- Laboratory for Maritime Transport, School of Naval Architecture and Marine Engineering, National Technical University of Athens, Athens, Greece
The potential leakage of oil trapped in the hull of fully or partially submerged shipwrecks has been a growing concern worldwide mainly due to major uncertainties therein and the great potential for environmental damage. The high cost of either preventing or reacting to potential oil discharges from shipwrecks into the environment mandates the use of risk analysis methods in order to identify the most dangerous cases and prioritize the allocation of resources. The purpose of this study is to present a probabilistic risk assessment method that will incorporate the various uncertainties into a structured and quantitative approach. One of the main factors affecting the release of oil from a shipwreck is the amount and progression of corrosion of the steel structure of the wreck, which is a dynamic process. The proposed model will take this into account by applying a time dependent approach that can be used to forecast how the risk rating, that is calculated for each case, changes as a function of time. The analysis will be concluded by applying a sensitivity analysis for the calibration of the model results. Geographically, this study will focus on Greece which presents a particularly interesting case due to its unique geomorphology and its rather developed maritime domain that is a direct consequence thereof. The great number of islands situated in Greece present an additional concern from a risk perspective because oil spill response operations are therefore constituted extremely difficult and costly. Another consideration, which is directly related to the high value of the various tourist activities, is the fact that even a small amount of spilled oil could be catastrophic for the local economies if the response is not fast enough. These issues indicate a growing need for the application of risk analysis tools that will aid in the decision making process relating to the optimum allocation of resources that are needed to efficiently address the problem as well as the prioritization of the most environmentally dangerous shipwrecks.
Introduction
Michel et al. (2005) have reported that there are approximately 8500 shipwrecks across the globe, containing estimated remaining oil quantities that range from 2.5 to as high as 20.4 million tons. This fact has been the starting point in recognizing and addressing shipwrecks as a significant source of oil pollution and consequently a major environmental threat. Another fact that has mobilized various administrative authorities is the confirmation that some of these shipwrecks have been chronically leaking oil, either at a small and steady rate or as sudden periodic discharges. The U.S. National Oceanic and Atmospheric Administration—NOAA (Helton, 2003) has investigated two such cases, i.e., the USS Mississinewa and the SS Jacob Luckenbach, which both sank during World War II (WWII) and have been submerged for over 60 years. Regarding the effects on both the environment and human health, sunken shipwrecks contain various pollutants, which include oil, asbestos and plastics (Kamm, 2011). These dangers are of particular concern, compared, for example, to accidental releases of oil from ships, because they may not be presented immediately but after many years following the incident. This fact affects both the immediate mobilization of competent authorities and public awareness.
This paper addresses the problem within Greek waters, which have been selected due to the unique geomorphology1 and the existence of a high value tourist industry. Consequently, oil spill response operations in Greece can be extremely difficult and hence costly, and the potential consequences to the local economies can be catastrophic. On this basis, the aim of this paper is to present a comprehensive risk assessment method based on the construction of a Dynamic Fault Tree (DFT). The specific objectives are to evaluate the probability of oil discharge from shipwrecks and the relevant consequence severity, as well as assess the performance of the developed model. The first section contains a literature review concerning major risk factors as well as existing methodologies for shipwreck risk assessment. The next section contains the theoretical basis for this paper and a detailed description of the developed model. The section documenting the results includes the outcome of the sensitivity analysis that was conducted as well as interesting insights from the application of the model to selected real-world cases. The paper will conclude with a discussion of key points and a number of interesting conclusions.
Shipwreck Risk Assessment
As reported in the work of Monfils (2005), the majority of existing shipwrecks (about 7800 documented cases) sank during WWII operations. Since most of these shipwrecks have been submerged for over 60 years, and therefore have been extensively corroded, it is becoming more likely that trapped oil will be discharged. Corrosion has been recognized as the main driver affecting potential oil discharges from shipwrecks and is a dynamic phenomenon that depends on various environmental factors (Macleod et al., 2004). The growing concern for the environmental impact of shipwrecks has resulted in various studies examining the effects of corrosion on modern day shipwrecks such as the USS Arizona (Johnson et al., 2006). Alcaro et al. (2007), in the context of the DEEPP Project (DEvelopment of European guidelines for Potentially Polluting shipwrecks), have conducted a comprehensive analysis of the factors affecting shipwreck corrosion and also presented relevant data from site surveys. Despite the existing research on shipwreck corrosion, the effect of depth and hydrostatic pressure remains unclear. Overfield (2005) has examined corrosion as a function of depth by monitoring a number of deep water WWII shipwrecks situated in the Gulf of Mexico and concluded that deep water shipwrecks were in a worse state compared to shallow water shipwrecks. This conclusion contradicted the prior assumption that shipwrecks in deep water are less exposed to various ocean elements and therefore better protected against corrosion.
Theoretical work on corrosion has also led to the development of various models for indirectly predicting corrosion rates on shipwrecks. The following are some notable examples from the relevant literature. Russell et al. (2006) developed a novel prediction method based on the physical and chemical properties of the concretion layer that builds up on the hull of the shipwreck. Hafiz (2010) used experimental data from 84 specimens to construct a Radial Basis Function Neural Network (RBFNN) model and validate the predictions. Johnson et al. (2011) proposed the use of the Weins number, which is a dimensionless ratio of calculated to estimated corrosion rate and is based on temperature, concretion thickness and oxygen concentration. Another interesting approach is the work by Foecke et al. (2010), who incorporated corrosion rate data into a Finite Elements Model (FEM) that was applied to the USS Arizona for predicting the future deterioration of the ship's structure.
A major problem with shipwreck risk assessment is the effects of uncertainties, mostly related to the scarcity of available information (especially for older shipwrecks), on the risk results. It is not uncommon for information regarding accident details (e.g., even the exact location of a particular shipwreck), the state of the shipwreck on the sea floor, or the amount of hazardous substances still trapped inside, to be unavailable. For example, the uncertainty on the exact amount of trapped oil has a significant impact on the decision to take remediation actions, considering that cleanup cost of oil spills can get extremely high per ton of oil (Etkin, 1999), which often results in indecisiveness and inaction. Another source of uncertainty is the one related to gaps in knowledge. For example, the insufficient knowledge of the way structural integrity is affected by either the dynamic (corrosion and fatigue) or static (damage to the hull by a change of position) processes at play. The need to effectively deal with these uncertainties highlights the importance of applying a structured risk assessment approach to this problem. In particular, the importance of shipwreck risk assessment lies in identifying the potential dangers, prioritizing the most hazardous shipwrecks and ultimately allocating resources either to monitor (shipwreck surveys etc.) or take appropriate remediation actions.
One of the earliest attempts at a comprehensive risk assessment for shipwrecks was made by Michel et al. (2005). This work included compiling a global shipwreck database, developing a method for estimating remaining oil quantities, assessing the technological feasibility of oil removal from shipwrecks, and proposing specific risk control options. Under the auspices of the NOAA, Symons (2013) has presented an extensive risk assessment for 87 potentially polluting shipwrecks along the U.S. coastline. They classified each case in clearly defined risk categories and prioritized them according to the necessary remediation actions. Another noteworthy attempt is the work conducted by Svensson (2010), which aimed at compiling a shipwreck database and ranking each case according to the estimated level of oil spill risk, as well as collecting a variety of data from on-site shipwreck surveys. Most of these methodologies have taken a qualitative approach to risk modeling, as pointed out by Landquist et al. (2013) in a detailed review of the risk assessment methods. However, research aiming at producing quantitative models has already surfaced, such as the work by Landquist et al. (2014), where a quantitative method (VRAKA) to estimate the risk of oil discharge from a shipwreck, was developed. VRAKA uses a fault tree to calculate the probability of oil discharge, on an annual basis, and expert opinion to estimate probabilities of basic events. As part of the work preceding this paper, a semi-quantitative Fuzzy Inference System (FIS) for assessing the danger of oil release from shipwrecks located in Greek national waters (Ventikos et al., 2013) has been constructed. This approach involved compiling a database of 402 shipwrecks in Greece, and applying spatial analysis through a specially developed GIS system. The existence of these independently developed attempts at shipwreck risk assessment, indicates that awareness of the problem is building up.
Methodology
The methodology applied in this paper involves the construction of a DFT model to calculate the probability of oil discharge and defined consequence indicators for assessing the severity of the consequences.
The DFT approach was selected because of its extended capability in dealing with events that are inter-dependent and in modeling complex systems by taking into account order dependent failures, i.e., infeasible failure sequences (Dugan et al., 1992). DFTs are also used for modeling time-dependent failures, which means that combined events must happen in a particular order for failure to occur (Distefano and Puliafito, 2007). The additional gates used in a DFT are called dynamic gates and are used for modeling the following complex system behaviors: redundancy (SPARE gate), common cause failures with the Functional Dependency (FDEP) gate, and order-dependent failures with the Sequential (SEQ) and Priority-AND (PAND) gates. A key difference between a classic fault tree and a DFT is the method of calculating the probability of the top event. Since the events connected with dynamic gates are not independent from each other, it is not possible to calculate the probability of the top event by using the same equations as for static gates. The most commonly used approach to solving a DFT is by converting it into a Continuous Time Markov Chains (CTMC) and solving the resulting differential equations numerically (Boudali et al., 2007). The main challenge with the application of the DFT approach lies in the unavailability of relevant historical data, which severely limits the ability to calculate frequencies or probabilities. This analysis deals with this issue by approximating the initial probabilities as a function of site and wreck specific data that are usually available (e.g., depth, location, time since the sinking of the ship, accident type and ship type) combined with estimations from expert opinion.
The severity of the potential consequences is evaluated on defined scales that are used to rank each shipwreck, according to information such as the estimated oil quantity in the shipwreck, the oil type, the distance and coast type of the nearest shore etc. Due to the quantitative nature of the probability calculation and the semi-quantitative nature of the consequence ranking, a risk matrix was employed to combine the two dimensions and calculate the final risk value (Table 1). The risk matrix used in this approach is similar to the one proposed by the International Maritime Organization (IMO) for conducting Formal Safety Assessments (FSAs) for the maritime industry.
As defined by the IMO (2013), the Risk Index (RI) is calculated as a function of the Frequency Index (FI) and the Severity Index (SI). The FI has seven (7) levels of probability (ranging from extremely remote to probable) and the SI four (4) levels of consequence severity (Minor, Significant, Severe, and Catastrophic). Since the values of the FI and the SI are measured on logarithmic scales, the RI is calculated by using the following formula:
To obtain the final risk values, the probabilities that were calculated from the DFT were assigned to specific levels of the FI. Similarly, the different consequence indicators were aggregated into a Consequence Index (CI) for each shipwreck, whose values were assigned to specific levels of the SI. The application of this method aimed at taking advantage of the defined levels of the FI and SI.
Description of the DFT Model
The DFT model was constructed in such a way as to capture a wide range of different event combinations that lead to the discharge of oil from a shipwreck. The examination of actually documented cases of oil leaking from a shipwreck, reveals that this undesired event can occur either due to a breach of the hull (e.g., caused by the effect of corrosion), or due to a shift in the position of the shipwreck (e.g., caused by external factors). The latter was the case for the USS Mississinewa, from which a leak was initiated after it was shifted by a typhoon that hit the area (Monfils et al., 2006). The main failure modes considered for the occurrence of a hull breach were corrosion, structural fatigue and structural damage that is effected by a change of position under the influence of an external factor. It is important to note that corrosion and fatigue are both dynamic processes that evolve continuously through time and have a major impact on the structural integrity of the hull of the shipwreck. The structure of the developed DFT (i.e., basic events and types of connection gates) is described in more detail below.
The event “Oil discharge due to hull breach” depends on the basic events “Existence of oil on ship” and “Existence of oil on specific point of the ship” and the intermediate event “Hull breach,” which in turn are combined using a PAND gate (Figure 1). The dynamic gate imposes the condition that the connected events need to occur in a specific order (from left to right) for the resulting event to occur. This structure reflects the following conditions for oil to be discharged into the marine environment: (a) there must be some quantity of oil present somewhere in the shipwreck, (b) the hull must be breached, and (c) oil needs to be present at the specific point where the hull was breached.
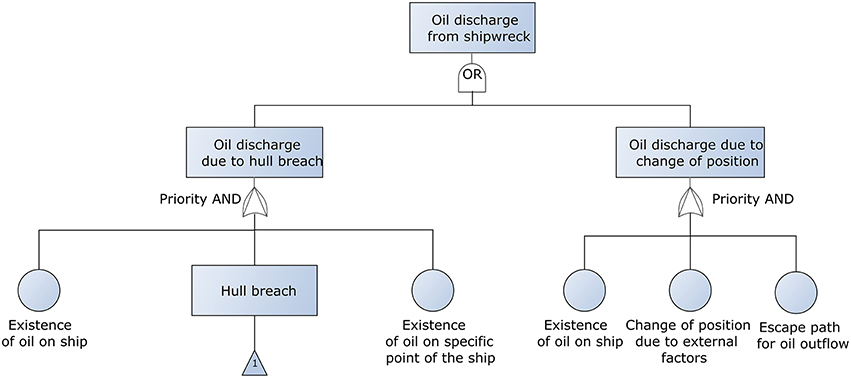
Figure 1. The part of the developed Dynamic Fault Tree (DFT) that calculates the probability of oil discharge.
Similarly, the event “Oil discharge due to change of position” depends on the basic events “Existence of oil on ship,” “Change of position due to external factors,” and “Escape path for oil outflow,” which are combined with a PAND gate (Figure 1). This structure reflects the following conditions for oil to be discharged into the marine environment: (a) there must be some quantity of oil present somewhere in the shipwreck, (b) an external factor needs to affect the position of the shipwreck, and (c) there must be an available path through the structure for the oil to escape. It must be noted that the basic event “Existence of oil on ship” was added to the DFT to take into account the high level of uncertainty regarding the potential quantities of trapped oil inside a shipwreck.
The probability of the hull breaching is calculated by the structure shown in Figure 2, where the various events are connected with static OR gates, which implies that the occurrence of any one of the connected events is sufficient for the resulting event to occur. For example, the hull will be breached either due to fatigue, or corrosion, or due to a change of position under the influence of an external factor. Structural fatigue can be attributed to the effect of periodic (usually tidal) and non-periodic sea-water movements such as waves and sea currents (Bartholomew et al., 1992). Structural damage due to the change of position can be better understood by considering the scenario when a shipwreck lies unsteadily on a rocky slope and is pushed deeper toward the sea bottom after the occurrence of an earthquake.
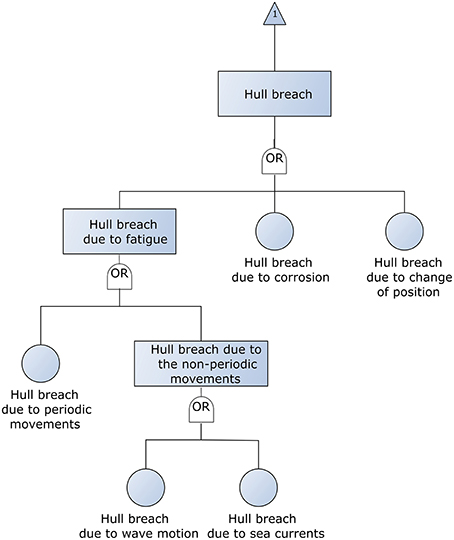
Figure 2. The part of the developed DFT that calculates the probability of the shipwreck hull to be breached.
Probabilities of Basic Events
The data that are usually available for a shipwreck, are both qualitative (e.g., accident type, ship type, etc.) and quantitative (e.g., depth, age of shipwreck, distance from the nearest shore, etc.). Since the availability of qualitative data might be greater compared to that of quantitative data, it is useful to combine these different types of information for calculating specific probability values for the basic events. However, qualitative data are often vague, a fact that consequently limits their reliability. In this paper, basic events probabilities are either directly defined by correlating specific values of probabilities to qualitative data (based on expert opinion), or indirectly by deterministically updating an initial probability (based on “site-specific” quantitative data). The initial probability has been estimated according to the relevant classification applied in the SWERA Project, as referenced in Symons (2013). More details on how various basic event probabilities were defined are given below:
• The probability of oil being present in the shipwreck is defined by the type of accident2, which indirectly affects the extent of structural damage the ship suffered prior to sinking. More extensive initial structural damage, means that more oil could have leaked from the ship prior to sinking and consequently, less oil is probably still inside the shipwreck.
• The probability of oil being present at the specific point where the hull has been breached is defined by the ship type3, which indirectly whether a ship carries oil as cargo as well as the specific tank arrangement. With increasing values of the total oil tank volume to total ship volume ratio, it is less probable that oil will be present at any specific point where the hull has been breached.
• The probability that an escape path for oil outflow is available is defined by the type of the accident. This probability is assumed to increase with more extensive initial structural damage.
• The probability of the hull breaching due to a change of the shipwreck position has been defined as a function of the possible effect of an earthquake (i.e., the seismic hazard linked to a specific location). Other external factors (e.g., extreme storms, typhoons, or tsunamis) have been excluded from the analysis because they were considered irrelevant for Greek waters. The Greek Seismic Code(EPPO 2000) distinguishes three regions of seismic hazard (low, moderate, and high) according to the average recorded seismic intensity. Therefore, the initial probability for a location of moderate seismic activity has been assumed equal to 10−6.
• The probability of a potential oil discharge due to a change of position has been defined as a function of the orientation of the shipwreck (i.e., secured, semi-secured or not secured against a violent impact on the sea bottom due to a change of position). For example, a shipwreck that lies on a sandy sea bottom upright is considered secured. Therefore, the initial probability for a semi-secured shipwreck has been assumed equal to 10−5.
• The probability of the hull breaching due to fatigue has been calculated as a function of the time the structure has been exposed to alternating stresses, caused by periodic and non-periodic movements of the sea water. The probabilities for the basic events corresponding to the hull breaching due to periodic movements, wave motions and sea currents, have been initially set equal to 10−6, for shipwrecks that have been submerged for 54 years. This is the average value for cases recorded in the NTUA Shipwreck Database (Ventikos et al., 2013). To calculate the case-specific probability we assumed a positive linear relationship and used the following formula:
For the calculation of this probability, the following assumptions have been made: (a) the effect of wave motions on the structure of the shipwreck is negligible below 10 m depth, and (b) the effect of periodic water movements on the structure of the shipwreck are solely attributed to tidal motions.
• The probability of the hull breaching due to corrosion has been calculated as a function of the time the structure has been exposed to the deteriorating effects of the marine environment. Considering an average hull thickness of 20 mm (Hammer 2010), we have assumed that there will certainly be a breach in the hull, when the amount of material removed due to corrosion equals 20 mm. The corresponding initial probability is therefore assumed to be PHull Breach | Corrosion = 20mm = 1. It is important to note that this assumption possibly leads to an underestimation of the probability, since the hull might be breached far sooner. The next step was to estimate the total amount of hull corrosion by estimating the corrosion rate for each shipwreck. Finally, the probability was calculated assuming a positive linear relationship by using the following formula:
The corrosion rate for each shipwreck was estimated using Wein's number, as described by Johnson et al. (2011). According to this approach, the corrosion rate can be estimated provided temperature, oxygen concentration and concretion thickness are known for a specific location. These environmental parameters have been approximated as a function of shipwreck depth, as for example found in the website Corrosion Doctors(2015) for a specific test site in the Pacific Ocean.
Ranking of Potential Consequences
The potential oil discharge from sunken shipwrecks has a multitude of possible consequences, ranging from impacts on the biota of the marine environment to impacts on the fishing and tourist activities. This paper presents a high-level qualitative evaluation approach, by ranking each shipwreck on defined consequence indicators that correspond to different consequence categories. The ranking process enables a relative comparison between shipwrecks accounting for a wide array of consequences according to the availability of relevant information. For each consequence indicator, a custom scale has been created with values ranging from 1 to 10. Subsequently, the different consequence indicators are aggregated in the total CI by averaging the different values. The various indicators are classified into different consequence categories, as described below:
Difficulty of Remediation
This category includes the following consequence indicators: the potential remaining oil quantity in the shipwreck, the type of remaining oil (light, crude or heavy) and the nearest coast type (rocky coast, beach, etc.). As highlighted by Michel et al. (2005), the viscosity of the remaining oil has a major impact on the difficulty of removal and remediation operations. It should be noted that the coast types have been taken from the coast line dataset of the European Environment Agency (EEA 2015).
Immediacy of Impact
The immediacy of potential consequences is estimated by the distance of the shipwreck from the nearest shore. For example, the consequences of an oil leak from a shipwreck that is located at a great distance from the nearest shore will not be immediate. The depth of the shipwreck has also been taken into account for the calculation of the distance from the shore, accounting for the vertical distance the oil will have to travel before it reaches the surface of the sea.
Potential Impact on Economic Activities
This category accounts for the possible disruptions to the fishing, aquaculture and tourist activities in a specific geographic location by a potential oil leak. Based on data available from the Greek Ministry of Environment and Energy(MEE 2015) and the Ministry of Rural Development and Food(MRDF 2015), the analysis has defined two consequence indicators that show how developed the relevant local activities are. It has been assumed that the possible disruptions will be greater for more developed activities.
Potential Impact on Human Health
This category includes a consequence indicator related to population density, which was based on publicly available data from the Hellenic Statistical Authority(Geodata 2015). It has been assumed that the severity of potential consequences from an oil leak will increase with the population density in the area that is in the proximity of the shipwreck location.
Potential Ecological Impact
This category accounts for the consequences to the biota of especially fragile marine environments, such as the protected areas in the European Natura 2000 network(GBWC 2015). It has been assumed that the consequence severity is a function of the distance of the shipwreck location from a protected area.
Results
This section of the paper attempts to evaluate the performance of the proposed model. The presented methodology includes an appropriate sensitivity analysis, to identify the input variables with the most significant effect on the values of the RI, and testing the model on selected real-world cases. The aim is to test the validity and evaluate the usefulness of the results from the proposed risk assessment process.
Sensitivity Analysis
The sensitivity analysis presented in this paper is based on the “one-factor-at-a-time” approach (Rohmer et al., 2014), and aims to determine the input variables that have the greatest effect on the RI and to evaluate the developed DFT model. The base case for the sensitivity analysis was taken from the NTUA Shipwreck Database. The profile of the base case is a non-tanker cargo ship that sank due to heavy weather damage, has been submerged for about 10 years, at a depth of about 10 m and lies semi-secured on the sea bottom. The trapped oil quantity in the shipwreck is estimated at about 170 tons of light fuel oil. The shipwreck is located in a non-protected area of moderate seismic hazard, 0.037 km from a shore with man-made structures. The area is highly populated, with highly developed tourist and fishing activities. The probability of an oil leak from this shipwreck is estimated as reasonably probable (FI = 5), the potential consequences to the marine environment and local area are estimated as significant (SI = 2) and the overall risk is estimated as major (RI = 7). The analysis examined the variation of the probability of oil discharge, the CI, as well as the variation of the RI.
As shown in Figure 3, the depth of the shipwreck has the greatest effect on the probability of oil discharge, which decreases by increasing depth. This is due to the fact that depth affects both fatigue (i.e., water motions are less pronounced at great depths), and corrosion (i.e., lower temperatures and oxygen concentrations at great depths result in lower corrosion rates). The age of the shipwreck has a proportionate relationship to the probability, which accounts for both fatigue and corrosion, i.e., the probability of a hull breach increases when the structure has been submerged for long time periods. The third most important variable is the type of accident, which is directly linked to varying damage to the shipwreck. The probability of oil discharge is reduced with increasing damage extent, because it is more likely for oil to have leaked during the accident, and therefore not remain trapped in the shipwreck. It must be noted that the variables “Orientation” and “Seismic hazard” have a minimal effect on this probability.
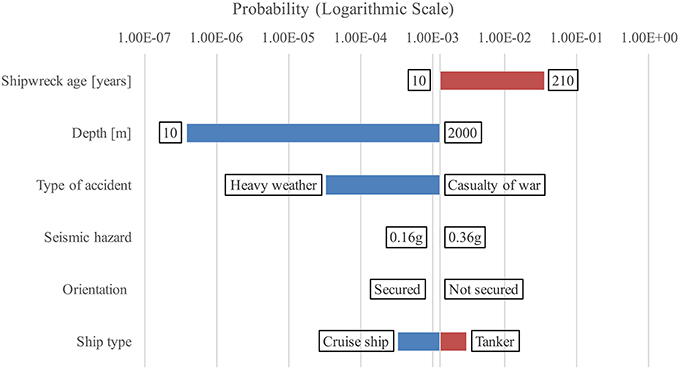
Figure 3. Tornado diagram showing the effect of the input variables on the probability of oil discharge.
Regarding the variation of the CI, the analysis yielded the results as shown in Figure 4. Evidently, the estimated remaining oil quantity has the greatest effect on the severity of the consequences, which can be argued that it may vary widely depending on the accuracy of this estimation. The type of oil has the second largest effect on the CI, which shows that oil viscosity affects greatly the difficulty of the potential remediation actions. Another factor that significantly affects the potential remediation actions is the coast type of the nearest shore. Additionally, the variable “Distance from the nearest shore” has a measurable inversely related effect on the value of the CI, which accounts for the fact that the farther away a shipwreck is situated from a shoreline, the consequences will be less immediate. It must be noted that the variables determining whether the area is protected, how developed the local tourist and fishing activities are as well as the population density have a minimal proportionate effect on the severity of the consequences.
The same methodology was applied to investigate the variation of the RI (Figure 5), which is a function of both the probability of oil discharge and the CI. The variables with the greatest effect on the RI are the depth of the shipwreck and the accident type, which are also two of the major contributing factors to the value of the probability of oil discharge. It should be noted that the variation of the variables “Shipwreck age,” “Estimated quantity of oil,” “Oil type,” and “Nearest coast type” have an equal effect on the RI. An interesting result was that the RI remained unchanged with varying values of the seismic hazard of the area, how securely the shipwreck is oriented on the sea bottom, whether the shipwreck is in a protected area, how developed the fishing and tourist activities are and how densely populated the nearby area is. The results of the sensitivity analysis led to the conclusion that the outlined risk assessment methodology is quite stable, i.e., wide variations in the input variables result in relatively narrow variation in the final risk value. However, it is important to note that this insensitivity is largely owed to the use of the risk matrix to combine the values of the FI and SI.
The sensitivity analysis concludes with an examination of how the probability of oil discharge varies as a function of time. The time-dependent sensitivity analysis was conducted for a period of 60 years, on 3 specific non-tanker cargo ships that were taken from the NTUA Shipwreck Database (Figure 6). The input variable that changed as a function of time is the age of the shipwreck, which consequently affects the basic events in the DFT connected to fatigue and corrosion. The probability for Test Case 1, which is the passenger vessel Corfu Island (submerged for 29 years), increased by 68%. The probability for Test Case 2, which is the general cargo ship Evripos (submerged for 6 years), increased by 91%. Finally, the probability for Test Case 3, which is the warship Alcyone (submerged for 74 years), increased by 43%. It must be noted that while the probability of oil discharge increases as a function of time for all test cases, the increase is sharper for newer shipwrecks compared to older shipwrecks. Another interesting result is that the probability is inverse to the age of the shipwreck, which accounts for the fact that corrosion rates are not constant, but rather follow a logarithmic growth. This means that the corrosion rate tends to converge to a certain value over a period of time, due to the formation of protective layers of concretion on the hull plating (Overfield, 2005).
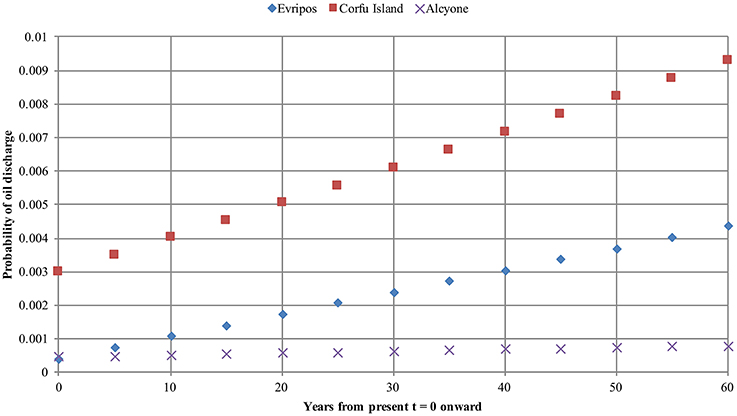
Figure 6. Time-dependent sensitivity analysis applied on test cases taken from the NTUA Shipwreck Database.
Model Test on Real-World Shipwrecks
The aim of the model test was to evaluate the usefulness of the risk assessment results, for example by comparing with authoritative assessments of how dangerous a shipwreck is considered to be. The test cases were selected according to the availability of adequate information regarding their status and condition. Another criterion was that the test cases should cover a wide range of input values and hence facilitate the discovery of misleading results.
The test cases were taken from the NTUA Shipwreck Database, which contains information on 402 potentially polluting shipwrecks that sank from the years 1940 up to 2009 in Greek waters. The test sample consisted of 21 cases, including 6 tankers, 10 non-tanker cargo ships (bulk carriers, general cargoes, container ships, and passenger ferries) and 5 warships. These shipwrecks sank under various circumstances, ranging from groundings and collisions to casualties of war, in various locations across Greek waters (ranging from 0.04 to 50 km distance from the nearest shore). Moreover, they are located in depths ranging from 8.3 to 4100 m and have been submerged for time periods ranging from 6 to 75 years. The potential remaining oil quantities were taken from the work by Ventikos et al. (2013), which combined the estimation methodology proposed by Michel et al. (2005) with actual information, where available. It is important to note that these estimations were used to obtain a Low and High value for the RI, accounting for the relevant uncertainty. The results for the tanker, non-tanker cargo ships and warship test cases are shown in Tables 2–4 respectively.
The difference between the resulting low and high risk values (per ship type) is illustrated in Figure 7. The risk values for warships remain unaffected by the uncertainty in the estimated quantity of oil, as it has been assumed that most of the oil they carried will have leaked while the ship was sinking. Additionally, the difference between the resulting low and high risk values for tanker ships is owed to the assumption that they contain very large trapped oil quantities.
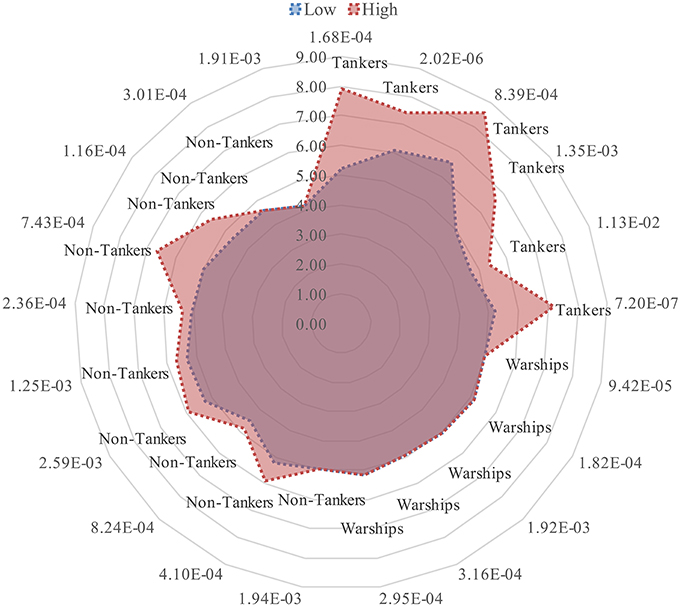
Figure 7. Polar diagram that illustrates the difference between the low and high risk rating of the test cases from the NTUA Shipwreck Database per ship type. The angular dimension is the probability of oil release and the linear dimension is the value of the CI.
To simplify the comparison between different ship types, the risk values in the following figures have been based on the average of the low and high estimations for the remaining oil quantity. Figure 8 shows that, compared to non-tanker cargo ships and warships, tankers have the largest risk rankings, i.e., high values in both probability and consequence severity. Additionally, although non-tanker cargo ships and warships present lower values of consequence severity compared to tankers, the range of consequences is more dispersed and the probability values are higher.
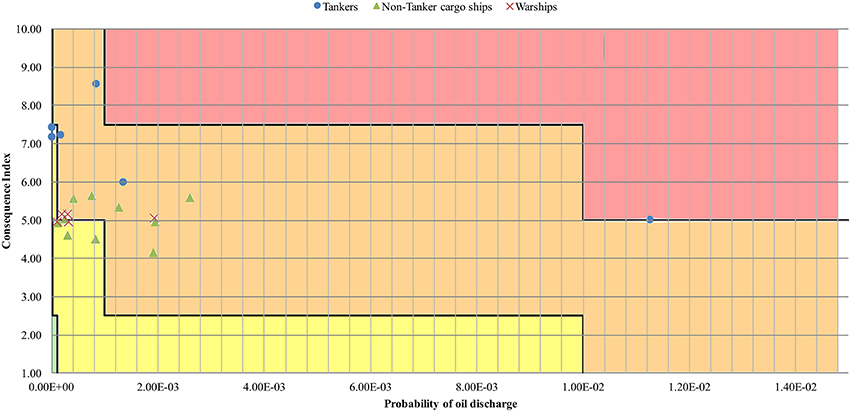
Figure 8. Risk values for each test case as a function of the probability of oil discharge and the CI per ship type.
The following results demonstrate the dependence of the probability of oil discharge on depth and age of the shipwreck respectively. Shipwrecks of any ship type that are located in depths over 200 m generally present lower probabilities of oil discharge (Figure 9), indicative of the fact that corrosion is slower at great depths. Furthermore, the probability of oil discharge increases with the age of shipwrecks for cases up to 40 years (Figure 10). However, shipwrecks that have been submerged for over 60 years present relatively low probability values. These cases include ships that were lost as a casualty of World War II and hence it has been assumed that the existence of oil in these shipwrecks is less probable. At this point it must be argued that this assumption might not be valid for tanker ships that were casualties of war. The existence of trapped oil in these shipwrecks could be more probable due to the smaller damage they might have suffered compared to warships.
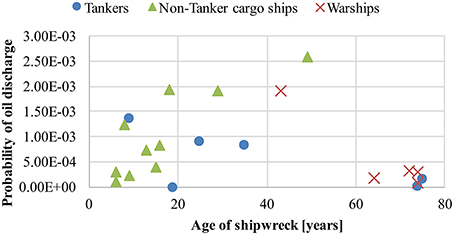
Figure 10. Probability of oil discharge as a function of the shipwreck age, in years, per ship type.
From the sensitivity analysis, it was concluded that the distance of the shipwreck location from the nearest shore has a measurable effect on the severity of the consequences. Figure 11 illustrates this effect across the various ship types that were examined. Evidently, oil leaking from shipwrecks that are located at very small distances from the nearest shore will have severe or catastrophic consequences even when the oil quantities are relatively small. Moreover, large oil quantities leaking from shipwrecks that are located at large distances from the nearest shore will have similarly severe consequences.
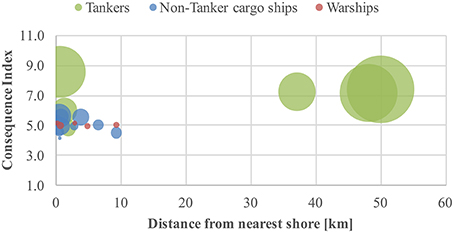
Figure 11. CI as a function of the average estimation for potential remaining oil quantity and the distance from the nearest shore.
The majority of shipwrecks contained in the NTUA shipwreck database, and especially that do not constitute a navigational hazard, have not been authoritatively characterized as dangerous or otherwise. However, one of the shipwrecks that have indeed been assessed is the tanker Irenes Serenade (which was used for the model test). This shipwreck is not deemed a threat to the environment and therefore no further oil removal actions are to be taken. The tanker Irenes Serenade, which sank as a result of an explosion, has been submerged for 35 years in a depth of 40 m, at a very small distance from the nearest shore (0.6 km). At the time of the sinking it was carrying 100,000 t of oil cargo, which was completely removed a short time after the incident. The results for this test case were: probability of oil discharge between extremely remote and remote and severe consequences. Consequently, the resulting risk ranking was high. Although this result might seem counter-intuitive, it is owed to the fact that an estimated bunker fuel quantity between 1080 and 8600 tons still remain in the shipwreck. Therefore, while the oil cargo was completely removed, the release of the bunkers could result to equally devastating consequences to the local environment. This conclusion highlights the importance of focusing removal actions not only on the potentially remaining oil cargo but also on the potentially remaining bunker fuel.
Discussion
The comparison of the results from the developed DFT model with the corresponding results from the FIS that was developed by Ventikos et al. (2013), resulted in the conclusion that the two approaches are generally consistent with each other. In particular, both approaches resulted in comparable risk ratings, especially for shipwrecks that are located in depths up to 500 m. However, for shipwrecks that are located in depths over 500 m, the DFT approach resulted in significantly lower risk ratings, compared to the FIS approach. This can be attributed to the fact that the DFT approach takes into account lower corrosion rates at great depths and the larger distance the leaked oil has to travel before it reaches the surface. The FIS approach considered depth only as a factor of potential damage to the ship hull during its descent to the sea bottom. Furthermore, the DFT approach incorporates a wider array of consequence factors (i.e., dangers to human health and impacts on the fishing and tourist activities), which resulted in comparatively higher risk ratings. It might be argued that the FIS approach underestimated the severity of the consequences and overestimated the probability of oil leaking from a shipwreck. Therefore, the FIS could be further improved on both of these aspects.
The methodology that has been demonstrated takes a step closer toward a more quantitative approach to shipwreck risk assessment, which is a direction supported in the relevant literature by the work of Landquist et al. (2014). The rational results obtained from feeding real-world data into the model show that such a risk assessment can be benefited from more accurate modeling of the complex relationships between the various factors. Furthermore, the outlined methodology has addressed the need to effectively combine quantitative with qualitative data to obtain useful results that are based on often scarcely available information. Some indicative limitations of the presented approach are its semi-quantitative nature, its insensitivity to varying estimations of the remaining quantity of oil, and that certain special cases (such as tanker ships that sank during World War II) need to be investigated further. Another limitation that should be considered is the use of a risk matrix that was not tailored to fit the specific application and therefore is not appropriately calibrated.
Some issues to consider for the future direction of this research are data accuracy and improving upon various assumptions. The verification of the data stored in shipwreck databases (such as the NTUA Shipwreck Database) with data collected, for example, by dedicated shipwreck surveys, would lead to increased confidence in the results of the risk assessment and would also provide a means for validating the developed models. In terms of assumptions, the inclusion of more factors and the application of improved models would provide with more accurate estimations of the basic event probabilities as well as the severity of the potential consequences. However, although increasing accuracy is often desirable, the level of detail that is actually needed to obtain a useful risk value should be carefully examined. The benefit should be carefully weighed against the cost in resources for data collection or more complex modeling. Another point would be to further examine the effects of the various sources of uncertainty, such as the uncertainty inherently found in expert opinion, on the risk results.
Conclusions
The main conclusion from the results presented in this paper is that the application of DFT analysis, as well as the application of other similar quantitative methods, in the field of shipwreck risk assessment appears to be promising. The DFT approach has the potential to yield useful results, because the submerged shipwreck is dynamically linked to its local environment, often in uncertain and complex ways. Additionally, in terms of accurately modeling the various relationships, the DFT appears to be an improvement over the FIS approach, which, however, could still contribute significantly to modeling uncertainties (especially those involved in expert opinion) and combining qualitative with quantitative data. A significant drawback of the proposed approach is that it is based on many assumptions and hypotheses that need to be improved upon in order to maximize the confidence in the resulting risk rankings. Therefore, further research should be directed toward developing more quantitative shipwreck risk assessments, which will be based on more data than assumptions.
Regarding the hazard of oil leaks from submerged shipwrecks, a significant conclusion is that environmental threat is multi-faceted and therefore any relevant risk assessment effort should consider a multitude of parameters and factors. The example of the tanker Irenes Serenade is illustrative of the fact that while oil cargo is the main factor contributing to the environmental threat, the leak of potentially significant quantities of bunker fuel should not be discounted. In addition, Greece is an indicative example for the need for a proactive approach to this problem because its high value tourist and aquaculture activities are especially dependent on a pristine marine environment. Furthermore, as demonstrated in the presented sensitivity analysis, the calculation of the oil discharge probability or the severity of the potential consequences are affected by various sources of uncertainty that ultimately subtract from the confidence in the resulting risk values. Further investigation in the effect of the ensuing uncertainties is consequently needed for effectively managing the risks from potentially polluting shipwrecks. This paper concludes with the note that future research in the field of shipwreck risk assessment should focus on formulating a unified risk framework, for addressing this particular threat in a uniform manner, as well as developing appropriate risk acceptance criteria that would aid in maintaining a certain level of cost-effectiveness for any proposed measures of risk mitigation.
Author Contributions
NV is the head and supervisor of this research effort and co-authored the paper. KL, AK, and PD had an equal contribution to conduncting the research and authoring the paper.
Conflict of Interest Statement
The authors declare that the research was conducted in the absence of any commercial or financial relationships that could be construed as a potential conflict of interest.
Footnotes
1. ^About 15,000 km of coastline and a total of 2500 islands and islets.
2. ^The accident types considered for this analysis are: foundering due to heavy weather, mechanical failures, hull cracks, collisions, groundings, severe structural failures, explosions, war casualties.
3. ^The ship types considered for this analysis are: tankers, non-tanker cargo ships, passenger vessels, cruise ships.
References
Alcaro, L., Amato, E., Cabioch, F., Farchi, C., and Gouriou, V. (2007). DEEPP Project Development of European Guidelines for Potentially Polluting Shipwrecks. Rome: DEEPP Project.
Bartholomew, C. A., Marsh, B., Hooper, R., and United States Naval Sea Systems Command. (1992). U.S. Navy Salvage Engineer's Handbook: Salvage Engineering. Washington, DC: Direction of Commander, Naval Sea Systems Command.
Boudali, H., Crouzen, P., and Stoelinga, M. (2007). “Dynamic fault tree analysis using input/output interactive markov chains,” in Proceedings of the 37th Annual IEEE/IFIP International Conference on Dependable Systems and Networks (Edinburgh), 708–717.
Distefano, S., and Puliafito, A. (2007). “Dynamic reliability block diagrams vs. dynamic fault trees,” in Annual Reliability and Maintainability Symposium, RAMS (Orlando, FL), 71–76.
Dugan, J. B., Bavuso, S. J., and Boyd, M. A. (1992). Dynamic fault-tree models for fault-tolerant computer systems. IEEE Trans. Reliab. 41, 363–377.
Etkin, D. S. (1999). Estimating cleanup costs for oil spills. Int. Oil Spill Conf. Proc. 1999, 35–39. doi: 10.7901/2169-3358-1999-1-35
Foecke, T., Ma, L., Russell, M. A., Conlin, D. L., and Murphy, L. E. (2010). Investigating archaeological site formation processes on the battleship USS Arizona using finite element analysis. J. Archaeol. Sci. 37, 1090–1101. doi: 10.1016/j.jas.2009.12.009
Hafiz, M. H. (2010). A novel predict corrosion rate model based on RBFNN. Modern Appl. Sci. 4, 51–57. doi: 10.5539/mas.v4n9p51
Helton, D. (2003). “Wreck removal: a federal perspective,” in National Maritime Salvage Conference (Seattle), 1–15.
IMO (2013). Revised Guidelines For Formal Safety Assessment (FSA) for Use in the IMO Rule-Making Process. MSC-MEPC.2/Circ.12. London, UK.
Johnson, D. L., Medlin, D. J., Murphy, L. E., Carr, J. D., and Conlin, D. L. (2011). Corrosion rate trajectories of concreted iron and steel shipwrecks and structures in seawater-the weins number. Corrosion 67, 125005-1–125005-9. doi: 10.5006/1.3666859
Johnson, D. L., Wilson, B. M., Carr, J. D., Russell, M. A., Murphy, L. E., and Conlin, D. L. (2006). Corrosion of steel shipwreck in the marine environment: USS arizona-part 1. Mater. Perform. 45, 40–45.
Kamm, N. (2011). An Overview of Pollution from Shipwrecks. Ph.D. dissertation, University of Cape Town.
Landquist, H., Hassellöv, I. M., Rosén, L., Lindgren, J. F., and Dahllöf, I. (2013). Evaluating the needs of risk assessment methods of potentially polluting shipwrecks. J. Environ. Manage. 119, 85–92. doi: 10.1016/j.jenvman.2012.12.036
Landquist, H., Rosén, L., Lindhe, A., Norberg, T., Hassellöv, I.-M., Lindgren, J. F., et al. (2014). A fault tree model to assess probability of contaminant discharge from shipwrecks. Mar. Pollut. Bull. 88, 239–248. doi: 10.1016/j.marpolbul.2014.08.037
Macleod, I. D., Morrison, P., Richards, V., and West, N. (2004). “Corrosion monitoring and the environmental impact of decommissioned naval vessels as artificial reefs,” in Metal 2004: Proceedings of the International Conference on Metals Conservation (Canberra, ACT: National Museum of Australia), 53–74.
Michel, J., Etkin, D. S., Gilbert, T., Urban, R., Waldron, J., and Blocksidge, C. T. (2005). “Potentially polluting wrecks in marine waters,” in 2005 International Oil Spill Conference (Miami Beach, FL).
Monfils, R. (2005). “The global risk of marine pollution from WWII shipwrecks: examples from the seven seas,” in 2005 International Oil Spill Conference (Miami Beach, FL), 1–18.
Monfils, R., Gilbert, T., and Nawadra, S. (2006). Sunken WWII Shipwrecks of the Pacific and East Asia: the need for regional collaboration to address the potential marine pollution threat. Ocean Coastal Manage. 49, 779–88. doi: 10.1016/j.ocecoaman.2006.06.011
Overfield, M. L. (2005). “Corrosion on deep gulf shipwrecks of World War II,” in 2005 International Oil Spill Conference, IOSC 2005 (Miami Beach, FL), 11242–11248.
Rohmer, J., Douglas, J., Bertil, D., Monfort, D., and Sedan, O. (2014). Weighing the importance of model uncertainty against parameter uncertainty in earthquake loss assessments. Soil Dyn. Earthquake Eng. 58, 1–9. doi: 10.1016/j.soildyn.2013.11.006
Russell, M. A., Conlin, D. L., Murphy, L. E., Johnson, D.L., Wilson, B. M., and Carr, J. D. (2006). A minimum-impact method for measuring corrosion rate of steel-hulled shipwrecks in seawater. Int. J. Naut. Archaeol. 35, 310–318. doi: 10.1111/j.1095-9270.2006.00101.x
Svensson, E. (2010). Potential Shipwreck Pollution in the Baltic Sea Overview of Work in the Baltic Sea States. Gothenburg: Swedish Maritime Administration and Lighthouse.
Symons, L. (2013). National Oceanic and Atmospheric Administration. 2013. Risk Assessment for Potentially Polluting Wrecks in U.S. Waters. Silver Spring, MD: National Oceanic and Atmospheric Administration. 127 + appendices.
Keywords: shipwreck, risk assessment, oil spill, dynamic fault tree, probabilistic approach
Citation: Ventikos NP, Louzis K, Koimtzoglou A and Delikanidis P (2016) Enhanced Decision Making through Probabilistic Shipwreck Risk Assessment: Focusing on the Situation in Greece. Front. Mar. Sci. 3:97. doi: 10.3389/fmars.2016.00097
Received: 30 November 2015; Accepted: 30 May 2016;
Published: 14 June 2016.
Edited by:
Francois Galgani, Institut Français de Recherche pour l'Exploitation de la Mer, FranceReviewed by:
Rodrigo Riera, Atlantic Environmental Marine Center (Centro de Investigaciones Medioambientales del Atlántico SL), SpainJongseong Ryu, Anyang University, South Korea
Copyright © 2016 Ventikos, Louzis, Koimtzoglou and Delikanidis. This is an open-access article distributed under the terms of the Creative Commons Attribution License (CC BY). The use, distribution or reproduction in other forums is permitted, provided the original author(s) or licensor are credited and that the original publication in this journal is cited, in accordance with accepted academic practice. No use, distribution or reproduction is permitted which does not comply with these terms.
*Correspondence: Nikolaos P. Ventikos, bml2ZW5AZGVzbGFiLm50dWEuZ3I=; bml2ZW5AbWFpbC5udHVhLmdy