- 1Center for Intelligence and Security Studies, University of the Bundeswehr Munich, Neubiberg, Germany
- 2Department of Economics and Management, University of the Bundeswehr Munich, Neubiberg, Germany
- 3Aleph Alpha, Heidelberg, Germany
- 4Institute for Software Technology, University of the Bundeswehr Munich, Neubiberg, Germany
This study explores the potential of AI to support the work of military intelligence analysts. In the study, 30 participants were randomly assigned to an experimental condition (in which they could use a proprietary AI tool) and a control group (no access to the AI tool). In both conditions, participants had access to the same dataset of 50 media articles and were asked to provide a comprehensive picture in response to a series of realistic military intelligence tasks. The proprietary AI tool included text search, automatic text summarization, and named entity recognition (NER) capabilities. It was shown that under time pressure, the use of the AI features resulted in better assessments than the control group. It was also shown that the probability estimates of the experimental group were closer to those of the experts. Despite these demonstrably better analysis results and probability estimates in the experimental group, no higher confidence in the sources used for the analysis task was found. Finally, the paper identifies the limitations of using AI in military intelligence, particularly in the context of analyzing ambiguous and contradictory information.
1 Introduction
The sheer volume of data that can be observed today makes it clear that military intelligence requires the use of artificial intelligence (AI) (Gartin, 2019). The benefits of using AI can come from many different angles and need to be clearly understood to identify their potential added value (Vogel et al., 2021). The primary role of military intelligence is to gather and analyze information to help military leaders make informed decisions. From an academic perspective, military intelligence represents a transdisciplinary field of research that draws on a multitude of disciplines, including political science, economics, sociology, and psychology, among others (Albrecht et al., 2022; Svendsen, 2017).
Military intelligence is thus concerned with the collection and analysis of information to provide a comprehensive picture of the situation. This may entail the collection of data on the armed forces and the examination of the plans and operations of other nations, as well as the gathering of information on developments affecting a nation's security (Sadiku and Musa, 2021).
The analyst is responsible for collecting, analyzing and presenting data and information for military intelligence. New developments in AI and its integration as an analytical tool promise a wide range of support capabilities for the analyst (Cho et al., 2020). The use of AI technologies is expected to reduce the burden on analysts, allowing them to focus on the core content of analyzing, assessing, and presenting the military intelligence situation (Hare and Coghill, 2016). It should be emphasized that the analyst should not be replaced by AI systems, but rather assisted. In particular, it must be ensured that analysts are always able to understand the information on which they are making an assessment (Blanchard and Taddeo, 2023).
This study describes the results of experiments that were conducted when deployment of a propriety AI tool. This tool was designed to support analysts in their role of capturing, analyzing and producing intelligence from data pertaining to national security. The capabilities of the programme, called deepCOM, are mainly based on a Large Language Model (LLM). The core functionality of deepCOM is semantic search. This allows the user to ask direct questions which are answered by the system, indicating the sources used. In addition, deepCOM can automatically summarize each report in the database, allowing the analyst to identify relevant sources from a summary of a few sentences. An additional Named Entity Recognition (NER) implemented in the system labels all reports fully automatically: if present in the text, tags are derived from mentions of time, places, organizations, and people, which are highlighted for the user both when identifying relevant sources and when reading (Devlin, 2018).
In general, AI is already being used in almost all military domains (Rashid et al., 2023). The interaction between humans and computers plays a special role in this research topic. This includes forms of dialogue and information transfer via various media. The aim is to enable computers to interact with people in a similar way to how people interact with one another. With the help of AI technologies, this interaction should be increasingly simplified and, ideally, improved. In particular, previous work has shown how AI can enhance individual creativity, productivity and decision-making (Dell'Acqua et al., 2025). Issues such as trust in human-computer interaction (McNeese et al., 2021) and networking actors to create a shared situational awareness (Gorman et al., 2017) also play a pivotal role in the context of our study.
The goal of this study is to evaluate the added value of using AI in the military analysis process. While previous research in the field of intelligence has mainly focused on the contribution of AI to the management of big data (Horlings, 2023), this study focuses on the support AI provides to human analysis and assessment. To be able to make empirically validated statements, an experiment was conducted in this study. Against the backdrop of the relationships identified, we discuss key issues for the effective use of AI in military intelligence analysis. These include the important areas of trust in human-computer interaction and how this can be improved through greater transparency of AI.
In order to answer the research question of the added value of AI in military intelligence analysis, the structure of this article is as follows: Section 2 provides an overview of the military analysis process based on the intelligence cycle. Section 3 then presents the AI functions that were investigated and how they support military analysts. Section 4 explains the experimental design, while Section 5 presents the resulting findings. Section 6 discusses the results of the experiment. Finally, Section 7 offers concluding remarks.
2 The intelligence cycle as the starting point of the military analysis process
In order to assess the support provided by AI systems in the military analysis process, it is first necessary to clarify how the intelligence process works in general (Horlings, 2023). The starting point is the so-called intelligence cycle, which describes the ideal process from the decision maker's request for information to the intelligence product (Lowenthal, 2022). It should be noted that the process is not a linear sequence of individual steps, but includes feedback loops (Hulnick, 2006).
Figure 1 illustrates the typical intelligence cycle.
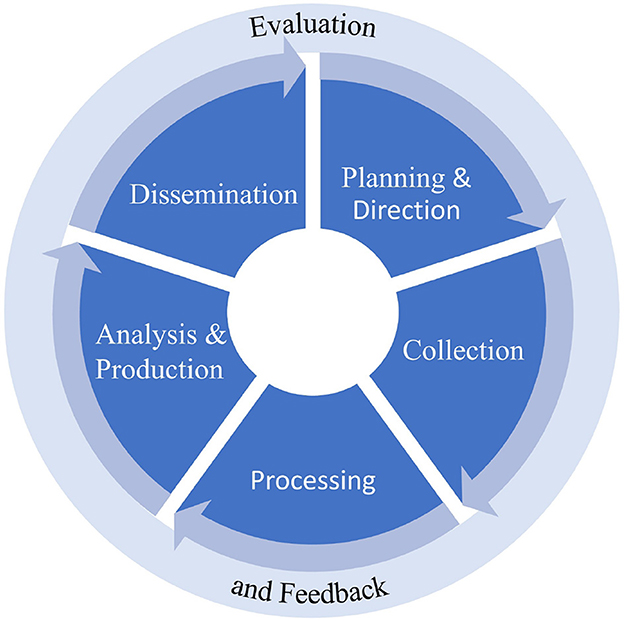
Figure 1. Intelligence cycle (Werro et al., 2024).
The process begins with the Planning & Direction phase. In this phase, a client or customer, in our cases a military decision maker, formulates a need for intelligence. This need is usually expressed in terms of questions that the customer believes must be answered in order to be able to make an informed decision. This defines an intelligence problem (Clark, 2019; Phythian et al., 2013).
Once the mandate is given, the second phase of the cycle, Collection, begins. This involves gathering information needed to produce the finished intelligence product (Phythian et al., 2013). Today, collection can be based on a variety of different intelligence disciplines: Human Intelligence (HUMINT), Imagery Intelligence (IMINT), Signals Intelligence (SIGINT), and Open Source Intelligence (OSINT) (Clark, 2013; NATO, 2016).
The Collection phase is followed by the Processing phase, in which the collected information is processed (Phythian et al., 2013). This includes translating foreign language texts, decoding, and organizing the information from human sources into a standardized reporting format (Clark, 2013). The main challenge in processing is that there is often more data from different sources than can be processed in a reasonable amount of time (Johnson, 1986).
The intelligence product is created in the Analysis & Production phase. This is done by integrating, evaluating, and analyzing all available information into an overall picture, taking into account the knowledge already available (Phythian et al., 2013). The analyst faces the challenge that the available information may be incomplete and contradictory. The goal of this phase is to obtain an assessment of ambiguous events and possible future events, thus providing the customer with a basis for an informed decision, for example, by recommending a course of action (Clark, 2019).
Finally, in the Dissemination phase, the intelligence product is distributed to the client. This may take the form of a written report or a verbal briefing (Clark, 2013). The decisions made by the customer may directly lead to further intelligence requirements or at least influence the content requirements for future finished intelligence, so the circle from Dissemination to Planning & Direction closes at this point (Phythian et al., 2013).
3 AI capabilities in the deepCOM demonstrator in support of military intelligence
The deepCOM demonstrator is an analysis tool with integrated AI capabilities designed to support the work of military analysts. The AI functions experimentally analyzed are described below. Two of the three AI functions tested in deepCOM, namely AI search and automated summarization, are based on an LLM. The third AI function tested is Named Entity Recognition. Although the intelligence community in Germany works in English due to international structures such as NATO, the United Nations and the EU, its own products are created in German. Accordingly, deepCOM's user interface and output are in German.
3.1 Artificial intelligence search in text databases
Standard searches in text databases are based on the frequency of occurrence of words. Accordingly, texts that contain more of the search terms will appear higher up on the list. In this method, also known as Bag of Words (BOW), the sheer frequency of the individual words determines a good search hit, not their relationship to one another (Qader et al., 2019). The BOW search typically starts with a question in the user's mind, which the user must break down into several keywords (Bohne et al., 2011), rather than typing the entire question into the search box. This type of search is usually the only way for the military analyst to search text, as the text databases need to be in a secure environment.
Such an approach is inefficient for several reasons: Firstly, information is lost during the interaction between the user and the query due to the forced reduction to keywords. Even if the search still works despite the omission of prepositions, cases, numbers and conjugations, the information it contains can help to produce better search results. Furthermore, the search process is not intuitive. When a question is already formulated, it is more straightforward to enter it into the search box without making any modifications. This is a standard practice for all major search engines on the Internet. Finally, BOW's search is based exclusively on the frequency of words in texts. However, this approach may result in the retrieval of irrelevant documents that contain the keywords in question, despite the documents' lack of relevance to the user's actual query.
The problems of BOW search can be solved by AI search. AI search is able to process a question as a whole. This results in less loss of information. In deepCOM, this is achieved by first displaying the answer of the AI search in the output, which is either formulated by the system itself or taken directly from a text, depending on the complexity of the question.
As can be seen in Figure 2, the answer given by the LLM-based AI search is independent of the exact wording of the questions. In addition, the AI search can deal with different spellings of identical entities (in the example shown, the different transliteration of an Arabic proper name). Full text references are always included. This increases the reliability of the answers and reduces the level of hallucination. Hallucinations sometimes occur in the LLM when the model produces false or invented information that still sounds convincing. This can make it difficult to fully trust the model's answers, even when full text references are provided. We will come back to how to deal with these issues—and how to improve trust in the results generated—in Section 7.
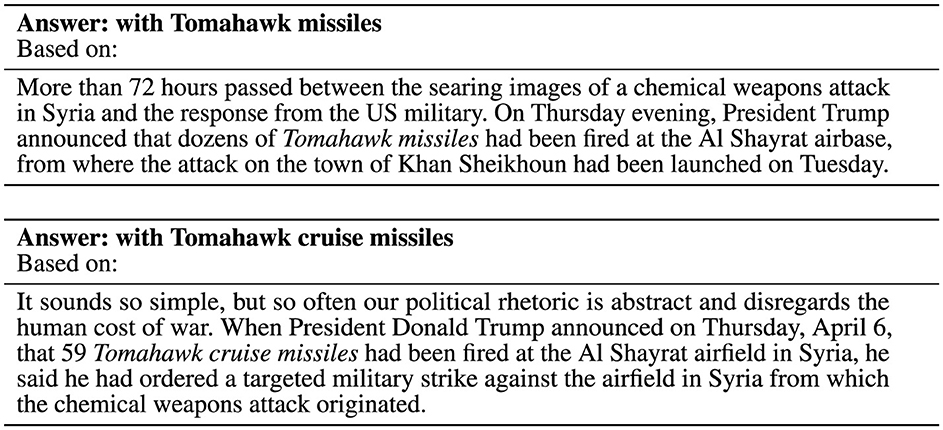
Figure 2. Sample AI search answers to the questions “How was the US air strike on Ash Sha‘irat carried out?” and “How did the US attack Al Shayrat airfield?” [source material translated from German to English].
3.2 Named Entity Recognition
Named Entity Recognition (NER) refers to the extraction of entities from unstructured text and their classification into predefined categories (Lample et al., 2016). The NER implemented in deepCOM is based on a German retraining of the Bidirectional Encoder Representations from Transformers models originally published by Google (Devlin, 2018; Yadav and Bethard, 2019). It automatically identifies time, place, organization, and person entities, even without optimization for specific text corpora. Since entities in texts usually occur in inflected form, lemmatization is also required to convert them to their base form and thus make them comparable (e.g., Mittelmeers, Mittelmeere → Mittelmeer). Both works largely correctly, although rare entities are sometimes incorrectly categorized or incorrectly converted to an uninflected base form. A detailed description of the use of NER in military intelligence can be found in Nitzl et al. (2024a).
In military intelligence, source code is still sometimes labeled manually, which is time consuming. NER can help the analyst to get a first impression of the content of a source. They can then more quickly decide whether a source is of interest. The color coding also speeds up the search for relevant information when reading full text. Finally, the automatically extracted entities can be displayed as locations on a world map in deepCOM. This allows the analyst to quickly locate events and integrate them into a situational picture. A heat map can also be used to identify clusters of locations and associated events.
Figure 3 shows an example of the use of NER for the above explanations.
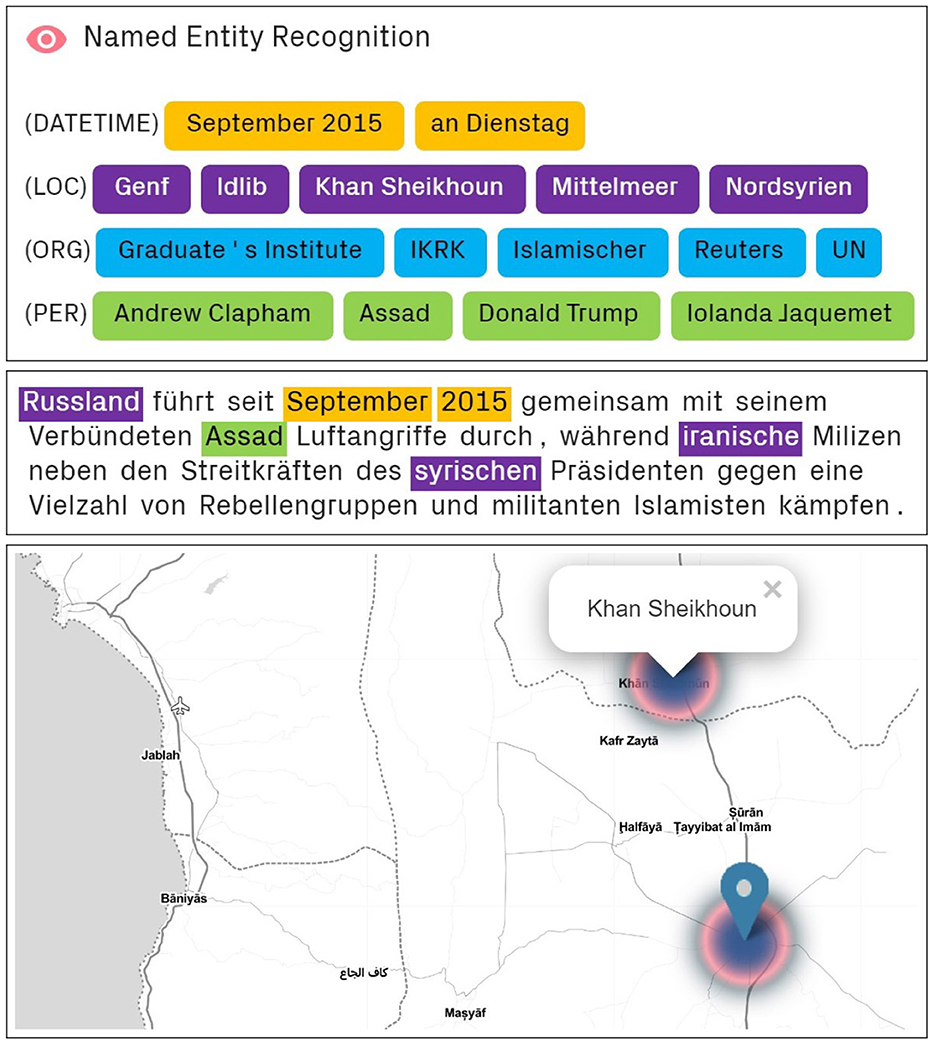
Figure 3. Top image: NER automatically extracts time, place, organization, and person names from the text. Middle image: Color coding of recognized entities in the [German] text. Bottom image: Display of recognized locations on a map.
3.3 Automated text summaries
Irrespective of the possible full-text presentation, the references in deepCOM are also reduced to about one-third to one-half of their original length by summarizing each paragraph into one sentence. Automated summarization thus serves a similar purpose to NER. Both allow a quick assessment of the importance of a text for an analysis. The conflict between the length of the text and the depth of detail that summarization provides must be decided on a situation-by-situation basis.
Automatic summarization is enabled by the LLM in the deepCOM back-end. The neural network algorithm allows to merge paragraphs or omit parts of sentences in such a way that the summary is a coherent image of the original text.
The implemented automatic summarization primarily uses the omission of individual sentence parts. Further tests with different settings for text summarization have shown that the summary generally works very well, but that too much world knowledge is added from the LLM training data.
Figure 4 shows an example of automated summarization.
4 Description of the experimental study
4.1 Military analysis scenario
The starting point of the experimental study is a realistic scenario from military intelligence analysis. A total of 50 source texts was collected from publicly accessible news portals on the internet. The sources were stored in deepCOM and served as a text database. The sources were selected to provide a comprehensive picture, including articles from both national and international news sites. These included news portals publishing in French, Russian, Arabic and Persian.
An overview of the sources used can be found in Appendix A.
The news texts refer to the poison gas attack in Khan Shaykhun in the Idlib governorate in northwestern Syria. On April 4, 2017, at least 86 people were killed and several hundred injured by sarin gas. The release of the poison gas is uncontested, but explanations of how it happened vary widely: According to the US, UK, French, and German accounts, the gas was deliberately dropped by an air strike by the Assad government's Syrian air force. The Idlib governorate and the town of Khan Shaykhun were considered a stronghold of the Syrian government's opposition at the time of the incident. However, according to Syrian, Russian, and Iranian accounts, the sarin was released because the Syrian Air Force had bombed an insurgent poison gas storage facility or factory with conventional weapons. In response to the poison gas attack, the US, under President Trump, launched cruise missile strikes on the Syrian military airfield of Al Shayrat, from which the attack on Khan Shaykhun is believed to have originated. The central task in the analysis process is to identify and select the relevant sources that provide the necessary information for a correct assessment of the situation. In order to meet this challenge in the experiment, the 50 source texts were selected in such a way that about one third could be used directly for the analysis, another third dealt with the poison gas attack in Khan Shaykhun only in passing (e.g., mention in stock market news), and the last third had no reference to the incident to be analyzed. What all texts have in common, however, is that they contain the keywords “Syria” and “poison gas.” The last third of the texts is deliberately used as a distraction in both the BOW and AI searches in order to divert attention from the relevant topic.
The analysis task begins four days after the poison gas attack in Khan Shaykhun on April 7, 2017. A military leader needs detailed information about the poison gas attack in Syria and its aftermath in order to make a decision on how to proceed, and therefore wants to be briefed in writing about the developments so far. A set of relevant questions was defined to specify the information required.
The participants were supposed to answer these questions in their own words in the first part of the analysis task. In order to achieve the best possible comparability between the experimental and the control groups, these questions had to be answered in a few key points. In addition, at least one source had to be cited for each answer. On the one hand, the sources mentioned by the participants reflect the reality in practice. On the other hand, it can be excluded that the participants arrive at the correct answers by guessing or through prior knowledge of the poison gas attack in Khan Shaykhun.
In the second part of the analysis task, participants were presented with propositions and asked to rate how likely they occurred. Expressing the uncertainty associated with predictions in the context of intelligence assessments exclusively in non-standardized textual form leads to limited comparability of different intelligence products, as the interpretation of verbal probability assessments depends heavily on the individual analyst (Heuer, 1999). NATO (2016) therefore requires that the presumed probability of occurrence of ambiguous events addressed in the analysis be assessed using a standardized scale. The guideline for assessing this probability serves as a answer to the question: “How likely is it that the event has occurred or will occur?” The answers are given on a five-point scale from very likely to very unlikely.
In the second part of the analysis task, participants were also asked to indicate how confident they were in their own assessment of the source situation (confidence). As the sources can be varied, numerous and consistent, confidence is asked in addition to the probability assessment. Participants answer the question: “Given the quality of the information available, how confident am I in my own judgments?” The response options were a three-point scale from high to medium to low.
This probability and confidence measure and its scales follow the guidelines of the NATO Allied Joint Doctrine for Intelligence Procedures (NATO, 2016). They are summarized in Table 1.
Figure 5 shows examples of items from both parts of the analysis task. The complete list of all items can be found in Appendix B.
Before the experiment began, the participants were individually introduced to the deepCOM interface and had the opportunity to ask questions about using the software. There was also a general briefing on the experimental procedure. Both groups used the same browser interface, except that the AI functions were deactivated in the control group. The AI search was replaced by a BOW search and the automatic summarization was replaced by a display of the first words of a paragraph. NER was deactivated in the control group with no equivalent.
The processing time for the analysis task was set to 30 min. Based on a pilot study, 25 min was estimated for the first analysis task and 5 minutes for the second analysis task. The processing time was indicated by a continuously visible timer, which switched from the first to the second analysis task after 25 min and ended the processing option completely after a further 5 min. All deepCOM functions were available during the entire processing time, depending on the assigned experimental or control group.
Additional questions such as the System Usability Scale (SUS) and demographic information were excluded from the 30-minute time limit. Participants could choose an individual start time for the experiment. The questions were completed on their own computer.
4.2 Selection and description of the participants in the experiment
A total of 30 participants from the “Master of Intelligence and Security Studies” (MISS) program were recruited to participate in the experiment at an information session and via email.1 Upon completion of the data collection process, the data were deleted according to the procedure outlined in the original advertisement. No other personal information was collected as part of the questionnaire. The data were collected via the SoSci server of the University of the Bundeswehr Munich (survey.unibw.de). The raw data used in the statistical analysis were anonymized before the analysis. It is not possible to trace the results back to individual persons. In addition, seven active duty soldiers, among others working in military intelligence as well as intelligence services were recruted. As part of the survey, participants were asked to provide information about their age, gender, and previous military assignments. Data were collected via a digital questionnaire in PDF format. The email address used to send the questionnaire was deleted after the survey was completed. Raw data from the experts included in the statistical analysis were anonymized, as well. Participants in the study were randomly assigned to either the experimental or control group.
An expert survey was conducted as a basis for evaluating the analysis performance of the participants. Experienced military analysts worked on the same analysis task as the participants in the experiment. They worked under the same conditions as the control group. However, the experts were not given a time limit to complete the analysis scenario. The expert answers in the first part and their evaluations about propability and confidence in the second part determined what was considered correct in the assessment. They therefore form the basis of what is achievable, the ground truth.
Vouchers were raffled off to encourage participation. Of the original 30 participants, one person had to be excluded for technical reasons. Therefore, the experimental group consisted of 14 participants and the control group consisted of 15 participants. All participants are active duty soldiers. They range in age from 20 to 33 years old (M = 26.6; SD = 4.3). Seven of the participants were women and 22 of the participants were men. In addition, seven military intelligence experts completed the analysis task. They were between 31 and 57 years old (M = 41.1; SD = 8.0) and all male. On average, they had been soldiers for 21.5 years (SD = 8.4). On average, the experts took 3 h and 49 min to complete the analysis task.
5 Analysis of the experimental results
In order to assess the participants' performance in the first part of the analysis task, the correct answers for each item were determined from the experts' answers. This resulted in a catalog. This catalog was used to assess the participants' analysis performance. The maximum possible score resulted from the average depth and complexity of the experts' answers. The possible number of points per item varied between 1 and 3. The average score per item was then calculated separately for the experimental and control groups. In this way, it was possible to determine how close the participants in both groups were to the experts' judgment.
The statistical analysis first checked whether there were statistically significant differences between the experimental group and the control group as a whole. The related items were then tested as blocks representing an analysis task. Task 6, which had an above-average number of six items and dealt with both the US air strike and the reaction of several nations to it, was divided up into two sub-tasks.
Table 2 shows the means (M) and standard deviations (SD) of the experimental and control groups. The χ2 and p-values (p) are also shown in the table. A two-sided likelihood ratio test (LRT) for differences in means for independent samples was used to test statistical significance.
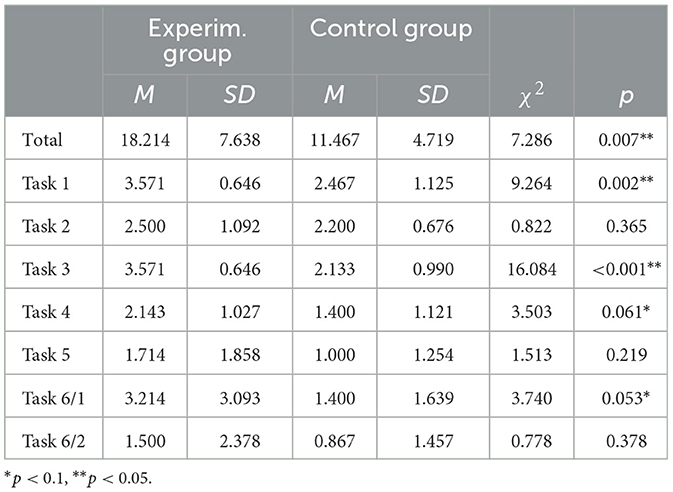
Table 2. Participants' scores on the first part of the analysis task, separated into total performance and performance per task.
The experimental group with AI support scored higher overall than that of the control group without AI support. On average, the experimental group exhibited a score that was more than six and a half points higher than the control group. Looking at the individual tasks, it can be seen that the AI support did not add significant value in all cases. The experimental group solved tasks 1, 3, 4, and 6/1 significantly better than the control group. No significant differences were found for the other tasks. Analysis performance did not correlate significantly with participant age (χ2 = 1.823; p = 0.177) or gender (χ2 = 1.910; p = 0.167).
The next step goes even deeper and shows the results for each individual item. Figure 6 shows the average percentages achieved per item. Since a score closer to 100% means a higher average agreement with the expert judgment, a higher score is considered to be better. As the total of 21 items had different scores to be achieved due to their varying complexity, they have been standardized to values between 0 and 100 so that they can also be understood as percentages.
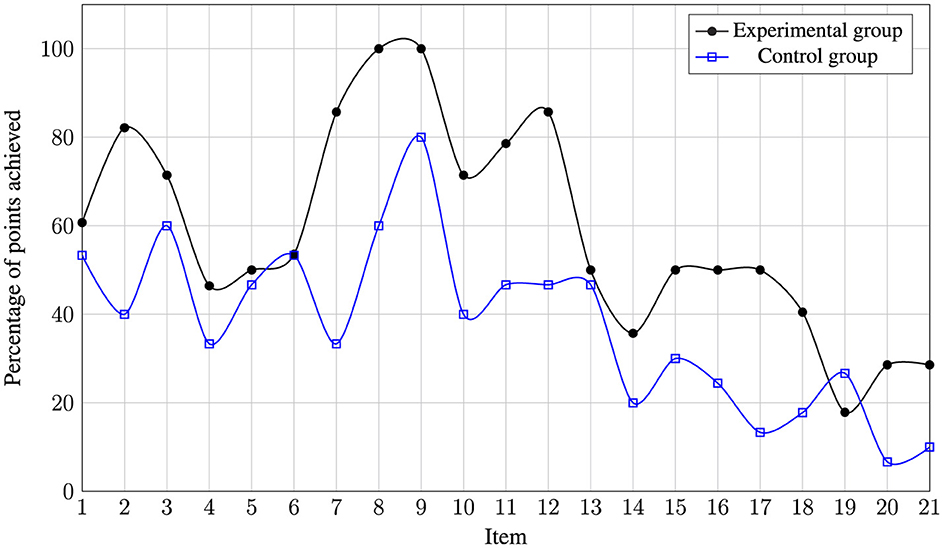
Figure 6. Percentage mean value per item separately for experimental and control groups in the first part of the analysis task.
The experimental group always performed better than the control group, except for Item 19. Furthermore, the items were of varying difficulty, as the curves are similar for both groups. For example, the solutions for Items 4 to 6 turned out to be significantly poorer than for Items 8 and 9, regardless of whether the AI functions were available to the subjects or not. Finally, it is noticeable that both groups were able to solve fewer and fewer items toward the end of the first part. This is due to the 25-min time limit for the first part of the analysis task.
In the second part of the analysis task, participants were asked to indicate the probability of pre-formulated propositions. They were also asked to indicate how confident they were that their assessment was correct based on the sources. Participants' assessments of probability and confidence in the second part of the analysis task were also evaluated by comparison with the experts' assessments. As these can be compared directly, no scoring standard is needed here. Furthermore, the assessment differs from the first part of the analysis task in that a deviation from the expert base is possible in both directions, i.e., an over- or underestimation of probability and confidence. Therefore, the discrepancy between each individual rating and the meaning of the expert ratings for that item was first determined. Then, the mean (M) and standard deviation (SD) of this discrepancy from the expert judgment were calculated.
Table 3 shows the results of the tests for two-sided significance for independent samples for the overall score (total) and for the individual blocks of tasks.
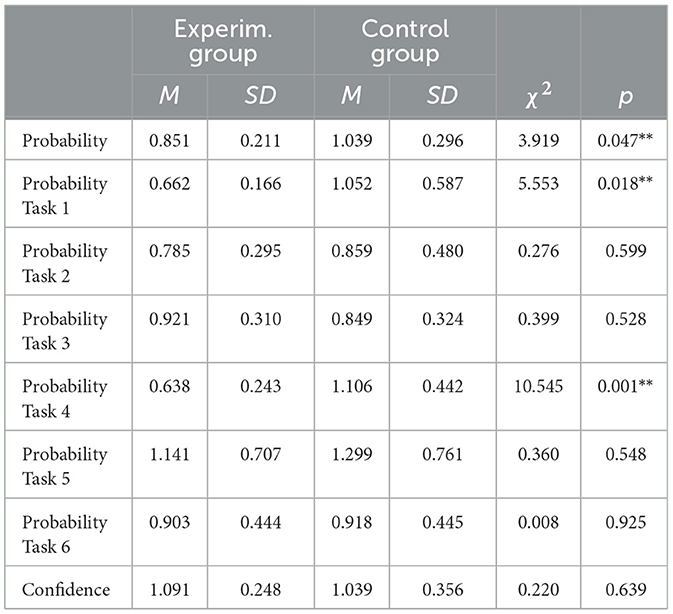
Table 3. Deviation of the participant's probability and confidence from the expert's judgment, measured as absolute value and the standard deviation of the item.
Overall, the experimental group with AI support was able to make significantly more estimates (probability) in line with the experts than the control group. As with the evaluation of the first part of the analysis task, both probability and confidence were analyzed on a task-by-task basis. On this task-by-task basis, only for Tasks 1 and 4 were the AI-assisted participants able to make a significantly higher assessment of probability in accordance with the expert group than the control group. There was no significant difference between the two groups in terms of confidence in the sources (therefore, these are not reported in Table 3). However, it can be observed that the experts, with a mean of 2.38 compared to the participants with a mean of 2.02, rated the confidence significantly higher over all propositions. In addition, for each of the propositions tested in the experiment, this higher rating of confidence by the experts compared to the participants can be observed.
Figure 7 illustrates the results for the probability scores, with lower values representing a more favorable outcome.
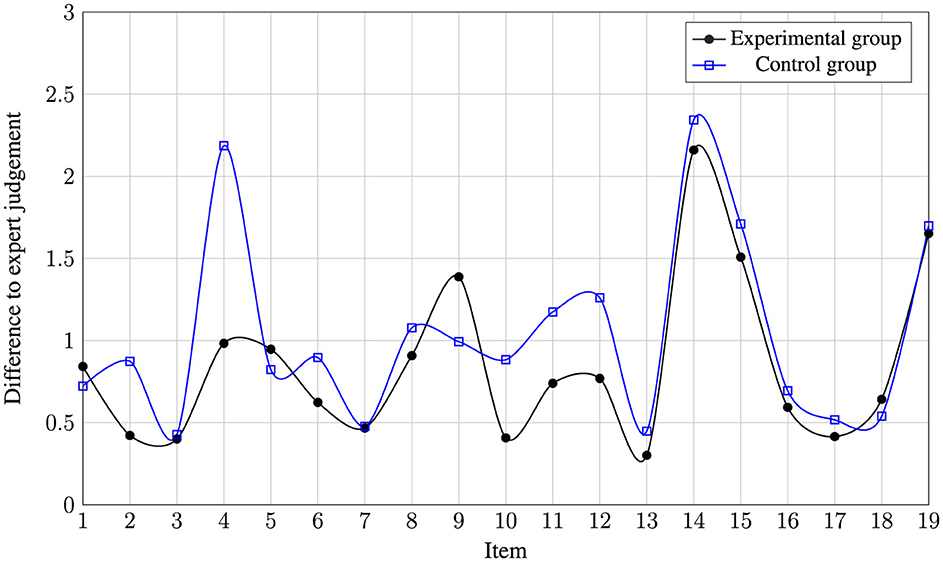
Figure 7. Absolute value of the difference between the experimental group and the control group from the probability expert judgment, measured as the standard deviation of the respective item.
Although the experimental group still outperformed the control group on most items, the difference is graphically less clear than in the first part of the analysis task. The poorer performance of the control group on Item 4 is still striking, as this was an assessment of whether the agent released at Khan Shaykhun could have been a gas other than sarin, chlorine or a mixture of the two. The experts were almost unanimous in their assessment that this was “highly unlikely'.
This view was shared mainly by the experimental group, but not by the control group. The latter rated this thesis on average as “unlikely'. A similar picture emerges for Items 14 and 15, but here for both groups. In conclusion it can be said that, in contrast to the first part of the analysis task, there is no decline in performance toward the end of the task. Probability estimation does not correlate significantly with age (χ2 = 3.564; p = 0.060) or gender (χ2 = 0.688; p = 0.407).
A post-hoc survey was also conducted after the experiment to determine whether deepCOM was perceived by the participants as having above-average usability, whether the use of deepCOM could lead to an increase in the speed of military analysis, and whether the three AI functions investigated in the experiment (AI search, automated summarization, NER) were considered suitable for use in military analysis. The post-hoc survey was conducted only among the 14 participants in the experimental group, as they were the only ones who had worked with the AI functions. The existing System Usability Scale (SUS) was used to assess usability (Lewis, 2018). For the remaining items of the post-hoc survey, separate questions were developed, which are listed in Appendix C. These were surveyed with several questions per evaluation criterion and summarized by averaging. Negatively answered questions were recoded.
With the exception of the SUS measurement, there are no reference or standard values for respondent behavior from other surveys. Therefore, the mean of the five-point Likert scale shown, i.e., 3, was taken as the baseline. For the SUS, the mean of 68 out of a maximum of 100 points was used based on existing surveys (Brooke, 2013; Sauro, 2011). Therefore, the values of 3 and 68 were used as the null hypothesis for the two-sided LRT. The results are presented in Table 4.
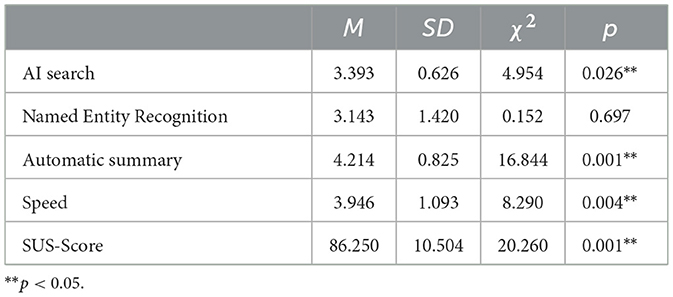
Table 4. Evaluation of the System Usability Scale (SUS), speed increase, and AI functions by experimental group participants.
With regard to the individual AI features, the subjects rated the automated summary (M = 4.2) and the AI search (M = 3.4) as significantly more suitable than average for military analysis. No such statement can be made for the NER. The experimental group also considers a significant increase in analysis speed to be achievable (M = 3.9). The System Usability Scale (SUS) was rated above average with a value of 86. The participants therefore believe that the user interface can support military analysis efficiently, effectively and satisfactorily.
6 Discussion
6.1 Comparison of the analytical performance of the experimental group with that of the control group
For the first part of the analysis task, the experiment showed that the use of AI functions leads to a demonstrable increase in performance. However, a more detailed analysis shows that this is not the case for all tasks. Since these blocks of tasks belong together thematically, further conclusions can be drawn for the AI functions. For this purpose, the tasks are divided up into three groups: Tasks in which the experimental group performed highly significantly (Group 1: Tasks 1 and 3), weakly significantly (Group 2: Tasks 4 and 6/1) and not significantly better (Group 3: Tasks 2, 5, and 6/2). All the questions in Group 1 have in common that they can be answered in a few words and in a factual manner. The sample items shown in Figure 8 can be answered with “insurgents” (1c) and “between 100 and 400” (3a).
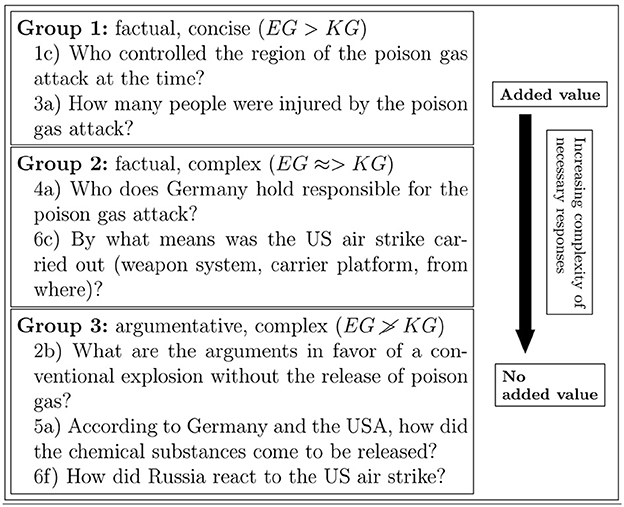
Figure 8. Examples of items for each of the three groups of items, in order of complexity of response required.
Group 2 consists of questions with similar characteristics to Group 1, except that the answers are more complex: Possible answers for Item 4a are “Syrian government,” “President Assad,” or “Syrian air force,” and for Item 6c “cruise missiles,” “tomahawks,” “warships,” and “destroyers.” All items in Group 2 can be answered largely factually. The items in Group 3, where no statement can be made about the added value of AI functions, require more complex answers. These are mainly argumentative and less clear than those in the first two Groups 1 and 2, requiring comparatively long answers. It can be concluded that the AI functions analyzed provide added value mainly for questions that require a direct and factual answer. As the complexity and ambiguity of the information increases, the benefit decreases.
A similar statement cannot be made for the second part of the analysis task regarding probability. It is true that the participants with AI support were able to give more accurate ratings than the control group on the overall scale and on Tasks 1 and 4. However, this observation is difficult to interpret because the tasks are grouped thematically in the same way, but do not differ in terms of complexity or other characteristics.
The poorer analytical performance of the control group, which had already been shown, led to less knowledge about the background of the American air strike. Since the control group had fewer source texts to analyze due to time pressure, their judgments in this regard were less reliable. Nevertheless, the conclusion for Task 4 remains an important observation, as time pressure is a realistic situation for military intelligence. Thus, the overall conclusion that the AI-assisted participants were able to make more accurate judgments than the control group remains valid.
The demonstrated superior analysis performance of the AI-assisted experimental group suggests that this would also be associated with greater confidence in the estimated probabilities based on the sources. However, a higher level of confidence in the assessment of the probability cannot be observed. This is also seen in the detailed analysis by task block. One explanation for this could be that the confidence assessment in both groups led to uncertainties in the assessment of the source situation due to time pressure. It is possible that the participants had the impression that not all sources that could have provided further information could be analyzed due to the short time available. This assumption is supported by the fact that the participants in both groups on average rated confidence considerably lower than the experts. The latter were not bound by time constraints when completing the tasks. However, it should be noted that the benefits of AI could not be fully exploited by considering only 50 sources. For example, AI can be used to analyze different data sources, such as image sources. By facilitating the triangulation of information from different data sources, AI can be expected to have a positive impact on analysts' confidence in the estimated probabilities.
6.2 Post-hoc survey of the experimental group
The evaluation of the post-hoc survey shows that the experimental group perceives a speed gain in military analysis through AI functions. However, it should be noted that the participants are not experts in the field of military analysis. Yet, their evaluation is in line with the findings by Perboli et al. (2021). In the context of the labeling of aviation accident documents, the use of AI functions for the partial automation of expert work has reduced the overall investigation time by 30%. For our experimental design, it should be noted that speed and workload are not be measured separately. They were measured jointly as analysis performance. Theoretically, it is possible that the AI functions contribute to a more accurate and comprehensive analysis, but the task itself takes the same amount of time. This would not be a problem, as the improved analysis performance would still be an added value. In this respect, the participants' assessment can be seen as an indication that the speed of intelligence analysis can be increased. This correlation was also confirmed in the personal feedback discussions with the participants. In particular, they felt that the direct answering of questions by the AI search had the potential to increase speed. Comparing the average time taken by the experts surveyed (3 h 49 min) with the experimental group (30 min), it can be assumed that the use of AI can lead to time savings.
In general, participants rated the automated summary in particular as being above average for use in military analysis. The NER and the AI search, on the other hand, were rated as being of average suitability. Three reasons for this can be deduced from the personal feedback interviews, in which the NER was also criticized by the trial participants: Firstly, the participants could not see any added value in the NER beyond the automatic summarization. Both AI functions were used with the aim of identifying suitable source texts more quickly. However, in a head-to-head comparison, the participants in the experimental group favored the automatic summarization. It can therefore be assumed that the NER is not completely unsuitable for use in AI analysis, but appears to be less suitable than the automated summary in a direct comparison due to the overlapping scope of application. Another reason given for the average rating was the sometimes incorrect labeling. In this context, three participants noted that they had wanted to use the NER to identify relevant source texts at the beginning of the study, but then refrained from doing so because, for example, people were incorrectly classified as places. Finally, several participants in the experimental group reported that some of the sub-functions of the NER, such as the color coding in the text or the display on a world map, were perceived as useful, but then simply overlooked due to the number of other features. Participants also attributed this to the time constraints of the experiment. In summary, the majority of respondents rated the NER as having average added value to the military analysis process due to competition from automated summarization, functionality that still needs improvement in some cases, and other AI functions.
The System Usability Scale (SUS) was rated above average by the participants. This shows that the user interface of the deepCOM demonstrator was successfully designed to be intuitive. This is also confirmed by the experience of getting used to deepCOM before the experiment. Not only did this rarely take longer than 5 min, but there were generally no questions about the user interface as it was perceived as self-explanatory. In the personal feedback interviews at the end of the experiment, the participants were also unable to make any suggestions for improving the design of the user interface.
6.3 The challenges associated with the use of artificial intelligence in military analysis
The study identified several challenges in applying AI to the military analysis process; see also Devanny et al. (2023) and Horlings (2023). For example, the AI search encountered problems when the underlying texts contained no or insufficient information for the answer. LLM always gives the answer with the highest probability. If the sources are poor, this may lead to an accumulation of wrong answers. This was also evident in the first part of the analysis task which contained two trick questions. The correct answer to the question “Which nations were also involved in the US air strike?” was “None,” and the possible answer to the question “What are the signs of a conventional explosion without the release of poison gas?” was “Nothing.” In both cases, the AI search gave the wrong answer. The problem is that LLM always finds a passage in the source text that could answer the question posed.
There is also room for improvement in the NER. Although entities are almost always recognized and extracted as such, the classification into different groups (person, place, etc.) still fails too often. Lemmatization is not perfect in some cases, either, especially in the case of military terms and proper names, which are reduced to incorrect stems. These problems could probably be solved by retraining. The NER used was not adapted for a specific purpose, but was trained for German texts in general. However, it could be specialized for entities that occur exclusively in military source texts (e.g., names of weapons, names of military leaders). This would require retraining on as large a dataset of military reports as possible. The automatic creation of domain-specific dictionaries based on military reports would be another possible improvement in this context (Häffner et al., 2023).
A final challenge is the volume of text to be processed in military intelligence. The analysis scenario of the experiment involved only 50 reports, a quantity that can also be processed with a BOW search in a reasonable amount of time. Scaling up to several thousand texts, for example, could quickly overwhelm the BOW search, which is based on word frequency and does not really capture the content of the texts. The semantic understanding of an LLM, on the other hand, allows the same word occurrences to be interpreted in the context of the text or paragraph, making larger volumes of text manageable. In the subsequent phase, more sophisticated AI algorithms will undertake extractive summarization across multiple documents, employing unsupervised techniques such as Lamsiyah et al. (2021). Utilizing sentence embedding representations, they will identify pivotal sentences based on a combined scoring system. This system will then assess the relevance, novelty, and positional importance of sentence content, ensuring that the summary encompasses the most crucial and diverse information from the documents. The fact remains, however, that the performance of any analysis-supporting AI can never be better than the quality of the military source reports it works with (Vogel et al., 2021). So, while the use of AI makes it possible to sift through and analyze more sources, AI does not guarantee that the sources are reliable (Horlings, 2023).
Also, drawing from more sources (e.g., satellite feeds, intercepted communications, social media, etc.) can provide a more complete picture, and with enough high-quality data, AI models tend to generalize better and make fewer mistakes. In addition, AI can verify information by comparing across sources—if multiple places are reporting the same event, that adds credibility. On the other hand, sifting through low-quality, misleading, or noisy data can confuse the model and degrade performance. At the extreme, without good filtering and relevance scoring, the AI might surface unimportant things, miss important information, or inherit and amplify a bias. In addition, more data sources means more processing power, longer training times, potentially slower results, and less alignment with analyst expectations and confidence—especially in real-time scenarios (Li et al., 2025)—or alignment with AI accountability guidelines (Floridi, 2019; EU, 2024). More is not always better.
6.4 Limitations of the design of the study
One limitation to the validity of this study is that the time allowed for completion was limited to 30 min. The experts were used as a reference for the assessment of analytic performance, but were not under time constraints in completing the analysis tasks. In practice, however, it is often the case that intelligence products are produced under great time pressure. In this respect, the restriction of the processing time for the participants in the experiment can be regarded as a real situation in practice. In the first part of the analysis task, the experimental group achieved on average 100% of the baseline for two items, i.e., they performed as well as the experts. It cannot be excluded that the experimental group performed better than the experts due to the support of the AI functions, but that this could not be measured. It is also likely that the participants' answers to the confidence and probability questions were influenced by the time limit.
Another critical point regarding the study design is that the added value of the AI functions was demonstrated only in the context of a single analysis scenario. The scenario was designed to best model a real-world military intelligence scenario based on unclassified sources. However, it can be assumed that running multiple scenarios will result in a greater learning effect in the use of the AI tools and thus a different assessment of the benefits. Furthermore, only textual sources were used as a basis for the analysis. In practice, it is likely that additional sources, such as satellite imagery, will be included in the analysis. While the responses of experienced military analysts were used as a baseline, the subjects in the experimental group were all students. The evaluation of the usefulness of the AI tools can distinguish between experienced military analysts and students without professional experience. It can therefore be assumed that experienced military analysts perceive the added value of using AI tools even more strongly. It should also be noted that AI is still in a massive development phase. For example, the limitations in the use of NER identified in the study due to incorrect tagging may have been improved since the time of the study.
Finally, it should be noted that due to the design of the study, it is not possible to make statements about the AI functions individually, as their added value was only analyzed in combination. It is possible that one of the AI functions did not add value on its own, but only benefited from the added value of the others. To account for this, only three AI functions were included in the experimental study from the outset. Conceptually, these three functions provided added value to the military analysis. An additional post-hoc survey was conducted to take this into account. However, these are subjective assessments of the participants and do not allow any causal conclusions to be drawn. Therefore, a limitation of the experiment that cannot be eliminated without further research is that while the three AI functions as a whole provide significant added value, the added value of the individual functions is not necessarily given.
7 Conclusion
A demonstrator was used to test three AI functions designed to support the work of military analysts in providing the most accurate situational awareness possible. These AI functions are essentially AI functions that have been made possible by advances in the field of LLM. In addition, there is a wide range of other possible applications of AI in military analysis that could not be included in this study in the interest of brevity. A further increase in the performance of the analyzed AI functions can be expected from new developments, especially in the training of German or European LLMs. Data protection and confidentiality also play a central role in the use of artificial intelligence in military analysis. The present study has shown experimentally that the three AI functions of AI search, NER, and automated summarization in combination provide added value for military analysts. Not only does analysis performance increase, but so does the ability to make more accurate assessments. In particular, the participants in the experiment saw an advantage in the increased speed of military analysis.
Using the intelligence cycle, the AI functions were positioned within military intelligence analysis. It was found that there are numerous potential applications for the AI functions proposed here. In practice, however, it is often necessary to specify which AI functions can provide concrete support in military analysis (Cho et al., 2020). However, there are also areas of military analysis that may never be supported by AI due to ethical considerations (Blanchard and Taddeo, 2023). Hallucinated information can also be very dangerous, especially in the critical decision-making environments of military intelligence. If the LLM draws false conclusions or misinterprets data, it could lead analysts astray. One promising approach is retrieval-augmented generation (RAG), in which the language model works with external, reliable sources in addition to its trained world knowledge (Gao et al., 2024; Ovadia et al., 2024). Domain-specific fine-tuning on curated specialist data has also proven useful to focus the model on consistent and verifiable knowledge. In addition, a downstream fact check—using hybrid AI-human workflows (Borghoff et al., 2025) or manual—will further ensure the correctness of the content. Farquhar et al. (2024) present another promising statistics-based approach, proposing entropy-based uncertainty estimators for large language models to detect a specific type of hallucination known as confabulations. Unlike traditional methods that focus on word sequences, this approach measures uncertainty at the semantic level, recognizing that a single idea can be conveyed through different phrasings.
For the practical implementation of these principles in deepCOM, it was shown that the model's semantic understanding allows for the targeted omission of individual parts of sentences without compromising the coherence of the content. Previous approaches in which the AI formulated the content in “its own words” led to a greater tendency to hallucinate, as additional world knowledge from the training data was incorporated. The selective abbreviation method favored in deepCOM therefore proved to be more robust.
Future research could complement the present study in that the added value of AI in supporting analysis also applies to the application of other AI functions. Extending the findings to different analysis scenarios could also contribute to the generalizability of the results. In the future, the necessity for trust and transparency in AI systems, particularly in the context of military applications, highlights the requirement for well-defined methodologies to address the “black box” nature of generative AI. If analysts don't trust what the AI has to say, they may choose to ignore its recommendations, even if they are right.
Costa and Pedreira (2023), Seidel et al. (2018), and Seidel and Borghoff (2025) emphasize that symbolic models such as decision trees, finite state machines (used for classification or as transducers), and behavior trees can effectively represent the underlying decision logic of the (convolutional) neural networks of LLMs. Among these options, decision trees emerge as the most suitable symbolic equivalent, thanks to their compatibility with internal network processes, ease of interpretation, and strong alignment with the goals of Explainable AI (XAI) (Dwivedi et al., 2023). The provision of such clarity will facilitate a more nuanced understanding and trust in AI decisions and the “rational” actions they generate among stakeholders, including customers, developers, and regulators. While not a prerequisite in the transparent study of well-understood documents, the application of XAI becomes imperative in more intricate, real-world scenarios. XAI enhances the interpretability and user-friendliness of AI systems, fosters trust in their decision-making processes, and aligns development with ethical and regulatory standards (Chamola et al., 2023).
Data availability statement
All relevant data are included in the article. The datasets analyzed for this study can be found in the Supplementary material. Further inquiries can be directed to the corresponding author.
Ethics statement
The study involving humans was approved by the Ethics Committee at University of the Bundeswehr Munich under Ethics Committee Approval - EK UniBw M 24-68. Written informed consent from the participants was not required to participate in this study in accordance with the national legislation and the institutional requirements. Furthermore, all procedures were carried out in full compliance with the General Data Protection Regulation (GDPR).
Author contributions
CN: Writing – original draft, Writing – review & editing. AC: Writing – original draft, Writing – review & editing. SK: Writing – original draft, Writing – review & editing. UB: Writing – original draft, Writing – review & editing.
Funding
The author(s) declare that financial support was received for the research and/or publication of this article. Open access funding provided by the University of the Bundeswehr Munich.
Acknowledgments
A preprint of this article has been published on arXiv by Nitzl et al. (2024b). The authors would like to acknowledge the use of DEEPL TRANSLATOR and DEEPL WRITE in the preparation of this paper. These tools assisted in enhancing the language quality and translating certain sections originally written in German. Naturally, the content remains a faithful reflection of the authors' original research and intellectual contributions.
Conflict of interest
The authors declare that the research was conducted in the absence of any commercial or financial relationships that could be construed as a potential conflict of interest.
Generative AI statement
The author(s) declare that no Gen AI was used in the creation of this manuscript.
Publisher's note
All claims expressed in this article are solely those of the authors and do not necessarily represent those of their affiliated organizations, or those of the publisher, the editors and the reviewers. Any product that may be evaluated in this article, or claim that may be made by its manufacturer, is not guaranteed or endorsed by the publisher.
Supplementary material
The Supplementary Material for this article can be found online at: https://www.frontiersin.org/articles/10.3389/fhumd.2025.1540450/full#supplementary-material
Footnotes
1. ^See Scheffler et al. (2016), Borghoff et al. (2024), and Berger et al. (2025) for a detailed description of intelligence programs and the German MISS.
References
Albrecht, K., Nitzl, C., and Borghoff, U. M. (2022). “Transdisciplinary software development for early crisis detection,” in Computer Aided Systems Theory - EUROCAST 2022- 18th International Conference, Las Palmas de Gran Canaria, Spain, Feb 20–25, 2022, Revised Selected Papers, Lecture Notes in Computer Science 13789 (Springer), 3–10. doi: 10.1007/978-3-031-25312-6_1
Berger, L., Borghoff, U. M., Conrad, G., and Pickl, S. (2025). Intelligence education made in Europe: critical reflections on the German experience. Int. J. Intell. CounterIntell. 2025, 1–20. doi: 10.1080/08850607.2025.2460940
Blanchard, A., and Taddeo, M. (2023). The ethics of artificial intelligence for intelligence analysis: a review of the key challenges with recommendations. Digital Soc. 2:12. doi: 10.1007/s44206-023-00036-4
Bohne, T., Rönnau, S., and Borghoff, U. M. (2011). “Efficient keyword extraction for meaningful document perception,” in Proceedings of the 2011 ACM Symposium on Document Engineering, Mountain View, CA, USA, Sep 19–22, 2011, eds. M. R. B. Hardy, and F. W. Tompa (New York: ACM), 185–194. doi: 10.1145/2034691.2034732
Borghoff, U. M., Berger, L., and Fischer, F. (2024). The intelligence college in Europe: an effort to create a European intelligence community. Connections 23, 1–13. doi: 10.11610/Connections.23.1.03
Borghoff, U. M., Bottoni, P., and Pareschi, R. (2025). Human-artificial interaction in the age of agentic AI: a system-theoretical approach. Front. Hum. Dyn. 7:1579166. doi: 10.3389/fhumd.2025.1579166
Chamola, V., Hassija, V., Sulthana, A. R., Ghosh, D., Dhingra, D., and Sikdar, B. (2023). A review of trustworthy and explainable artificial intelligence (XAI). IEEE Access 11, 78994–79015. doi: 10.1109/ACCESS.2023.3294569
Cho, S., Shin, W., Kim, N., Jeong, J., and In, H. P. (2020). Priority determination to apply artificial intelligence technology in military intelligence areas. Electronics 9:2187. doi: 10.3390/electronics9122187
Clark, R. M. (2019). Intelligence Analysis: A Target-Centric Approach. California, USA: CQ Press, SAGE Publications.
Costa, V. G., and Pedreira, C. E. (2023). Recent advances in decision trees: an updated survey. Artif. Intell. Rev. 56, 4765–4800. doi: 10.1007/s10462-022-10275-5
Dell'Acqua, F., Ayoubi, C., Lifshitz-Assaf, H., Sadun, R., Mollick, E. R., Mollick, L., et al. (2025). The Cybernetic Teammate: A Field Experiment on Generative AI Reshaping Teamwork and Expertise. Working Paper 25–043, Harvard Business School. doi: 10.3386/w33641
Devanny J. Dylan H. and E. G. (2023). Generative AI and intelligence assessment. RUSI J. 168, 16–25. doi: 10.1080/03071847.2023.2286775
Devlin, J. (2018). Bert: Pre-training of deep bidirectional transformers for language understanding. preprint arXiv:1810.04805.
Dwivedi, R. (2023). Explainable AI (XAI): core ideas, techniques, and solutions. ACM Comput. Surv. 55, 1–33. doi: 10.1145/3561048
EU (2024). Regulation (EU) 2024/... of the European Parliament and of the Council on Harmonised Rules on Artificial Intelligence (Artificial Intelligence Act). OJ L, 2024. Final text pending publication in the Official Journal of the European Union. Available online at: https://eur-lex.europa.eu/eli/reg/2024/1689/oj/eng (accessed May 10, 2025).
Farquhar, S., Kossen, J., Kuhn, L., and Gal, Y. (2024). Detecting hallucinations in large language models using semantic entropy. Natural 630, 625–630. doi: 10.1038/s41586-024-07421-0
Floridi, L. (2019). Translating principles into practices of digital ethics. Philos. Technol. 32, 185–193. doi: 10.1007/s13347-019-00354-x
Gao, Y., Xiong, Y., Gao, X., Jia, K., Pan, J., Bi, Y., et al. (2024). Retrieval-augmented generation for large language models: a survey. preprint arXiv:2312.10997.
Gorman, J. C., Cooke, N. J., and Winner, J. L. (2017). “Measuring team situation awareness in decentralized command and control environments,” in Situational Awareness (London: Routledge), 183–196. doi: 10.4324/9781315087924-11
Häffner, S., Hofer, M., Nagl, M., and Walterskirchen, J. (2023). Introducing an interpretable deep learning approach to domain-specific dictionary creation: a use case for conflict prediction. Polit. Anal. 31, 481–499. doi: 10.1017/pan.2023.7
Hare, N., and Coghill, P. (2016). The future of the intelligence analysis task. Intell. Nat. Secur. 31, 858–870. doi: 10.1080/02684527.2015.1115238
Heuer, R. J. (1999). Psychology of Intelligence Analysis. Reston: Center for the Study of Intelligence.
Horlings, T. (2023). Dealing with data: coming to grips with the information age in intelligence studies journals. Intell. Nat. Secur. 38, 447–469. doi: 10.1080/02684527.2022.2104932
Hulnick, A. S. (2006). What's wrong with the intelligence cycle. Intell. Nat. Secur. 21, 959–979. doi: 10.1080/02684520601046291
Johnson, L. K. (1986). Making the intelligence “cycle” work. Int. J. Intell. Count. Intell. 1, 1–23. doi: 10.1080/08850608608435033
Lamsiyah, S., El Mahdaouy, A., Espinasse, B., and Ouatik, S. E. A. (2021). An unsupervised method for extractive multi-document summarization based on centroid approach and sentence embeddings. Expert Syst. Appl. 167:114152. doi: 10.1016/j.eswa.2020.114152
Lewis, J. R. (2018). The system usability scale: past, present, and future. Int. J. Hum. Comput. Interact. 34, 577–590. doi: 10.1080/10447318.2018.1455307
Li, J., Yang, Y., Liao, Q. V., Zhang, J., and Lee, Y.-C. (2025). As confidence aligns: Exploring the effect of ai confidence on human self-confidence in human-AI decision making. arXiv Preprint, abs-2501.12868.
Lowenthal, M. M. (2022). Intelligence: From Secrets to Policy. California, USA: CQ Press, SAGE Publications.
McNeese, N. J., Demir, M., Chiou, E. K., and Cooke, N. J. (2021). Trust and team performance in human-autonomy teaming. Int. J. Electr. Commer. 25, 51–72. doi: 10.1080/10864415.2021.1846854
NATO (2016). AJP-2.1 Allied joint doctrine for intelligence procedures. Available online at: https://jadl.act.nato.int/ILIAS/data/testclient/lm_data/lm_152845/Linear/JISR04222102/sharedFiles/AJP21.pdf (accessed May 10, 2025).
Nitzl, C., Cyran, A., Krstanovic, S., and Borghoff, U. M. (2024a). “The application of named entity recognition in military intelligence,” in Computer Aided Systems Theory - EUROCAST 2024- 19th International Conference, Las Palmas de Gran Canaria, Spain, February 25- March 1, 2024, Revised Selected Papers, Part I, Lecture Notes in Computer Science 15172, eds. A. Q. Arencibia, M. Affenzeller, and R. Moreno-Díaz (Springer), 15–22. doi: 10.1007/978-3-031-82949-9_2
Nitzl, C., Cyran, A., Krstanovic, S., and Borghoff, U. M. (2024b). The use of artificial intelligence in military intelligence: An experimental investigation of added value in the analysis process. preprint arXiv:2412.03610.
Ovadia, O., Brief, M., Mishaeli, M., and Elisha, O. (2024). Fine-tuning or retrieval? Comparing knowledge injection in LLMs. arXiv:2312.05934.
Perboli, G., Gajetti, M., Fedorov, S., and Giudice, S. L. (2021). Natural language processing for the identification of human factors in aviation accidents causes: an application to the shel methodology. Expert Syst. Appl. 186, 115694. doi: 10.1016/j.eswa.2021.115694
Phythian, M. (2013). Understanding the Intelligence Cycle. London: Routledge. doi: 10.4324/9780203558478
Qader, W. A., Ameen, M. M., and Ahmed, B. I. (2019). “An overview of bag of words; importance, implementation, applications, and challenges,” in IEEE International Engineering Conference (IEC), 200–204. doi: 10.1109/IEC47844.2019.8950616
Rashid, A. B., Kausik, A. K., Al Hassan Sunny, A., and Bappy, M. H. (2023). Artificial intelligence in the military: an overview of the capabilities, applications, and challenges. Int. J. Intell. Syst. 2023:8676366. doi: 10.1155/2023/8676366
Sadiku, M. N. O., and Musa, S. M. (2021). Military Intelligence. New York: Springer, 249–262. doi: 10.1007/978-3-030-77584-1_20
Sauro, J. (2011). A Practical Guide to the System Usability Scale: Background, Benchmarks &Best Practices. Denver: Measuring Usability LLC.
Scheffler, A. C., Jeraj, B., and Borghoff, U. M. (2016). The rise of intelligence studies: a model for Germany? Connections 15, 79–106. doi: 10.11610/Connections.15.1.06
Seidel, S., and Borghoff, U. M. (2025). Deriving equivalent symbol-based decision models from feedforward neural networks. arXiv Preprint, abs-2504.12446, 1–15. doi: 10.48550/arXiv.2504.12446
Seidel, S., Schimmler, S., and Borghoff, U. M. (2018). “Understanding neural network decisions by creating equivalent symbolic AI models,” in Intelligent Systems and Applications - Proceedings of the 2018 Intelligent Systems Conference, IntelliSys 2018, London, UK, September 6–7, 2018, Volume 1, number 868 in Advances in Intelligent Systems and Computing, eds. K. Arai, S. Kapoor, and R. Bhatia (Springer), 616–637. doi: 10.1007/978-3-030-01054-6_45
Svendsen, A. D. M. (2017). Intelligence Engineering: Operating Beyond the Conventional. Lanham: Rowman &Littlefield.
Vogel, K. M., Reid, G., Kampe, C., and Jones, P. (2021). The impact of AI on intelligence analysis: tackling issues of collaboration, algorithmic transparency, accountability, and management. Intell. Nat. Secur. 36, 827–848. doi: 10.1080/02684527.2021.1946952
Werro, A., Nitzl, C., and Borghoff, U. M. (2024). On the role of intelligence and business wargaming in developing foresight. preprint arXiv:2405.06957. doi: 10.48550/arXiv.2405.06957
Keywords: military intelligence, artificial intelligence, open source intelligence, analysis process, experiment
Citation: Nitzl C, Cyran A, Krstanovic S and Borghoff UM (2025) The use of artificial intelligence in military intelligence: an experimental investigation of added value in the analysis process. Front. Hum. Dyn. 7:1540450. doi: 10.3389/fhumd.2025.1540450
Received: 05 December 2024; Accepted: 28 April 2025;
Published: 21 May 2025.
Edited by:
Claire M. Mason, Commonwealth Scientific and Industrial Research Organisation (CSIRO), AustraliaReviewed by:
Haohui Chen, Commonwealth Scientific and Industrial Research Organisation (CSIRO), AustraliaJessica Irons, Commonwealth Scientific and Industrial Research Organisation (CSIRO), Australia
Copyright © 2025 Nitzl, Cyran, Krstanovic and Borghoff. This is an open-access article distributed under the terms of the Creative Commons Attribution License (CC BY). The use, distribution or reproduction in other forums is permitted, provided the original author(s) and the copyright owner(s) are credited and that the original publication in this journal is cited, in accordance with accepted academic practice. No use, distribution or reproduction is permitted which does not comply with these terms.
*Correspondence: Uwe M. Borghoff, dXdlLmJvcmdob2ZmQHVuaWJ3LmRl