- 1Virology Unit and Bioinformatics Centre, Institute of Microbial Technology, Council of Scientific and Industrial Research (CSIR), Sector 39-A, Chandigarh, India
- 2Academy of Scientific and Innovative Research (AcSIR), Ghaziabad, India
miRNAs play an essential role in promoting viral infections as well as modulating the antiviral defense. Several miRNA repositories have been developed for different species, e.g., human, mouse, and plant. However, ‘VIRmiRNA’ is the only existing resource for experimentally validated viral miRNAs and their targets. We have developed a ‘AntiVIRmiR’ resource encompassing data on host/virus miRNA expression during viral infection. This resource with 22,741 entries is divided into four sub-databases viz., ‘DEmiRVIR’, ‘AntiVmiR’, ‘VIRmiRNA2’ and ‘VIRmiRTar2’. ‘DEmiRVIR’ has 10,033 differentially expressed host-viral miRNAs for 21 viruses. ‘AntiVmiR’ incorporates 1,642 entries for host miRNAs showing antiviral activity for 34 viruses. Additionally, ‘VIRmiRNA2’ includes 3,340 entries for experimentally validated viral miRNAs from 50 viruses along with 650 viral isomeric sequences for 14 viruses. Further, ‘VIRmiRTar2’ has 7,726 experimentally validated targets for viral miRNAs against 21 viruses. Furthermore, we have also performed network analysis for three sub-databases. Interactions between up/down-regulated human miRNAs and viruses are displayed for ‘AntiVmiR’ as well as ‘DEmiRVIR’. Moreover, ‘VIRmiRTar2’ interactions are shown among different viruses, miRNAs, and their targets. We have provided browse, search, external hyperlinks, data statistics, and useful analysis tools. The database available at https://bioinfo.imtech.res.in/manojk/antivirmir would be beneficial for understanding the host-virus interactions as well as viral pathogenesis.
Introduction
MiRNAs are short non-coding endogenous RNA (ncRNA) molecules with 18–23 nucleotides (nt) in length. They help in the post-transcriptional regulation of gene expression (Treiber et al., 2019). The miRNA biogenesis involves two types of processing pathways, viz. Canonical and non-canonical pathways (O’Brien et al., 2018). The canonical miRNA biogenesis includes four main processing steps. Firstly, it begins with the transcription of primary miRNA (pri-miRNA) from the genomic DNA (intronic, intergenic, or polycistronic loci) by RNA polymerase II in the nucleus. Secondly, the pri-miRNA consists of a hairpin structure that is further cleaved into ∼70 nt precursor miRNA (pre-miRNA) using the RNase III enzyme Drosha (Ha and Kim, 2014). This drosha enzyme is found in a multiprotein complex known as microprocessor that also include ds-RNA binding protein, DGCR8 (DiGeorge syndrome chromosomal region 8), also known as Pasha in Caenorhabditis elegans and Drosophila (Denli et al., 2004; Yeom et al., 2006). DGCR8 helps in the binding of dsRNA substrate by recognizing the “UGU” motif and helps drosha in the cleavage of pri-miRNA by binding to the basal “UG” motif (Han et al., 2004; Auyeung et al., 2013). Thirdly, this pre-miRNA is then exported to cytoplasm using Exportin-5 (XPO5)/RanGTP complex, where the hairpin loop is cleaved by the Dicer-transactivating response RNA-binding protein (TRBP) complex enzyme and results in miRNA duplex. Finally, the miRNA duplex is loaded onto the Argonaute protein (AGO)-containing RNA-induced silencing complex (RISC) complex to form a mature miRNA strand and its complementary passenger strand. The mature miRNA binds to the complementary strand of target mRNA and results in translational repression or mRNA degradation (Rupaimoole and Slack, 2017). However, in most cases, the passenger strand is degraded in the cytoplasm. For example, endonuclease C3PO degraded the passenger strand in C. elegans (Ye et al., 2011). But in some cases, the passenger strand plays a pivotal role in the cells where the mature strand works synergistically with the passenger strand (Shan et al., 2013; Yang et al., 2013).
However, non-canonical pathways are drosha/DGCR8 or dicer-independent pathways e.g., mirtrons produced from the pre-miRNA/introns during splicing (Ruby et al., 2007); 7-methylguanosine (m7G)-capped pre-miRNA exported to cytoplasm via PHAX-exportin one pathway (Xie M. et al., 2013). The first miRNA, lin-4 was discovered in 1993 by the Ambros and Ruvkun groups in Caenorhabditis elegans (Lee et al., 1993). Then, in 2000, a second miRNA, i.e. let-7 was also discovered in Caenorhabditis elegans (Reinhart et al., 2000). Since then, miRNAs have been discovered for organisms like human, worms, flies, mouse, rats, etc. (Lee and Ambros, 2001; Takada et al., 2006).
MiRNAs play an essential role in biological processes like cell division, stress conditions, cancer, proliferation, apoptotic cell death, viral infection, tumorigenesis, etc. (Huang et al., 2011). Host miRNA also help in replicating and propagating viruses by generating pri-miRNAs using RNA polymerase II (Grundhoff and Sullivan, 2011; Zhuo et al., 2013). miRNAs are also encoded from the viral genome and may assist in causing viral infection (Skalsky and Cullen, 2010; Bruscella et al., 2017). Host miRNAs can also target viral mRNAs that result in inhibiting the viral life cycle. For example, hsa-miR-296–5p targets IFN beta (Scagnolari et al., 2010) and VP1 and VP3 (Zheng et al., 2013), during Hepatitis C virus (HCV) and Enterovirus 71 (EV71) infections respectively. Contrary, a few host miRNAs e.g. hsa-miR-122, hsa-miR-151–5p, and hsa-miR-17–5p also result in promoting HCV infection (Li et al., 2017).
The host miRNAs play an essential role in the viral life cycle. Likewise, many viruses have their own miRNAs. The first viral miRNA was identified for Epstein-Barr virus (EBV) in 2004 (Pfeffer et al., 2004). Since then, miRNAs were identified in different viruses like Kaposi’s sarcoma-associated herpesvirus (KSHV) (Lin et al., 2012), Human cytomegalovirus (HCMV) (Pfeffer et al., 2005), Human immunodeficiency virus type 1 (HIV-1) (Su et al., 2018) etc. There are 1,308 viral miRNAs for 44 viruses reported in the ‘VIRmiRNA’ database (Qureshi et al., 2014). Since then, numerous miRNAs and their isomiRs have been discovered for different species (Lagos-Quintana et al., 2002; Glazov et al., 2010). IsomiRs are miRNA sequences with variations in length at the 5’ or 3’ termini that result in nucleotide addition or deletion (Glogovitis et al., 2020).
Various computational resources have been developed that collect data for experimentally validated miRNA. The latest release of miRBase22 database has 38,589 miRNAs for 271 organisms. Likewise, there are many other databases for miRNA collecting data for different organisms. For example, miRBase (Kozomara et al., 2019), miRviewer (Kiezun et al., 2012), miRNEST (Szcześniak et al., 2012), PMRD (Zhang et al., 2010); mirPub (Vergoulis et al., 2015), HMDD (Li et al., 2014; Huang et al., 2019), EpimiRBase (Mooney et al., 2016), HumiR (Solomon et al., 2020), miR2Disease (Jiang et al., 2009), miRCancer (Xie B. et al., 2013) etc. Also, repositories have been developed for experimentally validated or predicted miRNA targets. For example, targetHub (Manyam et al., 2013), miRTarBase (Hsu et al., 2011; Chou et al., 2018; Huang et al., 2020), dbMTS (Li et al., 2020), maTE (Yousef et al., 2019), miRPathDB (Backes et al., 2017; Kehl et al., 2020) etc. Similarly, there are web servers for differentially expressed miRNA developed using different miRNA profiling methods, namely, miRExpress (Wang et al., 2009), miRGator (Nam et al., 2008; Cho et al., 2013), miRmine (Panwar et al., 2017) etc.
Likewise, few repositories have been developed for viruses, viz., ViTa (Hsu et al., 2007) predicts targets for host miRNA on viruses; Vir-Mir db (Li et al., 2008) predicts viral miRNA hairpin; vHoT (Kim et al., 2012) database shows interactions between viral microRNA and host genomes. Also, our lab has developed a dedicated virus-encoded resource that has information for experimental viral miRNA and their targets, i.e. ‘VIRmiRNA’ (Qureshi et al., 2014). Since, there are several studies for host miRNAs that are involved in viral infection. But still, a dedicated web resource for host miRNAs involved in viral diseases is lacking. Therefore, we have developed the ‘AntiVIRmiR’ database that encompasses information on host encoded antiviral miRNA with their expression and experimentally validated viral miRNA along with their targets. It includes four sub-databases, viz. DEmiRVIR, AntiVmiR, VIRmiRNA2 and VIRmiRTar2. DEmiRVIR sub-database incorporates differentially expressed host and viral miRNA data, AntiVmiR sub-database has host encoded antiviral and proviral miRNA data, VIRmiRNA2 sub-database provides experimentally validated viral miRNA data and VIRmiRTar2 sub-database includes experimentally validated viral miRNA target information. This resource would be helpful for the researchers that are focusing on host miRNA-virus interaction.
Materials and methods
Data collection
The literature search was carried out using the advanced search option of PubMed using the following query:
Using this search, ∼2,300 articles were obtained as of March 2021. We focused majorly on screening miRNAs pertaining to important human viruses. After screening, ∼1,200 articles were retrieved to obtain the relevant information. Besides these, we also removed those articles that provide information about the predicted miRNA and some other research aspects of miRNAs. Finally, 620 articles were used to extract viral and antiviral miRNA information (Supplementary Figure S1). Further, we will update the data yearly or whenever sufficient data is available.
Database organization
‘AntiVIRmiR’ resource is divided into four sub-databases, namely, ‘DEmiRVIR’, ‘AntiVmiR’ ‘VIRmiRNA2’, and ‘VIRmiRTar2’.
‘DEmiRVIR’' comprises data for differentially expressed miRNA found in the viral infection. It includes the following data: 1) DEmiRVIR ID, 2) virus, 3) taxonomy, 4) nomenclature, 5) miRNA name, 6) miRNA sequence, 7) miRNA expression (up/down), 8) organism, 9) cell line, 10) experimental method, 11) score, and (xiii) references.
‘AntiVmiR’ module incorporates information regarding the host miRNA that acts mainly as an antiviral or sometimes as a proviral in different disease conditions. Following fields are incorporated in the sub-database, viz. 1) AntiVmiR ID, 2) virus, 3) taxonomy, 4) nomenclature, 5) miRNA name, 6) target gene, 7) uniprot id, 8) target organism, 9) target process, 10) cell line, 11) experimental method, 12) target region and 13) references.
‘VIRmiRNA2’ sub-database provides data for experimentally validated viral miRNAs that are reported in literature. It incorporates information about the following fields: 1) VIRmiRNA2 ID, 2) virus, 3) taxonomy, 4) nomenclature, 5) viral miRNA name 6) viral miRNA sequence, 7) length, 8) GC content, 9) pre-miRNA, 10) arm, 11) cell line, 12) experimental method and 13) references.
‘VIRmiRTar2’ sub-database has information about experimentally validated miRNA targets. It includes the following fields: 1) VIRmiRTar2 ID, 2) virus, 3) taxonomy, 4) nomenclature, 5) viral miRNA name, 6) target gene, 7) uniprot id, 8) target organism, 9) cell line, 10) experimental method, 11) target region, 12) target reference and 13) references.
Network-based analysis
We have constructed the networks for three sub-databases, viz. ‘DEmiRVIR’, ‘AntiVmiR’, and ‘VIRmiRTar2’. Interactions are shown among viruses, miRNAs, and targets as input in the cytoscape software (Shannon et al., 2003). Different shapes and colors were used to represent the virus, miRNA and targets in different sub-databases. Using ‘DEmiRVIR’ data, networks developed between human miRNA and virus. miRNA name is given in ellipse shape (pink color), upregulated human miRNA is denoted in diamond shape (red color); downregulated human miRNA in hexagon shape (purple color). While the human miRNAs found upregulated or downregulated in different studies are assigned octagon shape (green color). In ‘AntiVmiR’, networks developed for human miRNA with different viruses where virus name is displayed in ellipse shape (red color) and human miRNA in octagon shape (pink color). While in ‘VIRmiRTar2’, networks developed between virus, viral miRNA and their targets. The virus name is provided in ellipse shape (red color), viral miRNA in octagon shape (pink color) and targets in triangular shape (green color).
Implementation
‘AntiVIRmiR’ resource is developed user-friendly to access the data using different web pages like search, browse, analysis tools and network analysis. Further, a user manual is also provided on the help page to assist in data exploration. The database is designed using LAMP software that uses linux as an operating system and apache as a web server. The front-end of the web interface is developed using scripting languages viz., Javascript, HTML, CSS and PHP. While back-end of the interface is implemented using MySQL database. The detailed architecture of ‘AntiVIRmiR’ is shown in Figure 1.
Results
‘AntiVIRmiR’ resource has been subdivided into four databases, viz. ‘DEmiRVIR’, ‘AntiVmiR’, ‘VIRmiRNA2’, and ‘VIRmiRTar2’.
Database statistics
We have provided exhaustive database statistics for each sub-category as depicted in Figure 2.
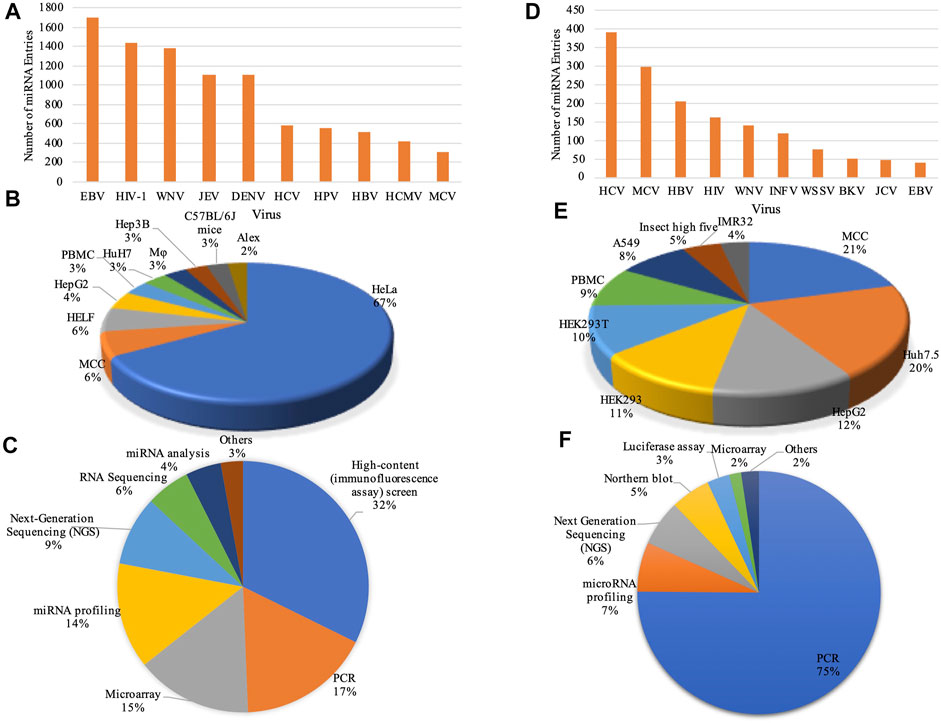
FIGURE 2. Data statistics: Bar graphs represent the number of entries in top 10 viruses for (A) ‘DEmiRVIR’ and (D) ‘AntiVmiR’. Pie charts representing statistical distribution of cell lines in (B) ‘DEmiRVIR’ and (E) ‘AntiVmiR’ and experimental methods in (C) ‘DEmiRVIR’ and (F) ‘AntiVmiR’ (HCV, Hepatitis C virus; MCV, Merkel cell polyomavirus; HBV, Hepatitis B virus; HIV, Human immunodeficiency virus; WNV, West Nile Virus; INFV, Influenza A virus; WSSV, White spot syndrome virus; BKV, BK polyomavirus; JCV, JC polyomavirus; EBV, Epstein Barr virus; JEV, Japanese encephalitis virus; DENV, Dengue virus; HPV, Human papillomavirus and HCMV, Human cytomegalovirus).
1. DEmiRVIR: ‘DEmiRVIR’ resource deals with the differentially expressed miRNA (DEmiRs) of hosts (primarily human) found against viruses. Currently, our database incorporates 10,033 miRNAs that are differentially expressed against 21 viruses. The maximum number of entries has been reported for EBV having 1705 entries followed by HIV-1 with 1,435 entries, West Nile Virus (WNV) with 1,386 entries, Japanese encephalitis virus (JEV) with 1,108 entries, Dengue virus (DENV) having 1,107 entries and so on (Figure 2A). The majority of these DEmiRs have been detected in cell lines like HeLa, MCC, HELF, HepG2, PBMC etc., as shown in Figure 2B. While, these DEmiRs are obtained by performing different experiments. The most commonly used experiments are High-content (immunofluorescence assay) screen, microarray, PCR, miRNA profiling, next-generation sequencing (NGS) etc. (Figure 2C).
2. AntiVmiR: This sub-database encompasses 1,642 entries reported for 34 viruses. The HCV has a maximum of 394 entries reported from 81 human miRNAs in different studies, followed by Merkel cell polyomavirus (MCV) with 297 entries encountered from 190 human miRNAs; Hepatitis B virus (HBV) having 204 entries from 42 human miRNAs and so on (Figure 2D). Different cell lines viz. MCC, Huh7.5, HepG2, HEK293 etc. Were used to test these miRNAs. Further, these human miRNAs were validated using experimental techniques like PCR, miRNA profiling, NGS, northern blot etc. (Figures 2E,F).
3. VIRmiRNA2: ‘VIRmiRNA2’ sub-database encompasses 3,340 viral miRNA entries from 50 viruses infecting different organisms. The majority of entries belongs to EBV with 604 viral miRNAs followed by 350 entries from Pseudorabies virus (PRV), 348 viral miRNAs from KSHV and other important viruses like HCMV, Rhesus lymphocryptovirus (RLCV), Herpes simplex virus 1 (HSV1) etc. As shown in Supplementary Figure S2A. These viral miRNA entries have been tested on different cell lines, like PK15, MDBK, IB-RS-2, HEK293T, MSB1, and Vero (Supplementary Figure S2B). Further, we found that experimental methods like PCR, deep sequencing, northern blot, next-generation sequencing (NGS) are the most commonly used methods for miRNA experiments, as depicted in Supplementary Figure S2C.
Isomirs: From 3,340 entries for the ‘VIRmiRNA2’ sub-database, we have found 650 unique viral miRNA isomeric sequences for 14 viruses. We have displayed a single viral miRNA entry with their isomeric sequence for individual viruses in Table 1. The maximum number of viral isomeric sequences have been reported for PRV with 276 isomirs for 26 viral miRNAs, followed by Rhesus rhadinovirus (RRV) and RLCV having 66 and 46 isomirs for 25 viral miRNAs respectively. Whereas, Herpes B virus has 11 viral miRNAs that include 57 isomirs. A complete list of viral miRNA isomeric sequences is given in Supplementary Table S1. In the case of individual viral miRNAs, the top six miRNAs belong to PRV, where prv-miR-11–1 has a maximum number of 40 entries, followed by prv-miR-9-5p, prv-miR-8-5p, prv-miR-5-5p, prv-miR-4-5p and prv-miR-7-5p with 32, 28, 25, 22 and 21 entries, respectively. Followed by Herpes B virus, hbv-miR-b8-5p and hbv-miR-b7-5p with 18 and 10 isomirs, respectively. Whereas, top hits for other viruses have less than 10 isomirs. For example, rlcv-miR-rl1-35–3p has seven isomirs; bfv-miR-bf2-3p, ebv-mir-bart16–3p and rrv-miR-rr1-7-5p having five isomirs; kshv-miR-K12-11–3p and rcmv-miR-r1-1 having four isomirs. Similarly, many miRNAs contain only single isomirs e.g., hcmv-miR-UL112–3p, hsv1-miR-H5-3p, mdv1-m11–5p, mdv2-m14–5p etc. These isomeric sequences are appended by # symbol after the miRNA sequence in the database.
4. VIRmiRTar2: ‘VIRmiRTar2’ section has 7,726 entries for experimentally validated miRNA targets obtained from 21 different viruses. The maximum number of targets have been reported for EBV, KSHV, Marek’s disease virus type 2 (MDV2), Marek’s disease virus type 1 (MDV1), HCMV, Bovine Leukemia Virus (BLV), Mouse gammaherpesvirus 68 (MGHV) with 3,846, 2,257, 859, 561, 107, 29, 25 entries respectively (Supplementary Figure S2D). While the remaining 42 entries have been reported for viruses like Herpes simplex virus (HSV), HIV-1, Simian virus 40 (SV40), JC polyomavirus (JCV) etc. In the database, ebv-miR-BART19–3p has a maximum of 372 entries, followed by ebv-miR-BART2-5p with 254 entries. In KSHV, kshv-miR-K12-11 has a maximum of 236 entries; kshv-miR-K12-4-3p with 223 entries; kshv-miR-K12-1 with 218 entries. In MDV, mdv2-miR-m16–5p and mdv1-miR-m6-5p have a maximum of 73 and 71 entries for respectively. Likewise, different cell lines have been used to identify miRNA targets e.g., BCBL-1, BC-1, BC-3, MSB1 and others (Supplementary Figure S2E), which includes cell lines like C666–1, B95-8, BJAB, Raji etc. Various experimental methods have been used for miRNA target identification. The most commonly used methods are Photoactivatable-Ribonucleoside-Enhanced Crosslinking and Immunoprecipitation (PAR-CLIP), Gene Set Enrichment Analysis (GSEA), PCR, luciferase assay, High Throughput Sequencing- Crosslinking and Immunoprecipitation (HITS-CLIP) (Supplementary Figure S2F). Whereas less commonly used methods for target identification are sequencing, RISC-Immunoprecipitation, microarray, ELISA etc.
Network analysis
We have performed network analysis for three sub-databases to show the interactions among virus, miRNAs, and targets.
1. DEmiRVIR network analysis: In ‘DEmiRVIR’, interaction analysis was performed for the human miRNA reported upon various viral infections. Human miRNAs express in host system either in human or cell line upon natural or artificial infection. In the database, different types of human miRNA belong to the same human miRNA family, e.g., let-7 (let-7a, let-7b, let-7c etc.), mir-130a, mir-18a, mir-146b etc. Similarly, different entries for 5p and 3p miRNA are shown, viz. mir-142–3p, mir-142–5p, mir-181a-2-3p etc. Therefore, we have used the original name of human miRNA by excluding its type, arm, e.g., let-7a and let-7b will be denoted as let-7, mir-142–3p will be used as mir-142. After this nomenclature, redundant entries were excluded from the analysis. Finally, 1,021 entries were left for upregulated miRNAs (507 unique) and 996 for downregulated miRNAs (426 unique). We have analyzed only those human miRNAs whose expression change during infection of five or more viruses in ‘DEmiRVIR’ sub-database. We found that 52 upregulated and 59 downregulated human miRNAs e.g., hsa-miR-146 was upregulated during 10 viral infections, followed by hsa-let-7, hsa-miR-18, hsa-miR-182 in eight viral infections and many more.
From the 52 upregulated and 59 downregulated human miRNAs during viral infections (of five or more viruses), we found 30 human miRNAs were both upregulated and downregulated in different studies. While the remaining 22 upregulated human miRNAs were also found downregulated in less than five viral infections. Whereas, from the remaining 29 downregulated human miRNAs, namely, hsa-miR-128, hsa-miR-138 and hsa-miR-654 were not found in upregulated human miRNA data. In Supplementary Figure S3, the bar graph depicts the number of human miRNAs that are upregulated, downregulated or both in five or more viral infections. The maximum 50 differentially expressed human miRNAs have been reported for viral infections of EBV and HIV-1, followed by HBV (47), HCV (45), HCMV 32) etc. While, human miRNAs that were both upregulated and downregulated in different studies were found to have a maximum 21 in case of HCV followed by EBV (18), HBV 16) etc. Further, there is no human miRNA found that is both upregulated and downregulated during HCMV infection. Whereas, there are only two upregulated human miRNAs found during Varicella-zoster virus (VZV) infection. Using this data, we have developed a heatmap as depicted in Figure 3. Heatmap represents the human miRNA expression as upregulated, downregulated or both during different viral infections as reported in the literature. We found hsa-miR-25 and hsa-miR-210 human miRNAs were upregulated in maximum seven viral infections followed by hsa-miR-96 and hsa-miR-532 during six viral infections. Contrary, hsa-miR-145 human miRNA was downregulated in eight viral infections followed by hsa-miR-23 in 7, hsa-miR-654, hsa-miR-100 and hsa-miR-139 in six viral infections respectively. There are many human miRNAs e.g., let-7, hsa-miR-30, hsa-miR-29, hsa-miR-125 etc. That were both upregulated and downregulated for the same viral infection in different studies.
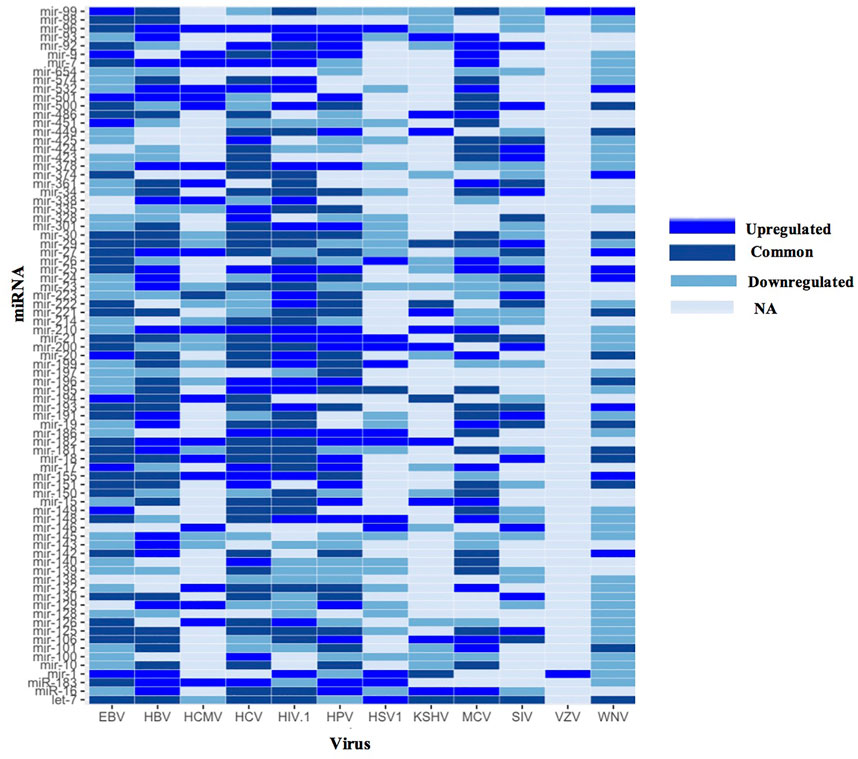
FIGURE 3. Heatmap showing human miRNA expression reported in different viruses (EBV, Epstein Barr virus; HBV, Hepatitis B virus; HCMV, Human cytomegalovirus; HIV, Human immunodeficiency virus; HPV, Human Papillomavirus; HSV1, Herpes simplex virus one; KSHV, Kaposi sarcoma-associated herpesvirus; MCV, Merkel cell polyomavirus; SIV, Simian immunodeficiency virus; VZV, Varicella-zoster virus and WNV, West Nile Virus).
Additionally, we have also developed networks using cytoscape software. In the network analysis, miRNA name is displayed in pink color (ellipse shape), whereas viral infections that help in the upregulation of human miRNA are denoted in red color (diamond shape) and downregulation in purple color (hexagon shape). Moreover, viral infections are assigned green color (octagon shape) if human miRNA expression was both upregulated and downregulated in different studies. For example, hsa-miR-9 human miRNA upregulated in five viral infections i.e., Human papillomavirus (HPV), MCV, HIV-1, HCMV, EBV and downregulated in WNV. Further, hsa-miR-9 human miRNA was both upregulated and downregulated in HCV in different studies (Figure 4A). Likewise, hsa-miR-129 human miRNA was found upregulated in three viral infections i.e., HBV, HCMV, HPV and downregulated in five viral infections i.e., WNV, HCV, Simian immunodeficiency virus (SIV), HSV1 and HIV-1. But in none of the viral infections, hsa-miR-129 human miRNA was found both upregulated and downregulated, as shown in Figure 4B. Further, hsa-let-7 human miRNA was upregulated in HSV1, downregulated in HPV and HCMV. Whereas it was upregulated or downregulated in eight viral infections viz., MCV, HBV, WNV, EBV, KSHV, SIV, HIV-1 and HCV (Supplementary Figure S4A). Moreover, hsa-miR-128 human miRNA was downregulated in five viral infections viz., EBV, HSV1, HBV, WNV and HIV-1 as displayed in Supplementary Figure S4B.
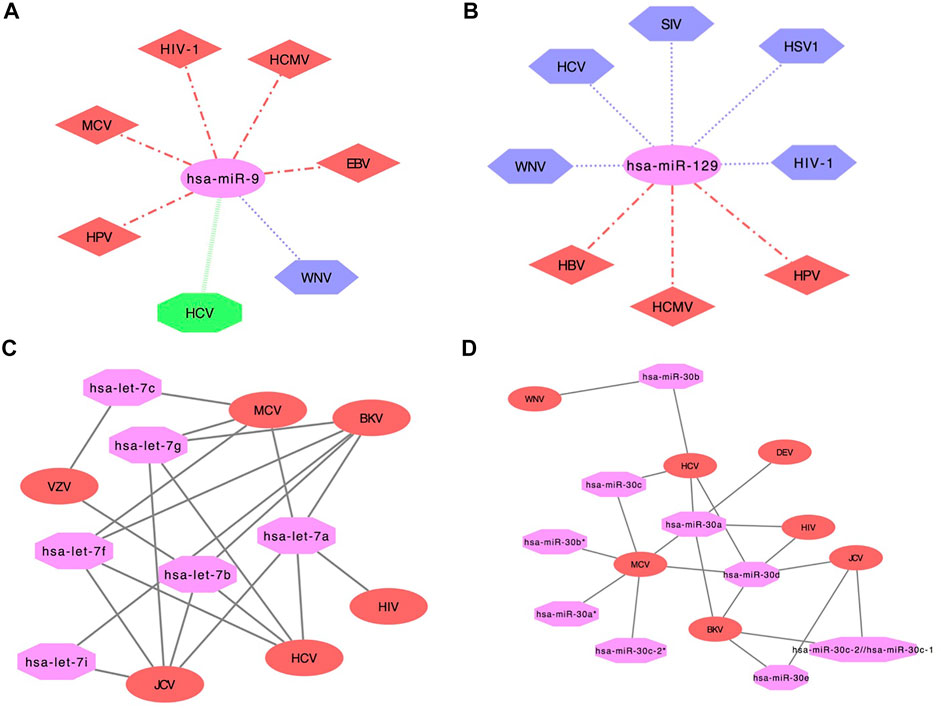
FIGURE 4. Network showing human miRNA expression reported in different viruses in ‘DEmiRVIR’ (A) hsa-miR-9 and (B) hsa-miR-129 (Viruses with hexagon shape (purple color) represent downregulation, diamond shape (red color) represents upregulation, octagon shape (green color) represents viruses found common in both upregulation as well as downregulation in different studies and miRNA is represented in ellipse shape (pink color)). Network interaction analysis for ‘AntiVmiR’ (C) let-7 and (D) mir-30. Virus name is written in ellipse shape (red color) and miRNA name in octagon shape (pink color).
2. AntiVmiR Network analysis: ‘AntiVmiR’ interaction has been performed for human miRNAs that were found common in different viruses. We have developed 82 interaction networks for antiviral human miRNA for 28 viruses using the cytoscape software available on the web server. In the network analysis, the human miRNA name is written in octagon shape having magenta color. While the virus name is displayed in an ellipse shape having red color. The maximum number of viruses has been reported for hsa-miR-21 and hsa-miR-181 that show its antiviral effect against 9 viruses. hsa-miR-125 and hsa-miR-155 were reported against eight viruses, followed by hsa-miR-30 and hsa-miR-221 with seven viruses and so on. While building the network, we have shown common viruses for different variants of miRNA in one class. e.g., miRNA hsa-let-7a, 7b, 7c, 7f, 7g, 7i belongs to the let-7 family (Figure 4C). The let-7 family has been found in six viruses, where let-7a was found in BK polyomavirus (BKV), MCV, JCV, HCV and HIV; followed by let-7b (BKV, VZV, JCV and HCV), let-7f (MCV, BKV, HCV and JCV) and let-7g (JCV, HCV, BKV and MCV) in four viruses, let-7c (VZV and MCV) and let-7i (JCV and BKV) in two viruses. Likewise, the hsa-miR-30 family has variants like 30a, 30b, 30c*. hsa-miR-30a (HCV, MCV, Duck enteritis virus (DEV), BKV and HIV) and hsa-miR-30 days (BKV, HCV, MCV, HIV and JCV) were found in five viruses; hsa-miR-30b (WNV and HCV), hsa-miR-30c (MCV and HCV) and hsa-miR-30e (BKV and JCV) were found in two viruses. Whereas, hsa-miRmir-30a*, hsa-miR-30b* and hsa-miR-30c-2* were found in MCV (Figure 4D).
3. VIRmiRTar2 Network analysis: We have also performed network-based analysis on viral miRNA target data for 20 viruses using Cytoscape software. We have used viruses, viral miRNAs and their target as input in the software for building a network. Different shapes and colors were used to represent the virus (ellipse shape having red color), miRNA (octagon shape with pink color), and target (triangle shape in green color). The network analysis for HIV and BLV has been shown in Supplementary Figure S5. The viral miRNA for HIV further interacts with their respective target (Supplementary Figure S5A). There are three viral miRNAs for BLV that have some common targets like TLR9, C4A, COLEC12 in blv-miR-B1-5p and blv-miR-B4-5p (Supplementary Figure S5A). Therefore, users can view viral miRNA target interaction analysis for different viruses on the web server.
Web server interface
We have provided a search and browse-by virus option to retrieve the data from each of the four different sub-databases.
1. Database browsing: Users can browse each sub-database of ‘AntiVIRmiR’ by selecting any virus from the given list. The virus name is written with the number of miRNAs for that virus, e.g., EBV has 1705 entries in the ‘DEmiRVIR’ sub-database. Users can click on the virus name, and a list of all the miRNAs from that virus will be displayed. By clicking on miRNA ID, the user can view more details. Further, we have also provided an external link for NCBI Taxonomy browser, Uniprot, miRBase and PubMed (Figure 5).
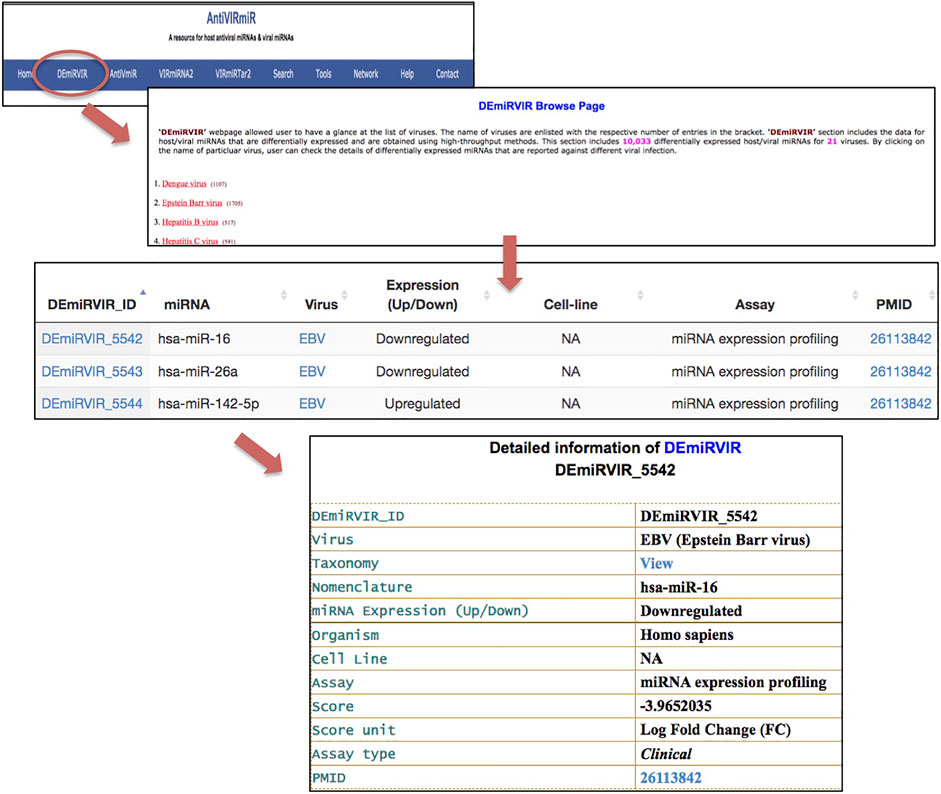
FIGURE 5. ‘AntiVIRmiR’ resource overview that includes a browse page, individual search output and detailed information of a miRNA.
2. Database search: Users can select any of the four sub-databases to search against the given keyword in the search option. The search output provides information about different aspects, i.e., miRNA, virus, miRNA expression, nomenclature, sequence, length, GC content, cell line, assay and pubmed. We have also provided sorting and filtering functionality in the search output. By clicking on the miRNA ID, users can view the complete details of that entry.
3. Analysis Tools: We also have integrated tools in the database like BLAST, Map and TarFind for miRNA sequence analysis. AntiVIRmiR-BLAST tool matches the user-provided miRNA sequence against the viral miRNA sequences (‘VIRmiRNA2’) that are already reported in the database. The output shows both tabular and text alignment of the matching miRNA sequences along with their alignment score. Map tool displays the list of perfectly matching miRNAs available in the database against the given input sequence. Map tool will help the user find how many miRNAs are available in the database against the user-provided sequence. TarFind tool identifies the target genes against the user-provided miRNA or seed sequence in the human reference gene sequence.
Discussion
Viral miRNA and host miRNA play an essential role in viral pathogenesis during infection. It helps in promoting viral replication by cell survival, proliferation, or modulating the immune response (Skalsky and Cullen, 2010). Viral miRNAs have been discovered as post-transcriptional gene regulators for the host and viral genes. They can bind to single or multiple targets, also known as multiplicity, as reported in different studies (John et al., 2004; Qureshi et al., 2014). For example, ebv-miR-BART19–3p targets RBM47 and UHRF1BP1L (Gottwein et al., 2011); kshv-miR-K12-4-3p targets ZFYVE20, MACROD2, CASP3, MBNL3 and C9orf41 etc. (Gottwein et al., 2011). Simultaneously, there were reports of cooperativity in which multiple viral miRNAs target a gene (John et al., 2004). Like TPM1 (Tumor suppressor Protein tropomyosin 1) has been targeted by multiple KSHV viral miRNAs like kshv-miR-K12-1, kshv-miR-K12-2, kshv-miR-K12-3, kshv-miR-K12-4 (Kieffer-Kwon et al., 2015). In another example, THBS1 (Thrombospondin 1) is also targeted by multiple KSHV viral miRNAs resulting in the reduction of its activity at mRNA and protein level (Samols et al., 2007). However, there are genes that can be targeted by different viral miRNAs encoded by different viruses, e.g. MICB (major histocompatibility complex class I-related chain B) gene is targeted by ebv-miR-BART2-5p, hcmv-miR-UL112 and kshv-miR-K12-7 that result in the reduction of stress-induced natural killer (NK) cell mediated killing of infected cells (Nachmani et al., 2009). Similarly, human miRNAs viz. hsa-miR-122, hsa-miR-199a, hsa-miR-30, hsa-miR-573, hsa-miR-411 help in HCV replication are also found to influence the viral infection (Lanford et al., 2010; Kumar, 2011; Li et al., 2017). Likewise, hsa-miR-155 results in EBV-regulated signal transduction pathways by targeting genes like BACH1, ZIC3, HIVEP2, CEBPB etc. (Yin et al., 2008). Similarly, hsa-miR-199a-3p (Santhakumar et al., 2010) and hsa-miR-613 (Wang et al., 2017) were found downregulated in HCMV infection targeting AKT1 and ARG2 genes, respectively. Few repositories have been developed that contain viral miRNA information for different organisms, e.g. ‘miRBase’ (Kozomara et al., 2019), ‘ViTa’ (Hsu et al., 2007), ‘Vir-Mir db’ (Li et al., 2008) and ‘VIRmiRNA’ (Qureshi et al., 2014). In this study, we provide an integrated platform for human/viral miRNA with their expression and targets.
‘DEmiRVIR’ sub-database has been developed to provide the list of differentially expressed miRNAs (DEmiRs) of human and viruses. These miRNAs have been identified using various profiling methods like RT-PCR, NGS-based techniques etc. This sub-database incorporates unique 507 and 426 human miRNAs that are upregulated and downregulated respectively. While 258 human miRNAs were both upregulated and downregulated during viral infections as reported in different studies. We found that human miRNAs hsa-miR-25 and hsa-miR-210 were upregulated in maximum seven viral infections. These miRNAs are shown to play important role in cancer cell proliferation, metastasis (Wong et al., 2012; Peta et al., 2018), and also involved in KSHV (Viollet et al., 2017) and HIV infections (Modai et al., 2019). Likewise, hsa-miR-145 was downregulated during eight viral infections. This miRNA helps in oncogene expression at pre- and post-transcriptional level (Ye et al., 2019) and was downregulated in MCV + ve samples associated with non-small cell lung cancer (NSCLC) (Lasithiotaki et al., 2017). Likewise, hsa-miR-23 was downregulated in seven viral infections and differentially expressed in burkitt lymphomas (BL) vs. extramedullary plasmacytoma (EMPC) (Ambrosio et al., 2017). This miRNA can be used as a biomarker in molecular diagnosis (Fatmi et al., 2020). Further, hsa-let-7 was both upregulated and downregulated during eight viral infections reported in different studies. For example, hsa-let-7a upregulated in EBV-positive cells (Mansouri et al., 2014) and downregulated in SNT16 cells, which is an EBV-infected cell line (Alles et al., 2016). Likewise, hsa-let-7e upregulated in peripheral blood mononuclear cells (PBMCs) of chronic hepatitis C (CHC) patients compared with the healthy donors HCV (Chang et al., 2014) and downregulated in human hepatoma cells (Steuerwald et al., 2010).
Few repositories have been developed that encompass miRNA expression data viz. ‘mirEX 2.0’ resource for plant miRNA expression profiling (Zielezinski et al., 2015), ‘HMED’ database for human miRNA expression profiling that includes tissue and disease-specific miRNAs (Gong et al., 2014), ‘PmiRExAt’ is a plant miRNA expression database (Gurjar et al., 2016), ‘miRmine’ is a resource for human miRNA expression profiling (Panwar et al., 2017) etc. The ‘miR2Disease’ database only contains information on hepatitis B and C virus showing descriptions of the miRNA expression pattern (Jiang et al., 2009). Therefore, ‘DEmiRVIR’ is the first dedicated resource representing miRNA expression data for 21 viral infections.
The ‘AntiVmiR’ sub-database holds information about the human and other host miRNAs that shows the antiviral effect. hsa-miR-21 is the most explored miRNA found in 9 viruses viz., BKV, HBV, HCV, HIV, HPV, JCV, KSHV, MCV and SIV. hsa-miR-21 plays an essential role in causing viral infections associated with cancer (Lasithiotaki et al., 2017). It can be used as a biomarker for HBV infection, a risk factor in causing hepatocellular carcinoma (HCC) (Liu et al., 2011). hsa-miR-155 is the second most explored miRNA that shows its antiviral activity against eight viruses viz., EBV, HBV, HIV, HSV1, MCV, SFV, VSV and WNV. This miRNA plays an important role in inflammation, autoimmunity, cancer etc. And can be used as a disease biomarker (Alivernini et al., 2017). Further, hsa-miR-155 also helps control hypoxia-inducible factor 1α (HIF-1α) and promotes angiogenesis (Yang et al., 2016). Further, a few miRNAs were also reported in the literature that shows their proviral activity against HCV, viz. hsa-miR-122, hsa-miR-135a, hsa-miR-151–5p, hsa-miR-514, hsa-miR-548, hsa-miR-607, hsa-miR-191*, hsa-miR-17–5p, hsa-miR-589, and hsa-miR-657 (Kim et al., 2016; Li et al., 2017; Sodroski et al., 2019). Sodroski C et al. study suggests that host factors like RIPK2 (receptor-interacting serine/threonine kinase 2), MYD88 (myeloid differentiation primary response 88) result in the proviral effect of hsa-miR-135a on HCV (Sodroski et al., 2019). Kim GW et al., shows the proviral activity of hsa-miR-122 by inhibiting GLD-2, a non-canonical cytoplasmic poly(A) polymerase (Kim et al., 2016). Therefore, ‘AntiVmiR’ is a dedicated repository that provides the information on human and other host miRNA showing antiviral effects.
‘VIRmiRNA2’ sub-database is an exhaustive resource for viral miRNA encompassing 3,340 viral miRNA entries for 50 viruses. In the previous repository, ‘VIRmiRNA’ contains 1,308 viral miRNA entries from 44 viruses, while ‘miRBase’ has 532 viral miRNA entries from 34 viruses (Qureshi et al., 2014). We have incorporated six new viruses in the database, i.e., Hepatitis A virus (HAV), Hepatitis B virus (HeBV), Japanese macaque rhadinovirus (JMRV), Murine (Mouse) cytomegalovirus (MCMV), Simian foamy virus (SFV) and Tiger frog virus (TFV). The current repository has about a 2.5-fold increase than ‘VIRmiRNA’ and a 6-fold increase than the miRBase data.
The ‘VIRmiRNA2’ sub-database also includes isomeric viral miRNA sequences for 14 viruses. Isomirs are miRNA variants generated from a single miRNA having variations in length and sequence. Majority of the isomirs were derived from the argonaute proteins, as reported in different studies (Martí et al., 2010; Cloonan et al., 2011). In our database, PRV has the maximum number of isomirs detected from the sequencing library using the infected PK-15 cells. These viral isomirs have a high sequence identity except terminal sequences/repeats (Wu et al., 2012). Likewise, viral isomirs for RRV were also identified using deep sequencing by analyzing the RNA expression using RRV-infected B-cell lymphoma and retroperitoneal fibromatosis tissues and their expression level was checked using 293T cells (Umbach et al., 2010). Therefore, ‘VIRmiRNA2’ provides exhaustive information about the viral miRNAs and isomirs.
‘VIRmiRTar2’ sub-database holds updated information of experimentally validated targets of viral miRNAs from 21 viruses. Gottwein E et al. study reported majority of target genes, i.e. 5060 targets for KSHV and EBV (Gottwein et al., 2011). The study suggested that KSHV miRNAs target >2000 genes during KSHV pathogenesis, while EBV miRNAs also target >2,900 of these genes by using distinct binding sites. Further, Parnas O et al. reported a total of 1,413 targets including 554 for MDV1 and 859 for MDV2 (Parnas et al., 2014). This study performs mRNA analysis targeted by miRNAs expressed in the chicken T-cell line, i.e. MSB1, which naturally gets infected with MDV-1 and MDV-2. Moreover, we identified CASP3 (Caspase 3) targeted by a maximum 23 miRNAs encoded by three viruses, viz., EBV, KSHV and MDV2. Harold C et al. study showed that CASP3 is a putative target for one or more EBV miRNAs. They cloned the 3′-UTR region of CASP3 using a dual-luciferase reporter vector and further co-transfected HEK293T cells with EBV miRNAs (Harold et al., 2016). Likewise, THBS1 (thrombospondin 1) is targeted by 17 miRNAs of KSHV. Samols MA et al. study revealed that THBS1 is down-regulated more than10-fold by KSHV miRNAs that leads to the reduction of TGF-beta activity (Samols et al., 2007). Repositories have been developed for experimentally validated miRNA targets, e.g. ‘miRTarBase’, that contain target information for only three viruses, i.e. EBV, KSHV and HCMV (Huang et al., 2020). ‘TargetScan’ predicted the miRNA target sites in the mammalian mRNAs (Agarwal et al., 2015). Likewise, there are few resources for predicted targets of viral miRNAs like ‘ViTa’ (Hsu et al., 2007), ‘RepTar’ (Elefant et al., 2011), ‘vHOT’ (Kim et al., 2012), ‘Vir-Mir db’ (Li et al., 2008) etc. But these resources only furnish information about the predicted targets of viral miRNA. In this study, we are providing experimentally validated targets of viral miRNAs encoded by 21 viruses.
Conclusion
‘AntiVIRmiR’ is a miRNA repository that includes the human and virus miRNA expression data during the viral infection. In this resource, we have provided exhaustive information about the differentially expressed human and viral miRNAs and miRNAs having antiviral activity. We have also provided information about the experimentally validated viral miRNAs along with the isomirs and their targets. The resource would assist in understanding the host-virus interactions and may also be useful in developing miRNA-based therapeutics.
Data availability statement
The datasets presented in this study can be found in online repositories. The names of the repository/repositories and accession number(s) can be found in the article/Supplementary Material.
Author contributions
MK conceived, designed, and supervised this study. AT performed the data collection, curation, network analysis and developed the web server. AT and MK performed the data interpretation and wrote the manuscript.
Funding
This work was supported by the grants from the CSIR-Institute of Microbial Technology, Council of Scientific and Industrial Research (CSIR) (STS0038) and Indian Council of Medical Research [(ICMR), ISRM/11 (18)/2017].
Acknowledgments
We acknowledge the infrastructure support of the Department of Biotechnology, Government of India (STS0038) and Indian Council of Medical Research [(ICMR), ISRM/11(18)/2017].
Conflict of interest
The authors declare that the research was conducted in the absence of any commercial or financial relationships that could be construed as a potential conflict of interest.
Publisher’s note
All claims expressed in this article are solely those of the authors and do not necessarily represent those of their affiliated organizations, or those of the publisher, the editors and the reviewers. Any product that may be evaluated in this article, or claim that may be made by its manufacturer, is not guaranteed or endorsed by the publisher.
Supplementary material
The Supplementary Material for this article can be found online at: https://www.frontiersin.org/articles/10.3389/fgene.2022.971852/full#supplementary-material
References
Agarwal, V., Bell, G. W., Nam, J.-W., and Bartel, D. P. (2015). Predicting effective microRNA target sites in mammalian mRNAs. eLife 4. doi:10.7554/eLife.05005
Alivernini, S., Gremese, E., McSharry, C., Tolusso, B., Ferraccioli, G., McInnes, I. B., et al. (2017). MicroRNA-155-at the critical interface of innate and adaptive immunity in arthritis. Front. Immunol. 8, 1932. doi:10.3389/fimmu.2017.01932
Alles, J., Menegatti, J., Motsch, N., Hart, M., Eichner, N., Reinhardt, R., et al. (2016). miRNA expression profiling of Epstein-Barr virus-associated NKTL cell lines by Illumina deep sequencing. FEBS open bio 6, 251–263. doi:10.1002/2211-5463.12027
Ambrosio, M. R., Mundo, L., Gazaneo, S., Picciolini, M., Vara, P. S., Sayed, S., et al. (2017). MicroRNAs sequencing unveils distinct molecular subgroups of plasmablastic lymphoma. Oncotarget 8, 107356–107373. doi:10.18632/oncotarget.22219
Auyeung, V. C., Ulitsky, I., McGeary, S. E., and Bartel, D. P. (2013). Beyond secondary structure: Primary-sequence determinants license pri-miRNA hairpins for processing. Cell 152, 844–858. doi:10.1016/j.cell.2013.01.031
Backes, C., Kehl, T., Stöckel, D., Fehlmann, T., Schneider, L., Meese, E., et al. (2017). miRPathDB: a new dictionary on microRNAs and target pathways. Nucleic Acids Res. 45, D90–D96. doi:10.1093/nar/gkw926
Bruscella, P., Bottini, S., Baudesson, C., Pawlotsky, J.-M., Feray, C., and Trabucchi, M. (2017). Viruses and miRNAs: More friends than foes. Front. Microbiol. 8, 824. doi:10.3389/fmicb.2017.00824
Chang, C.-C., Lin, C.-C., Hsieh, W.-L., Lai, H.-W., Tsai, C.-H., and Cheng, Y.-W. (2014). MicroRNA expression profiling in PBMCs: A potential diagnostic biomarker of chronic hepatitis C.. C. Dis. markers 2014, 367157. doi:10.1155/2014/367157
Cho, S., Jang, I., Jun, Y., Yoon, S., Ko, M., Kwon, Y., et al. (2013). MiRGator v3.0: A microRNA portal for deep sequencing, expression profiling and mRNA targeting. Nucleic Acids Res. 41, D252–D257. doi:10.1093/nar/gks1168
Chou, C.-H., Shrestha, S., Yang, C.-D., Chang, N.-W., Lin, Y.-L., Liao, K.-W., et al. (2018). miRTarBase update 2018: a resource for experimentally validated microRNA-target interactions. Nucleic Acids Res. 46, D296–D302. doi:10.1093/nar/gkx1067
Cloonan, N., Wani, S., Xu, Q., Gu, J., Lea, K., Heater, S., et al. (2011). MicroRNAs and their isomiRs function cooperatively to target common biological pathways. Genome Biol. 12, R126. doi:10.1186/gb-2011-12-12-r126
Denli, A. M., Tops, B. B. J., Plasterk, R. H. A., Ketting, R. F., and Hannon, G. J. (2004). Processing of primary microRNAs by the Microprocessor complex. Nature 432, 231–235. doi:10.1038/nature03049
Elefant, N., Berger, A., Shein, H., Hofree, M., Margalit, H., and Altuvia, Y. (2011). RepTar: A database of predicted cellular targets of host and viral miRNAs. Nucleic Acids Res. 39, D188–D194. doi:10.1093/nar/gkq1233
Fatmi, A., Rebiahi, S. A., Chabni, N., Zerrouki, H., Azzaoui, H., Elhabiri, Y., et al. (2020). miRNA-23b as a biomarker of culture-positive neonatal sepsis. Mol. Med. 26, 94. doi:10.1186/s10020-020-00217-8
Glazov, E. A., Horwood, P. F., Assavalapsakul, W., Kongsuwan, K., Mitchell, R. W., Mitter, N., et al. (2010). Characterization of microRNAs encoded by the bovine herpesvirus 1 genome. J. Gen. Virol. 91, 32–41. doi:10.1099/vir.0.014290-0
Glogovitis, I., Yahubyan, G., Würdinger, T., Koppers-Lalic, D., and Baev, V. (2020). isomiRs-hidden soldiers in the miRNA regulatory army, and how to find them? Biomolecules 11, 41. doi:10.3390/biom11010041
Gong, J., Wu, Y., Zhang, X., Liao, Y., Sibanda, V. L., Liu, W., et al. (2014). Comprehensive analysis of human small RNA sequencing data provides insights into expression profiles and miRNA editing. RNA Biol. 11, 1375–1385. doi:10.1080/15476286.2014.996465
Gottwein, E., Corcoran, D. L., Mukherjee, N., Skalsky, R. L., Hafner, M., Nusbaum, J. D., et al. (2011). Viral microRNA targetome of KSHV-infected primary effusion lymphoma cell lines. Cell Host Microbe 10, 515–526. doi:10.1016/j.chom.2011.09.012
Grundhoff, A., and Sullivan, C. S. (2011). Virus-encoded microRNAs. Virology 411, 325–343. doi:10.1016/j.virol.2011.01.002
Gurjar, A. K. S., Panwar, A. S., Gupta, R., and Mantri, S. S. (2016). PmiRExAt: Plant miRNA expression atlas database and web applications. Database. 2016, baw060. doi:10.1093/database/baw060
Ha, M., and Kim, V. N. (2014). Regulation of microRNA biogenesis. Nat. Rev. Mol. Cell Biol. 15, 509–524. doi:10.1038/nrm3838
Han, J., Lee, Y., Yeom, K.-H., Kim, Y.-K., Jin, H., and Kim, V. N. (2004). The Drosha-DGCR8 complex in primary microRNA processing. Genes Dev. 18, 3016–3027. doi:10.1101/gad.1262504
Harold, C., Cox, D., and Riley, K. J. (2016). Epstein-Barr viral microRNAs target caspase 3. Virol. J. 13, 145. doi:10.1186/s12985-016-0602-7
Hsu, P. W.-C., Lin, L.-Z., Hsu, S.-D., Hsu, J. B.-K., and Huang, H.-D. (2007). ViTa: Prediction of host microRNAs targets on viruses. Nucleic Acids Res. 35, D381–D385. doi:10.1093/nar/gkl1009
Hsu, S.-D., Lin, F.-M., Wu, W.-Y., Liang, C., Huang, W.-C., Chan, W.-L., et al. (2011). miRTarBase: a database curates experimentally validated microRNA-target interactions. Nucleic Acids Res. 39, D163–D169. doi:10.1093/nar/gkq1107
Huang, H.-Y., Lin, Y.-C.-D., Li, J., Huang, K.-Y., Shrestha, S., Hong, H.-C., et al. (2020). miRTarBase 2020: updates to the experimentally validated microRNA-target interaction database. Nucleic Acids Res. 48, D148–D154. doi:10.1093/nar/gkz896
Huang, Y., Shen, X. J., Zou, Q., Wang, S. P., Tang, S. M., and Zhang, G. Z. (2011). Biological functions of microRNAs: A review. J. Physiol. Biochem. 67, 129–139. doi:10.1007/s13105-010-0050-6
Huang, Z., Shi, J., Gao, Y., Cui, C., Zhang, S., Li, J., et al. (2019). HMDD v3.0: A database for experimentally supported human microRNA-disease associations. Nucleic Acids Res. 47, D1013–D1017. doi:10.1093/nar/gky1010
Jiang, Q., Wang, Y., Hao, Y., Juan, L., Teng, M., Zhang, X., et al. (2009). miR2Disease: a manually curated database for microRNA deregulation in human disease. Nucleic Acids Res. 37, D98–D104. doi:10.1093/nar/gkn714
John, B., Enright, A. J., Aravin, A., Tuschl, T., Sander, C., and Marks, D. S. (2004). Human MicroRNA targets. PLoS Biol. 2, e363. doi:10.1371/journal.pbio.0020363
Kehl, T., Kern, F., Backes, C., Fehlmann, T., Stöckel, D., Meese, E., et al. (2020). miRPathDB 2.0: a novel release of the miRNA Pathway Dictionary Database. Nucleic Acids Res. 48, D142–D147. doi:10.1093/nar/gkz1022
Kieffer-Kwon, P., Happel, C., Uldrick, T. S., Ramalingam, D., and Ziegelbauer, J. M. (2015). KSHV MicroRNAs repress tropomyosin 1 and increase anchorage-independent growth and endothelial tube formation. PloS one 10, e0135560. doi:10.1371/journal.pone.0135560
Kiezun, A., Artzi, S., Modai, S., Volk, N., Isakov, O., and Shomron, N. (2012). miRviewer: a multispecies microRNA homologous viewer. BMC Res. Notes 5, 92. doi:10.1186/1756-0500-5-92
Kim, G.-W., Lee, S.-H., Cho, H., Kim, M., Shin, E.-C., and Oh, J.-W. (2016). Hepatitis C virus core protein promotes miR-122 destabilization by inhibiting GLD-2. PLoS Pathog. 12, e1005714. doi:10.1371/journal.ppat.1005714
Kim, H., Park, S., Min, H., and Yoon, S. (2012). vHoT: a database for predicting interspecies interactions between viral microRNA and host genomes. Arch. Virol. 157, 497–501. doi:10.1007/s00705-011-1181-y
Kozomara, A., Birgaoanu, M., and Griffiths-Jones, S. (2019). miRBase: from microRNA sequences to function. Nucleic Acids Res. 47, D155–D162. doi:10.1093/nar/gky1141
Kumar, A. (2011). MicroRNA in HCV infection and liver cancer. Biochim. Biophys. Acta 1809, 694–699. doi:10.1016/j.bbagrm.2011.07.010
Lagos-Quintana, M., Rauhut, R., Yalcin, A., Meyer, J., Lendeckel, W., and Tuschl, T. (2002). Identification of tissue-specific microRNAs from mouse. Curr. Biol. 12, 735–739. doi:10.1016/s0960-9822(02)00809-6
Lanford, R. E., Hildebrandt-Eriksen, E. S., Petri, A., Persson, R., Lindow, M., Munk, M. E., et al. (2010). Therapeutic silencing of microRNA-122 in primates with chronic hepatitis C virus infection. Sci. (New York, N.Y.) 327, 198–201. doi:10.1126/science.1178178
Lasithiotaki, I., Tsitoura, E., Koutsopoulos, A., Lagoudaki, E., Koutoulaki, C., Pitsidianakis, G., et al. (2017). Aberrant expression of miR-21, miR-376c and miR-145 and their target host genes in Merkel cell polyomavirus-positive non-small cell lung cancer. Oncotarget 8, 112371–112383. doi:10.18632/oncotarget.11222
Lee, R. C., and Ambros, V. (2001). An extensive class of small RNAs in Caenorhabditis elegans. Sci. (New York, N.Y.) 294, 862–864. doi:10.1126/science.1065329
Lee, R. C., Feinbaum, R. L., and Ambros, V. (1993). The C. elegans heterochronic gene lin-4 encodes small RNAs with antisense complementarity to lin-14. Cell 75, 843–854. doi:10.1016/0092-8674(93)90529-y
Li, C., Mou, C., Swartz, M. D., Yu, B., Bai, Y., Tu, Y., et al. (2020). dbMTS: A comprehensive database of putative human microRNA target site SNVs and their functional predictions. Hum. Mutat. 41, 1123–1130. doi:10.1002/humu.24020
Li, Q., Lowey, B., Sodroski, C., Krishnamurthy, S., Alao, H., Cha, H., et al. (2017). Cellular microRNA networks regulate host dependency of hepatitis C virus infection. Nat. Commun. 8, 1789. doi:10.1038/s41467-017-01954-x
Li, S.-C., Shiau, C.-K., and Lin, W.-C. (2008). Vir-mir db: Prediction of viral microRNA candidate hairpins. Nucleic Acids Res. 36, D184–D189. doi:10.1093/nar/gkm610
Li, Y., Qiu, C., Tu, J., Geng, B., Yang, J., Jiang, T., et al. (2014). HMDD v2.0: A database for experimentally supported human microRNA and disease associations. Nucleic Acids Res. 42, D1070–D1074. doi:10.1093/nar/gkt1023
Lin, X., Li, X., Liang, D., and Lan, K. (2012). MicroRNAs and unusual small RNAs discovered in Kaposi’s sarcoma-associated herpesvirus virions. J. Virol. 86, 12717–12730. doi:10.1128/JVI.01473-12
Liu, W.-H., Yeh, S.-H., and Chen, P.-J. (2011). Role of microRNAs in Hepatitis B virus replication and pathogenesis. Biochim. Biophys. Acta 1809, 678–685. doi:10.1016/j.bbagrm.2011.04.008
Mansouri, S., Pan, Q., Blencowe, B. J., Claycomb, J. M., and Frappier, L. (2014). Epstein-Barr virus EBNA1 protein regulates viral latency through effects on let-7 microRNA and dicer. J. Virol. 88, 11166–11177. doi:10.1128/JVI.01785-14
Manyam, G., Ivan, C., Calin, G. A., and Coombes, K. R. (2013). targetHub: a programmable interface for miRNA-gene interactions. Bioinforma. Oxf. Engl. 29, 2657–2658. doi:10.1093/bioinformatics/btt439
Martí, E., Pantano, L., Bañez-Coronel, M., Llorens, F., Miñones-Moyano, E., Porta, S., et al. (2010). A myriad of miRNA variants in control and Huntington’s disease brain regions detected by massively parallel sequencing. Nucleic Acids Res. 38, 7219–7235. doi:10.1093/nar/gkq575
Modai, S., Farberov, L., Herzig, E., Isakov, O., Hizi, A., and Shomron, N. (2019). HIV-1 infection increases microRNAs that inhibit Dicer1, HRB and HIV-EP2, thereby reducing viral replication. PloS one 14, e0211111. doi:10.1371/journal.pone.0211111
Mooney, C., Becker, B. A., Raoof, R., and Henshall, D. C. (2016). EpimiRBase: A comprehensive database of microRNA-epilepsy associations. Bioinforma. Oxf. Engl. 32, 1436–1438. doi:10.1093/bioinformatics/btw008
Nachmani, D., Stern-Ginossar, N., Sarid, R., and Mandelboim, O. (2009). Diverse herpesvirus microRNAs target the stress-induced immune ligand MICB to escape recognition by natural killer cells. Cell Host Microbe 5, 376–385. doi:10.1016/j.chom.2009.03.003
Nam, S., Kim, B., Shin, S., and Lee, S. (2008). miRGator: an integrated system for functional annotation of microRNAs. Nucleic Acids Res. 36, D159–D164. doi:10.1093/nar/gkm829
O’Brien, J., Hayder, H., Zayed, Y., and Peng, C. (2018). Overview of MicroRNA biogenesis, mechanisms of actions, and circulation. Front. Endocrinol. 9, 402. doi:10.3389/fendo.2018.00402
Panwar, B., Omenn, G. S., and Guan, Y. (2017). miRmine: a database of human miRNA expression profiles. Bioinforma. Oxf. Engl. 33, 1554–1560. doi:10.1093/bioinformatics/btx019
Parnas, O., Corcoran, D. L., and Cullen, B. R. (2014). Analysis of the mRNA targetome of microRNAs expressed by Marek’s disease virus. mBio 5, e01060–13. doi:10.1128/mBio.01060-13
Peta, E., Sinigaglia, A., Masi, G., Di Camillo, B., Grassi, A., Trevisan, M., et al. (2018). HPV16 E6 and E7 upregulate the histone lysine demethylase KDM2B through the c-MYC/miR-146a-5p axys. Oncogene 37, 1654–1668. doi:10.1038/s41388-017-0083-1
Pfeffer, S., Sewer, A., Lagos-Quintana, M., Sheridan, R., Sander, C., Grässer, F. A., et al. (2005). Identification of microRNAs of the herpesvirus family. Nat. Methods 2, 269–276. doi:10.1038/nmeth746
Pfeffer, S., Zavolan, M., Grässer, F. A., Chien, M., Russo, J. J., Ju, J., et al. (2004). Identification of virus-encoded microRNAs. Sci. (New York, N.Y.) 304, 734–736. doi:10.1126/science.1096781
Qureshi, A., Thakur, N., Monga, I., Thakur, A., and Kumar, M. (2014). VIRmiRNA: A comprehensive resource for experimentally validated viral miRNAs and their targets. Database. 2014, bau103. doi:10.1093/database/bau103
Reinhart, B. J., Slack, F. J., Basson, M., Pasquinelli, A. E., Bettinger, J. C., Rougvie, A. E., et al. (2000). The 21-nucleotide let-7 RNA regulates developmental timing in Caenorhabditis elegans. Nature 403, 901–906. doi:10.1038/35002607
Ruby, J. G., Jan, C. H., and Bartel, D. P. (2007). Intronic microRNA precursors that bypass Drosha processing. Nature 448, 83–86. doi:10.1038/nature05983
Rupaimoole, R., and Slack, F. J. (2017). MicroRNA therapeutics: Towards a new era for the management of cancer and other diseases. Nat. Rev. Drug Discov. 16, 203–222. doi:10.1038/nrd.2016.246
Samols, M. A., Skalsky, R. L., Maldonado, A. M., Riva, A., Lopez, M. C., Baker, H. V., et al. (2007). Identification of cellular genes targeted by KSHV-encoded microRNAs. PLoS Pathog. 3, e65. doi:10.1371/journal.ppat.0030065
Santhakumar, D., Forster, T., Laqtom, N. N., Fragkoudis, R., Dickinson, P., Abreu-Goodger, C., et al. (2010). Combined agonist-antagonist genome-wide functional screening identifies broadly active antiviral microRNAs. Proc. Natl. Acad. Sci. U. S. A. 107, 13830–13835. doi:10.1073/pnas.1008861107
Scagnolari, C., Zingariello, P., Vecchiet, J., Selvaggi, C., Racciatti, D., Taliani, G., et al. (2010). Differential expression of interferon-induced microRNAs in patients with chronic hepatitis C virus infection treated with pegylated interferon alpha. Virol. J. 7, 311. doi:10.1186/1743-422X-7-311
Shan, S. W., Fang, L., Shatseva, T., Rutnam, Z. J., Yang, X., Du, W., et al. (2013). Mature miR-17-5p and passenger miR-17-3p induce hepatocellular carcinoma by targeting PTEN, GalNT7 and vimentin in different signal pathways. J. Cell Sci. 126, 1517–1530. doi:10.1242/jcs.122895
Shannon, P., Markiel, A., Ozier, O., Baliga, N. S., Wang, J. T., Ramage, D., et al. (2003). Cytoscape: A software environment for integrated models of biomolecular interaction networks. Genome Res. 13, 2498–2504. doi:10.1101/gr.1239303
Skalsky, R. L., and Cullen, B. R. (2010). Viruses, microRNAs, and host interactions. Annu. Rev. Microbiol. 64, 123–141. doi:10.1146/annurev.micro.112408.134243
Sodroski, C., Lowey, B., Hertz, L., Jake Liang, T., and Li, Q. (2019). MicroRNA-135a modulates hepatitis C virus genome replication through downregulation of host antiviral factors. Virol. Sin. 34, 197–210. doi:10.1007/s12250-018-0055-9
Solomon, J., Kern, F., Fehlmann, T., Meese, E., and Keller, A. (2020). HumiR: Web services, tools and databases for exploring human microRNA data. Biomolecules 10, E1576. doi:10.3390/biom10111576
Steuerwald, N. M., Parsons, J. C., Bennett, K., Bates, T. C., and Bonkovsky, H. L. (2010). Parallel microRNA and mRNA expression profiling of (genotype 1b) human hepatoma cells expressing hepatitis C virus. Liver Int. 30, 1490–1504. doi:10.1111/j.1478-3231.2010.02321.x
Su, B., Fu, Y., Liu, Y., Wu, H., Ma, P., Zeng, W., et al. (2018). Potential application of MicroRNA profiling to the diagnosis and prognosis of HIV-1 infection. Front. Microbiol. 9, 3185. doi:10.3389/fmicb.2018.03185
Szcześniak, M. W., Deorowicz, S., Gapski, J., Kaczyński, Ł., and Makalowska, I. (2012). miRNEST database: an integrative approach in microRNA search and annotation. Nucleic Acids Res. 40, D198–D204. doi:10.1093/nar/gkr1159
Takada, S., Berezikov, E., Yamashita, Y., Lagos-Quintana, M., Kloosterman, W. P., Enomoto, M., et al. (2006). Mouse microRNA profiles determined with a new and sensitive cloning method. Nucleic Acids Res. 34, e115. doi:10.1093/nar/gkl653
Treiber, T., Treiber, N., and Meister, G. (2019). Regulation of microRNA biogenesis and its crosstalk with other cellular pathways. Nat. Rev. Mol. Cell Biol. 20, 5–20. doi:10.1038/s41580-018-0059-1
Umbach, J. L., Strelow, L. I., Wong, S. W., and Cullen, B. R. (2010). Analysis of rhesus rhadinovirus microRNAs expressed in virus-induced tumors from infected rhesus macaques. Virology 405, 592–599. doi:10.1016/j.virol.2010.06.036
Vergoulis, T., Kanellos, I., Kostoulas, N., Georgakilas, G., Sellis, T., Hatzigeorgiou, A., et al. (2015). mirPub: a database for searching microRNA publications. Bioinforma. Oxf. Engl. 31, 1502–1504. doi:10.1093/bioinformatics/btu819
Viollet, C., Davis, D. A., Tekeste, S. S., Reczko, M., Ziegelbauer, J. M., Pezzella, F., et al. (2017). RNA sequencing reveals that Kaposi sarcoma-associated herpesvirus infection mimics hypoxia gene expression signature. PLoS Pathog. 13, e1006143. doi:10.1371/journal.ppat.1006143
Wang, W.-C., Lin, F.-M., Chang, W.-C., Lin, K.-Y., Huang, H.-D., and Lin, N.-S. (2009). miRExpress: analyzing high-throughput sequencing data for profiling microRNA expression. BMC Bioinforma. 10, 328. doi:10.1186/1471-2105-10-328
Wang, Y., Zhao, P., Qian, D., Hu, M., Zhang, L., Shi, H., et al. (2017). MicroRNA-613 is downregulated in HCMV-positive glioblastoma and inhibits tumour progression by targeting arginase-2. Tumour Biol. 39, 1010428317712512. doi:10.1177/1010428317712512
Wong, A. M. G., Kong, K. L., Tsang, J. W. H., Kwong, D. L. W., and Guan, X.-Y. (2012). Profiling of Epstein-Barr virus-encoded microRNAs in nasopharyngeal carcinoma reveals potential biomarkers and oncomirs. Cancer 118, 698–710. doi:10.1002/cncr.26309
Wu, Y.-Q., Chen, D.-J., He, H.-B., Chen, D.-S., Chen, L.-L., Chen, H.-C., et al. (2012). Pseudorabies virus infected porcine epithelial cell line generates a diverse set of host microRNAs and a special cluster of viral microRNAs. PloS one 7, e30988. doi:10.1371/journal.pone.0030988
Xie, B., Ding, Q., Han, H., and Wu, D. (2013a). miRCancer: a microRNA-cancer association database constructed by text mining on literature. Bioinforma. Oxf. Engl. 29, 638–644. doi:10.1093/bioinformatics/btt014
Xie, M., Li, M., Vilborg, A., Lee, N., Shu, M.-D., Yartseva, V., et al. (2013b). Mammalian 5’-capped microRNA precursors that generate a single microRNA. Cell 155, 1568–1580. doi:10.1016/j.cell.2013.11.027
Yang, D., Wang, J., Xiao, M., Zhou, T., and Shi, X. (2016). Role of mir-155 in controlling HIF-1α level and promoting endothelial cell maturation. Sci. Rep. 6, 35316. doi:10.1038/srep35316
Yang, X., Du, W. W., Li, H., Liu, F., Khorshidi, A., Rutnam, Z. J., et al. (2013). Both mature miR-17-5p and passenger strand miR-17-3p target TIMP3 and induce prostate tumor growth and invasion. Nucleic Acids Res. 41, 9688–9704. doi:10.1093/nar/gkt680
Ye, D., Shen, Z., and Zhou, S. (2019). Function of microRNA-145 and mechanisms underlying its role in malignant tumor diagnosis and treatment. Cancer Manag. Res. 11, 969–979. doi:10.2147/CMAR.S191696
Ye, X., Huang, N., Liu, Y., Paroo, Z., Huerta, C., Li, P., et al. (2011). Structure of C3PO and mechanism of human RISC activation. Nat. Struct. Mol. Biol. 18, 650–657. doi:10.1038/nsmb.2032
Yeom, K.-H., Lee, Y., Han, J., Suh, M. R., and Kim, V. N. (2006). Characterization of DGCR8/Pasha, the essential cofactor for Drosha in primary miRNA processing. Nucleic Acids Res. 34, 4622–4629. doi:10.1093/nar/gkl458
Yin, Q., McBride, J., Fewell, C., Lacey, M., Wang, X., Lin, Z., et al. (2008). MicroRNA-155 is an Epstein-Barr virus-induced gene that modulates Epstein-Barr virus-regulated gene expression pathways. J. Virol. 82, 5295–5306. doi:10.1128/JVI.02380-07
Yousef, M., Abdallah, L., and Allmer, J. (2019). maTE: discovering expressed interactions between microRNAs and their targets. Bioinforma. Oxf. Engl. 35, 4020–4028. doi:10.1093/bioinformatics/btz204
Zhang, Z., Yu, J., Li, D., Zhang, Z., Liu, F., Zhou, X., et al. (2010). Pmrd: Plant microRNA database. Nucleic Acids Res. 38, D806–D813. doi:10.1093/nar/gkp818
Zheng, Z., Ke, X., Wang, M., He, S., Li, Q., Zheng, C., et al. (2013). Human microRNA hsa-miR-296-5p suppresses enterovirus 71 replication by targeting the viral genome. J. Virol. 87, 5645–5656. doi:10.1128/JVI.02655-12
Zhuo, Y., Gao, G., Shi, J. A., Zhou, X., and Wang, X. (2013). miRNAs: biogenesis, origin and evolution, functions on virus-host interaction. Cell. Physiol. biochem. 32, 499–510. doi:10.1159/000354455
Keywords: microRNA, target, expression, antiviral, viruses, human host, network, web server
Citation: Thakur A and Kumar M (2022) AntiVIRmiR: A repository of host antiviral miRNAs and their expression along with experimentally validated viral miRNAs and their targets. Front. Genet. 13:971852. doi: 10.3389/fgene.2022.971852
Received: 17 June 2022; Accepted: 16 August 2022;
Published: 08 September 2022.
Edited by:
Aashish Srivastava, Haukeland University Hospital, NorwayReviewed by:
Gopal Pandi, Madurai Kamaraj University, IndiaZhichun Feng, Bayi Children’s Hospital, China
Copyright © 2022 Thakur and Kumar. This is an open-access article distributed under the terms of the Creative Commons Attribution License (CC BY). The use, distribution or reproduction in other forums is permitted, provided the original author(s) and the copyright owner(s) are credited and that the original publication in this journal is cited, in accordance with accepted academic practice. No use, distribution or reproduction is permitted which does not comply with these terms.
*Correspondence: Manoj Kumar, manojk@imtech.res.in