- 1School of Biomedical Sciences, Faculty of Medicine, The Chinese University of Hong Kong, Shatin, Hong Kong SAR, China
- 2Hong Kong Bioinformatics Centre, The Chinese University of Hong Kong, Shatin, Hong Kong SAR, China
- 3Department of Dermatology, The Third Affiliated Hospital off Sun Yat-Sen University, Guangzhou, Hong Kong SAR, China
- 4Department of Orthopaedics and Traumatology, Faculty of Medicine, The Chinese University of Hong Kong, Shatin, China
- 5Department of Orthopaedics and Limb Reconstruction/Paediatric Orthopaedics, South China Hospital of Shenzhen University, Shenzhen, China
- 6Advanced Medical Research Center, Zhengzhou Central Hospital Affiliated to Zhengzhou University, Zhengzhou, China
- 7Centre for Microbial Genomics and Proteomics, The Chinese University of Hong Kong, Shatin, Hong Kong SAR, China
Observational studies revealed altered gut microbial composition in patients with allergic diseases, which illustrated a strong association between the gut microbiome and the risk of allergies. However, whether such associations reflect causality remains to be well-documented. Two-sample mendelian randomization (2SMR) was performed to estimate the potential causal effect between the gut microbiota and the risk of allergic diseases. 3, 12, and 16 SNPs at the species, genus, and family levels respectively of 15 microbiome features were obtained as the genetic instruments of the exposure dataset from a previous study. GWAS summary data of a total of 17 independent studies related to allergic diseases were collected from the IEU GWAS database for the outcome dataset. Significant causal relationships were obtained between gut microbiome features including Ruminococcaceae, Eggerthella, Bifidobacterium, Faecalibacterium, and Bacteroides and the risk of allergic diseases. Furthermore, our results also pointed out a number of putative associations between the gut microbiome and allergic diseases. Taken together, this study was the first study using the approach of 2SMR to elucidate the association between gut microbiome and allergic diseases.
Introduction
Allergic diseases, also known as allergies, including hay fever, allergic rhinitis, asthma, and atopic dermatitis, are the most common chronic immunological diseases and are prevalent in adults and children and persists throughout life (Akdis, 2006). Allergic diseases are mainly caused by IgE-dependent immunological reaction to the allergens and are characterized by the T cell response, including high production of IL-4, IL-5, IL-9, IL-10, and IL-13 and low production of INF-γ (Veldhoen et al., 2008). On the other hand, allergic diseases are genetically predisposed (Aldakheel, 2021). In addition to genetic factors, the gut microbiome has gradually been considered as one of the important risk factors for allergic diseases in recent years due to its influence on the host’s immune response and its interaction with host genetic SNPs (Pascal et al., 2018).
The general term for the microbial community in the human intestine is known as the gut microbiome, disorders of which can cause the occurrence of various diseases such as immune-related, oncologic, neurologic, and metabolic diseases (Gomaa, 2020). Major factors affecting the gut microbiome are divided into three categories: host-extrinsic factors like diet, drugs and lifestyle, host-intrinsic factors such as age, gender, and genetic factors, and environmental factors (Schmidt et al., 2018). The gut microbiome plays a pivotal role in the host’s nutrient metabolism and immunity, which makes it one of the major influencing factors of allergic diseases (Pascal et al., 2018). There are numbers of cross-sectional studies that illustrates there is an altered gut microbiome composition in the patients with allergies. For example, the colonization of Bacteroides fragilis was identified to show a positive correlation with the prevalence of asthma during early childhood development. And a relative abundance of Ruminococcaceae and Bifidobacterium lead to an increase asthma and eczema in infants (Vael et al., 2008; Begley et al., 2018; Berni Canani et al., 2019; Wang et al., 2020; Zhu et al., 2020). However, the causal relationship between gut microbiota composition and allergic disease remains elusive.
The mendelian randomization (MR) model uses genetic variation, the single nucleotide polymorphism (SNP), as the instrumental variable to infer the causal effect size and direction between exposure factors and outcomes (Lawlor et al., 2008). Two-sample mendelian randomization (2SMR) applies the MR methods to estimate the causal effect size of the GWAS summary datasets of two independent studies. This method was wildly used in causal inference detection between the complex diseases such as psychiatric disorders, celiac disease, obesity, T2D, and cardiovascular diseases and specific genera in the host gut microbiome as well as their secretion. Alterations of the relative abundance of Bifidobacterium can reduce the risk of ischemic heart disease as well as obesity, and the concentration of the blood low-density lipoprotein. Moreover, changes in the relative abundance of several genera and species, such as Acidaminococcus, Aggregatibacter, Blautia, Desulfovibrio, and Faecalibacterium, are causes of type 2 diabetes (Yang et al., 2018). Furthermore, Serena et al. suggests that the increase in the content of propionic acid produced by the metabolism of the intestinal flora can also cause an increase in the risk of type 2 diabetes (Sanna et al., 2019). The rich experience of 2SMR and the mature and public GWAS summary databases make the utility of 2SMR on gut microbiome and allergic diseases possible.
In this study, we conducted the two-sample MR analysis to investigate the interplay between host genetics, gut microbiome composition, and allergic diseases including asthma, eczema, hay fever, as well as allergic rhinitis by using GWAS summary datasets from published studies. Our findings provide not only new directions for the treatment and the diagnostic but also valuable insights into early screening of the allergic diseases.
Materials and methods
Two-sample mendelian randomization analysis was performed using the R package TwoSampleMR (v.0.5.6) (Hemani et al., 2018) and the in-house R scripts used to perform 2SMR analysis and generate figures were available on GitHub (https://github.com/evyforjazz/2SMR). Supplementary Figure S1 illustrated the flowchart of the analysis process.
Exposure data preparation
Significant SNPs related to the relative abundance of the gut bacteria taxa were selected as the genetic instruments of the exposure data from a public microbiome-GWAS study. Briefly, Goodrich et al. (2016) analyzed the genetic association between 1,300,091 SNPs and 945 taxa of 1126 United Kingdom twin pairs. 307 SNPs were calculated to be correlated with 61 taxa and the summary dataset could be obtained from the Supplementary Table S5 of the original publication. To ensure that the genetic instruments of the exposure data were independent, after acquiring the significant SNPs list, the European (EUR) genotype in the 1,000 human genomes project was used as the reference template for linkage disequilibrium (LD) analysis. The maximum LD R-square value was set to be 0.1 and the distance of searching for LD R-square values was set to be 500 kb. Using a stricter threshold, p < 5 × 10−8 was the criteria for the selection of the significant SNPs. After performing the step of clumping, 3, 12, and 16 SNPs at the species, genus, and family levels respectively of 15 microbiome features were obtained as the genetic instruments of the exposure dataset for the following two-sample mendelian randomization analysis (Supplementary Table S1).
Outcome data collection from the IEU GWAS database
IEU GWAS Database (IGD) contains 39,603 GWAS summary studies from 18 batches, which can be obtained and applied to the mendelian randomization analysis using the R package, TwoSampleMR (Abrahamsson et al., 2012). GWAS summary data of a total of 17 independent studies with the trait of allergic asthma, eczema, hay fever, allergic rhinitis, pollen allergy, and medicine or food allergy were collected from IGD (Supplementary Table S2).
Data harmonization and causal effect evaluation
To make sure the effect of the same SNP of both exposure and outcome data were corresponding to the same allele, the harmonise_data() function of the TwoSampleMR(v0.5.6) package was performed to harmonize the exposure and outcome data. For microbiome features including multiple SNPs, an inverse variance weighted (IVW) was performed to evaluate the causal association. The IVW method is an effective analysis on the assumption that all genetic variations are effective instrumental variables and has a strong causality detection ability (Burgess et al., 2013). For microbiome features containing only 1 SNP, a wild ratio was used to measure the causal effect.
Sensitivity analysis
MR-Egger regression method was performed to test the horizontal pleiotropy and heterogeneity. Exposure data of the microbiome features containing over 3 SNPs could be used for MR-Egger regression analysis (Bowden et al., 2015). To estimate the causal effect size of each SNP in the microbiome feature allergic diseases, MR_Singlesnp() function was performed. For the microbiome feature with a p-value less than 0.05 using the IVW method and passing the heterogeneity analysis and the horizontal pleiotropy analysis, the leave-one-out method was applied for the sensitivity analysis to identify if a single SNP is driving the association.
Results
Mendelian randomization results of causal effects between the gut microbiome and atopic dermatitis
IVW and WR methods were performed to assess the causal relationship between the abundance of intestinal flora and atopic dermatitis. We found that the causal effect values of the three microbiome features of the same family Coriobacteriaceae and eczema were nominally significant (Table 1). MR-Egger regression was tested for heterogeneity and horizontal pleiotropy. And the instrumental variables showed no heterogeneity and pleiotropy, which could be ignored for the causal effect estimation (p > 0.05, Supplementary Table S3). The increase of the relative abundance of the Coriobateriaceae (p = 0.0074) at the family level, Eggerthella (p = 0.0074) at the genus level, and Eggerthella lenta (p = 0.0102) at the species level was positively and causally related to the elevating of the risk of atopic dermatitis (Table 1; Figure 1). Only one SNP was related to each significant microbiome feature and the exposure microbiome features Coriobacteriaceae at the family level and Eggerthella at the genus level shared the same genetic instrument rs1376236. Therefore, the result of the single SNP analysis was the same as the causal estimation analysis results (Figure 1).

FIGURE 1. Single SNP analysis between the gut microbiome and AD. Only significant results were displayed.
Causal effects of gut microbiota on the risk of hay fever, eczema, and allergic rhinitis
Significant causal relationships were evaluated between 5 gut microbiome features and hay fever, eczema, or allergic rhinitis (Table 2). The heterogeneity and horizontal pleiotropy could be ignored in the causal estimation between the gut microbiome and allergic diseases (Supplementary Table S4). Our results illustrated that 3 gut microbiome features including Bifidobacteriaceae at the family level, Bifidobacterium and Anaerostipes at the genus level were positively and causally correlated to the risk of hay fever, eczema, or allergic rhinitis. And the 2 microbiome features, Clostridiaceae at the family level and Dorea at the genus level were negatively and causally related to the risk of hay fever, allergic rhinitis, or eczema (Table 2). Only one SNP was related to each significant microbiome feature. Therefore, the causal effect size and direction of single SNP analysis results was the same as the 2SMR analysis. rs1446585, rs10055309, rs10233359, and rs12604607 were identified to be causally and considerably associated with the risk of hay fever, eczema, and rhinitis (Figure 2).
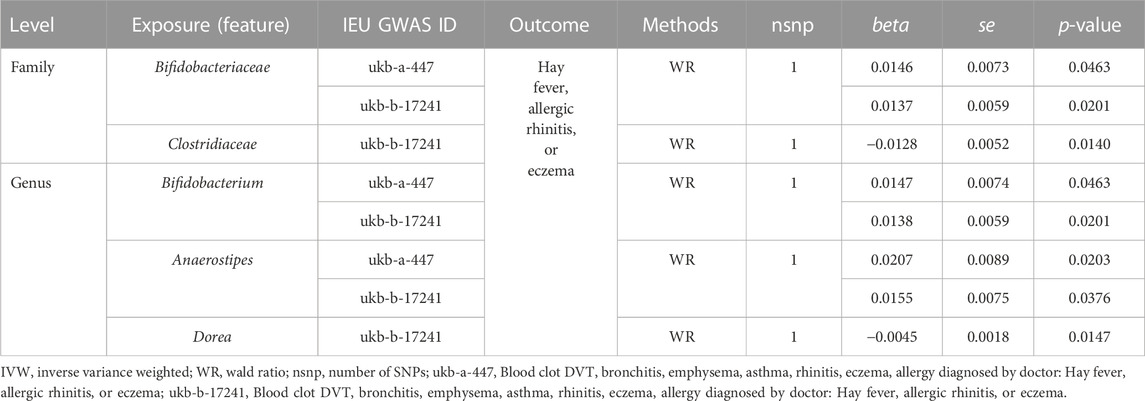
TABLE 2. Significant 2SMR analysis results between gut microbiome composition and hay fever, allergic rhinitis, or eczema.
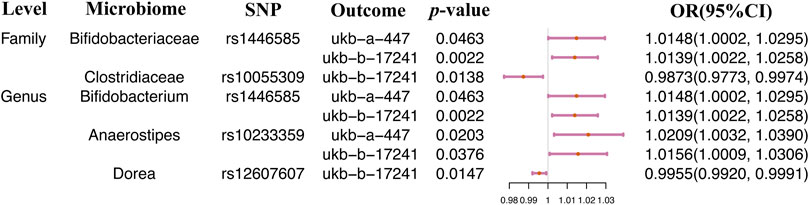
FIGURE 2. Single SNP analysis between the gut microbiome and eczema, hay fever, and allergic rhinitis.
Seven gut microbiome features were identified causally and significantly related to asthma risk
Significant causal relationships were evaluated between 7 gut microbiome features and asthma. The heterogeneity and horizontal pleiotropy could be ignored in the causal estimation between the gut microbiome and asthma (Supplementary Table S4). The increase of the relative abundance of 5 microbiome features, Bifidobacteriaceae, Bacteroidaceae, and Ruminococcaceae at the family level, Bifidobacterium and Bacteroides at the genus level, and the decrease of the relative abundance of 2 microbiome features, Faecalibacterium and Faecalibacterium prausnitzii, contribute to the risk of asthma (Table 3). A Leave-one-out analysis was performed on the microbiome features containing over 2SNPs, and the results were consistent with the 2SMR analysis (Supplementary Table S5). Single SNP analysis results were illustrated in Figure 3. 4 SNPs, rs146585 correlated with the microbiome features Bifidobacteriaceae at the family level and Bifidobacterium at the genus level, rs7486170 associated with the Faecalibacterium at the genus level and F. prausnitzii at the species level, rs70589 related to Ruminococcaceae, and rs10507725 correlated to Bacteroidaceae at the family level and Bacteroides at the genus level, was detected to demonstrate causal relationships with the risk of asthma (Figure 3).
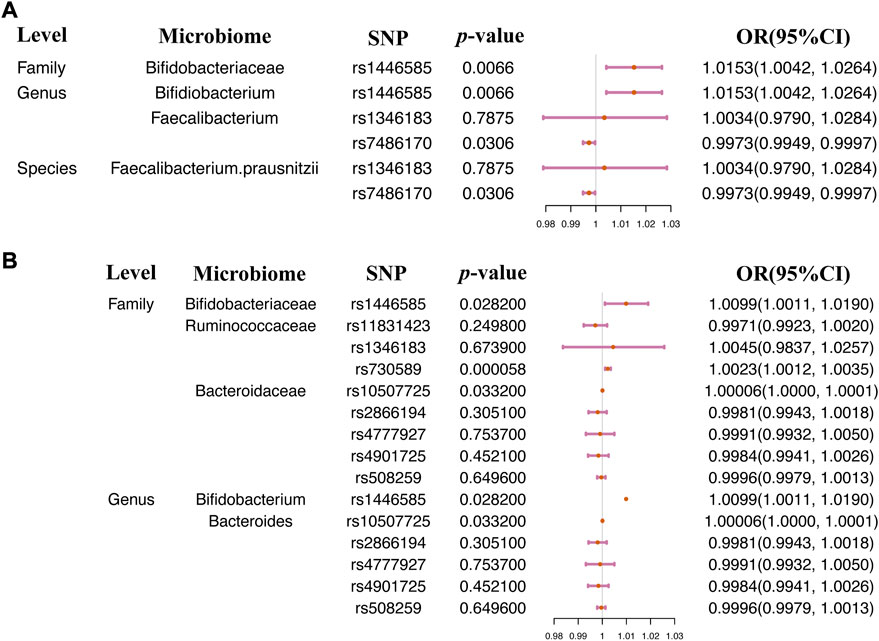
FIGURE 3. Single SNP analysis between the gut microbiome and asthma. Single SNP analysis was performed on two asthma GWAS summary datasets (A) ukb-a-446 and (B) ukb-b-20296.
Discussion
As far as we know, we are the first to perform the 2SMR approach to assess the potential causal associations between the gut microbiome and allergic diseases including eczema, asthma, hay fever, and allergic rhinitis. We found genetic liability to some gut microbiota causally associated with allergic diseases and some gut microbiota that might be potential risk factors for allergies.
Our results indicated that the enrichment of the Eggerthella, belonging to the family Coriobacteriaceae and related to rs1376236, as well as its species E. lenta correlated with rs1376235 were causally related to atopic dermatitis. Thomas et al. demonstrated that compared with healthy infants, the relative abundance of Eggerthella in the colon of the 12-month-old eczema infants raised significantly (Reddel et al., 2019). However, Sofia et al. reported an absolutely opposite result (Abrahamsson et al., 2012). This controversial condition might be attributed to the limited sample size and regional inconsistencies. Moreover, IVs using in causal inference were selected based on the relationship between the intestinal flora and host genetics. And the gut flora was not only affected by host genetic, but also by other factors such as diet, medication, and cultural habits. In addition, Eggerthella was detected to be significantly in the gut microbiome of allergic rhinitis patients by comparison with patients with eczema and measles (Su et al., 2021). Furthermore, E. lenta under the genera Eggerthella was shown to be highly enriched in the gut flora of patients with asthma and rheumatoid arthritis, comparing to the normal population (Chen et al., 2016; Wang et al., 2018). Additionally, the colonization of the E. lenta was evidenced to induce the activation of the Th17, leading to colitis (Alexander et al., 2019).
We also found that Ruminococcaceae at the family level, and Bifidobacterium and Bacteroides at the genus level were positively associated with the risk of asthma. Previous studies revealed that a higher abundance of Ruminococcaceae was colonized in the intestinal flora of infants with food allergies and atopic eczema by comparison with healthy infants (Berni Canani et al., 2019; Zhu et al., 2020). Besides, the results of a tracking study by Carl et al. on the gut microbiome of 117 infants supported our causal inference results regarding Bacteroides and the risk of asthma. Carl et al. found that the colonization of B. fragilis was positively correlated with the prevalence of asthma during early development. They also put forward a proposal that the enrichment of the B. fragilis in early life played a role as a potential biomarker of possible asthma (Vael et al., 2008). In addition, Begley et al. (2018) demonstrated that compared with normal children, the relative abundance of Bifidobacterium declined significantly in the gut bacteria community of children at high risk of asthma. Notably, certain strains of Bifidobacterium had been illustrated to alleviate asthma via inhibiting Th1/Th2 activation (Wang et al., 2020). The inconsistency between our causal estimation and the results of clinical trials as stated above might be traceable to the following reasons. First, as mentioned above, our causal evaluation was based on the host genetic variations. Both gut microbiome and human diseases were not only influenced by host inheritance but also affected by other factors such as diet and lifestyle. Second, pleiotropic testing and sensitivity analysis could not be performed because only one SNP, rs1446585, was selected as the exposure IV of Bifidobacterium. Thus, we failed to exclude the impact of the horizontal pleiotropy on causal inference between Bifidobacterium and asthma.
Conversely, we evidenced that the increase of F. prausnitzii potentially resulted in a lessening of the risk of asthma, which supported the findings of the longitudinal study conducted by Galazzo et al. (2020) (Alexander et al., 2019). In children who developed eczema and asthma, the deficiency of Faecalibacterium was detected in their intestinal microbiome during infancy. Similar conclusions were reached by Arrieta et al. (2015) and Stokholm et al. (2018) using sequencing approaches.
Interestingly, we found that the significant causal relationships between Clostridiaceae at the family level and Dorea at the genus level and the risk of hay fever, allergic rhinitis, or eczema allergic could not be replicated in two similar outcome studies. The same inconsistent results were also seen when assessing the causal association between Bacteroidaceae and Ruminococcaceae at the family level, Bacteroides and Faecalibacterium at the genus level, and asthma risk. First, this may be due to the limited number of SNPs included in this study, resulting in insufficient statistical significance to identify associations. Second, the gut microbiome composition is more affected by environmental factors. Genetic differences have a minimal impact on given exposure, which could just explain a small proportion of the alteration of the gut microbiome. So, in some cases, our analysis might have had insufficient power to detect a causal relationship.
The present study had several limitations. First, the IVs selected in this study were all correlated to the relative abundance of the individual bacteria in the gut microbiome, but the IVs related to the functional metabolic pathways of the gut flora were lacking. The metabolites of the intestinal microbiome might be directly related to human diseases and illustrate a more intuitive causal association with the diseases. Besides, the relatively small sample size of the microbiome-GWAS study (n = 1,126 United Kingdom twins) used in this study might lead to some bacteria that were found to be potentially causally related to allergic disease in one study, but could not be identified repeatedly in another study, which reduced the confidence of the true causal relationship. Moreover, most of the bacteria taxa analyzed in this study were at the genus level, and future analysis at the level of species and strains will improve the accuracy of causality. In addition, our study only used GWAS summary data to complete the analysis of 2SMR and ignored the bacterial composition across samples. In the future research, when the compositionality of the gut microbiome is addressed, GutBalance and DisBalance can be used to accurately predict the microbial biomarkers of the allergic diseases (Yang et al., 2021; Yang and Zou, 2021).
Conclusion
Taken together, this study supports the potential causal effects of gut microbial composition on allergies, including eczema, asthma, hay fever, and allergic rhinitis. Our research puts forward the hypothesis that Ruminococcaceae, Eggerthella, Bifidobacterium, Faecalibacterium, and Bacteroides might be potential risk factors for allergic diseases. In summary, our work can shed light on the comprehensive interactions between the host and the gut flora in allergic patients and provide new directions as well as novel strategies for the treatment, diagnosis, and early screening of atopic eczema, asthma, and related allergic diseases.
Data availability statement
The datasets presented in this study can be found in online repositories. The names of the repository/repositories and accession number(s) can be found in the article/Supplementary Material.
Author contributions
ST designed this study, obtained funding, recruit, supervised data analysis, provided guidance, and revised the manuscript. YW participated in the study design, collected data, performed two sample mendelian randomization study analysis, interpreted the results, and wrote the manuscript. TL and ZW assisted data collection and interpreted the results. JH and LW participated in the data analysis. LS revised the manuscript. All authors read and approved the final manuscript.
Funding
This work was made possible by General Research Fund from Research Grants Council of Hong Kong (Reference numbers: 475113, 14175617, 14119219, 14119420) and the Health and Medical Research Fund from Food and Health Bureau of Hong Kong (Reference numbers: 06171016, 07181266).
Conflict of interest
The authors declare that the research was conducted in the absence of any commercial or financial relationships that could be construed as a potential conflict of interest.
Publisher’s note
All claims expressed in this article are solely those of the authors and do not necessarily represent those of their affiliated organizations, or those of the publisher, the editors and the reviewers. Any product that may be evaluated in this article, or claim that may be made by its manufacturer, is not guaranteed or endorsed by the publisher.
Supplementary material
The Supplementary Material for this article can be found online at: https://www.frontiersin.org/articles/10.3389/fgene.2023.1153847/full#supplementary-material
References
Abrahamsson, T. R., Jakobsson, H. E., Andersson, A. F., Björkstén, B., Engstrand, L., and Jenmalm, M. C. (2012). Low diversity of the gut microbiota in infants with atopic eczema. J. Allergy Clin. Immunol. 129 (2), 434-40. doi:10.1016/j.jaci.2011.10.025
Akdis, C. A. (2006). Allergy and hypersensitivity: Mechanisms of allergic disease. Curr. Opin. Immunol. 18 (6), 718–726. doi:10.1016/j.coi.2006.09.016
Aldakheel, F. M. (2021). Allergic diseases: A comprehensive review on risk factors, immunological mechanisms, link with COVID-19, potential treatments, and role of allergen bioinformatics. Int. J. Environ. Res. Public Health 18 (22), 12105. doi:10.3390/ijerph182212105
Alexander, M., Ang, Q. Y., and Turnbaugh, P. J. (2019). A diet-dependent enzyme from the human gut microbiome promotes Th17 accumulation and colitis. bioRxiv, 766899. doi:10.1101/766899
Arrieta, M. C., Stiemsma, L. T., Dimitriu, P. A., Thorson, L., Russell, S., Yurist-Doutsch, S., et al. (2015). Early infancy microbial and metabolic alterations affect risk of childhood asthma. Sci. Transl. Med. 7 (307), 307ra152. doi:10.1126/scitranslmed.aab2271
Begley, L., Madapoosi, S., Opron, K., Ndum, O., Baptist, A., Rysso, K., et al. (2018). Gut microbiota relationships to lung function and adult asthma phenotype: A pilot study. BMJ open Respir. Res. 5 (1), e000324. doi:10.1136/bmjresp-2018-000324
Berni Canani, R., Paparo, L., Nocerino, R., Di Scala, C., Della Gatta, G., Maddalena, Y., et al. (2019). Gut microbiome as target for innovative strategies against food allergy. Front. Immunol. 10, 191. doi:10.3389/fimmu.2019.00191
Bowden, J., Davey Smith, G., and Burgess, S. (2015). Mendelian randomization with invalid instruments: Effect estimation and bias detection through egger regression. Int. J. Epidemiol. 44 (2), 512–525. doi:10.1093/ije/dyv080
Burgess, S., Butterworth, A., and Thompson, S. G. (2013). Mendelian randomization analysis with multiple genetic variants using summarized data. Genet. Epidemiol. 37 (7), 658–665. doi:10.1002/gepi.21758
Chen, J., Wright, K., Davis, J. M., Jeraldo, P., Marietta, E. V., Murray, J., et al. (2016). An expansion of rare lineage intestinal microbes characterizes rheumatoid arthritis. Genome Med. 8 (1), 43. doi:10.1186/s13073-016-0299-7
Galazzo, G., van Best, N., Bervoets, L., Dapaah, I. O., Savelkoul, P. H., Hornef, M. W., et al. (2020). Development of the microbiota and associations with birth mode, diet, and atopic disorders in a longitudinal analysis of stool samples, collected from infancy through early childhood. Gastroenterology 158 (6), 1584–1596. doi:10.1053/j.gastro.2020.01.024
Gomaa, E. Z. (2020). Human gut microbiota/microbiome in health and diseases: A review. Ant. Van Leeuwenhoek 113 (12), 2019–2040. doi:10.1007/s10482-020-01474-7
Goodrich, J. K., Davenport, E. R., Beaumont, M., Jackson, M. A., Knight, R., Ober, C., et al. (2016). Genetic determinants of the gut microbiome in UK twins. Cell. Host Microbe 19 (5), 731–743. doi:10.1016/j.chom.2016.04.017
Hemani, G., Zheng, J., Elsworth, B., Wade, K. H., Haberland, V., Baird, D., et al. (2018). The MR-Base platform supports systematic causal inference across the human phenome. Elife 7, e34408. doi:10.7554/eLife.34408
Lawlor, D. A., Harbord, R. M., Sterne, J. A., Timpson, N., and Davey Smith, G. (2008). Mendelian randomization: Using genes as instruments for making causal inferences in epidemiology. Stat. Med. 27 (8), 1133–1163. doi:10.1002/sim.3034
Pascal, M., Perez-Gordo, M., Caballero, T., Escribese, M. M., Lopez Longo, M. N., Luengo, O., et al. (2018). Microbiome and allergic diseases. Front. Immunol. 9, 1584. doi:10.3389/fimmu.2018.01584
Reddel, S., Del Chierico, F., Quagliariello, A., Giancristoforo, S., Vernocchi, P., Russo, A., et al. (2019). Gut microbiota profile in children affected by atopic dermatitis and evaluation of intestinal persistence of a probiotic mixture. Sci. Rep. 9 (1), 4996. doi:10.1038/s41598-019-41149-6
Sanna, S., van Zuydam, N. R., Mahajan, A., Kurilshikov, A., Vich Vila, A., Võsa, U., et al. (2019). Causal relationships among the gut microbiome, short-chain fatty acids and metabolic diseases. Nat. Genet. 51 (4), 600–605. doi:10.1038/s41588-019-0350-x
Schmidt, T. S. B., Raes, J., and Bork, P. (2018). The human gut microbiome: From association to modulation. Cell. 172 (6), 1198–1215. doi:10.1016/j.cell.2018.02.044
Stokholm, J., Blaser, M. J., Thorsen, J., Rasmussen, M. A., Waage, J., Vinding, R. K., et al. (2018). Maturation of the gut microbiome and risk of asthma in childhood. Nat. Commun. 9 (1), 141. doi:10.1038/s41467-017-02573-2
Su, Y.-J., Luo, S.-D., Hsu, C.-Y., and Kuo, H.-C. (2021). Differences in gut microbiota between allergic rhinitis, atopic dermatitis, and skin urticaria: A pilot study. Medicine 100 (9), e25091. doi:10.1097/MD.0000000000025091
Vael, C., Nelen, V., Verhulst, S. L., Goossens, H., and Desager, K. N. (2008). Early intestinal Bacteroides fragilis colonisation and development of asthma. BMC Pulm. Med. 8, 19. doi:10.1186/1471-2466-8-19
Veldhoen, M., Uyttenhove, C., Van Snick, J., Helmby, H., Westendorf, A., Buer, J., et al. (2008). Transforming growth factor-β'reprograms' the differentiation of T helper 2 cells and promotes an interleukin 9–producing subset. Nat. Immunol. 9 (12), 1341–1346. doi:10.1038/ni.1659
Wang, Q., Li, F., Liang, B., Liang, Y., Chen, S., Mo, X., et al. (2018). A metagenome-wide association study of gut microbiota in asthma in UK adults. BMC Microbiol. 18 (1), 114. doi:10.1186/s12866-018-1257-x
Wang, W., Luo, X., Zhang, Q., He, X., Zhang, Z., and Wang, X. (2020). Bifidobacterium infantis relieves allergic asthma in mice by regulating Th1/Th2. Med. Sci. Monit. Int. Med. J. Exp. Clin. Res. 26, e920583. doi:10.12659/MSM.920583
Yang, F., and Zou, Q. (2021). DisBalance: A platform to automatically build balance-based disease prediction models and discover microbial biomarkers from microbiome data. Briefings Bioinforma. 22 (5), bbab094. doi:10.1093/bib/bbab094
Yang, F., Zou, Q., and Gao, B. (2021). GutBalance: A server for the human gut microbiome-based disease prediction and biomarker discovery with compositionality addressed. Briefings Bioinforma. 22 (5), bbaa436. doi:10.1093/bib/bbaa436
Yang, Q., Lin, S. L., Kwok, M. K., Leung, G. M., and Schooling, C. M. (2018). The roles of 27 genera of human gut microbiota in ischemic heart disease, type 2 diabetes mellitus, and their risk factors: A mendelian randomization study. Am. J. Epidemiol. 187 (9), 1916–1922. doi:10.1093/aje/kwy096
Keywords: mendelian randomization, allergic disease, genetic varients, genetics, MWAS, gut microbiota (GM)
Citation: Wang Y, Liu T, Wan Z, Wang L, Hou J, Shi M and Tsui SKW (2023) Investigating causal relationships between the gut microbiota and allergic diseases: A mendelian randomization study. Front. Genet. 14:1153847. doi: 10.3389/fgene.2023.1153847
Received: 30 January 2023; Accepted: 28 March 2023;
Published: 12 April 2023.
Edited by:
Shitao Rao, Fujian Medical University, ChinaReviewed by:
Xuhui Zheng, University of Washington, United StatesFenglong Yang, Fujian Medical University, China
Copyright © 2023 Wang, Liu, Wan, Wang, Hou, Shi and Tsui. This is an open-access article distributed under the terms of the Creative Commons Attribution License (CC BY). The use, distribution or reproduction in other forums is permitted, provided the original author(s) and the copyright owner(s) are credited and that the original publication in this journal is cited, in accordance with accepted academic practice. No use, distribution or reproduction is permitted which does not comply with these terms.
*Correspondence: Stephen Kwok Wing Tsui, kwtsui@cuhk.edu.hk
†ORCID: Stephen Kwok Wing Tsui, orcid.org/0000-0003-0686-4259