- 1Department of Genetics, Louisiana State University Health Sciences Center, New Orleans, LA, United States
- 2School of Medicine, Louisiana State University Health Sciences Center, New Orleans, LA, United States
- 3Department of Microbiology, Immunology, and Parasitology, Lousiana State University Health Sciences Center (LSUHSC), New Orleans, LA, United States
- 4Research and Development, Oschner Medical Center, New Orleans, LA, United States
- 5Department of Pathology, Louisiana State University Health Sciences Center, New Orleans, LA, United States
- 6Research and Development, BioInfoExperts, LLC, Thibodaux, LA, United States
Long-term immunity against severe acute respiratory syndrome coronavirus 2 (SARS-CoV-2) requires the identification of T-cell epitopes affecting host immunogenicity. In this computational study, we explored the CD8+ epitope diversity estimated in 27 of the most common HLA-A and HLA-B alleles, representing most of the United States population. Analysis of 16 SARS-CoV-2 variants [B.1, Alpha (B.1.1.7), five Delta (AY.100, AY.25, AY.3, AY.3.1, AY.44), and nine Omicron (BA.1, BA.1.1, BA.2, BA.4, BA.5, BQ.1, BQ.1.1, XBB.1, XBB.1.5)] in analyzed MHC class I alleles revealed that SARS-CoV-2 CD8+ epitope conservation was estimated at 87.6%–96.5% in spike (S), 92.5%–99.6% in membrane (M), and 94.6%–99% in nucleocapsid (N). As the virus mutated, an increasing proportion of S epitopes experienced reduced predicted binding affinity: 70% of Omicron BQ.1-XBB.1.5 S epitopes experienced decreased predicted binding, as compared with ~3% and ~15% in the earlier strains Delta AY.100–AY.44 and Omicron BA.1–BA.5, respectively. Additionally, we identified several novel candidate HLA alleles that may be more susceptible to severe disease, notably HLA-A*32:01, HLA-A*26:01, and HLA-B*53:01, and relatively protected from disease, such as HLA-A*31:01, HLA-B*40:01, HLA-B*44:03, and HLA-B*57:01. Our findings support the hypothesis that viral genetic variation affecting CD8 T-cell epitope immunogenicity contributes to determining the clinical severity of acute COVID-19. Achieving long-term COVID-19 immunity will require an understanding of the relationship between T cells, SARS-CoV-2 variants, and host MHC class I genetics. This project is one of the first to explore the SARS-CoV-2 CD8+ epitope diversity that putatively impacts much of the United States population.
1 Introduction
Since the emergence of severe acute respiratory syndrome coronavirus 2 (SARS-CoV-2) in late 2019, the scientific community rapidly developed several therapeutic monoclonal antibodies and mRNA vaccines. Current vaccines elicit a short-lived humoral response against the SARS-CoV-2 spike protein, lasting an average of 3–4 months and requiring periodic boosters (1–3). Intriguingly, coronavirus disease 2019 (COVID-19) patients lacking humoral immune response due to treatment of hematological malignancies did not exhibit increased disease severity or mortality, suggesting that B-cell-mediated immunity may not be sufficient to confer long-term immunity against SARS-CoV-2 (4–6). In contrast, convalescent macaque models depleted of CD8+ T cells exhibited loss of host protection following reinfection, highlighting the importance of T-cell immunity in COVID-19 clinical presentation (7).
Cytotoxic CD8+ T cells are essential for the clearance of intracellular viral pathogens, such as SARS-CoV-2 (8–10). T-cell activation occurs through T-cell receptors binding to T-cell epitopes, described as peptide antigens bound by a human heterodimeric glycoprotein, known as a major histocompatibility complex (MHC). CD8+ T-cell antigen recognition is determined by MHC class I genes, which control antigenic peptide presentation on MHC class I molecules (11). Unlike the invariant β2-microglobulin subunit, the α subunit of MHC class I proteins is highly polymorphic, with the most polymorphic genes being human leukocyte antigens (HLA) HLA-A, HLA-B, and HLA-C; these subunits have an estimated 1,939, 2,577, and 1,595 allotypes, respectively (11, 12). Therefore, the considerable individual diversity generated from HLA polymorphism is a proposed explanation for the differential clinical severity of COVID-19 variants seen between individuals, since the epitope repertoire from one patient is likely to be substantially different from the next (13–15). Select studies have sequenced the HLA alleles and SARS-CoV-2 T-cell epitopes of convalescent patients (4, 15–18). However, current research on T-cell response to COVID-19, especially analysis exploring the relationship between HLA molecules and viral CD8+ epitopes on a population/epidemiological level, remains limited. Previously published research has already identified several HLA alleles associated with increased (HLA-A*25:01, HLA-B*46:01, and HLA-B*27:07) or decreased (HLA-B*07:02, HLA-B*15:03, and HLA-B*51:01) clinical severity in convalescent patients (Table 1) (13, 19–21), but none have explored the entire epitope repertoire of variants of concern (VOC) gene products in the most common HLA allotypes.
SARS-CoV-2 VOC and subvariants accumulate mutations in the genes for their protein products, such as spike, membrane, and nucleocapsid proteins, potentially affecting the binding affinity and immunogenicity of T-cell epitopes. These mutations and the resulting alterations to MHC-I binding affinity may influence COVID-19 clinical characteristics, such as viral transmissibility, protection against neutralizing antibodies, risks of reinfection, and disease severity (15, 22, 23), as well as the risk of post-acute sequelae of COVID-19 infection (PASC or long COVID) (24–26). Given that an estimated 3% of CD8+ T-cell epitopes are affected by mutations conferred in various VOCs, certain HLA alleles may have more (or less) propensity to be strongly affected by mutations in specific VOCs (15).
This manuscript distinguishes SARS-CoV-2 variant-specific CD8+ T-cell epitopes of spike, membrane, and nucleocapsid gene products for 27 of the most frequent HLA-A and HLA-B alleles. The purpose of this computational study was to model the immunogenic effects and clinical severity of SARS-CoV-2 variants in the most common MHC class I alleles in the United States population. Our bioinformatics approach integrates the use of Ensembl’s COVID-19 genome browser, Immune Epitope Database and Analysis Resource tool TepiTool, and ExPASy translate tool (27–29).
2 Materials and methods
2.1 SARS-CoV-2 viral genome sequencing
Specimens were received by the LSUHSC Precision Medicine Laboratory from various collection sites representing the Louisiana patient population for public health screening purposes. RNA extraction was performed using the Zymo Quick DNA/RNA Viral MagBead kit automated on a Tecan Fluent liquid handling workstation. The resulting viral RNA was used for library generation and next-generation sequencing using the Illumina COVID-Seq workflow as per the manufacturer’s instructions. Libraries were pooled (up to 192 samples/run) and loaded on an Illumina NextSeq550Dx in RUO mode, with 74 cycles of paired-end sequencing using a 150-cycle mid output reagent cartridge and flow cell. Initial data processing and QC was performed using the DRAGEN COVID-Seq Test (EUA) v.1.2.2 application on the cloud-based BaseSpace sequence analysis hub hosted by Illumina. BaseSpace project share links were provided to BioInfoExperts, LLC for sequence processing and analysis in FoxSeq software (www.foxseqllc.com). Briefly, sequences were quality-filtered using Trimmomatic (30) and mapped to the reference using Bowtie2 (31). Variant calling and consensus sequence generation were performed using bcftools (32). Nucleotides at any position were only assigned if the sequencing depth was >200 and the allele frequency was 80%. Lineages were assigned using pangolin (https://cov-lineages.org). Consensus sequences were uploaded to GISAID and NCBI SARS-CoV-2 viral genome data repositories.
2.2 SARS-CoV-2 variant sequence comparison and protein peptide sequence generation
Genome sequences of SARS-CoV-2 variants were blasted against the originally sequenced Wuhan strain (INSDC accession CGA_009858895.3) using Ensembl’s (RRID: SCR_002344) SARS-CoV-2 genome browser (29). Variant-specific cDNA sequences for transcripts were generated from Ensembl’s SARS-CoV-2 genome browser (RRID: SCR_024704). SARS-CoV-2 variant-specific cDNA for spike, membrane, and nucleocapsid was converted to amino acid (protein) sequences, using the ExPASy translate tool (RRID: SCR_024703) (27).
2.3 TepiTool IEDB analysis of coronavirus T-cell epitopes
The prediction of MHC-I epitope binding to variant-specific S, M, and N was generated through the Immune Epitope Database and Analysis Resource (IEDB) (RRID: SCR_006604), via TepiTool utilizing the IEDB-recommended default prediction (33). Spike, membrane, and nucleocapsid were selected because, for the most part, spontaneous CD8+ responses against SARS-CoV-2 T-cell epitopes target the proteins they encode (16). A panel of 27 most frequent A and B alleles was used for MHC-I epitope binding analysis. The specific alleles included were as follows: HLA-A*01:01, HLA-A*02:01, HLA-A*02:03, HLA-A*02:06, HLA-A*03:01, HLA-A*11:01, HLA-A*23:01, HLA-A*24:02, HLA-A*26:01, HLA-A*30:01, HLA-A*30:02, HLA-A*31:01, HLA-A*32:01, HLA-A*33:01, HLA-A*68:01, HLA-A*68:02, HLA-B*07:02, HLA-B*08:01, HLA-B*15:01, HLA-B*35:01, HLA-B*40:01, HLA-B*44:02, HLA-B*44:03, HLA-B*51:01, HLA-B*53:01, HLA-B*57:01, and HLA-B*58:01. IEDB’s default prediction method reflects consensus across ANN, SMM, and CombLib predictors and was used to select peptides with predicted consensus percentile ranks ≤1 (28). Low scores correspond to high predicted affinities.
2.4 FoldX peptide docking of HLA-B*15:01
Molecular docking was adapted from Mazumder et al. (34). The RepairPDB method from FoldX (RRID: SCR_008522) Suite 5.0 was initially used to repair the structures obtained from the RCSB Protein Data Bank (Supplementary Table S1) (35). This allows for the use of the structures in downstream FoldX tools. BuildModel was used to convert from the peptide in the structure to the original SARS-COV-2 CD8+ peptide. BuildModel was used again to convert from the original SARS-COV-2 CD8+ peptide to the mutated peptide. The estimated ∆G (kcal/mol) was then used to create a heatmap in R (v4.2.1) with the ComplexHeatmap function (36). Python scripts used to run FoldX can be found at github.com/elnaggarj/FoldX-PeptideDocking.
3 Results
3.1 Spike, membrane, and nucleocapsid nucleotide alterations between one pre-Alpha, one Alpha, five Delta, and nine Omicron SARS-CoV-2 variants over time
B.1, Alpha (B.1 and B.1.1.7), five Delta (AY.100, AY.25, AY.3, AY.3.1, and AY.44), and nine Omicron (BA.1, BA.1.1, BA.2, BA.4, BA.5, BQ.1, BQ.1.1, XBB.1, and XBB.1.5) VOCs were sequenced from the Louisiana patient population between 9 April 2020 and January 2023. Variant FASTAs were compared with the ancestral Wuhan strain (NCBI: NC_045512.2) using BLAST to determine nucleotide differences (Figure 1). Alpha and Delta strains displayed minimal variance, with Delta exhibiting 12–15, 1, and 4–6 nucleotide (NT) variations in S, M, and N, respectively. B.1 and B.1.1.7 showed alterations in spike (2 NT in B.1; 10 NT in B.1.1.7) and nucleocapsid (5 NT in B.1.1.7) although M remained identical to the original Wuhan strain. In comparison, Omicron variants exhibited 37–55, 3–4, and 13–16 NT variations in S, M, and N, respectively (Figure 1). Among the three protein products analyzed, membrane and nucleocapsid sequences were highly conserved, with M experiencing only 0–4 NT changes between the 16 variants analyzed (M: 665–669/669 NT = 99.4%–100% conservation; N: 1,244–1,256/1,260 = 98.7%–99.8%; S: 3,830–3,776/3,831 = 98.5%–99.9%). Additionally, there was limited mutational divergence seen in Omicron variants between March 2022 and December 2022, suggesting a possible plateau in genetic drift within the Omicron family of SARS-CoV-2.
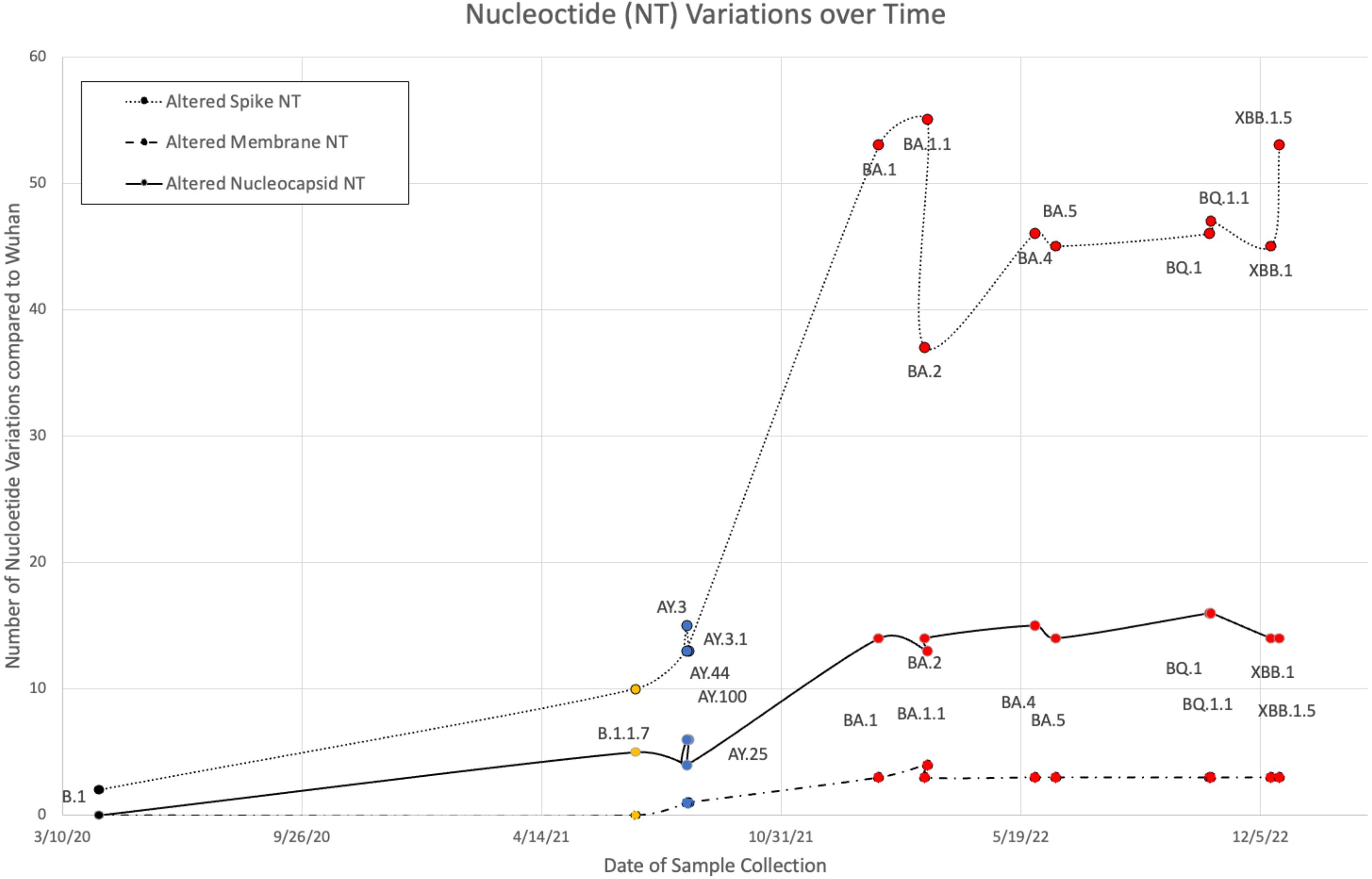
Figure 1 Nucleotide (NT) variations of spike, membrane, and nucleocapsid over time between B.1 (colored black), Alpha (orange), five Delta (blue), and nine Omicron (red) variants when compared against the original Wuhan strain.
3.2 Epitope differences between 16 variants of spike, membrane, and nucleocapsid when compared against the ancestral Wuhan strain
We generated predictive estimates of MHC-I epitopes to variant-specific S, M, and N using the IEDB Resource TepiTool, utilizing the IEDB-recommended default prediction. A panel of 27 most frequent A and B alleles were used for MHC-I epitope binding analysis, which encompassed 16 HLA-A (HLA-A*01:01, HLA-A*02:01, HLA-A*02:03, HLA-A*02:06, HLA-A*03:01, HLA-A*11:01, HLA-A*23:01, HLA-A*24:02, HLA-A*26:01, HLA-A*30:01, HLA-A*30:02, HLA-A*31:01, HLA-A*32:01, HLA-A*33:01, HLA-A*68:01, and HLA-A*68:02) and 11 HLA-B (HLA-B*07:02, HLA-B*08:01, HLA-B*15:01, HLA-B*35:01, HLA-B*40:01, HLA-B*44:02, HLA-B*44:03, HLA-B*51:01, HLA-B*53:01, HLA-B*57:01, and HLA-B*58:01) alleles. Utilizing the haplotype frequency estimates provided by the National Marrow Donor Program (37), the 16 HLA-A alleles make up 92.4% of the population in Caucasians, 69.2% in African Americans, 74% in Asian, and 83% in Hispanics. Similarly, the 11 HLA-B alleles represent 67.7% of Caucasians, 44.8% of African Americans, 39.2% of Asians, and 39.8% of Hispanics. CD8+ epitope repertoires, comprising the 27 most common HLA-A and HLA-B alleles, were generated for 16 SARS-CoV-2 variants and the ancestral Wuhan strain. The original S, M, and N protein products resulted in a repertoire of 1,081, 237, and 289 predicted CD8+ epitopes, respectively. From the 16 SARS-CoV-2 variant spike proteins, we identified a range of 1,077–1,115 CD8+ T-cell epitopes. Variant-specific membrane epitopes ranged between 236 and 241, with nucleocapsid CD8+ repertoires comprising 289–298 epitopes for the 27 HLA alleles analyzed.
Wuhan S, M, and N repertoires were compared against 16 variants (B.1, Alpha, five Delta, and nine Omicron) to identify epitopes that were lost, gained, or altered in estimated HLA binding affinity between variants (Figure 2). In general, a balanced number of epitopes were lost and gained for all variants; however, BA.1.1 M, BA.4 N, B.1.1.7 S, and BQ.1.1 S repertoires sustained greater epitope loss than gain (Figures 2A–C, 3, bottom), which may contribute to explaining the increased transmission and breakthrough cases seen in these subvariants (39, 40). Additionally, spike epitopes in the early variants (B.1 and B.1.1.7) and Omicron VOCs experienced a greater number of epitopes predicted to have reduced binding affinity than increased affinity.
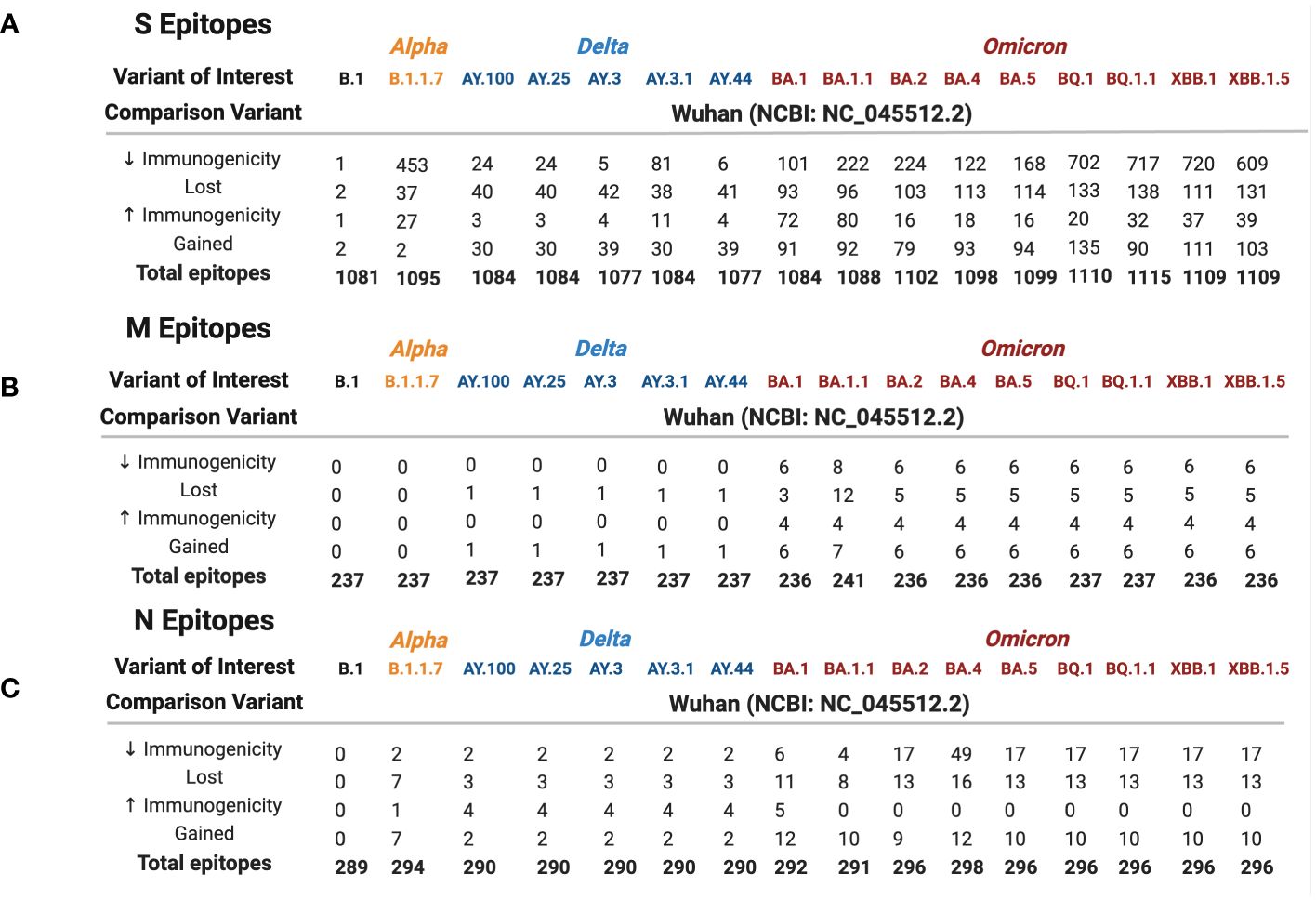
Figure 2 Spike (A), membrane (B), and nucleocapsid (C) epitope differences between variants of interest (B.1 labeled black, Alpha in orange, Delta in blue, and Omicron in red) when compared against the ancestral Wuhan strain. Predicted binding of SARS-CoV-2 S, M, and N epitopes was generated using the IEDB database TepiTool for the 27 most common HLA-A and HLA-B alleles.
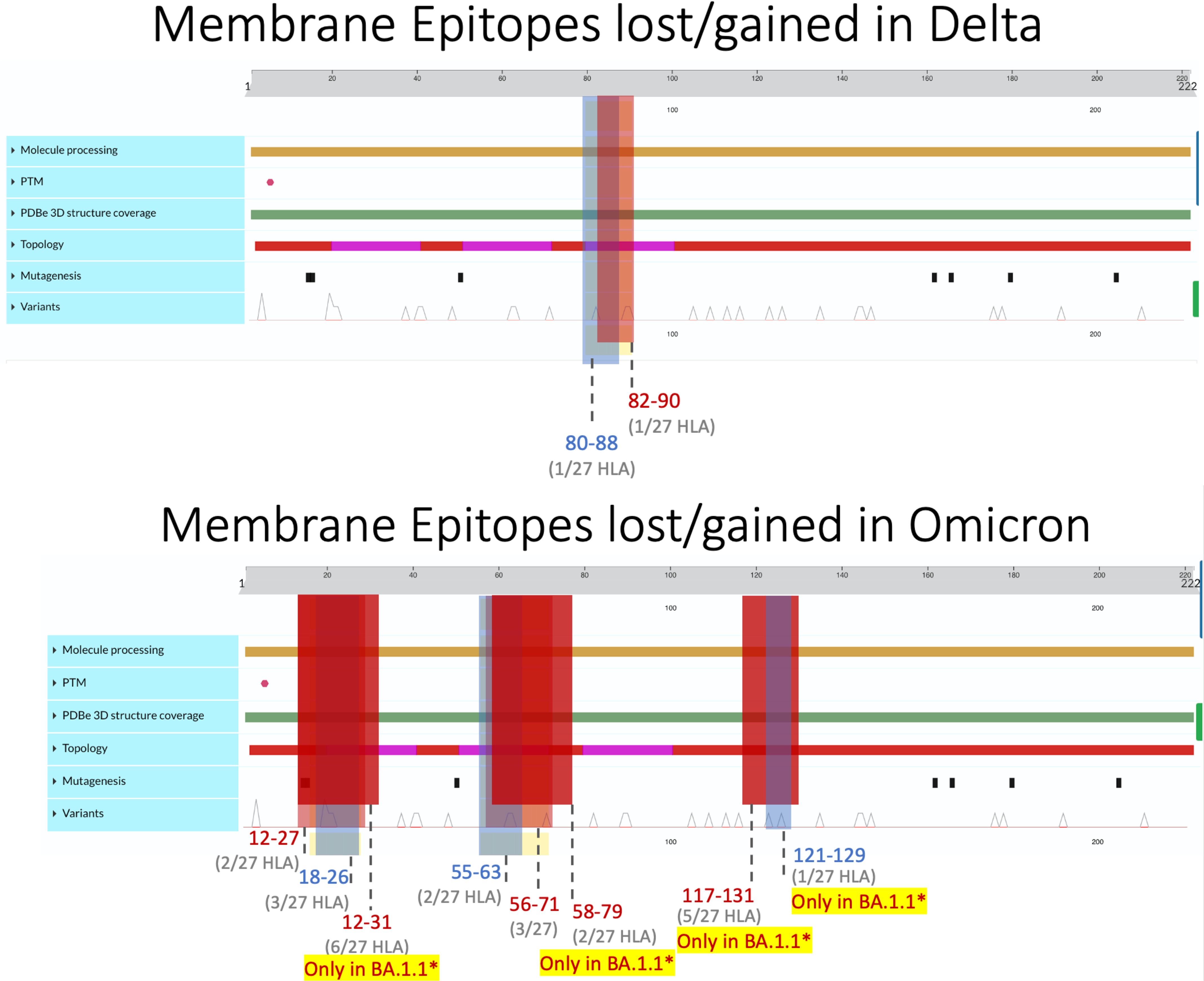
Figure 3 Membrane epitopes lost (regions colored red) and gained (colored blue) in Delta (top) and Omicron (bottom) when compared against the ancestral Wuhan strain. Protein characteristics were generated using UniProt’s Feature Viewer (38).
3.2.1 Spike epitopes were the least conserved, compared with membrane and nucleocapsid
Among the three viral proteins we examined, spike epitopes were least conserved, with S, M, and N epitopes experiencing 87.6%–99.8%, 92.5%–100%, and 94.6%–100% conservation, respectively. Across all the variants studied, Omicron BQ.1.1 S epitopes experienced the most loss, with 138/1,115 = 12.4% affected, while strain B.1 only lost 2 epitopes out of 1,081 total (0.0019%) compared with the original Wuhan strain. Among the 14 Delta and Omicron spike proteins, the largest area of conservation, defined as a region experiencing no epitope loss or gain, was found between amino acids (AA) 987–1,205 within the 1273 AA protein (Figure 4). As seen in the two other protein products, S epitopes that were lost were generally replaced by alternate epitopes that were gained in the same regions. However, all Delta variants lost two epitopes (VSSQCNLR and SQCVNLRTR), affecting HLA-A*31:01 and HLA-A*68:01, without experiencing epitope gains (Supplementary Figure 2). These epitopes spanned the AA 11–21 region, affecting the tail end of the hydrophobic signal peptide and the S1 subunit in the S protein.
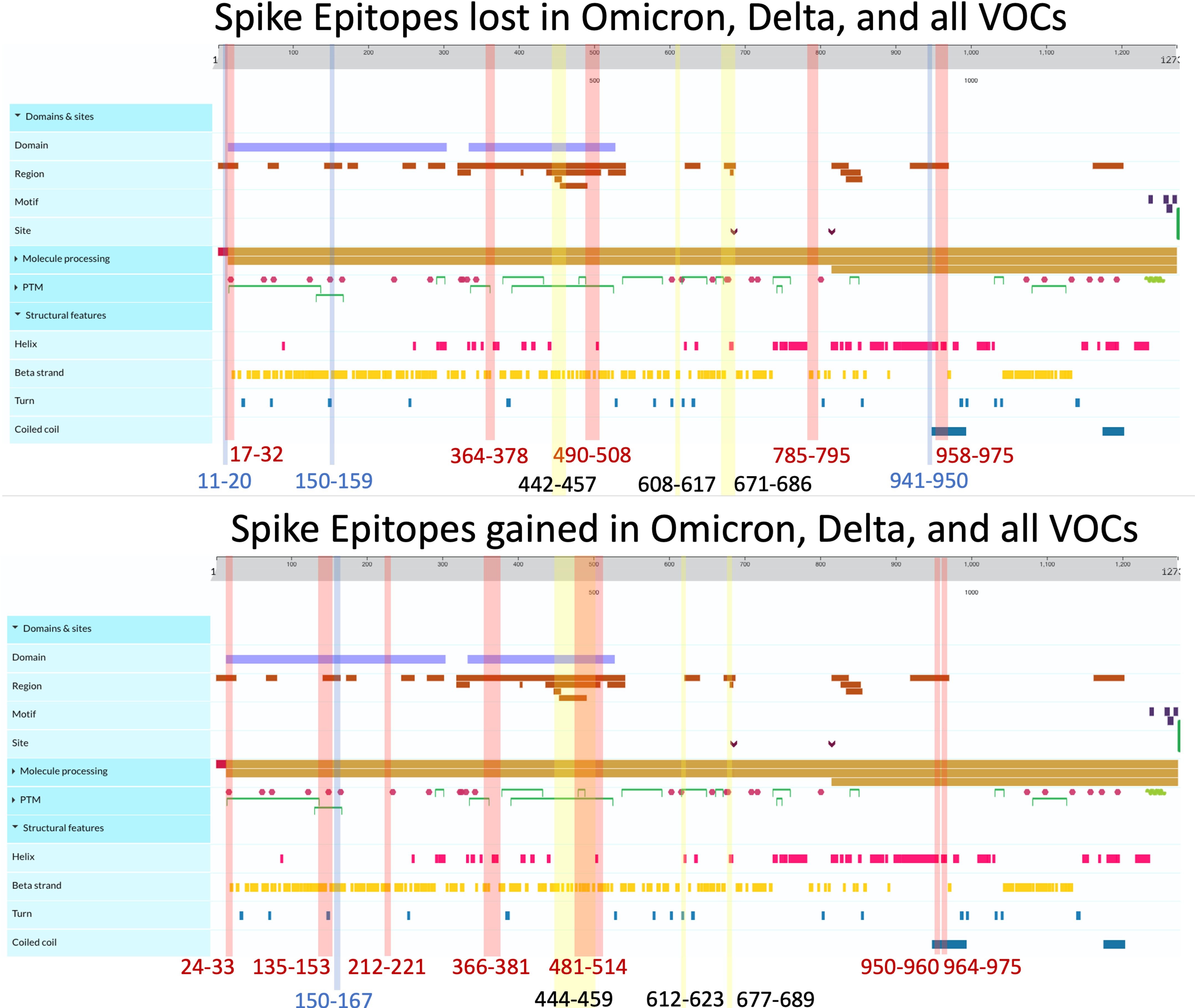
Figure 4 Spike epitopes gained (top) and lost (bottom) when compared against the ancestral Wuhan strain. Colored regions and numbers refer to amino acid locations of predicted epitope alterations, with red indicating changes seen in Omicron, blue for Delta, and yellow for epitopes affected in all 16 variants. Protein characteristics were generated using UniProt’s Feature Viewer (38).
As shown in Figure 2A, 41.4% of B.1.1.7 (Alpha VOC) spike epitopes were estimated to have reduced immunogenicity, while only 2.5% (27/1,092 epitopes) demonstrated increased predicted HLA binding. As SARS-CoV-2 mutated, an increasing proportion of epitopes were predicted to have reduced HLA binding, with 70% of Omicron BQ.1–XBB.1.5 S epitope repertoires experiencing decreased predicted binding affinity (as compared with the roughly 3% and 15% affected in Delta AY.100–AY.44 and Omicron BA.1–BA.5 variants, respectively) (Figures 1, 2A). When compared with the ancestral Wuhan spike, XBB.1 S epitopes experienced the greatest decrease in predicted immunogenicity, with 64.9% (720/1,109 epitopes; Figure 2A) of its CD8- T-cell repertoire demonstrating a reduction in estimated binding affinity, while only 37 epitopes (3.3%) were estimated to have increased HLA binding. Additionally, all 27 HLA-A and HLA-B alleles had decreased predicted binding affinity for B.1.1.7 and BA.1–XBB.1.5 spike epitopes.
3.2.2 Membrane epitopes were most conserved with balanced gain and loss maintained in all variants
Membrane epitopes sustained minimal alterations, with BA.1.1 losing the most (18/241 = 7.5%) and AY.100–AY.44 losing the least (1/237 = 0.04%) epitopes between Delta and Omicron variants (Figures 1, 2B). Alpha membrane epitopes were conserved unaltered from the original Wuhan variant sequenced. In general, M epitope loss was accompanied by balanced epitope gains across all VOCs, with similar patterns seen between epitopes with altered predicted binding affinity (Figure 2A). For all Delta variants, HLA-A*68:02 lost the ability to bind epitope TAMACLVGL, while HLA-B*51:01 gained IAIAMCLV between AA 80 and 90. Likewise, for the nine Omicron variants, two membrane segments (AA 12–27 and AA 55–71) experienced balanced epitope loss and gain (Figure 3).
BA.1.1 M protein lost significantly more epitopes than the other variants, affecting 15/27 HLA alleles, while the other 8 Omicron variants sustained epitope loss in only 5 HLA alleles (Figures 2B, 3, bottom). Additionally, BA.1.1 M contained a third region between 117 and 129 AA wherein HLA-A*03:01, HLA-A*26:01, HLA-A*30:02, HLA-A*31:01, and HLA-A*33:01 endured epitope loss of NILLNVPLY and PLYGTILTR (Figure 3, bottom). Unlike other regions, only HLA-B*08:01 gained an epitope within the AA 117–129 segment. The region between AA 132 and 222 was found to be conserved, with no predicted epitopes being lost or gained.
3.2.3 Unbalanced nucleocapsid epitope gain/loss and alterations in predicted binding, with more epitopes experiencing decreased predicted binding
Like M, N epitopes were highly conserved, with the greatest loss seen in BA.4 (16/298 = 5.4%) and conservation in AY.100/AY.25/AY.44 (3/290 = 1%) among Omicron and Delta variants. N epitopes experienced no loss or gain between AA 66–194 and AA 237–401 in all VOCs. Although nucleocapsid epitopes experienced numerically balanced gain and loss across VOCs (Figure 2C), further analysis revealed AA 192–209 to be the only region where epitopes were both gained and lost, including the Alpha variant (Figure 5). Within this region, more HLAs sustained gain/loss in Omicron (6/27 HLA gain; 5/27 HLA loss) and Alpha (6/27 HLA gain; 5/27 HLA loss) VOCs than Delta VOCs (1/27 HLA gain; 1/27 HLA loss) in this region. Unlike the other two SARS-CoV-2 VOC families, Alpha only experienced epitope loss/gain between the AA 195 and 237 region, wherein epitope SSRGTSPAR was gained in HLA-A:03:01, HLA-A*11:01, HLA-A*30:01, HLA-A*31:01, HLA-A:33:01, and HLA-A:68:01, while RNSTPGSSK and NSTPGSSKR were lost in HLA-A*03:01, HLA-A*11:01, HLA-A*30:01, HLA-A*33:01, and HLA-A*68:01.
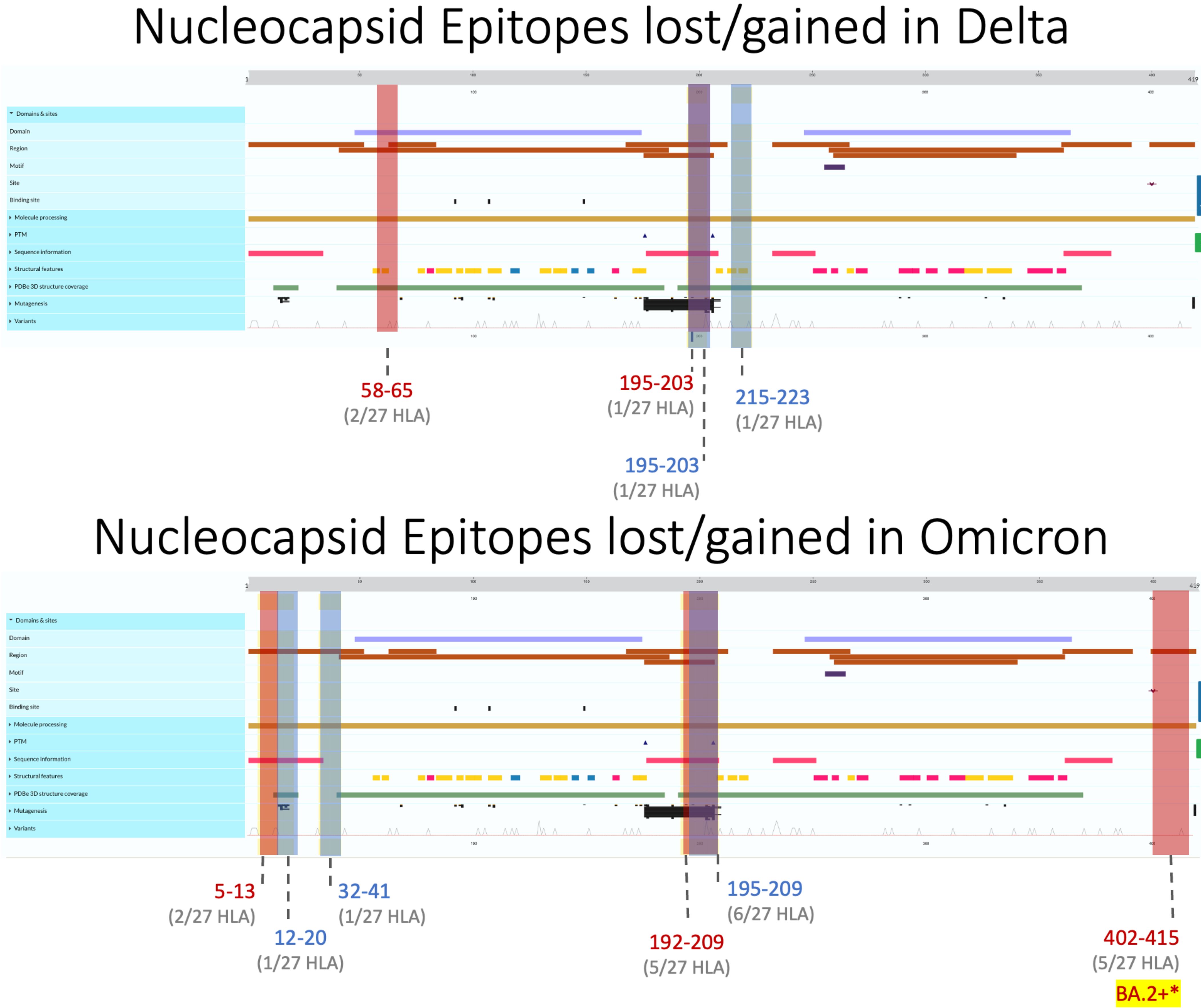
Figure 5 Nucleocapsid epitopes lost (regions colored red) and gained (in blue) in Delta (top) and Omicron (bottom) variants when compared against the ancestral Wuhan strain. Protein characteristics were generated using UniProt’s Feature Viewer.
Across all Delta N epitope repertoires, HLA-A*23:01 and HLA-A*24:02 lost the ability to bind to QHGKEGLKF between 58 and 65 AA, while HLA-B*40:01 gained binding to GDAALALLL in AA 215–223 (Figure 4, top). Between the nine Omicron variants, HLA-B*07:02 gained APTRITFGGP epitope binding between 12 and 20 AA, while HLA-A*31:01 gained two epitopes (RSGARSKQR and SGARSKQRR) between AA 32 and 41. Omicron-specific N epitope loss was found between AA 5 and 13, where HLA-B*07:02 and HLA-B*08:01 lost the ability to bind to GPQNQRNAL. In addition, SSRGTSPAR (AA 402–415) loss was found in BA.2–XBB.1.5 VOCs for 5/27 alleles: HLA-A*03:01, HLA-A*11:01, HLA-A*30:01, HLA-A*33:01, and HLA-A*68:01. Omicron VOCs BA.1.1–XBB.1.5 sustained decreased predicted binding affinity in epitopes [4 epitopes (BA.1.1 N)–49 epitopes (BA.4 N)], with zero epitopes gaining predicted binding (Figure 2C). BA.4 had 40 unique peptides affecting 16/16 HLA-A and 8/11 HLA-B alleles (Supplementary Figure 1).
3.2.4 Gained epitopes conserved in Omicron and Delta variants
Several epitopes were gained or conserved across Delta and Omicron families, including the spike epitopes, GVYYHKNNK, QTNSPRRAR, VGGNYNYLY, NYNYLYRLF, and YNYLYRLFR, as well as the nucleocapsid epitope RNSTPGSSR (Figures 6, 7). Of the gained S epitopes, ASFSTFKCY encompassed the greatest number of HLAs analyzed (9/27), estimated to affect 6/16 HLA-A encompassing 31.2% of the population in Caucasian American (EUR), 22.2% of African American (AFA), 30.3% of Asian American and Pacific Islander (API), and 21.8% of Hispanic and Latino Americans (HIS), and 3/11 HLA-B alleles (11% EUR, 5% AFA, 11.3% API, and 5.5% HIS). Likewise, the nucleocapsid epitope SSRGTSPAR was gained in 6/16 HLA-A alleles, comprising of 27.2% EUR, 23.5% AFA, 27.8% API, and 26.1% HIS population in the United States (Figure 7).
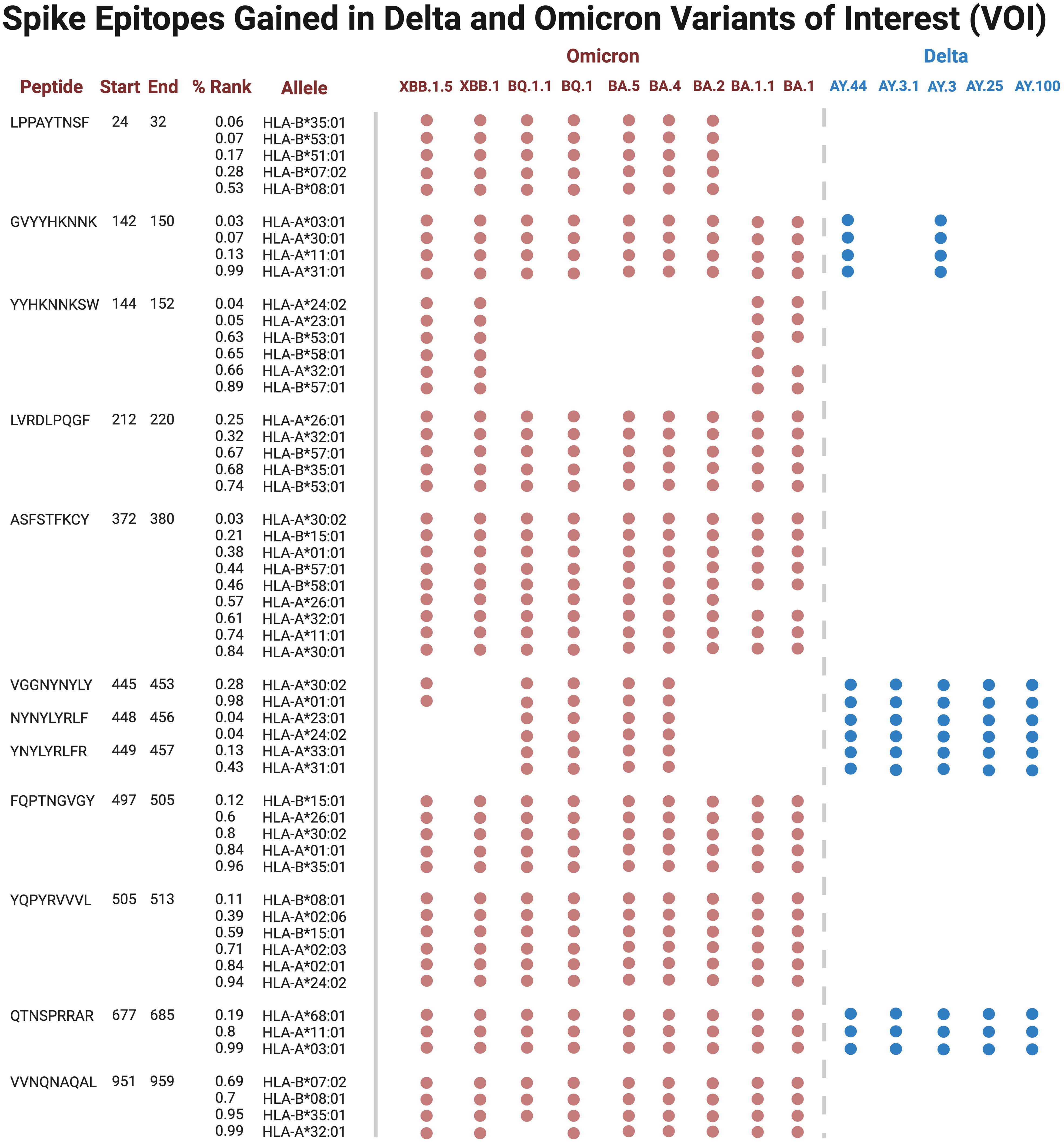
Figure 6 Spike epitopes gained in nine Omicron and five Delta variants, when compared against the original Wuhan strain. Figures were generated using BioRender (RRID: SCR_018361).
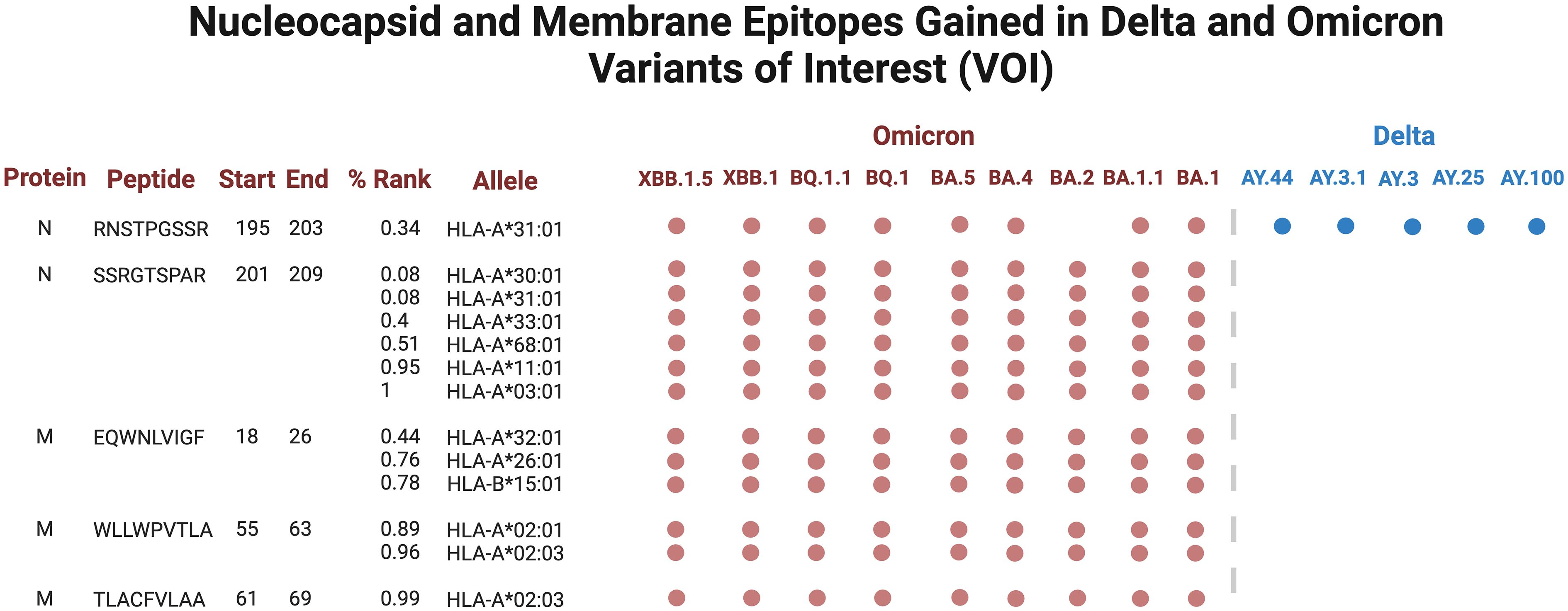
Figure 7 Nucleocapsid (N) and membrane (M) epitopes gained in nine Omicron (colored red) and five Delta (blue) variants when compared against the original Wuhan strain. Figures were generated using BioRender (RRID: SCR_018361).
3.3 Secondary in-silico structural epitope binding using FoldX
Protein-peptide binding free energy of SARS-CoV-2 peptides and HLA-B*15:01 (n = 7, Supplementary Table 1) was computationally determined using FoldX (Tables 1, 2, Supplementary Tables 1–3). HLA-B*15:01 was selected because the allele is both common and has been the focus of recent publications (Tables 3, 4) (47–49). Of the seven HLA-B*15:01 structures downloaded from the Protein Data Bank, only one structure, 8ELG, was complexed with SARS-CoV-2 epitopes (Supplementary Table 1). Protein-peptide binding analysis for all seven HLA-B*15:01 structures returned a 58% match between FoldX-generated binding free energy/ΔG and IEDB-predicted consensus percentile ranks. The predicted binding match rate jumped to 64% in HLA-B*15:01 complexed with Coronaviridae peptides 8ELG and 3C9N (Tables 1, 2, Supplementary Table 1). The nucleocapsid peptide KLDDKGPNF, which had mutated from KLDDKDPNF (Wuhan) to KLDDKGPNF (BA.1.1), exhibited the greatest match rate (85.7% = 6/7; Figure 8, Supplementary Table 2).
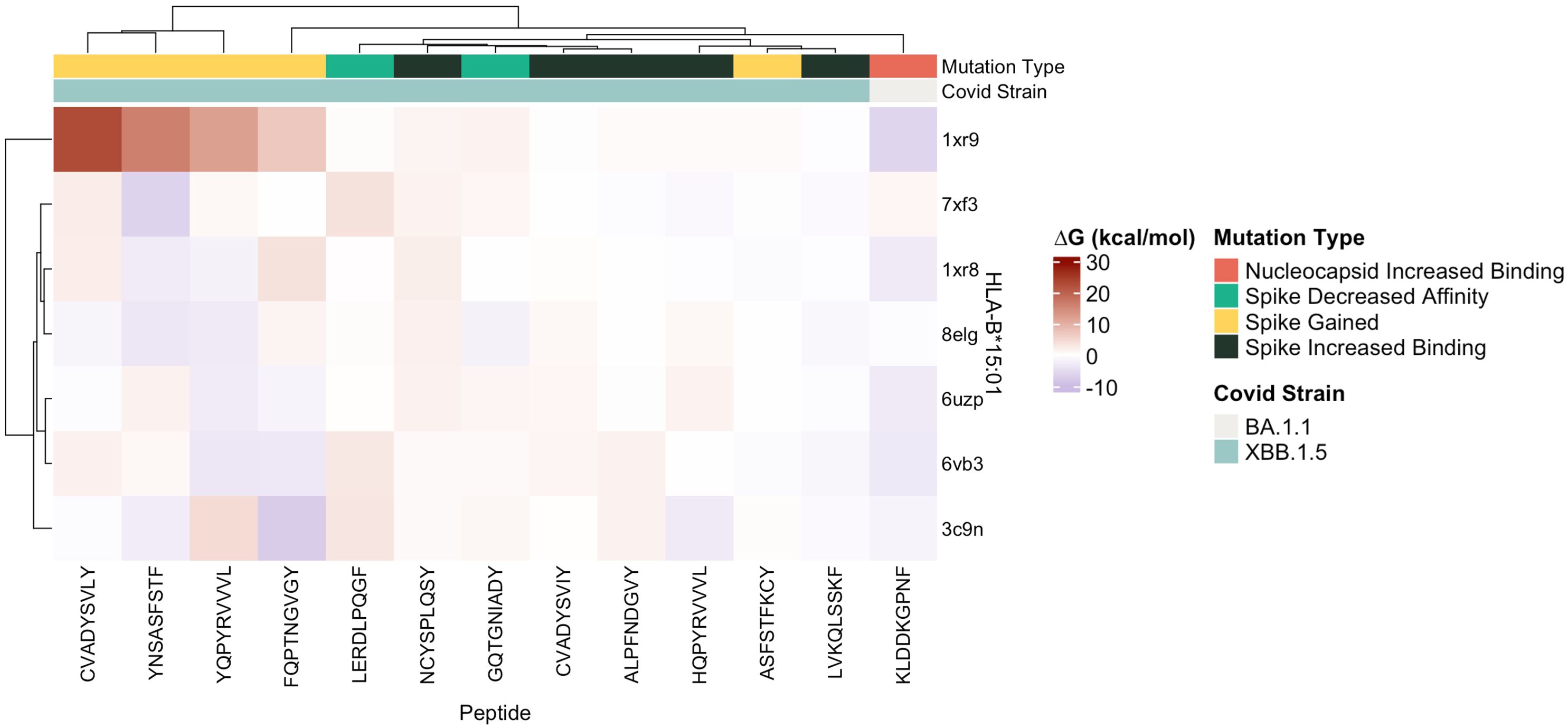
Figure 8 Heatmap of estimated ΔG (kcal/mol) values predicted for ligands docked with target SARS-CoV-2 epitopes with crystalized HLA-B*15:01 structures (n = 7) by FoldX.
3.4 Frequencies of affected HLA alleles in B.1.1.7 S, BQ.1.1 S, BA.1.1 M, and BA.4 N
To estimate how much of the United States population was potentially affected by the unbalanced epitope loss in BA.1.1 M, BA.4 N, and BQ.1.1 S protein variants, we utilized the haplotype frequencies cited by the US National Bone Marrow Donor Program (Table 3) (37). BQ.1.1 S epitopes sustained loss in 16/16 HLA-A alleles (making up approximately 92.3% population in EUR, 69.2% of AFA, 74% of API, 83% of HIS) and in 10/11 HLA-B alleles (62% EUR, 43.5% AFA, 31.2% API, and 38.5% HIS). BQ.1.1 S epitope gain was seen in all 16 HLA-A and 8/11 HLA-B alleles (48% EUR, 36% AFA, 26.2% API, and 29% HIS), with HLA-B*44:02 and HLA-B*44:03 experiencing only lost epitopes.
B.1.1.7 S and BA.4 N epitopes sustained decreased predicted immunogenicity in all 27 HLA alleles analyzed (16/16 HLA-A = 92.3% EUR, 69.2% AFA, 74% API, and 83% HIS; 11/11 HLA-B = 67.6% EUR, 44.8% AFA, 39.2% API, and 39.8% HIS) although only a fraction of HLAs analyzed experienced increased binding affinity in both repertoires (8/16 HLA-A and 6/11 HLA-B alleles in B.1.1.7 S). Likewise, only six HLA-A alleles (27.1% EUR, 23.5% AFA, 27.7% API, and 26% HIS) and one HLA-B allele (18.5% EUR, 9.5% AFA, 8.9% APA, and 11.2% HIS) experienced increased predicted binding in BA.4 N. BA.1.1 M repertoires lost epitopes for 9/16 HLA-A alleles (55.3% EUR, 39.3% AFA, 25.5% API, and 19.4% HIS), while only 4/16 HLA-A alleles experienced gains (35.7% EUR, 15.3% AFA, 17.8% API, and 2.9% HIS). Similarly, 6/11 HLA-B (29.2% EUR, 16% AFA, 27% API, and 19.4% HIS) lost epitopes, with only HLA-B*15:01 and HLA-B*08:01 gaining epitopes (19.1% EUR, 4.8% AFA, 5.1% API, and 7.3% HIS).
3.5 Predicted HLA clinical correlates of CD8+ T-cell epitope diversity
To summarize epitope difference between HLA and variant-specific S, M, and N, the number of unique epitopes experiencing loss, gain, and altered predicted binding was tabulated for the 16 HLA-A alleles and 11 HLA-B alleles analyzed (Table 1). The following equation was utilized to predict clinical severity of the 27 HLA haplotypes analyzed for individual protein products (Equation 1) and SARS-CoV-2 more broadly (Equation 2). Equation 2 was structured to reflect that clinical characteristics are affected by the net CD8+ T-cell epitope repertoire differences for all protein products.
Equation 1: Predicted clinical severity, X, of an HLA allele specific for a SARS-CoV-2 protein product (spike, membrane, or nucleocapsid).
Equation 2: HLA predicted clinical severity for all SARS-CoV-2 VOCs and protein product, spike (S), membrane (M), and nucleocapsid (N).
Utilizing Equation 2, HLA-A*32:01, HLA-A*30:02, HLA-A*26:01, HLA-B*08:01, HLA-B*35:01, and HLA-B*51:01 were predicted to have worse clinical correlates when infected with SARS-CoV-2. Collectively, these six alleles are expected to affect approximately 7.0% of EUR, 9.1% of AFA, 5.3% of API, and 8.4% of HIS population for HLA-A and 22.8% of EUR, 12.5% of AFA, 12.2% of API, and 16.6% of HIS population for HLA-B alleles in the United States. Favorable clinical outcomes were predicted in HLA-A*01:01, HLA-A*02:01, HLA-A*31:01, HLA-A*68:02, HLA-B*15:01, HLA-B*40:01, HLA-B*44:03, and HLA-B*57:01 (Tables 2, 3) (HLA-A: 50% EUR, 24.8% AFA, 17.8% API, and 33.4% HIS; HLA-B: 21.1% EUR, 8.2% AFA, 17.8% API, and 11.5% HIS). Our predicted clinical severity matched the reported clinical observations (42–48, 50), excluding HLA-A*11:01, which is explored further in the discussion (Table 1).
4 Discussion
Select studies have previously sequenced the HLA allele and viral epitopes of convalescent patients (16, 51, 52), but to our knowledge, none have explored the entire epitope repertoire of multiple SARS-CoV-2 variants with respect to the most common HLA allotypes. Although epitope screening has been conducted in cell lines (53, 54), no analysis of the COVID-19 peptidome exists on a population/epidemiological level. Therefore, our team utilized a computational approach aimed to model the immunogenic effects and clinical severity of SARS-CoV-2 variants in the most common MHC class I alleles comprising the United States population. Our bioinformatics analysis is consistent with the percentages of CD8+ epitope conservation (S: 87.6%–96.5%, M: 92.5%–99.6%, N: 94.6%–99%) found by Tarke et al. (15) (97%). As the virus mutated, an increasing proportion of spike epitopes experienced reduced predicted HLA binding, with 70% of Omicron BQ.1–XBB.1.5 S epitope repertoires experiencing decreased predicted HLA binding affinity (as compared with the roughly 3% and 15% affected in Delta AY.100–AY.44 and Omicron BA.1–BA.5 variants, respectively) (Figures 1, 2A). The changes experienced by spike CD8+ epitopes highlight both the remarkable structural plasticity of the S protein and the selective pressures experienced by its gene, particularly following the widespread availability of vaccines in mid-2021 (Figure 1). Our findings suggest that viral genetic variation affecting CD8 T-cell epitope immunogenicity contributes to determining the clinical severity of acute COVID-19.
Our findings support the hypothesis that long-lasting immunity against SARS-CoV-2 variants will be difficult to achieve through vaccines based solely on the spike protein and using neutralizing antibodies as an efficacy endpoint. One strategy to achieve long-term immunity against COVID-19 is the development of T-cell vaccines (9, 55). When designing such vaccines, it is important that the epitopes selected are as invariant as possible and cover the maximum number of HLA haplotypes with even affinity distribution between HLA alleles (56). Our research identified several predicted epitopes that were gained and conserved between variants (Figures 6, 7), including highly conserved nucleocapsid (n = 2) and membrane (n = 3) peptides predicted to elicit immune response through multiple HLA alleles (Figure 7). Additionally, the CD8+ T cell epitopes in this manuscript have been evidenced in previously published datasets (Table 5). To develop a pan-coronavirus vaccine, epitopes affecting conserved protein product regions should also be considered, such as AA 987–1205 in spike, AA 132–222 in membrane, and the AA 66–194 and 210–401 regions in nucleocapsid described in our findings. Lastly, considering that several HLA haplotypes, including HLA-A*11:01, HLA-A*24:02, and HLA-B*08:01, are associated with COVID-induced autoimmune disease (44), epitopes affecting these alleles must be carefully considered to minimize the risk of autoimmune adverse effects. In-silico and in-vitro experiments will be needed to confirm the bioinformatically predicted epitope gains and remove promiscuous peptides.
An alternative path to prevent or treat severe COVID-19 immunity is the development of personalized vaccines and/or treatment strategies. This requires the identification of haplotypes at risk of or protected from severe illness, which can be added to non-genetic risk factors to estimate the overall risk of severe outcomes. Our findings are significant because this study is one of the first to explore SARS-CoV-2 CD8+ epitope diversity in the context of HLA alleles found in most of the United States population. Our predicted clinical severity, Xtotal (Equation 2), is consistent with previously published findings (Tables 2–4, 6, 7) and identified several novel candidate haplotypes that may be susceptible to severe disease, notably HLA-A*32:01, HLA-A*26:01, and HLA-B*53:01, and relatively protected from disease, such as HLA-A*01:01, HLA-A*31:01, HLA-B*40:01, HLA-B*44:03, and HLA-B*57:01 (Tables 2, 3). All referenced clinical associations were consistent with our predicted estimates, except HLA-A*11:01, which was reported to have severe disease and COVID-induced autoimmune effects despite a low Xtotal (−19), and HLA-A*01:01, which was reported to have severe infection in Russia despite a low Xtotal (−17). The inconsistency of predicted/reported severity seen in HLA-A*11:01 may be explained through a combination of factors, including an association with COVID-induced autoimmune disease (42–44) and limited availability of CD8+ hepatitis B epitopes, with some reports (66) suggesting that chronic hepatitis B patients with this allele had less than 10% of known HBV epitopes. Therefore, with these findings being considered (66–68), HLA-A*11:01 patients with chronic, untreated, or poorly managed hepatitis B co-infection may be at greater risk of experiencing severe COVID-19 infection, even if the allele alone may not confer an increased risk of clinical severity. It is also important to be mindful of the considerable diversity generated from HLA polymorphism. A patient heterozygous for both HLA-A and HLA-B loci would have to account for the predicted clinical severity, Xtotal, of all four haplotypes to determine a true net predicted effect (not including the other MHC class I loci, -C). Therefore, clinical studies will be needed to confirm these findings. We hope that our computation study will encourage groups with access to large numbers of peripheral blood mononuclear cells from COVID-19 patients, such as the RECOVER cohorts, to analyze SARS-CoV-2 peptidomes in association with HLA haplotypes.
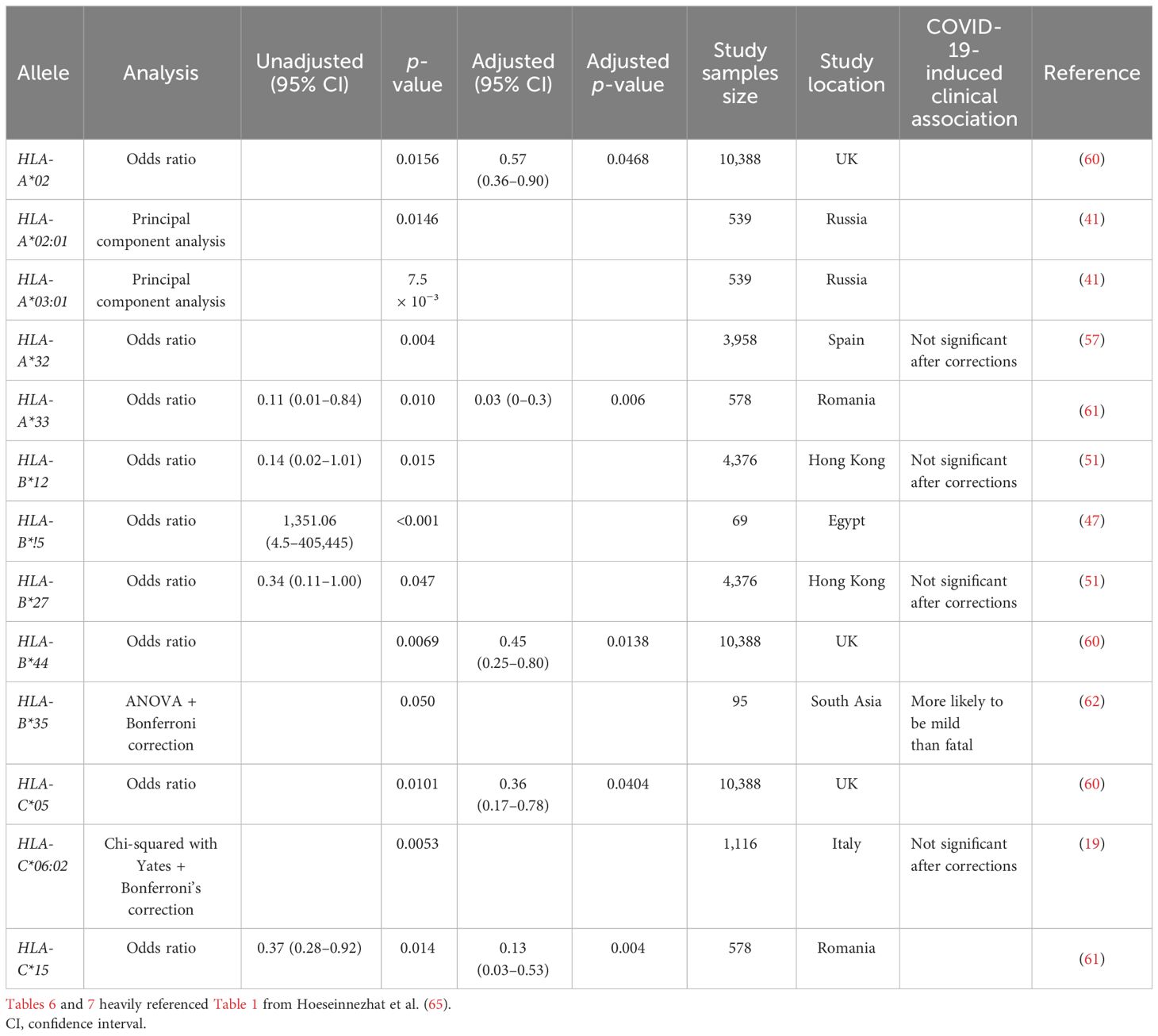
Table 7 Global summary of HLA Class I allele associated with low risk of or protection from COVID-19 infection.
Data availability statement
The data presented in this study are deposited in the Figshare portal, figshare.com/s/e47f99c210177912283a, and github, github.com/elnaggarj/FoldX-PeptideDocking. All SARS-CoV-2 viral sequences generated by the LSUHSC Precision Medicine Laboratory were deposited into both GISAID and NCBI databases and are publicly available.
Ethics statement
The viral sequences used for this study were obtained from nasopharyngeal swab samples collected by Ochsner Health clinics throughout Louisiana as part of routine medical care and retained as medical waste. Collection of these samples was authorized by the Ochsner IRB under protocol # 2021.221. Ochsner Health retained patient identifiers for medical waste under the State of Louisiana pandemic declaration, which mandated reporting of each COVID-19 case. However, no patient identifiers were used in this study. Fully de-identified samples were provided to the LSUHSC Precision Medicine laboratory. Results were analyzed by BIE and returned to Ochsner Health via a secure, HIPAA-compliant server. All samples are already publicly accessible in both GISAID and at the NCBI.
Author contributions
GK: Conceptualization, Data curation, Formal Analysis, Funding acquisition, Investigation, Methodology, Project administration, Visualization, Writing – original draft, Writing – review & editing. JE: Formal Analysis, Methodology, Software, Visualization, Writing – original draft. MV: Formal Analysis, Investigation, Writing – review & editing. AF: Writing – review & editing, Data curation, Project administration. DT: Formal Analysis, Writing – review & editing. RR: Writing – review & editing, Formal Analysis. SL: Writing – review & editing, Formal Analysis. MS: Writing – review & editing, Validation. NN: Writing – review & editing, Validation. EG: Writing – review & editing, Formal Analysis. DF: Data curation, Writing – review & editing, Project administration. JC: Conceptualization, Data curation, Supervision, Writing – review & editing. LM: Conceptualization, Data curation, Funding acquisition, Supervision, Writing – review & editing.
Funding
The author(s) declare financial support was received for the research, authorship, and/or publication of this article. Research reported in this publication was supported by the National Center for Advancing Translational Sciences of the National Institutes of Health under award number TL1TR003106 and by the National Institute of General Medical Sciences under award number U54GM104940. The content is solely the responsibility of the authors and does not necessarily represent the official views of the National Institutes of Health
Acknowledgments
The VBA script used for IEDB T-cell epitope analysis was generated by David Allen White, Jr. Sample collection was conducted at Ochsner Medical Center, New Orleans, LA with assistance provided by Courtney Parke. The authors acknowledge the additional support of Fannie Jackson and Dr. Gordon Love in the Louisiana State University Health Science Center Precision Medicine Laboratory.
Conflict of interest
Authors RR and SL are employed by the company BioInfoExperts, LLC.
The remaining authors declare that the research was conducted in the absence of any commercial or financial relationships that could be construed as a potential conflict of interest.
Publisher’s note
All claims expressed in this article are solely those of the authors and do not necessarily represent those of their affiliated organizations, or those of the publisher, the editors and the reviewers. Any product that may be evaluated in this article, or claim that may be made by its manufacturer, is not guaranteed or endorsed by the publisher.
Author disclaimer
The content is solely the responsibility of the authors and does not necessarily represent the official views of the National Institutes of Health.
Supplementary material
The Supplementary Material for this article can be found online at: https://www.frontiersin.org/articles/10.3389/fimmu.2024.1357731/full#supplementary-material
References
1. Wang Q, Guo Y, Iketani S, Nair MS, Li Z, Mohri H, et al. Antibody evasion by SARS-CoV-2 Omicron subvariants BA.2.12.1, BA.4 and BA.5. Nature. (2022) 608:603–8. doi: 10.1101/2022.05.26.493517
2. Harvey WT, Carabelli AM, Jackson B, Gupta RK, Thomson EC, Harrison EM, et al. SARS-CoV-2 variants, spike mutations and immune escape. Nat Rev Microbiol. (2021) 19:409–24. doi: 10.1038/s41579-021-00573-0
3. Mistry P, Barmania F, Mellet J, Peta K, Strydom A, Viljoen IM, et al. SARS-CoV-2 variants, vaccines, and host immunity. Front Immunol. (2021) 12:809244. doi: 10.3389/fimmu.2021.809244
4. Stanevich OV, Alekseeva EI, Sergeeva M, Fadeev AV, Komissarova KS, Ivanova AA, et al. SARS-CoV-2 escape from cytotoxic T cells during long-term COVID-19. Nat Commun. (2023) 14:149. doi: 10.1038/s41467-022-34033-x
5. Noh JY, Jeong HW, Kim JH, Shin EC. T cell-oriented strategies for controlling the COVID-19 pandemic. Nat Rev Immunol. (2021) 21:687–8. doi: 10.1038/s41577-021-00625-9
6. Baker D, Roberts CAK, Pryce G, Kang AS, Marta M, Reyes S, et al. COVID-19 vaccine-readiness for anti-CD20-depleting therapy in autoimmune diseases. Clin Exp Immunol. (2020) 202:149–61. doi: 10.1111/cei.13495
7. McMahan K, Yu J, Mercado NB, Loos C, Tostanoski LH, Chandrashekar A, et al. Correlates of protection against SARS-CoV-2 in rhesus macaques. Nature. (2021) 590:630–4. doi: 10.1038/s41586-020-03041-6
8. Moss P. The T cell immune response against SARS-CoV-2. Nat Immunol. (2022) 23:186–93. doi: 10.1038/s41590-021-01122-w
9. Dolgin E. T-cell vaccines could top up immunity to COVID, as variants loom large. Nat Biotechnol. (2022) 40:3–4. doi: 10.1038/d41587-021-00025-3
10. Yang Y, Miller H, Byazrova MG, Cndotti F, Benlagha K, Camara NOS, et al. The characterization of CD8+ T-cell responses in COVID-19. Emerg Microbes Infect. (2023) 13:2287118. doi: 10.1080/22221751.2023.2287118
11. Parham P, Janeway C. The immune system. Fourth edition Vol. 1. New York, NY: Garland Science, Taylor & Francis Group (2015).
12. Wieczorek M, Abualrous ET, Sticht J, Alvaro-Benito M, Stolzenberg S, Noe F, et al. Major histocompatibility complex (MHC) class I and MHC class II proteins: conformational plasticity in antigen presentation. Front Immunol. (2017) 8:292. doi: 10.3389/fimmu.2017.00292
13. Tavasolian F, Rashidi M, Hatam GR, Jeddi M, Hosseini AZ, Mosawi SH, et al. HLA, immune response, and susceptibility to COVID-19. Front Immunol. (2020) 11:601886. doi: 10.3389/fimmu.2020.601886
14. Fricke-Galindo I, Falfan-Valencia R. Genetics insight for COVID-19 susceptibility and severity: A review. Front Immunol. (2021) 12:622176. doi: 10.3389/fimmu.2021.622176
15. Tarke A, Sidney J, Methot N, Yu ED, Zhang Y, Dan JM, et al. Impact of SARS-CoV-2 variants on the total CD4(+) and CD8(+) T cell reactivity in infected or vaccinated individuals. Cell Rep Med. (2021) 2:100355. doi: 10.1016/j.xcrm.2021.100355
16. Ferretti AP, Kula T, Wang Y, Nguyen DMV, Weinheimer A, Dunlap GS, et al. Unbiased screens show CD8(+) T cells of COVID-19 patients recognize shared epitopes in SARS-CoV-2 that largely reside outside the spike protein. Immunity. (2020) 53:1095–107.e3. doi: 10.1016/j.immuni.2020.10.006
17. Keeton R, Tincho MB, Ngomti A, Baguma R, Benede N, Suzuki A, et al. T cell responses to SARS-CoV-2 spike cross-recognize Omicron. Nature. (2022) 603:488–92. doi: 10.1038/s41586-022-04460-3
18. Jin X, Liu X, Shen C. A systemic review of T-cell epitopes defined from the proteome of SARS-CoV-2. Virus Res. (2023) 324:199024. doi: 10.1016/j.virusres.2022.199024
19. Novelli A, Andreani M, Biancolella M, Liberatoscioli L, Passarelli C, Colona VL, et al. HLA allele frequencies and susceptibility to COVID-19 in a group of 99 Italian patients. HLA. (2020) 96:610–4. doi: 10.1111/tan.14047
20. Nguyen A, David JK, Maden SK, Wood MA, Weeder BR, Nellore A, et al. Human leukocyte antigen susceptibility map for severe acute respiratory syndrome coronavirus 2. J Virol. (2020) 94:e00510–20. doi: 10.1128/JVI.00510-20
21. Guerini FR, Bolognesi E, Lax A, Bianchi LNC, Caronni A, Zanzottera M, et al. HLA allele frequencies and association with severity of COVID-19 infection in northern italian patients. Cells. (2022) 11:1792. doi: 10.3390/cells11111792
22. Tao K, Tzou PL, Nouhin J, Gupta RK, de Oliveira T, Kosakovsky Pond SL, et al. The biological and clinical significance of emerging SARS-CoV-2 variants. Nat Rev Genet. (2021) 22:757–73. doi: 10.1038/s41576-021-00408-x
23. Tregoning JS, Flight KE, Higham SL, Wang Z, Pierce BF. Progress of the COVID-19 vaccine effort: viruses, vaccines and variants versus efficacy, effectiveness and escape. Nat Rev Immunol. (2021) 21:626–36. doi: 10.1038/s41577-021-00592-1
24. Su Y, Yuan D, Chen DG, Ng RH, Wang K, Choi J, et al. Multiple early factors anticipate post-acute COVID-19 sequelae. Cell. (2022) 185:881–95.e20. doi: 10.1016/j.cell.2022.01.014
25. Peluso MJ, Deitchman AN, Torres L, Iyer NS, Munter SE, Nixon CC, et al. Long-term SARS-CoV-2-specific immune and inflammatory responses in individuals recovering from COVID-19 with and without post-acute symptoms. Cell Rep. (2021) 36:109518. doi: 10.1016/j.celrep.2021.109518
26. Patterson BK, Guevara-Coto J, Yogendra R, Francisco EB, Long E, Pise A, et al. Immune-based prediction of COVID-19 severity and chronicity decoded using machine learning. Front Immunol. (2021) 12:700782. doi: 10.3389/fimmu.2021.700782
27. Duvaud S, Gabella C, Lisacek F, Stockinger H, Ioannidis V, Durinx C. Expasy, the Swiss Bioinformatics Resource Portal, as designed by its users. Nucleic Acids Res. (2021) 49:W216–W27. doi: 10.1093/nar/gkab225
28. Paul S, Sidney J, Sette A, Peters B. TepiTool: A pipeline for computational prediction of T cell epitope candidates. Curr Protoc Immunol. (2016) 114:1891–924. doi: 10.1002/cpim.12
29. Howe KL, Achuthan P, Allen J, Allen J, Alvarez-Jarreta J, Amode MR, et al. Ensembl 2021. Nucleic Acids Res. (2021) 49:D884–D91. doi: 10.1093/nar/gkaa942
30. Bolger AM, Lohse M, Usadel B. Trimmomatic: a flexible trimmer for Illumina sequence data. Bioinformatics. (2014) 30:2114–20. doi: 10.1093/bioinformatics/btu170
31. Langmead B, Salzberg SL. Fast gapped-read alignment with Bowtie 2. Nat Methods. (2012) 9:357–9. doi: 10.1038/nmeth.1923
32. Danecek P, Bonfield JK, Liddle J, Marshall J, Ohan V, Pollard MO, et al. Twelve years of SAMtools and BCFtools. Gigascience. (2021) 10:giab008. doi: 10.1093/gigascience/giab008
33. Vita R, Mahajan S, Overton JA, Dhanda SK, Martini S, Cantrell JR, et al. The immune epitope database (IEDB): 2018 update. Nucleic Acids Res. (2019) 47:D339–D43. doi: 10.1093/nar/gky1006
34. Mazumder L, Hasan MR, Fatema K, Begum S, Azad AK, Islam MA. Identification of B and T cell epitopes to design an epitope-based peptide vaccine against the cell surface binding protein of monkeypox virus: an immunoinformatics study. J Immunol Res. (2023) 2023:2274415. doi: 10.1155/2023/2274415
35. Berman HM, Westbrook J, Feng Z, Gilliland G, Bhat TN, Weissig H, et al. The protein data bank. Nucleic Acids Res. (2000) 28:235–42. doi: 10.1093/nar/28.1.235
36. Gu Z, Eils R, Schlesner M. Complex heatmaps reveal patterns and correlations in multidimensional genomic data. Bioinformatics. (2016) 32:2847–9. doi: 10.1093/bioinformatics/btw313
37. Gragert L, Madbouly A, Freeman J, Maiers M. Six-locus high resolution HLA haplotype frequencies derived from mixed-resolution DNA typing for the entire US donor registry. Hum Immunol. (2013) 74:1313–20. doi: 10.1016/j.humimm.2013.06.025
38. UniProt C. UniProt: the universal protein knowledgebase in 2023. Nucleic Acids Res. (2023) 51:D523–D31. doi: 10.1093/nar/gkac1052
39. Mushtaq MZ, Nasir N, Mahmood SF, Khan S, Kanji A, Nasir A, et al. Exploring the relationship between SARS-CoV-2 variants, illness severity at presentation, in-hospital mortality and COVID-19 vaccination in a low middle-income country: A retrospective cross-sectional study. Health Sci Rep. (2023) 6:e1703. doi: 10.1002/hsr2.1703
40. Yuan Z, Shao Z, Ma L, Guo R. Clinical severity of SARS-CoV-2 variants during COVID-19 vaccination: A systematic review and meta-analysis. Viruses. (2023) 15:1994. doi: 10.3390/v15101994
41. Shkurnikov M, Nersisyan S, Jankevic T, Galatenko A, Gordeev I, Vechorko V, et al. Association of HLA class I genotypes with severity of coronavirus disease-19. Front Immunol. (2021) 12:641900. doi: 10.3389/fimmu.2021.641900
42. Khor SS, Omae Y, Nishida N, Sugiyama M, Kinoshita N, Suzuki T, et al. HLA-A*11:01:01:01, HLA-C*12:02:02:01-HLA-B*52:01:02:02, age and sex are associated with severity of Japanese COVID-19 with respiratory failure. Front Immunol. (2021) 12:658570. doi: 10.3389/fimmu.2021.658570
43. Wang F, Huang S, Gao R, Zhou Y, Lai C, Li Z, et al. Initial whole-genome sequencing and analysis of the host genetic contribution to COVID-19 severity and susceptibility. Cell Discovery. (2020) 6:83. doi: 10.1038/s41421-020-00231-4
44. Karami Fath M, Jahangiri A, Ganji M, Sefid F, Payandeh Z, Hashemi ZS, et al. SARS-CoV-2 proteome harbors peptides which are able to trigger autoimmunity responses: implications for infection, vaccination, and population coverage. Front Immunol. (2021) 12:705772. doi: 10.3389/fimmu.2021.705772
45. Schindler E, Dribus M, Duffy BF, Hock K, Farnsworth CW, Gragert L, et al. HLA genetic polymorphism in patients with Coronavirus Disease 2019 in Midwestern United States. HLA. (2021) 98:370–9. doi: 10.1111/tan.14387
46. Dobrijevic Z, Gligorijevic N, Sunderic M, Penezic A, Miljus G, Tomic S, et al. The association of human leucocyte antigen (HLA) alleles with COVID-19 severity: A systematic review and meta-analysis. Rev Med Virol. (2023) 33:e2378. doi: 10.1002/rmv.2378
47. Abdelhafiz AS, Ali A, Fouda MA, Sayed DM, Kamel MM, Kamal LM, et al. HLA-B*15 predicts survival in Egyptian patients with COVID-19. Hum Immunol. (2022) 83:10–6. doi: 10.1016/j.humimm.2021.09.007
48. Augusto DG, Hollenbach JA. HLA variation and antigen presentation in COVID-19 and SARS-CoV-2 infection. Curr Opin Immunol. (2022) 76:102178. doi: 10.1016/j.coi.2022.102178
49. Augusto DG, Yusufali T, Sabatino JJ Jr., Peyser ND, Murdolo LD, Butcher X, et al. A common allele of HLA mediates asymptomatic SARS-CoV-2 infection. medRxiv. (2022). doi: 10.1101/2021.05.13.21257065
50. Sahin Tekin M, Yorulmaz G, Yantir E, Gunduz E, Colak E. A novel finding of an HLA allele’s and a haplotype’s relationship with SARS-CoV-2 vaccine-associated subacute thyroiditis. Vaccines (Basel). (2022) 10:1986. doi: 10.3390/vaccines10121986
51. Yung YL, Cheng CK, Chan HY, Xia JT, Lau KM, Wong RSM, et al. Association of HLA-B22 serotype with SARS-CoV-2 susceptibility in Hong Kong Chinese patients. HLA. (2021) 97:127–32. doi: 10.1111/tan.14135
52. Vishnubhotla R, Sasikala M, Ketavarapu V, Reddy DN. High-resolution HLA genotyping identifies alleles associated with severe COVID-19: A preliminary study from India. Immun Inflammation Dis. (2021) 9:1781–5. doi: 10.1002/iid3.481
53. Weingarten-Gabbay S, Klaeger S, Sarkizova S, Pearlman LR, Chen DY, Gallagher KME, et al. Profiling SARS-CoV-2 HLA-I peptidome reveals T cell epitopes from out-of-frame ORFs. Cell. (2021) 184:3962–80 e17. doi: 10.1016/j.cell.2021.05.046
54. Nagler A, Kalaora S, Barbolin C, Gangaev A, Ketelaars SLC, Alon M, et al. Identification of presented SARS-CoV-2 HLA class I and HLA class II peptides using HLA peptidomics. Cell Rep. (2021) 35:109305. doi: 10.1016/j.celrep.2021.109305
55. Sepand MR, Bigdelou B, Ho JQ, Sharaf M, Lannigan AJ, Sullivan IM, et al. Long-term immunity and antibody response: challenges for developing efficient COVID-19 vaccines. Antibodies (Basel). (2022) 11:35. doi: 10.3390/antib11020035
56. Kim Y, Sette A, Peters B. Applications for T-cell epitope queries and tools in the Immune Epitope Database and Analysis Resource. J Immunol Methods. (2011) 374:62–9. doi: 10.1016/j.jim.2010.10.010
57. Lorente L, Martin MM, Franco A, Barrios Y, Caceres JJ, Sole-Violan J, et al. HLA genetic polymorphisms and prognosis of patients with COVID-19. Med Intensiva (Engl Ed). (2021) 45:96–103. doi: 10.1016/j.medin.2020.08.004
58. Mashayekhi P, Omrani MD, Yassin Z, Dehghanifard A, Ashouri L, Aghabozorg Afjeh SS, et al. Influence of HLA-A, -B, -DR polymorphisms on the severity of COVID-19: A case-control study in the Iranian population. Arch Iran Med. (2023) 26:261–6. doi: 10.34172/aim.2023.40
59. Littera R, Campagna M, Deidda S, Angioni G, Cipri S, Melis M, et al. Human leukocyte antigen complex and other immunogenetic and clinical factors influence susceptibility or protection to SARS-CoV-2 infection and severity of the disease course. Sardinian Experience. Front Immunol. (2020) 11:605688. doi: 10.3389/fimmu.2020.605688
60. Poulton K, Wright P, Hughes P, Savic S, Welberry Smith M, Guiver M, et al. A role for human leucocyte antigens in the susceptibility to SARS-Cov-2 infection observed in transplant patients. Int J Immunogenet. (2020) 47:324–8. doi: 10.1111/iji.12505
61. Vica ML, Dobreanu M, Curocichin G, Matei HV, Balici S, Vuscan ME, et al. The influence of HLA polymorphisms on the severity of COVID-19 in the Romanian population. Int J Mol Sci. (2024) 25:1326. doi: 10.3390/ijms25021326
62. Naemi FMA, Al-Adwani S, Al-Khatabi H, Al-Nazawi A. Association between the HLA genotype and the severity of COVID-19 infection among South Asians. J Med Virol. (2021) 93:4430–7. doi: 10.1002/jmv.27003
63. Hovhannisyan A, Madelian V, Avagyan S, Nazaretyan M, Hyussyan A, Sirunyan A, et al. HLA-C*04:01 affects HLA class I heterozygosity and predicted affinity to SARS-CoV-2 peptides, and in combination with age and sex of Armenian patients contributes to COVID-19 severity. Front Immunol. (2022) 13:769900. doi: 10.3389/fimmu.2022.769900
64. Sakuraba A, Haider H, Sato T. Population difference in allele frequency of HLA-C*05 and its correlation with COVID-19 mortality. Viruses. (2020) 12:1333. doi: 10.3390/v12111333
65. Hoseinnezhad T, Soltani N, Ziarati S, Behboudi E, Mousavi MJ. The role of HLA genetic variants in COVID-19 susceptibility, severity, and mortality: A global review. J Clin Lab Anal. (2024) 38:e25005. doi: 10.1002/jcla.25005
66. Chen X, Wang W, Wang S, Meng G, Zhang M, Ni B, et al. An immunodominant HLA-A*1101-restricted CD8+ T-cell response targeting hepatitis B surface antigen in chronic hepatitis B patients. J Gen Virol. (2013) 94:2717–23. doi: 10.1099/vir.0.052167-0
67. Liu Z, Song L, Chen J, Zhou Y, Wang Y, Tang L, et al. Causal associations between chronic hepatitis B and COVID-19 in East Asian populations. Virol J. (2023) 20:109. doi: 10.1186/s12985-023-02081-4
Keywords: SARS-CoV-2, T cell epitope, COVID-19, bioinformatics, CD8 T cell epitope, HLA, vaccine design
Citation: Kim GJ, Elnaggar JH, Varnado M, Feehan AK, Tauzier D, Rose R, Lamers SL, Sevalia M, Nicholas N, Gravois E, Fort D, Crabtree JS and Miele L (2024) A bioinformatic analysis of T-cell epitope diversity in SARS-CoV-2 variants: association with COVID-19 clinical severity in the United States population. Front. Immunol. 15:1357731. doi: 10.3389/fimmu.2024.1357731
Received: 18 December 2023; Accepted: 05 April 2024;
Published: 09 May 2024.
Edited by:
Gabriela Angélica Martínez-Nava, National Institute of Rehabilitation Luis Guillermo Ibarra Ibarra, MexicoCopyright © 2024 Kim, Elnaggar, Varnado, Feehan, Tauzier, Rose, Lamers, Sevalia, Nicholas, Gravois, Fort, Crabtree and Miele. This is an open-access article distributed under the terms of the Creative Commons Attribution License (CC BY). The use, distribution or reproduction in other forums is permitted, provided the original author(s) and the copyright owner(s) are credited and that the original publication in this journal is cited, in accordance with accepted academic practice. No use, distribution or reproduction is permitted which does not comply with these terms.
*Correspondence: Lucio Miele, Lmiele@LSUHSC.edu