- 1Program in Metabolism, Broad Institute of MIT and Harvard, Cambridge, MA, United States
- 2Center for Research on Genomics and Global Health, National Human Genome Research Institute, US National Institutes of Health, Bethesda, MD, United States
- 3Department of Computational Biology, Institut Pasteur, Université Paris Cité, Paris, France
- 4Human Genetics Center, Department of Epidemiology, Human Genetics, and Environmental Sciences, School of Public Health, The University of Texas Health Science Center at Houston, Houston, TX, United States
- 5Division of Preventive Medicine, Brigham and Women’s Hospital, Harvard Medical School, Boston, MA, United States
- 6Division of Statistical Genomics, Department of Genetics, Washington University School of Medicine, St. Louis, MO, United States
- 7Department of Epidemiology, Gillings School of Global Public Health, University of North Carolina at Chapel Hill, Chapel Hill, NC, United States
- 8Biostatistics, Department of Preventive Medicine, University of Southern California, Los Angeles, CA, United States
- 9Clinical and Translational Epidemiology Unit, Massachusetts General Hospital, Boston, MA, United States
- 10The Population Sciences Branch, National Heart, Lung, and Blood Institute, National Institutes of Health, Bethesda, MA, United States
- 11Division of Biostatistics, Washington University School of Medicine, St. Louis, MO, United States
- 12Program in Genetic Epidemiology and Statistical Genetics, Harvard T.H. Chan School of Public Health, Boston, MA, United States
- 13Department of Medicine and Harvard Medical School, Boston, MA, United States
Though both genetic and lifestyle factors are known to influence cardiometabolic outcomes, less attention has been given to whether lifestyle exposures can alter the association between a genetic variant and these outcomes. The Cohorts for Heart and Aging Research in Genomic Epidemiology (CHARGE) Consortium’s Gene-Lifestyle Interactions Working Group has recently published investigations of genome-wide gene-environment interactions in large multi-ancestry meta-analyses with a focus on cigarette smoking and alcohol consumption as lifestyle factors and blood pressure and serum lipids as outcomes. Further description of the biological mechanisms underlying these statistical interactions would represent a significant advance in our understanding of gene-environment interactions, yet accessing and harmonizing individual-level genetic and ‘omics data is challenging. Here, we demonstrate the coordinated use of summary-level data for gene-lifestyle interaction associations on up to 600,000 individuals, differential methylation data, and gene expression data for the characterization and prioritization of loci for future follow-up analyses. Using this approach, we identify 48 genes for which there are multiple sources of functional support for the identified gene-lifestyle interaction. We also identified five genes for which differential expression was observed by the same lifestyle factor for which a gene-lifestyle interaction was found. For instance, in gene-lifestyle interaction analysis, the T allele of rs6490056 (ALDH2) was associated with higher systolic blood pressure, and a larger effect was observed in smokers compared to non-smokers. In gene expression studies, this allele is associated with decreased expression of ALDH2, which is part of a major oxidative pathway. Other results show increased expression of ALDH2 among smokers. Oxidative stress is known to contribute to worsening blood pressure. Together these data support the hypothesis that rs6490056 reduces expression of ALDH2, which raises oxidative stress, leading to an increase in blood pressure, with a stronger effect among smokers, in whom the burden of oxidative stress is greater. Other genes for which the aggregation of data types suggest a potential mechanism include: GCNT4×current smoking (HDL), PTPRZ1×ever-smoking (HDL), SYN2×current smoking (pulse pressure), and TMEM116×ever-smoking (mean arterial pressure). This work demonstrates the utility of careful curation of summary-level data from a variety of sources to prioritize gene-lifestyle interaction loci for follow-up analyses.
1 Introduction
Lifestyle and environmental exposures have been shown to modify the associations of common genetic variants with traits linked to cardiometabolic disease (Grarup et al., 2008; Montasser et al., 2009; Higashibata et al., 2012; Manning et al., 2012; Sung et al., 2014). Recent large-scale studies have expanded the genetic architecture of interaction effects with genome-wide interaction analyses with tens of thousands of people from diverse ancestral backgrounds (Feitosa et al., 2018; Sung et al., 2018; Bentley et al., 2019; de Vries et al., 2019; Sung et al., 2019). These studies implicate genetic loci for which the genetic effect on a phenotype of interest is modified by either alcohol consumption or cigarette smoking. These lifestyle exposures could impact the gene regulatory mechanisms through which the trait-associated alleles influence gene expression. Statistical tests that evaluate these models would require human cohorts with exposure and outcome data, genetic data, and methylation, gene expression or other ‘omics data. The process of finding, obtaining, harmonizing, and analyzing individual-level data from human cohorts presents a challenge, as these data are ‘controlled-access’ and require numerous regulatory steps for the researcher, the researcher’s institution and the entity managing access to the data. On the other hand, it is now common to publish summary-level association statistics from human cohorts. Therefore, an analysis of both genetic and non-genetic data that relies on summary data alone is particularly valuable in this context.
To this end, we leveraged multiple summary-level association datasets, both genetic and epigenomic, to evaluate possible mechanisms of gene-environment interactions effects. We use data from the CHARGE Consortium’s Gene-Lifestyle Interactions Working Group (Rao et al., 2017), published in five recent papers (Feitosa et al., 2018; Sung et al., 2018; Bentley et al., 2019; de Vries et al., 2019; Sung et al., 2019) together with differential methylation and gene expression data (Lonsdale et al., 2013; Dekkers et al., 2016; Huan et al., 2016; Joehanes et al., 2016; Parker et al., 2017; Richard et al., 2017; Liu et al., 2018; Nikodemova et al., 2018). We demonstrate that by considering diverse epigenetic associations at the same genetic loci and linking multiple forms of evidence to a common gene, gene-environment interaction effects may be more fully characterized and prioritized for future follow-up analyses.
2 Methods
2.1 Gene-lifestyle interaction (GLI) summary statistics
Summary statistics from four genome-wide interaction studies performed within the Cohorts for Heart and Aging Research in Genomic Epidemiology (CHARGE) consortium were gathered, covering blood pressure and lipid trait measures and cigarette smoking and alcohol consumption environmental exposures (Feitosa et al., 2018; Sung et al., 2018; de Vries et al., 2019; Sung et al., 2019). Each of these projects used the following GLI model: Y = β0 + βG G + βCC + βL L + βGL G × L, where G represents the genetic variant, C the covariates (age, sex, and field center, where appropriate), and L the lifestyle exposure. The GLI models used additive allele effects and produced genome-wide joint tests of main effects and interaction effects. Sample sizes ranged from 175,000 to 602,000 individuals of multiple, self-identified ancestries (Supplemental Table S1).
We extracted individual variants with interaction p-value in any model less than 5 × 10−5 from each study for four subgroups, defined by the CHARGE Gene-Lifestyle Interaction Working Group: European ancestry (EA), African ancestry (AA), Hispanic ancestry (HA) and Asian Ancestry (ASA). Variants from trans-ancestry meta-analyses were also extracted for each project (Supplemental Table S1). Lipid trait measures included high-density lipoprotein cholesterol (HDL), low-density lipoprotein cholesterol (LDL), and triglycerides (TG). Four blood pressure traits were used: systolic blood pressure (SBP), diastolic BP (DBP), mean arterial pressure (MAP), and pulse pressure (PP). Environmental exposures were defined as: current drinking (yes/no), regular drinking (≥2 drinks per week/<2 drinks per week), drinking habits (≥8 glasses per week [heavy]/< 8 glasses per week [light]), current smoking (yes/no regular smoking in the past year), and ever smoked (yes/no 100 cigarettes smoked in lifetime).
Given the minor differences in filtering strategies for each of these projects, all meta-analysis results were re-processed using a common pipeline, as is described in more detail in (Laville et al., 2022). Briefly, SNPs were excluded for low MAF (<1%) or significant heterogeneity across included cohorts (p < 10–6). Meta-analyses were first conducted within ancestry groups, and these ancestry-specific results were meta-analyzed to produce the trans-ancestry meta-analysis results. All meta-analyses were implemented in METAL using the inverse variance scheme.
2.2 Epigenomic and transcriptomic data
Data describing the molecular signatures of the two environmental exposures were gathered from the literature, focusing on epigenomics and transcriptomics from population-based cohort studies (Table 1). For each of these sources, we used the statistical significance as defined within the publication or resource to determine which association results to use in our analysis. We used differential gene expression analysis (DExpr analysis) from studies of cigarette smoking (Huan et al., 2016; Parker et al., 2017; Nikodemova et al., 2018) and alcohol consumption (Liu et al., 2018). For genes, locus boundaries were defined by the start of the first exon and the end of the final exon, regardless of transcript, extended by 500 kb. Boundaries for DNA methylation (DNAm) sites were similarly defined by 500 kb on either side. Differential methylation analyses (DMe analysis) yield DNAm sites whose methylation is associated with a trait or exposure. These sites can be further associated with gene expression, defining expression quantitative trait methylation sites (eQTM). DNAm sites and eQTM were gathered from studies for lipids (Dekkers et al., 2016), blood pressure (Richard et al., 2017), cigarette smoking (Joehanes et al., 2016), and alcohol consumption (Liu et al., 2018), with some also providing mQTL analyses results (Richard et al., 2017; Liu et al., 2018). We also extracted significant expression quantitative trait loci (eQTL) from the Genetic Tissue-Expression (GTEx) project (Lonsdale et al., 2013) portal, version 8.
2.3 Locus prioritization based on accumulation of evidence
Our prioritization schema consisted of several steps (Figure 1). First, we identified overlapping loci from the GLI association studies with DMe, mQTL and DExpr analyses. Starting from the set of GLI variants from the association studies (p < 5 × 10−5), we found significantly associated DNAm sites within 500 kb of the variants for the trait or exposure of interest. We next identified those genes for which both 1) the GLI variant was a significant eQTL and 2) the DNAm was a significant eQTM (“prioritized loci”). Finally, we selected genes from these prioritized loci that additionally had significant DExpr effects for the exposure of interest in the GLI association (“further prioritized loci”).
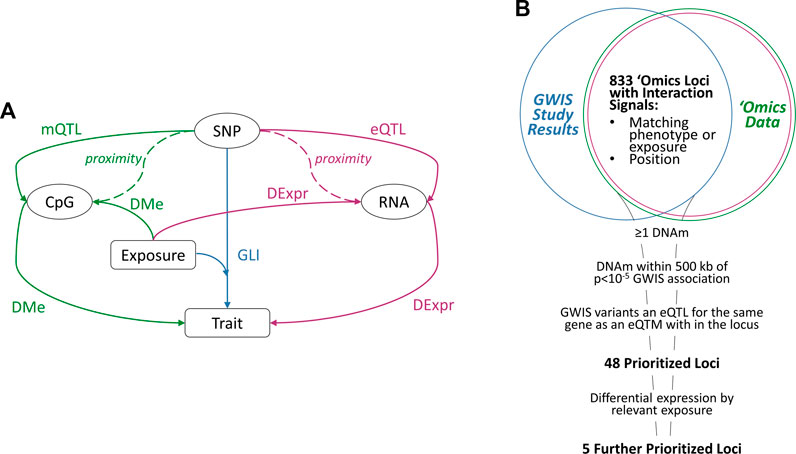
FIGURE 1. (A) Illustration of Possible Mechanisms Underlying Interaction Signals; rectangles represent study traits or measured lifestyle exposures; ovals represent three types of molecular risk factors: SNP genotype, RNA expression, and DNA methylation. Interactions are depicted by the “SNP-Exposure-Trait” path (GLI), with possible underlying molecular mechanisms shown along the sides for the transcriptomic (RNA expression) and epigenomic level (DNAm). The molecular effects are represented by solid arrows: expression QTL effect of a SNP allele on RNA expression levels (eQTL analysis) or methylation (mQTL analysis); differential methylation of a CpG site by trait or exposure (DMe analysis); and differential expression by trait or exposure (DExpr analysis). Dashed lines indicate physical proximity between elements. (B) Prioritization of GLI Loci; a panel of GLI loci from multiple genome-wide interaction studies (blue) were intersected with publicly-available epigenomic (green) and transcriptomic (purple) data for the relevant variants, lifestyle exposures, and traits. The resulting data were then prioritized using the listed criteria to yield 48 loci with multiple sources of molecular evidence underlying the interaction and five loci with differential expression by the exposure of interest.
3 Results
3.1 Overview
We obtained genetic summary statistics from genome-wide interaction analyses of cigarette smoking habits and alcohol consumption with lipid traits (HDL, LDL, and TG) and blood pressure traits (SBP, DBP, MAP, and PP) (Table 1; Supplemental Table S1). The generation (Feitosa et al., 2018; Sung et al., 2018; Bentley et al., 2019; de Vries et al., 2019; Sung et al., 2019) and harmonization (Laville et al., 2020; Laville et al., 2022) of these summary statistics has been previously described, resulting in 140 sets of loci in four race/ancestry groups and one trans-ancestry meta-analysis (Supplementary Figure S1).
To restrict our efforts to the variants most likely to have both genetic associations and molecular associations, we considered genetic variants with an interaction p-value less than an arbitrary cut-off of 5 × 10−5 in the following model: Y = β0 + βG G + βCC + βL L + βGL G × L, where G represents the genetic variant, C the covariates (age, sex, and field center, where appropriate), and L the lifestyle exposure. This filtering resulted in 897 variants (Figure 2). Of these variants, 682 were seen in smoking behavior interaction models, 511 were seen in models focusing on blood pressure traits, and 674 were observed in the analyses in the African ancestry subset (Figure 3).
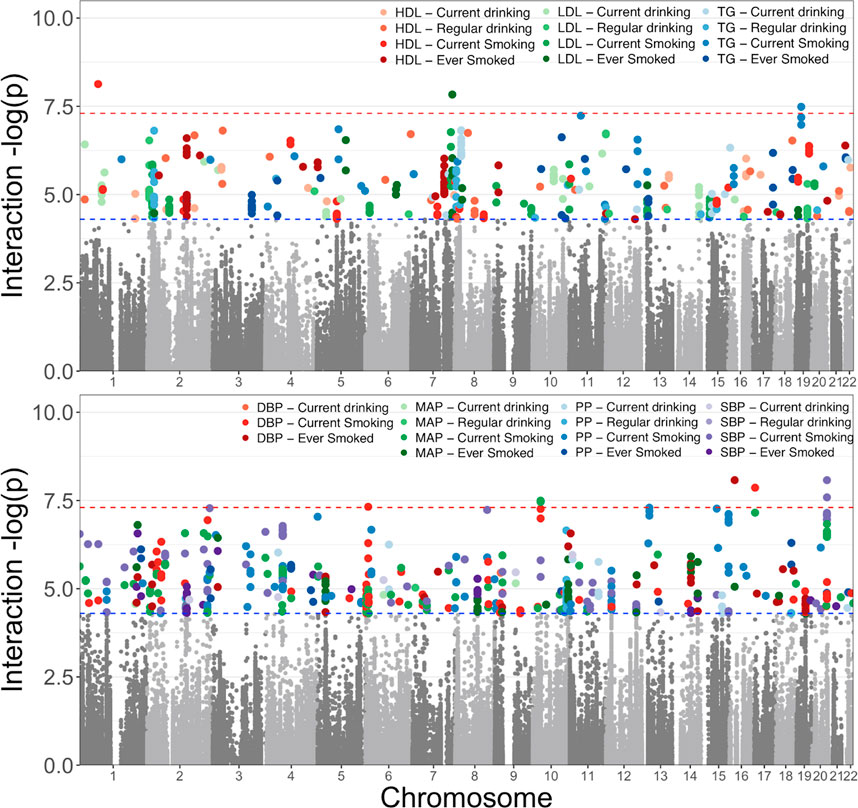
FIGURE 2. Selection of GLI Associations for Evaluation; a total of 897 variants were selected from meta-analyses of four ancestral groups and one trans-ancestry group of the variant interactions with two smoking and two alcohol exposure variables on three lipid measures (top figure) and four blood pressure traits (bottom figure). Here we show the most statistically significant associations across the five meta-analyses for each of the phenotype-exposure combinations. The results separated by ancestry group are available in Supplemental Figure 1.
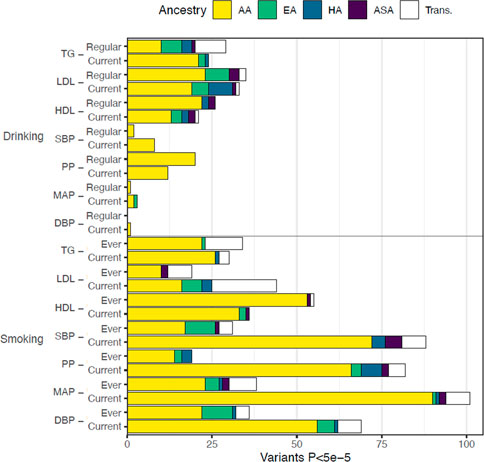
FIGURE 3. Distribution of GLI Associations across phenotypes and exposures. We display the total number of associated genetic variants discovered in each trait-exposure-ancestry group. The associations in models utilizing alcohol-related exposures are shown in the top figure and represent the minority of observed associations. The alcohol-related associates were dominated by analyses studying blood lipids. The associations related to cigarette smoking exposure are shown in the bottom figure with the majority of observations among analyses of blood pressure measures.
3.2 Harmonization of association and omics data
We determined which of these variants also demonstrated significant epigenomic (methylation and gene-expression) associations with clinical outcomes, smoking behavior and/or alcohol consumption habits (Figure 1A). We used a variety of epigenetic association data from cohort studies, including differential methylation analysis (DMe analysis; see Methods) with both clinical outcomes (lipid traits, blood pressure traits) and lifestyle outcomes (smoking behaviors and alcohol consumption habits), and differential gene expression analysis (DExpr analysis; see Methods) with smoking behaviors only, as no expression data by alcohol consumption data were found at the time of our literature search (Table 1). For the traits and exposures considered in the genetic association analysis, we compiled a list of loci with significantly differentiated genes or differentially methylated methylation sites (DNAm) for the trait or exposure, extending the borders of the gene (defined by exon boundaries) or site by 500 KB. This intersection of genetic, epigenetic, and transcriptomic data resulted in 833 unique genes (Figure 1B).
3.2.1 Prioritizing GLI associations
To further analyze these regions, we required loci to contain at least one DNAm site associated with expression of a gene (eQTM analysis; see Methods) and at least one of the GLI variants to be associated with the expression of the same gene in at least one tissue (eQTL analysis; see Methods). Using these criteria, we prioritized 48 loci. Of these, 37 loci were linked to smoking behavior and 28 loci were linked to lipid outcomes (Table 2). The majority of the prioritized loci were derived from analyses in individuals of African ancestry, a total of 30 loci, 26 of which were related to smoking behavior. These loci were evenly distributed between lipid and blood pressure traits. Our final prioritization step matched the lifestyle exposure from the GLI effect at the locus with significant genes from the DExpr analysis of the same exposure. At the 48 prioritized loci, five genes showed differential expression with a smoking exposure: GCNT4, PTPRZ1, SYN2, ALDH2, TMEM116. Each of these loci is discussed further below (Supplementary Figures S2–8) and details regarding the different types of evidence harmonized are available (Supplemental Tables S3–S9).
3.2.2 Further prioritized loci
At the GCNT4 locus, the common A allele at the rs3761743 variant was associated with decreased HDL levels among current smokers in an African-ancestry subset of cohorts, an effect that was attenuated among non-smokers (Interaction p = 1.5 × 10−5). GCNT4 is a glycosyltransferase expressed primarily in the thymus (Schwientek et al., 2000) but rs3761743 was associated with decreased expression of GCNT4 in the aorta (p = 3.5 × 10−6) and increased expression in testis (p = 6.7 × 10−14) (Supplemental Table S3, Supplementary Figure S2). Notably, GCNT4 is upregulated among smokers (Parker et al., 2017) (p = 9.8 × 10−5) while methylation of the DNAm site cg21158503 was decreased with smoking exposure (Joehanes et al., 2016) (p = 6.6 × 10−6).
In the analysis of lipids and the ‘ever-smoking’ exposure in the African ancestry subset of cohorts, the association between the rs77810251 variant at the PTPRZ1 locus and HDL levels was found to differ between exposure strata (Interaction p = 9.5 × 10−7), with a positive association of the minor A allele in the ‘never-smoking’ exposure group and no association among the ‘ever-smoking’ exposure group (Bentley et al., 2019) (Figure 4A, Supplemental Table S4, Supplementary Figure S3). rs77810251 is an eQTL for PTPRZ1 in aorta tissue, with the A allele associated with decreased gene expression. A DNAm site within the locus, cg00826384, shows increased methylation among smokers (Joehanes et al., 2016). PTPRZ1 was shown to be downregulated in nicotine-treated cells (Wang et al., 2011). These generate a potential hypothesis of the A allele of rs77810251 decreasing expression of PTPRZ1, which then causes an increase in HDL levels through unknown mechanisms. Smoking, which is associated with increased methylation of PTPRZ1, may perturb the PTPRZ1-HDL pathway, and abolished the association between rs77810251 and HDL. PTPRZ1 is a protein tyrosine phosphatase receptor, which is constitutively active and is inactivated through binding with heparin-binding growth factors pleiotrophin and midkine. Its inactivation leads to increased tyrosine phosphorylation of target genes. This gene has a broad spectrum of substrates that may mediate multiple pathways (Shi et al., 2017). Of interest, both PTPRZ1 and LRP6, a member of the well-established lipids signaling family of LDL-receptor related proteins, are regulated through binding with midkine (Sakaguchi et al., 2003), suggesting a potential connection of this locus with a lipids pathway.
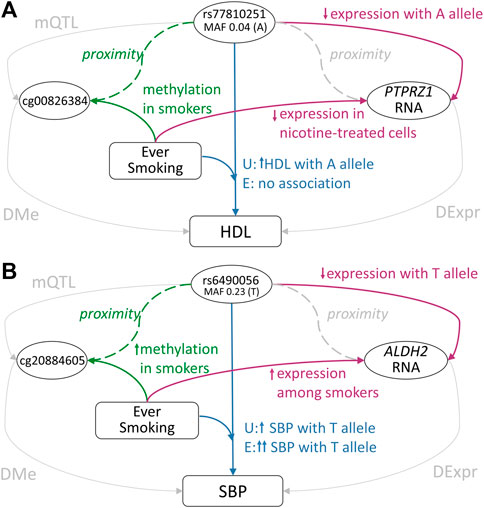
FIGURE 4. Summary of evidence for two GLI loci (see text for further details). Pathways without evidence for the association have been grayed out (A) PTPRZ1 GLI Locus: the GLI variant rs77810251 in PTPRZ1 is also an eQTL with downregulated expression in aortic tissue, and was in close proximity to the DNAm site cg00826384, which shows elevated methylation among smokers (B) ALDH2 GLI Locus: the GLI variant rs6490056 in ALDH2 is also an eQTL with downregulated expression in multiple tissues and was in close proximity to the DNAm site cg20884605, which shows elevated methylation among smokers. U: unexposed individuals, E: exposed individuals.
SYN2 was prioritized from the PP and ‘current smoking’ GLI analysis among the African ancestry subset of cohorts. A rare T allele at a single variant in the locus, rs4135300, was associated with PP (p = 8.72 × 10−7) with a positive effect in the ‘non-current smoking’ exposure group and a negative effect in the ‘current smoking’ exposure group (Interaction P = 3 × 10−7; Supplemental Table S5). rs4135300 is an eQTL for SYN2 in aorta tissue, with the T allele associated with decreased expression levels (Supplementary Figure S4). The DNAm site identified within the locus, cg10245988, has increased methylation on average among smokers (Joehanes et al., 2016). Expression of SYN2 is increased in human neuroblastoma cells treated with nicotine. SYN2 has previously been associated with schizophrenia (Mirnics et al., 2000).
The gene ALDH2 was prioritized based on multiple analyses of smoking and blood pressure among the Asian ancestry subset of cohorts, specifically, the SBP with ‘current smoking’ (Figure 4B; Supplemental Table S7; Supplementary Figure S6), SBP with ‘ever smoking’ (Supplemental Table S8; Supplementary Figure S7), and MAP with ‘ever smoking’ Supplemental Table S6; Supplementary Figure S5) GLI analyses. As a representative example, the common T allele of rs6490056 was associated with increased SBP levels (p = 1.9 × 10−10) with a larger effect in the ‘current smoking’ exposure strata (Interaction p = 2.6 × 10−5). This variant and other associated variants in this locus are eQTLs for ALDH2 in multiple tissues, including in the lungs and esophagus. ALDH2 is a part of the major oxidative pathway for alcohol metabolism, and an Asian ancestry-specific variant (rs671) in this gene is well known for causing acetaldehyde accumulation with alcohol intake, leading to unpleasant side effects (Chen et al., 2014). Acetaldehyde and other toxic aldehydes are also components of tobacco smoke (Hoffman and Evans, 2013), and decreased ALDH2 activity leads to increased reactive aldehyde species and oxidative stress (Yasue et al., 2019). In individuals deficient in ALDH2 activity, smoking amplifies risk of oxidative stress-related conditions (Yasue et al., 2019). Importantly, oxidative stress is a known contributor to worsening blood pressure (Ahmad et al., 2017; Guzik and Touyz, 2017). Based on these data, it appears possible that rs6490056 reduces expression of ALDH2, raising oxidative stress, and causing a concomitant increase in blood pressure. Under the additional burden of oxidative stress introduced by smoking, an even stronger effect on blood pressure traits may be observed through this locus.
TMEM116 is a transmembrane protein, expressed in nearly all measured tissues (Uhlén et al., 2015). Two variants at this locus (rs6490056, rs10849962) were associated in MAP with ‘ever smoking’ GLI analyses among the Asian ancestry subset of cohorts (Supplemental Table S9, Supplementary Figure S8). The common T allele of rs6490056 was associated with increased MAP measures (p = 5.7 × 10−11) with a larger effect in ‘ever smoking’ exposure group (Interaction p = 3.3 × 10−5). These variants were significant eQTLs with the T allele of rs6490056 associated with lower TMEM116 expression levels in atrial and adipose tissues and the A allele of rs10849962 associated with higher TMEM116 expression levels in a variety of tissues, including esophageal tissues, heart tissue, adipose, and whole blood. TMEM116 is upregulated among smokers with corresponding demethylation of a nearby DNAm site, cg08528204 (Huan et al., 2016).
4 Discussion
Although gene × environment interactions are often cited as a potential source for “missing heritability” (Simon et al., 2016; Mayhew and Meyre, 2017), molecular evidence of these interactions remains limited. The establishment of CHARGE’s Gene Lifestyle Interactions Working Group resulted in a number of large-scale, trans-ancestry evaluations of gene × environment interaction effects (Feitosa et al., 2018; Sung et al., 2018; Bentley et al., 2019; de Vries et al., 2019; Sung et al., 2019). In this analysis, we leveraged publicly available epigenomic and transcriptomic summary association data to identify a subset of interactions from these analyses among which multiple forms of evidence point toward potential mechanistic explanations. We identified 833 genes for which evidence of a statistical interaction can be supported with functional data from at least one source, with 48 of these having multiple sources of functional support. We also observed an overrepresentation of prioritized interaction loci drawn from meta-analyses of African-ancestry populations. An overrepresentation of findings with the smoking vs. alcohol exposure may be a result of the greater amount of available omics data for smoking (Table 1).
A striking finding from this work is the large proportion of interactions that are observed among African ancestry meta-analyses compared to other ancestries or to Trans-ancestry analyses. This phenomenon was observed among both the full number of interactions identified (79.7%) as well as the prioritized loci (62.5%), and three of the five further prioritized loci were from African ancestry meta-analyses. This phenomenon reflects the underlying GLI association results from which our study draws. Notably, despite the shared methodology, this predominance of findings from African ancestry meta-analyses was evenly distributed among traits considered but was not evenly distributed among exposures, observed in studies of smoking with blood pressure and lipids, but not with alcohol exposure on either trait. Similarly, in these analyses, 26 of 30 of the prioritized loci based on meta-analyses of those of African ancestry were studies of the smoking exposure. A more complete discussion of these GLI association results are available in the primary publications for these projects. Briefly, some of the associations are for variants that are in higher frequency or present only among African ancestry populations, they are generally of low frequency (MAF 0.01–0.05) with high imputation scores, and with consistent associations across contributing African ancestry cohorts (Sung et al., 2018; Bentley et al., 2019; Sung et al., 2019; Laville et al., 2020). Of the 30 loci prioritized in this study based on African ancestry meta-analyses, the lead associated variant was African ancestry-specific (only present in 1 KG AFR populations) for only 2, while for most the lead variants were available in all ancestries, but not associated in the meta-analyses of other ancestries. These results suggest that the source of this observation relates to a smoking exposure-related difference by ancestry.
Although we did not have the data for a detailed evaluation of smoking patterns by ancestry in our studies, there are pronounced differences in smoking patterns across ancestry groups in the US (U.S. Department of Health and Human Services, 1998). Notably, there is a marked difference in the type of preferred cigarette, as shown in data from the National Survey on Drug Use and Health, which is designed to be representative of the US population: 88% of African Americans smokers vs. 26% of non-Hispanic white smokers used menthol cigarettes (Giovino et al., 2015). Menthol cigarettes are marketed more aggressively to African Americans (Lee et al., 2015; Mendez and Le, 2021). Additionally, some differences in preference may stem from variations in bitter taste perception by ancestry, with menthol cigarettes more palatable to those with stronger bitter taste perception as the menthol flavor additive masks the bitterness of nicotine. An African ancestry-specific genetic locus, MRGPRX4, associated with a five- to 8-fold increased odds of menthol cigarette smoking was recently identified (Kozlitina et al., 2019), although only a small minority of African Americans carry this variant.
Menthol cigarettes have long been targeted by the public health community based on evidence that they facilitate deeper smoke inhalation by decreasing nicotine-induced irritation (Ton et al., 2015; Mayhew and Meyre, 2017). This deeper inhalation may lead to a subsequent higher absorption of the myriad harmful components within cigarette smoke (U.S. Department of Health and Human Services, 1998; Ross et al., 2016). Consistent with this observation, ancestry differences in smoking-related metabolites and carcinogens have been reported (Pérez-Stable et al., 1998; Benowitz et al., 2011; Khariwala et al., 2014; Jain, 2015), and different levels of these compounds may underlie the observed differences by ancestry in genetic interactions upon smoking exposure. Additionally, there is some evidence for greater systemic oxidative stress (Il’yasova et al., 2012; Morris et al., 2012; Annor et al., 2017; Kim et al., 2020) and inflammation (Albert et al., 2004; Khera et al., 2005; Akinyemiju et al., 2019; Zahodne et al., 2019) among Americans of African vs. European ancestry. Exposure to cigarette smoke, a rich source of oxidants, on a background of elevated oxidative stress and inflammation may provoke a greater response among these individuals, manifesting as an interaction with smoking that differs by ancestry.
One key motivation for conducting analyses of gene × lifestyle interactions is the relative ease of practical translation, as results suggest a modifiable risk, i.e. individuals with a certain genotype might reduce exposures associated with exacerbated risk. In our efforts to map existing functional information to loci of interest, we identified several areas where improvements in available data might facilitate stronger inferences. For instance, there are limited data to evaluate differential gene expression by alcohol exposure, making it difficult to further investigate the loci identified in gene-alcohol interaction analyses. Additionally, more tissue-specific data would be useful. Specific tissue types are of greater interest for each phenotype (e.g. liver for lipids) and for each exposure (e.g. lung for smoking). Further, the reliance on whole blood, which is the most available in cohort studies, will limit our understanding of the underlying biology. Data linking methylation to gene expression is also limited. Although it was beyond the study design of this project, it would be useful to collect individual-level data to better elucidate these loci. Importantly, while some of the patterns observed in our data fit with expectations (e.g. the direction of RNA expression and DNA methylation for GCNT4, PTPRZ1, TMEM116), some did not, and individual-level data is needed to correctly model these associations.
A strength of these analyses is the use of the expansive genome-wide interaction results from large epidemiological meta-analyses. These data are drawn from discovery data on up to 133,805 individuals, important given the statistical power needed to detect gene-environment interaction effects. Additionally, the CHARGE Gene × Lifestyle Interactions Working Group went to great effort to include studies of diverse ancestries, such that relatively large proportions of historically underrepresented ancestry groups, such as African ancestry, were achieved. Given the preponderance of African ancestry-identified associations among our results, this inclusion was of key significance. This work could have been improved with omics data derived from individuals of diverse ancestries to explore associations that differed by ancestry. The exposure data considered in these analyses was represented using binary variables in order to maximize sample sizes for detection of interactions, although the true effects of exposures are certain to be more complex, with variations with timing and dose of exposure. Similarly, the exposures and phenotypes we selected for these analyses were relatively straightforward to measure, however, a wide range of exposures may be involved in gene-lifestyle interactions on a wide range of phenotypes, and it is unknown whether these findings are representative of gene-lifestyle interactions in general. Also, additional experimental data will be necessary to confirm biological pathways suggested by these findings in order to advance the evidence from this work towards clinical translation.
In summary, this work provides preliminary evidence from publicly available transcriptomics and epigenomics data to support the biological mechanisms underlying statistical gene × lifestyle interactions. These data suggest compelling evidence for how gene × lifestyle interactions may occur, motivating future studies that include individual-level epigenomic and transcriptomic data, other environmental exposures and outcomes, and more complex characterization of exposure.
Data availability statement
All of the data used in this work are publicly available. Both the original GWAS summary results and the re-processed statistics generated as part of this study are available via dbGaP (accession number phs000930).
Author contributions
TM, AB, CG, AM wrote the manuscript and performed the analysis. VL, PV, KS, provided summary statistics for analysis. And everyone else reviewed and revised the manuscript.
Funding
This work was partly supported by grants R01 HL118305 and R01 HL156991 from the National Heart, Lung, and Blood Institute (NHLBI) of the National Institutes of Health (NIH). This work was also supported in part by the Intramural Research Program of the National Human Genome Research Institute of the National Institutes of Health through the Center for Research on Genomics and Global Health (CRGGH). The CRGGH is supported by the National Human Genome Research Institute, the National Institute of Diabetes and Digestive and Kidney Diseases, the Center for Information Technology, and the Office of the Director at the National Institutes of Health (Z01HG200362).
Acknowledgments
The authors would like to acknowledge the contributions of L. Adrienne Cupples to the Framingham Heart Study and the development of the TOPMed ecosystem.
Conflict of interest
The authors declare that the research was conducted in the absence of any commercial or financial relationships that could be construed as a potential conflict of interest.
Publisher’s note
All claims expressed in this article are solely those of the authors and do not necessarily represent those of their affiliated organizations, or those of the publisher, the editors and the reviewers. Any product that may be evaluated in this article, or claim that may be made by its manufacturer, is not guaranteed or endorsed by the publisher.
Supplementary material
The Supplementary Material for this article can be found online at: https://www.frontiersin.org/articles/10.3389/fgene.2022.954713/full#supplementary-material
Abbreviations
GLI, Gene-lifestyle interaction; LDL, low density lipoprotein; HDL, high density lipoprotein; TG, triglycerides; DBP, diastolic blood pressure; SBP, systolic blood pressure; MAP, mean arterial pressure; PP, pulse pressure; eQTL, expression quantitative trait loci; eQTM, expression quantitative trait methylation; mQTL, methylation quantitative trait loci (SNPs whose genotype correlates with DNA methylation); DNAm, DNA methylation; DMe, differential methylation; DExpr, differential gene expression.
References
Ahmad, K. A., Yuan Yuan, D., Nawaz, W., Ze, H., Zhuo, C. X., Talal, B., et al. (2017). Antioxidant therapy for management of oxidative stress induced hypertension. Free Radic. Res. 51 (4), 428–438. doi:10.1080/10715762.2017.1322205
Akinyemiju, T., Moore, J. X., Pisu, M., Goodman, M., Howard, V. J., Safford, M., et al. (2019). Association of baseline inflammatory biomarkers with cancer mortality in the REGARDS cohort. Oncotarget 10 (47), 4857–4867. doi:10.18632/oncotarget.27108
Albert, M. A., Glynn, R. J., Buring, J., and Ridker, P. M. (2004). C-reactive protein levels among women of various ethnic groups living in the United States (from the Women's Health Study). Am. J. Cardiol. 93 (10), 1238–1242. doi:10.1016/j.amjcard.2004.01.067
Annor, F., Goodman, M., Thyagarajan, B., Okosun, I., Doumatey, A., Gower, B. A., et al. (2017). African ancestry gradient is associated with lower systemic F2-isoprostane levels. Oxid. Med. Cell. Longev. 2017, 8319176. doi:10.1155/2017/8319176
Benowitz, N. L., Dains, K. M., Dempsey, D., Wilson, M., and Jacob, P. (2011). Racial differences in the relationship between number of cigarettes smoked and nicotine and carcinogen exposure. Nicotine Tob. Res. 13 (9), 772–783. doi:10.1093/ntr/ntr072
Bentley, A. R., Sung, Y. J., Brown, M. R., Winkler, T. W., Kraja, A. T., Ntalla, I., et al. (2019). Multi-ancestry genome-wide gene-smoking interaction study of 387, 272 individuals identifies new loci associated with serum lipids. Nat. Genet. 51 (4), 636–648. doi:10.1038/s41588-019-0378-y
Chen, C. H., Ferreira, J. C., Gross, E. R., and Mochly-Rosen, D. (2014). Targeting aldehyde dehydrogenase 2: New therapeutic opportunities. Physiol. Rev. 94 (1), 1–34. doi:10.1152/physrev.00017.2013
de Vries, P. S., Brown, M. R., Bentley, A. R., Sung, Y. J., Winkler, T. W., Ntalla, I., et al. (2019). Multiancestry genome-wide association study of lipid levels incorporating gene-alcohol interactions. Am. J. Epidemiol. 188 (6), 1033–1054. doi:10.1093/aje/kwz005
Dekkers, K. F., van Iterson, M., Slieker, R. C., Moed, M. H., Bonder, M. J., van Galen, M., et al. (2016). Blood lipids influence DNA methylation in circulating cells. Genome Biol. 17 (1), 138. doi:10.1186/s13059-016-1000-6
Feitosa, M. F., Kraja, A. T., Chasman, D. I., Sung, Y. J., Winkler, T. W., Ntalla, I., et al. (2018). Novel genetic associations for blood pressure identified via gene-alcohol interaction in up to 570K individuals across multiple ancestries. PLoS One 13 (6), e0198166. doi:10.1371/journal.pone.0198166
Giovino, G. A., Villanti, A. C., Mowery, P. D., Sevilimedu, V., Niaura, R. S., Vallone, D. M., et al. (2015). Differential trends in cigarette smoking in the USA: Is menthol slowing progress? Tob. Control 24 (1), 28–37. doi:10.1136/tobaccocontrol-2013-051159
Grarup, N., Andreasen, C. H., Andersen, M. K., Albrechtsen, A., Sandbaek, A., Lauritzen, T., et al. (2008). The -250G>A promoter variant in hepatic lipase associates with elevated fasting serum high-density lipoprotein cholesterol modulated by interaction with physical activity in a study of 16, 156 Danish subjects. J. Clin. Endocrinol. Metab. 93 (6), 2294–2299. doi:10.1210/jc.2007-2815
Guzik, T. J., and Touyz, R. M. (2017). Oxidative stress, inflammation, and vascular aging in hypertension. Hypertension 70 (4), 660–667. doi:10.1161/hypertensionaha.117.07802
Higashibata, T., Hamajima, N., Naito, M., Kawai, S., Yin, G., Suzuki, S., et al. (2012). eNOS genotype modifies the effect of leisure-time physical activity on serum triglyceride levels in a Japanese population. Lipids Health Dis. 11, 150. doi:10.1186/1476-511X-11-150
Hoffman, A. C., and Evans, S. E. (2013). Abuse potential of non-nicotine tobacco smoke components: Acetaldehyde, nornicotine, cotinine, and anabasine. Nicotine Tob. Res. 15 (3), 622–632. doi:10.1093/ntr/nts192
Huan, T., Joehanes, R., Schurmann, C., Schramm, K., Pilling, L. C., Peters, M. J., et al. (2016). A whole-blood transcriptome meta-analysis identifies gene expression signatures of cigarette smoking. Hum. Mol. Genet. 25 (21), 4611–4623. doi:10.1093/hmg/ddw288
Il'yasova, D., Wang, F., Spasojevic, I., Base, K., D'Agostino, R. B., and Wagenknecht, L. E. (2012). Racial differences in urinary F2-isoprostane levels and the cross-sectional association with BMI. Obes. (Silver Spring) 20 (10), 2147–2150. doi:10.1038/oby.2012.170
Jain, R. B. (2015). Distributions of selected urinary metabolites of volatile organic compounds by age, gender, race/ethnicity, and smoking status in a representative sample of U.S. adults. Environ. Toxicol. Pharmacol. 40 (2), 471–479. doi:10.1016/j.etap.2015.07.018
Joehanes, R., Just, A. C., Marioni, R. E., Pilling, L. C., Reynolds, L. M., Mandaviya, P. R., et al. (2016). Epigenetic signatures of cigarette smoking. Circ. Cardiovasc. Genet. 9 (5), 436–447. doi:10.1161/circgenetics.116.001506
Khariwala, S. S., Scheuermann, T. S., Berg, C. J., Hayes, R. B., Nollen, N. L., Thomas, J. L., et al. (2014). Cotinine and tobacco-specific carcinogen exposure among nondaily smokers in a multiethnic sample. Nicotine Tob. Res. 16 (5), 600–605. doi:10.1093/ntr/ntt194
Khera, A., McGuire, D. K., Murphy, S. A., Stanek, H. G., Das, S. R., Vongpatanasin, W., et al. (2005). Race and gender differences in C-reactive protein levels. J. Am. Coll. Cardiol. 46 (3), 464–469. doi:10.1016/j.jacc.2005.04.051
Kim, C., Slaughter, J. C., Terry, J. G., Jacobs, D. R., Parikh, N., Appiah, D., et al. (2020). Antimüllerian hormone and F2-isoprostanes in the coronary artery risk development in young adults (CARDIA) study. Fertil. Steril. 114 (3), 646–652. doi:10.1016/j.fertnstert.2020.04.028
Kozlitina, J., Risso, D., Lansu, K., Olsen, R. H. J., Sainz, E., Luiselli, D., et al. (2019). An African-specific haplotype in MRGPRX4 is associated with menthol cigarette smoking. PLoS Genet. 15 (2), e1007916. doi:10.1371/journal.pgen.1007916
Laville, V., Majarian, T., Sung, Y. J., Schwander, K., Feitosa, M. F., Chasman, D., et al. (2020). Large-scale multivariate multi-ancestry Interaction analyses point towards different genetic mechanisms by population and exposure. 562157. doi:10.1101/562157%JbioRxiv
Laville, V., Majarian, T., Sung, Y. J., Schwander, K., Feitosa, M. F., Chasman, D. I., et al. (2022). Gene-lifestyle interactions in the genomics of human complex traits. Eur. J. Hum. Genet. 30, 730–739. doi:10.1038/s41431-022-01045-6
Lee, J. G. L., Henriksen, L., Rose, S. W., Moreland-Russell, S., and Ribisl, K. M. (2015). A systematic review of neighborhood disparities in point-of-sale tobacco marketing. Am. J. Public Health 105 (9), e8–e18. doi:10.2105/ajph.2015.302777
Liu, C., Marioni, R. E., Hedman, Å. K., Pfeiffer, L., Tsai, P. C., Reynolds, L. M., et al. (2018). A DNA methylation biomarker of alcohol consumption. Mol. Psychiatry 23 (2), 422–433. doi:10.1038/mp.2016.192
Lonsdale, J., Thomas, J., Salvatore, M., Phillips, R., Lo, E., Shad, S., et al. (2013). The genotype-tissue expression (GTEx) project. Nat. Genet. 45 (6), 580–585. doi:10.1038/ng.2653
Manning, A. K., Hivert, M. F., Scott, R. A., Grimsby, J. L., Bouatia-Naji, N., Chen, H., et al. (2012). A genome-wide approach accounting for body mass index identifies genetic variants influencing fasting glycemic traits and insulin resistance. Nat. Genet. 44 (6), 659–669. doi:10.1038/ng.2274
Mayhew, A. J., and Meyre, D. (2017). Assessing the heritability of complex traits in humans: Methodological challenges and opportunities. Curr. Genomics 18 (4), 332–340. doi:10.2174/1389202918666170307161450
Mendez, D., and Le, T. T. T. (2021). Consequences of a match made in hell: The harm caused by menthol smoking to the african American population over 1980–2018. tobaccocontrol-2021-056748. doi:10.1136/tobaccocontrol-2021-056748%JTobaccoControl
Mirnics, K., Middleton, F. A., Marquez, A., Lewis, D. A., and Levitt, P. (2000). Molecular characterization of schizophrenia viewed by microarray analysis of gene expression in prefrontal cortex. Neuron 28 (1), 53–67. doi:10.1016/s0896-6273(00)00085-4
Montasser, M. E., Shimmin, L. C., Hanis, C. L., Boerwinkle, E., and Hixson, J. E. (2009). Gene by smoking interaction in hypertension: Identification of a major quantitative trait locus on chromosome 15q for systolic blood pressure in Mexican-Americans. J. Hypertens. 27 (3), 491–501. doi:10.1097/hjh.0b013e32831ef54f
Morris, A. A., Zhao, L., Patel, R. S., Jones, D. P., Ahmed, Y., Stoyanova, N., et al. (2012). Differences in systemic oxidative stress based on race and the metabolic syndrome: The morehouse and emory team up to eliminate health disparities (META-Health) study. Metab. Syndr. Relat. Disord. 10 (4), 252–259. doi:10.1089/met.2011.0117
Nikodemova, M., Yee, J., Carney, P. R., Bradfield, C. A., and Malecki, K. M. (2018). Transcriptional differences between smokers and non-smokers and variance by obesity as a risk factor for human sensitivity to environmental exposures. Environ. Int. 113, 249–258. doi:10.1016/j.envint.2018.02.016
Parker, M. M., Chase, R. P., Lamb, A., Reyes, A., Saferali, A., Yun, J. H., et al. (2017). RNA sequencing identifies novel non-coding RNA and exon-specific effects associated with cigarette smoking. BMC Med. Genomics 10 (1), 58. doi:10.1186/s12920-017-0295-9
Pérez-Stable, E. J., Herrera, B., Jacob, P., and Benowitz, N. L. (1998). Nicotine metabolism and intake in black and white smokers. Jama 280 (2), 152–156. doi:10.1001/jama.280.2.152
Rao, D. C., Sung, Y. J., Winkler, T. W., Schwander, K., Borecki, I., Cupples, L. A., et al. (2017). Multiancestry study of gene-lifestyle interactions for cardiovascular traits in 610 475 individuals from 124 cohorts: Design and rationale. Circ. Cardiovasc. Genet. 10 (3), e001649. doi:10.1161/CIRCGENETICS.116.001649
Richard, M. A., Huan, T., Ligthart, S., Gondalia, R., Jhun, M. A., Brody, J. A., et al. (2017). DNA methylation analysis identifies loci for blood pressure regulation. Am. J. Hum. Genet. 101 (6), 888–902. doi:10.1016/j.ajhg.2017.09.028
Ross, K. C., Dempsey, D. A., St Helen, G., Delucchi, K., and Benowitz, N. L. (2016). The influence of puff characteristics, nicotine dependence, and rate of nicotine metabolism on daily nicotine exposure in african American smokers. Cancer Epidemiol. Biomarkers Prev. 25 (6), 936–943. doi:10.1158/1055-9965.Epi-15-1034
Sakaguchi, N., Muramatsu, H., Ichihara-Tanaka, K., Maeda, N., Noda, M., Yamamoto, T., et al. (2003). Receptor-type protein tyrosine phosphatase zeta as a component of the signaling receptor complex for midkine-dependent survival of embryonic neurons. Neurosci. Res. 45 (2), 219–224. doi:10.1016/s0168-0102(02)00226-2
Schwientek, T., Yeh, J. C., Levery, S. B., Keck, B., Merkx, G., van Kessel, A. G., et al. (2000). Control of O-glycan branch formation. Molecular cloning and characterization of a novel thymus-associated core 2 beta1, 6-n-acetylglucosaminyltransferase. J. Biol. Chem. 275 (15), 11106–11113. doi:10.1074/jbc.275.15.11106
Shi, Y., Ping, Y. F., Zhou, W., He, Z. C., Chen, C., Bian, B. S., et al. (2017). Tumour-associated macrophages secrete pleiotrophin to promote PTPRZ1 signalling in glioblastoma stem cells for tumour growth. Nat. Commun. 8, 15080. doi:10.1038/ncomms15080
Simon, P. H., Sylvestre, M. P., Tremblay, J., and Hamet, P. (2016). Key considerations and methods in the study of gene-environment interactions. Am. J. Hypertens. 29 (8), 891–899. doi:10.1093/ajh/hpw021
Sung, Y. J., de las Fuentes, L., Schwander, K. L., Simino, J., and Rao, D. C. (2014). Gene–smoking interactions identify several novel blood pressure loci in the Framingham heart study. Am. J. Hypertens. 28 (3), 343–354. doi:10.1093/ajh/hpu149
Sung, Y. J., Winkler, T. W., de Las Fuentes, L., Bentley, A. R., Brown, M. R., Kraja, A. T., et al. (2018). A large-scale multi-ancestry genome-wide study accounting for smoking behavior identifies multiple significant loci for blood pressure. Am. J. Hum. Genet. 102 (3), 375–400. doi:10.1016/j.ajhg.2018.01.015
Sung, Y. J., de las Fuentes, L., Winkler, T. W., Chasman, D. I., Bentley, A. R., Kraja, A. T., et al. (2019). A multi-ancestry genome-wide study incorporating gene–smoking interactions identifies multiple new loci for pulse pressure and mean arterial pressure. Hum. Mol. Genet. 28 (15), 2615–2633. doi:10.1093/hmg/ddz070
Ton, H. T., Smart, A. E., Aguilar, B. L., Olson, T. T., Kellar, K. J., and Ahern, G. P. (2015). Menthol enhances the desensitization of human α3β4 nicotinic acetylcholine receptors. Mol. Pharmacol. 88 (2), 256–264. doi:10.1124/mol.115.098285
Uhlén, M., Fagerberg, L., Hallström, B. M., Lindskog, C., Oksvold, P., Mardinoglu, A., et al. (2015). Proteomics. Tissue-based map of the human proteome. Science 347 (6220), 1260419. doi:10.1126/science.1260419
U.S. Department of Health and Human Services (1998). Tobacco use among U.S. Racial/ethnic minority groups—african Americans, American Indians and Alaska natives, asian Americans and pacific islanders, and hispanics: A report of the surgeon general. Atlanta, GA: U.S. Department of Health and Human Services, Centers for Disease Control and Prevention, National Center for Chronic Disease Prevention and Health.
Wang, J., Cui, W., Wei, J., Sun, D., Gutala, R., Gu, J., et al. (2011). Genome-wide expression analysis reveals diverse effects of acute nicotine exposure on neuronal function-related genes and pathways. Front. Psychiatry 2, 5. doi:10.3389/fpsyt.2011.00005
Yasue, H., Mizuno, Y., and Harada, E. (2019). Coronary artery spasm - clinical features, pathogenesis and treatment. Proc. Jpn. Acad. Ser. B Phys. Biol. Sci. 95 (2), 53–66. doi:10.2183/pjab.95.005
Keywords: multi-omics, gene-lifestyle interactions, smoking, alcohol, serum lipids, blood pressure, summary data
Citation: Majarian TD, Bentley AR, Laville V, Brown MR, Chasman DI, de Vries PS, Feitosa MF, Franceschini N, Gauderman WJ, Marchek C, Levy D, Morrison AC, Province M, Rao DC, Schwander K, Sung YJ, Rotimi CN, Aschard H, Gu CC and Manning AK (2022) Multi-omics insights into the biological mechanisms underlying statistical gene-by-lifestyle interactions with smoking and alcohol consumption. Front. Genet. 13:954713. doi: 10.3389/fgene.2022.954713
Received: 27 May 2022; Accepted: 18 November 2022;
Published: 05 December 2022.
Edited by:
Hui-Qi Qu, Children’s Hospital of Philadelphia, United StatesReviewed by:
Liliana Ciobanu, University of Adelaide, AustraliaEvan Yi-Wen Yu, Southeast University, China
Copyright © 2022 Majarian, Bentley, Laville, Brown, Chasman, de Vries, Feitosa, Franceschini, Gauderman, Marchek, Levy, Morrison, Province, Rao, Schwander, Sung, Rotimi, Aschard, Gu and Manning. This is an open-access article distributed under the terms of the Creative Commons Attribution License (CC BY). The use, distribution or reproduction in other forums is permitted, provided the original author(s) and the copyright owner(s) are credited and that the original publication in this journal is cited, in accordance with accepted academic practice. No use, distribution or reproduction is permitted which does not comply with these terms.
*Correspondence: Alisa K. Manning, amanning@broadinstitute.org
†These authors have contributed equally to this work and share first authorship