- 1Department of Emergency Medicine, Massachusetts General Hospital, Harvard Medical School, Boston, MA, United States
- 2Department of Health Science, University of Yamanashi, Chuo, Yamanashi, Japan
- 3Program in Genetic Epidemiology and Statistical Genetics, Harvard T. H. Chan School of Public Health, Boston, MA, United States
- 4Department of Biostatistics, Harvard T. H. Chan School of Public Health, Boston, MA, United States
- 5Division of Pediatric Pulmonary Medicine, UPMC Children’s Hospital of Pittsburgh, University of Pittsburgh, Pittsburgh, PA, United States
- 6Center for Genetic Medicine Research, Children’s National Hospital, Washington, DC, United States
- 7Department of Pediatrics, George Washington University School of Medicine and Health Sciences, Washington, DC, United States
- 8Division of Infectious Diseases, Children’s National Hospital, Washington, DC, United States
- 9Division of Nephrology, Department of Medicine, Massachusetts General Hospital, Harvard Medical School, Boston, MA, United States
- 10Division of Emergency Medicine, Children’s National Hospital, Washington, DC, United States
Background: Infants with bronchiolitis are at high risk for developing childhood asthma. While genome-wide association studies suggest common genetic susceptibilities between these conditions, the mechanisms underlying the link remain unclear.
Objective: Through integrated genetics-metabolomics analysis in this high-risk population, we sought to identify genetically driven metabolites associated with asthma development and genetic loci associated with both these metabolites and asthma susceptibility.
Methods: In a multicenter prospective cohort study of infants hospitalized for bronchiolitis, we profiled the nasopharyngeal metabolome and genotyped the whole genome at hospitalization. We identified asthma-related metabolites from 283 measured compounds and conducted metabolite quantitative trait loci (mtQTL) analyses. We further examined the mtQTL associations by testing shared genetic loci for metabolites and asthma using colocalization analysis and the concordance between the loci and known asthma-susceptibility genes.
Results: In 744 infants hospitalized with bronchiolitis, 28 metabolites (e.g., docosapentaenoate [DPA], 1,2-dioleoyl-sn-glycero-3-phosphoglycerol, sphingomyelin) were associated with asthma risk. A total of 349 loci were associated with these metabolites—161 for non-Hispanic white, 120 for non-Hispanic black, and 68 for Hispanics. Of these, there was evidence for 30 shared loci between 16 metabolites and asthma risk (colocalization posterior probability ≥0.5). The significant SNPs within loci were aligned with known asthma-susceptibility genes (e.g., ADORA1, MUC16).
Conclusion: The integrated genetics-metabolomics analysis identified genetically driven metabolites during infancy that are associated with asthma development and genetic loci associated with both these metabolites and asthma susceptibility. Identifying these metabolites and genetic loci should advance research into the functional mechanisms of the infant bronchiolitis-childhood asthma link.
Introduction
Bronchiolitis is the leading cause of infant hospitalization in the U.S., accounting for 110,000 hospitalizations each year (1). Its chronic morbidity is also substantial. Among infants hospitalized with bronchiolitis (i.e., severe bronchiolitis), ~30% subsequently develop childhood asthma (2–6). Yet, the mechanisms underlying the bronchiolitis-asthma link remain unclear. Our limited understanding has hindered the development of asthma prevention strategies.
Asthma is a complex syndrome that is influenced by both genetic and environmental factors (e.g., early-life virus infection) (7). Metabolomics systematically profiles small molecules in a biological system, which represent the downstream functional products of these genetic and environmental interactions. Studies have suggested metabolites involved in asthma pathobiology—e.g., sphingolipids (e.g., sphingomyelins) (8, 9), phospholipids (e.g., phosphatidylglycerol [PG]) (10), and fatty acids (e.g., docosapentaenoate [DPA]) (11). In addition to metabolomics, genome-wide association studies (GWASs) have identified genetic loci for childhood asthma susceptibility (12–17). For example, ORMDL3 located at chromosome 17q21—a major regulator of sphingolipid metabolism—plays an important role in asthma development (12, 15). Metabolomics and genetics studies have independently attempted to elucidate the mechanisms underlying asthma pathobiology. However, no study has yet examined the integrated relationship of genetics, airway metabolome, and asthma development in children—let alone in infants at high risk for asthma development.
To address this knowledge gap, we applied an integrative genetics-metabolomics approach to data from a multicenter prospective cohort study of infants with severe bronchiolitis. We sought to identify the genetically driven metabolites and the genetic loci regulating those metabolites associated with the development of childhood asthma.
Materials and methods
Study design, setting, and participants
We analyzed data from the 35th Multicenter Airway Research Collaboration (MARC-35) study—a multicenter prospective cohort study (18). Details of the study design, setting, participants, data collection, testing, and statistical analysis may be found in the Supplementary Methods. Briefly, investigators enrolled 1,016 infants (age <1 year) hospitalized with attending physician-diagnosis of bronchiolitis at 17 sites across 14 U.S. states (Table S1) in 2011–2014. The diagnosis of bronchiolitis was made according to the American Academy of Pediatrics bronchiolitis guidelines (19), defined as an acute respiratory illness with a combination of rhinitis, cough, tachypnea, wheezing, crackles, or chest retractions. We excluded infants with a known heart-lung disease, immunodeficiency, immunosuppression, or gestational age of <32 weeks. All patients were treated at the discretion of the treating physicians.
Of 1,016 infants enrolled in the MARC-35 cohort, the current analysis investigated 744 infants who underwent both genotyping and nasopharyngeal metabolome profiling (Table S2). The institutional review board at each participating hospital approved the study with written informed consent obtained from the parent or guardian.
Data collection
Clinical data (patients’ demographic characteristics, medical, environmental, and family history, and details of the acute illness) were collected via structured interviews and chart reviews using a standardized protocol (8, 9). After the index hospitalization for bronchiolitis, trained interviewers began interviewing parents/legal guardians by telephone at 6-month intervals in addition to medical record review by physicians. All data were reviewed at the Emergency Medicine Network Coordinating Center at Massachusetts General Hospital (Boston, Massachusetts, USA) (18). By using a standardized protocol (8), investigators collected peripheral blood specimens (for genotyping) and nasopharyngeal specimens (for metabolome profiling) within 24 hours of hospitalization. We described the details of the data collection and measurement methods in the Supplementary Methods.
Genotyping
We used the Illumina Multi-Ethnic Genotyping Array (San Diego, California) for genotyping. For genotype imputation, we used the TOPMed reference panel on the TOPMed Imputation Server (20) and removed variants with an imputation score of <0.6 from the imputed dataset. We also removed rare variants with a minor allele frequency of <0.01 from the dataset. Subsequently, we included a total of 10,852,874 autosomal variants for the downstream association study. We described the details of genotype imputation and quality control in the Supplementary Methods.
Nasopharyngeal airway metabolome profiling
We profiled the nasopharyngeal metabolome using liquid chromatography with tandem mass spectrometry (LC-MS/MS) at Metabolon (Morrisville, North Carolina). The laboratory processed the blinded specimens in random order. Instrument variability was 4%, as determined by calculating the median relative standard deviation for the internal standards. The metabolome profiling identified 283 known metabolites from 76 sub-pathways within 7 super-pathways. We described the details of metabolome profiling in a previous study (21) and Supplementary Methods.
Clinical outcome measure
The clinical outcome of interest is the development of asthma by age 6 years. Asthma was defined using a commonly used epidemiologic definition: physician-diagnosis of asthma, with either asthma medication use (e.g., albuterol, inhaled corticosteroids) or asthma-related symptoms (e.g., wheezing, nocturnal cough) in the preceding year (22).
Statistical analysis
The objectives of the present study are (i) to identify genetically driven metabolites that are associated with the risk of developing asthma and (ii) to examine the genetic loci that are associated with both these metabolites and asthma susceptibility. The analytic workflow is summarized in Figure 1. The details of the statistical analysis may be found in the Supplementary Methods.
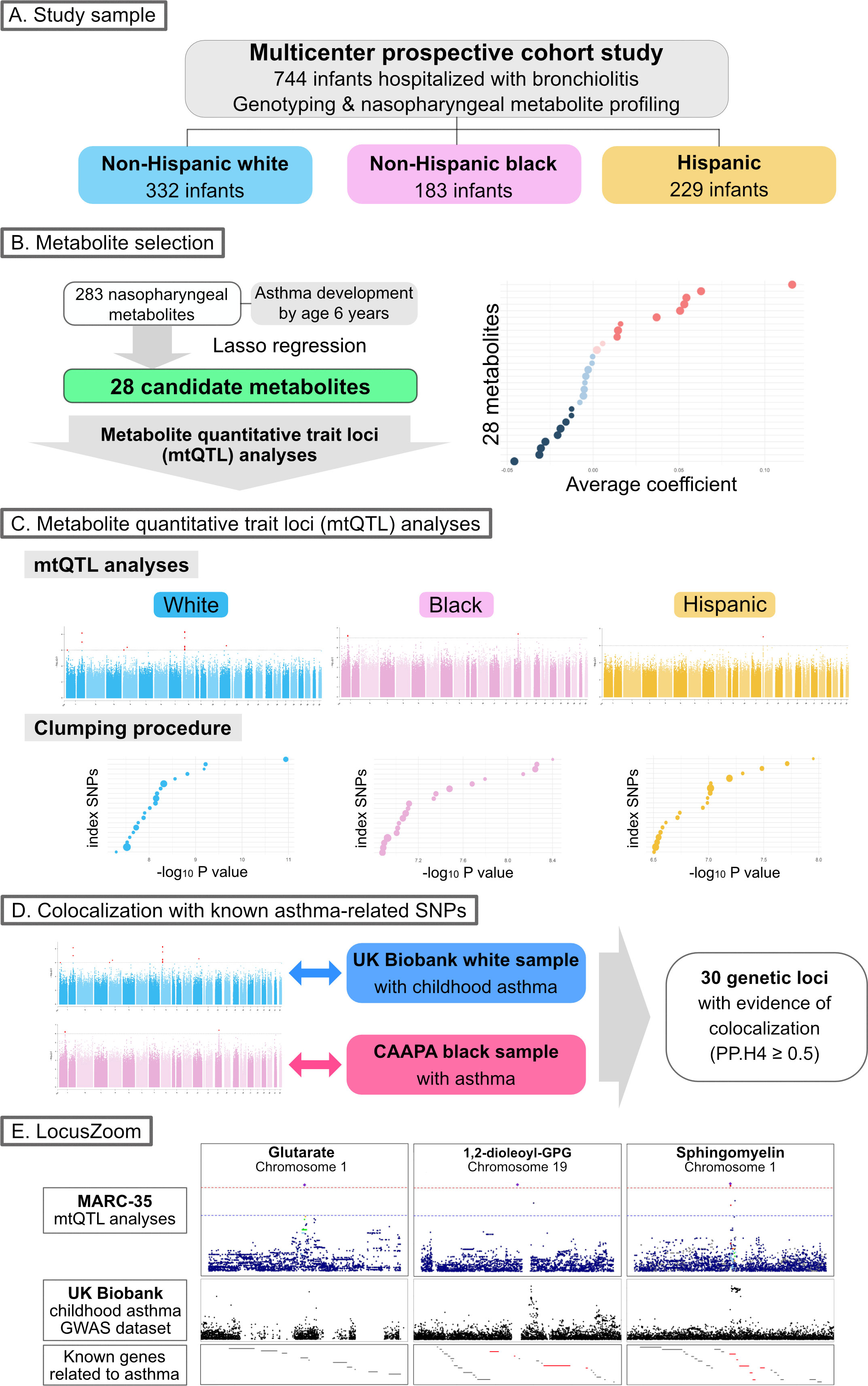
Figure 1 Analytic workflow of the study (A). Of 744 infants (age <1 year) hospitalized with bronchiolitis, the current analysis investigated 744 infants—332 (45%) were non-Hispanic white, 183 (25%) were non-Hispanic black, and 229 (31%) were Hispanics—who underwent both genotyping and nasopharyngeal metabolome profiling. (B). The association of 283 nasopharyngeal metabolites of the infants with asthma development by age 6 years was examined—by constructing Lasso regression models—to identify candidate metabolites for the downstream metabolite quantitative trait loci (mtQTL) analysis. (C). The mtQTL analysis was performed to examine the association between 28 candidate metabolites and genotypes in each of the three major racial/ethnic subgroups of infants with bronchiolitis. The clumping procedure was also conducted to determine mtQTLs that are independent of each other. (D). Bayesian colocalization analysis using summary statistics of the mtQTLs of MARC-35, UK Biobank (white sample with childhood asthma), and CAAPA (African-admixed sample with asthma) was conducted to identify shared genetic loci between the mtQTLs and the independent GWAS summary statistics for asthma. (E). The association peaks of the 30 loci with colocalization evidence were compared between the GWAS summary statistics of the mtQTL analysis of MARC-35 and the UK Biobank. The association peaks of the three loci were aligned with those in the same genetic regions of UK Biobank summary statistics for childhood asthma, and there were concordances between the peaks of the two loci and known genes related to asthma. Abbreviations: CAAPA, Consortium on Asthma among African-ancestry Populations in the Americas; GPG, glycero-3-phosphoglycerol; GWAS, genome-wide association studies; MARC, multicenter airway research collaboration; PP.H4, posterior probability of hypothesis 4; SNP, single nucleotide polymorphism.
Briefly, we first constructed logistic regression models with Lasso regularization that examines the association of the nasopharyngeal metabolome with asthma development to identify candidate metabolites for the subsequent metabolite quantitative trait loci (mtQTL) analysis. Second, we performed the mtQTL analysis with an additive linear regression genetic association analysis adjusting for age, sex, and the first two ancestry principal components to examine the association between the genotypes and candidate metabolites in each of the three major racial/ethnic samples (non-Hispanic white, non-Hispanic black, and Hispanics) in the MARC-35 cohort. The significance threshold for these analyses was set to P<1×10-6. Third, we conducted clumping procedures to determine mtQTLs that are independent of each other. Fourth, we conducted pathway analyses to examine the biological significance of the genetic loci identified in the mtQTL analysis by using all genes within the clumped region of those loci. Fifth, we conducted Bayesian colocalization analyses to examine whether genetic loci for candidate metabolites are shared with those for asthma by using the GWAS summary statistics of the mtQTL analysis from MARC-35 and those of two independent datasets—the UK Biobank (white sample with childhood asthma (23–25)) and the Consortium on Asthma among African-ancestry Populations in the Americas (CAAPA; African-admixed sample with asthma (26)). We selected variants within 500 kb of the index SNP—the SNP with the smallest (i.e., most significant) P-value in each clumped region—at each of the shared loci in the non-Hispanic white and non-Hispanic black samples, and estimated the posterior probability that the two traits (i.e., each metabolite and asthma risk) share one common causal variant (a posterior probability of hypothesis 4 [PP.H4]). We considered loci with a posterior probability of ≥0.5 to colocalize. Lastly, we visualized the index SNPs colocalized with childhood asthma-risk loci from the UK Biobank GWAS summary statistics by using LocusZoom (27).
Results
Of 1,016 infants enrolled in the MARC-35 cohort, the current study focused on 744 infants with severe bronchiolitis who underwent both genotyping and nasopharyngeal metabolome profiling. The analytic (n=744) and non-analytic (n=272) cohorts did not differ in the patient characteristics (P≥0.05; Table S2), except for the proportion of racial/ethnicity and rhinovirus infection. Of the infants in the analytic cohort, the median age was 3 (interquartile range [IQR], 2-6) months and 40% were female; 45% were non-Hispanic white, 25% were non-Hispanic black, and 31% were Hispanics (Table 1). Overall, 26% subsequently developed asthma by age 6 years (Table S3).
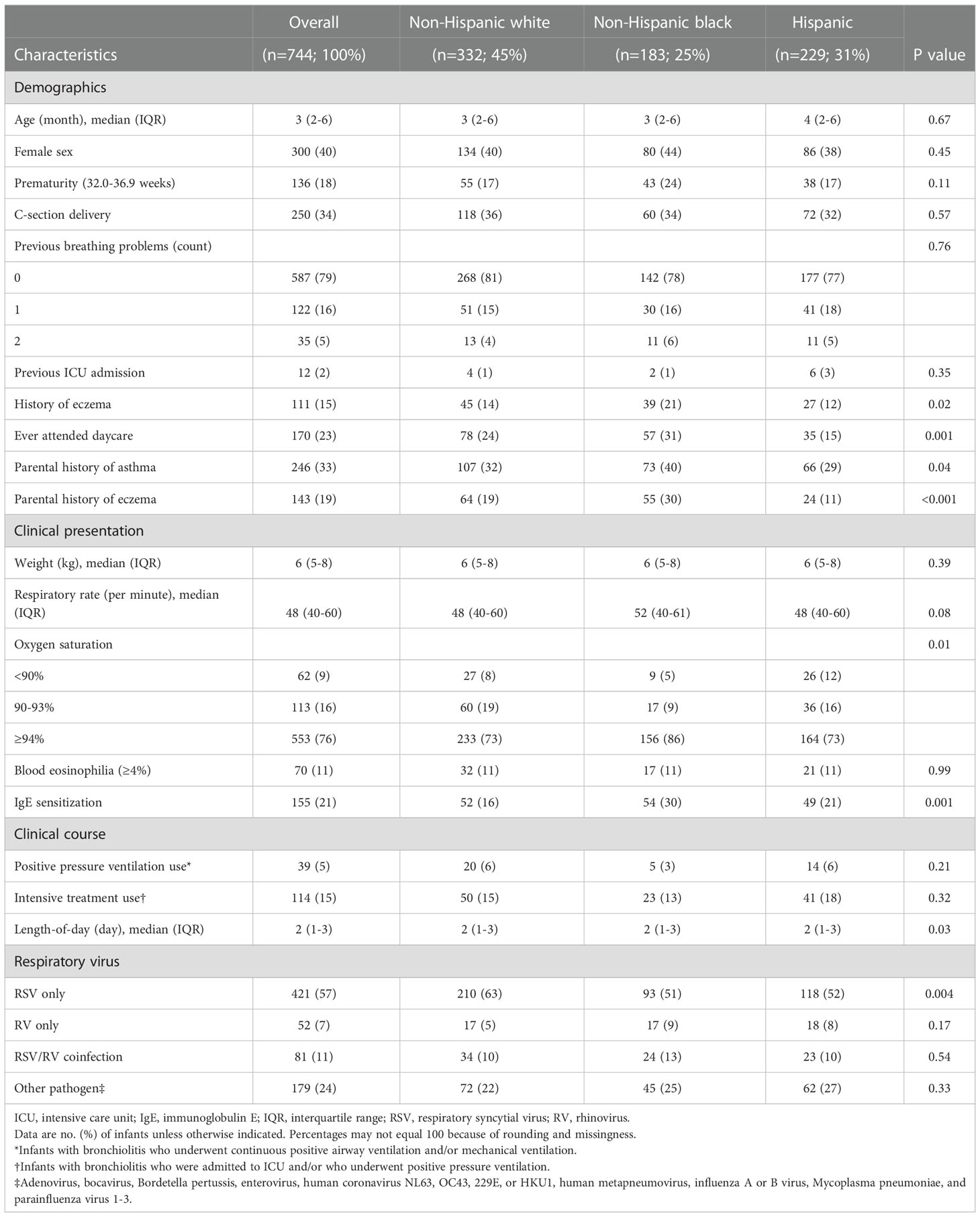
Table 1 Baseline characteristics and clinical course of 744 infants hospitalized with bronchiolitis, according to race/ethnicity.
mtQTL analysis reveals nasopharyngeal airway metabolites at infant bronchiolitis that are genetically driven
Of 283 nasopharyngeal metabolites identified in infants with bronchiolitis, 28 candidate metabolites were associated with the risk of developing asthma based on the Lasso regression models (Figure 2). Of these 28 candidate metabolites, 13 were lipids (e.g., DPA, 1,2-dioleoyl-sn-glycero-3-phosphoglycerol [GPG], glutarate, sphingomyelin [d17:1/16:0, d18:1/15:0, d16:1/17:0]), 5 were amino acids (e.g., N-acetylarginine), 4 were carbohydrates (e.g., arabitol), and 6 were other classes of metabolites.
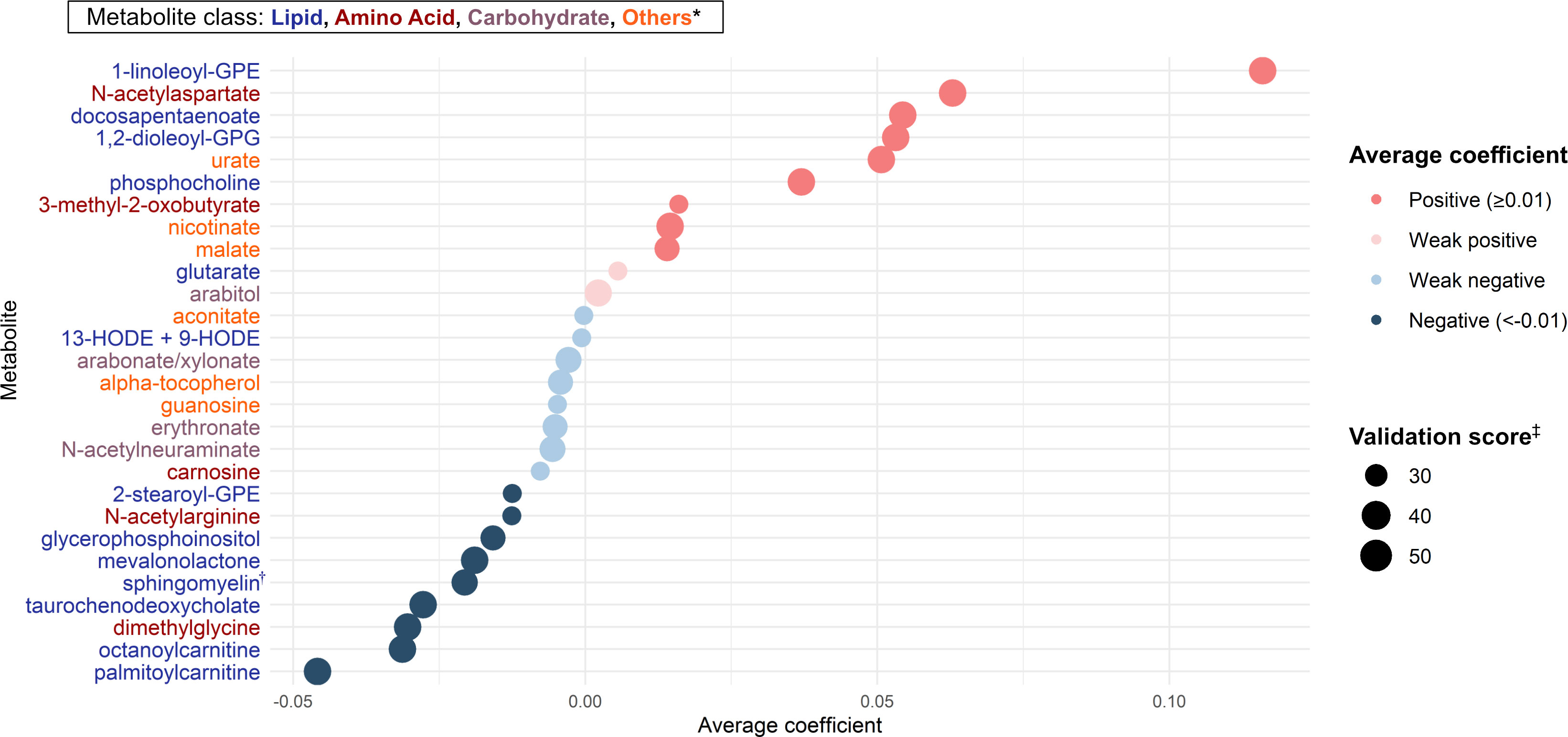
Figure 2 Metabolites selection for the mtQTL analyses By applying the Lasso regression models on nasopharyngeal metabolome (283 metabolites) data and the asthma outcome data of 744 infants hospitalized for bronchiolitis, we identified 28 candidate metabolites for the downstream mtQTL analyses. The average coefficient indicates the magnitude of the metabolite-outcome association averaged over times selected as a feature in 100 Lasso regression model executions. * Of 28 metabolites, 13 were lipids, 5 were amino acids, 4 were carbohydrates, and 6 were other classes (e.g., vitamins, nucleic acids) of metabolites. † Sphingomyelin (d17:1/16:0, d18:1/15:0, d16:1/17:0) ‡ The number of times selected as a feature of the Lasso regression model in 100 model executions. Abbreviations: GPE, glycerophosphorylethanolamine; GPG, glycero-3-phosphoglycerol; HODE, hydroxyoctadecadienoic acid.
Based on the mtQTL analysis for each of the 28 candidate metabolites, 900 SNPs were at a suggestive significance level (28) (P <1×10-6)—524 in non-Hispanic white, 259 in non-Hispanic black, and 117 in Hispanics (Figure S1 and Table S4). The clumping procedure for these SNPs showed that 349 loci were independently associated with the candidate metabolites—161 loci associated with 26 metabolites in non-Hispanic white, 120 loci with 28 metabolites in non-Hispanic black, and 68 loci with 25 metabolites in Hispanics (Figure 3, Tables S5, S6). The pathway analysis showed the biological importance of these loci with significant pathways (FDR<0.05; Figure S2), which are relevant to both bronchiolitis and asthma development—e.g., interferon-α/β (29), tumor necrosis factor receptor-associated factor 6 (TRAF6) mediated interferon regulatory factor 7 (IRF7) activation pathways (30).
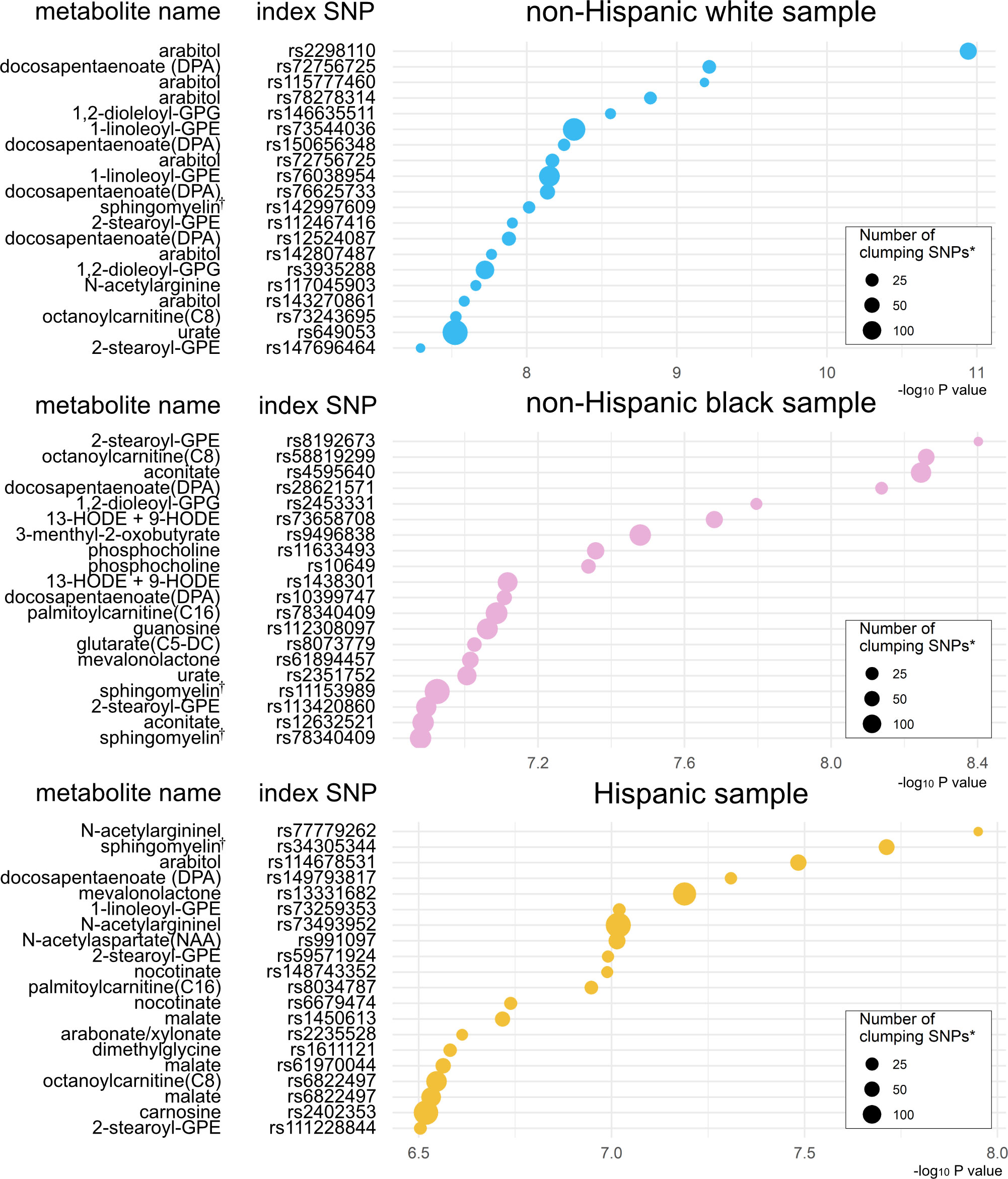
Figure 3 Significant index SNPs identified in metabolite quantitative trait loci (mtQTL) analyses The 20 index SNPs with the lowest P-values associated with the candidate metabolites were identified in the mtQTL analysis of each racial/ethnic sample after the clumping procedure. * Variants with a P value of <1 × 10−3, R2 of ≥0.2, and <500 kb away from the index SNP were assigned to the clump. † Sphingomyelin (d17:1/16:0, d18:1/15:0, d16:1/17:0) Abbreviations: GPE, glycerophosphorylethanolamine; GPG, glycero-3-phosphoglycerol; HODE, hydroxyoctadecadienoic acid.
Colocalization analysis demonstrates 30 genetic loci associated with both metabolites and asthma-susceptibility
To test for genetic loci that are in common between the candidate metabolites and asthma risk, colocalization analyses were performed for the 281 loci identified in the clumping procedure—161 in non-Hispanic white and 120 in non-Hispanic black—by comparing the GWAS summary statistics of the mtQTL analysis in MARC-35 to two independent datasets—the UK Biobank and CAAPA (Table S5 and Figure S3). There was evidence for 30 shared genetic loci between 16 metabolites and asthma risk (PP.H4 ≥0.5; Table 2)—27 loci in UK Biobank (e.g., chromosome 6q26 with DPA, chromosome 1q21 with glutarate, chromosome 1q32 with sphingomyelin [d17:1/16:0, d18:1/15:0, d16:1/17:0]), 1 locus in CAAPA (chromosome 6q12 with N-acetylarginine), and 2 loci with both datasets (e.g., chromosome 14q31 with 1,2-dioleoyl-GPG).
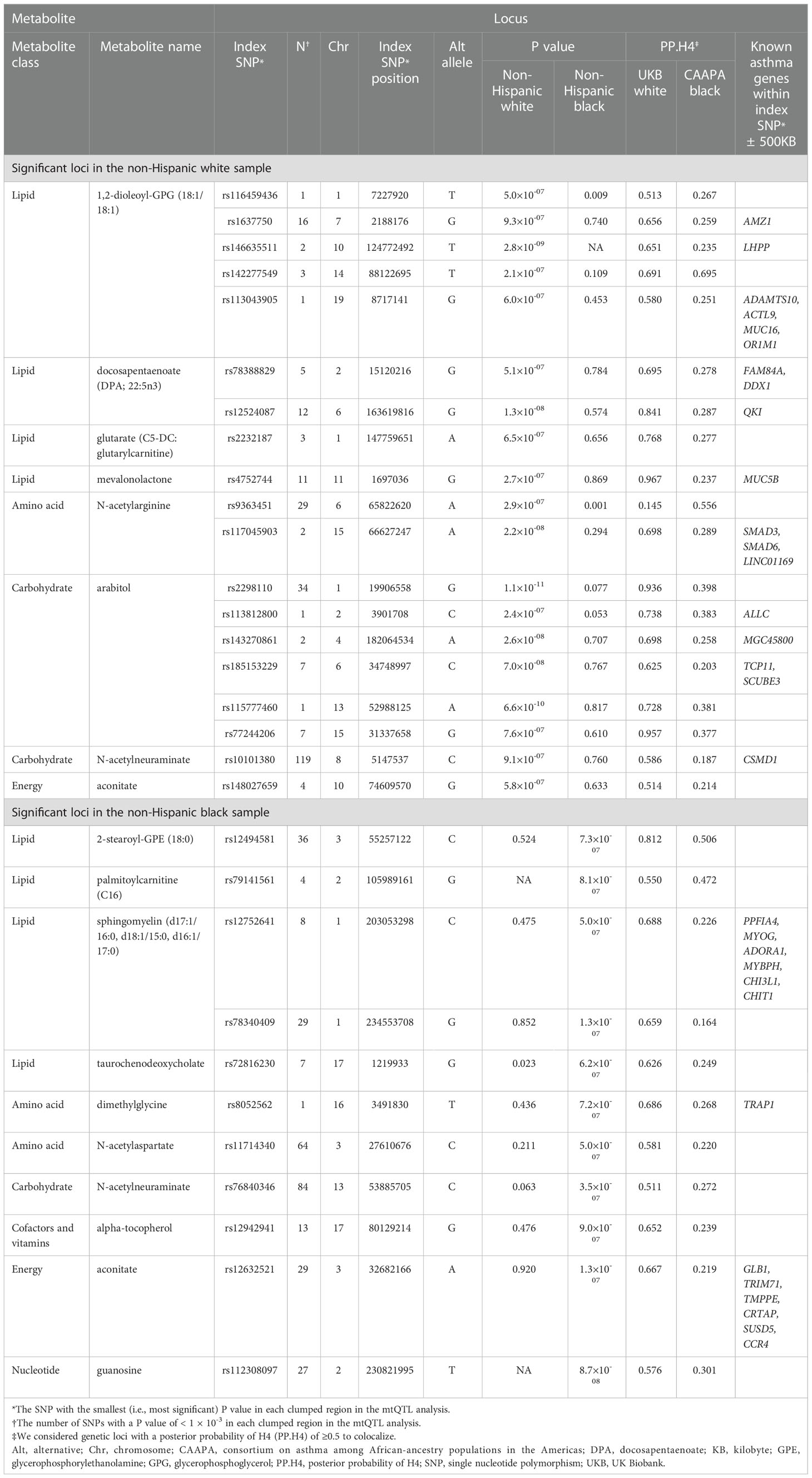
Table 2 Summary of the 30 genetic loci associated with both the candidate metabolites and asthma risk.
Genetic loci are concordant to known asthma-susceptibility genes
The association peaks for the 30 loci with colocalization evidence were compared between the mtQTL analysis and UK Biobank (Figure S4). For example, the association peaks of glutarate on chromosome 1q21 (e.g., rs2232187), 1,2-dioleoyl-GPG on chromosome 19p13 (e.g., rs113043905), and sphingomyelin [d17:1/16:0, d18:1/15:0, d16:1/17:0] on chromosome 1q32 (e.g., rs12752641) were aligned with the association peak in the same genetic region from the UK Biobank statistics of childhood asthma. Furthermore, there were apparent concordances between the association peaks for two loci (i.e., chromosome 19p13 with 1,2-dioleoyl-GPG, chromosome 1q32 with sphingomyelin [d17:1/16:0, d18:1/15:0, d16:1/17:0]) and genes that are known to be related to asthma (e.g., MUC16, ADORA1; Figure 4).
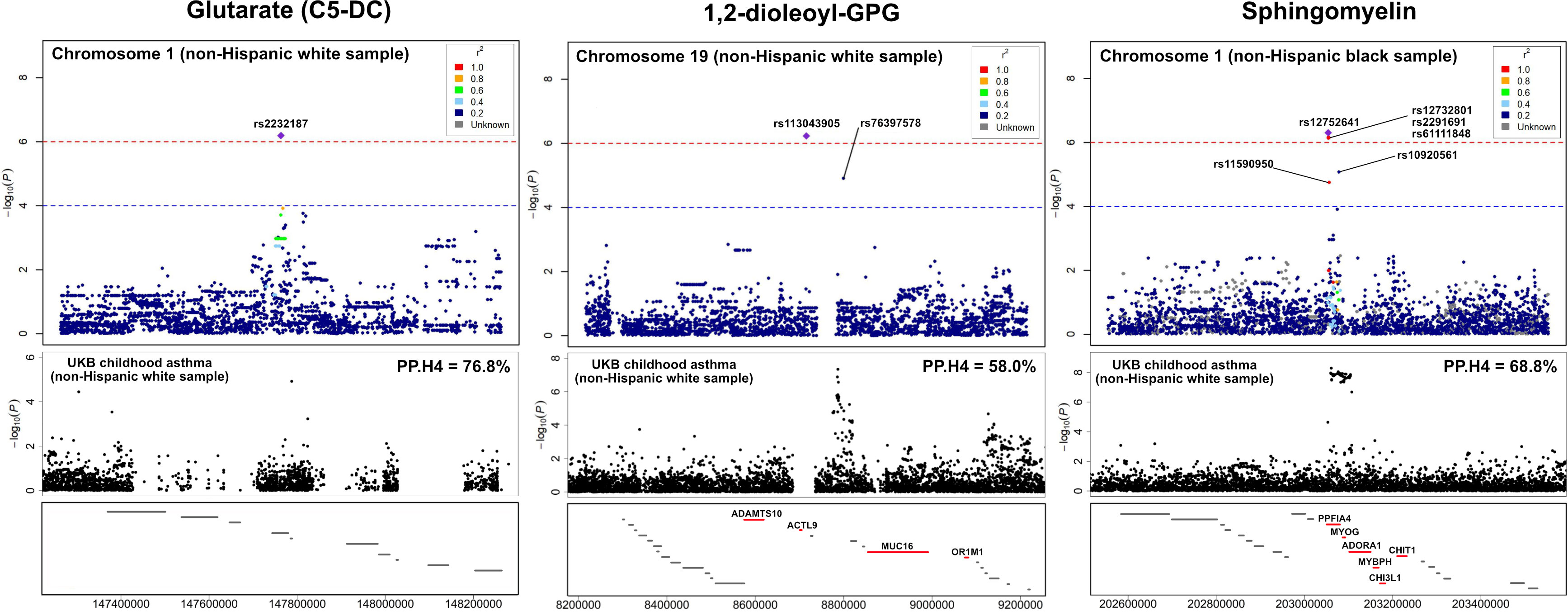
Figure 4 LocusZoom for the genetic loci with the UK Biobank summary statistics of childhood asthma To compare the association peaks of the loci between the mtQTL analysis in infants with bronchiolitis and the UK Biobank summary statistics for childhood asthma, we applied LocusZoom to glutarate (C5-DC: glutarylcarnitine) on chromosome 1q21, 1,2-dioleoyl-GPG on chromosome 19p13, and sphingomyelin [d17:1/16:0, d18:1/15:0, d16:1/17:0] on chromosome 1q32. The association peaks of these three loci were aligned with those in the same genetic regions of UK Biobank summary statistics for childhood asthma. The red lines at the bottom boxes represent the genetic location of the genes that are known to be related to asthma. There were concordances between the association peaks of two loci (i.e., chromosome 19p13 with 1,2-dioleoyl-GPG, chromosome 1q32 with sphingomyelin) and known asthma genes (e.g., ADORA1, MUC16). Abbreviations: GPG, glycero-3-phosphoglycerol; PP.H4, posterior probability of hypothesis 4; UKB, UK Biobank.
Discussion
By applying an integrated genetics-metabolomics approach to multicenter prospective cohort data of 744 infants with severe bronchiolitis, we identified 28 metabolites associated with asthma development and 349 independent genetic loci associated with these metabolites. Additionally, of these loci, colocalization analysis (with independent GWAS datasets for asthma) revealed 30 shared loci between 16 metabolites and asthma risk. Furthermore, the significant SNPs within two loci were aligned with known asthma-susceptibility genes (e.g., ADORA1, MUC16). To the best of our knowledge, this is the first study that has investigated infant bronchiolitis-childhood asthma link with an integrative genetics-metabolomics approach and demonstrated genetically driven metabolites and related genetic loci associated with asthma risk.
Childhood asthma is a heterogeneous syndrome that results from complex interactions between genetic and environmental factors in early childhood (7). Recent research has studied the mechanisms by applying high-throughput approaches to survey the metabolome—the downstream functional products of a child’s genetic make-up and environmental exposures (e.g., virus respiratory infection) (21, 31–40). Consistent with our findings, for example, recent studies of infants with acute respiratory infection have reported associations of the upper airway (21, 34–38), serum (36, 38), and urine (39) metabolome signature (e.g., altered sphingolipid, phospholipid, and fatty acid metabolism)—with the subsequent development of asthma. Independent from these metabolomics investigations, GWASs have identified genetic regions associated with respiratory syncytial virus (RSV) infection (41–43), severe bronchiolitis (41, 42, 44), and asthma risk (12–17, 43, 45–49). For example, multiple studies have identified ORMDL3 as an asthma susceptibility gene (12, 15, 46, 48) with asthma risk. ORMDL3 is a major regulator of serine palmitoyltransferase—the rate-limiting enzyme of sphingolipid biosynthesis (50). While most research independently have applied genetics and metabolomics, few studies have examined the integrated relationships of genetic variants and altered metabolism with prevalent asthma in adults (51, 52). For example, Johnson et al. recently conducted an mtQTL analysis of 348 adults (59 with prevalent asthma) from Tangier Island and found several serum metabolites (e.g., linoleoyl ethanolamide) associated with asthma risk (51). Our multicenter prospective study—integrating the genome and nasopharyngeal airway metabolome data from high-risk infants—corroborates these earlier reports, and extends them by demonstrating genetic loci and genetically driven metabolites associated with the risk of developing asthma.
The exact mechanisms underlying the observed relationship of genetic loci and metabolites—e.g., genes within chromosome 1q32 (e.g., ADORA1, PPFIA4) and sphingomyelin (d17:1/16:0, d18:1/15:0, d16:1/17:0)—with asthma risk warrant further clarification. Studies have shown that genes on chromosome 1q32, including ADORA1 and PPFIA4 are associated with asthma risk (13, 53, 54), and ADORA1 may interact with sphingolipids to enhance airway inflammation (55–59). For example, adenosine receptor A1 (ADORA1) encoded by the ADORA1 gene contributes to bronchoconstriction, mucus secretion, and inflammation in bronchial epithelial cells through the sphingolipid signaling pathway (55, 56). Experimental studies have also shown that ADORA1 regulated cyclic adenosine monophosphate (cAMP) and sphingomyelin-derived lipids to mobilize intracellular calcium stores in bronchial smooth muscle cells, leading to the contraction of the bronchial smooth muscles and airway remodeling (57–59). Sphingolipids are not only integrated components of the human cell membrane (60) but also have molecular signaling functions with roles in the immune response to infections, inflammation, and cell proliferation, thereby contributing to asthma pathobiology (61). Accordingly, studies have suggested ADORA1 (62) and sphingolipids (63) as therapeutic targets for asthma.
In addition to sphingomyelins, we also observed a relationship between genes on chromosome 19p13 (e.g., MUC16), 1,2-dioleoyl-GPG, and asthma risk. 1,2-dioleoyl-GPG is one of the phosphatidylglycerols (PGs)—a main component of pulmonary surfactant (64). An experimental study has suggested that PG inhibits proinflammatory protein expression in alveolar macrophages through downregulation of NF-κB activation (65, 66). In contrast, the depletion of pulmonary surfactant PG leads to asthma-associated surfactant dysfunction (10). Another study has also reported that PG inhibits RSV infection by blocking viral attachment to epithelial cells (67). Besides, Mucin-16—also known as CA125 and encoded regulated by the MUC16 gene—is the largest membrane-associated mucin synthesized in the endoplasmic reticulum (ER) of the bronchial epithelial cells (68–70). Research has demonstrated that the expression of mucin-16 was promoted by NF-κB activation and protects against ER stress (71). ER stress regulates proinflammatory signaling in epithelial cells through pulmonary surfactant dysfunction that is also induced by PG depletion (72). Accordingly, MUC16 gene, mucin-16, and GPG jointly play roles in ER stress (73). Notwithstanding the complexity of these potential mechanisms, the identification of genetically driven metabolites associated with the development of childhood asthma is an important finding. Our data—in conjunction with the literature—should advance further research into the pathobiological mechanisms underlying the bronchiolitis-asthma link.
The current study has several potential limitations. First, the study did not have “healthy controls.” Yet, the objective of the study was not to identify the genetic loci and metabolites related to incident bronchiolitis (i.e., bronchiolitis yes vs. no) but to investigate the functional consequences of genetic risk factors for asthma (i.e., genetically driven metabolites) in this high-risk population. Second, compared to the non-analytic cohort, the analytic cohort had an overrepresentation of solo rhinovirus infection (3% vs. 7%), which might have led to selection bias. Third, it is possible that asthma diagnosis is misclassified and that some children are going to develop asthma at a later age. To address these potential limitations, the cohort is currently being followed up to age 9 years. In addition, in the current study, children who had asthma-related symptoms but did not receive asthma medication might have been over-diagnosed with asthma. Fourth, the sample size of the current analysis was relatively small, partially because of the stratified analysis across the different racial/ethnic subgroups. To accommodate the limited statistical power, we performed the mtQTL analyses with the significance threshold (P <1×10-6) proposed in a previous study (28). The statistical power of the analyses calculated using the GAS Power Calculator (74) was 0.72 in non-Hispanic white, 0.71 in non-Hispanic black, and 0.71 in Hispanics. Fifth, the CAAPA dataset provided summary statistics for an African-admixed population with asthma (26). Therefore, the sample from the other racial/ethnic groups might have affected the colocalization estimates of our non-Hispanic black sample due to population stratification (75). Sixth, the lack of publicly available asthma GWAS data in the Hispanic sample precluded us from conducting colocalization analysis for our Hispanic sample. Lastly, our inferences may not be generalizable to infants without severe bronchiolitis (i.e., infants with mild-to-moderate bronchiolitis). Nonetheless, our observations remain directly relevant to the 110,000 infants hospitalized annually in the U.S (1).—a large population with a substantial morbidity burden.
Conclusions
By integrating the genetics and nasopharyngeal airway metabolomics data from a multicenter, prospective cohort study of infants hospitalized for bronchiolitis, we identified genetically driven metabolites (e.g., 1,2-dioleoyl-GPG, sphingomyelin) associated with asthma development and genetic loci associated with both these metabolites and asthma susceptibility genes (e.g., ADORA1, MUC16). These associations were also confirmed by colocalization analyses with independent GWAS datasets for asthma. Identifying these metabolites and genetic loci should advance research into the functional consequences of genetic risk factors for the development of asthma. Furthermore, these findings will, in turn, accelerate the understanding of the bronchiolitis-asthma link and the development of prevention strategies for childhood asthma.
Data availability statement
The data presented in the study are deposited in the ImmPort repository, accession number SDY2157.
Ethics statement
The studies involving human participants were reviewed and approved by Alfred I. duPont Hospital for Children, Wilmington, DE; Arnold Palmer Hospital for Children, Orlando, FL; Boston Children’s Hospital, Boston, MA; Children’s Hospital of Los Angeles, Los Angeles, CA; Children’s Hospital of Philadelphia, Philadelphia, PA; Children’s Hospital of Pittsburgh, Pittsburgh, PA; The Children’s Hospital at St. Francis, Tulsa, OK; The Children’s Mercy Hospital & Clinics, Kansas City, MO; Children’s National Medical Center, Washington, D.C.; Cincinnati Children’s Hospital and Medical Center, Cincinnati, OH; Connecticut Children’s Medical Center, Hartford, CT; Dell Children’s Medical Center of Central Texas, Austin, TX; Norton Children’s Hospital, Louisville, KY; Massachusetts General Hospital, Boston, MA; Phoenix Children’s Hospital, Phoenix, AZ; Seattle Children’s Hospital, Seattle, WA; Texas Children’s Hospital, Houston, TX. Written informed consent to participate in this study was provided by the participants’ legal guardian/next of kin.
Author contributions
TO carried out the main statistical analysis, drafted the initial manuscript, and approved the final manuscript as submitted. ZZ carried out the data processing, developed the methodology, analyzed and interpreted data, reviewed and revised the initial manuscript, and approved the final manuscript as submitted. LL, JC, BH, AH, ER, and RF collected the study data, reviewed and revised the initial manuscript, and approved the final manuscript as submitted. CC and KH conceptualized the study, obtained funding, supervised the statistical analysis, reviewed and revised the initial manuscript, and approved the final manuscript as submitted. All authors contributed to the article and approved the submitted version.
Funding
This study was supported by grants (UG3/UH3 OD-023253, R01 AI-127507, R01 AI-134940, R01 AI-137091, R01 AI-148338, and K01 AI-153558) from the National Institutes of Health (Bethesda, MD). The content of this manuscript is solely the responsibility of the authors and does not necessarily represent the official views of the National Institutes of Health. The funding organization was not involved in the collection, management, or analysis of the data; preparation or approval of the manuscript; or decision to submit the manuscript for publication.
Acknowledgments
We thank the MARC-35 study hospitals and research personnel for their ongoing dedication to bronchiolitis and asthma research (see Table S1). This research has been conducted using the UK Biobank Resource under application number 88976. We would like to thank the participants and researchers from UK Biobank who significantly contributed or collected data. We thank the CAAPA consortium for providing GWAS summary statistic data.
Conflict of interest
JC received research materials from Merck Rahway, NJ in order to provide medications free of cost to participants in an NIH-funded study, unrelated to the current work.
The remaining authors declare that the research was conducted in the absence of any commercial or financial relationships that could be constructed as a potential conflict of interest.
Publisher’s note
All claims expressed in this article are solely those of the authors and do not necessarily represent those of their affiliated organizations, or those of the publisher, the editors and the reviewers. Any product that may be evaluated in this article, or claim that may be made by its manufacturer, is not guaranteed or endorsed by the publisher.
Supplementary material
The Supplementary Material for this article can be found online at: https://www.frontiersin.org/articles/10.3389/fimmu.2022.1111723/full#supplementary-material
Abbreviations
ADORA1, adenosine receptor A1; CAAPA, Consortium on Asthma among African-ancestry Populations in the Americas; cAMP, cyclic adenosine monophosphate; DPA, docosapentaenoate; ER, endoplasmic reticulum; FDR, false discovery rate; GWAS, genome-wide association studies; GPE, glycerophosphorylethanolamine; GPG, glycero-3-phosphoglycerol; IL, interleukin; IRF7, interferon regulatory factor 7; MARC, multicenter airway research collaboration; mtQTL, metabolite quantitative trait loci; NF-κB, nuclear factor-κB; PG, phosphatidylglycerol; PP.H4, posterior probability of hypothesis 4; RSV, respiratory syncytial virus; SNP, single nucleotide polymorphisms; TRAF6, tumor necrosis factor receptor associated factor 6.
References
1. Fujiogi M, Goto T, Yasunaga H, Fujishiro J, Mansbach JM, Camargo CA Jr, et al. Trends in bronchiolitis hospitalizations in the united states: 2000-2016. Pediatrics (2019) 144:e20192614. doi: 10.1542/peds.2019-2614
2. Régnier SA, Huels J. Association between respiratory syncytial virus hospitalizations in infants and respiratory sequelae: systematic review and meta-analysis. Pediatr Infect Dis J (2013) 32:820–6. doi: 10.1097/INF.0b013e31829061e8
3. Koponen P, Helminen M, Paassilta M, Luukkaala T, Korppi M. Preschool asthma after bronchiolitis in infancy. Eur Respir J (2012) 39:76–80. doi: 10.1183/09031936.00040211
4. Törmänen S, Lauhkonen E, Riikonen R, Koponen P, Huhtala H, Helminen M, et al. Risk factors for asthma after infant bronchiolitis. Allergy. (2018) 73:916–22. doi: 10.1111/all.13347
5. Carroll KN, Wu P, Gebretsadik T, Griffin MR, Dupont WD, Mitchel EF, et al. The severity-dependent relationship of infant bronchiolitis on the risk and morbidity of early childhood asthma. J Allergy Clin Immunol (2009) 123:1055–61,1061.e1. doi: 10.1016/j.jaci.2009.02.021
6. Henderson J, Hilliard TN, Sherriff A, Stalker D, Al Shammari N, Thomas HM. Hospitalization for RSV bronchiolitis before 12 months of age and subsequent asthma, atopy and wheeze: a longitudinal birth cohort study. Pediatr Allergy Immunol (2005) 16:386–92. doi: 10.1111/j.1399-3038.2005.00298.x
7. Hernandez-Pacheco N, Kere M, Melén E. Gene-environment interactions in childhood asthma revisited; expanding the interaction concept. Pediatr Allergy Immunol (2022) 33:e13780. doi: 10.1111/pai.13780
8. Stewart CJ, Mansbach JM, Wong MC, Ajami NJ, Petrosino JF, Camargo CA Jr, et al. Associations of nasopharyngeal metabolome and microbiome with severity among infants with bronchiolitis. A multiomic analysis. Am J Respir Crit Care Med (2017) 196:882–91. doi: 10.1164/rccm.201701-0071OC
9. Stewart CJ, Mansbach JM, Ajami NJ, Petrosino JF, Zhu Z, Liang L, et al. Serum metabolome is associated with the nasopharyngeal microbiota and disease severity among infants with bronchiolitis. J Infect Dis (2019) 219:2005–14. doi: 10.1093/infdis/jiz021
10. Hite RD, Seeds MC, Bowton DL, Grier BL, Safta AM, Balkrishnan R, et al. Surfactant phospholipid changes after antigen challenge: a role for phosphatidylglycerol in dysfunction. Am J Physiol Lung Cell Mol Physiol (2005) 288:L610–7. doi: 10.1152/ajplung.00273.2004
11. Adams S, Lopata AL, Smuts CM, Baatjies R, Jeebhay MF. Relationship between serum omega-3 fatty acid and asthma endpoints. Int J Environ Res Public Health (2019) 16(1):43. doi: 10.3390/ijerph16010043
12. Moffatt MF, Kabesch M, Liang L, Dixon AL, Strachan D, Heath S, et al. Genetic variants regulating ORMDL3 expression contribute to the risk of childhood asthma. Nature. (2007) 448:470–3. doi: 10.1038/nature06014
13. Pividori M, Schoettler N, Nicolae DL, Ober C, Im HK. Shared and distinct genetic risk factors for childhood-onset and adult-onset asthma: genome-wide and transcriptome-wide studies. Lancet Respir Med (2019) 7:509–22. doi: 10.1016/S2213-2600(19)30055-4
14. Cookson W, Moffatt M, Strachan DP. Genetic risks and childhood-onset asthma. J Allergy Clin Immunol (2011) 128:266–72. doi: 10.1016/j.jaci.2011.06.026
15. Ferreira MAR, Mathur R, Vonk JM, Szwajda A, Brumpton B, Granell R, et al. Genetic architectures of childhood- and adult-onset asthma are partly distinct. Am J Hum Genet (2019) 104:665–84. doi: 10.1016/j.ajhg.2019.02.022
16. Bønnelykke K, Sleiman P, Nielsen K, Kreiner-Møller E, Mercader JM, Belgrave D, et al. A genome-wide association study identifies CDHR3 as a susceptibility locus for early childhood asthma with severe exacerbations. Nat Genet (2014) 46:51–5. doi: 10.1038/ng.2830
17. Eliasen AU, Pedersen CET, Rasmussen MA, Wang N, Soverini M, Fritz A, et al. Genome-wide study of early and severe childhood asthma identifies interaction between CDHR3 and GSDMB. J Allergy Clin Immunol (2022) 150(3):622–30. doi: 10.1016/j.jaci.2022.03.019
18. Hasegawa K, Mansbach JM, Bochkov YA, Gern JE, Piedra PA, Bauer CS, et al. Association of rhinovirus c bronchiolitis and immunoglobulin e sensitization during infancy with development of recurrent wheeze. JAMA Pediatr (2019) 173:544–52. doi: 10.1001/jamapediatrics.2019.0384
19. Ralston SL, Lieberthal AS, Meissner HC, Alverson BK, Baley JE, Gadomski AM, et al. Clinical practice guideline: the diagnosis, management, and prevention of bronchiolitis. Pediatrics. (2014) 134:e1474–502. doi: 10.1542/peds.2014-2742
20. Taliun D, Harris DN, Kessler MD, Carlson J, Szpiech ZA, Torres R, et al. Sequencing of 53,831 diverse genomes from the NHLBI TOPMed program. Nature. (2021) 590:290–9. doi: 10.1038/s41586-021-03205-y
21. Zhu Z, Camargo CA, Raita Y, Fujiogi M, Liang L, Rhee EP, et al. Metabolome subtyping of severe bronchiolitis in infancy and risk of childhood asthma. J Allergy Clin Immunol (2022) 149:102–12. doi: 10.1016/j.jaci.2021.05.036
22. Camargo CA Jr, Ingham T, Wickens K, Thadhani R, Silvers KM, Epton MJ, et al. Cord-blood 25-hydroxyvitamin d levels and risk of respiratory infection, wheezing, and asthma. Pediatrics. (2011) 127:e180–7. doi: 10.1542/peds.2010-0442
23. Zhu Z, Lee PH, Chaffin MD, Chung W, Loh PR, Lu Q, et al. A genome-wide cross-trait analysis from UK biobank highlights the shared genetic architecture of asthma and allergic diseases. Nat Genet (2018) 50:857–64. doi: 10.1038/s41588-018-0121-0
24. Zhu Z, Zhu X, Liu CL, Shi H, Shen S, Yang Y, et al. Shared genetics of asthma and mental health disorders: a large-scale genome-wide cross-trait analysis. Eur Respir J (2019) 54:1901507. doi: 10.1183/13993003.01507-2019
25. Zhu Z, Guo Y, Shi H, Liu CL, Panganiban RA, Chung W, et al. Shared genetic and experimental links between obesity-related traits and asthma subtypes in UK biobank. J Allergy Clin Immunol (2020) 145:537–49. doi: 10.1016/j.jaci.2019.09.035
26. Daya M, Rafaels N, Brunetti TM, Chavan S, Levin AM, Shetty A, et al. Association study in African-admixed populations across the americas recapitulates asthma risk loci in non-African populations. Nat Commun (2019) 10:880. doi: 10.1038/s41467-019-08469-7
27. Pruim RJ, Welch RP, Sanna S, Teslovich TM, Chines PS, Gliedt TP, et al. LocusZoom: regional visualization of genome-wide association scan results. Bioinformatics. (2010) 26:2336–7. doi: 10.1093/bioinformatics/btq419
28. Duggal P, Gillanders EM, Holmes TN, Bailey-Wilson JE. Establishing an adjusted p-value threshold to control the family-wide type 1 error in genome wide association studies. BMC Genomics (2008) 9:516. doi: 10.1186/1471-2164-9-516
29. Makrinioti H, Bush A, Gern J, Johnston SL, Papadopoulos N, Feleszko W, et al. The role of interferons in driving susceptibility to asthma following bronchiolitis: controversies and research gaps. Front Immunol (2021) 12:761660. doi: 10.3389/fimmu.2021.761660
30. Read JF, Bosco A. Decoding susceptibility to respiratory viral infections and asthma inception in children. Int J Mol Sci (2020) 21:6372. doi: 10.3390/ijms21176372
31. Zhu Z, Camargo CA Jr, Hasegawa K. Metabolomics in the prevention and management of asthma. Expert Rev Respir Med (2019) 13:1135–8. doi: 10.1080/17476348.2019.1674650
32. Kelly RS, Dahlin A, McGeachie MJ, Qiu W, Sordillo J, Wan ES, et al. Asthma metabolomics and the potential for integrative omics in research and the clinic. Chest. (2017) 151:262–77. doi: 10.1016/j.chest.2016.10.008
33. Rago D, Pedersen CET, Huang M, Kelly RS, Gürdeniz G, Brustad N, et al. Characteristics and mechanisms of a sphingolipid-associated childhood asthma endotype. Am J Respir Crit Care Med (2021) 203:853–63. doi: 10.1164/rccm.202008-3206OC
34. Raita Y, Pérez-Losada M, Freishtat RJ, Harmon B, Mansbach JM, Piedra PA, et al. Integrated omics endotyping of infants with respiratory syncytial virus bronchiolitis and risk of childhood asthma. Nat Commun (2021) 12:3601. doi: 10.1038/s41467-021-23859-6
35. Raita Y, Camargo CA Jr, Bochkov YA, Celedón JC, Gern JE, Mansbach JM, et al. Integrated-omics endotyping of infants with rhinovirus bronchiolitis and risk of childhood asthma. J Allergy Clin Immunol (2021) 147:2108–17. doi: 10.1016/j.jaci.2020.11.002
36. Fujiogi M, Camargo CA Jr, Raita Y, Zhu Z, Celedón JC, Mansbach JM, et al. Integrated associations of nasopharyngeal and serum metabolome with bronchiolitis severity and asthma: A multicenter prospective cohort study. Pediatr Allergy Immunol (2021) 32:905–16. doi: 10.1111/pai.13466
37. Fujiogi M, Zhu Z, Raita Y, Ooka T, Celedon JC, Freishtat R, et al. Nasopharyngeal lipidomic endotypes of infants with bronchiolitis and risk of childhood asthma: a multicentre prospective study. Thorax (2022) 77:1059–69. doi: 10.1136/thorax-2022-219016. thoraxjnl-2022-219016.
38. Kyo M, Zhu Z, Nanishi M, Shibata R, Ooka T, Freishtat RJ, et al. Association of nasopharyngeal and serum glutathione metabolism with bronchiolitis severity and asthma risk: A prospective multicenter cohort study. Metabolites (2022) 12:674. doi: 10.3390/metabo12080674
39. Turi KN, Romick-Rosendale L, Gebretsadik T, Watanabe M, Brunwasser S, Anderson LJ, et al. Using urine metabolomics to understand the pathogenesis of infant respiratory syncytial virus (RSV) infection and its role in childhood wheezing. Metabolomics. (2018) 14:135. doi: 10.1007/s11306-018-1431-z
40. Carraro S, Bozzetto S, Giordano G, El Mazloum D, Stocchero M, Pirillo P, et al. Wheezing preschool children with early-onset asthma reveal a specific metabolomic profile. Pediatr Allergy Immunol (2018) 29:375–82. doi: 10.1111/pai.12879
41. Janssen R, Bont L, Siezen CLE, Hodemaekers HM, Ermers MJ, Doornbos G, et al. Genetic susceptibility to respiratory syncytial virus bronchiolitis is predominantly associated with innate immune genes. J Infect Dis (2007) 196:826–34. doi: 10.1086/520886
42. Forton JT, Rowlands K, Rockett K, Hanchard N, Herbert M, Kwiatkowski DP, et al. Genetic association study for RSV bronchiolitis in infancy at the 5q31 cytokine cluster. Thorax. (2009) 64:345–52. doi: 10.1136/thx.2008.102111
43. Larkin EK, Hartert TV. Genes associated with RSV lower respiratory tract infection and asthma: the application of genetic epidemiological methods to understand causality. Future Virol (2015) 10:883–97. doi: 10.2217/fvl.15.55
44. Pasanen A, Karjalainen MK, Bont L, Piippo-Savolainen E, Ruotsalainen M, Goksör E, et al. Genome-wide association study of polymorphisms predisposing to bronchiolitis. Sci Rep (2017) 7:41653. doi: 10.1038/srep41653
45. Moffatt MF, Gut IG, Demenais F, Strachan DP, Bouzigon E, Heath S, et al. A large-scale, consortium-based genomewide association study of asthma. N Engl J Med (2010) 363:1211–21. doi: 10.1056/NEJMoa0906312
46. Demenais F, Margaritte-Jeannin P, Barnes KC, Cookson WOC, Altmüller J, Ang W, et al. Multiancestry association study identifies new asthma risk loci that colocalize with immune-cell enhancer marks. Nat Genet (2018) 50:42–53. doi: 10.1038/s41588-017-0014-7
47. Miller RL, Grayson MH, Strothman K. Advances in asthma: New understandings of asthma’s natural history, risk factors, underlying mechanisms, and clinical management. J Allergy Clin Immunol (2021) 148:1430–41. doi: 10.1016/j.jaci.2021.10.001
48. Galanter J, Choudhry S, Eng C, Nazario S, Rodríguez-Santana JR, Casal J, et al. ORMDL3 gene is associated with asthma in three ethnically diverse populations. Am J Respir Crit Care Med (2008) 177:1194–200. doi: 10.1164/rccm.200711-1644OC
49. Chang X, March M, Mentch F, Qu H, Liu Y, Glessner J, et al. Genetic architecture of asthma in African American patients. J Allergy Clin Immunol (2022). doi: 10.1016/j.jaci.2022.09.001
50. James B, Milstien S, Spiegel S. ORMDL3 and allergic asthma: From physiology to pathology. J Allergy Clin Immunol (2019) 144:634–40. doi: 10.1016/j.jaci.2019.07.023
51. Johnson RK, Brunetti T, Quinn K, Doenges K, Campbell M, Arehart C, et al. Discovering metabolite quantitative trait loci in asthma using an isolated population. J Allergy Clin Immunol (2022) 149:1807–1811.e16. doi: 10.1016/j.jaci.2021.11.002
52. Ried JS, Baurecht H, Stückler F, Krumsiek J, Gieger C, Heinrich J, et al. Integrative genetic and metabolite profiling analysis suggests altered phosphatidylcholine metabolism in asthma. Allergy. (2013) 68:629–36. doi: 10.1111/all.12110
53. Rathcke CN, Holmkvist J, Husmoen LLN, Hansen T, Pedersen O, Vestergaard H, et al. Association of polymorphisms of the CHI3L1 gene with asthma and atopy: a populations-based study of 6514 Danish adults. PloS One (2009) 4:e6106. doi: 10.1371/journal.pone.0006106
54. March ME, Sleiman PM, Hakonarson H. Genetic polymorphisms and associated susceptibility to asthma. Int J Gen Med (2013) 6:253–65. doi: 10.2147/IJGM.S28156
55. Kanehisa M, Goto S. KEGG: kyoto encyclopedia of genes and genomes. Nucleic Acids Res (2000) 28:27–30. doi: 10.1093/nar/28.1.27
56. Brown RA, Spina D, Page CP. Adenosine receptors and asthma. Br J Pharmacol (2008) 153 Suppl 1:S446–56. doi: 10.1038/bjp.2008.22
57. Pyne S, Pyne NJ. The differential regulation of cyclic AMP by sphingomyelin-derived lipids and the modulation of sphingolipid-stimulated extracellular signal regulated kinase-2 in airway smooth muscle. Biochem J (1996) 315:917–23. doi: 10.1042/bj3150917
58. Billington CK, Ojo OO, Penn RB, Ito S. cAMP regulation of airway smooth muscle function. Pulm Pharmacol Ther (2013) 26:112–20. doi: 10.1016/j.pupt.2012.05.007
59. Ethier MF, Madison JM. Adenosine A1 receptors mediate mobilization of calcium in human bronchial smooth muscle cells. Am J Respir Cell Mol Biol (2006) 35:496–502. doi: 10.1165/rcmb.2005-0290OC
60. Gault CR, Obeid LM, Hannun YA. An overview of sphingolipid metabolism: from synthesis to breakdown. Adv Exp Med Biol (2010) 688:1–23. doi: 10.1007/978-1-4419-6741-1_1
61. Maceyka M, Spiegel S. Sphingolipid metabolites in inflammatory disease. Nature. (2014) 510:58–67. doi: 10.1038/nature13475
62. Adikusuma W, Chou WH, Lin MR, Ting J, Irham LM, Perwitasari DA, et al. Identification of druggable genes for asthma by integrated genomic network analysis. Biomedicines. (2022) 10(1):113. doi: 10.3390/biomedicines10010113
63. Sturgill JL. Sphingolipids and their enigmatic role in asthma. Adv Biol Regul (2018) 70:74–81. doi: 10.1016/j.jbior.2018.09.001
64. Liu Q, Guan J, Song R, Zhang X, Mao S. Physicochemical properties of nanoparticles affecting their fate and the physiological function of pulmonary surfactants. Acta Biomater. (2022) 140:76–87. doi: 10.1016/j.actbio.2021.11.034
65. Choudhary V, Uaratanawong R, Patel RR, Patel H, Bao W, Hartney B, et al. Phosphatidylglycerol inhibits toll-like receptor-mediated inflammation by danger-associated molecular patterns. J Invest Dermatol (2019) 139:868–77. doi: 10.1016/j.jid.2018.10.021
66. Wu YZ, Medjane S, Chabot S, Kubrusly FS, Raw I, Chignard M, et al. Surfactant protein-a and phosphatidylglycerol suppress type IIA phospholipase A2 synthesis via nuclear factor-kappaB. Am J Respir Crit Care Med (2003) 168:692–9. doi: 10.1164/rccm.200304-467OC
67. Numata M, Nagashima Y, Moore ML, Berry KZ, Chan M, Kandasamy P, et al. Phosphatidylglycerol provides short-term prophylaxis against respiratory syncytial virus infection. J Lipid Res (2013) 54:2133–43. doi: 10.1194/jlr.M037077
68. Blalock TD, Spurr-Michaud SJ, Tisdale AS, Heimer SR, Gilmore MS, Ramesh V, et al. Functions of MUC16 in corneal epithelial cells. Invest Ophthalmol Vis Sci (2007) 48:4509–18. doi: 10.1167/iovs.07-0430
69. Davies JR, Kirkham S, Svitacheva N, Thornton DJ, Carlstedt I. MUC16 is produced in tracheal surface epithelium and submucosal glands and is present in secretions from normal human airway and cultured bronchial epithelial cells. Int J Biochem Cell Biol (2007) 39:1943–54. doi: 10.1016/j.biocel.2007.05.013
70. Das S, Batra SK. Understanding the unique attributes of MUC16 (CA125): Potential implications in targeted therapy. Cancer Res (2015) 75:4669–74. doi: 10.1158/0008-5472.CAN-15-1050
71. Lei Y, Zang R, Lu Z, Zhang G, Huang J, Liu C, et al. ERO1L promotes IL6/sIL6R signaling and regulates MUC16 expression to promote CA125 secretion and the metastasis of lung cancer cells. Cell Death Dis (2020) 11:853. doi: 10.1038/s41419-020-03067-8
72. Maguire JA, Mulugeta S, Beers MF. Endoplasmic reticulum stress induced by surfactant protein c BRICHOS mutants promotes proinflammatory signaling by epithelial cells. Am J Respir Cell Mol Biol (2011) 44:404–14. doi: 10.1165/rcmb.2009-0382OC
73. Kim SR, Kim DI, Kang MR, Lee KS, Park SY, Jeong JS, et al. Endoplasmic reticulum stress influences bronchial asthma pathogenesis by modulating nuclear factor κB activation. J Allergy Clin Immunol (2013) 132:1397–408. doi: 10.1016/j.jaci.2013.08.041
74. Johnson JL, Abecasis GR. GAS power calculator: web-based power calculator for genetic association studies. BioRxiv (2017) 2017:164343. doi: 10.1101/164343
Keywords: asthma, bronchiolitis, childhood asthma, genetics, integrated-omics, metabolomics, phosphatidylglycerol, sphingolipids
Citation: Ooka T, Zhu Z, Liang L, Celedon JC, Harmon B, Hahn A, Rhee EP, Freishtat RJ, Camargo CA Jr. and Hasegawa K (2023) Integrative genetics-metabolomics analysis of infant bronchiolitis-childhood asthma link: A multicenter prospective study. Front. Immunol. 13:1111723. doi: 10.3389/fimmu.2022.1111723
Received: 30 November 2022; Accepted: 28 December 2022;
Published: 02 February 2023.
Edited by:
Hideaki Morita, National Center for Child Health and Development (NCCHD), JapanReviewed by:
Esther Herrera Luis, University of La Laguna, SpainShohei Akagawa, Kansai Medical University, Japan
Copyright © 2023 Ooka, Zhu, Liang, Celedon, Harmon, Hahn, Rhee, Freishtat, Camargo and Hasegawa. This is an open-access article distributed under the terms of the Creative Commons Attribution License (CC BY). The use, distribution or reproduction in other forums is permitted, provided the original author(s) and the copyright owner(s) are credited and that the original publication in this journal is cited, in accordance with accepted academic practice. No use, distribution or reproduction is permitted which does not comply with these terms.
*Correspondence: Tadao Ooka, tooka@mgh.harvard.edu
†These authors have contributed equally to this work