- 1Department of Critical Care Medicine & Department of Emergency Medicine, Xiangyang Central Hospital, Affiliated Hospital of Hubei University of Arts and Science, Xiangyang, Hubei, China
- 2Medical College, Hubei University of Arts and Science, Xiangyang, Hubei, China
- 3Institute of Neuroscience and Brain Diseases, Xiangyang Central Hospital, Affiliated Hospital of Hubei University of Arts and Science, Xiangyang, Hubei, China
- 4Gucheng People’s Hospital, Affiliated Hospital of Hubei University of Arts and Science, Xiangyang, Hubei, China
Introduction: Acute respiratory distress syndrome (ARDS) is a major cause of death among critically ill patients in intensive care settings, underscoring the need to identify biomarkers capable of predicting ARDS patient clinical status and prognosis at an early time point. This study specifically sought to explore the utility and clinical relevance of TM9SF1 as a biomarker for the early prediction of disease severity and prognostic outcomes in patients with ARDS.
Methods: This study enrolled 123 patients with severe ARDS and 116 patients with non-severe ARDS for whom follow-up information was available. The mRNA levels of TM9SF1 and cytokines in peripheral blood mononuclear cells from these patients were evaluated by qPCR. The predictive performance of TM9SF1 and other clinical indicators was evaluated using received operating characteristic (ROC) curves. A predictive nomogram was developed based on TM9SF1 expression and evaluated for its ability in the early prediction of severe disease and mortality in patients with ARDS.
Results: TM9SF1 mRNA expression was found to be significantly increased in patients with severe ARDS relative to those with non-severe disease or healthy controls. ARDS severity increased in correspondence with the level of TM9SF1 expression (odds ratio [OR] = 2.43, 95% confidence interval [CI] = 2.15–3.72, P = 0.005), and high TM9SF1 levels were associated with a greater risk of mortality (hazard ratio [HR] = 2.27, 95% CI = 2.20–4.39, P = 0.001). ROC curves demonstrated that relative to other clinical indicators, TM9SF1 offered superior performance in the prediction of ARDS severity and mortality. A novel nomogram incorporating TM9SF1 expression together with age, D-dimer levels, and C-reactive protein (CRP) levels was developed and was used to predict ARDS severity (AUC = 0.887, 95% CI = 0.715–0.943). A separate model incorporating TM9SF1 expression, age, neutrophil-lymphocyte ratio (NLR), and D-dimer levels (C-index = 0.890, 95% CI = 0.627–0.957) was also developed for predicting mortality.
Conclusion: Increases in ARDS severity and patient mortality were observed with rising levels of TM9SF1 expression. TM9SF1 may thus offer utility as a novel biomarker for the early prediction of ARDS patient disease status and clinical outcomes.
1 Introduction
Acute respiratory distress syndrome (ARDS) is a serious clinical condition wherein extensive alveolar capillary injury occurs following pulmonary insults such as shock, trauma, or severe infection, resulting in characteristic symptoms including tachypnea, distress, and progressive hypoxemia together with diffuse alveolar infiltration evident on X-ray imaging (1, 2). While there have been many improvements to the management of ARDS in patients over recent years such as the advent of strategies for mechanical ventilation that protect lung integrity (3, 4), prone positioning, and extracorporeal membrane oxygenation (5), adequately treating ARDS patients in intensive care unit (ICU) settings remains a pressing challenge. ARDS affects a large percentage of critical patients admitted to the ICU (6), culminating in a mortality rate as high as 35% among these severely ill individuals (3). In recent reports, disease severity was found to be strongly dependent on a range of host factors, underscoring the importance of fully characterizing these individual responses at a molecular level (7, 8). While severe genes reportedly linked with ARDS progression have been identified to date, the predictive performance as tools for evaluating disease prognosis and severity remains unsatisfactory. These factors thus hamper clinical efforts to predict the deterioration of patient vital status during the earlier stages of disease. There is thus a critical need for the investigation of individualized biomarkers capable of predicting ARDS patient status and clinical outcomes.
At the tissue level, ARDS pathophysiology is characterized by diffuse alveolar epithelial cell apoptosis or necrosis as a result of exposure to pathogens or noninfectious irritants (9, 10), with the clinical status of affected patients thus being profoundly shaped by multiple proinflammatory mechanisms. ARDS and associated progression to the development of multi-organ failure (MOF) have been proposed as forms of secondary bystander tissue damage that lie along a spectrum and stem from the effects of dysregulated or excessive inflammatory responses to a primary infectious or non-infectious insult (11). Factors associated with the regulation of such inflammation thus hold great promise as potential biomarkers that may be analyzed to more reliably assess ARDS severity and the prognosis of affected patients.
In a previous study, transmembrane 9 superfamily member 1 (TM9SF1) was identified as a key player in the processes that shape inflammatory lung injury (12). TM9SF1 is a TM9SF family protein with 9 transmembrane domains and a large non-cytoplasmic region, and its expression at the mRNA level is altered in a mouse model of lipopolysaccharide (LPS)-induced acute lung injury (12). Mice in which TM9SF1 has been knocked out also presented with significant changes in lung injury progression, including altered inflammatory cytokine expression and infiltration by inflammatory cells. Given these prior results linking the expression of TM9SF1 to immune regulation and inflammatory lung injury progression, this suggested the possibility that changes in TM9SF1 levels may play a role in ARDS incidence and the development of additional severe lung injury as a consequence of its regulatory effects on immune-mediated inflammatory activity.
In this study, a prospective cohort analysis was conducted enrolling both severe and non-severe ARDS patients admitted to Xiangyang Central Hospital, as well as healthy control (HC) subjects collected during this same period of time. These analyses were designed to clarify the differences in TM9SF1 expression in ARDS patients, to better probe the clinical relevance and possible functional role of TM9SF1 as a novel personalized biomarker for the early-stage prediction of ARDS severity and patient prognosis, and to construct predictive models capable of the early identification of patients with severe disease and gauging their prognosis.
2 Materials and methods
2.1 Study population
This was a prospective cohort study of adult ARDS patients admitted to the medical floor or the medical ICU of Xiangyang Central Hospital from November 1, 2022 through January 31, 2023, with follow-up having been performed through March 31, 2023. Patients were considered eligible for the study if they had been admitted to the hospital for symptoms consistent with ARDS caused by pneumonia, aspiration, trauma, and sepsis, among other conditions. ARDS was diagnosed as per the Berlin Definition.
ARDS disease severity was used to classify patients into severe and nonsevere groups (13). Patients were classified in the severe group if they were admitted to the ICU and exhibited any: (1) respiratory failure, as characterized by a respiratory rate ≥ 30 breaths/min and resting arterial oxygen saturation ≤ 93% mainly for patients without invasive mechanical ventilation; and/or (2) an arterial blood oxygen partial pressure/fraction of inspiration O2 (PaO2/FiO2) ≤ 150 mmHg mainly for those with invasive mechanical ventilation. Patients were classified in the nonsevere group if they were hospitalized and admitted to the internal medicine or pneumology wards without the need for critical care. Any ARDS patients not meeting the criteria set forth for the severe group were included as nonsevere cases.
ARDS patients were excluded from this study if they met any of the following criteria: (1) patients ≤ 18 years of age; (2) patients that died within 24 h following admission; (3) patients with a history of chronic respiratory disease (such as chronic obstructive pulmonary disease and chronic pulmonary fibrosis), immunodeficiency, or malignant tumors; (4) patients for whom severity status was not established; or (5) patients missing details regarding age, sex, or the results of key clinical laboratory tests. In addition, 52 healthy subjects who had undergone physical examinations in the Physical Examination Center and who generally matched the age and sex distributions of the enrolled ARDS patients were included in this study as HCs.
2.2 Clinical details and sample collection
Basic ARDS patient clinical data including demographic, clinical, and laboratory data were extracted from electronic medical records by trained research staff using standardized forms.
Laboratory results collected within 24 h of patient admission were collected for analysis, including results pertaining to the following: white blood cells (WBCs), hemoglobin (HGB), hematocrit (HCT), lymphocytes, lymphocyte % (LYM%), monocytes, monocyte % (MON%), neutrophils, neutrophil % (NEU%), platelet lymphocyte ratio (PLR), monocyte lymphocyte ratio (MLR), neutrophil-lymphocyte ratio (NLR), platelets (PLTs), platelet distribution width (PDW), mean platelet volume (MPV), C-reactive protein (CRP), procalcitonin (PCT), activated partial thromboplastin time (APTT), prothrombin time (PT), thrombin time (TT), D-dimer, international normalized ratio (INR), and erythrocyte sedimentation rate (ESR). Oxygenation status in ARDS patients was evaluated based on the PaO2/FiO2 ratio, while the numbers of organ failures on ICU admission were assessed based on Sequential Organ Failure Assessment (SOFA) scores (14).
The biochemical test results for these patients detailed above were collected through analyses conducted with an Atellica Solution Immunoassay and Clinical Chemistry Analyzer (Siemens Healthcare Diagnostics, Erlangen, Germany). WBCs and lymphocytes were estimated with a Sysmex XE-2100 (TOA Medical Electronics, Kobe, Japan), while a Sysmex CS-5100 System (Siemens Healthcare Diagnostics, Erlangen, Germany) was used to measure APTT, PT, INR, fibrinogen, and D-dimer levels. Levels of CRP were quantified with an i-CHROMA instrument (BodiTech Med Inc., Chuncheon, Korea), whereas the Vacuette ESR System (Greiner Bio-One GmbH, Frickenhausen, Germany) was used to quantify ESR values.
The blood samples analyzed for this study were obtained on enrollment from remaining blood used for routine complete blood counts in the clinic after admission to the ICU or clinical ward. Additionally, blood samples from 61 ARDS patients were obtained when patients were both experiencing severe disease and in stable remission. “Remission” was defined as successful completion of the SBT test for 120 min and with no need for re-intubation after extubation for at least 48 h or tracheotomy status after invasive mechanical ventilation without ventilator support lasting longer than 48 h.
2.3 Follow-up
Patients were followed from the time of hospital admission to discharge or death, with no interference with medical decision-making for the enrolled patients. The follow-up for the patients included in this study continued until March 31, 2023. The endpoint for follow-up for patients with severe ARDS was death or recovery from illness. The outcome-related information was collected for further analysis, including survival status, discharge date, days of hospitalization, and date of death date.
2.4 Separation of peripheral blood mononuclear cells
Blood samples remaining in the clinic after routine complete blood count (CBC) examinations were collected for the separation of PBMCs. The PBMCs were isolated from 2 mL whole blood by density centrifugation at 3000 rpm for 20 min with 3 mL of Human Lymphocyte Separation Medium (Solarbio, P8610), with collection of the PBMCs from the second layer (layers from the top to the bottom: plasma, PBMCs, separation medium, and erythrocyte layers). The PBMCs were resuspended in 1 mL of TRIzol reagent (Invitrogen, 15596026) prior to storage at – 80 °C.
2.5 RNA extraction
The PBMC samples in TRIzol were removed from the –80°C freezer and were thawed on ice before the addition of 200 μL of chloroform, followed by thorough mixing and incubation on ice for 15 min. The samples were then centrifuged at 12 000 rpm for 15 min at 4°C. The upper aqueous phase was transferred to another EP tube, taking care not to aspirate the interphase, and an equal volume of isopropanol was added, followed by thorough mixing and incubation on ice for 15 min. This was followed by further centrifugation (12 000 rpm, 15 min, 4°C), the supernatant was discarded, and the RNA was allowed to pellet at the bottom of the tube. After the addition of 1 mL of 75% ethanol, the pellet was resuspended with gentle shaking, and centrifuged (7500 rpm, 5 min, 4°C). The supernatant was discarded as much as possible and the pellet was air-dried at room temperature for 5–10 min. The RNA was dissolved in 40 μL of 0.1% DEPC water (adding DEPC water according to the amount of pellet) and stored at -20°C until use.
2.6 Reverse transcription
The RNA was reverse-transcribed to cDNA using an iScript cDNA Synthesis Kit (Bio-Rad, 1708891) according to the provided directions. The reverse transcription was performed in a reaction volume of 20 µL, including 15 μL of RNA solution together with 1000 ng RNA, 4 μL of 5x reverse-transcription reaction mix, and 1μL of iScript reverse transcriptase at 25°C for 5 min, followed by 46°C for 5 min and 95°C for 1 min. After the reaction, the cDNA was collected and stored at 4°C or -20°C.
2.7 qPCR
Relative gene expression levels were calculated using the 2-ΔΔCt method, using GAPDH as a reference as in prior reports (15). Primer sequences used for this study are presented in Supplementary Table S1. qPCR was performed on the ABI7500 system by using the iTaq Universal SYBR Green Supermix (Bio-Rad, 1725122). The reaction volume was 20 µL consisting of 3.0 μL of diluted cDNA, 1.0 μL of the forward and reverse primers (10 μM), 10 μL of iTaq Universal SYBR Green Supermix (Bio-Rad, 1725122), and 5 µL of water with the following conditions: 95°C for 5 min; 40 cycles at 95°C for 10 s; 60°C for 20 s.
2.8 Western blotting
Western blotting was performed as previously described (16). The primary antibodies used for the reactions included anti- GAPDH (GoodHere, AB-P-R 001, China), and anti-TM9SF1 (PA5–84406, Invitrogen, USA).
2.9 Statistical analysis
Continuous data are presented as means ± standard deviation, while qualitative data are presented as N (percentage), and these data types were respectively compared with Student’s t-tests or chi-square tests, with one-way ANOVAs being used to compare 3 or more groups. Linear correlation analyses were used to assess correlations between TM9SF1 expression and cytokines. The association between TM9SF1 levels and ARDS severity was examined through univariate and multivariate logistic regression analyses in which odds ratios (ORs) and 95% confidence intervals (CIs) were calculated. The composite outcome of disease progression and death was analyzed with Cox regression analyses, calculating hazard ratios (HRs) and corresponding 95% CIs. Analyses were adjusted for demographic characteristics including age, sex, smoking status, drinking status, and history of disease.
The predictive performance of TM9SF1, other key indicators, and developed predictive models as tools for assessing ARDS severity and mortality was evaluated using receiver operating characteristic (ROC) curves. Predictive models for ARDS severity and mortality were developed through a multivariate analysis of those variables significant in univariate logistic regression analyses (P < 0.05), incorporating all variables that remained significant (P < 0.05) after adjustment for a range of covariates. Multivariate analysis results were also used to construct a nomogram related to ARDS severity. Nomogram performance was evaluated through discrimination analyses based on the concordance index and through the use of calibration curves and the Hosmer–Lemeshow calibration test, with bootstrapping (1,000x resampling).
The threshold for significance was a two-sided P-value < 0.05. Figures were constructed with GraphPad Prism 5 and R v3.6.3, while all statistical analyses were performed with SAS 9.4 software (SAS Institute, NC, USA).
3 Results
3.1 Study participant characteristics
Between November 1, 2022 and January 31, 2023, 239 total adult ARDS patients who were admitted to Xiangyang Central Hospital were enrolled in this study. Of these patients, 123 and 116 were respectively diagnosed with severe and nonsevere ARDS. ARDS patient demographic and clinical characteristics are presented in Table 1. Sex did not differ significantly between groups (female: 42.2% vs. 47.2%, P = 0.445), whereas the average age of severe ARDS patients was higher than that of individuals with nonsevere disease (73.67 ± 13.26 vs. 65.98 ± 17.03 , P < 0.001). Of these ARDS patients, 68.2% exhibited at least one comorbidity, among which hypertension (43.9%), diabetes mellitus (26.4%), and cardiovascular disease (18.0%) were the most common. Patients with severe disease were more likely than nonsevere patients to have hypertension (Table 1). The median duration of hospitalization for the overall ARDS patient cohort was 15 [8–23] days, with a significantly longer duration of hospitalization among individuals with severe disease as compared to those with nonsevere disease (19 [6–45] vs. 12 [8–18] days, P = 0.017).
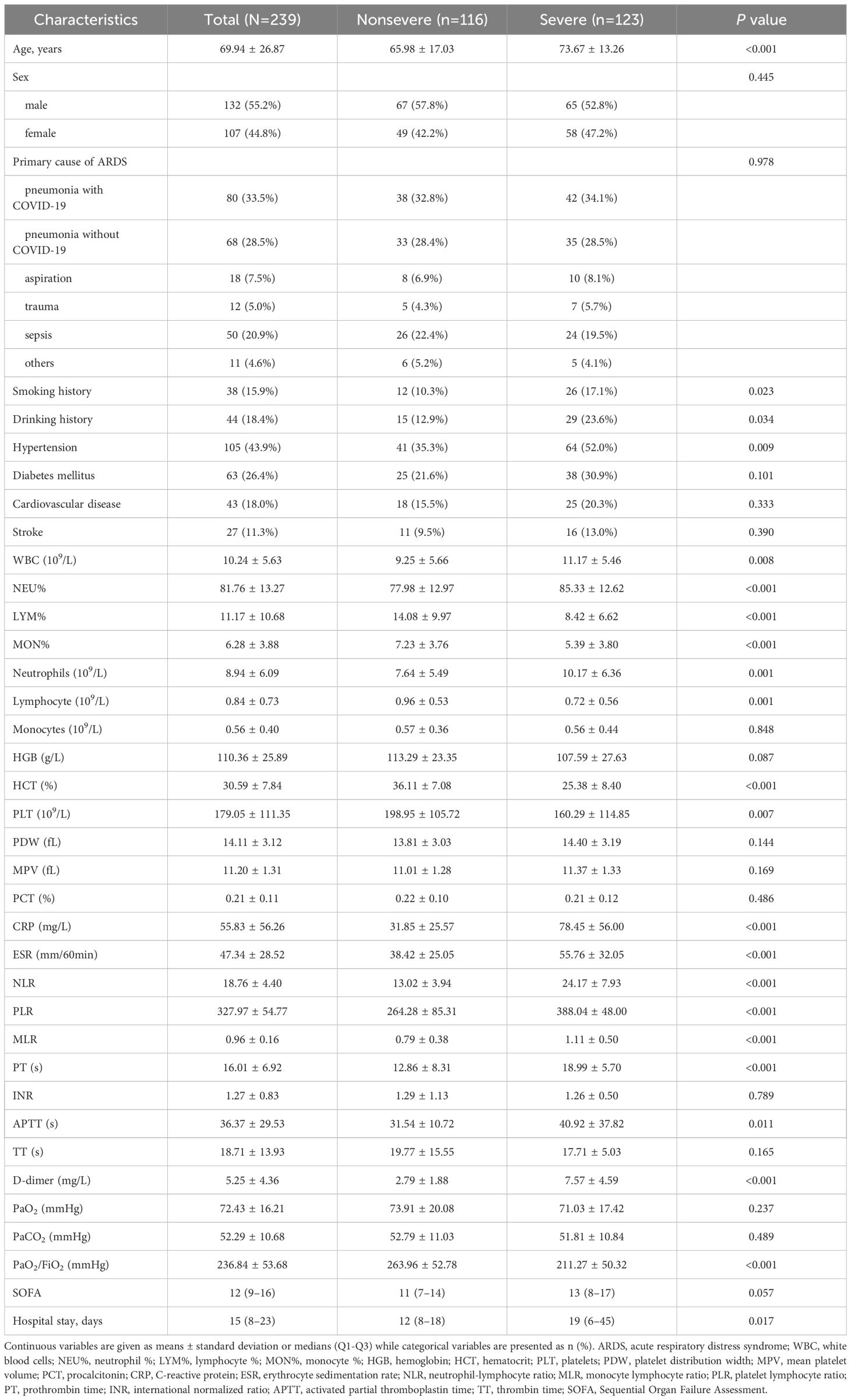
Table 1 ARDS patient clinical and demographic characteristics classified according to disease severity.
Laboratory findings from patients grouped according to disease severity are shown in Table 1. Routine blood analyses revealed that patients with severe ARDS presented with higher WBCs (P = 0.008) and neutrophil counts (P = 0.001) together with reduced lymphocyte counts as compared to nonsevere patients (P = 0.001). HCT, lymphocyte %, monocyte %, neutrophil %, NLR, PLR, and MLR values also differed significantly among groups (all P values < 0.001). With respect to platelet and coagulation indices, individuals with severe disease presented with higher APTT, PT, and D-dimer levels (all P values < 0.05). CRP and ESR levels were also significantly elevated in individuals suffering from severe disease as compared to those with nonsevere disease (all P-values < 0.001). The PaO2/FiO2 ratio was also significantly reduced among severe patients (P < 0.001), while SOFA scores exhibited the opposite trend to those for the PaO2/FiO2 ratio.
All patients with ARDS completed the follow-up period through March 31, 2023, and were included in these analyses. Over the course of this follow-up interval, 64 (26.8%) patients died and 175 (73.2%) patients recovered. Similar differences in clinical and demographic characteristics were observed between patients in the severe ARDS group that died and recovered (Table 2). Relative to patients in the severe group that progressed, through who died exhibited several worse laboratory parameters including higher WBCs, neutrophil %, NLR, PLR, MLR, D-dimer, CRP, and SOFA score values together with decreases in lymphocyte %, monocyte %, and PaO2/FiO2 ratio (all P-values < 0.05).
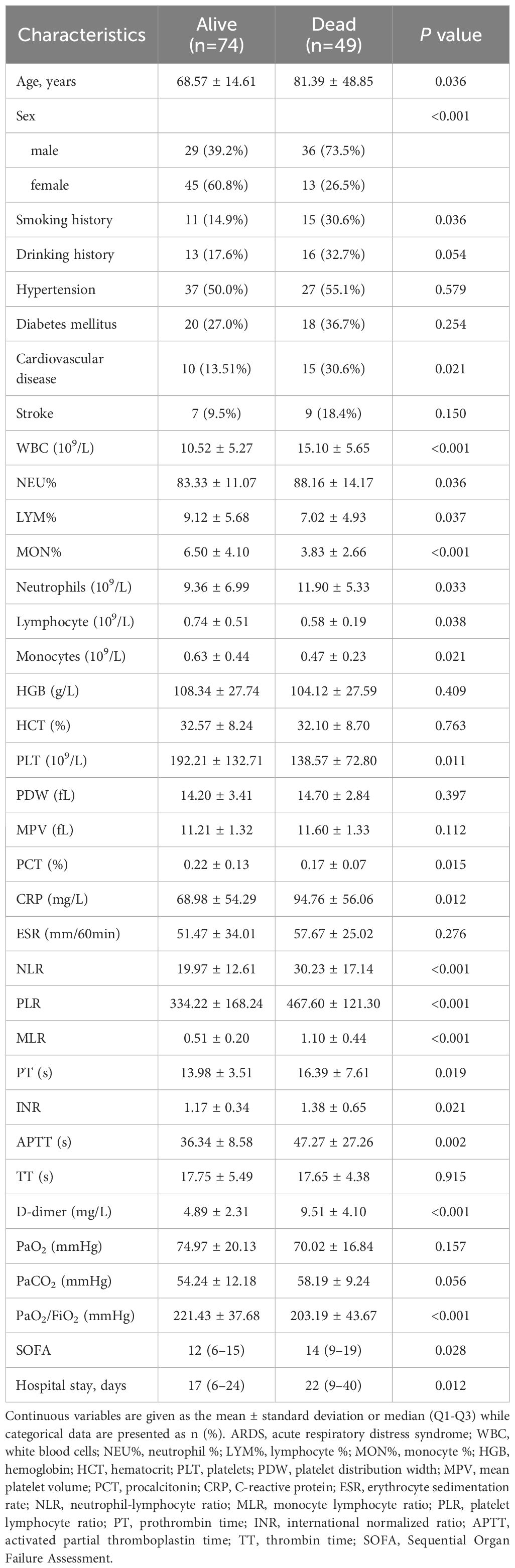
Table 2 Demographic and clinical characteristics of ARDS patients with severe disease classified according to survival status.
3.2 Patients with severe ARDS exhibit increased TM9SF1 expression
Differential TM9SF1 expression was assessed in ARDS patients and HCs (Figure 1). Blood samples from 61 ARDS patients were obtained during both periods of severe and stable remission, and samples were collected from 52 HCs at the same time. Significant increases in TM9SF1 mRNA levels were detected among patients with severe ARDS relative to individuals with nonsevere disease (0.21 ± 0.03 vs. 0.08 ± 0.02, P < 0.001). When TM9SF1 expression levels were analyzed in individuals during both severe disease and stable remission (61 pairs), TM9SF1 expression was found to be significantly reduced with disease resolution (0.28 ± 0.04 vs. 0.10 ± 0.02, P < 0.001) (Figure 1B). There were also significantly higher TM9SF1 expression levels in ARDS patients relative to HCs (0.15 ± 0.02 vs. 0.06 ± 0.01, P = 0.003) (Figure 1C). Independent-sample t-tests revealed that TM9SF1 expression levels were also significantly higher among deceased ARDS patients as compared to surviving patients (0.29 ± 0.06 vs. 0.10 ± 0.01, P < 0.001) (Figure 1D).
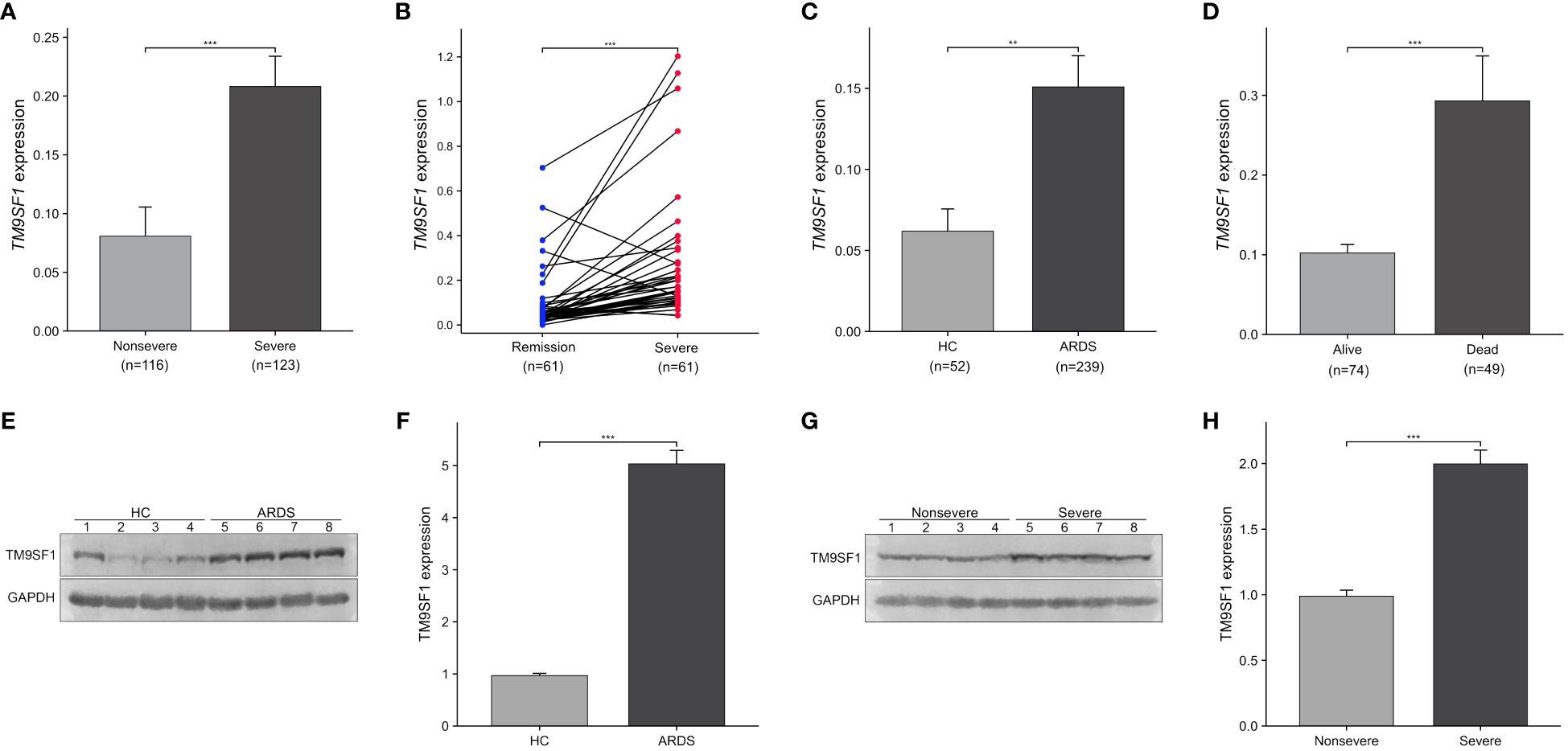
Figure 1 Severe ARDS patients exhibit elevated TM9SF1 expression. (A) Patients with severe and nonsevere ARDS. (B) Paired samples from patients with ARDS, in the remission and severe groups. (C) Patients with ARDS and HCs. (D) Patients with severe ARDS that did or did not survive. (E, F) Comparison of TM9SF1 protein expression in PBMCs from patients with ARDS and healthy controls, assessed by western blotting followed by semi-quantification of band intensities using Image J software with GAPDH as the loading control. (G, H) TM9SF1 protein expression in PBMCs from patients with ARDS on transition from severe to non-severe disease, evaluated by western blotting and semi-quantification of band intensities using Image J software with GAPDH as the loading control. ARDS, acute respiratory distress syndrome; HC, health control. **P < 0.01; ***P < 0.001.
In addition, we performed Western blotting to verify the TM9SF1 protein levels in PBMCs from 4 patients with ARDS and 4 healthy controls. It was found that the protein levels of TM9SF1 were upregulated 5.19-fold in patients with ARDS compared to healthy controls (Figures 1E, F). Additionally, TM9SF1 protein levels in PBMCs from patients with severe ARDS were also upregulated 2.02-fold compared to those from patients with non-severe ARDS (Figures 1G, H).
3.3 TM9SF1 expression is significantly correlated with cytokines in ARDS patients
The release of excessively high cytokine levels is a hallmark of ARDS that can result in severe lung damage (17). To probe the relationship between TM9SF1 mRNA levels and ARDS patient cytokine levels, correlation analyses were next conducted (Figure 2). This approach revealed that TM9SF1 mRNA levels were significantly positively correlated with interferon (IFN)-γ (r = 0.577, P < 0.001) and tumor necrosis factor (TNF)-α (r = 0.857, P < 0.001) levels, with the same also being true for interleukin (IL)-6 (r = 0.664, P < 0.001), IL-17A (r = 0.844, P < 0.001), and the transcription factor forkhead box P3 (FOXP3) (r = 0.731, P < 0.001). When these same correlation analyses were only conducted in patients with severe ARDS, these correlations remained intact, with particularly strong correlations between the expression of TM9SF1 and IFN-γ (r = 0.821, P < 0.001), TNF-α (r = 0.640, P < 0.001), and IL-17A (r = 0.613, P < 0.001). These data support a relationship between TM9SF1 expression and plasma cytokines in ARDS patients, identifying this gene as a potential regulator of ARDS pathogenesis. The cytokine levels during remission and severe disease in patients with ARDS are presented in Supplementary Figure S1.
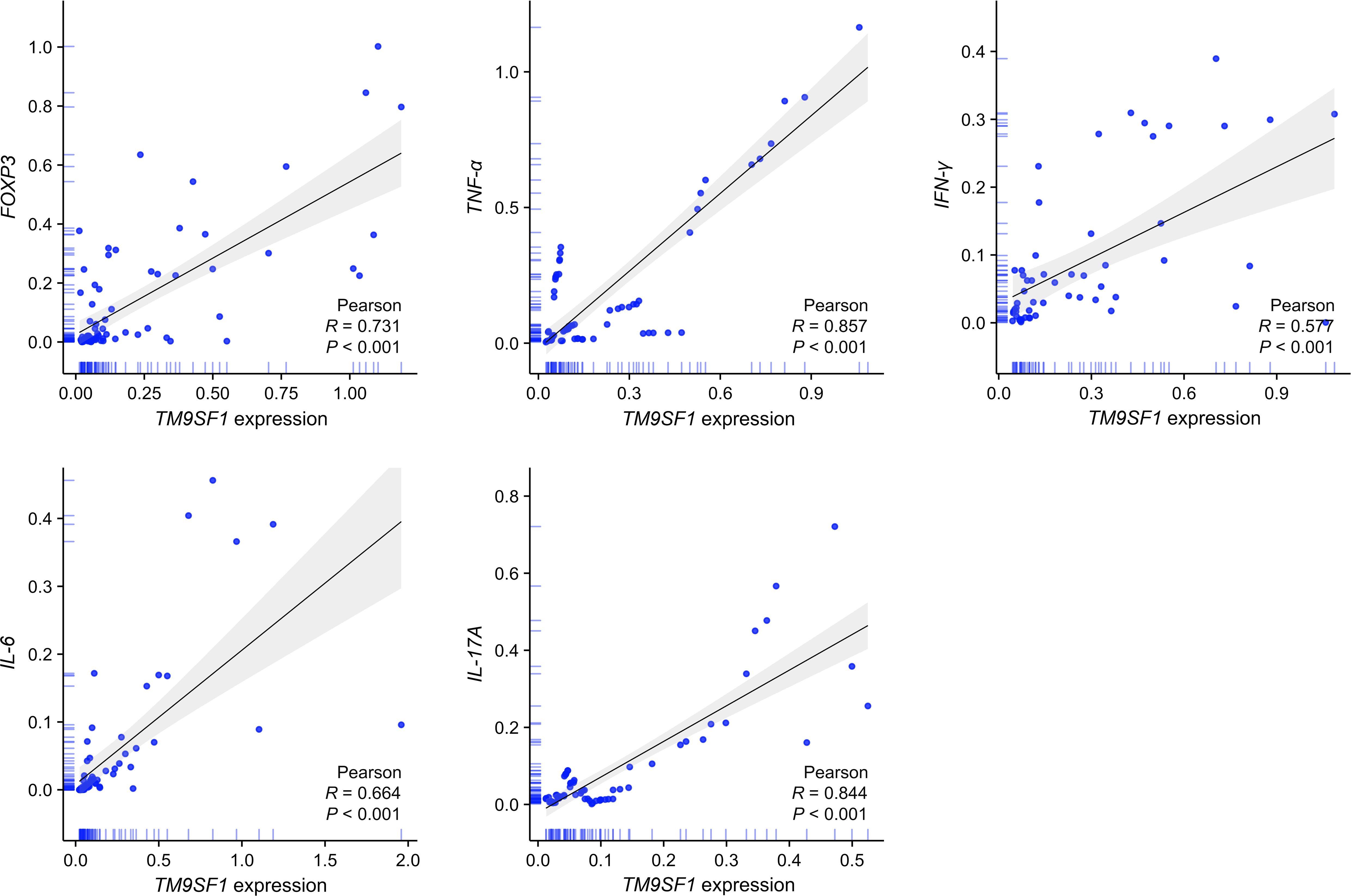
Figure 2 TM9SF1 expression is significantly correlated with cytokine levels in ARDS patients. ARDS, acute respiratory distress syndrome; FOXP3, forkhead box P3; TNF-α, tumor necrosis factor-α; IFN-γ, interferon-γ; IL-6, interleukin-6; IL-17A, interleukin-17A. The shadows represent the 95% confidence intervals of the fitted line.
3.4 High levels of TM9SF1 expression may contribute to increased ARDS severity and mortality
The link between the expression of TM9SF1 and ARDS severity was next probed through univariate and multivariate analyses (Supplementary Table S2). When these analyses were adjusted for patient demographic characteristics including age, sex, smoking status, drinking status, and history of disease, ARDS severity rose with increases in TM9SF1 expression (OR = 2.43, 95% CI = 2.15–3.72, P = 0.005). When median TM9SF1 expression levels were used to stratify patients into low- and high-risk groups, high-risk patients presented with a 3.03-fold increase in ARDS severity as compared to low-risk patients (OR = 4.03, 95% CI = 3.75–6.36, P < 0.001).
Cox regression analyses were further used to probe the link between the expression of TM9SF1 and patient mortality (Supplementary Table S3). When these analyses were adjusted for demographic characteristics including age, sex, smoking status, drinking status, and history of disease, ARDS patient mortality risk rose as TM9SF1 levels increased (HR = 2.27, 95% CI = 2.20–4.39, P = 0.001). Relative to patients with lower levels of TM9SF1 expression, the survival time of patients expressing higher levels of this gene was reduced (HR = 3.10, 95% CI = 2.03–4.32, P = 0.008).
3.5 TM9SF1 can predict ARDS severity and mortality more effectively than other clinical indicators
ROC curve analyses were used to gauge the relative performance of TM9SF1 and a range of other clinical indicators (WBCs, lymphocytes, lymphocyte %, monocytes, monocyte %, neutrophils, neutrophil %, NLR, MLR, PLR, CRP, D-dimer, PaO2/FiO2 and SOFA) when predicting ARDS severity (Table 3). Of the analyzed variables, TM9SF1 exhibited the highest area under the ROC curve (AUC) when predicting disease severity (0.871, 95% CI = 0.785–0.924). The corresponding AUC values for MLR and D-dimer when predicting ARDS severity were 0.798 (95% CI = 0.645–0.847) and 0.811 (95% CI = 0.764–0.883). These results thus suggested that TM9SF1 was the most efficacious marker for use when distinguishing between ARDS patients with severe and nonsevere disease. When using a TM9SF1 expression cut-off value of 0.07, the respective sensitivity and specificity values were 87.3% and 84.7%.
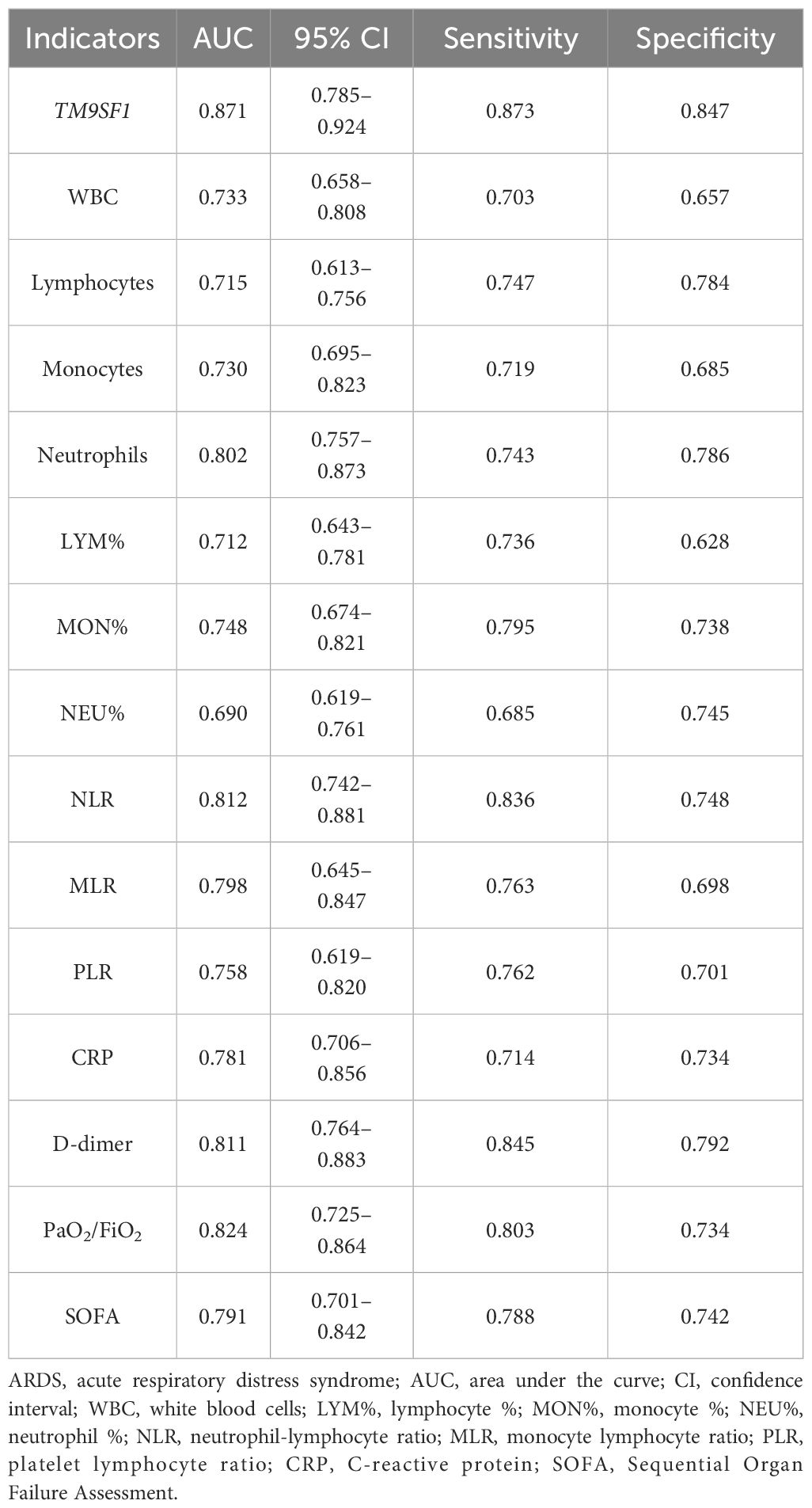
Table 3 The predictive performance of TM9SF1 and a range of other clinical indicators when predicting ARDS severity.
Time-dependent ROC analyses were further used to probe the performance of TM9SF1 and these other variables when predicting patient mortality (Table 4). In these analyses, TM9SF1 levels again exhibited superior predictive utility with a C-index of 0.846 (95% CI = 0.752–0.917) for patient mortality, with corresponding sensitivity and specificity values of 87.5% and 82.6% at a cut-off value of 0.15. The respective C-index values for CRP, PaO2/FiO2, and SOFA scores when predicting ARDS patient mortality were 0.783 (95% CI = 0.646–0.905), 0.816 (95% CI = 0.719–0.853), and 0.797 (95% CI = 0.711–0.852). These results demonstrate that TM9SF1 exhibits predictive performance better than that of a wide range of other clinical indicators when assessing ARDS patient disease severity and mortality risk. As such, TM9SF1 may offer value as a novel biomarker to predict ARDS severity and patient survival.
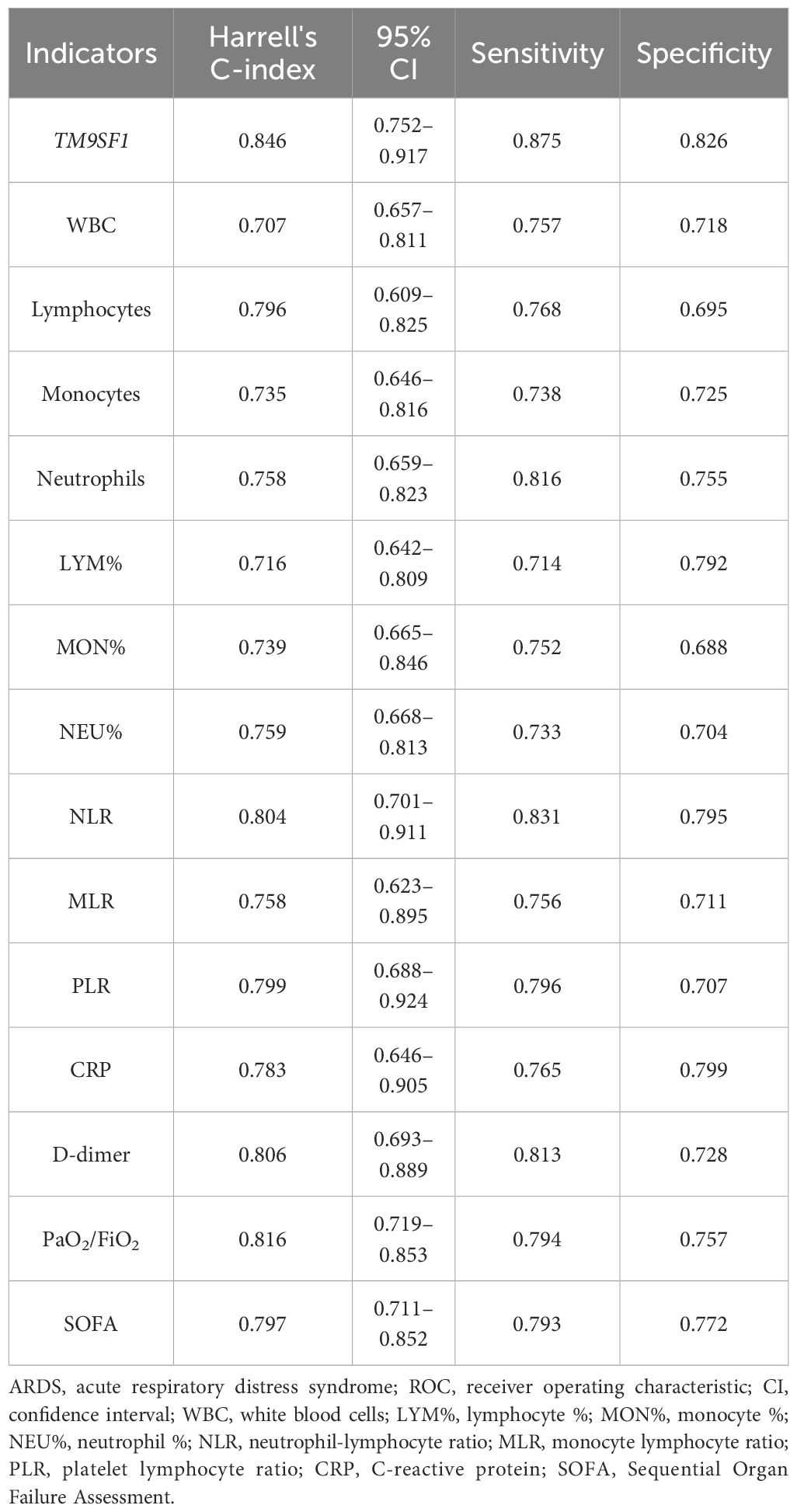
Table 4 The predictive performance of TM9SF1 and a range of other clinical indicators when assessing ARDS patient mortality through a time-dependent ROC analysis.
3.6 Nomogram development and validation
Multivariate logistic regression analyses incorporating all results that were significantly associated with ARDS severity in univariate analyses (P < 0.05) were next conducted to develop a predictive model. Those variables that were significant in this model following adjustment for covariates (P < 0.05) were considered independently associated with ARDS severity, and included TM9SF1 levels, age, D-dimer, and CRP levels, with respective ORs of 2.31 (95% CI = 1.12–4.67, P = 0.017), 1.07 (95% CI = 1.02–1.11, P = 0.024), 1.48 (95% CI = 1.31–2.05, P = 0.018), and 1.02 (95% CI = 1.01–1.05, P = 0.035).
Multivariable Cox proportional hazards regression analyses similarly identified elevated TM9SF1 expression (HR = 2.47, 95% CI = 2.18–5.49, P = 0.011), older age (HR = 1.36, 95% CI 1.22–4.19, P = 0.038), elevated NLR levels (HR = 1.81, 95% CI 1.01–3.25, P = 0.048), and elevated D-dimer levels (HR = 1.51, 95% CI = 1.08–2.12, P = 0.017) as being independently associated with the risk of mortality among patients with severe ARDS.
A nomogram for use in predicting severe ARDS development was established incorporating TM9SF1 levels, age, CRP levels, and D-dimer levels based on the above multivariate analysis results (Figure 3). The odds of developing severe ARDS were computed for individual patients by summing the scores for each of these individual risk factors. Similarly, a predictive nomogram for severe ARDS patient mortality risk was developed incorporating TM9SF1 expression, age, NLR, and D-dimer levels (Figure 4).
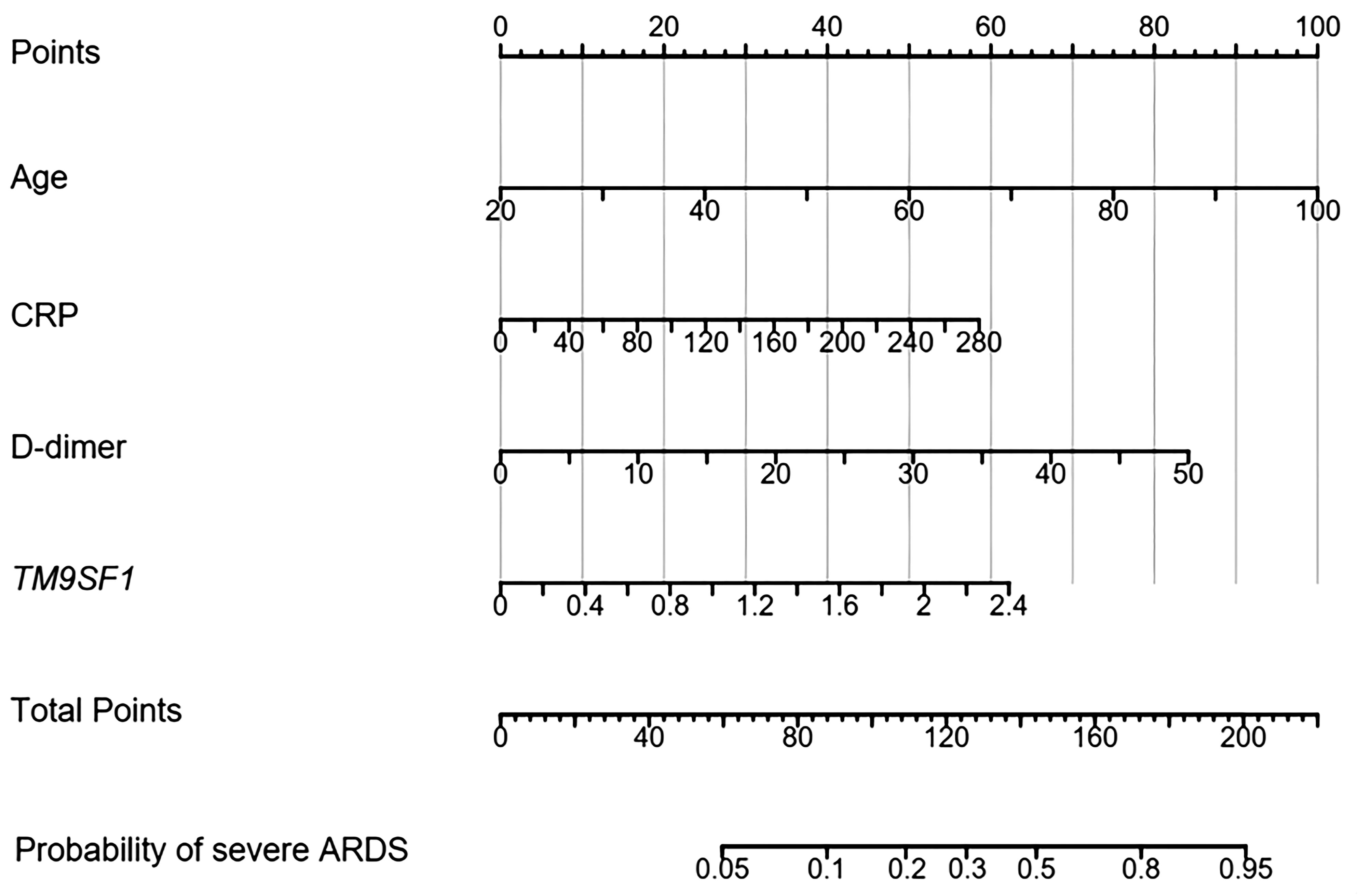
Figure 3 Nomogram developed to predict ARDS severity. ARDS, acute respiratory distress syndrome; CRP, C-reactive protein. Points: the individual scores corresponding to each predictor in the model in different groups/values. Total Points: the total score of each score corresponding to the values of all predictors. Probability: the predicted probability of event occurrence at the corresponding total point value.
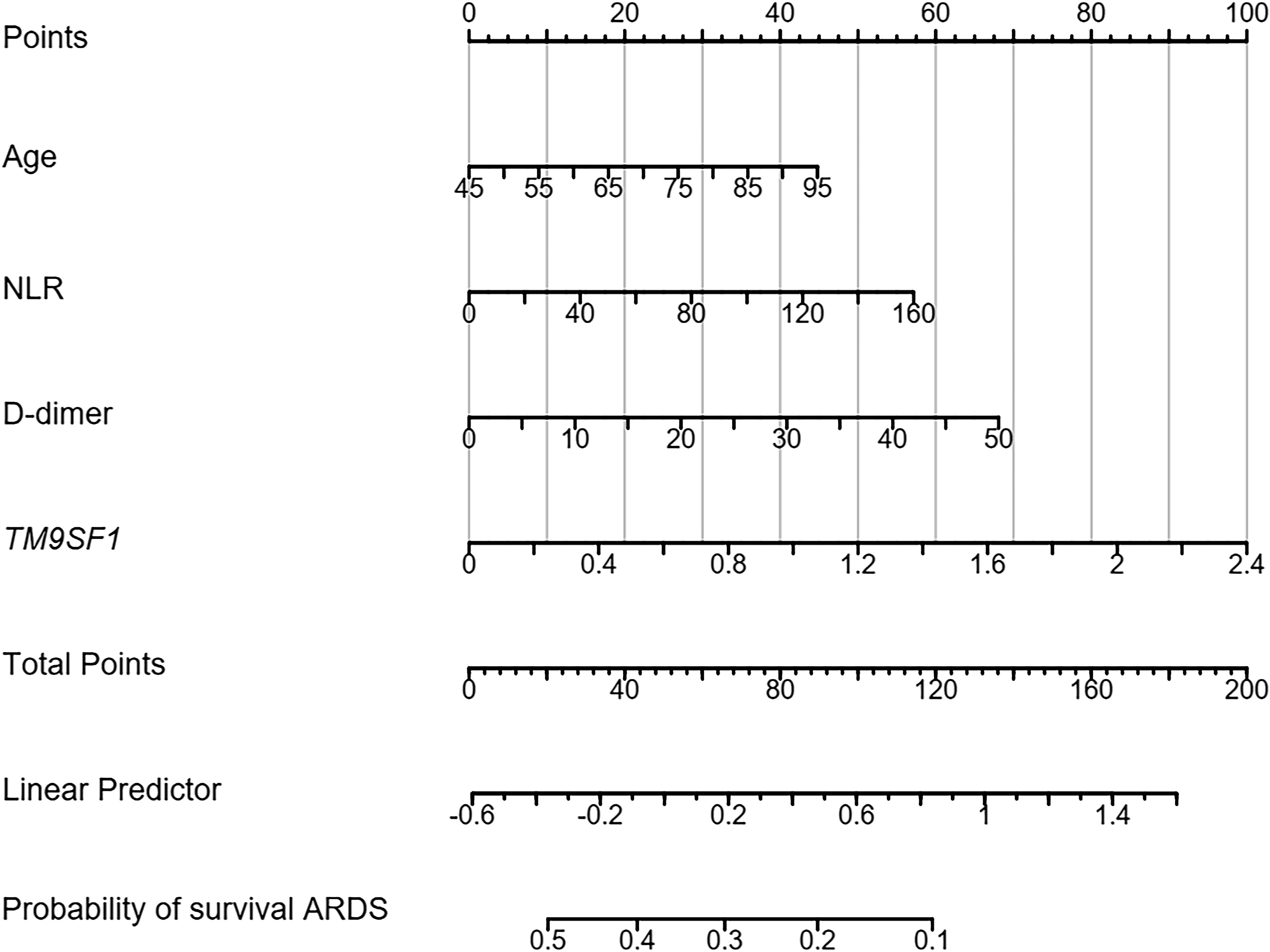
Figure 4 Nomogram developed to predict ARDS patient mortality risk. ARDS, acute respiratory distress syndrome; CRP, C-reactive protein. Points: the individual scores corresponding to each predictor in the model in different groups/values. Total Points: the total score of each score corresponding to the values of all predictors. Probability: the predicted probability of event occurrence at the corresponding total point value.
ROC curves were used to validate the nomogram designed to predict ARDS severity, yielding an AUC of 0.887 (95% CI = 0.715–0.943), a sensitivity of 87.9%, and a specificity of 86.8%. The validation of the nomogram used when predicting severe ARDS patient mortality yielded a C-index of 0.890 (95% CI = 0.627–0.957). Patients were separated into low- and high-risk groups based on total points to evaluate prognostic outcomes, revealing an optimal risk stratification value of 112.4 points.
4 Discussion
This prospective cohort study explored the utility of TM9SF1 as an early predictor of ARDS severity and patient survival outcomes. Severe ARDS patients presented with significantly higher TM9SF1 expression as compared to those with nonsevere disease and HCs. Significantly higher TM9SF1 mRNA levels were also detected in patients who experienced fatal ARDS as compared to those who did not. Multivariate logistic regression approaches indicated that increases in TM9SF1 levels were associated with greater disease severity, and higher TM9SF1 expression was also associated with greater patient mortality risk in Cox regression analysis. ROC curves revealed that the predictive performance of TM9SF1 was superior to that of many other clinical indicators associated with ARDS severity and patient mortality. Predictive models incorporating TM9SF1 expression levels were also established to assess ARDS severity and mortality risk, and these models exhibited satisfactory predictive performance. Preliminary investigation focused on the biological link between TM9SF1 and immune regulation in ARDS patients further revealed that TM9SF1 mRNA levels were strongly correlated with plasma cytokines. These findings suggest that TM9SF1 may offer utility as a novel biomarker for the personalized assessment of ARDS patient disease status and prognosis. In addition, the findings of the study provide a scientific basis for the individualized treatment of ARDS.
Many ARDS-related biomarkers and clinical parameters have been described to date (18–22). For example, Medford et al. explored polymorphisms in the VEGF gene and determined that patients with the T allele for a VEGF polymorphism exhibited greater ARDS incidence (18). Tejera et al. similarly noted that the rs324420-FAAH polymorphism was strongly correlated with ARDS (19). Many studies have also revealed that NLR, PLR, and/or MLR values can offer excellent utility as a predictor of ARDS clinical course and the risk of mortality among ARDS patients (23, 24). In a separate multicenter study, risk factors associated with ARDS severity were found to include age, sex, PaO2/FiO2 ratio, and SOFA scores (25). While these studies probed the predictive performance of individual biomarkers when assessing ARDS severity and/or mortality risks, none constructed multi-factor predictive models or evaluated both clinical and epigenetic biomarkers in order to simultaneously consider the effects of these various factors in a comprehensive manner. Here, TM9SF1 levels were found to outperform a range of clinical indicators with respect to their ability to predict ARDS severity and affected patient mortality. When predictive models incorporating TM9SF1 were established, they exhibited satisfactory predictive performance for ARDS severity and patient prognostic outcomes, outperforming any predictive models reported in these prior studies.
TM9SF family proteins are highly conserved across species, and consist of 9 putative transmembrane domains together with a large non-cytoplasmic domain (26, 27). In mammals, four members of this protein family (TM9SF1–4) have thus far been identified (26), with a limited number of prior reports having documented the relationships between these proteins and certain cancers (28–31). TM9SF1 is ubiquitously expressed at the protein level in many human tissues, and it shares 27–31% sequence identity with its yeast counterpart, suggesting that it likely plays important intracellular functions (32). While there have been prior analyses focused on patterns of TM9SF1 expression (33, 34), its functional characteristics remain poorly understood. Work from our group recently unveiled an increase in TM9SF1 expression levels in the lungs of mice suffering from LPS-induced acute lung injury, while TM9SF1 knockdown in these animals was found to alleviate the pulmonary inflammation induced by LPS through the enhancement of autophagic activity (12).
Elevated levels of many cytokines such as IL-6, IL-17A, TNF-α, and IFN-γ and the onset, severity, and prognosis of ARDS have long been documented (17). Elevated IL-6 expression has been observed among patients at risk of ARDS or with established disease, with particularly high levels among non-survivors (35–38). The IL-17 cytokine family consists of six proteins (IL-17A/B/C/D/E/F), and IL-17A levels have previously been shown to be positively correlated with various measures of lung function, inflammation, and disease severity in ARDS patients (39, 40). The production of TNF-α is central to acute inflammatory processes and it is enhanced in ARDS patients, individuals with severe COVID-19, and individuals with other forms of severe pneumonia (35, 36, 38). As the only type II IFN protein, IFN-γ serves as a central regulator of innate and adaptive immune function, although its relationship with ARDS has only recently been explored. For example, one recent study included IFN-γ in a panel of plasma biomarkers used to define a “reactive” disease subtype associated with higher rates of mortality in the ICU (41). Analyses of ARDS cases associated with SARS-CoV-2, bacterial pneumonia, human adenovirus (HAdV), and IAV/H1N1 have also documented significant increases in levels of IFN-γ and multiple other cytokines as compared to HCs (42). Notably, more pronounced increases in IFN-γ levels were observed in patients with HAdV infection who developed ARDS relative to patients who did not develop this complication, and a negative correlation was documented between the levels of this cytokine and the PaO2/FiO2 ratio (42). FOXP3 is an important transcription factor that controls the functions of regulatory T cells (Tregs) (43). Some reports have suggested that ARDS patients exhibit increases in Treg percentages of FOXP3 expression (43), whereas FOXP3 levels reportedly return to normal levels in convalescent or recovering patients (44). In another recent report, increases in CD25+ CD127+ FOXP3+ Tregs were observed together with the enhancement of the suppressive activity of these cells in individuals with severe COVID-19 (45). Bronchoalveolar lavage fluid samples from COVID-19 patients with ARDs have been shown to exhibit increases in FOXP3+Tregs and Th17 cells together with overall reductions in T cell numbers (46). Our group previously demonstrated that LPS-induced lung injury was associated with TM9SF1 upregulation at the mRNA and protein levels, while knocking down its expression was sufficient to protect against lung inflammation and to inhibit the production of cytokines including sTNF-α. In the present clinical study, a positive correlation was detected between TM9SF1 expression and levels of cytokines including IL-6, IL-17A, TNF-α, and IFN-γ as well as increases in FOXP3 levels, offering further support for the link between elevated TM9SF1 levels and greater ARDS severity. However, the precise mechanisms that link TM9SF1 and inflammatory immune regulation in patients with ARDS remain uncertain and warrant future investigation.
Here, TM9SF1 expression levels in PBMCs from ARDS patients were found, for the first time, to be significantly elevated. This, coupled with the observed positive correlation between TM9SF1 expression and ARDS severity, offered further support for the involvement of TM9SF1 in the incidence of pulmonary inflammation (12). We also performed Western blotting to verify the TM9SF1 protein levels in PBMCs. It was found that the protein levels of TM9SF1 were upregulated 5.19-fold in patients with ARDS compared to healthy controls. Additionally, TM9SF1 protein levels in PBMCs from patients with severe ARDS were also upregulated 2.02-fold compared to those from patients with non-severe ARDS. TM9SF1 has also been linked to a range of other conditions, including certain tumors (47, 48), ruptured intracranial aneurysms (34), and varicose spermatic veins (49). First identified as a marker associated with autophagy (50), TM9SF1 has since been confirmed to play roles in autophagic activity and regulating inflammatory factors (12). The precise molecular mechanisms whereby TM9SF1 functions, however, remain poorly understood. Efforts to clarify the functions of TM9SF1 are further hampered by the complex structure of this protein with its nine transmembrane domains. As such, while our team previously documented a role for this protein as a regulator of autophagy that controls lung inflammation in a mouse model system (12), the precise mechanisms linking TM9SF1 to inflammatory activity await further characterization.
This study is the first to have explored the functions or documented the performance of TM9SF1 as an early predictor of disease severity and mortality in ARDS patients. The novel predictive models and associated nomograms incorporating TM9SF1 designed herein were able to effectively predict ARDS patient status and clinical outcomes. However, this study is subject to some limitations. For one, this study only enrolled patients from a single hospital. As the overall patient cohort size was large and these patients were from many cities throughout China, however, these results are likely to be more representative and robust. In the future, a multicenter study will be performed to validate the present conclusions. Secondly, the average PaO2/FiO2 levels in patients with severe ARDS were not that low. In terms of the criteria by which the severe group was defined, the high PaO2/FiO2 level was apparent mainly in patients without invasive mechanical ventilation. When these patients were treated with analgesic sedation and high PEEP and were mechanically ventilated, the detected PaO2/FiO2 levels were higher than those in patients with invasive mechanical ventilation. As a result, the overall average PaO2/FiO2 levels in patients with severe ARDS were slightly higher. In addition, further research will be vital to clarify the immunological mechanisms whereby TM9SF1 exerts its effects on the pathogenesis of ARDS.
5 Conclusions
In summary, the present analyses revealed significantly increased TM9SF1 mRNA expression in patients with severe ARDS, and ARDS severity rose with increasing TM9SF1 expression. In addition, high TM9SF1 levels were associated with a greater risk of mortality among those ARDS patients with severe disease. TM9SF1 outperformed a range of other clinical indicators with respect to its ability to predict ARDS patient mortality and disease severity, and predictive models incorporating TM9SF1 were ultimately developed and found to exhibit satisfactory performance as a means of assessing ARDS severity and patient mortality risk. Based on these findings, TM9SF1 can serve as a novel biomarker for the individualized assessment of ARDS severity and patient prognosis in individuals suffering from ARDS.
Data availability statement
The raw data supporting the conclusions of this article will be made available by the authors, without undue reservation.
Ethics statement
The studies involving humans were approved by the Ethics Committee of Hubei University of Arts and Science. The studies were conducted in accordance with the local legislation and institutional requirements. The participants provided their written informed consent to participate in this study.
Author contributions
FC: Data curation, Investigation, Methodology, Writing – original draft. LZ: Conceptualization, Data curation, Formal Analysis, Methodology, Writing – original draft. ZZ: Data curation, Investigation, Methodology, Validation, Writing – review & editing. XS: Data curation, Formal Analysis, Investigation, Validation, Writing – review & editing. JiX: Data curation, Investigation, Methodology, Resources, Writing – review & editing. ZY: Data curation, Investigation, Methodology, Resources, Writing – review & editing. BG: Data curation, Investigation, Methodology, Validation, Writing – review & editing. ML: Data curation, Investigation, Methodology, Software, Validation, Writing – review & editing. HC: Data curation, Investigation, Methodology, Validation, Writing – review & editing. HX: Conceptualization, Data curation, Investigation, Resources, Validation, Writing – review & editing. MH: Data curation, Investigation, Methodology, Visualization, Writing – review & editing. YL: Data curation, Formal Analysis, Investigation, Methodology, Writing – review & editing. GQ: Formal Analysis, Investigation, Methodology, Software, Writing – review & editing. KW: Data curation, Funding acquisition, Methodology, Resources, Software, Validation, Writing – review & editing. FZ: Data curation, Methodology, Resources, Software, Validation, Writing – review & editing. JuX: Investigation, Software, Validation, Visualization, Writing – review & editing, Funding acquisition.
Funding
The author(s) declare that financial support was received for the research, authorship, and/or publication of this article. This work was supported by the Key R&D Program of Hubei province (2022BCE014), the Hubei Provincial Natural Science Foundation of China (2022CFC032) and the Research Foundation for Teacher Cultivation of Hubei University of Arts and Science (2022pygpzk10).
Acknowledgments
The authors would like to thank all the reviewers who participated in the review and MJEditor (www.mjeditor.com) for its linguistic assistance during the preparation of this manuscript.
Conflict of interest
The authors declare that the research was conducted in the absence of any commercial or financial relationships that could be construed as a potential conflict of interest.
Publisher’s note
All claims expressed in this article are solely those of the authors and do not necessarily represent those of their affiliated organizations, or those of the publisher, the editors and the reviewers. Any product that may be evaluated in this article, or claim that may be made by its manufacturer, is not guaranteed or endorsed by the publisher.
Supplementary material
The Supplementary Material for this article can be found online at: https://www.frontiersin.org/articles/10.3389/fimmu.2024.1408406/full#supplementary-material
References
1. Bos LDJ, Ware LB. Acute respiratory distress syndrome: causes, pathophysiology, and phenotypes. Lancet. (2022) 400:1145–56. doi: 10.1016/S0140-6736(22)01485-4
2. Force ADT, Ranieri VM, Rubenfeld GD, Thompson BT, Ferguson ND, Caldwell E, et al. Acute respiratory distress syndrome: the Berlin Definition. JAMA. (2012) 307:2526–33. doi: 10.1001/jama.2012.5669
3. Guerin C, Papazian L, Reignier J, Ayzac L, Loundou A, Forel JM, et al. Effect of driving pressure on mortality in ARDS patients during lung protective mechanical ventilation in two randomized controlled trials. Crit Care. (2016) 20:384. doi: 10.1186/s13054-016-1556-2
4. Briel M, Meade M, Mercat A, Brower RG, Talmor D, Walter SD, et al. Higher vs lower positive end-expiratory pressure in patients with acute lung injury and acute respiratory distress syndrome: systematic review and meta-analysis. JAMA. (2010) 303:865–73. doi: 10.1001/jama.2010.218
5. Peek GJ, Mugford M, Tiruvoipati R, Wilson A, Allen E, Thalanany MM, et al. Efficacy and economic assessment of conventional ventilatory support versus extracorporeal membrane oxygenation for severe adult respiratory failure (CESAR): a multicentre randomised controlled trial. Lancet. (2009) 374:1351–63. doi: 10.1016/S0140-6736(09)61069-2
6. Mahmood I, Tawfeek Z, El-Menyar A, Zarour A, Afifi I, Kumar S, et al. Outcome of concurrent occult hemothorax and pneumothorax in trauma patients who required assisted ventilation. Emerg Med Int. (2015) 2015:859130. doi: 10.1155/2015/859130
7. de la Rica R, Borges M, Gonzalez-Freire M. COVID-19: in the eye of the cytokine storm. Front Immunol. (2020) 11:558898. doi: 10.3389/fimmu.2020.558898
8. Potey PM, Rossi AG, Lucas CD, Dorward DA. Neutrophils in the initiation and resolution of acute pulmonary inflammation: understanding biological function and therapeutic potential. J Pathol. (2019) 247:672–85. doi: 10.1002/path.5221
9. Blondonnet R, Constantin JM, Sapin V, Jabaudon M. A pathophysiologic approach to biomarkers in acute respiratory distress syndrome. Dis Markers. (2016) 2016:3501373. doi: 10.1155/2016/3501373
10. Meyer NJ, Gattinoni L, Calfee CS. Acute respiratory distress syndrome. Lancet. (2021) 398:622–37. doi: 10.1016/S0140-6736(21)00439-6
11. Matthay MA, Zemans RL, Zimmerman GA, Arabi YM, Beitler JR, Mercat A, et al. Acute respiratory distress syndrome. Nat Rev Dis Primers. (2019) 5:18. doi: 10.1038/s41572-019-0069-0
12. Xiao J, Shen X, Chen H, Ding L, Wang K, Zhai L, et al. TM9SF1 knockdown decreases inflammation by enhancing autophagy in a mouse model of acute lung injury. Heliyon. (2022) 8:e12092. doi: 10.1016/j.heliyon.2022.e12092
13. Tasaka S, Ohshimo S, Takeuchi M, Yasuda H, Ichikado K, Tsushima K, et al. ARDS clinical practice guideline 2021. J Intensive Care. (2022) 10:32. doi: 10.1186/s40560-022-00615-6
14. Vincent JL, de Mendonca A, Cantraine F, Moreno R, Takala J, Suter PM, et al. Use of the SOFA score to assess the incidence of organ dysfunction/failure in intensive care units: results of a multicenter, prospective study. Working group on "sepsis-related problems" of the European Society of Intensive Care Medicine. Crit Care Med. (1998) 26:1793–800. doi: 10.1097/00003246-199811000-00016
15. Livak KJ, Schmittgen TD. Analysis of relative gene expression data using real-time quantitative PCR and the 2(-Delta Delta C(T)) Method. Methods. (2001) 25:402–8. doi: 10.1006/meth.2001.1262
16. Xiao J, Liu W, Chen Y, Deng W. Recombinant human PDCD5 (rhPDCD5) protein is protective in a mouse model of multiple sclerosis. J Neuroinflamm. (2015) 12:117. doi: 10.1186/s12974-015-0338-0
17. Fadanni GP, Calixto JB. Recent progress and prospects for anti-cytokine therapy in preclinical and clinical acute lung injury. Cytokine Growth Factor Rev. (2023) 71–72:13–25. doi: 10.1016/j.cytogfr.2023.07.002
18. Medford AR, Ibrahim NB, Millar AB. Vascular endothelial growth factor receptor and coreceptor expression in human acute respiratory distress syndrome. J Crit Care. (2009) 24:236–42. doi: 10.1016/j.jcrc.2008.04.003
19. Tejera P, Meyer NJ, Chen F, Feng R, Zhao Y, O'Mahony DS, et al. Distinct and replicable genetic risk factors for acute respiratory distress syndrome of pulmonary or extrapulmonary origin. J Med Genet. (2012) 49:671–80. doi: 10.1136/jmedgenet-2012-100972
20. Sapru A, Liu KD, Wiemels J, Hansen H, Pawlikowska L, Poon A, et al. Association of common genetic variation in the protein C pathway genes with clinical outcomes in acute respiratory distress syndrome. Crit Care. (2016) 20:151. doi: 10.1186/s13054-016-1330-5
21. O'Mahony DS, Glavan BJ, Holden TD, Fong C, Black RA, Rona G, et al. Inflammation and immune-related candidate gene associations with acute lung injury susceptibility and severity: a validation study. PloS One. (2012) 7:e51104. doi: 10.1371/journal.pone.0051104
22. Liang Y, Yang N, Pan G, Jin B, Wang S, Ji W. Elevated IL-33 promotes expression of MMP2 and MMP9 via activating STAT3 in alveolar macrophages during LPS-induced acute lung injury. Cell Mol Biol Lett. (2018) 23:52. doi: 10.1186/s11658-018-0117-x
23. Ma A, Cheng J, Yang J, Dong M, Liao X, Kang Y. Neutrophil-to-lymphocyte ratio as a predictive biomarker for moderate-severe ARDS in severe COVID-19 patients. Crit Care. (2020) 24:288. doi: 10.1186/s13054-020-03007-0
24. Favre PA, de Molliens L, Petit L, Biais M, Carrie C. May the neutrophil-to-lymphocyte ratio at admission predict the occurrence and the severity of ARDS after blunt chest trauma patients? A retrospective study. Am J Emerg Med. (2021) 39:137–42. doi: 10.1016/j.ajem.2020.09.050
25. Cunha MCA, Schardong J, Righi NC, Lunardi AC, Sant'Anna GN, Isensee LP, et al. Aging-related predictive factors for oxygenation improvement and mortality in COVID-19 and acute respiratory distress syndrome (ARDS) patients exposed to prone position: A multicenter cohort study. Clinics (Sao Paulo). (2023) 78:100180. doi: 10.1016/j.clinsp.2023.100180
26. Pruvot B, Laurens V, Salvadori F, Solary E, Pichon L, Chluba J. Comparative analysis of nonaspanin protein sequences and expression studies in zebrafish. Immunogenetics. (2010) 62:681–99. doi: 10.1007/s00251-010-0472-x
27. Froquet R, Cherix N, Birke R, Benghezal M, Cameroni E, Letourneur F, et al. Control of cellular physiology by TM9 proteins in yeast and Dictyostelium. J Biol Chem. (2008) 283:6764–72. doi: 10.1074/jbc.M704484200
28. Clark CR, Maile M, Blaney P, Hellweg SR, Strauss A, Durose W, et al. Transposon mutagenesis screen in mice identifies TM9SF2 as a novel colorectal cancer oncogene. Sci Rep. (2018) 8:15327. doi: 10.1038/s41598-018-33527-3
29. Oo HZ, Sentani K, Sakamoto N, Anami K, Naito Y, Oshima T, et al. Identification of novel transmembrane proteins in scirrhous-type gastric cancer by the Escherichia coli ampicillin secretion trap (CAST) method: TM9SF3 participates in tumor invasion and serves as a prognostic factor. Pathobiology. (2014) 81:138–48. doi: 10.1159/000357821
30. Shen L, Du X, Ma H, Mei S. miR-1193 suppresses the proliferation and invasion of human T-cell leukemia cells through directly targeting the transmembrane 9 superfamily 3 (TM9SF3). Oncol Res. (2017) 25:1643–51. doi: 10.3727/096504017X14908284471361
31. Paolillo R, Spinello I, Quaranta MT, Pasquini L, Pelosi E, Lo Coco F, et al. Human TM9SF4 is a new gene down-regulated by hypoxia and involved in cell adhesion of leukemic cells. PloS One. (2015) 10:e0126968. doi: 10.1371/journal.pone.0126968
32. Chluba-de Tapia J, de Tapia M, Jaggin V, Eberle AN. Cloning of a human multispanning membrane protein cDNA: evidence for a new protein family. Gene. (1997) 197:195–204. doi: 10.1016/S0378-1119(97)00263-1
33. Schlegel J, Neff F, Piontek G. Serial induction of mutations by ethylnitrosourea in PC12 cells: a new model for a phenotypical characterization of the neurotoxic response to 6-hydroxydopamine. J Neurosci Methods. (2004) 137:215–20. doi: 10.1016/j.jneumeth.2004.02.010
34. Wang C, Qu B, Wang Z, Ju J, Wang Y, Wang Z, et al. Proteomic identification of differentially expressed proteins in vascular wall of patients with ruptured intracranial aneurysms. Atherosclerosis. (2015) 238:201–6. doi: 10.1016/j.atherosclerosis.2014.11.027
35. Meduri GU, Headley S, Kohler G, Stentz F, Tolley E, Umberger R, et al. Persistent elevation of inflammatory cytokines predicts a poor outcome in ARDS. Plasma IL-1 beta and IL-6 levels are consistent and efficient predictors of outcome over time. Chest. (1995) 107:1062–73. doi: 10.1378/chest.107.4.1062
36. Meduri GU, Kohler G, Headley S, Tolley E, Stentz F, Postlethwaite A. Inflammatory cytokines in the BAL of patients with ARDS. Persistent elevation over time predicts poor outcome. Chest. (1995) 108:1303–14. doi: 10.1378/chest.108.5.1303
37. Park WY, Goodman RB, Steinberg KP, Ruzinski JT, Radella F 2nd, Park DR, et al. Cytokine balance in the lungs of patients with acute respiratory distress syndrome. Am J Respir Crit Care Med. (2001) 164:1896–903. doi: 10.1164/ajrccm.164.10.2104013
38. Wang J, Yang X, Li Y, Huang JA, Jiang J, Su N. Specific cytokines in the inflammatory cytokine storm of patients with COVID-19-associated acute respiratory distress syndrome and extrapulmonary multiple-organ dysfunction. Virol J. (2021) 18:117. doi: 10.1186/s12985-021-01588-y
39. Li Q, Gu Y, Tu Q, Wang K, Gu X, Ren T. Blockade of interleukin-17 restrains the development of acute lung injury. Scand J Immunol. (2016) 83:203–11. doi: 10.1111/sji.12408
40. Mikacenic C, Hansen EE, Radella F, Gharib SA, Stapleton RD, Wurfel MM. Interleukin-17A is associated with alveolar inflammation and poor outcomes in acute respiratory distress syndrome. Crit Care Med. (2016) 44:496–502. doi: 10.1097/CCM.0000000000001409
41. Bos LD, Schouten LR, van Vught LA, Wiewel MA, Ong DSY, Cremer O, et al. Identification and validation of distinct biological phenotypes in patients with acute respiratory distress syndrome by cluster analysis. Thorax. (2017) 72:876–83. doi: 10.1136/thoraxjnl-2016-209719
42. Li J, Wei J, Xu Z, Jiang C, Li M, Chen J, et al. Cytokine/Chemokine expression is closely associated disease severity of human adenovirus infections in immunocompetent adults and predicts disease progression. Front Immunol. (2021) 12:691879. doi: 10.3389/fimmu.2021.691879
43. Dhawan M, Rabaan AA, Alwarthan S, Alhajri M, Halwani MA, Alshengeti A, et al. Regulatory T cells (Tregs) and COVID-19: unveiling the mechanisms, and therapeutic potentialities with a special focus on long COVID. Vaccines (Basel). (2023) 11:699. doi: 10.3390/vaccines11030699
44. Samaan E, Elmaria MO, Khedr D, Gaber T, Elsayed AG, Shenouda RN, et al. Characterization of regulatory T cells in SARS-CoV-2 infected hemodialysis patients: relation to clinical and radiological severity. BMC Nephrol. (2022) 23:391. doi: 10.1186/s12882-022-03024-x
45. Vick SC, Frutoso M, Mair F, Konecny AJ, Greene E, Wolf CR, et al. A regulatory T cell signature distinguishes the immune landscape of COVID-19 patients from those with other respiratory infections. Sci Adv. (2021) 7:eabj0274. doi: 10.1126/sciadv.abj0274
46. Ronit A, Berg RMG, Bay JT, Haugaard AK, Ahlstrom MG, Burgdorf KS, et al. Compartmental immunophenotyping in COVID-19 ARDS: A case series. J Allergy Clin Immunol. (2021) 147:81–91. doi: 10.1016/j.jaci.2020.09.009
47. Hu YX, Zheng MJ, Zhang WC, Li X, Gou R, Nie X, et al. Systematic profiling of alternative splicing signature reveals prognostic predictor for cervical cancer. J Transl Med. (2019) 17:379. doi: 10.1186/s12967-019-02140-x
48. Zhuo W, Sun M, Wang K, Zhang L, Li K, Yi D, et al. m(6)Am methyltransferase PCIF1 is essential for aggressiveness of gastric cancer cells by inhibiting TM9SF1 mRNA translation. Cell Discovery. (2022) 8:48. doi: 10.1038/s41421-022-00395-1
49. Zhang C, Li X, Chen J, Zhao L, Wei X, Dong Y, et al. Transcriptome and genome sequencing investigating the molecular characteristics of patients with varicocele infertility. Andrologia. (2022) 54:e14542. doi: 10.1111/and.14542
Keywords: ARDS, TM9SF1, prediction model, severity, mortality
Citation: Cao F, Zhang L, Zhao Z, Shen X, Xiong J, Yang Z, Gong B, Liu M, Chen H, Xiao H, Huang M, Liu Y, Qiu G, Wang K, Zhou F and Xiao J (2024) TM9SF1 offers utility as an efficient predictor of clinical severity and mortality among acute respiratory distress syndrome patients. Front. Immunol. 15:1408406. doi: 10.3389/fimmu.2024.1408406
Received: 28 March 2024; Accepted: 20 May 2024;
Published: 03 June 2024.
Edited by:
Ramcés Falfán-Valencia, National Institute of Respiratory Diseases-Mexico (INER), MexicoReviewed by:
Chenming Zhang, Henan University of Chinese Medicine, ChinaIngrid Fricke-Galindo, Instituto Nacional de Enfermedades Respiratorias-México (INER), Mexico
Copyright © 2024 Cao, Zhang, Zhao, Shen, Xiong, Yang, Gong, Liu, Chen, Xiao, Huang, Liu, Qiu, Wang, Zhou and Xiao. This is an open-access article distributed under the terms of the Creative Commons Attribution License (CC BY). The use, distribution or reproduction in other forums is permitted, provided the original author(s) and the copyright owner(s) are credited and that the original publication in this journal is cited, in accordance with accepted academic practice. No use, distribution or reproduction is permitted which does not comply with these terms.
*Correspondence: Fengqiao Zhou, zhoufengqiao@hbuas.edu.cn; Ke Wang, wangk201912@hbuas.edu.cn; Juan Xiao, xiaoj@hbuas.edu.cn
†These authors have contributed equally to this work