- 1Department of Pharmacology and Toxicology, University of Toronto, Toronto, ON, Canada
- 2Department of Medical Genetics and Molecular Medicine, School of Medicine, Mashhad University of Medical Sciences, Mashhad, Iran
- 3Medical Genetics Research Center, Faculty of Medicine, Mashhad University of Medical Sciences, Mashhad, Iran
- 4Student Research Committee, Faculty of Medicine, Mashhad University of Medical Sciences, Mashhad, Iran
- 5Department of Pharmaceutical Biotechnology, School of Pharmacy, Guilan University of Medical Sciences, Rasht, Iran
- 6Laboratory of Pharmacogenomics, Department of Molecular Neuropharmacology, Maj Institute of Pharmacology Polish Academy of Sciences, Kraków, Poland
- 7Department of Pharmacy Practice and Science, University of Arizona R. Ken Coit College of Pharmacy, Tucson, AZ, United States
- 8Department of Biomedical Informatics, Vanderbilt University Medical Center, Nashville, TN, United States
Long-read sequencing (LRS) was introduced as the third generation of next-generation sequencing technologies with a high accuracy rate in genomic variant identification for some of its platforms. Due to the structural complexity of many pharmacogenes, the presence of rare variants, and the limitations of genotyping and short-read sequencing approaches in detecting pharmacovariants, LRS methods are likely to become increasingly utilized in the near future. In this review, we aim to provide a comprehensive discussion of current and future applications of long-read genotyping methods by introducing the opportunities and advantages as well as the challenges and disadvantages of state-of-the-art LRS platforms for the implementation of pharmacogenomic tests in clinical and research settings. New approaches to data processing, as well as the challenges and pitfalls of performing such tests in daily practice, will be explored in detail. We provide references to resources for those who are interested or intend to employ LRS in pharmacogenomics screening, both in clinical and research settings.
Introduction
The field of pharmacogenomics (PGx) is poised to integrate clinical genetic testing to predict the drug response into daily clinical practice (Borden et al., 2021). PGx testing is now available for many gene–drug interactions, which are now cataloged and curated in well-established databases (Barbarino et al., 2018; Cannon et al., 2024). PGx tests have the potential to enable drug-response stratification and implementation of personalized medicine across diverse populations. Many clinical and research centers are now considering PGx profiling in addition to existing genome screening and considering extraction of PGx markers out of exome or genome sequencing data (van der Lee et al., 2020).
Increased accuracy of genotyping approaches may add significant insights into treatment outcomes and prevent potential adverse drug-reactions (ADRs) (Golubenko et al., 2023). The PGx data interpretation workflow mainly relies on haplotype phasing, complex genomic region analysis for pharmacogenes, diplotype detection, allele imputation, and phenotype prediction. Although current genotyping methods mostly use array-based or short-read sequencing (SRS) technologies for single-nucleotide variant (SNV) detection within drug-related genes, more accurate approach(es) should be considered, especially for those genes with clear guidelines and actionable clinical recommendations.
Pharmacogenes contain highly polymorphic and/or homologous regions, repeated variants in the non-coding part of the genome, or rare structural variants (SVs), such as large insertions or deletions and segmental duplications or homopolymers (van der Lee et al., 2022). The advent of long-read sequencing (LRS) technologies, known as third-generation sequencing approaches, and their integration into PGx investigations highlight opportunities for resolving such variations (Table1). From providing unambiguous alignment of genomic regions to addressing challenges in achieving uniform coverage of GC-rich positions, LRS platforms can perform accurate genotyping in analytically challenging pharmacogenes without specific needs for DNA treatment, and they can perform full phasing and resolve complex diplotypes while decreasing the occurrence of false-negative results in a single assay (Caspar et al., 2018; Turner et al., 2023). Figure 1 displays the read length and coverage for some core pharmacogenes, decoded by LRS technologies in clinical PGx setting.
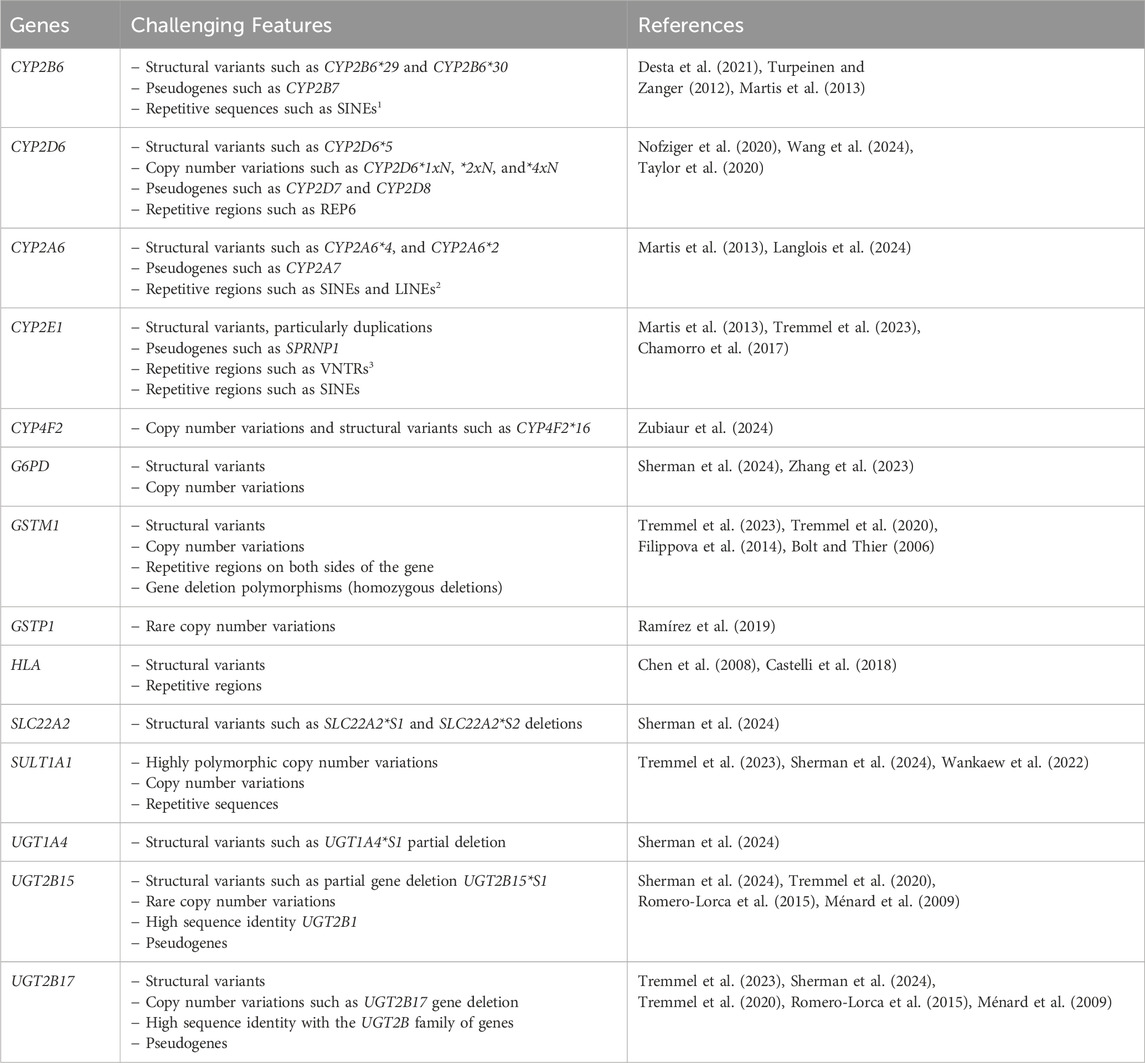
Table 1. Pharmacogenes and their particular characteristics that would take advantage of long-read sequencing.
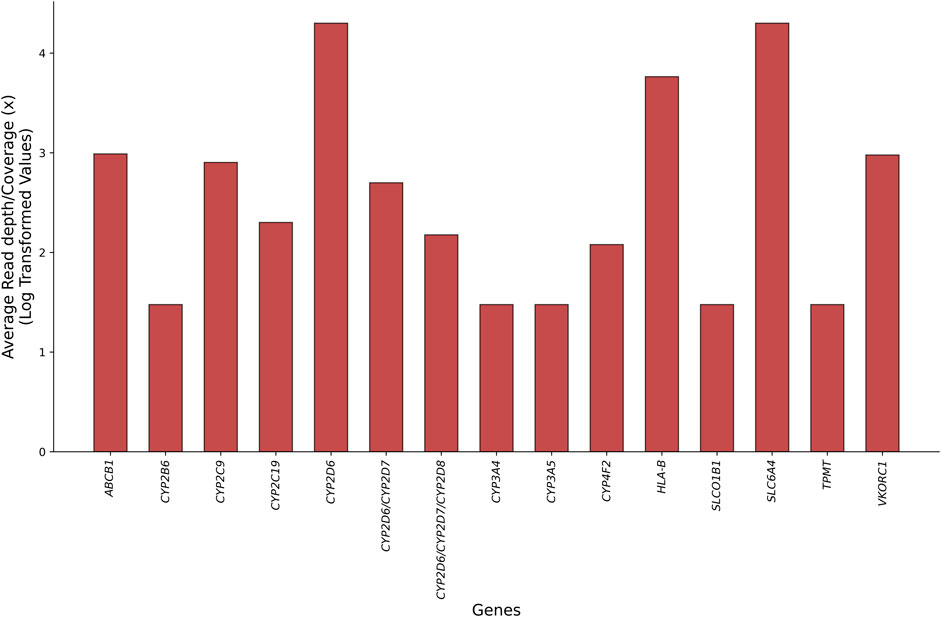
Figure 1. Comparison of average read-length distribution and coverage for core pharmacogenes sequenced by currently available long-read technologies within clinical pharmacogenomic tests to recall the ambiguous and novel genotypes (see the main text and Table 3 for references).
The aim of this review is to introduce and highlight the current and potential applications of LRS in PGx profiling of individuals through discussion of its advantages alongside challenges and limitations. While other literature works addressed LRS for PGx testing in a general view (Neu et al., 2023), this review explores applications of LRS in PGx in greater depth and provides more topic-specific contexts. We start with a brief overview on technologies and up-to-date workflows for data processing and pharmacovariant identification within the LRS output. Then, we take a broader look at the opportunities of applying LRS in PGx studies. Several real-world examples of using LRS for decoding complex core drug-related genes, such as CYP2D6 and HLA-B, are illustrated in detail as a proof of principle. Finally, potential challenges and existing limitations for utilization of the technologies are explored and discussed. We believe the article constitutes a valuable reference for those who are interested or intend to employ LRS in PGx studies, both in clinical and research settings.
Methods
A literature review was conducted to identify studies exploring the use of LRS technologies in PGx testing and clinical applications for articles published between 2010 and 2025. The search began with general studies on LRS technologies and their functionality, followed by a focused investigation using targeted search terms relevant to our review objectives and workflow. Comprehensive literature search: databases such as PubMed, Scopus, and Web of Science were searched, complemented by manual examination of reference lists from the selected publications. Search terms and combinations: [(“Long-read sequencing” OR “PacBio sequencing” OR “Nanopore sequencing” [Mesh]) AND “Pharmacogenomics” (Mesh)] AND (“Genetic Variation” [Mesh] OR “Drug Response Biomarkers, Genetics” [Mesh] OR “Drug Targeting” [Mesh] OR “Personalized Medicine” [Mesh]) [(“Long-read sequencing” OR “PacBio sequencing” OR “Nanopore sequencing”) AND (“CYP2B6” OR “CYP2C19”OR “CYP2C9” OR “CYP2D6” OR “CYP3A4” OR “CYP3A5” OR “DPYD” OR “SLCO1B1” OR “TPMT” OR “UGT1A1” OR “HLA” OR “VKORC1” OR “NAT1” OR “NUDT11”)]. For general terms, it was [(“Long-read sequencing” OR “PacBio sequencing” OR “Nanopore sequencing”) AND (“Genetic variation” OR “Polymorphism, genetic” OR “Genetic variation”) AND (“Gene identification” OR “Gene discovery”)]. Inclusion criteria included the following: a) studies investigating the application of LRS technologies (e.g., PacBio and Nanopore) in PGx; b) studies using LRS to analyze genetic variants associated with actionable genes, including drug response and drug target genes; c) studies evaluating clinical applications of LRS in PGx; d) studies decoding genetic variants in patients with adverse drug reaction-like clinical manifestations; e) publications in English with the full text available. Exclusion criteria included the following: a) studies not utilizing LRS for analyzing PGx-related genetic variants; b) studies focused on non-pharmacogenomic applications or unrelated diseases; c) publications not available in English or full text (e.g., conference abstracts); d) studies published before 2010, given the relatively recent development of the technology. Outcome measures included the following: a) studies demonstrating the application of LRS for decoding variants in actionable PGx genes and b) research using LRS to identify genetic variants in complex genomic regions, challenging to resolve with SRS or other standard assays. A total of 152 published articles met the inclusion criteria and were included in the current literature review.
Long-read sequencing (a brief overview of technology and data processing)
Third-generation next-generation sequencing platforms
With continuous development of sequencing technologies and decrease in LRS costs, applications will likely shift toward third-generation sequencing platforms due to their longer read length and the capability for using real-time single-molecule sequencing approaches. The ability to read long stretches of DNA without the need for amplification minimizes errors introduced during the amplification process (Athanasopoulou et al., 2021). Recent versions of LRS technologies have also improved error correction algorithms that reduce the inaccuracy. As a result, LRS has the potential to become an essential tool for various applications, including genome assembly, structural variant detection, epigenetic analysis, and PGx profiling (Amarasinghe et al., 2020a). Available platforms now directly produce uninterrupted sequences of native DNA, ranging from 10 kilobases to megabases in length with an accuracy up to 99.9% (Sequel II and PromethION) (Mantere et al., 2019; Pollard et al., 2018). In this review, we focus on two LRS technologies, Pacific Biosciences’ (PacBio) and Oxford Nanopore Technologies (ONT), which have been used extensively in genomic and PGx research. Table 2 provides an overview of these technologies, including the advantages and disadvantages specific to PGx profiling.
PacBio and ONT are LRS platforms that offer reads ranging from 50 kilobase pairs (kbp) to the current record of 2.3 million base pairs (Mb), respectively (Shendure and Ji, 2008). The accuracy of base-calling in both of these technologies has significantly improved in recent years, and it is now claimed that the raw base-called error rate has been lowered to <1% for PacBio and <5% for nanopore sequences (Payne et al., 2019). These error rates are computed by comparing the base calls generated by the sequencing platform to a known reference or consensus sequence. Various factors contribute to sequencing errors, including machine errors, chemistry limitations, and other technical challenges. Improvements in base-calling algorithms, sequencing chemistry, and overall technology advancements have collectively contributed to the reduction in these error rates over time (Amarasinghe et al., 2020a; Wenger et al., 2019).
The PacBio single-molecule real-time (SMRT) sequencing technology uses a circular DNA molecule template, referred to as SMRTbell, which has a unique topological structure. The SMRTbell is composed of a double-stranded DNA insert and single-stranded hairpin adapters located on both ends, facilitating effective and high-precision sequencing of DNA. The length of the DNA insert can range from 1 to 100 kilobases, making it possible to generate lengthy sequencing reads. After the successful assembly of the SMRTbell, a DNA polymerase binds to it before loading onto an SMRT Cell. The SMRT Cell, which houses up to 8 million zero-mode waveguides (see the mini glossary), is then utilized for sequencing (Kronenberg et al., 2018; Audano et al., 2019; Huddleston et al., 2017; Chaisson et al., 2015). Recently, PacBio developed two new platforms, named Revio and VEGA system, which promise highly advanced genome profiling approaches, such as direct detection of genome methylation in a very competitive turnaround time and/or a highly accurate LRS platform. ONT, on the other hand, enables the efficient and affordable analysis of extremely small amounts of DNA without manipulating cells (Ozsolak, 2012). While SMRT sequencers (RSII, Sequel, and Sequel II) recognize fluorescent signals, core nanopore sequencers (MinION, GridION, and PromethION) assess the variations in ionic current that arise as single-stranded nucleic acids traverse nanopores (Merker et al., 2018). A nanopore is a very small pore that is typically made of a solid material or protein embedded within a membrane and immersed in an electrolyte solution. The nucleic acid to be sequenced is passed through the nanopore, causing disruptions in the electrical current passing through the pores, and each base has a distinct effect on the current flow. These changes are recorded and analyzed. One advantage of nanopores is their portability for some sequencing devices (Stahl-Rommel et al., 2021; Burton et al., 2020; Castro-Wallace et al., 2017). Furthermore, DNA or RNA can be directly sequenced, whereas previous technologies required the synthesis of nucleic acids (Ozsolak, 2012; Marx, 2023). Recent efforts in ONT development have shifted their focus toward solid nanopores, with particular emphasis on materials like graphene and silicon. This shift is motivated by several factors, including the ability to achieve more precise control over the pore structure (Hall et al., 2010).
Both PacBio and ONT technologies are able to negotiate the most repetitive parts of the human genome. However, differences in their chemistry and sequence detection methods impact the length of their reads, the accuracy of the base pairs, and the amount of data they can produce (Kronenberg et al., 2018). Furthermore, the analysis of third-generation sequencing data necessitates use of specialized tools compared to second-generation platforms.
Bioinformatics of LRS technologies for PGx studies
Brief overview of PacBio and ONT data processing
The data generated by LRS platforms present unique challenges and opportunities for analysis. Routine workflow for LRS data analysis benefits from both common and LRS-specific tools, which may result in individual variant call format (VCF) or separate VCF files for different types of variants. On the other hand, mapping of long and sometimes noisy reads demands an accurate algorithm(s) of the seed-and-chain framework (see the mini glossary at the end of this article) for dealing with the initial 10%–20% error rates in some LRS reads. A valuable database is available to introduce all currently existing software designed for processing and analyzing LRS data, which is accessible through the web address https://long-read-tools.org/ (Amarasinghe et al., 2020a).
SMRT cells can be merged prior to assembly to achieve more coverage. PacBio comes with a basecall format referred to as bas.h5/bax.h5 (now POD5) or BAM file format as the primary output from SMRT instruments. To convert h5 or POD5 to Fastq files, several tools with different features have been developed, which may be used for multiple manipulations of PacBio data from basecalling to read alignment (Li, 2018). Other tools also have also been introduced recently and are available, outperforming in terms of sequence identity, the count of mapped bases, and runtime efficiency (Chaisson and Tesler, 2012; Hufnagel et al., 2020).
Previous ONT basecalling utilized an “official” state-of-the-art tool named Guppy for converting Fast5 format to Fastq. The tool was a neural network basecaller, which could also filter and remove low-quality reads, clip ONT adapters, and estimate the probability of methylation signatures for each base (Wick et al., 2019). However, recent advancements in nanopore sequencing include the transition to POD5 as the raw data storage format and Dorado as the default basecaller. These updates enhance data processing efficiency and accuracy. Dorado is a data processing toolkit that includes alignment, modified base detection, and barcode demultiplexing and normal basecalling. For modified bases, currently, it can identify 5mC, 5hmC, 4mC + 5mC, and 6mA for DNA and m6A and pseudouridine for RNA (https://nanoporetech.com/document/data-analysis; De Coster et al., 2018).
In addition to Dorado, which uses Minimap2 as a built-in algorithm, genome alignment also applies general standalone tools (Sedlazeck et al., 2018a). However, such tools have high computational needs.
Phasing, haplotype phasing, and haplotype/diplotype imputation
Phasing in PGx testing refers to the process of assigning individual alleles in a diploid individual to specific copies of a chromosome. It is an essential part of PGx data processing because it identifies whether genetic variants associated with the drug response are located on the same or different alleles (cis or trans). This is often crucial for understanding compound heterozygosity and predicting an individual’s drug response and potential adverse reactions accurately. Correct phasing ensures personalized and effective medication strategies, maximizing the benefits of PGx testing. Haplotype phasing is a crucial step in the identification of diplotype determination and subsequent phenotype prediction for a PGx test. It can be the decisive factor in distinguishing between a poor metabolizer (characterized by two loss-of-function variants located on distinct alleles) and an intermediate metabolizer (defined by having two loss-of-function variants on a single allele with no variants on the opposite allele). Hence, improved accuracy of haplotype phasing in PGx has the potential to improve phenotype determination (van der Lee et al., 2020). Currently, WhatsHap and HapCUT2 (by using sorted BAM files from Minimap2) are available for direct haplotype phasing of long-read WGS genotyping results. Genotype imputation and admixture analysis are also likely to benefit from improved phasing (Martin et al., 2016). The recently developed tool LRphase utilizes haplotype-resolved heterozygous variants, derived from various genomes including maternal and paternal genomes, in two different modes of scoring and matching to the observed genotype (Holmes et al., 2023).
PGx variant calling with LRS
We start this section with a summary of the tools applied for LRS variant calling as many of PGx variant callers accept the LRS VCF files as their input data for subsequent diplotype/phenotype prediction. The quality of the input data will have a significant impact on the accuracy for variant callers. Both PacBio and ONT require a minimum amount of coverage for read-based and/or assembly-based variant calling. However, the assembly-based method is preferred since the error correction step would be run during the genome assembly, thus reducing the false detection of SVs and making it a more appropriate choice for individualized sequencing of large and complex genomic regions (Lee et al., 2023).
SNVs and short insertion–deletions (InDels) in the LRS result can be identified by common callers or PacBio/ONT-related tools. Among the variant callers that were tested for LRS data from the Genome in a Bottle (GIAB) and HGSVC Freeze 4 resources (Helal et al., 2022; Byrska-Bishop et al., 2022), DeepVariant and Clair3 have been evaluated for PGx variant calling and consistently demonstrated superior performance and robustness (van der Lee et al., 2022; Barbitoff et al., 2022). For SV detection, Sniffles2 utilizes an innovative scoring system, which considers factors such as the position, size, type, and coverage of candidate SVs to exclude false calls. This approach effectively mitigates the high indel error rates in LRS results, ensuring precise detection of SVs in both germline and somatic variations in population-level analyses for PacBio and ONT read data (Sedlazeck et al., 2018a). Another common variant caller for LRS data is SVIM (pronounced swim), with the ability to mark and classify six types of SVs, namely, insertions, deletions, tandem duplications, interspersed duplications, inversions, and translocations. In addition, both PacBio and ONT platforms possess their own structural variant caller tools, identifying SVs within the related sequencing data. For instance, SMRTlink pbsv, which accepts pre-processed data named SvSIG, works with PacBio SMRT reads, while other tools such as Evince work with ONT reads (Bolognini and Magi, 2021).
Currently, ONT variant calling relies on NextFlow’s variant caller tool human variation workflow (epi2me-labs/wf-human-variation). It can perform basecalling of Fast5 and calling all types of variants in ONT reads, including SNVs, SVs, methylation signatures, CNVs, and short tandem repeats (STRs), simultaneously. The recently introduced tool NanoCaller uses deep learning and neural networks to call novel variants within complex genomic regions (Ahsan et al., 2021).
SV annotation databases like AnnotSV (https://www.lbgi.fr/AnnotSV/) may be utilized for the interpretation of potential pathogen SVs in human genome reads. The webserver identifies possible false-positive variants among all detected SVs and visualizes the range of variants present. It provides improved annotation from various sources, three output formats, innovative user interfaces, including an interactive Circos view, and SV scoring (0–1) based on human phenotype ontology (HPO) and Exomiser (Geoffroy et al., 2023). However, once the phased LRS-VCF files are ready, PGx-dedicated bioinformatic tools and algorithms with the capacity to accept LRS reads as the input data must be applied for accurate diplotype determination and subsequent phenotype prediction. The data may also be used to predict a PGx phenotype through the assignment of a “gene activity score.” This score is assessed by calculating the sum of individual activity scores assigned to each allele, and when there is more than one copy of an allele, the score is multiplied by the number of copies (Charnaud et al., 2022).
Numerous PGx tools are available for star allele calling using sequence data. Recently developed tools demonstrate promise in star allele calling and phenotype estimation in NGS data, including WGS (Tafazoli et al., 2022). Here, we provide a brief overview of the tools with the capability of processing LRS-VCFs for allele imputation and diplotype assignment in clinically important pharmacogenes. Aldy (version 4.0.1 and more) announced the adaptation with the LRS result in its latest update. While other tools such as Stargazer, Astrolabe, PharmCAT, StellarPGx, and Pnno do not support LRS reads natively, Aldy4 accepts different types of sequencing data, including LRS, using new phasing strategies and improved variant calling models. Tested by two PacBio HiFi datasets and compared with other tools, Aldy4 was found to demonstrate superior performance than other tools in the detection of the star alleles, except for a small number of CYP2D6 fusion and CNV alleles and one CYP2C9 call, where discrepancies were observed. However, finding and phasing novel unreported alleles within pharmacogenes like CYP2C19, CYP2B6, CYP3A4, DPYD, and SLCO1B1 is considered a major advantage for Aldy4 when applying for LRS variant calling (Hari et al., 2023).
Another computational algorithm with built-in compatibility for decoding LRS variants is Cyrius. The tool demonstrated its unique ability to call both SNVs and known SVs in CYP2D6, including *5, *13, *36, *68, and duplications. However, currently, Cyrius is only adapted for star allele calling within CYP2D6 and its pseudogene CYP2D7 (Chen et al., 2021). Other bioinformatic solutions such as Stargazer and PharmCAT may be used for LRS despite limitations for the identification of some distinct SV alleles (Lee et al., 2019). The standard pipeline must be provided phased input data, using BAM/CRAM (e.g., to create GDF for Stargazer), GVCF, and VCF files. The workflow itself may be modified by adding additional parameters (similar to “-S” in PharmCAT) when there are pooled multi-coverage samples. Merged VCF files also require separation in batches by tools like BCFtools. The Stargazer tool has now been superseded by PyPGx (Lee et al., 2022). This Python package works with both SRS and LRS alongside SNVs from SNP array data. Through a machine learning-based approach, PyPGx will predict star-allele diplotypes and phenotypes. This promised completely free and open-source package supports both the command line interface (CLI) and application programming interface (API) along with comprehensive and supportive documentations. Compatible with both the common genome builds (hg19 and hg38), PyPGx covers 87 pharmacogenes. The tool is highly appreciated and used by many reference centers and projects, as well as the genetic testing reference materials coordination program (GeT-RM), a program under the Centers for Disease Control and Prevention (CDC), and the 1000 Genomes Project (1KGP) (N = 2,504) (PyPGx documentation, accessed on 03-04-2025). Supplementary Table S1 provides details of currently available tools for converting raw LRS data into related VCFs and PGx annotated output.
Applications and opportunities
LRS has facilitated advances in genomic and PGx studies by unveiling previously concealed pharmacovariants within core pharmacogenes and genes associated with pharmacogenetic pathways. A brief description of the potential impact of this technology on PGx testing is given below, showcasing opportunities that can be obtained through its application in both clinical and research settings. Also, Figure 2 provides a quick overview on such applications in PGx setting.
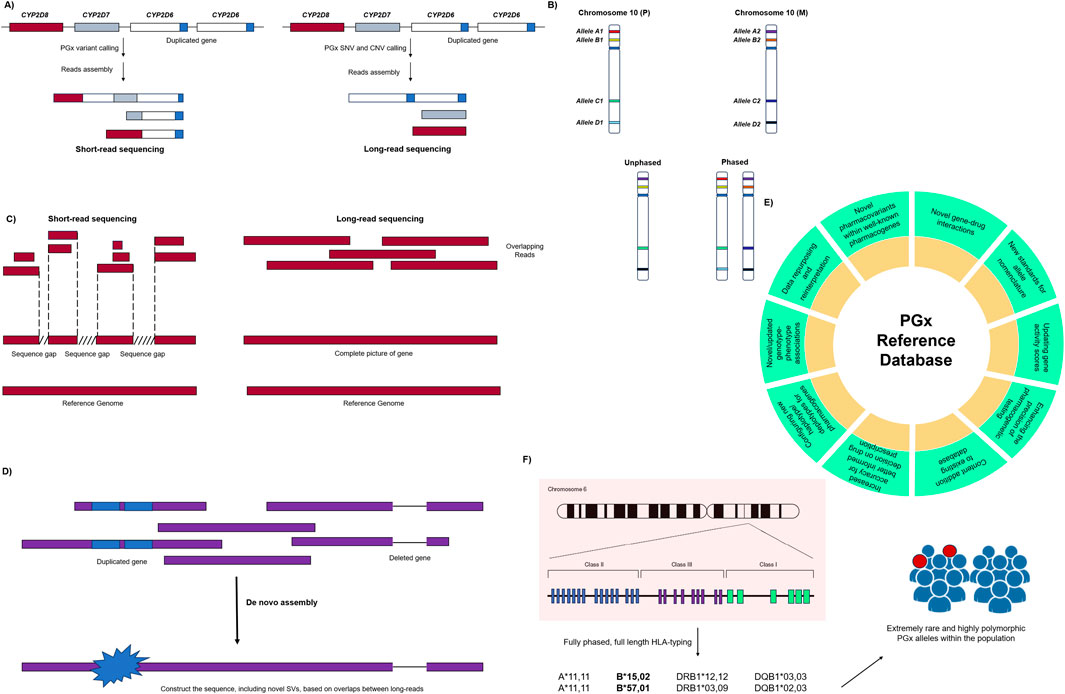
Figure 2. Main applications of long-read sequencing technologies in pharmacogenomic testing. (A) Decoding complex pharmacogenes: LRS may provide more accurate sequencing reads compared to the short-read sequencing result for the genomic region with high polymorphic and structural rearrangement. This leads to correct variant calls while dealing with CNVs and other SVs in core pharmacogenes. (B) Haplotype characterization and phasing: LRS makes the true allele decomposition, which may lead to the comprehensive picture of genome for target pharmacogenes. This includes assigning the right position of each allele on paternal/maternal chromosomes for better understanding of allele inheritance and haplotype definition. (C) Resolving ambiguous genotype calls: short-read sequencing may result in some ambiguity while trying to map the reads to the reference genome. This mostly happens when there are gaps between sequence reads, where there is no overlapping. On the other side, LRS reads have completely overlapped sequences, which makes them easier in both genome alignment and de novo assembly. (D) De novo genome assembly: rare or unique mutations and SVs could be covered successfully by LRS reads, which results in better genome assembly for individual PGx genes. (E) Update the PGx references: LRS can add multiple aspects for content creation and/or updating in PGx reference organizations and databases as well as PharmVAR, PharmGKB, etc. (F) Drug hypersensitivity discovery: extremely rare and highly polymorphic PGx alleles (including HLA genes) are now covered and deciphered by the utilization of custom-made LRS-dependent HLA-typing approaches. PGx: pharmacogenomics; SNV: single-nucleotide variant; CNV: copy number variation; SV: structural variant.
Complex genomic regions in pharmacogenes
Pharmacovariants in complex genomic regions are not always accurately detected by genotyping technologies that rely on either SRS or SNV arrays (van der Lee et al., 2020). LRS techniques have the ability to analyze complex PGx regions effectively (Wenger et al., 2019; Bowden et al., 2019; Midha et al., 2019). For example, LRS has successfully identified variants and haplotypes within complex pharmacogenes like CYP2D6 and HLA-A, which contain repeats or segmental duplications. LRS technologies also have advantages in haplotype phasing of variants within complex loci (van der Lee et al., 2022), de novo genome assembly (Cai et al., 2020), and transcriptome assembly (Tilgner et al., 2014).
Haplotype characterization and resolving uncertain haplotype phasing
While linkage disequilibrium (LD) assessment is utilized for PGx diplotype phasing and can yield accurate haplotype phasing at the population level, its accuracy at the individual scale is limited (van der Lee et al., 2022). Other tools for rapid and accurate statistical phasing of large sequencing datasets with an error rate of <5% have been developed (Hofmeister et al., 2023). Statistical phasing does not require supplementary experiments, but it is contingent upon the availability of representative datasets encompassing population genetic information. Despite demonstrating notable precision at a local level, the inherent limitation of statistical phasing becomes apparent in the form of multiple errors (referred to as “switch errors”), particularly at recombination hotspots. This occurs because software that uses LD only considers the co-inheritance of alleles that are closely located along the chromosome (Xu and Dixon, 2020).
LRS does not require pedigree or computational approaches, which makes haplotyping more straightforward and higher throughput, thus improving the accuracy of genotype imputation (van der Lee et al., 2022) and enabling more accurate PGx phenotype prediction (van der Lee et al., 2020). LRS has the potential to generate fragments of DNA sequences long enough to decode an entire pharmacogene in a single read, such as the 6.6-kb CYP2D6 gene (Zhou et al., 2022). LRS has been used to accurately identify the haplotype of complex genes such as HLA and CYP2D6 (Ammar et al., 2015; Liau et al., 2019a). PacBio HiFi long-reads have also been used for direct phasing and haplotype calling of the NUDT15 gene for more comprehensive diplotyping of star (*) alleles (Scott et al., 2022).
Resolving ambiguous genotype calls
LRS platforms also allow resolution of ambiguous and inconsistent calls that might be due to novel alleles and sub-allele calls (Gaedigk et al., 2022). Data obtained from 137 DNA samples recharacterized by employing the LRS technology and three genotype calling algorithms identified a previously unresolved CYP2C19 diplotype. The result decoded two novel sub-alleles, CYP2C19*35.002 and CYP2C19*2.011, which were previously assigned as CYP2C19*1 (*15)/*2 (indeterminate metabolizer) but have been updated to CYP2C19*2/*35 (poor metabolizer). The findings from LRS also indicated that CYP2C9*10 and *12 alleles are not located in a trans configuration, as previously thought, but rather in a cis arrangement, giving rise to a new and unique haplotype (Gaedigk et al., 2022).
Updating the existing knowledge and adding novel PGx reference alleles
LRS has the potential to detect novel variants and generate accurate reference alleles in diverse populations. A reference genome was created by integrating de novo assemblies of three Japanese individuals using LRS technologies (Takayama et al., 2021). LRS alongside SRS was utilized to obtain the genome of an individual from Saudi Arabia for creating the first draft of the genome based on de novo genome assembly, which was then refined (Kulmanov et al., 2022).
The Egyptian Genome Reference (EgyptRef) project was initiated in 2020 using a combination of long- and short-read sequencing technologies, including 10x Genomics, Illumina, and Pacific Biosciences (PacBio). In this project, the data obtained from 109 individuals demonstrated 1,198 Egyptian population-specific variants, including 49 novel ones (Ateia et al., 2023). Another study on Arabs recently investigated the genomes of 43 individuals from diverse Arab ethnicities using an ultra-long-read sequencing kit (ULK), high fidelity (HiFi), PacBio, and ONT. The study aimed to construct the Arab pan-genome reference and resulted in the detection of millions of base pairs of novel sequences (Uddin et al., 2023). In previous studies, the investigation of the genomes of diverse populations using mostly array-based technologies has resulted in novel PGx discoveries. For instance, in a study on the Spanish population, a significant number of potentially damaging variants that were not previously associated with PGx were identified (Nunez-Torres et al., 2023). Another study discovered a new link between a genetic variation in FPGS and reduced the need for warfarin dosage in the African American population (Giri et al., 2014). Moreover, the association between a novel non-function variant, NQO1*2, and resistance to warfarin was discovered in a Puerto Rican case (Hernandez-Suarez et al., 2016). Moving forward, LRS is likely to enhance the feasibility of detecting novel variants and may be used to update PGx references, especially in diverse populations that are not well-studied with respect to PGx (Kaye et al., 2017; Venner et al., 2024).
LRS revealed novel alleles in NUDT15, which were confirmed by Sanger sequencing and assigned as *1.007 and *20 by the PharmVar Consortium (Scott et al., 2022). SMRT sequencing has also led to characterization of new alleles and tandem arrangement, configuring diplotypes like CYP2D6*36+*41, facilitating prediction of a metabolizer phenotype and rate of drug response (Qiao et al., 2016). Discovering novel haplotypes, alleles, and sub-alleles through LRS, once confirmed and clinically validated, can accelerate the updating of PGx references. In addition, such technologies may offer a more rapid time-to-result approach for a PGx or other targeted tests. A nanopore sequencing-based approach led to reduced turnaround time from approximately 7 days to 24 h (Oehler et al., 2023). This rapid integration of verified genetic information has the potential to enable healthcare professionals to make more informed decisions about drug prescriptions and dosages with more speed and accuracy.
De novo genome assembly in personalized medicine
The capability of LRS in providing de novo assemblies is especially valuable in PGx, where it allows for the identification of individual-specific genetic variation within pharmacogenes. This variation can be critical in understanding an individual’s response to drugs and predicting adverse reactions or treatment efficacy. An extensive example of population-based, LRS-aided de novo genome assembly was provided by the Seo group in the Korean population. A total of 18,210 SVs were identified by direct comparison of their assembly with the human reference, which led to the identification of thousands of previously unreported breakpoints. Asian-specific SVs were decoded, and high-quality haplotyping of clinically relevant alleles was provided alongside successful characterization of HLA and CYP2D6 regions (Seo et al., 2016).
Long-read sequencing advantages in decoding PGx genes (proofs of principle)
LRS technologies are now widely used in locus characterization and for improving phenotype prediction in PGx investigations (Deserranno et al., 2023). Such studies successfully mitigated the ambiguity associated with challenging genotypes and missing heritability, resulting in conclusive findings. In this context, in addition to providing detailed examples of the application of LRS, focusing on the very important pharmacogenes CYP2D6 and HLA as proof of concept, Table 3 provides a summary of recent studies focusing on utilization of LRS technologies in clinical PGx testing.
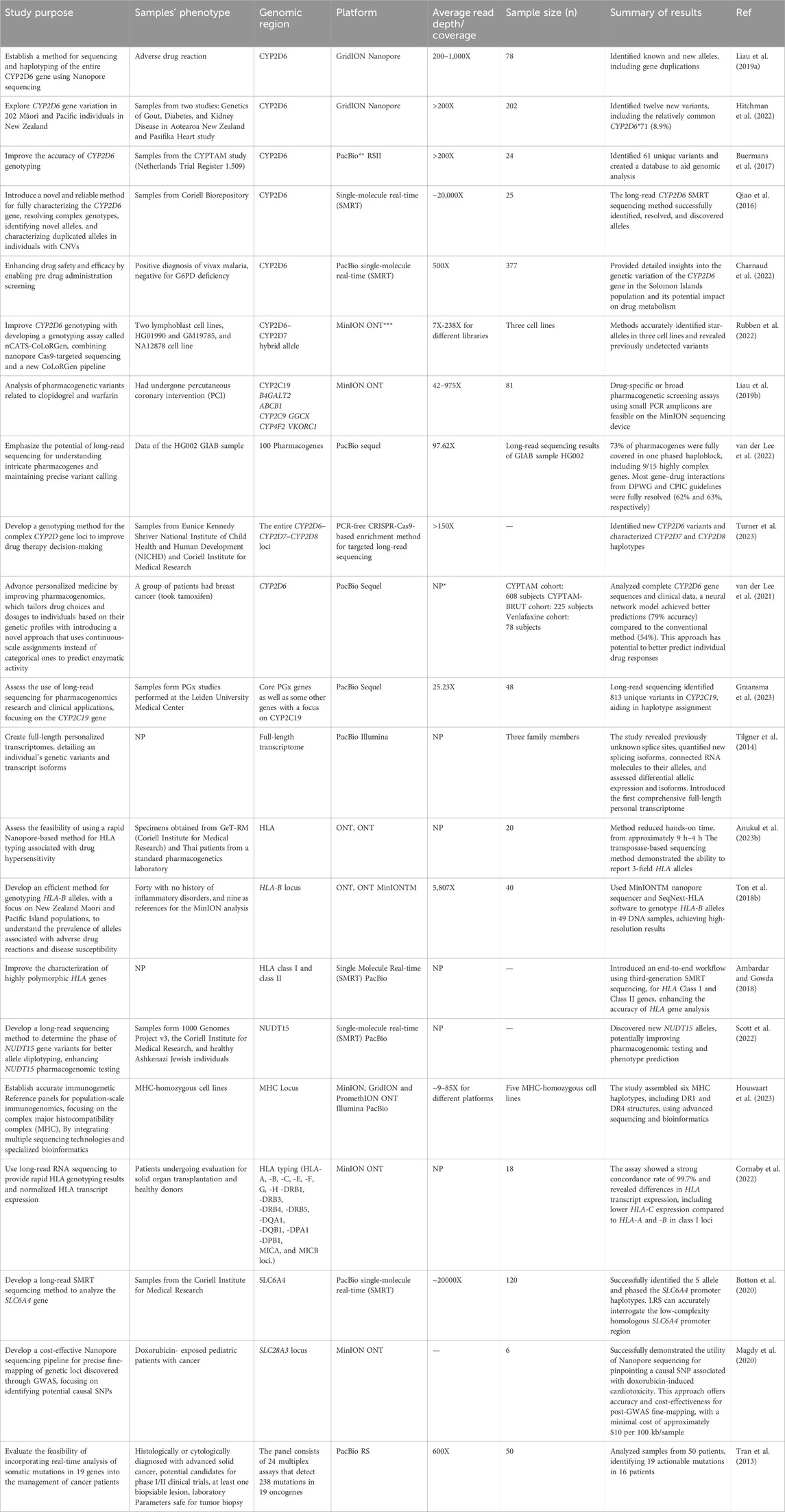
Table 3. Summary of studies with successful utilization of long-read sequencing technologies in clinical pharmacogenomics.
Long-read technologies and CYP2D6
With more than 170 frequently updating listed star (*) alleles, the cytochrome P450 2D6 (CYP2D6) gene is considered highly polymorphic. Recombination events generate gene deletions, duplications, and conversions, and the CYP2D6 pseudogene CYP2D7 also impacts the CYP2D6 structure (Kramer et al., 2009a; Kramer et al., 2009b). Hence, there is a high level of hybrid alleles and CNVs with clinical consequences in diverse populations. Furthermore, when a higher copy number is found, identification of duplicated alleles is required for reliable prediction of the CYP2D6 metabolizer status. In the SUPER-Finland study, the importance of identification of CYP2D6 copy number is highlighted in a large population with psychiatric disorders. A higher frequency of CYP2D6 ultrarapid metabolizers and a substantial enrichment of specific variants were observed within the Finnish population (Häkkinen et al., 2022). The potential benefit of long-read CYP2D6 sequencing is also indicated by the ability to reduce multiple alignments in single-end reads larger than 3 kb (Yang et al., 2017).
Hitchman et al. utilized nanopore sequencing for CYP2D6 alterations in 202 New Zealanders and reported 12 novel variants in this population (Hitchman et al., 2022). Buermans et al. introduced PacBio’s RSII with a two-step barcoding approach as a high-quality and cost-efficient assay for full-length CYP2D6 sequencing and haplotyping. They reported 61 unique alterations in CYP2D6 (Buermans et al., 2017). In a Solomon Islands population, the “PLASTER” (Phased Long Allele Sequence Typing with Error Removal) pipeline identified one of eight * alleles in 95.3% of patients and seven new * alleles in 4.7% patients, using long-read SMRT sequencing (Charnaud et al., 2022). Nanopore sequencing has also been used for high throughput and accurate haplotyping and identifying novel alterations and CNVs in challenging pharmacogenes like CYP2D6. A whole gene deletion (*5) along with 2484G>T (*33) variants were observed in one patient and confirmed on Sanger sequencing (*5/*33), resulting in an intermediate metabolizer phenotype. Furthermore, five novel rare variants have been found, including *17.003 (*17 allele with a 653C>T intronic variant), *41.004 (two variants of 1378C>G and 5814C>T in intron 2 and exon 3, respectively), *4.026 (contained 1404C>T in intron 2), and *1.025 (−365G>A) (Liau et al., 2019a). Rubben et al. introduced a new PCR-free nanopore Cas9-targeted sequencing (nCATS) for genotyping of allele-specific sequences of complex regions and a new extensive LRS (CoLoRGen) pipeline. These assays are efficient and accurate methods for determination of the complete CYP2D6–CYP2D7 sequence (Rubben et al., 2022). Ammar et al. demonstrated that MinION nanopore sequencer-based LRS technology can identify both alterations and haplotypes of key pharmacogenes including HLA-A, HLA-B, and CYP2D6. Because of the non-functional CYP2D6 enzyme associated with *3 and *4 alleles, decreased metabolism of codeine and olanzapine has been observed (Ammar et al., 2015). Several sub-alleles have been found in CYP2D6, which may cause misalignments in short-read NGS data. For example, rs61736524 (G>A) in exon 4 of CYP2D8 adjusts rs748851484 (G>A) in exon 4 of CYP2D6. To handle CYP2D6 and the related pseudogenes, single-molecule LRS has been used for the entire CYP2D6–CYP2D7–CYP2D8 locus, indicating that it could overcome the limitation and challenges of other genotyping assays like short-read NGS and SNP-arrays (10). Ten novel CYP2D6 haplotypes, including *128 to *137 alleles, were identified by a targeted long-read panel (PKSeq) and confirmed in vitro metabolic studies in a Japanese population and suggested to be nonfunctional alleles. In addition, the *135/*136 diplotype was described as a reduced function phenotype. The alleles *129, *134, *135, *136, and *137 all have the potential to create a reduced functional CYP2D6 phenotype through the decreased CYP2D6 activities (Fukunaga et al., 2021).
Long-read technologies and HLA genes
Variation in human leukocyte antigen (HLA) genes is considered a strong marker for multiple drug hypersensitivity reactions (Karnes et al., 2019). HLA typing at a high resolution can be clinically useful in predicting severe cutaneous adverse drug reactions to certain drugs (Fan et al., 2017). Hypersensitivity to abacavir and carbamazepine is associated with the carriage of HLA-B*57:01 (Illing et al., 2018) and HLA-B*15:02, respectively (Leckband et al., 2013). HLA-B typing can aid healthcare providers in selecting suitable drugs and mitigating the risk of adverse drug effects (Matern et al., 2020).
HLA typing methods have shifted from serologic testing to DNA sequencing. Because of extreme polymorphic zones and uncommon population-specific variation, it is challenging to choose an effective sequencing strategy. Recently, LRS was validated for HLA by Benedict et al. They reported a method for sequencing and typing of full-length HLA class-I molecules using the Oxford Nanopore MinION and the implementation of this method in a routine diagnostic setting (Matern et al., 2020). Ultrarapid and high-resolution HLA class-I typing using transposase-based nanopore sequencing was also applied in PGx testing by other groups, its potential for conducting testing that is independent of race and population was demonstrated, while significantly reducing both time and costs (Anukul et al., 2023a). However, the accuracy of typing results could be impacted by an imbalance in the PCR amplification of distinct haplotypes (Anukul et al., 2023a). Ton et al. outlined a method for HLA-B typing that combines data analysis with the SeqNext-HLA software package with NGS on the MinION™ nanopore sequencer. Alleles within this specific gene are described as crucial risk elements for hypersensitivity reactions triggered by drug intake. Researchers illustrated effective haplotyping facilitated by the nanopore sequencer’s long-read capacity and single-molecule reads. The HLA-B diversity in Pacific Islander and Maori population is not well-characterized, so this approach was utilized in both of these populations as well as reference samples (Ton et al., 2018a).
The Pac-Bio SMRT sequencing technology yields fully phased, clear, allele-level data for full-Length HLA typing (Ambardar and Gowda, 2018). Given the time limits for allocating deceased organ donors, a test that delivers both rapid allele-specific transcript quantitation and high-resolution HLA typing with LRS technologies is ideal. Overall, HLA typing with the accuracy of 99.68% is demonstrated by this method (Cornaby et al., 2022).
Long-read technologies and other pharmacogenes
Other PGx regions have been phased into haploblocks, mediated by long-read PacBio sequencing. The total CYP2D6 locus in one long read was covered using PacBio, and variants in other genes like rs776746 (6981A>G) of CYP3A5 and 1173 TT genotype (homozygous for 6484C>T) in VKORC1 lead to poor metabolizer and decreased activity phenotypes, respectively, and can be identified with LRS technologies (van der Lee et al., 2022). Other studies demonstrated a higher rate of on-target mapped nucleotides in pharmacogenes, which was seen in nanopore sequencing compared to Illumina, resulting in the longer length of sequencing (e.g., in CYP2C19 and CYP2D6) (Tilleman et al., 2022). Graansma et al. identified 813 unique variants in 37 samples and showed that the package of LRS and PGx-dedicated bioinformatics tools (Aldy, PharmCAT, and PharmKU) can recognize CYP2C19 variation and new haplotypes (Graansma et al., 2023). LRS affords high coverage of regions within these genes and addresses the high level of heterozygosity in individuals (Liau et al., 2019b).
NUDT15 encodes a Nudix hydrolase enzyme that is a negative regulator of thiopurine activation and toxicity. Mutations in this gene may lead to poor metabolism of thiopurines. In contrast to short-read genome sequencing, which was unable to phase star (*) allele-defining NUDT15 variants, long-read HiFi sequencing phased all variants across the NUDT15 amplicons, including the *2/*9 diplotype. This diplotype is interpreted as a possible poor metabolizer, which may pose the patient to high risk for toxicity with normal doses of thiopurines. As a result, this method was considered an innovative platform for allele discovery and new full gene haplotyping (Scott et al., 2022). Other examples include the serotonin (5-HT) transporter (5-HTT), produced by the SLC6A4 gene, which regulates 5-HT reuptake and is known as the major target of selective serotonin reuptake inhibitor (SSRI) antidepressants. The promoter of SLC6A4 consists of a variable number of homologous 20–24bp repeats (5-HTTLPR) and has rare population-specific alterations. A long-read SMRT sequencing method can provide an efficient technology for the investigation of the SLC6A4 promoter region that could not be determined using high-depth short-read capture-based sequencing (∼330X) (Botton et al., 2020). Solute carrier family 28 member 3, SLC28A3, impacts neurotransmission and nucleoside drug metabolism and transport. Recently, nanopore long-range PCR-based target enrichment for SLC28A3 amplicons through fine-mapping of a genome-wide association study (GWAS) revealed an association between an SNP (rs7853758G>A, L461L) in SLC28A3 and lower risk of cardiotoxicity in patients treated with doxorubicin. This investigation also provides a proof of principle for the application of LRS in pinpointing potential causal SNPs that could be used to optimize pharmacotherapy (Magdy et al., 2020).
Challenges and limitations in utilization of long-read sequencing for clinical practice and pharmacogenomic testing
Current LRS technologies have several limitations in both infrastructure and data production and/or management. At present, LRS instruments and reagents are more expensive, and it is not a cost-effective approach for many test centers (Amarasinghe et al., 2020b). Library preparation requires fresh material or intact cells, and there is a need for improvements in DNA isolation protocols and handling of ultra-long high-molecular weight DNA (Mantere et al., 2019). Obtaining high-quality DNA samples can be a challenging aspect of LRS, especially when dealing with specific sample sources such as degraded or low-yield samples. In addition, formation of PCR chimeras during PCR amplification and biases in reference alignment are challenges that must be taken into account when trying to phase variants with amplicon-based LRS technologies (Laver et al., 2016). An example of this issue is demonstrated by Ammar et al., who determined the HLA-A and HLA-B haplotypes in a manner similar to the CYP2D6 haplotypes, utilizing predefined markers from the HapMap project. Taking into account the errors in MinION sequencing and allowing for a single mismatch in the haplotype, the investigated diplotype for those genes revealed PCR bias during amplification, which likely influenced the relative proportion of haplotypes. Owing to the high error rates of nanopore reads, the identified HLA alleles did not match those found using HapMap data (Ammar et al., 2015).
Recent advancements in some platforms like PacBio HiFi produce 99.9% accuracy in long DNA sequencing reads and significantly decreased error rates (Hotaling et al., 2023). However, in general, these technologies have a higher rate of sequencing errors, ranging from 5% to 20%, compared to short-read next-generation sequencing (SR-NGS) outcomes, which typically have an error rate of less than 1% (Wenger et al., 2019; Goodwin et al., 2016). LRS errors are generally randomly distributed. These issues have the potential to affect the quality of assembled sequences and the accuracy of variant calling (Amarasinghe et al., 2020a).
Analyzing LRS data presents a significant computational challenge. Specialized bioinformatics tools and expertise are frequently essential for tasks such as error correction, alignment, and de novo assembly. These processes can be resource-intensive and demand substantial computational power and expertise (Ozsolak, 2012). Managing and archiving these large volumes of data can pose logistical challenges, especially for long-term projects that require data preservation and accessibility over extended periods (De Coster et al., 2021). Reference genomes that currently exist are primarily constructed using SRS data. When long reads are aligned to these reference genomes, it is important to be aware that biases can emerge, potentially resulting in misalignments and errors in variant calling (Sedlazeck et al., 2018b).
Achieving sufficient coverage depth and accurate de novo assembly could be considered an emerging challenge for LRS utilization in PGx studies. Although LRS shows unprecedented ability for uncovering genomic base modifications as well as DNA methylation, accurately pinpointing the precise location and type of these modifications presents another challenge. Specialized analysis methods are typically necessary to achieve accurate detection and characterization of base modifications through DNA samples (Eid et al., 2009; Johannesen et al., 2023). Finally, it is crucial to evaluate the accuracy and reliability of the results from long-read sequencing technologies, especially in clinical practice. Common orthogonal methods to validate findings from long-read sequencing include Sanger sequencing and quantitative RT-PCR, which are typically conducted to validate results in the proband and their family. These methods are also utilized to validate the results and refine the exact position of breakpoint junctions. Meanwhile, an automated quality control tool named LongQC is designed specifically for genomic datasets produced by third-generation sequencing technologies, including ONT and SMRT sequencing from PacBio, and it supports all major LRS platforms. Moreover, structural variant visualization tools, such as Ribbon, facilitate easy manual validation of SVs, allowing researchers to verify findings without relying solely on SV calling tools (https://v2.genomeribbon.com/) (see the Supplementary Table S2 for more details).
Future trends and conclusion
Despite the existing challenges and limitations, integration of LRS technologies into PGx testing is continually evolving, resulting in new possibilities for individualized treatment. Access to such technologies is increasing as an outsource point for many clinical and academic sections through the service providers, offering PacBio and ONT sequencing facilities and data analysis. Supplementary Table S3 introduces many of reliable and well-known centers, offering collaborations in this field. Future LRS-based PGx studies are likely to benefit from personal genome assembly, rapid decoding of novel and rare/underrepresented variants within core pharmacogenes, comparing ancestrally diverse samples, patient-specific primer design and optimization for challenging pharmacogenes, detecting and phasing de novo CNV and SV haplotypes, and resolution of previously ambiguous bases (Pirmohamed, 2023; Giardina and Zampatti, 2022). Fourth-generation (4G) sequencing machines with the ability for real-time sequencing of nucleotides in the fixed cells and tissues were also introduced through integration of nanopore sequencing into the single-molecule sequencing method. Advantages include the broader look into the arrangement of DNA reads across the sample, offering valuable insights into tissue heterogeneity using known markers. Moreover, the technology may lead to an ultrafast scan of the whole genome in 15 min (Pervez et al., 2022).
Such advancements are expected to be introduced into PGx testing as well. Current applications of LRS in stratified medicine utilizes drug-/treatment-related PGx panel for long-read technologies in order to find true gene–drug interactions in individuals. As the technology moves forward, more LRS-WGS will likely be performed as the predominant method for PGx profiling. The outcome may help in faster PGx implementation in clinical decision and drug stratification. Pre-emptive genotyping strategies may facilitate improvement of drug safety and efficacy, while reducing hospitalization and improving cost effectiveness. Other factors as well as sex, age, co-morbidity, drug–drug interactions, and organ functionality contribute significantly in overall drug response variability within individuals, yet genetic variation may constitute a crucial influence on those differences (Caspar et al., 2021). Comprehensive PGx marker screening and digitalization of the result for convenient and widespread access by healthcare professionals may facilitate utilization of the data for prescription modifications. To achieve this, the complexities in genome structure and function will need to be addressed by more advanced genotyping platforms. LRS technologies are promising tools to reach these goals through advancement in introducing highly accurate platforms (e.g., HIFI technologies) and compound bioinformatic algorithms to bring more precise genotype–phenotype correlations, aiding in PGx clinical evidence generation. This precision is particularly vital in modern pharmacology, where LRS can help guide therapeutic decisions, ensuring that patients receive medications tailored to their genetic makeup, maximizing both safety and efficacy.
Mini glossary
Complex genomic regions: genomic regions with intricate structural variations, making them challenging to analyze due to tandem repeats, duplications, deletions, and rearrangements. In addition, they often involve multiple genes/pseudogenes and regulatory elements, leading to complex phenotypic traits.
Circular consensus sequencing: a method that generates highly accurate DNA sequences by repeatedly reading circularized DNA fragments, reducing sequencing errors. Sequencing of the same DNA fragment in a circular manner, ensuring consensus on the base calls.
Diplotype: the combination of two distinct haplotypes for specific genes or genomic regions in an individual’s genome. The specific pair of alleles present at two linked loci on homologous chromosomes in a diploid organism, representing a combination of two distinct haplotypes.
Fine mapping: a detailed genetic mapping approach to pinpoint the precise location of a gene or variant associated with a specific trait or disease. It is a refined mapping approach that aims to identify the exact genetic variants contributing to a specific phenotype by narrowing down the location within a previously identified genetic region.
Fourth-generation of sequencing: cutting-edge sequencing technologies that surpass previous generations, often characterized by higher throughput, lower costs, and improved accuracy. The advanced sequencing methods that utilize innovative approaches, such as nanopore sequencing or single-molecule sequencing, characterized by improved speed, accuracy, and scalability.
Gene activity score: a numerical measure, often derived from high-throughput sequencing data, quantifying the level of gene expression or activity in a specific biological context, mostly used in genomic research.
Gene conversion: the process where the genetic material is transferred from one DNA molecule to another, leading to the exchange of genetic information. A recombination process where one allele replaces a homologous allele, leading to the transfer of genetic material between non-identical genes.
Genetic mapping: the process of determining the relative positions of genes or other genetic markers on a chromosome and establishing the distances between them. Genetic mapping can be done through various techniques, such as linkage analysis in families, association studies in populations, or physical mapping using DNA sequencing methods.
Genome-wide association studies: large-scale investigations exploring genetic variations across the entire genome to identify statistically significant associations between specific genetic markers and complex traits or diseases.
Genomic VCF (GVCF): genomic variant call format, a standard file format storing genetic variations (SNPs, indels, etc.) with associated metadata, commonly used in genomics research.
Haplotype: a set of genetic variations, or alleles, on a chromosome that are inherited together from a single parent due to low recombination frequencies.
Haploblock: a genomic region where genetic variations are inherited together due to low recombination rates, often used in association studies to identify linked variants. A contiguous genomic region with limited historical recombination, containing a set of closely linked genetic variants.
Hybrid gene: a gene formed by the fusion of sequences from two different genes, resulting from chromosomal rearrangements or translocations, leading to a novel genetic sequence with potential functional implications.
Long-read sequencing: sequencing technologies capable of reading longer DNA fragments, enabling the assembly of complex genomes, allowing for the analysis of complex genomic regions and the detection of structural variations.
Multiallelic CNV: copy number variants (CNVs) involving multiple alleles, indicating variations in the number of copies of a specific genomic segment among individuals.
Seed-and-chain framework: a computational algorithm used in sequence alignment, where short, exact matches (seeds) between sequences are identified and extended into longer alignments (chains) to detect similarities and differences between biological sequences, commonly used in genomics for sequence comparison and analysis.
Star allele: a specific variant of a gene associated with pharmacogenetic traits, often denoted with an asterisk (*), indicating distinct allelic forms that influence an individual’s response to drugs or other substances.
Zero-mode waveguides: Zero-mode waveguides (ZMWs) are nanoscale observation chambers used in single-molecule real-time (SMRT) sequencing technologies. These tiny wells confine the sequencing reaction to a volume so small that only a single DNA polymerase molecule and its associated template DNA strand can fit. This isolation enables real-time observation of the DNA synthesis process at the single-molecule level, contributing to the accuracy and efficiency of the sequencing method.
Author contributions
AT: conceptualization, data curation, investigation, methodology, project administration, software, visualization, writing – original draft, and writing – review and editing. MH: data curation, investigation, and writing – original draft. MR: data curation, investigation, and writing – original draft. MA: data curation, investigation, and writing – original draft. FK: data curation, investigation, and writing – original draft. MK: writing – review and editing. JK: conceptualization, funding acquisition, project administration, resources, supervision, and writing – review and editing.
Funding
The author(s) declare that financial support was received for the research and/or publication of this article. All APCs are covered by the Department of Pharmacy Practice and Science, University of Arizona (Dr. Jason Karnes).
Conflict of interest
The authors declare that the research was conducted in the absence of any commercial or financial relationships that could be construed as a potential conflict of interest.
The author(s) declared that they were an editorial board member of Frontiers, at the time of submission. This had no impact on the peer review process and the final decision.
Publisher’s note
All claims expressed in this article are solely those of the authors and do not necessarily represent those of their affiliated organizations, or those of the publisher, the editors and the reviewers. Any product that may be evaluated in this article, or claim that may be made by its manufacturer, is not guaranteed or endorsed by the publisher.
Supplementary material
The Supplementary Material for this article can be found online at: https://www.frontiersin.org/articles/10.3389/fgene.2025.1435416/full#supplementary-material
Abbreviations
4G sequencing, fourth-generation of sequencing; API, application programming interface; BAM, binary alignment mapped; CCS, circular consensus sequencing; CLI, command line interface; CNV, copy number variation; CRAM, compressed reference-oriented alignment map; GDF, genotype data file; GIAB, Genome in a Bottle; GVCF, genomic VCF; LD, linkage disequilibrium; LRS, long-read sequencing; NGS, next-generation sequencing; SMRT, single-molecule real-time; SNP, single-nucleotide polymorphism; SNVs, single-nucleotide variants; SRS, short-read sequencing; SVs, structural variants; VCF, variant call format.
Footnotes
1Short interspersed elements
2Long interspersed elements
3Variable number tandem repeats
References
Ahsan, M. U., Liu, Q., Fang, L., and Wang, K. (2021). NanoCaller for accurate detection of SNPs and indels in difficult-to-map regions from long-read sequencing by haplotype-aware deep neural networks. Genome Biol. 22, 261–333. doi:10.1186/s13059-021-02472-2
Amarasinghe, S. L., Su, S., Dong, X., Zappia, L., Ritchie, M. E., and Gouil, Q. (2020a). Opportunities and challenges in long-read sequencing data analysis. Genome Biol. 21, 30–16. doi:10.1186/s13059-020-1935-5
Amarasinghe, S. L., Su, S., Dong, X., Zappia, L., Ritchie, M. E., and Gouil, Q. (2020b). Opportunities and challenges in long-read sequencing data analysis. Genome Biol. 21, 30. doi:10.1186/s13059-020-1935-5
Ambardar, S., and Gowda, M. (2018). High-resolution full-length HLA typing method using third generation (Pac-Bio SMRT) sequencing technology. HLA Typing Methods Protoc. 1802, 135–153. doi:10.1007/978-1-4939-8546-3_9
Ammar, R., Paton, T. A., Torti, D., Shlien, A., and Bader, G. D. (2015). Long read nanopore sequencing for detection of HLA and CYP2D6 variants and haplotypes. F1000 Research 4, 17. doi:10.12688/f1000research.6037.1
Anukul, N., Jenjaroenpun, P., Sirikul, C., Wankaew, N., Nimsamer, P., Roothumnong, E., et al. (2023a). Ultrarapid and high-resolution HLA class I typing using transposase-based nanopore sequencing applied in pharmacogenetic testing. Front. Genet. 14, 1213457. doi:10.3389/fgene.2023.1213457
Anukul, N., Jenjaroenpun, P., Sirikul, C., Wankaew, N., Nimsamer, P., Roothumnong, E., et al. (2023b). Ultrarapid and high-resolution HLA class I typing using transposase-based nanopore sequencing applied in pharmacogenetic testing. Front. Genet. 14, 1213457. doi:10.3389/fgene.2023.1213457
Ateia, H., Ogrodzki, P., Wilson, H. V., Ganesan, S., Halwani, R., Koshy, A., et al. (2023). Population genome programs across the Middle East and north africa: successes, challenges, and future directions. Biomed. Hub. 8, 60–71. doi:10.1159/000530619
Athanasopoulou, K., Boti, M. A., Adamopoulos, P. G., Skourou, P. C., and Scorilas, A. (2021). Third-generation sequencing: the spearhead towards the radical transformation of modern genomics. Life 12, 30. doi:10.3390/life12010030
Audano, P. A., Sulovari, A., Graves-Lindsay, T. A., Cantsilieris, S., Sorensen, M., Welch, A. E., et al. (2019). Characterizing the major structural variant alleles of the human genome. Cell 176, 663–675. doi:10.1016/j.cell.2018.12.019
Barbarino, J. M., Whirl-Carrillo, M., Altman, R. B., and Klein, T. E. (2018). PharmGKB: a worldwide resource for pharmacogenomic information. Wiley Interdiscip. Rev. Syst. Biol. Med. 10, e1417. doi:10.1002/wsbm.1417
Barbitoff, Y. A., Abasov, R., Tvorogova, V. E., Glotov, A. S., and Predeus, A. V. (2022). Systematic benchmark of state-of-the-art variant calling pipelines identifies major factors affecting accuracy of coding sequence variant discovery. BMC genomics 23, 155. doi:10.1186/s12864-022-08365-3
Blanco, C., Verbanic, S., Seelig, B., and Chen, I. A. (2020). High throughput sequencing of in vitro selections of mRNA-displayed peptides: data analysis and applications. Phys. Chem. Chem. Phys. 22, 6492–6506. doi:10.1039/c9cp05912a
Bolognini, D., and Magi, A. (2021). Evaluation of germline structural variant calling methods for nanopore sequencing data. Front. Genet. 12, 761791. doi:10.3389/fgene.2021.761791
Bolt, H., and Thier, R. (2006). Relevance of the deletion polymorphisms of the glutathione S-transferases GSTT1 and GSTM1 in pharmacology and toxicology. Curr. drug Metab. 7, 613–628. doi:10.2174/138920006778017786
Borden, B. A., Jhun, E. H., Danahey, K., Schierer, E., Apfelbaum, J. L., Anitescu, M., et al. (2021). Appraisal and development of evidence-based clinical decision support to enable perioperative pharmacogenomic application. Pharmacogenomics J. 21, 691–711. doi:10.1038/s41397-021-00248-2
Botton, M. R., Yang, Y., Scott, E. R., Desnick, R. J., and Scott, S. A. (2020). Phased haplotype resolution of the SLC6A4 promoter using long-read single molecule real-time (SMRT) sequencing. Genes 11, 1333. doi:10.3390/genes11111333
Bowden, R., Davies, R. W., Heger, A., Pagnamenta, A. T., de Cesare, M., Oikkonen, L. E., et al. (2019). Sequencing of human genomes with nanopore technology. Nat. Commun. 10, 1869. doi:10.1038/s41467-019-09637-5
Buermans, H. P., Vossen, R. H., Anvar, S. Y., Allard, W. G., Guchelaar, H. J., White, S. J., et al. (2017). Flexible and scalable full-length CYP2D6 long amplicon PacBio sequencing. Hum. Mutat. 38, 310–316. doi:10.1002/humu.23166
Burton, A. S., Stahl, S. E., John, K. K., Jain, M., Juul, S., Turner, D. J., et al. (2020). Off earth identification of bacterial populations using 16S rDNA nanopore sequencing. Genes 11, 76. doi:10.3390/genes11010076
Byrska-Bishop, M., Evani, U. S., Zhao, X., Basile, A. O., Abel, H. J., Regier, A. A., et al. (2022). High-coverage whole-genome sequencing of the expanded 1000 Genomes Project cohort including 602 trios. Cell 185, 3426–3440. doi:10.1016/j.cell.2022.08.004
Cai, R., Dong, Y., Fang, M., Guo, C., and Ma, X. (2020). De novo genome assembly of a Han Chinese male and genome-wide detection of structural variants using Oxford Nanopore sequencing. Mol. Genet. Genomics 295, 871–876. doi:10.1007/s00438-020-01672-y
Cannon, M., Stevenson, J., Stahl, K., Basu, R., Coffman, A., Kiwala, S., et al. (2024). DGIdb 5.0: rebuilding the drug–gene interaction database for precision medicine and drug discovery platforms. Nucleic Acids Res. 52, D1227–D1235. doi:10.1093/nar/gkad1040
Caspar, S., Dubacher, N., Kopps, A., Meienberg, J., Henggeler, C., and Matyas, G. (2018). Clinical sequencing: from raw data to diagnosis with lifetime value. Clin. Genet. 93, 508–519. doi:10.1111/cge.13190
Caspar, S. M., Schneider, T., Stoll, P., Meienberg, J., and Matyas, G. (2021). Potential of whole-genome sequencing-based pharmacogenetic profiling. Pharmacogenomics 22, 177–190. doi:10.2217/pgs-2020-0155
Castelli, E. C., Paz, M. A., Souza, A. S., Ramalho, J., and Mendes-Junior, C. T. (2018). Hla-mapper: an application to optimize the mapping of HLA sequences produced by massively parallel sequencing procedures. Hum. Immunol. 79, 678–684. doi:10.1016/j.humimm.2018.06.010
Castro-Wallace, S. L., Chiu, C. Y., John, K. K., Stahl, S. E., Rubins, K. H., McIntyre, A. B., et al. (2017). Nanopore DNA sequencing and genome assembly on the international space station. Sci. Rep. 7, 18022. doi:10.1038/s41598-017-18364-0
Chaisson, M. J., Huddleston, J., Dennis, M. Y., Sudmant, P. H., Malig, M., Hormozdiari, F., et al. (2015). Resolving the complexity of the human genome using single-molecule sequencing. Nature 517, 608–611. doi:10.1038/nature13907
Chaisson, M. J., and Tesler, G. (2012). Mapping single molecule sequencing reads using basic local alignment with successive refinement (BLASR): application and theory. BMC Bioinforma. 13, 238–318. doi:10.1186/1471-2105-13-238
Chamorro, J. G., Castagnino, J. P., Aidar, O., Musella, R. M., Frías, A., Visca, M., et al. (2017). Effect of gene–gene and gene–environment interactions associated with antituberculosis drug-induced hepatotoxicity. Pharmacogenetics Genomics 27, 363–371. doi:10.1097/FPC.0000000000000300
Charnaud, S., Munro, J. E., Semenec, L., Mazhari, R., Brewster, J., Bourke, C., et al. (2022). PacBio long-read amplicon sequencing enables scalable high-resolution population allele typing of the complex CYP2D6 locus. Commun. Biol. 5, 168. doi:10.1038/s42003-022-03102-8
Chen, X., Shen, F., Gonzaludo, N., Malhotra, A., Rogert, C., Taft, R. J., et al. (2021). Cyrius: accurate CYP2D6 genotyping using whole-genome sequencing data. Pharmacogenomics J. 21, 251–261. doi:10.1038/s41397-020-00205-5
Chen, X. Y., Yan, W. H., Lin, A., Xu, H. H., Zhang, J. G., and Wang, X. X. (2008). The 14 bp deletion polymorphisms in HLA-G gene play an important role in the expression of soluble HLA-G in plasma. Tissue Antigens 72, 335–341. doi:10.1111/j.1399-0039.2008.01107.x
Cornaby, C., Montgomery, M. C., Liu, C., and Weimer, E. T. (2022). Unique molecular identifier-based high-resolution HLA typing and transcript quantitation using long-read sequencing. Front. Genet. 13, 901377. doi:10.3389/fgene.2022.901377
De Coster, W., D’hert, S., Schultz, D. T., Cruts, M., and Van Broeckhoven, C. (2018). NanoPack: visualizing and processing long-read sequencing data. Bioinformatics 34, 2666–2669. doi:10.1093/bioinformatics/bty149
De Coster, W., Weissensteiner, M. H., and Sedlazeck, F. J. (2021). Towards population-scale long-read sequencing. Nat. Rev. Genet. 22, 572–587. doi:10.1038/s41576-021-00367-3
Deserranno, K., Tilleman, L., Rubben, K., Deforce, D., and Van Nieuwerburgh, F. (2023). Targeted haplotyping in pharmacogenomics using Oxford Nanopore Technologies’ adaptive sampling. Front. Pharmacol. 14, 1286764. doi:10.3389/fphar.2023.1286764
Desta, Z., El-Boraie, A., Gong, L., Somogyi, A. A., Lauschke, V. M., Dandara, C., et al. (2021). PharmVar GeneFocus: CYP2B6. Clin. Pharmacol. and Ther. 110, 82–97. doi:10.1002/cpt.2166
Eid, J., Fehr, A., Gray, J., Luong, K., Lyle, J., Otto, G., et al. (2009). Real-time DNA sequencing from single polymerase molecules. Science 323, 133–138. doi:10.1126/science.1162986
Fan, W.-L., Shiao, M.-S., Hui, R.C.-Y., Su, S.-C., Wang, C.-W., Chang, Y.-C., et al. (2017). HLA association with drug-induced adverse reactions. J. Immunol. Res. 2017, 3186328. doi:10.1155/2017/3186328
Filippova, I., Khrunin, A., and Limborskaia, S. (2014). Analysis of a GSTM1 gene deletion in the context of the GSTM genomic cluster diversity in three Russian populations. Mikrobiol. i Virusol., 8–12.
Fukunaga, K., Hishinuma, E., Hiratsuka, M., Kato, K., Okusaka, T., Saito, T., et al. (2021). Determination of novel CYP2D6 haplotype using the targeted sequencing followed by the long-read sequencing and the functional characterization in the Japanese population. J. Hum. Genet. 66, 139–149. doi:10.1038/s10038-020-0815-x
Gaedigk, A., Boone, E. C., Scherer, S. E., Lee, S.-b., Numanagić, I., Sahinalp, C., et al. (2022). CYP2C8, CYP2C9, and CYP2C19 characterization using next-generation sequencing and haplotype analysis: a GeT-RM collaborative project. J. Mol. Diagnostics 24, 337–350. doi:10.1016/j.jmoldx.2021.12.011
Geoffroy, V., Lamouche, J.-B., Guignard, T., Nicaise, S., Kress, A., Scheidecker, S., et al. (2023). The AnnotSV webserver in 2023: updated visualization and ranking. Nucleic Acids Res., gkad426.
Giardina, E., and Zampatti, S. (2022). The future of pharmacogenomics requires new discoveries and innovative education. Genes (Basel) 13 (9), 1575. doi:10.3390/genes13091575
Giri, A. K., Khan, N. M., Grover, S., Kaur, I., Basu, A., Tandon, N., et al. (2014). Genetic epidemiology of pharmacogenetic variations in CYP2C9, CYP4F2 and VKORC1 genes associated with warfarin dosage in the Indian population. Pharmacogenomics 15, 1337–1354. doi:10.2217/pgs.14.88
Golubenko, E. O., Savelyeva, M. I., Sozaeva, Z. A., Korennaya, V. V., Poddubnaya, I. V., Valiev, T. T., et al. (2023). Predictive modeling of adverse drug reactions to tamoxifen therapy for breast cancer on base of pharmacogenomic testing. Drug Metabolism Personalized Ther. 38, 339–347. doi:10.1515/dmpt-2023-0027
Goodwin, S., McPherson, J. D., and McCombie, W. R. (2016). Coming of age: ten years of next-generation sequencing technologies. Nat. Rev. Genet. 17, 333–351. doi:10.1038/nrg.2016.49
Graansma, L. J., Zhai, Q., Busscher, L., Menafra, R., van den Berg, R. R., Kloet, S. L., et al. (2023). From gene to dose: long-read sequencing and*-allele tools to refine phenotype predictions of CYP2C19. Front. Pharmacol. 14, 1076574. doi:10.3389/fphar.2023.1076574
Häkkinen, K., Kiiski, J. I., Lähteenvuo, M., Jukuri, T., Suokas, K., Niemi-Pynttäri, J., et al. (2022). Implementation of CYP2D6 copy-number imputation panel and frequency of key pharmacogenetic variants in Finnish individuals with a psychotic disorder. Pharmacogenomics J. 22, 166–172. doi:10.1038/s41397-022-00270-y
Hall, A. R., Scott, A., Rotem, D., Mehta, K. K., Bayley, H., and Dekker, C. (2010). Hybrid pore formation by directed insertion of α-haemolysin into solid-state nanopores. Nat. Nanotechnol. 5, 874–877. doi:10.1038/nnano.2010.237
Hari, A., Zhou, Q., Gonzaludo, N., Harting, J., Scott, S. A., Qin, X., et al. (2023). An efficient genotyper and star-allele caller for pharmacogenomics. Genome Res. 33, 61–70. doi:10.1101/gr.277075.122
Helal, A. A., Saad, B. T., Saad, M. T., Mosaad, G. S., and Aboshanab, K. M. (2022). Evaluation of the available variant calling tools for oxford nanopore sequencing in breast cancer. Genes 13, 1583. doi:10.3390/genes13091583
Hernandez-Suarez, D. F., Claudio-Campos, K., Mirabal-Arroyo, J. E., Torres-Hernández, B. A., López-Candales, A., Melin, K., et al. (2016). Potential of a pharmacogenetic-guided algorithm to predict optimal warfarin dosing in a high-risk hispanic patient: role of a novel NQO1* 2 polymorphism. J. Investigative Med. High Impact Case Rep. 4, 2324709616682049. doi:10.1177/2324709616682049
Hitchman, L. M., Faatoese, A., Merriman, T. R., Miller, A. L., Liau, Y., Graham, O. E., et al. (2022). Allelic diversity of the pharmacogene CYP2D6 in New Zealand Māori and Pacific peoples. Front. Genet. 13, 1016416. doi:10.3389/fgene.2022.1016416
Hofmeister, R. J., Ribeiro, D. M., Rubinacci, S., and Delaneau, O. (2023). Accurate rare variant phasing of whole-genome and whole-exome sequencing data in the UK Biobank. Nat. Genet. 55, 1243–1249. doi:10.1038/s41588-023-01415-w
Holmes, M. J., Mahjour, B., Castro, C. P., Farnum, G. A., Diehl, A. G., and Boyle, A. P. (2023). LRphase: an efficient method for assigning haplotype identity to long reads. bioRxiv. doi:10.1101/2023.01.18.524565
Hotaling, S., Wilcox, E. R., Heckenhauer, J., Stewart, R. J., and Frandsen, P. B. (2023). Highly accurate long reads are crucial for realizing the potential of biodiversity genomics. BMC genomics 24, 117–119. doi:10.1186/s12864-023-09193-9
Houwaart, T., Scholz, S., Pollock, N. R., Palmer, W. H., Kichula, K. M., Strelow, D., et al. (2023). Complete sequences of six major histocompatibility complex haplotypes, including all the major MHC class II structures. Hla 102, 28–43. doi:10.1111/tan.15020
Huddleston, J., Chaisson, M. J., Steinberg, K. M., Warren, W., Hoekzema, K., Gordon, D., et al. (2017). Discovery and genotyping of structural variation from long-read haploid genome sequence data. Genome Res. 27, 677–685. doi:10.1101/gr.214007.116
Hufnagel, D. E., Hufford, M. B., and Seetharam, A. S. (2020). SequelTools: a suite of tools for working with PacBio Sequel raw sequence data. BMC Bioinforma. 21, 429–511. doi:10.1186/s12859-020-03751-8
Illing, P. T., Pymm, P., Croft, N. P., Hilton, H. G., Jojic, V., Han, A. S., et al. (2018). HLA-B57 micropolymorphism defines the sequence and conformational breadth of the immunopeptidome. Nat. Commun. 9, 4693. doi:10.1038/s41467-018-07109-w
Johannesen, K. M., Tümer, Z., Weckhuysen, S., Barakat, T. S., and Bayat, A. (2023). Solving the unsolved genetic epilepsies: current and future perspectives. Epilepsia 64, 3143–3154. doi:10.1111/epi.17780
Karnes, J. H., Miller, M. A., White, K. D., Konvinse, K. C., Pavlos, R. K., Redwood, A. J., et al. (2019). Applications of immunopharmacogenomics: predicting, preventing, and understanding immune-mediated adverse drug reactions. Annu. Rev. Pharmacol. Toxicol. 59, 463–486. doi:10.1146/annurev-pharmtox-010818-021818
Kaye, J. B., Schultz, L. E., Steiner, H. E., Kittles, R. A., Cavallari, L. H., and Karnes, J. H. (2017). Warfarin pharmacogenomics in diverse populations. J. Hum. Pharmacol. Drug Ther. 37, 1150–1163. doi:10.1002/phar.1982
Kramer, W. E., Walker, D. L., O’Kane, D. J., Mrazek, D. A., Fisher, P. K., Dukek, B. A., et al. (2009a). CYP2D6: novel genomic structures and alleles. Pharmacogenetics genomics 19, 813–822. doi:10.1097/FPC.0b013e3283317b95
Kramer, W. E., Walker, D. L., O'Kane, D. J., Mrazek, D. A., Fisher, P. K., Dukek, B. A., et al. (2009b). CYP2D6: novel genomic structures and alleles. Pharmacogenetics genomics 19, 813–822. doi:10.1097/FPC.0b013e3283317b95
Kronenberg, Z. N., Fiddes, I. T., Gordon, D., Murali, S., Cantsilieris, S., Meyerson, O. S., et al. (2018). High-resolution comparative analysis of great ape genomes. Science 360, eaar6343. doi:10.1126/science.aar6343
Kulmanov, M., Tawfiq, R., Al Ali, H., Abdelhakim, M., Alarawi, M., Aldakhil, H., et al. (2022). A personal, reference quality, fully annotated genome from a Saudi individual. bioRxiv.
Langlois, A. W., Chenoweth, M. J., Twesigomwe, D., Scantamburlo, G., Whirl-Carrillo, M., Sangkuhl, K., et al. (2024). PharmVar GeneFocus: CYP2A6. Clin. Pharmacol. and Ther. 116, 948–962. doi:10.1002/cpt.3387
Laver, T. W., Caswell, R. C., Moore, K. A., Poschmann, J., Johnson, M. B., Owens, M. M., et al. (2016). Pitfalls of haplotype phasing from amplicon-based long-read sequencing. Sci. Rep. 6, 21746. doi:10.1038/srep21746
Leckband, S., Kelsoe, J., Dunnenberger, H., George, A., Tran, E., Berger, R., et al. (2013). Clinical Pharmacogenetics Implementation Consortium guidelines for HLA-B genotype and carbamazepine dosing. Clin. Pharmacol. and Ther. 94, 324–328. doi:10.1038/clpt.2013.103
Lee, H., Kim, J., and Lee, J. (2023). Benchmarking datasets for assembly-based variant calling using high-fidelity long reads. BMC genomics 24, 148. doi:10.1186/s12864-023-09255-y
Lee, S.-b., Shin, J.-Y., Kwon, N.-J., Kim, C., and Seo, J.-S. (2022). ClinPharmSeq: a targeted sequencing panel for clinical pharmacogenetics implementation. PLoS One 17, e0272129. doi:10.1371/journal.pone.0272129
Lee, S.-b., Wheeler, M. M., Patterson, K., McGee, S., Dalton, R., Woodahl, E. L., et al. (2019). Stargazer: a software tool for calling star alleles from next-generation sequencing data using CYP2D6 as a model. Genet. Med. 21, 361–372. doi:10.1038/s41436-018-0054-0
Li, H. (2018). Minimap2: pairwise alignment for nucleotide sequences. Bioinformatics 34, 3094–3100. doi:10.1093/bioinformatics/bty191
Liau, Y., Cree, S. L., Maggo, S., Miller, A. L., Pearson, J. F., Gladding, P. A., et al. (2019b). A multiplex pharmacogenetics assay using the MinION nanopore sequencing device. Pharmacogenetics Genomics 29, 207–215. doi:10.1097/FPC.0000000000000385
Liau, Y., Maggo, S., Miller, A. L., Pearson, J. F., Kennedy, M. A., and Cree, S. L. (2019a). Nanopore sequencing of the pharmacogene CYP2D6 allows simultaneous haplotyping and detection of duplications. Pharmacogenomics 20, 1033–1047. doi:10.2217/pgs-2019-0080
Magdy, T., Kuo, H. H., and Burridge, P. W. (2020). Precise and cost-effective nanopore sequencing for post-GWAS fine-mapping and causal variant identification. Iscience 23, 100971. doi:10.1016/j.isci.2020.100971
Mantere, T., Kersten, S., and Hoischen, A. (2019). Long-read sequencing emerging in medical genetics. Front. Genet. 10, 426. doi:10.3389/fgene.2019.00426
Martin, M., Patterson, M., Garg, S., O Fischer, S., Pisanti, N., Klau, G. W., et al. (2016). WhatsHap: fast and accurate read-based phasing. BioRxiv, 085050.
Martis, S., Mei, H., Vijzelaar, R., Edelmann, L., Desnick, R. J., and Scott, S. A. (2013). Multi-ethnic cytochrome-P450 copy number profiling: novel pharmacogenetic alleles and mechanism of copy number variation formation. pharmacogenomics J. 13, 558–566. doi:10.1038/tpj.2012.48
Marx, V. (2023). Method of the year: long-read sequencing. Nat. Methods 20, 6–11. doi:10.1038/s41592-022-01730-w
Matern, B. M., Olieslagers, T. I., Groeneweg, M., Duygu, B., Wieten, L., Tilanus, M. G., et al. (2020). Long-read nanopore sequencing validated for human leukocyte antigen class I typing in routine diagnostics. J. Mol. Diagnostics 22, 912–919. doi:10.1016/j.jmoldx.2020.04.001
Ménard, V., Eap, O., Harvey, M., Guillemette, C., and Lévesque, É. (2009). Copy-number variations (CNVs) of the human sex steroid metabolizing genes UGT2B17 and UGT2B28 and their associations with a UGT2B15 functional polymorphism. Hum. Mutat. 30, 1310–1319. doi:10.1002/humu.21054
Merker, J. D., Wenger, A. M., Sneddon, T., Grove, M., Zappala, Z., Fresard, L., et al. (2018). Long-read genome sequencing identifies causal structural variation in a Mendelian disease. Genet. Med. 20, 159–163. doi:10.1038/gim.2017.86
Midha, M. K., Wu, M., and Chiu, K.-P. (2019). Long-read sequencing in deciphering human genetics to a greater depth. Hum. Genet. 138, 1201–1215. doi:10.1007/s00439-019-02064-y
Neu, M., Yang, Y., and Scott, S. A. (2023). Long-read sequencing for germline pharmacogenomic testing. Adv. Mol. Pathology 6, 99–109. doi:10.1016/j.yamp.2023.08.004
Nofziger, C., Turner, A. J., Sangkuhl, K., Whirl-Carrillo, M., Agúndez, J. A., Black, J. L., et al. (2020). PharmVar genefocus: CYP2D6. Clin. Pharmacol. and Ther. 107, 154–170. doi:10.1002/cpt.1643
Nunez-Torres, R., Pita, G., Peña-Chilet, M., López-López, D., Zamora, J., Roldán, G., et al. (2023). A comprehensive analysis of 21 actionable pharmacogenes in the Spanish population: from genetic characterisation to clinical impact. Pharmaceutics 15, 1286. doi:10.3390/pharmaceutics15041286
Oehler, J. B., Wright, H., Stark, Z., Mallett, A. J., and Schmitz, U. (2023). The application of long-read sequencing in clinical settings. Hum. genomics 17, 73. doi:10.1186/s40246-023-00522-3
Ozsolak, F. (2012). Third-generation sequencing techniques and applications to drug discovery. Expert Opin. drug Discov. 7, 231–243. doi:10.1517/17460441.2012.660145
Payne, A., Holmes, N., Rakyan, V., and Loose, M. (2019). BulkVis: a graphical viewer for Oxford nanopore bulk FAST5 files. Bioinformatics 35, 2193–2198. doi:10.1093/bioinformatics/bty841
Pervez, M. T., Abbas, S. H., Moustafa, M. F., Aslam, N., and Shah, S. S. M. (2022). A comprehensive review of performance of next-generation sequencing platforms. BioMed Res. Int. 2022, 3457806. doi:10.1155/2022/3457806
Petersen, L. M., Martin, I. W., Moschetti, W. E., Kershaw, C. M., and Tsongalis, G. J. (2019). Third-generation sequencing in the clinical laboratory: exploring the advantages and challenges of nanopore sequencing. J. Clin. Microbiol. 58, e01315. doi:10.1128/jcm.01315-19
Pirmohamed, M. (2023). Pharmacogenomics: current status and future perspectives. Nat. Rev. Genet. 24, 350–362. doi:10.1038/s41576-022-00572-8
Pollard, M. O., Gurdasani, D., Mentzer, A. J., Porter, T., and Sandhu, M. S. (2018). Long reads: their purpose and place. Hum. Mol. Genet. 27, R234–R241. doi:10.1093/hmg/ddy177
Qiao, W., Yang, Y., Sebra, R., Mendiratta, G., Gaedigk, A., Desnick, R. J., et al. (2016). Long-read single molecule real-time full gene sequencing of cytochrome P450-2D6. Hum. Mutat. 37, 315–323. doi:10.1002/humu.22936
Ramírez, B., Niño-Orrego, M. J., Cárdenas, D., Ariza, K. E., Quintero, K., Contreras Bravo, N. C., et al. (2019). Copy number variation profiling in pharmacogenetics CYP-450 and GST genes in Colombian population. BMC Med. Genomics 12, 1–9.
Romero-Lorca, A., Novillo, A., Gaibar, M., Bandrés, F., and Fernández-Santander, A. (2015). Impacts of the glucuronidase genotypes UGT1A4, UGT2B7, UGT2B15 and UGT2B17 on tamoxifen metabolism in breast cancer patients. PLoS One 10, e0132269. doi:10.1371/journal.pone.0132269
Rubben, K., Tilleman, L., Deserranno, K., Tytgat, O., Deforce, D., and Van Nieuwerburgh, F. (2022). Cas9 targeted nanopore sequencing with enhanced variant calling improves CYP2D6-CYP2D7 hybrid allele genotyping. PLoS Genet. 18, e1010176. doi:10.1371/journal.pgen.1010176
Satam, H., Joshi, K., Mangrolia, U., Waghoo, S., Zaidi, G., Rawool, S., et al. (2023). Next-generation sequencing technology: current trends and advancements. Biology 12, 997. doi:10.3390/biology12070997
Scott, E. R., Yang, Y., Botton, M. R., Seki, Y., Hoshitsuki, K., Harting, J., et al. (2022). Long-read HiFi sequencing of NUDT15: phased full-gene haplotyping and pharmacogenomic allele discovery. Hum. Mutat. 43, 1557–1566. doi:10.1002/humu.24457
Sedlazeck, F. J., Lee, H., Darby, C. A., and Schatz, M. C. (2018b). Piercing the dark matter: bioinformatics of long-range sequencing and mapping. Nat. Rev. Genet. 19, 329–346. doi:10.1038/s41576-018-0003-4
Sedlazeck, F. J., Rescheneder, P., Smolka, M., Fang, H., Nattestad, M., Von Haeseler, A., et al. (2018a). Accurate detection of complex structural variations using single-molecule sequencing. Nat. methods 15, 461–468. doi:10.1038/s41592-018-0001-7
Seo, J.-S., Rhie, A., Kim, J., Lee, S., Sohn, M.-H., Kim, C.-U., et al. (2016). De novo assembly and phasing of a Korean human genome. Nature 538, 243–247. doi:10.1038/nature20098
Shendure, J., and Ji, H. (2008). Next-generation DNA sequencing. Nat. Biotechnol. 26, 1135–1145. doi:10.1038/nbt1486
Sherman, C. A., Claw, K. G., and Lee, S.-b. (2024). Pharmacogenetic analysis of structural variation in the 1000 genomes project using whole genome sequences. Sci. Rep. 14, 22774. doi:10.1038/s41598-024-73748-3
Stahl-Rommel, S., Jain, M., Nguyen, H. N., Arnold, R. R., Aunon-Chancellor, S. M., Sharp, G. M., et al. (2021). Real-time culture-independent microbial profiling onboard the international space station using nanopore sequencing. Genes 12, 106. doi:10.3390/genes12010106
Tafazoli, A., van der Lee, M., Swen, J. J., Zeller, A., Wawrusiewicz-Kurylonek, N., Mei, H., et al. (2022). Development of an extensive workflow for comprehensive clinical pharmacogenomic profiling: lessons from a pilot study on 100 whole exome sequencing data. Pharmacogenomics J. 22, 276–283. doi:10.1038/s41397-022-00286-4
Takayama, J., Tadaka, S., Yano, K., Katsuoka, F., Gocho, C., Funayama, T., et al. (2021). Construction and integration of three de novo Japanese human genome assemblies toward a population-specific reference. Nat. Commun. 12, 226. doi:10.1038/s41467-020-20146-8
Taylor, C., Crosby, I., Yip, V., Maguire, P., Pirmohamed, M., and Turner, R. M. (2020). A review of the important role of CYP2D6 in pharmacogenomics. Genes 11, 1295. doi:10.3390/genes11111295
Tilgner, H., Grubert, F., Sharon, D., and Snyder, M. P. (2014). Defining a personal, allele-specific, and single-molecule long-read transcriptome. Proc. Natl. Acad. Sci. 111, 9869–9874. doi:10.1073/pnas.1400447111
Tilleman, L., Rubben, K., Van Criekinge, W., Deforce, D., and Van Nieuwerburgh, F. (2022). Haplotyping pharmacogenes using TLA combined with Illumina or Nanopore sequencing. Sci. Rep. 12, 17734. doi:10.1038/s41598-022-22499-0
Ton, K. N., Cree, S. L., Gronert-Sum, S. J., Merriman, T. R., Stamp, L. K., and Kennedy, M. A. (2018a). Multiplexed nanopore sequencing of HLA-B locus in Māori and Pacific Island samples. Front. Genet. 9, 152. doi:10.3389/fgene.2018.00152
Ton, K. N., Cree, S. L., Gronert-Sum, S. J., Merriman, T. R., Stamp, L. K., and Kennedy, M. A. (2018b). Multiplexed nanopore sequencing of HLA-B locus in Māori and Pacific Island samples. Front. Genet. 9, 152. doi:10.3389/fgene.2018.00152
Tran, B., Brown, A. M., Bedard, P. L., Winquist, E., Goss, G. D., Hotte, S. J., et al. (2013). Feasibility of real time next generation sequencing of cancer genes linked to drug response: results from a clinical trial. Int. J. cancer 132, 1547–1555. doi:10.1002/ijc.27817
Tremmel, R., Klein, K., Battke, F., Fehr, S., Winter, S., Scheurenbrand, T., et al. (2020). Copy number variation profiling in pharmacogenes using panel-based exome resequencing and correlation to human liver expression. Hum. Genet. 139, 137–149. doi:10.1007/s00439-019-02093-7
Tremmel, R., Zhou, Y., Schwab, M., and Lauschke, V. M. (2023). Structural variation of the coding and non-coding human pharmacogenome. NPJ Genomic Med. 8, 24. doi:10.1038/s41525-023-00371-y
Turner, A. J., Derezinski, A. D., Gaedigk, A., Berres, M. E., Gregornik, D. B., Brown, K., et al. (2023). Characterization of complex structural variation in the CYP2D6-CYP2D7-CYP2D8 gene loci using single-molecule long-read sequencing. Front. Pharmacol. 14, 1195778. doi:10.3389/fphar.2023.1195778
Turpeinen, M., and Zanger, U. M. (2012). Cytochrome P450 2B6: function, genetics, and clinical relevance. Drug Metabolism Drug Interact. 27, 185–197. doi:10.1515/dmdi-2012-0027
Uddin, M., Nassir, N., Almarri, M., Kumail, M., Mohamed, N., Balan, B., et al. (2023). A draft Arab pangenome reference. Cell, 51. doi:10.2139/ssrn.4891977
van der Lee, M., Allard, W. G., Bollen, S., Santen, G. W., Ruivenkamp, C. A., Hoffer, M. J., et al. (2020). Repurposing of diagnostic whole exome sequencing data of 1,583 individuals for clinical pharmacogenetics. Clin. Pharmacol. and Ther. 107, 617–627. doi:10.1002/cpt.1665
van der Lee, M., Allard, W. G., Vossen, R. H., Baak-Pablo, R. F., Menafra, R., Deiman, B. A., et al. (2021). Toward predicting CYP2D6-mediated variable drug response from CYP2D6 gene sequencing data. Sci. Transl. Med. 13, eabf3637. doi:10.1126/scitranslmed.abf3637
van der Lee, M., Rowell, W. J., Menafra, R., Guchelaar, H.-J., Swen, J. J., and Anvar, S. Y. (2022). Application of long-read sequencing to elucidate complex pharmacogenomic regions: a proof of principle. pharmacogenomics J. 22, 75–81. doi:10.1038/s41397-021-00259-z
Venner, E., Patterson, K., Kalra, D., Wheeler, M. M., Chen, Y.-J., Kalla, S. E., et al. (2024). The frequency of pathogenic variation in the All of Us cohort reveals ancestry-driven disparities. Commun. Biol. 7, 174. doi:10.1038/s42003-023-05708-y
Wang, D., Bolleddula, J., Coenen-Stass, A., Grombacher, T., Dong, J. Q., Scheuenpflug, J., et al. (2024). Implementation of whole-exome sequencing for pharmacogenomics profiling and exploring its potential clinical utilities. Pharmacogenomics 25, 197–206. doi:10.2217/pgs-2023-0243
Wankaew, N., Chariyavilaskul, P., Chamnanphon, M., Assawapitaksakul, A., Chetruengchai, W., Pongpanich, M., et al. (2022). Genotypic and phenotypic landscapes of 51 pharmacogenes derived from whole-genome sequencing in a Thai population. Plos one 17, e0263621. doi:10.1371/journal.pone.0263621
Wenger, A. M., Peluso, P., Rowell, W. J., Chang, P.-C., Hall, R. J., Concepcion, G. T., et al. (2019). Accurate circular consensus long-read sequencing improves variant detection and assembly of a human genome. Nat. Biotechnol. 37, 1155–1162. doi:10.1038/s41587-019-0217-9
Wick, R. R., Judd, L. M., and Holt, K. E. (2019). Performance of neural network basecalling tools for Oxford Nanopore sequencing. Genome Biol. 20, 129–210. doi:10.1186/s13059-019-1727-y
Xu, Z., and Dixon, J. R. (2020). Genome reconstruction and haplotype phasing using chromosome conformation capture methodologies. Briefings Funct. Genomics 19, 139–150. doi:10.1093/bfgp/elz026
Yang, Y., Botton, M. R., Scott, E. R., and Scott, S. A. (2017). Sequencing the CYP2D6 gene: from variant allele discovery to clinical pharmacogenetic testing. Pharmacogenomics 18, 673–685. doi:10.2217/pgs-2017-0033
Zhang, X., Wang, Z., Zhuo, R., Wang, L., Qin, Y., Han, W., et al. (2023). G6PD drives glioma invasion by regulating SQSTM1 protein stability. Gene 874, 147476. doi:10.1016/j.gene.2023.147476
Zhou, Y., Tremmel, R., Schaeffeler, E., Schwab, M., and Lauschke, V. M. (2022). Challenges and opportunities associated with rare-variant pharmacogenomics. Trends Pharmacol. Sci. 43, 852–865. doi:10.1016/j.tips.2022.07.002
Keywords: long-read sequencing, clinical pharmacogenomics, applications and challenges, third-generation sequencing, rare pharmacovariants
Citation: Tafazoli A, Hemmati M, Rafigh M, Alimardani M, Khaghani F, Korostyński M and Karnes JH (2025) Leveraging long-read sequencing technologies for pharmacogenomic testing: applications, analytical strategies, challenges, and future perspectives. Front. Genet. 16:1435416. doi: 10.3389/fgene.2025.1435416
Received: 20 May 2024; Accepted: 07 April 2025;
Published: 30 April 2025.
Edited by:
Pawel Mroz, University of Minnesota Twin Cities, United StatesReviewed by:
Simran D. S. Maggo, School of Pharmacy, Shenandoah University, United StatesRenata Posmyk, Medical University of Bialystok, Poland
Copyright © 2025 Tafazoli, Hemmati, Rafigh, Alimardani, Khaghani, Korostyński and Karnes. This is an open-access article distributed under the terms of the Creative Commons Attribution License (CC BY). The use, distribution or reproduction in other forums is permitted, provided the original author(s) and the copyright owner(s) are credited and that the original publication in this journal is cited, in accordance with accepted academic practice. No use, distribution or reproduction is permitted which does not comply with these terms.
*Correspondence: Jason H. Karnes, a2FybmVzQGFyaXpvbmEuZWR1