- 1Australian Centre for Precision Health, University of South Australia, Adelaide, SA, Australia
- 2UniSA Allied Health and Human Performance, University of South Australia, Adelaide, SA, Australia
- 3South Australian Health and Medical Research Institute (SAHMRI), Adelaide, SA, Australia
- 4Division of Animal Breeding and Genetics, National Institute of Animal Science (NIAS), Cheonan, Republic of Korea
- 5Department of Genetics and Animal Breeding, Faculty of Veterinary Medicine, Chattogram Veterinary and Animal Sciences University (CVASU), Chattogram, Bangladesh
- 6Cardiovascular Research Centre, Massachusetts General Hospital, Boston, MA, United States
- 7Center for Genomic Medicine, Massachusetts General Hospital, Boston, MA, United States
- 8Program in Medical and Population Genetics and the Cardiovascular Disease Initiative, Broad, Institute of Harvard and Massachusetts Institute of Technology (MIT), Cambridge, MA, United States
- 9School of Environmental and Rural Science, University of New England, Armidale, NSW, Australia
The H-matrix best linear unbiased prediction (HBLUP) method has been widely used in livestock breeding programs. It can integrate all information, including pedigree, genotypes, and phenotypes on both genotyped and non-genotyped individuals into one single evaluation that can provide reliable predictions of breeding values. The existing HBLUP method requires hyper-parameters that should be adequately optimised as otherwise the genomic prediction accuracy may decrease. In this study, we assess the performance of HBLUP using various hyper-parameters such as blending, tuning, and scale factor in simulated and real data on Hanwoo cattle. In both simulated and cattle data, we show that blending is not necessary, indicating that the prediction accuracy decreases when using a blending hyper-parameter <1. The tuning process (adjusting genomic relationships accounting for base allele frequencies) improves prediction accuracy in the simulated data, confirming previous studies, although the improvement is not statistically significant in the Hanwoo cattle data. We also demonstrate that a scale factor,
1 Introduction
Genomic prediction can achieve a more accurate prediction of additive genetic values at an early life stage, compared to the conventional pedigree-based prediction. Genomic prediction has been applied to a broad range of disciplines, including animal breeding (Hayes et al., 2009) and human disease risk prediction (Abraham et al., 2016; Inouye et al., 2018; Khera et al., 2018). The accuracy of genomic prediction is important, which depends on several factors such as marker density, linkage disequilibrium (LD) between the quantitative trait loci (QTLs) and markers, the sample size of reference, the heritability of the trait, the number of QTLs, and the distribution of QTL effects. The prediction accuracy is also determined by the method used (Yan et al., 2022).
Genomic prediction requires genotypic information for both discovery and target samples. Genome-wide single nucleotide polymorphisms (SNPs) are typically used to estimate the genomic relationship matrix (GRM) for the genotyped samples so that breeding values (in livestock) can be estimated for the target samples, given the phenotypic information of discovery samples (De Los Campos et al., 2009; VanRaden et al., 2009). In many cases, we may have individuals with useful phenotypic information that are not genotyped, but they may be linked with genotyped samples through a pedigree, i.e., missing genotype data. To address this problem, a single-step genomic best linear unbiased prediction (ssGBLUP) method was introduced, in which phenotypic information on both genotyped and non-genotyped individuals in the pedigree can be used simultaneously to maximise the prediction accuracy of genotyped target individuals (Legarra et al., 2009; Christensen and Lund, 2010; Christensen et al., 2012; McWhorter et al., 2022).
SsGBLUP uses an H-matrix that is a harmonised matrix of a pedigree-based numerator relationship matrix (NRM) and a GRM; therefore, we will use the term H-matrix best linear unbiased prediction (HBLUP). The H-matrix allows us to use the information of non-genotyped individuals in genomic prediction using a data augmentation technique (see (Legarra et al., 2009; Misztal et al., 2009) and Legarra et al., 2014). HBLUP has been widely used in the genetic evaluation of livestock and has been employed in the national genetic evaluation program in many countries (Gao et al., 2012; McMillan and Swan, 2017; Brown et al., 2018; Chung et al., 2018; Johnston et al., 2018; Meyer et al., 2018; Teissier et al., 2018; Oliveira et al., 2019; Mäntysaari et al., 2020; Alkhoder and Liu, 2021). There are numerous studies reporting that HBLUP outperforms traditional GBLUP (Baloche et al., 2014; Gao et al., 2018; Gowane et al., 2019; Mancisidor et al., 2021).
In HBLUP, there are two main hyper-parameters that can determine its performance. First, blending is one of the hyper-parameters that can provide a weighted sum of genomic and numerator relationships, using an arbitrary weight typically ranging from 0.5 to 0.99 (Meyer et al., 2018). This process is essential because it ensures GRM, which is a positive definite matrix, avoids numerical problems in HBLUP (VanRaden, 2008; Legarra et al., 2009). Second, tuning is another important hyper-parameter that can adjust GRM, accounting for the allele frequencies in the base population that are inferred from the information of NRM (Legarra et al., 2009; Misztal et al., 2009; Chen et al., 2011; Vitezica et al., 2011). Note that GRM is typically based on genotyped samples in the last few generations, whereas NRM includes the information of founders in the base population through the pedigree. Third, a scale factor is a novel hyper-parameter for HBLUP to be introduced in this study, which can generate different kinds of GRMs, accounting for the relationship between allele frequency and per-allele effect size, i.e., per-allele effect sizes vary, depending on a function proportional to [p (1 − p)] α, where p is the allele frequency (Speed et al., 2012; Speed et al., 2017; Schoech et al., 2019; Momin et al., 2023). Negative
In this study, we investigate the three hyper-parameters, blending, tuning, and
2 Material and methods
2.1 Simulated data
QMSim software (Sargolzaei and Schenkel, 2009) was used for simulation since it can efficiently generate a large-scale dataset including genotypic and pedigree information. We simulated three different scenarios that differed in terms of the effective population size, mating design, and family structure. Two different effective population sizes are determined at 100 and 1,000 individuals with 100 generations in order to mimic livestock (a half-sib design) and human (a full-sib design) populations.
I. The historical population consists of 100 generations. For the initial 95 generations, the effective population size (
II. In the second simulation scenario,
III. In the third scenario,
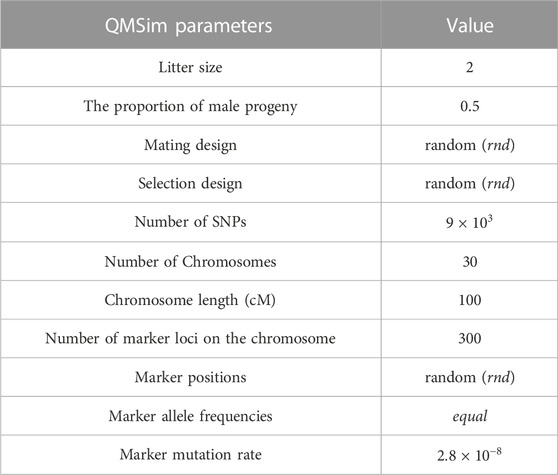
TABLE 1. Parameters of historical population and genotyping data simulation in the first scenario using QMSim software.
In order to simulate the phenotypes of a complex trait based on the simulated genotyped data, we used a model,
where
In the HBLUP analysis, for three simulation scenarios, it is assumed that the pedigree information is available for the last five generations (101–105th generations), and the genotypic information is available for the individuals from the last two generations (104–105th generations), noting that the sample size in each of the last five generations is 1,000. Furthermore, it is noted that the phenotypes are available for all individuals. We conducted 3,000 replicates of the simulations under three different scenarios with specified simulation parameters. By running multiple replicates, we were able to estimate the variance and uncertainty in the results and obtain a more accurate assessment of the effects of different factors on the population. Replicating the simulation multiple times is a common practice in simulation studies as it can increase the reliability and validity of the results by reducing the impact of chance events and providing a more robust assessment of the effects of the factors being studied.
2.2 Real data
2.2.1 Hanwoo cattle data
In this study, we applied statistical analyses to genotypic and phenotypic data from Hanwoo beef cattle. The total number of animals with pedigree information was 84,020, and among them, 13,800 animals were genotyped for 52,791 genome-wide SNPs, and 25,502 animals were recorded for their phenotypes. The number of animals available for both genotypic and phenotypic information was 9,072. The following criteria were applied for quality control (QC) using PLINK: minor allele frequency below 0.01 (MAF), filtering SNPs with a call rate lower than 95% (GENO = 0.05), individual missingness more than 5% (MIND = 0.05), and Hardy–Weinberg Equilibrium p-value threshold lower than 1e-04 (HWE). After QC, the number of individuals did not change, and the SNPs number was 42,795. The Hanwoo beef cattle data included five carcass traits: carcass weight, eye muscle area, back fat thickness, marbling score, and adjusted 12 months weight. The total number of animals with non-missing records for each carcass trait with and without genotypic information can be seen in Table 2.
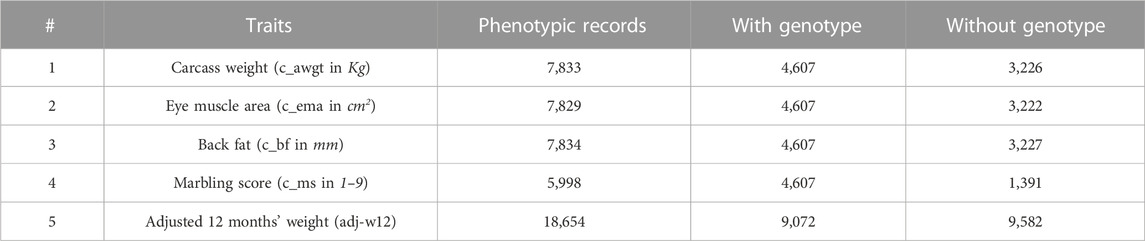
TABLE 2. The number of individuals available for phenotypes with and without genotypic information for five carcass traits in the Hanwoo cattle dataset.
In the HBLUP analysis for the Hanwoo cattle data, animals available for phenotypes and genotypes (
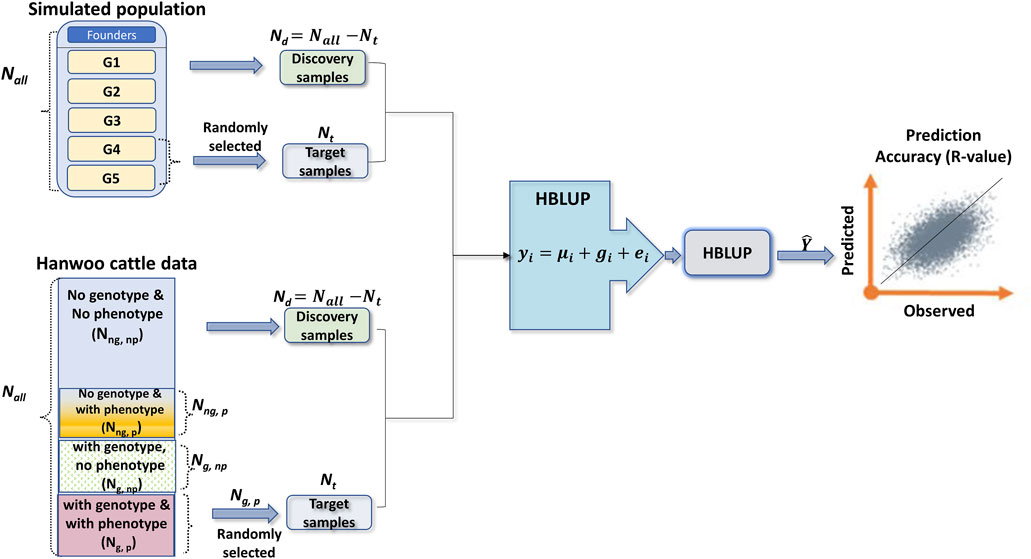
FIGURE 1. A diagram showing the experimental designs and how to select the target and discovery samples for simulated and Hanwoo cattle datasets. In the simulated dataset, the number of founders depends on the simulation scenarios (
2.3 Estimating NRM, GRM, and HRM
2.3.1 Numerator relationship matrix
NRM is denoted as A which is estimated based on the pedigree and has been used in Henderson’s mixed model equation (Henderson, 1975) to obtain estimated breeding values. Following Legarra et al., 2014, A matrix can be formulated as follows.
Where
2.3.2 Scale factor ( ) and GRM
Following Momin et al., 2023, the variance of the
where
where
Note that Eq. 4 with
In the HBLUP analysis, we will vary
2.3.3 H-matrix (HRM) best linear unbiased prediction
In the HBLUP analysis, GRM (G) is computed based on genotypic information, and NRM (A) is estimated using the pedigree information of the population. Following Legarra et al., 2009, given estimated G and A (from Eqs 3, 4), the H matrix can be derived as
In the HBLUP analysis, the simulated data were divided into two groups; one group included the individuals in the first three generations, and the other group included individuals in the last two generations in the current population (101–105th generations). We used the genotypic information of the last two generations and the full pedigree information across the five generations to estimate the H matrix. In cattle data, animals available for phenotypes and genotypes were considered (see Table 2) to estimate GRM, and then the HRM was estimated using a combination of NRM estimated based on whole pedigree (84,020 individuals) and GRM.
2.3.4 Blending
GRM is typically a non-positive definite matrix. In the process of HBLUP, it is usually required to modify GRM to be positive definite so that it can be inverted without any numerical problem (VanRaden, 2008). This modification method is called “blending” which shrinks the genomic relationships toward the pedigree relationships, using an arbitrary weight,
2.3.5 Tuning
The tuning process adjusts GRM, accounting for the allele frequencies in the base population, using the information from NRM that includes the information of founders in the base population through the pedigree (Legarra et al., 2009; Misztal et al., 2009; Chen et al., 2011; Vitezica et al., 2011; Hsu et al., 2017). The tuned GRM (
where J is a matrix with the same size as GRM, all elements are equal to one, and
where I is an array with the size of
Please note that Eqs 8, 9 have been implemented in BLUPf90 (Misztal et al., 2018) as the second and fourth tuning options (i.e., TunedG = 2 or 4).
2.4 Linear mixed model
In the analyses, we used a linear mixed model that can be written as
where
We employed the restricted maximum likelihood (REML) method, fitting the
2.5 Grid search to find optimal hyper-parameters
One of the well-known methods to find the best configuration of hyper-parameters is the grid search (LaValle et al., 2014). In the grid search, all possible combinations of hyper-parameters are considered to evaluate the performance of prediction models. We considered two tuning methods and without tuning (Tune = 0, 1, and 2). The blending step size in the grid search is 0.1 from 0 to 1 and 0.02 from 0.9 to 1.0. Meanwhile, the step size for
2.6 Key performance metrics
This study uses critical performance metrics to evaluate the accuracy and effectiveness of the prediction and estimation methods. The specific performance metrics will depend on the specific research question, the data type, and the prediction method’s goals. Using multiple performance metrics to provide a more comprehensive assessment of the model’s performance is common.
2.6.1 Root Mean squared error (RMSE)
In genomic analysis studies, we often use a performance metric called RMSE to see how good a model is at making predictions. It's like a measuring stick to compare the model’s guesses with the real answers. We calculate RMSE by taking the differences between the model’s predictions and the actual values, squaring them, and then finding the average of those squared differences. Finally, we take the square root of that average. RMSE is useful because it's simple to understand and tells us how far off the model’s predictions are from the real answers, on average. The smaller the RMSE is related the better the model is at making accurate predictions, which means the guesses are closer to the real answers.
2.6.2 R-value
In the study of genes and their effects on physical traits, scientists often use a tool called the Pearson correlation coefficient (R-value). This helps them figure out if there’s a connection between the two things they’re studying. If the coefficient is high and positive, that means when one thing goes up, the other thing tends to go up too. If it’s high and negative, that means when one thing goes up, the other thing tends to go down.
2.6.3 Akaike information criteria (AIC)
In genomic analysis studies, researchers use statistical models to understand the relationship between genes and traits. The Akaike Information Criteria (AIC) is a metric that compares different models and determines the best one. It was developed by Hirotugu Akaike in 1974 (Akaike, 1974) and is based on the principle of maximum likelihood, which aims to estimate the parameters of a statistical model that is most likely to have produced the observed data. The AIC value represents the amount of information the model loses when it approximates the true underlying process. A lower AIC value indicates a better model fit and a higher likelihood of accurately predicting new data. AIC is a valuable metric for model selection because it takes into account both the goodness of fit and the complexity of the model. It penalizes models with more parameters, which can help prevent overfitting and improve the generalisability of the model to new data. Furthermore, AIC can be used in a wide range of statistical models, including linear regression, generalized linear models, and mixed effects models. It plays a crucial role in model selection, allowing us to choose the model that best fits the data while avoiding overfitting and ensuring that the model is generalizable to new data.
3 Results
3.1 Simulated data
Figure 2A shows that the tuning process can improve the phenotypic prediction accuracy (referred to as R-value) when using the simulated data, which is a Pearson correlation coefficient between the observed and predicted phenotypes in the target dataset, confirming previous studies. However, it should be noted that the improvement in prediction accuracy between Blend = 0.9 and Blend = 1 is only 0.003, which may be considered relatively small. The tuning process with the first option (tune = 1; Eq. 8) appears to better perform than the second option (tune = 2; Eq. 9) for this simulated data. However, this shows that tuning GRM before blending had a negligible impact on genomic predictions (McWhorter et al., 2022). Furthermore, blending (
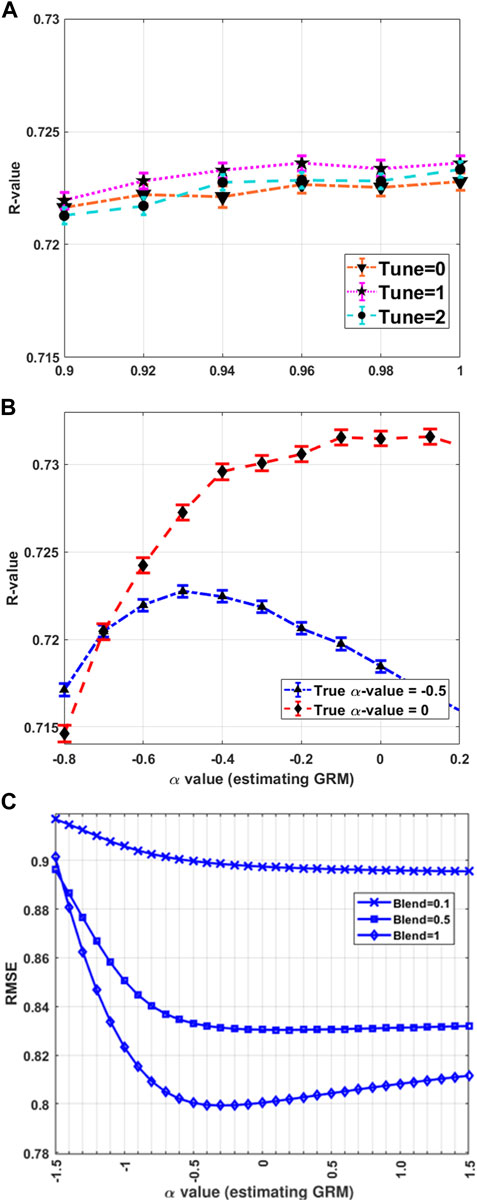
FIGURE 2. HBLUP accuracy and hyper-parameters. (A) The HBLUP accuracy (R-value) improves when using tune = 1 (Eq. 8) or tune = 2 (Eq. 9). However, blending (
Mimicking a real dataset in which multiple replicates are not possible, we used a single simulation data to assess the HBLUP accuracy, varying hyper-parameters (Figure 3). All possible configurations of tuning, blending, and
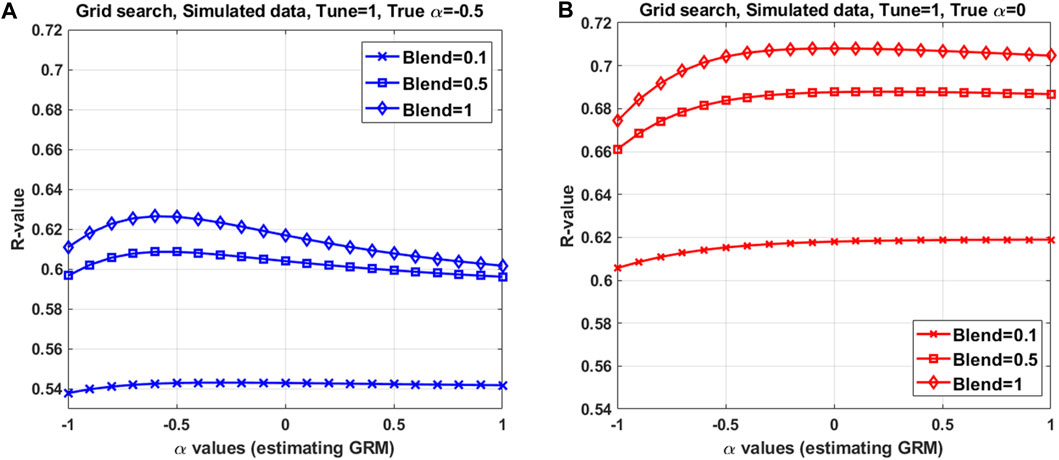
FIGURE 3. HBLUP accuracy averaged over 5-fold cross validation in a grid search with various configurations of the hyper-parameters, using a single simulation dataset. The best configuration found in the grid search consists of (A) and tune = 1, blend = 0.9, and α = −0.5 when using α = −0.5 in the simulation, and (B) tune = 1, blend = 1 and α = 0 (in estimating GRM) when using α = 0 in the simulation. The population parameters used in the simulation are h2 = 0.8, Ne = 100 for 100 historical generations, NSNPs = 9000, chromosome number = 30 and α = 0 or −0.5. Mimicking livestock population, a half-sib design (50 sires, 10 dams per sire and 2 offspring per dam) was applied to the last 5 generations. Full pedigree across the 5 generations were used in HBLUP. Among 2000 offspring in the last 2 generations, 5 subsets each with a random 400 individuals were used as target datasets in the 5-fold cross validation. To predict for each target dataset, the remaining 5150 (across the 5 generations) were used as the discovery dataset.
3.2 Cattle data
We used pedigree, genotype, and phenotype data of Korean native cattle (Hanwoo), which is a unique and important breed in the beef industry (Kim et al., 2017; Srivastava et al., 2021), to assess the HBLUP accuracy with various hyper-parameters including
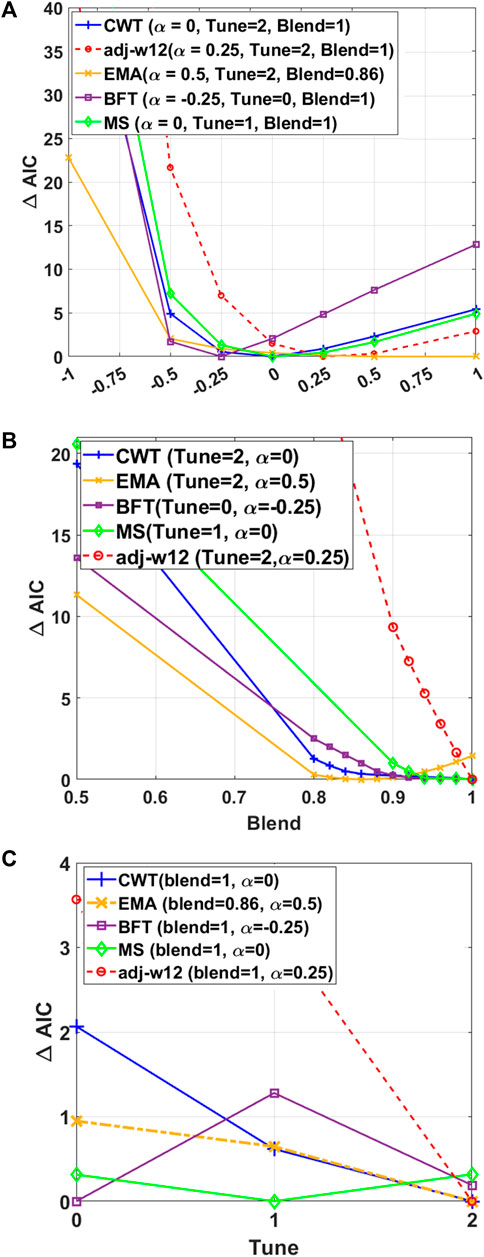
FIGURE 4. HREML estimation accuracy depends on
We also used a grid search to assess the performance of all hyper-parameters (Figure 5) in which HBLUP accuracies of all possible configurations of tuning, blending, and
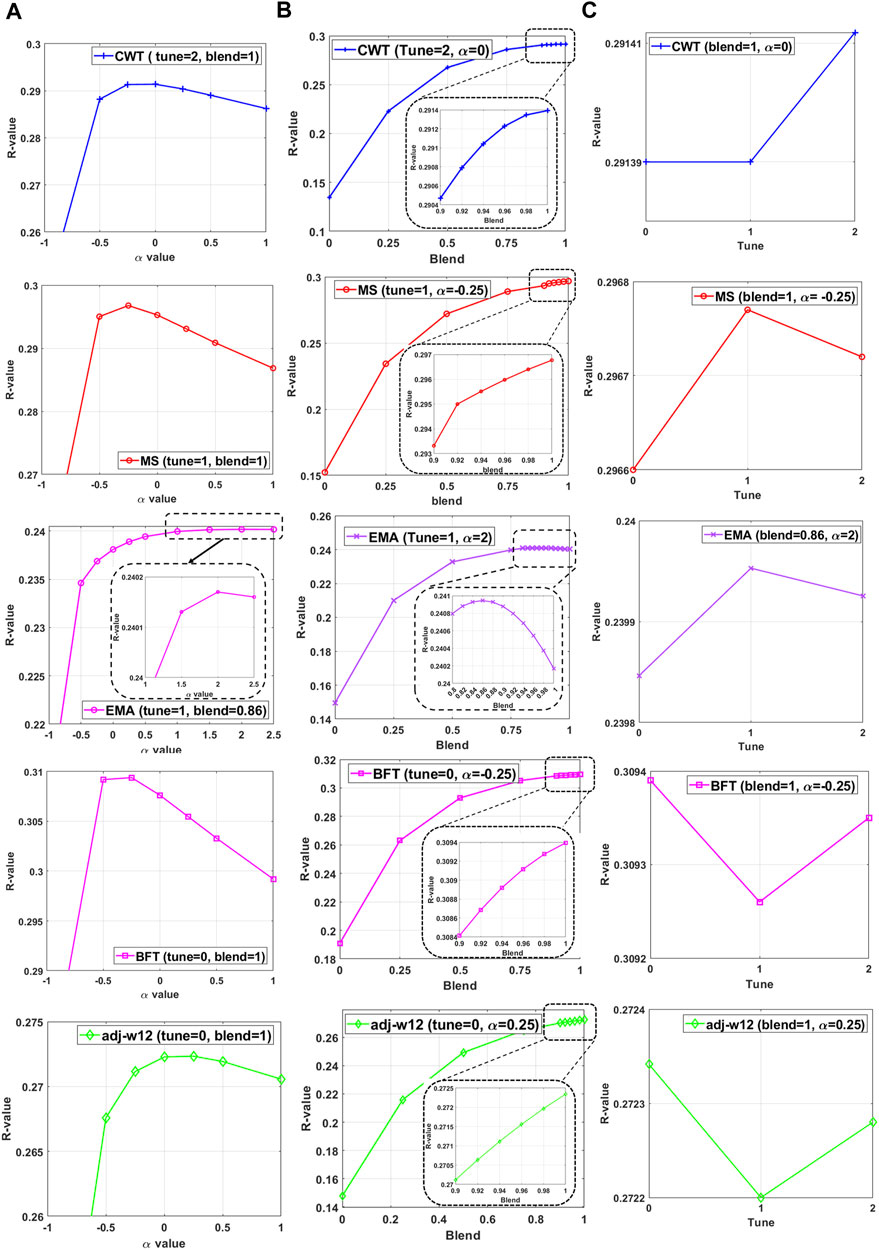
FIGURE 5. The performance of HBLUP when (A) varying
4 Discussion
HBLUP or ssGBLUP has been widely used in livestock breeding programs (De Los Campos et al., 2009; VanRaden et al., 2009). The HBLUP method (e.g., BLUPf90) requires hyper-parameters to integrate the information of genomic and pedigree relationship matrices, which should be optimised to increase the accuracy of genomic prediction (Legarra et al., 2009; Chen et al., 2011; Vitezica et al., 2011; Meyer et al., 2018). In this study, we evaluated the performance of HBLUP with various hyper-parameters such as blending, tuning, and scale factor, using simulated and real Hanwoo cattle datasets.
In our simulation scenario, we employed random mating and random selection instead of artificial selection based on phenotypes or estimated breeding values because the purpose of this simulation study is to demonstrate how the novel hyper-parameter, alpha, works in a simplified simulation setting. Nevertheless, we have applied our approach to real cattle data that have been subjected to artificial selection. By doing so, we believe that we have verified the performance of the hyper-parameter in a realistic setting. In both simulation and real data, allele frequencies can be altered significantly due to genetic drift and selection (Falconer and Mackay, 1996; Hartl and Clark, 1997; Lynch and Walsh, 1998).
The scale factor,
In both simulated and cattle data, blending (
The tuning process adjusts GRM, accounting for the allele frequencies in the base population, assuming that the founders in the base population are not genotyped but are linked through the pedigree. As expected, the widely used tuning method (tune = 1 (Chen et al., 2011) implemented in BLUPf90 option 2) could improve the prediction accuracy in the simulated data, indicating that the base allele frequencies are correctly accounted for. However, the improvement caused by tune = 1 or 2 was not remarkable in the Hanwoo cattle data. This is probably due to the fact that the pedigree information in the real data is not accurate enough to trace the founders, or the genotypes may capture substantial information about the base allele frequencies.
The grid search benefits include being able to provide reproducible results, being fast to implement, being simple to develop for parallel computing, and being efficient in exploring a low-dimensional hyper-parameter space. Moreover, for the large-scale hyper-parameters search space, there are a large number of other hyper-parameters optimisation methods, such as genetic/evolutionary algorithms, swarm intelligence methods, stochastic/random search techniques, and co-evolutionary algorithms (Dudzik et al., 2021). These methods are able to provide robust performance in exploring the multi-modal search space (Kuyu and Vatansever, 2021).
In conclusion, existing hyper-parameters such as blending and tuning in HBLUP are important in general, and their optimal values or options should be properly sought to achieve a reliable genetic evaluation. Depending on the data, optimal values can vary, and unnecessary or over-parametrised blending or tuning can produce adverse effects on the prediction accuracy. The scale factor, a novel hyper-parameter to be introduced in HBLUP, should be explicitly optimised to increase the prediction accuracy, given that the impact of the scale factor is competitive with other hyper-parameters, blending and tuning. We suggest including the scale factor,
Data availability statement
The data analyzed in this study is subject to the following licenses/restrictions: The SNP genotypic data and phenotypic data of Korean Hanwoo cattle used in this study are deposited and available at the digital repository of NIAS, South Korea (https://www.nias.go.kr/). Moreover, the simulated data generated and used in this study is available by https://github.com/a1708192/HBLUP_gridsearch.git. Requests to access these datasets should be directed to SoL, bGh5dW5nbUBrb3JlYS5rcg== (bGh5dW5nbUBnbWFpbC5jb20= https://www.nias.go.kr/.
Ethics statement
The current study was approved by the Animal Care and Use Committee of the National Institute of Animal Science (NIAS), Rural Development Administration (RDA), and Nonghyup Hanwoo Improvement Center, South Korea. SL (bGh5dW5nbUBrb3JlYS5rcg==).
Author contributions
Conceptualization: MN, SL, BT, JW, and SHL. methodology: MN and SHL. Data curation: MN, SL, and BT formal analysis: MN, SL, and MM investigation: MN and SHL. Software: MN, MM, and SHL. Validation: MN visualization: MN resources: SL and BT funding acquisition: SL and SHL. Writing–original draft preparation: MN and SHL. Writing–review and editing: MN, MM, BT, JW, and SHL. Project administration: SL and SHL. Supervision: SHL. All authors contributed to the article and approved the submitted version.
Funding
We acknowledge the support of the Cooperative Research Program for Agriculture Science and Technology Development (PJ01609901) from the Rural Development Administration, Republic of Korea, in sharing the Hanwoo cattle dataset and technical details. This research is also supported by the Australian Research Council (DP190100766).
Conflict of interest
The authors declare that the research was conducted in the absence of any commercial or financial relationships that could be construed as a potential conflict of interest.
Publisher’s note
All claims expressed in this article are solely those of the authors and do not necessarily represent those of their affiliated organizations, or those of the publisher, the editors and the reviewers. Any product that may be evaluated in this article, or claim that may be made by its manufacturer, is not guaranteed or endorsed by the publisher.
Supplementary material
The Supplementary Material for this article can be found online at: https://www.frontiersin.org/articles/10.3389/fgene.2023.1104906/full#supplementary-material
References
Abraham, G., Havulinna, A. S., Bhalala, O. G., Byars, S. G., De Livera, A. M., Yetukuri, L., et al. (2016). Genomic prediction of coronary heart disease. Eur. heart J. 37 (43), 3267–3278. doi:10.1093/eurheartj/ehw450
Akaike, H. (1974). A new look at the statistical model identification. IEEE Trans. Automatic Control 19 (6), 716–723. doi:10.1109/TAC.1974.1100705
Alkhoder, H., and Liu, Z. (2021). Application of a Single-Step SNP BLUP Model to Conformation Traits of German Holsteins. (INTERBULL BULLETIN: Leeuwarden, The Netherlands) 56, 30–40.
Baloche, G., Legarra, A., Sallé, G., Larroque, H., Astruc, J. M., Robert-Granié, C., et al. (2014). Assessment of accuracy of genomic prediction for French Lacaune dairy sheep. J. Dairy Sci. 97 (2), 1107–1116. doi:10.3168/jds.2013-7135
Bergstra, J., Bardenet, R., Bengio, Y., and Kégl, B. (2011). Algorithms for hyper-parameter optimization. Advances in Neural Information Processing Systems 24 (NIPS 2011) (ACM: Granada, Spain).
Bouwman, A. C., Hayes, B. J., and Calus, M. P. (2017). Estimated allele substitution effects underlying genomic evaluation models depend on the scaling of allele counts. Genet. Sel. Evol. 49 (1), 1–13. doi:10.1186/s12711-017-0355-9
Brown, D. J., Swan, A. A., Boerner, V., Li, L., Gurman, P. M., McMillan, A. J., et al. (2018). “Single-step genetic evaluations in the Australian sheep industry,” in Proceedings of the world congress on genetics applied to livestock production, (Armidale, Australia: University of New England).
Chen, C. Y., Misztal, I., Aguilar, I., Legarra, A., and Muir, W. M. (2011). Effect of different genomic relationship matrices on accuracy and scale. J. animal Sci. 89 (9), 2673–2679. doi:10.2527/jas.2010-3555
Christensen, O. F., and Lund, M. S. (2010). Genomic prediction when some animals are not genotyped. Genet. Sel. Evol. 42 (1), 1–8. doi:10.1186/1297-9686-42-2
Christensen, O. F., Madsen, P., Nielsen, B., Ostersen, T., and Su, G. (2012). Single-step methods for genomic evaluation in pigs. animal 6 (10), 1565–1571. doi:10.1017/S1751731112000742
Chung, K. Y., Lee, S. H., Cho, S. H., Kwon, E. G., and Lee, J. H. (2018). Current situation and future prospects for beef production in South Korea—a review. Asian-Australasian J. Animal Sci. 31 (7), 951. doi:10.5713/ajas.18.0187
De Los Campos, G., Naya, H., Gianola, D., Crossa, J., Legarra, A., Manfredi, E., et al. (2009). Predicting quantitative traits with regression models for dense molecular markers and pedigree. Genetics 182 (1), 375–385. doi:10.1534/genetics.109.101501
Dudzik, W., Nalepa, J., and Kawulok, M. (2021). Evolving data-adaptive support vector machines for binary classification. Knowledge-Based Syst. 227, 107221. doi:10.1016/j.knosys.2021.107221
Falconer, D. S., and Mackay, T. F. C. (1996). Introduction to quantitative genetics. Essex, England: Longman, 254–256.
Gao, H., Christensen, O. F., Madsen, P., Nielsen, U. S., Zhang, Y., Lund, M. S., et al. (2012). Comparison on genomic predictions using three GBLUP methods and two single-step blending methods in the Nordic Holstein population. Genet. Sel. Evol. 44 (1), 1–8. doi:10.1186/1297-9686-44-8
Gao, H., Koivula, M., Jensen, J., Strandén, I., Madsen, P., Pitkänen, T., et al. (2018). Genomic prediction using different single-step methods in the Finnish red dairy cattle population. J. dairy Sci. 101 (11), 10082–10088. doi:10.3168/jds.2018-14913
Gowane, G. R., Lee, S. H., Clark, S., Moghaddar, N., Al-Mamun, H. A., and van der Werf, J. H. (2019). Effect of selection and selective genotyping for creation of reference on bias and accuracy of genomic prediction. J. Animal Breed. Genet. 136 (5), 390–407. doi:10.1111/jbg.12420
Hartl, D. L., and Clark, A. G. (1997). Principles of population genetics. Sunderland, MA, USA: Sinauer Associates.
Hayes, B. J., Bowman, P. J., Chamberlain, A. J., and Goddard, M. E. (2009). Invited review: Genomic selection in dairy cattle: Progress and challenges. J. dairy Sci. 92 (2), 433–443. doi:10.3168/jds.2008-1646
Henderson, C. R. (1975). Best linear unbiased estimation and prediction under a selection model. Biometrics 31, 423–447. doi:10.2307/2529430
Henderson, C. R. (1953). Estimation of variance and covariance components. Biometrics 9 (2), 226–252. doi:10.2307/3001853
Hsu, W. L., Garrick, D. J., and Fernando, R. L. (2017). The accuracy and bias of single-step genomic prediction for populations under selection. G3 Genes, Genomes, Genet. 7 (8), 2685–2694.
Inouye, M., Abraham, G., Nelson, C. P., Wood, A. M., Sweeting, M. J., Dudbridge, F., et al. (2018). Genomic risk prediction of coronary artery disease in 480,000 adults: Implications for primary prevention. J. Am. Coll. Cardiol. 72 (16), 1883–1893. doi:10.1016/j.jacc.2018.07.079
Johnston, D. J., Ferdosi, M. H., Connors, N. K., Boerner, V., Cook, J., Girard, C. J., et al. (2018). “Implementation of single-step genomic BREEDPLAN evaluations in Australian beef cattle,” in Proceedings of the world congress on genetics applied to livestock production (Armidale, Australia: University of New England).
Kang, H. M., Zaitlen, N. A., Wade, C. M., Kirby, A., Heckerman, D., Daly, M. J., et al. (2008). Efficient control of population structure in model organism association mapping. Genetics 178 (3), 1709–1723. doi:10.1534/genetics.107.080101
Khera, A. V., Chaffin, M., Aragam, K. G., Haas, M. E., Roselli, C., Choi, S. H., et al. (2018). Genome-wide polygenic scores for common diseases identify individuals with risk equivalent to monogenic mutations. Nat. Genet. 50 (9), 1219–1224. doi:10.1038/s41588-018-0183-z
Kim, S., Alam, M., and Park, M. N. (2017). Breeding initiatives for Hanwoo cattle to thrive as a beef industry–A review study. J. Anim. Breed. Genom 1, 103. doi:10.12972/jabng.20170011
Kuyu, Y. Ç., and Vatansever, F. (2021). Advanced metaheuristic algorithms on solving multimodal functions: Experimental analyses and performance evaluations. Archives Comput. Methods Eng., 28 1–3. doi:10.1007/s11831-021-09555-0
LaValle, S. M., Branicky, M. S., and Lindemann, S. R. (2004). On the relationship between classical grid search and probabilistic roadmaps. Int. J. Robotics Res. 23 (7-8), 673–692. doi:10.1177/0278364904045481
Lee, S. H., Clark, S., and Van Der Werf, J. H. (2017). Estimation of genomic prediction accuracy from reference populations with varying degrees of relationship. PloS one 12 (12), e0189775. doi:10.1371/journal.pone.0189775
Lee, S. H., and Van Der Werf, J. H. (2006). An efficient variance component approach implementing an average information REML suitable for combined LD and linkage mapping with a general complex pedigree. Genet. Sel. Evol. 38 (1), 1–19. doi:10.1186/1297-9686-38-1-25
Lee, S. H., and Van der Werf, J. H. (2016). MTG2: An efficient algorithm for multivariate linear mixed model analysis based on genomic information. Bioinformatics 32 (9), 1420–1422. doi:10.1093/bioinformatics/btw012
Legarra, A., Christensen, O. F., Aguilar, I., and Misztal, I. (2014). Single Step, a general approach for genomic selection. Livest. Sci. 166, 54–65. doi:10.1016/j.livsci.2014.04.029
Legarra, A., Aguilar, I., and Misztal, I. (2009). A relationship matrix including full pedigree and genomic information. J. dairy Sci. 92 (9), 4656–4663. doi:10.3168/jds.2009-2061
Lynch, M., and Walsh, B. (1998). Genetics and analysis of quantitative traits. Sunderland, MA: Sinauer Associates.
Mancisidor, B., Cruz, A., Gutiérrez, G., Burgos, A., Morón, J. A., Wurzinger, M., et al. (2021). ssGBLUP method improves the accuracy of breeding value prediction in huacaya alpaca. Animals 11 (11), 3052. doi:10.3390/ani11113052
Mäntysaari, E. A., Koivula, M., and Strandén, I. (2020). Symposium review: Single-step genomic evaluations in dairy cattle. J. dairy Sci. 103 (6), 5314–5326. doi:10.3168/jds.2019-17754
McMillan, A. J., and Swan, A. A. (2017). Weighting of genomic and pedigree relationships in single step evaluation of carcass traits in Australian sheep. Proceedings of the 22nd Conference of the Association for the Advancement of Animal Breeding and Genetics (AAABG), Townsville, Queensland, Australia, July 2017 22, 557–560.
McWhorter, T. M., Bermann, M., Garcia, A. L., Legarra, A., Aguilar, I., Misztal, I., et al. (2022). Implication of the order of blending and tuning when computing the genomic relationship matrix in single-step GBLUP. J. Animal Breed. Genet. 140, doi:10.1111/jbg.12734
Meyer, K., Tier, B., and Swan, A. (2018). Estimates of genetic trend for single-step genomic evaluations. Genet. Sel. Evol. 50 (1), 1–11.
Misztal, I., Legarra, A., and Aguilar, I. (2009). Computing procedures for genetic evaluation including phenotypic, full pedigree, and genomic information. J. dairy Sci. 92 (9), 4648–4655.
Misztal, I., Tsuruta, S., Lourenco, D. A. L., Masuda, Y., Aguilar, I., Legarra, A., et al. (2018). Manual for blupf90 family of programs. Athens, Georgia: University of Georgia.
Momin, M. M., Shin, J., Lee, S., Truong, B., Benyamin, B., and Lee, S. H. (2023). A method for an unbiased estimate of cross-ancestry genetic correlation using individual-level data. Nat. Commun. 14, 722. doi:10.1038/s41467-023-36281-x
Oliveira, H. R., Lourenco, D. A. L., Masuda, Y., Misztal, I., Tsuruta, S., Jamrozik, J., et al. (2019). Application of single-step genomic evaluation using multiple-trait random regression test-day models in dairy cattle. J. dairy Sci. 102 (3), 2365–2377. doi:10.3168/jds.2018-15466
Sargolzaei, M., and Schenkel, F. S. (2009). QMSim: A large-scale genome simulator for livestock. Bioinformatics 25 (5), 680–681. doi:10.1093/bioinformatics/btp045
Schoech, A. P., Jordan, D. M., Loh, P. R., Gazal, S., O’Connor, L. J., Balick, D. J., et al. (2019). Quantification of frequency-dependent genetic architectures in 25 UK Biobank traits reveals action of negative selection. Nat. Commun. 10 (1), 1–10. doi:10.1038/s41467-019-08424-6
Speed, D., Cai, N., Johnson, M. R., Nejentsev, S., and Balding, D. J. (2017). Reevaluation of SNP heritability in complex human traits. Nat. Genet. 49 (7), 986–992. doi:10.1038/ng.3865
Speed, D., Hemani, G., Johnson, M. R., and Balding, D. J. (2012). Improved heritability estimation from genome-wide SNPs. Am. J. Hum. Genet. 91 (6), 1011–1021. doi:10.1016/j.ajhg.2012.10.010
Speed, D., Holmes, J., and Balding, D. J. (2020). Evaluating and improving heritability models using summary statistics. Nat. Genet. 52 (4), 458–462. doi:10.1038/s41588-020-0600-y
Srivastava, S., Lopez, B. I., Kumar, H., Jang, M., Chai, H. H., Park, W., et al. (2021). Prediction of Hanwoo cattle phenotypes from genotypes using machine learning methods. Animals 11 (7), 2066. doi:10.3390/ani11072066
Teissier, M., Larroque, H., and Robert-Granié, C. (2018). Weighted single-step genomic BLUP improves accuracy of genomic breeding values for protein content in French dairy goats: A quantitative trait influenced by a major gene. Genet. Sel. Evol. 50 (1), 1–12. doi:10.1186/s12711-018-0400-3
VanRaden, P. M. (2008). Efficient methods to compute genomic predictions. J. dairy Sci. 91 (11), 4414–4423. doi:10.3168/jds.2007-0980
VanRaden, P. M., Van Tassell, C. P., Wiggans, G. R., Sonstegard, T. S., Schnabel, R. D., Taylor, J. F., et al. (2009). Invited review: Reliability of genomic predictions for North American Holstein bulls. J. dairy Sci. 92 (1), 16–24. doi:10.3168/jds.2008-1514
Vitezica, Z. G., Aguilar, I., Misztal, I., and Legarra, A. (2011). Bias in genomic predictions for populations under selection. Genet. Res. 93 (5), 357–366. doi:10.1017/S001667231100022X
Yan, X., Zhang, T., Liu, L., Yu, Y., Yang, G., Han, Y., et al. (2022). Accuracy of genomic selection for important economic traits of cashmere and meat goats assessed by simulation study. Front. Veterinary Sci. 9. doi:10.3389/fvets.2022.770539
Yang, J., Benyamin, B., McEvoy, B. P., Gordon, S., Henders, A. K., Nyholt, D. R., et al. (2010). Common SNPs explain a large proportion of the heritability for human height. Nat. Genet. 42 (7), 565–569. doi:10.1038/ng.608
Keywords: genomic prediction, single-step genetic evaluation, hyper-parameters, scale factor, harmonised matrix
Citation: Neshat M, Lee S, Momin MM, Truong B, van der Werf JHJ and Lee SH (2023) An effective hyper-parameter can increase the prediction accuracy in a single-step genetic evaluation. Front. Genet. 14:1104906. doi: 10.3389/fgene.2023.1104906
Received: 22 November 2022; Accepted: 23 May 2023;
Published: 08 June 2023.
Edited by:
Kui Zhang, Michigan Technological University, United StatesReviewed by:
Jakub Nalepa, Silesian University of Technology, PolandMarco Bink, Hendrix Genetics Research, Netherlands
Copyright © 2023 Neshat, Lee, Momin, Truong, van der Werf and Lee. This is an open-access article distributed under the terms of the Creative Commons Attribution License (CC BY). The use, distribution or reproduction in other forums is permitted, provided the original author(s) and the copyright owner(s) are credited and that the original publication in this journal is cited, in accordance with accepted academic practice. No use, distribution or reproduction is permitted which does not comply with these terms.
*Correspondence: Mehdi Neshat, TWVoZGkuTmVzaGF0QHVuaXNhLmVkdS5hdQ==; S. Hong Lee, SG9uZy5MZWVAdW5pc2EuZWR1LmF1