- 1Beijing Key Laboratory for Genetics of Birth Defects, Beijing Pediatric Research Institute, MOE Key Laboratory of Major Diseases in Children, Beijing Children’s Hospital, Capital Medical University, National Center for Children’s Health, Beijing, China
- 2Department of Psychiatry, Beijing Children’s Hospital, Capital Medical University, National Center for Children’s Health, Beijing, China
- 3National Center for Children’s Health, Beijing, China
Purpose: To establish an effective genomic diagnosis pipeline for children with autism spectrum disorder (ASD) for its genetic etiology and intervention.
Methods: A cohort of 354 autism spectrum disorder patients were obtained from Beijing Children’s Hospital, Capital Medical University. Peripheral blood samples of the patients were collected for whole genome sequencing (WGS) and RNA sequencing (RNAseq). Sequencing data analyses were performed for mining the single nucleotide variation (SNV), copy number variation (CNV) and structural variation (SV). Sanger sequencing and quantitative PCR were used to verify the positive results.
Results: Among 354 patients, 9 cases with pathogenic/likely pathogenic copy number variation and 10 cases with pathogenic/likely pathogenic single nucleotide variations were detected, with a total positive rate of 5.3%. Among these 9 copy number variation cases, 5 were de novo and 4 were inherited. Among the 10 de novo single nucleotide variations, 7 were previously unreported. The pathological de novo mutations account for 4.2% in our cohort.
Conclusion: Rare mutations of copy number variations and single nucleotide variations account for a relatively small proportion of autism spectrum disorder children, which can be easily detected by a genomic testing pipeline of combined whole genome sequencing and RNA sequencing. This is important for early etiological diagnosis and precise management of autism spectrum disorder with rare mutations.
Introduction
Autism spectrum disorder (ASD) is a group of neurodevelopmental disorder with onset at early childhood. According to the Diagnostic and Statistical Manual of Mental Disorders (Fifth Edition, DSM-V), autism, Asperger’s syndrome, childhood disintegrating disorder and unclassified pervasive developmental disorder are collectively referred to ASD (American Psychiatric Association, 2013). The two core symptoms of ASD are 1) impaired social communication and interaction, 2) repetitive and stereotyped behaviors, interests, and activities (Lobar, 2016). The incidence of ASD has been on the rise in recent years. According to the Centers for Disease Control and Prevention (CDC) reports, 1 in 54 children are diagnosed with ASD (Maenner et al., 2020), with a significantly higher proportion of male patients than that of female patients, and the ratio of male to female patients is about 4 : 1 (Rutherford et al., 2016; Loomes et al., 2017; Maenner et al., 2020). Except for the core symptoms, ASD is also accompanied by other comorbidities including but not limited to intellectual disability, epilepsy, facial deformities, neurological imaging abnormalities, movement disorders, attention deficit hyperactivity disorder (ADHD), aggressive behavior, cardiovascular abnormalities, gastrointestinal disorders, sleep disorders, convulsions, oppositional defiant disorder, anxieties, obsessions and compulsions and depression (Simonoff et al., 2008; Popow et al., 2021). Due to the wide spectrum of symptoms, missing diagnosis or misdiagnosis often occurs. Genetic diagnosis is now a routine method for precision diagnosis and intervention of ASD.
The clinical manifestations of ASD are diverse and complex, and its etiology is still largely unknown. ASD shows great heterogeneity in clinical symptoms and genetic alterations (Sandin et al., 2017). More attention has been paid to the genetic factors associated with ASD. Known genetic abnormalities are copy number variants (CNVs), de novo single nucleotide variants (SNVs), common genetic variants, mosaicism, non-coding and regulatory pathogenic variations, and inherited recessive variants (Zhang et al., 2021). More than 1,000 genes related to ASD have been described in the SFARI database (https://gene.sfari.org). The reported single gene mutation related to ASD accounts for about 5% (Gaugler et al., 2014), and CNV accounts for 8%–20% of ASD (Sebat et al., 2007). However, to date, at least 70% of the affected individuals have no known genetic etiology (Dias and Walsh, 2020).
The clinical implementation of trio-whole exome/genome sequencing (WES/WGS) has been a significant contribution to the discovery of de novo SNVs to autism risk (Neale et al., 2012; Sanders et al., 2012; Iossifov et al., 2014). As these variants usually link to a single gene, it is particularly important in emphasizing the underlying neurobiology of de novo SNVs associated with autism. CNVs refer to large deletions or duplications often involving in several genes. The association of phenotype with gene dosage exists, but the confirmation of relationship is often difficult. In 2007, comparative genomic hybridization was used to establish a significant association between de novo submicroscopic structural variation (SV) and autism (Sebat et al., 2007). From then on, more CNVs related to autism have been identified. In the genomics era, more genetic architectural changes including SNVs, CNVs and SVs are associated with ASD in different disease cohorts of different populations. Nevertheless, functional experiments are crucial for these validations to better understand the pathogenicity.
Enhanced bioinformatics analyses integrate evolutionary constraints to identify risk genes with a false discovery rate less than or equal to 0.1. In addition to utilizing probability of loss of function (pLI), missense badness, PolyPhen-2 constraint score, researchers are able to identify variants affecting gene functions by predicted impact (Lek et al., 2016). These analyses not only confirm enrichment of de novo loss-of-function mutations which affect highly constrained genes, but also identify pathogenic missense mutations. Currently, there are a variety of molecular testing platforms in diagnosing ASD. Trio-WES and CNV sequencing (CNVseq) or chromosomal microarray analysis (CMA) are commonly used first-tier techniques in molecular diagnosis of ASD. In the next-generation sequencing era, with the cost of sequencing declining, WGS is more widely applied to detect SNVs, CNVs and SVs simultaneously to uncover both coding and non-coding variants. RNA sequencing (RNAseq) can increase the diagnostic rate by assessing the gene expression changes. Thus, we here integrate both WGS and RNAseq for the genomic analysis of our ASD cohort to evaluate its efficacy and clinical application in a single-center level, in order to characterize the genetic etiology of the patients for both known ASD genes or new candidate ASD genes by focusing on de novo SNVs and CNVs.
Materials and methods
General patient information
The patients who met the diagnostic criteria of ASD by DSM-V were all from the Department of Psychiatry, Beijing Children’s Hospital. From July 2019 to May 2021, a total of 354 cases from 345 families were enrolled, including 9 families with two affected siblings. The ASD patients aged at 1–12 years, including 279 males and 75 females, with a male-to-female ratio of 3.72 : 1, very close to the ratio of male-to-female of about 4 : 1 (Maenner et al., 2020). The clinical data, imaging reports (if any) and ASD assessment scales of the patients were collected, including the Autism Behavior Checklist (ABC), Clancy Autism Behavior Scale (CABS), Childhood Autism Rating Scale (CARS) and DSM-V Diagnostic Scale (Supplementary Material). The head circumference and eye distance of the children were measured, and the front and side images were captured. The enrollment and diagnosis process are summarized in Figure 1. 5 mL of venous blood from the patient and 2 mL of venous blood from each parent were collected, and genomic DNA and total RNA were extracted. All parents have signed their own written informed consents as well as in representative of their children’s guardians. This study has been approved by the Ethics Committee of Beijing Children’s Hospital.
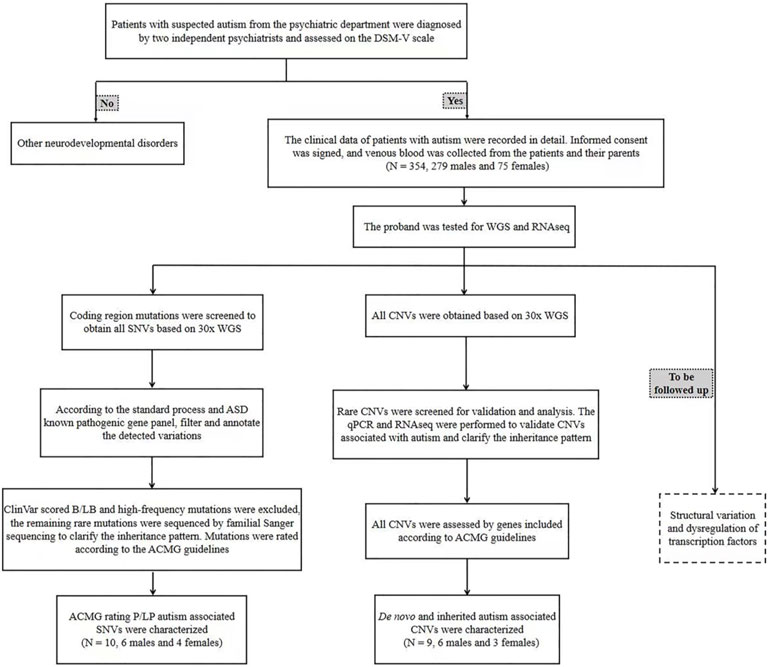
FIGURE 1. The clinical enrollment and diagnosis flowchart of ASD patients. qPCR: quantitative PCR; WGS: whole genome sequencing; SNV: single nucleotide variation; CNV: copy number variation; RNAseq: RNA sequencing.
Whole genome sequencing (WGS)
DNA of all collected samples was extracted and purified using DNA Blood Mini Kit (Qiagen, Hilden, Germany). DNA concentration was measured by Qubit DNA Assay Kit in Qubit 2.0 Flurometer (Life Technologies, CA, USA).
Library preparation
A total amount of 1 µg DNA sample from each proband was used as the input material for DNA library preparation. Sequencing library was generated using CleanNGS DNA Kit (CleanNA, Waddinxveen, Netherlands) following the manufacturer’s recommendations and index codes were added to each sample. Briefly, genomic DNA sample was enzymatically digested to fragments of about 350 bp. Then DNA fragments were end-polished, A-tailed, and ligated with the full-length adapter for sequencing, followed by further PCR amplification. After PCR products were purified by AMPure XP System (Beckman Coulter, CA, USA), libraries were analyzed for size distribution by Agilent 2100 Bioanalyzer (Agilent Technologies, CA, USA) and quantified by real-time PCR.
Clustering and sequencing
The clustering of the index-coded samples was performed on a cBot Cluster Generation System using Novaseq5000/6000 S4 Reagent Kit (Illumina, San Diego, CA, USA) according to the manufacturer’s instructions. After cluster generation, the DNA libraries were sequenced on Illumina NovaSeq 6000 platform and 150 bp paired-end reads were generated.
Quality control
Raw data (raw reads) of fastq format were firstly processed through primary quality control. In this step, clean data (clean reads) were obtained by removing read pairs that contain more than three N or the proportion of base with quality value below 5 is more than 20%, in any end, or adapter sequence was founded. All the downstream analyses were based on the clean data with high quality.
Reads mapping to reference sequences
Clean reads were compared with reference human genome (UCSC hg19) by using BWA software (http://bio-bwa.sourceforge.net), and the results were converted into bam format and sorted by Samtools software (https://github.com/samtools/samtools/releases). Finally, basic information statistics and map comparison statistics were conducted. The average depth of WGS is about 30×.
RNA sequencing (RNAseq)
Total RNA of all collected samples was extracted and purified from the fresh venous whole blood using PAXgene Blood RNA Kit (Qiagen). Total RNA concentration was measured by Qubit RNA Assay Kit in Qubit 2.0 Flurometer (Life Technologies).
RNA quality check
The purity of the sample was determined by NanoPhotometer (Implen, CA, USA), the concentration and integrity of RNA samples were detected by Agilent 2100 RNA Nano 6000 Assay Kit.
Library preparation
A total amount of 1–3 μg RNA per sample from each proband was used as the input material for RNA sample preparation. Sequencing libraries were generated using VAHTS Universal V6 RNA-seq Library Prep Kit (Illumina, NR604-01/02) following the manufacturer’s recommendations and index codes were added to attribute sequences to each sample. Briefly, mRNA was purified from total RNA using poly-T oligo-attached magnetic beads. Then we added fragmentation buffer to break the mRNA into short fragments. First strand cDNA was synthesized using random hexamer primer and RNase H. Second strand cDNA synthesis was subsequently performed using buffer, dNTPs, DNA polymerase I and RNase H. The double stranded cDNA was purified by AMPure XP beads (Beckman Coulter). The purified double stranded cDNA was repaired at the end, added a tail and connected to the sequencing connector, then the fragment size was selected, and the final cDNA library was constructed by PCR enrichment.
Library check
RNA concentration of library was measured using Qubit RNA Assay Kit in Qubit 3.0 (Life Technologies) for quantification and then diluted to 1 ng/μL. Insert size was assessed using the Agilent Bioanalyzer 2100 system. After the insert size met the requirement, the CFX 96 fluorescence quantitative PCR instrument (Bio-Rad, CA, USA) was used to quantify the library effective concentration (library effective concentration >10 nm) using Bio-Rad iQ SYBR GRN Kit.
Library clustering and sequencing
1) HiSeq x ten platform (Illumina). The clustering of the index-coded samples was performed on a cBot cluster generation system using HiSeq PE Cluster Kit v4-cBot-HS according to the manufacturer’s instructions. After cluster generation, the libraries were sequenced on an Illumina platform and 150 bp paired-end reads were generated. 2) Novaseq 6000 S4 platform (Illumina). The cluster generation and sequencing were performed on Novaseq 6000 S4 platform, using NovaSeq 6000 S4 Reagent Kit V1.5.
Sanger sequencing
Amplification primers were designed for the gene variants, and PCR amplification and sequencing verification were performed on the gene products in the family of the indicated patient. ABI 3730XL sequencer (Applied Biosystem, CA, USA) was used for Sanger sequencing.
Quantitative PCR (qPCR)
The CNVs were validated by quantitative PCR (qPCR). qPCR validation was performed using the Roche LightCycler 480 Ⅱ System (Roche, Basel, Switzerland). One pair of primers was selected from the middle of each CNV. The samples from the family of the indicated patient were analyzed in triplicate in a 10 μL reaction mixture (200 nM each primer, LightCycler 480 SYBR Green Master Mix (2X) and 5 or 10 ng of genomic DNA). The values were evaluated using LightCycler 480 Software (Applied Biosystems). Data analysis was performed using the qBase method (Hellemans et al., 2007). GAPDH was used as the reference gene for the minimal coefficient of variation. Data were normalized by setting a normal control to a value of 1.
Bioinformatic analyses of sequencing data and variants
Verita TreKKer (v1.9.3, Berry Genomics, Beijing, China) was used to identify SNP/InDels. CNVnator(v1.2.2) (Abyzov et al., 2011) and BIC-Seq (v0.7.2) (Xi et al., 2011) were used for CNV detection, while Manta (Chen et al., 2016) was used for SV discovery. EnliVen (v1.9.3, Berry Genomics) was performed to annotate SNP/InDels/CNV/SV. EnliVen and ANNOVAR (Wang et al., 2010) were executed for VCF (variant call format) files. dbSNP (http://www.ncbi.nlm.nih.gov/snp), 1,000 Genomics Project (http://browser.1000genomes.org) and other related existing databases were applied to annotate the variants. By focusing on exonic variants, gene transcript annotation databases, such as Consensus CDS protein set (http://www.ncbi.nlm.nih.gov/CCDS), RefSeq Gene (http://www.ncbi.nlm.nih.gov/RefSeq), Ensembl (https://www.ensembl.org) and UCSC (http://genome.ucsc.edu), were used to determine amino acid changes. Variants were analyzed for pathogenicity according to the American College of Medical Genetics and Genomics (ACMG) grading criteria (Richards et al., 2015).
The clean reads were aligned on genome hg19 along with annotated genes in the Ensembl website using STAR v2.7 aligner (Dobin et al., 2013). Gene expression level was quantified using DROP pipeline with the default parameters (Yépez et al., 2021). Differential gene expression was used to validate the effect of candidate SNVs and CNVs if the gene is stably expressed in blood. Candidate CNV regions cover up to hundreds of in-CNV genes. The average expression level of in-CNV genes were compared to that of equal number of randomly selected out-CNV genes outside the CNV region on the same chromosome. The difference between in-CNV and out-CNV genes was quantified by Z-score and log2-fold change. The duplication/loss CNVs were expected to show greater/smaller Z-score and log2-fold change, respectively.
Results
Intellectual disability and language delay are the two most common features of ASD
Among 354 patients enrolled, 19 were molecular positive, including 12 males and 7 females, aged from 2 to 6 years. The diagnosis pipeline is summarized in Figure 2. The male-to-female ratio is less than about 4:1 as these positive cases were mostly having de novo mutations. The specific clinical features of 19 patients with positive diagnosis are shown in Table 1. Except for the ASD0330 patient who lost follow-up, 17 of the remaining 18 patients had clinical symptoms of intellectual disability and language delay which are less common in those molecularly unknown ASD patients, suggesting that the co-occurrence of these two symptoms could be suggestive of inborn errors in genetic alterations in ASD patients.
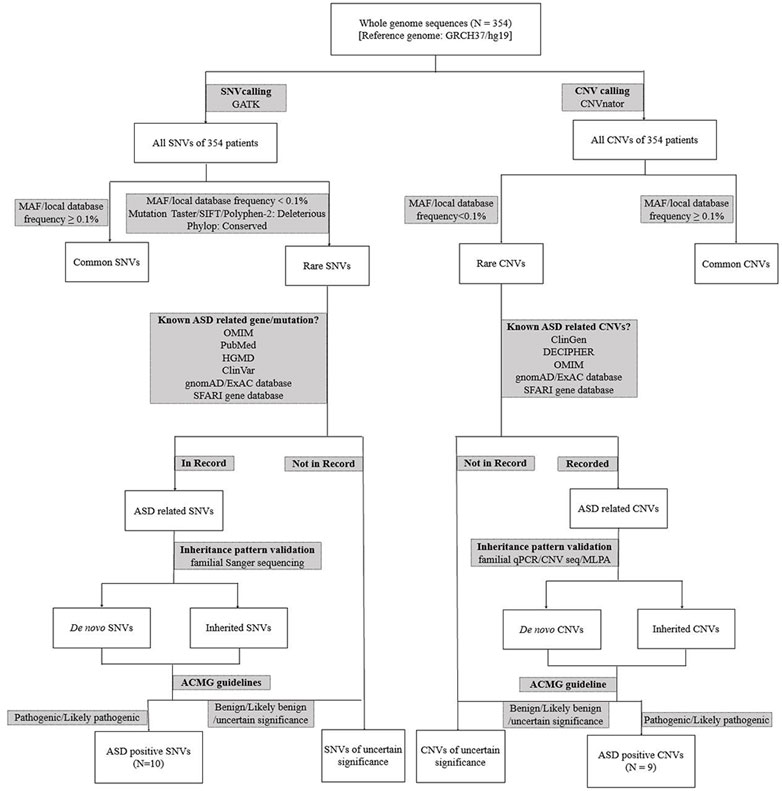
FIGURE 2. The detailed genomics diagnosis pipeline of ASD patients. qPCR: quantitative PCR; WGS: whole genome sequencing; SNV: single nucleotide variation; CNV: copy number variation; CNV seq: CNV sequencing.
CNVs detected in this ASD cohort
We applied low-depth WGS CNVseq-algorithm to analyze genomic alterations in 354 ASD patients. The distribution and burden analysis of CNV calls were calculated and summarized in Supplementary Figure S1. Among these CNVs, we found 9 cases were positive for pathological CNVs (Table 2). The resolution of CNVs reached 100 kb (Lord et al., 2018). To verify the CNV results, qPCR was performed on the positive cases, which are all in agreement (Supplementary Figure S2). Among these CNVs, 6 were duplicated CNVs, other 3 were deletional CNVs. Some of these deletions/duplications are known syndromes. Among these 9 CNV cases, 5 were de novo and 4 were inherited.
Duplications
ASD0018 carried a de novo duplication of the 22q11.2 proximal (A-D) region, which is associated with a highly variable clinical phenotype, ranging from apparently normal to a broad range of clinical features, including non-specific phenotypes (intellectual disability, learning disability, developmental delays, autism, psychiatric disorder growth delays, hypotonia) as well as phenotypes that overlap clinical findings of DiGeorge syndrome (DGS; OMIM #188400) or velocardiofacial syndrome (VCFS; OMIM #192,430) (ClinGen, 2018). 22q11.2 duplications are frequently inherited and incomplete penetrance has been demonstrated. The 22q11.2 duplication is often found in an apparently normal parent of a proband (Firth, 1993).
ASD0027 carried a de novo duplication of the 7q11.23 recurrent region (Williams-Beuren syndrome, OMIM #609757, including the ELN gene). Clinical findings in this syndrome may include speech delay, autistic features, motor delay, seizures, hypotonia, brain anomalies, joint laxity, and craniofacial abnormalities. Williams-Beuren region duplication syndrome is considered to be highly penetrant with patients showing variable expressivity. Both inherited and de novo cases of 7q11.23 duplications have been reported in the literature. The population frequency of the chromosome 7q11.23 duplication syndrome was estimated to be 1 in 13,000 to 20,000 (Van der Aa et al., 2009; Levy et al., 2011). This patient presented wide dental space, which has not been reported.
ASD0144 carried a duplication of the 16p13.11 recurrent region (BP2-BP3) (including the MYH11 gene) inherited from his phenotypically normal father. Duplication of the 16p13.11 region has been associated with a variable clinical presentation including developmental delay, intellectual disability/learning difficulties, behavioral abnormalities (including ASD and ADHD), hypotonia, congenital heart disease (such as tetralogy of Fallot), and variable dysmorphic features. The majority of patients with this duplication are inherited, mostly from an apparently unaffected parent or a parent with abnormal phenotypes similar to the child’s phenotype but not that much severe (Khattabi et al., 2020). Penetrance for any clinical phenotype associated with this duplication was estimated to be 7% (Kendall et al., 2019).
ASD0203 and ASD0330 both carried a duplication of 15q11q13 recurrent region (PWS/AS, BP2-BP3, Class 2), which is associated with chromosome 15q11-q13 duplication syndrome (OMIM #608636). Common clinical symptoms of this syndrome are autism, intellectual disability, ataxia, epilepsy, developmental delay and mental and behavioral problems (Bundey et al., 1994; Burnside et al., 2011). Most of the reported cases were familial inherited cases, and few were de novo mutations (Christian et al., 2008). The syndrome showed incomplete penetrance, and the clinical manifestations of different patients were highly heterogeneous. ASD0203 inherited the duplication from her phenotypic normal mother, while ASD0330 carried a de novo duplication.
ASD0343 carried a duplication of the 1q21.1 recurrent region (BP3-BP4, distal, including the GJA5 gene) inherited from his mother, who had congenital heart disease. Duplication of the 1q21.1 region has been associated with chromosome 1q21.1 duplication syndrome (OMIM #612475). The clinical phenotypes of the syndrome include varying degrees of intellectual impairment, macrocephaly, hypotonia, congenital heart disease (such as tetralogy of Fallot), and malformation features. Mental and behavioral disorders include ASD, ADHD and others. Most of the reported cases were inherited from unaffected parents with normal or abnormal phenotypes similar to the child but not severe, and the clinical phenotypes of patients are highly variable (Brunetti-Pierri et al., 2008; Bernier et al., 2016).
Deletions
ASD0060 carried a deletion at 2p16.3 involving exons of a haploinsufficiency gene NRXN1 inherited from his phenotypic normal father, which is associated with susceptibility to autism, schizophrenia (SCZD17), developmental delay, intellectual disability, and dysmorphic features. The phenotypes are highly variable and show incomplete penetrance (Schaaf et al., 2012; Dabell et al., 2013). Sleeping disorder of ASD0060 has not been reported in previous studies.
ASD0214 carried a de novo deletion covering a haploinsufficiency gene TBL1XR1, Heterozygous mutation or deletion of this gene is associated with autosomal dominant inherited intellectual disability type 41. The common clinical symptoms of patients include autism, intellectual disability, developmental delay, spasticity and facial deformations. The clinical manifestations of different patients are highly heterogeneous (O'Roak et al., 2012a; O'Roak et al., 2012b; Saitsu et al., 2014).
ASD0222 carried a de novo deletion of the 3q29 recurrent region (including the DLG1 gene). Deletion of this region is associated with chromosome 3q29 deletion syndrome (OMIM #609425). Clinical findings in this syndrome are mild to moderate developmental delay, intellectual disability, ASD, speech delay, walking delay, microcephaly and mild dysmorphic features. Most 3q29 deletions are de novo mutations, and a small number of cases are inherited from parents (Ballif et al., 2008; Quintero-Rivera et al., 2010; Città et al., 2013).
SNVs detected in this ASD cohort
We used the 30× WGS data for analyzing SNVs. Among 354 children with ASD, 10 were found with de novo deleterious SNVs (non-sense splicing or frameshift mutation, Table 3). The mutation sites and parental origins were verified by Sanger sequencing (Supplementary Figure S3). Among these mutational alleles, 7 were previously unreported alleles (PUAs).
ASD0063, ASD0221 and ASD0326 all carried de novo mutations of the SCN2A gene, which encodes the α-subunit family of voltage-gated sodium channel that is responsible for the action potentials in neurons and muscles. Mutations in SCN2A have been linked to epilepsy and ASD (Sanders et al., 2012; Tavassoli et al., 2014). The c. 4550_4551del of the SCN2A gene in ASD0063 was a PUA, while the c.605 + 1G>A mutation in ASD0221 and the c.1570C>T mutation in ASD0326 have been previously reported. ASD0063 had developmental and epileptic encephalopathy, seizures, benign familial infantile. ASD0221 and ASD0326 had no seizures, which is consistent with the clinical symptoms of patients with the same mutation reported previously (Kothur et al., 2018; van der Werf et al., 2020).
ASD0046 carried a PUA c. 8595delT of the ASH1L gene. The histone methyltransferase encoded by this gene is involved in chromatin epigenetic modifications and is associated with the transcriptional regulation of developmentally important genes. Mutations in the ASH1L gene can lead to intellectual developmental disorder, autosomal dominant 52. About 60% of the patients have ASD (Stessman et al., 2017).
ASD0061 carried a PUA c.4242T>G of the EP300 gene, which encodes p300, a histone acetyltransferase that regulates transcription via chromatin remodeling and is important in the processes of cell proliferation and differentiation. Mutations in the EP300 gene can lead to Menke-Hennekam syndrome-2 (MKHK2), characterized by variable impairment of intellectual development and facial dysmorphisms. Feeding difficulties, autistic behavior, recurrent upper airway infections, and hearing impairment are also frequently seen (Hamilton et al., 2016; Menke et al., 2018).
ASD0134 carried a de novo c.2156dupA mutation of the ADNP gene, which encodes a zinc finger protein with a homeodomain that has the transcription factor activity and is essential for brain formation. Mutations of the ADNP gene can lead to Helsmoortel-van der Aa syndrome, which includes intellectual disability, developmental delay, ASD and facial dysmorphic features (Helsmoortel et al., 2014; Breen et al., 2020). The incidence of this gene mutation in ASD population is 0.17% (Helsmoortel et al., 2014). The c.2156dupA of ADNP gene has been reported. Patients with this mutation had neurodevelopmental disorder, ADNP-related multiple congenital anomalies, intellectual disability, and ASD (DDD Study, 2015; Chérot et al., 2018).
ASD0148 carried a PUA c.4728_4740del of the SHANK3 gene. The scaffold protein encoded by this gene is enriched in the postsynaptic compact of excitatory synapses and is associated with epithelial tubule development and excitatory synaptic transmission in the renal and enteric nervous systems. Mutations in the SHANK3 gene can lead to Phelan McDermid syndrome (Prasad et al., 2000). Phelan McDermid syndrome is a developmental disorder with a variety of abnormal clinical manifestations, including neonatal hypotonia, global developmental delay, normal to accelerated growth, severe language delay to language loss, autistic behavior, and mild dysmorphic features (Prasad et al., 2000; Durand et al., 2007). Some studies have shown that mutations in the SHANK3 gene are found in about 0.75% of ASD patients (Moessner et al., 2007). ASD0148 had increased pain tolerance, which has not been previously reported.
ASD0162 carried a PUA c.7395-2A>T of the NF1 gene. This gene encodes neurofibrin, which is mainly expressed in neurons, Schwann cells, oligodendrocytes and leukocytes. Mutations in the NF1 gene can lead to neurofibroma type I, and about 10%–40% of patients with neurofibroma type I have been reported to have ASD (Walsh et al., 2013; Eijk et al., 2018).
ASD0219 carried a PUA c.41_71del of the PRKD1 gene. The protein encoded by PRKD1 is a serine/threonine kinase that regulates a variety of cellular functions, including membrane receptor signaling, transport at the Golgi, protection from oxidative stress at the mitochondria, gene transcription, and regulation of cell shape, motility, and adhesion. Mutations in the PRKD1 gene can lead to congenital heart defects and ectodermal dysplasia (Sifrim et al., 2016). Case-control studies have shown that de novo mutations in the PRKD1 gene occur more frequently in patients with autism than in the general population (Ellaway et al., 2013; Iossifov et al., 2014; Rubeis et al., 2014; DDD Study, 2015).
ASD0294 carried a PUA c.546dupA of the PTEN gene. This gene encodes a ubiquitously expressed tumor suppressor dual-specificity phosphatase that antagonizes the PI3K signaling pathway through its lipid phosphatase activity and negatively regulates the MAPK pathway through its protein phosphatase activity. Mutations in the PTEN gene lead to macrocephaly/autism syndrome, characterized by increased head circumference, abnormal facial features, and delayed psychomotor development resulting in autistic behavior or intellectual disability (Herman et al., 2007; Page et al., 2009). Some patients may have a primary immunodeficiency disorder with recurrent infections associated with variably abnormal T- and B-cell function (Tsujita et al., 2016).
RNAseq validation of positive cases in this ASD cohort
RNAseq is often used for candidate disease gene discovery. Hear we use RNAseq to evaluate the expressional changes in the blood samples of the ASD patients with deleterious mutations.
RNA expression level of detected positive CNVs
For each positive CNV, we first calculate background expression level of all genes located on the same chromosome of the patient. Next, we randomly selected three set of out-CNV genes on the chromosome where the CNV was located as negative controls of the patient. Then we calculated the average expression level of in-CNV and out-CNV genes. Finally, we calculated the value of z-score and log2-fold change of the in-CNV and out-CNV genes. Except that the genes affected in ASD0060 were not expressed in the peripheral blood, the z-score values and log2-fold changes of all other positive CNV cases were consistent with our genomic analysis results (duplication or deletion) (Table 4).
RNA expression level of detected positive SNVs
We calculated the mutant gene expression level for SNV positive cases and used the average expression level of this gene in all other patients as the control. Several cases (the SCN2A gene of ASD0063, ASD0221 and ASD0326, the SHANK3 gene of ASD0148 and the PRKD1 gene of ASD0219) were excluded from this validation analysis because these genes were not expressed in peripheral blood. Indeed, the expression of mutant genes in the other five SNV-positive cases decreased compared with the average value of RNA expression level of 354 patients (Table 5).
Discussion
Large-scale genomic studies have revealed multiple CNVs and SNVs in the pathogenesis of ASD (Ruzzo et al., 2019; Satterstrom et al., 2020). In this study, we found 10 SNVs in 354 ASD children with a positive rate of 2.8%, and 9 CNVs with a positive rate of 2.5%. The total positive rate (5.3%) is relatively lower than previous studies (Sebat et al., 2007; Gaugler et al., 2014). The major reason is that we mostly focused on the known CNVs and de novo dominant protein-truncating SNVs. These mutations explain the genetic etiology of ASD with rare mutations. The pathological de novo mutations accounts for 4.2% (15/354) in our cohort. Other types of mutations, such as missense mutation of ASD genes, recessive SNVs, mosaicism, regulatory pathogenic variations or non-coding variants, may explain a large number of ASD in its genetic etiology (Zhang et al., 2021). The diagnostic rate of autism by other Chinese researchers ranges from 4% to 19% (Jiang et al., 2013; Wang et al., 2016; Du et al., 2018; Wu et al., 2018). The variable molecular diagnostic rate may be due to different cohorts of patients, detection methods and mutational types. It is possible in our pipeline that some pathogenic SNVs are missed due to the lower sequencing depth (∼30×) compared to regular trio-WES depth (usually > 100×). In addition, we did not exclude the patients with fragile X syndrome or those cases with inborn error of metabolism who present autistic symptoms by biochemical screening. It must be noted that we have uncovered dozens of candidate variants that are characterized as variants of uncertain significance (VUS) to be verified by functional assays as causative variants or genes for ASD.
Three patients with SNVs in our cohort were in the SCN2A gene, and two cases with CNVs were in the 15q11q13 recurrent region (PWS/AS, BP2-BP3, Class 2). This data suggests that these ASD genes are more common in Chinese ASD, which agrees with previous findings by other researchers (Wang et al., 2016; Guo et al., 2017).
The combination of WGS and CNVseq can improve the diagnostic rate to a certain extent. However, since the cause of most ASD is unclear, complex SV may be one of the genetic alterations. In subsequent studies, we will explore non-coding region variation and SV in relation to ASD to improve diagnostic rate, as well as to explore new ASD-causative genes in combination with functional assays and animal models.
The RNA sequencing results of peripheral blood of our positive patients were well consistent with expected changes, suggesting that peripheral blood RNAseq can also be used as a means of detection and verification for patients with autism. The gene expression level could be inferred from the expression in peripheral blood detection (Xu et al., 2020). However, some ASD genes are not expressed in peripheral blood, which will have certain limitations for the diagnosis of autism patients.
In our study, autistic patients with comorbidity are more likely to find the genetic etiology, especially those with intellectual disability and language retardation. This also suggests that WGS together with RNAseq is effective in identifying the cause of autism when it is accompanied by other comorbidities, which is important for early diagnosis and precise intervention of ASD.
Data availability statement
According to national legislation/guidelines, specifically the Administrative Regulations of the People’s Republic of China on Human Genetic Resources (http://www.gov.cn/zhengce/content/2019-06/10/content_5398829.htm, http://english.www.gov.cn/policies/latest_releases/2019/06/10/content_281476708945462.htm), no additional raw data is available at this time. The raw sequence data reported in this paper have been deposited in the Genome Sequence Archive (Genomics, Proteomics & Bioinformatics 2021, 19(4):578-583) in National Genomics Data Center, China National Center for Bioinformation/Beijing Institute of Genomics, Chinese Academy of Sciences (GSA-Human: HRA004176/Genetic Diagnostic Yields of 354 Chinese ASD Children with Rare Mutations by a Pipeline of Genomic Tests) that are publicly accessible at https://ngdc.cncb.ac.cn/gsa-human. Data of this project can be accessed after an approval application to the project leader, WL. Please refer to email: liwei@bch.com.cn for detailed application guidance. The accession number HRA004176 should be included in the application.
Ethics statement
The studies involving human participants were reviewed and approved by The Ethics Committee of Beijing Children’s Hospital. Written informed consent to participate in this study was provided by the participants’s legal guardian/next of kin.
Author contributions
WL, YC, CH and XN designed and supervised the study; YZ and WL wrote the manuscript; YZ, RG, and WJX performed the experiments and analyzed the data. YL and YC collected the patient information. XL, CZ, and QG participated in data analysis. WSX collected the samples and prepared DNAs/RNAs.
Funding
This work was partially supported by the Ministry of Science and Technology of China (2019YFA0802104; 2016YFC1000306); the National Natural Science Foundation of China (31830054); and the Beijing Municipal Health Commission (JingYiYan 2018-5).
Conflict of interest
The authors declare that the research was conducted in the absence of any commercial or financial relationships that could be construed as a potential conflict of interest.
Publisher’s note
All claims expressed in this article are solely those of the authors and do not necessarily represent those of their affiliated organizations, or those of the publisher, the editors and the reviewers. Any product that may be evaluated in this article, or claim that may be made by its manufacturer, is not guaranteed or endorsed by the publisher.
Supplementary material
The Supplementary Material for this article can be found online at: https://www.frontiersin.org/articles/10.3389/fgene.2023.1108440/full#supplementary-material
References
Abyzov, A., Urban, A. E., Snyder, M., and Gerstein, M. (2011). CNVnator: An approach to discover, genotype, and characterize typical and atypical CNVs from family and population genome sequencing. Genome Res. 21, 974–984. doi:10.1101/gr.114876.110
American Psychiatric Association (2013). Diagnostic and statistical manual of mental disorders. 5th ed. Washington, DC: American Psychiatric Publishing. doi:10.1176/appi.books.9780890425596
Ballif, B. C., Theisen, A., Coppinger, J., Gowans, G. C., Hersh, J. H., Madan-Khetarpal, S., et al. (2008). Expanding the clinical phenotype of the 3q29 microdeletion syndrome and characterization of the reciprocal microduplication. Mol. Cytogenet 1, 8. doi:10.1186/1755-8166-1-8
Bernier, R., Steinman, K. J., Reilly, B., Wallace, A. S., Sherr, E. H., Pojman, N., et al. (2016). Clinical phenotype of the recurrent 1q21.1 copy-number variant. Genet. Med. 18, 341–349. doi:10.1038/gim.2015.78
Breen, M. S., Garg, P., Tang, L., Mendonca, D., Levy, T., Barbosa, M., et al. (2020). Episignatures stratifying Helsmoortel-Van Der Aa Syndrome show modest correlation with phenotype. Am. J. Hum. Genet. 107, 555–563. doi:10.1016/j.ajhg.2020.07.003
Brunetti-Pierri, N., Berg, J. S., Scaglia, F., Belmont, J., Bacino, C. A., Sahoo, T., et al. (2008). Recurrent reciprocal 1q21.1 deletions and duplications associated with microcephaly or macrocephaly and developmental and behavioral abnormalities. Nat. Genet. 40, 1466–1471. doi:10.1038/ng.279
Bundey, S., Hardy, C., Vickers, S., Kilpatrick, M. W., and Corbett, J. A. (1994). Duplication of the 15q11-13 region in a patient with autism, epilepsy and ataxia. Dev. Med. Child. Neurol. 36, 736–742. doi:10.1111/j.1469-8749.1994.tb11916.x
Burnside, R. D., Pasion, R., Mikhail, F. M., Carroll, A. J., Robin, N. H., Youngs, E. L., et al. (2011). Microdeletion/microduplication of proximal 15q11.2 between BP1 and BP2: A susceptibility region for neurological dysfunction including developmental and language delay. Hum. Genet. 130, 517–528. doi:10.1007/s00439-011-0970-4
Chen, X., Schulz-Trieglaff, O., Shaw, R., Barnes, B., Schlesinger, F., Källberg, M., et al. (2016). Manta: Rapid detection of structural variants and indels for germline and cancer sequencing applications. Bioinformatics 32, 1220–1222. doi:10.1093/bioinformatics/btv710
Chérot, E., Keren, B., Dubourg, C., Carré, W., Fradin, M., Lavillaureix, A., et al. (2018). Using medical exome sequencing to identify the causes of neurodevelopmental disorders: Experience of 2 clinical units and 216 patients. Clin. Genet. 93, 567–576. doi:10.1111/cge.13102
Christian, S. L., Brune, C. W., Sudi, J., Kumar, R. A., Liu, S., Karamohamed, S., et al. (2008). Novel submicroscopic chromosomal abnormalities detected in autism spectrum disorder. Biol. Psychiatry 63, 1111–1117. doi:10.1016/j.biopsych.2008.01.009
Città, S., Buono, S., Greco, D., Barone, C., Alfei, E., Bulgheroni, S., et al. (2013). 3q29 microdeletion syndrome: Cognitive and behavioral phenotype in four patients. Am. J. Med. Genet. A 161A, 3018–3022. doi:10.1002/ajmg.a.36142
ClinGen (2018). 22q11.2 recurrent (DGS/VCFS) region (proximal, A-D) (includes TBX1). Available from: https://search.clinicalgenome.org/kb/gene-dosage/region/ISCA-37446.
Dabell, M. P., Rosenfeld, J. A., Bader, P., Escobar, L. F., El-Khechen, D., Vallee, S. E., et al. (2013). Investigation of NRXN1 deletions: Clinical and molecular characterization. Am. J. Med. Genet. A 161A, 717–731. doi:10.1002/ajmg.a.35780
DDD Study (2015). Large-scale discovery of novel genetic causes of developmental disorders. Nature 519, 223–228. doi:10.1038/nature14135
Dias, C. M., and Walsh, C. A. (2020). Recent advances in understanding the genetic architecture of autism. Annu. Rev. Genomics Hum. Genet. 21, 21289–21304. doi:10.1146/annurev-genom-121219-082309
Dobin, A., Davis, C. A., Schlesinger, F., Drenkow, J., Zaleski, C., Jha, S., et al. (2013). STAR: Ultrafast universal RNA-seq aligner. Bioinformatics 29, 15–21. doi:10.1093/bioinformatics/bts635
Du, X., Gao, X., Liu, X., Shen, L., Wang, K., Fan, Y., et al. (2018). Genetic diagnostic evaluation of trio-based whole exome sequencing among children with diagnosed or suspected autism spectrum disorder. Front. Genet. 9, 594. doi:10.3389/fgene.2018.00594
Durand, C. M., Betancur, C., Boeckers, T. M., Bockmann, J., Chaste, P., Fauchereau, F., et al. (2007). Mutations in the gene encoding the synaptic scaffolding protein SHANK3 are associated with autism spectrum disorders. Nat. Genet. 39, 25–27. doi:10.1038/ng1933
Eijk, S., Mous, S. E., Dieleman, G. C., Dierckx, B., Rietman, A. B., de Nijs, P. F. A., et al. (2018). Autism spectrum disorder in an unselected cohort of children with neurofibromatosis type 1 (NF1). J. Autism Dev. Disord. 48, 2278–2285. doi:10.1007/s10803-018-3478-0
Ellaway, C. J., Ho, G., Bettella, E., Knapman, A., Collins, F., Hackett, A., et al. (2013). 14q12 microdeletions excluding FOXG1 give rise to a congenital variant Rett syndrome-like phenotype. Eur. J. Hum. Genet. 21, 522–527. doi:10.1038/ejhg.2012.208
Firth, H. V. (1993). 22q11.2 Duplication – archived Chapter, for historical reference only. GeneReviews.
Gaugler, T., Klei, L., Sanders, S. J., Bodea, C. A., Goldberg, A. P., Lee, A. B., et al. (2014). Most genetic risk for autism resides with common variation. Nat. Genet. 46, 881–885. doi:10.1038/ng.3039
Guo, H., Peng, Y., Hu, Z., Li, Y., Xun, G., Ou, J., et al. (2017). Genome-wide copy number variation analysis in a Chinese autism spectrum disorder cohort. Sci. Rep. 7, 44155. doi:10.1038/srep44155
Hamilton, M. J., Newbury-Ecob, R., Holder-Espinasse, M., Yau, S., Lillis, S., Hurst, J. A., et al. (2016). Rubinstein-taybi syndrome type 2: Report of nine new cases that extend the phenotypic and genotypic spectrum. Clin. Dysmorphol. 25, 135–145. doi:10.1097/MCD.0000000000000143
Hellemans, J., Mortier, G., Paepe, A. D., Speleman, F., and Vandesompele, J. (2007). qBase relative quantification framework and software for management and automated analysis of real-time quantitative PCR data. Genome Biol. 8, R19. doi:10.1186/gb-2007-8-2-r19
Helsmoortel, C., Vulto-van Silfhout, A. T., Coe, B. P., Vandeweyer, G., Rooms, L., van den Ende, J., et al. (2014). A SWI/SNF-related autism syndrome caused by de novo mutations in ADNP. Nat. Genet. 46, 380–384. doi:10.1038/ng.2899
Herman, G. E., Butter, E., Enrile, B., Pastore, M., Prior, T. W., and Sommer, A. (2007). Increasing knowledge of PTEN germline mutations: Two additional patients with autism and macrocephaly. Am. J. Med. Genet. A 143A, 589–593. doi:10.1002/ajmg.a.31619
Iossifov, I., O'Roak, B. J., Sanders, S. J., Ronemus, M., Krumm, N., Levy, D., et al. (2014). The contribution of de novo coding mutations to autism spectrum disorder. Nature 515, 216–221. doi:10.1038/nature13908
Jiang, Y. H., Yuen, R. K., Jin, X., Wang, M., Chen, N., Wu, X., et al. (2013). Detection of clinically relevant genetic variants in autism spectrum disorder by whole-genome sequencing. Am. J. Hum. Genet. 93, 249–263. doi:10.1016/j.ajhg.2013.06.012
Kendall, K. M., Bracher-Smith, M., Fitzpatrick, H., Lynham, A., Rees, E., Escott-Price, V., et al. (2019). Cognitive performance and functional outcomes of carriers of pathogenic copy number variants: Analysis of the UK biobank. Br. J. Psychiatry 214, 297–304. doi:10.1192/bjp.2018.301
Khattabi, L. A. E., Heide, S., Caberg, J-H., Andrieux, J., Fenzy, M. D., Vincent-Delorme, C., et al. (2020). 16p13.11 microduplication in 45 new patients: Refined clinical significance and genotype-phenotype correlations. J. Med. Genet. 57, 301–307. doi:10.1136/jmedgenet-2018-105389
Kothur, K., Holman, K., Farnsworth, E., Ho, G., Lorentzos, M., Troedson, C., et al. (2018). Diagnostic yield of targeted massively parallel sequencing in children with epileptic encephalopathy. Seizure 59, 132–140. doi:10.1016/j.seizure.2018.05.005
Lek, M., Karczewski, K. J., Minikel, E. V., Samocha, K. E., Banks, E., Fennell, T., et al. (2016). Analysis of protein-coding genetic variation in 60,706 humans. Nature 536, 285–291. doi:10.1038/nature19057
Levy, D., Ronemus, M., Yamrom, B., Lee, Y-H., Leotta, A., Kendall, J., et al. (2011). Rare de novo and transmitted copy-number variation in autistic spectrum disorders. Neuron 70, 886–897. doi:10.1016/j.neuron.2011.05.015
Lobar, S. L. (2016). DSM-V changes for autism spectrum disorder (ASD): Implications for diagnosis, management, and care coordination for children with ASDs. J. Pediatr. Health Care 30, 359–365. doi:10.1016/j.pedhc.2015.09.005
Loomes, R., Hull, L., and Mandy, W. P. L. (2017). What is the male-to-female ratio in autism spectrum disorder? A systematic review and meta-analysis. J. Am. Acad. Child. Adolesc. Psychiatry 56, 466–474. doi:10.1016/j.jaac.2017.03.013
Lord, C., Elsabbagh, M., Baird, G., and Veenstra-Vanderweele, J. (2018). Autism spectrum disorder. Lancet 392, 508–520. doi:10.1016/S0140-6736(18)31129-2
Maenner, M. J., Shaw, K. A., Baio, J., Washington, A., Patrick, M., et al. EdS1 (2020). Prevalence of autism spectrum disorder Among children aged 8 Years - autism and developmental disabilities monitoring network, 11 sites, United States, 2016. Morbidity and mortality weekly report, 69. Washington, D.C., 1–12. Surveillance summaries. doi:10.15585/mmwr.ss6904a1
Menke, L. A., Gardeitchik, T., Hammond, P., Heimdal, K. R., Houge, G., et al. DDD study (2018). Further delineation of an entity caused by CREBBP and EP300 mutations but not resembling Rubinstein-Taybi syndrome. Am. J. Med. Genet. A 176, 862–876. doi:10.1002/ajmg.a.38626
Moessner, R., Marshall, C. R., Sutcliffe, J. S., Skaug, J., Pinto, D., Vincent, J., et al. (2007). Contribution of SHANK3 mutations to autism spectrum disorder. Am. J. Hum. Genet. 81, 1289–1297. doi:10.1086/522590
Neale, B. M., Kou, Y., Liu, L., Ma'ayan, A., Samocha, K. E., Sabo, A., et al. (2012). Patterns and rates of exonic de novo mutations in autism spectrum disorders. Nature 485, 242–245. doi:10.1038/nature11011
O'Roak, B. J., Vives, L., Girirajan, S., Karakoc, E., Krumm, N., Coe, B. P., et al. (2012). Sporadic autism exomes reveal a highly interconnected protein network of de novo mutations. Nature 485, 246–250. doi:10.1038/nature10989
O'Roak, B. J., Vives, L., Fu, W., Egertson, J. D., Stanaway, I. B., Phelps, I. G., et al. (2012). Multiplex targeted sequencing identifies recurrently mutated genes in autism spectrum disorders. Science 338, 1619–1622. doi:10.1126/science.1227764
Page, D. T., Kuti, O. J., Prestia, C., and Sur, M. (2009). Haploinsufficiency for Pten and Serotonin transporter cooperatively influences brain size and social behavior. Proc. Natl. Acad. Sci. U.S.A. 106, 1989–1994. doi:10.1073/pnas.0804428106
Popow, C., Ohmann, S., and Plener, P. (2021). Practitioner's review: Medication for children and adolescents with autism spectrum disorder (ASD) and comorbid conditions. Neuropsych 35, 113–134. doi:10.1007/s40211-021-00395-9
Prasad, C., Prasad, A. N., Chodirker, B. N., Lee, C., Dawson, A. K., Jocelyn, L. J., et al. (2000). Genetic evaluation of pervasive developmental disorders: The terminal 22q13 deletion syndrome may represent a recognizable phenotype. Clin. Genet. 57, 103–109. doi:10.1034/j.1399-0004.2000.570203.x
Quintero-Rivera, F., Sharifi-Hannauer, P., and Martinez-Agosto, J. A. (2010). Autistic and psychiatric findings associated with the 3q29 microdeletion syndrome: Case report and review. Am. J. Med. Genet. A 152A, 2459–2467. doi:10.1002/ajmg.a.33573
Richards, S., Aziz, N., Bale, S., Bick, D., Das, S., Gastier-Foster, J., et al. (2015). Standards and guidelines for the interpretation of sequence variants: A joint consensus recommendation of the American College of medical genetics and genomics and the association for molecular pathology. Genet. Med. 17, 405–424. doi:10.1038/gim.2015.30
Rubeis, S. D., He, X., Goldberg, A. P., Poultney, C. S., Samocha, K., Cicek, A. E., et al. (2014). Synaptic, transcriptional and chromatin genes disrupted in autism. Nature 515, 209–215. doi:10.1038/nature13772
Rutherford, M., McKenzie, K., Johnson, T., Catchpole, C., O'Hare, A., McClure, I., et al. (2016). Gender ratio in a clinical population sample, age of diagnosis and duration of assessment in children and adults with autism spectrum disorder. Autism 20, 628–634. doi:10.1177/1362361315617879
Ruzzo, E. K., Pérez-Cano, L., Jung, J-Y., Wang, L-K., Kashef-Haghighi, D., Hartl, C., et al. (2019). Inherited and de novo genetic risk for autism impacts shared networks. Cell 178, 850–866.e26. doi:10.1016/j.cell.2019.07.015
Saitsu, H., Tohyama, J., Walsh, T., Kato, M., Kobayashi, Y., Lee, M., et al. (2014). A girl with West syndrome and autistic features harboring a de novo TBL1XR1 mutation. J. Hum. Genet. 59, 581–583. doi:10.1038/jhg.2014.71
Sanders, S. J., Murtha, M. T., Gupta, A. R., Murdoch, J. D., Raubeson, M. J., Willsey, A. J., et al. (2012). De novo mutations revealed by whole-exome sequencing are strongly associated with autism. Nature 485, 237–241. doi:10.1038/nature10945
Sandin, S., Lichtenstein, P., Kuja-Halkola, R., Hultman, C., Larsson, H., and Reichenberg, A. (2017). The heritability of autism spectrum disorder. J. Am. Med. Assoc. 318, 1182–1184. doi:10.1001/jama.2017.12141
Satterstrom, F. K., Kosmicki, J. A., Wang, J., Breen, M. S., Rubeis, S. D., An, J-Y., et al. (2020). Large-scale exome sequencing study implicates both developmental and functional changes in the neurobiology of autism. Cell 180, 568–584.e23. doi:10.1016/j.cell.2019.12.036
Schaaf, C. P., Boone, P. M., Sampath, S., Williams, C., Bader, P. I., Mueller, J. M., et al. (2012). Phenotypic spectrum and genotype-phenotype correlations of NRXN1 exon deletions. Eur. J. Hum. Genet. 20, 1240–1247. doi:10.1038/ejhg.2012.95
Sebat, J., Lakshmi, B., Malhotra, D., Troge, J., Lese-Martin, C., Walsh, T., et al. (2007). Strong association of de novo copy number mutations with autism. Science 316, 445–449. doi:10.1126/science.1138659
Sifrim, A., Hitz, M-P., Wilsdon, A., Breckpot, J., Turki, S., Thienpont, B., et al. (2016). Distinct genetic architectures for syndromic and nonsyndromic congenital heart defects identified by exome sequencing. Nat. Genet. 48, 1060–1065. doi:10.1038/ng.3627
Simonoff, E., Pickles, A., Charman, T., Chandler, S., Loucas, T., and Baird, G. (2008). Psychiatric disorders in children with autism spectrum disorders: Prevalence, comorbidity, and associated factors in a population-derived sample. J. Am. Acad. Child. Adolesc. Psychiatry 47, 921–929. doi:10.1097/CHI.0b013e318179964f
Stessman, H. A. F., Xiong, B., Coe, B. P., Wang, T., Hoekzema, K., Fenckova, M., et al. (2017). Targeted sequencing identifies 91 neurodevelopmental-disorder risk genes with autism and developmental-disability biases. Nat. Genet. 49, 515–526. doi:10.1038/ng.3792
Tavassoli, T., Kolevzon, A., Wang, A. T., Curchack-Lichtin, J., Halpern, D., Schwartz, L., et al. (2014). De novo SCN2A splice site mutation in a boy with Autism spectrum disorder. BMC Med. Genet. 15, 35. doi:10.1186/1471-2350-15-35
Tsujita, Y., Mitsui-Sekinaka, K., Imai, K., Yeh, T-W., Mitsuiki, N., Asano, T., et al. (2016). Phosphatase and tensin homolog (PTEN) mutation can cause activated phosphatidylinositol 3-kinase δ syndrome-like immunodeficiency. J. Allergy Clin. Immunol. 138, 1672–1680.e10. doi:10.1016/j.jaci.2016.03.055
Van der Aa, N., Rooms, L., Vandeweyer, G., van den Ende, J., Reyniers, E., Fichera, M., et al. (2009). Fourteen new cases contribute to the characterization of the 7q11.23 microduplication syndrome. Eur. J. Med. Genet. 52, 94–100. doi:10.1016/j.ejmg.2009.02.006
van der Werf, I. M., Jansen, S., de Vries, P. F., Gerstmans, A., van de Vorst, M., Dijck, A. V., et al. (2020). Overrepresentation of genetic variation in the AnkyrinG interactome is related to a range of neurodevelopmental disorders. Eur. J. Hum. Genet. 28, 1726–1733. doi:10.1038/s41431-020-0682-0
Walsh, K. S., Vélez, J. I., Kardel, P. G., Imas, D. M., Muenke, M., Packer, R. J., et al. (2013). Symptomatology of autism spectrum disorder in a population with neurofibromatosis type 1. Dev. Med. Child. Neurol. 55, 131–138. doi:10.1111/dmcn.12038
Wang, K., Li, M., and Hakonarson, H. (2010). Annovar: Functional annotation of genetic variants from high-throughput sequencing data. Nucleic Acids Res. 38, e164. doi:10.1093/nar/gkq603
Wang, T., Guo, H., Xiong, B., Stessman, H. A. F., Wu, H., Coe, B. P., et al. (2016). De novo genic mutations among a Chinese autism spectrum disorder cohort. Nat. Commun. 7, 13316. doi:10.1038/ncomms13316
Wu, J., Yu, P., Jin, X., Xu, X., Li, J., Li, Z., et al. (2018). Genomic landscapes of Chinese sporadic autism spectrum disorders revealed by whole-genome sequencing. J. Genet. Genomics 45, 527–538. doi:10.1016/j.jgg.2018.09.002
Xi, R., Hadjipanayis, A. G., Luquette, L. J., Kim, T-M., Lee, E., Zhang, J., et al. (2011). Copy number variation detection in whole-genome sequencing data using the Bayesian information criterion. Proc. Natl. Acad. Sci. U.S.A. 108, E1128–E1136. doi:10.1073/pnas.1110574108
Xu, W., Leng, F., Liu, X., and Li, W. (2020). Blood-based multi-tissue gene expression inference with Bayesian ridge regression. Bioinformatics 36, 3788–3794. doi:10.1093/bioinformatics/btaa239
Yépez, V. A., Mertes, C., Müller, M. F., Klaproth-Andrade, D., Wachutka, L., Frésard, L., et al. (2021). Detection of aberrant gene expression events in RNA sequencing data. Nat. Protoc. 16, 1276–1296. doi:10.1038/s41596-020-00462-5
Keywords: autism spectrum disorder, whole-genome sequencing, RNA sequencing, copy number variation, single nucleotide variation
Citation: Zhang Y, Li Y, Guo R, Xu W, Liu X, Zhao C, Guo Q, Xu W, Ni X, Hao C, Cui Y and Li W (2023) Genetic diagnostic yields of 354 Chinese ASD children with rare mutations by a pipeline of genomic tests. Front. Genet. 14:1108440. doi: 10.3389/fgene.2023.1108440
Received: 26 November 2022; Accepted: 15 March 2023;
Published: 23 March 2023.
Edited by:
Hu Hao, The Sixth Affiliated Hospital of Sun Yat-sen University, ChinaReviewed by:
Nora Urraca, University of Tennessee Health Science Center (UTHSC), United StatesMingbang Wang, South China Hospital of Shenzhen University, China
Copyright © 2023 Zhang, Li, Guo, Xu, Liu, Zhao, Guo, Xu, Ni, Hao, Cui and Li. This is an open-access article distributed under the terms of the Creative Commons Attribution License (CC BY). The use, distribution or reproduction in other forums is permitted, provided the original author(s) and the copyright owner(s) are credited and that the original publication in this journal is cited, in accordance with accepted academic practice. No use, distribution or reproduction is permitted which does not comply with these terms.
*Correspondence: Wei Li, liwei@bch.com.cn; Yonghua Cui, cuiyonghua@bch.com.cn; Chanjuan Hao, hchjhchj@163.com; Xin Ni, nixin@bch.com.cn
†These authors have contributed equally to this work