- Research and Development Team, Environmental Defense Fund Oceans Program, San Francisco, CA, USA
The importance of scaling initiatives that promote environmental protection and conservation is almost universally recognized. But how is scaling best achieved? We empirically evaluated the relationship of a list of factors that have been postulated to facilitate successful scaling to the degree of scaling success achieved in 56 case studies from a variety of sectors. We identified 23 factors that are significantly associated with successful scaling, defined as self-replication: an innovation that is congruent with local sociocultural patterns, takes advantage of existing scaled infrastructure, and facilitates a paradigm shift; adequate resources and constituencies for scaling, secured from the start, drawn from both within and outside the system; pilot sites that reflect conditions at future sites rather than ideal conditions; clear and deliberate scaling expectations and strategy; capitalization on economies of scale; a project team that has a unifying vision, includes both individuals who helped design the innovation and members of the target audience, and empowers users with the requisite skills; target audiences that take ownership of the project; the provision of long-term support systems; ongoing learning about the factors influencing scaling; direct management of relevant supply and demand streams; targeted marketing and dissemination efforts; and the evaluation of scaling success indicators. We also explored correlations between these principles, and identified a group of principles that together explain nearly 40% of the variance in success: the provision of long-term support systems (or one of its surrogates: turning users into partners, a user organization with wide reach, and the empowerment of the target audience with requisite skills); resources mobilized from within and outside the system; user organizations that have the capacity to implement the innovation; innovations that are platform solutions and that provide rapid feedback; and pilot sites that have realistic conditions relative to future sites. Our results suggest that for scaling to be successful: (1) scaling must be considered at all stages of a project; (2) the context must be managed and barriers to scaling must be identified and removed and (3) deliberate attention must be paid to scaling methods, marketing and dissemination efforts, and long-term monitoring of scaling progress.
Introduction
Many environmental problems have been solved at local scales, but it is becoming increasingly clear that these solutions do not always spread across larger geographies. For example, marine protected areas demonstrably achieve many ocean conservation goals at local scales (Lester et al., 2009) when they are designed and implemented well (Airamé et al., 2003; Mora, 2006; Edgar et al., 2014) but cover less than 2% of the ocean's surface after over 20 years of implementation efforts (Heal and Rising, 2014). Ideally of course, successful conservation initiatives would scale spontaneously, with little or no expenditure of financial or human capital. However, cases of such spontaneous scaling appear to be rare (Middleton et al., 2002; World Health Organization and ExpandNet, 2009; Management Systems International, 2012). More often than not organizations put extensive time and effort into designing and implementing pilot projects to ensure that their innovation will be successful, but not into preparing the ground for scaling up (World Health Organization ExpandNet, 2011; Hartmann et al., 2013). In fact, pilots are often executed where and when conditions are thought to maximize success, leaving organizations unsure of how to apply pilot project lessons and experiences to new sites (World Health Organization ExpandNet, 2011). This in turn often leads to scaling failures where excessive effort and capacity must be expended in order to ensure the success of new projects (World Health Organization ExpandNet, 2011; Management Systems International, 2012), or where the innovation simply fails to catch on at new targeted sites (World Health Organization ExpandNet, 2011).
Many authors across a variety of disciplines have written about factors that should facilitate efforts to spread ideas or innovations or ensure scaling success. For example, in 1978 Granovetter detailed his Threshold Models of Collective Behavior, which seek to explain how new behaviors spread and new norms emerge as different individuals' “thresholds” at which they will adopt an innovation or new behavior are crossed (Granovetter, 1978). These thresholds are determined by the variable cost/benefit ratio of adopting the given innovation to that specific person, and they dictate how many of the person's peers must first adopt the new behavior before that person will do so (Granovetter, 1978). Shortly after the Threshold Models were being developed, Prochaska and DiClemente (1982) developed the Trans-Theoretical Model of Change, which describes the conceptual stages individuals transition through when attempting to change their behavior: encountering the new behavior, considering adoption, determining to adopt, adoption, and finally maintenance (Prochaska and DiClemente, 1982; Butler et al., 2013). This concept of adoption stages has been adapted and applied by a variety of individuals and groups seeking to facilitate the spread of a new innovation or idea.
The Diffusion of Innovations theory, first published by Rogers (1962), attempts to explain scaling by identifying key target audiences that vary in their thresholds for adopting an innovation. Five distinct audience segments are delineated: innovators; early adopters; early majority; late majority; and laggards, each of which will take more or less time, and need different types of information and levels of confidence, to adopt a new innovation (Rogers, 1962).
In his 1991 book Crossing the Chasm, Moore modifies Rogers' original five adopter groups, calling them innovators, visionaries (early adopters), pragmatists (early majority), conservatives (late majority), and laggards. He goes on to describe a “chasm” between the visionaries and the pragmatists, explaining that the innovators and visionaries are actively seeking new technologies simply for the sake of advancing a given field and are accepting of some element of risk, while the pragmatists and conservatives (which essentially differ only in their thresholds for adoption) need to understand how the innovation will benefit them personally and want a stable and proven product before they will be willing to try it out (Moore, 2002). The key insight is that visionaries and pragmatists want different things, so different marketing strategies must be used for each (in contrast to the previously-prevalent marketing model, which suggested using product success in each group as a base for marketing to the next group) (Moore, 2002).
The principles described above have recently been embraced by the discipline known as “social marketing” to facilitate the spread of ideas, behaviors, or innovations to improve public wellbeing and address social problems. Social marketing campaigns have been in use for a number of years in the fields of public health and international development to encourage behavior changes such as healthy eating and quitting smoking, and spread innovations in health care and microfinancing for remote communities (e.g., Simmons et al., 2007; Rosenberg, 2011). More recently, groups like Rare (http://www.rare.org/) and individuals like Doug Mckenzie-Mohr (http://www.cbsm.com/) have pioneered the use of these tenets in efforts to spread conservation-relevant behaviors such as sustainable agriculture recycling, and water and electricity conservation (Fuller et al., 2010; Ardoin et al., 2013; Butler et al., 2013; Mckenzie-Mohr, 2013).
Importantly, most of the above-mentioned work has been directed toward understanding and applying tools to facilitate and enhance the spread of behaviors or innovations from one individual to another. Significantly less work has been done around understanding the factors that might improve the spread of group or community-scale behaviors from one geographically distinct area to another, as is generally necessary in the scaling of a conservation innovation. Although many of the factors and principles that authors have suggested will improve the individual-individual transmission of ideas may also be useful when considered in a larger-scale context, there are other factors that appear to be important in the spread of innovations between groups. For example, Kern et al. (2001) describe conditions likely to facilitate or hinder the spread of environmentally-relevant policies from one country to another. In their book Scaling Up Excellence, Sutton and Rao (2014) detail an extensive set of principles and tactics they argue can ease and ensure the successful growth of companies and organizations across sectors. The World Health Organization, ExpandNet, Management Systems International, the United Nations Development Program, and Stanford's ChangeLabs have all put forth guidelines, tools, frameworks, and principles for ensuring the success of efforts to scale social and environmental innovations (UNDP Small Grants Programme, World Health Organization and ExpandNet, 2009; Management Systems International, 2012; Stanford ChangeLabs, unpublished).
We extend this literature first, by enumerating all of the factors that have been suggested by authors across disciplines to facilitate efforts to scale (“putative scaling principles”), and second, by elucidating those factors that are statistically associated with initiatives that have and have not successfully scaled, as measured by specific metrics that we evaluated using data extracted from case studies.
Methods
We extracted principles associated with scaling in the literature, scored cases with respect to scaling success, and then identified associations between scaling principles and success scores. We began with an extensive literature review, from which we compiled a comprehensive list of “putative scaling principles.” We then consolidated this list of principles, and organized them into roughly the order that they might be applied in an innovation or project scaling effort. Next, we returned to the literature to collect a set of case studies in which attempts were made to scale pilot projects. After categorizing these cases by the degree of scaling success reached, we recorded the presence, absence, and author-reported importance of each of the putative scaling principles in each case. Finally, we evaluated the resulting data through the application of Fisher's exact tests to determine which principles are statistically correlated with higher and lower degrees of scaling success. We also characterized the degree of auto-correlation between scaling principles using regression tree analysis.
Putative Principles for Scaling Success
We conducted a systematic literature review across a range of disciplines by searching Google and Google Scholar using combinations of one keyword from each of the following three groups: (1) “principles,” “factors,” “aspects,” “characteristics,” “framework,” or “how to;” 2) “success,” “successful,” “facilitate,” or “improve;” and 3) “scale,” “scaling,” “scaling up,” “scale up,” “scaling out,” “scale out,” or “to scale.” We included articles, books, and white papers that directly addressed the question of “how to facilitate scaling,” providing concrete advice and guidance directed toward individuals, companies, and/ or organizations seeking to spread innovations or expand operations. We excluded literature that discussed the need for scaling in a theoretical or academic manner. This resulted in a list of factors that various authors have posited as important or necessary for successfully scaling up and/or out from a pilot stage (Rogers, 1962, 2010; Rogers and Shoemaker, 1971; Granovetter, 1978; Kemp et al., 1998; Kern et al., 2001; Moore, 2002; Gershon, 2009; Rosenberg, 2011; World Health Organization ExpandNet, 2011; Management Systems International, 2012; Ardoin et al., 2013; Butler et al., 2013; Sutton and Rao, 2014, UNDP Small Grants Programme, Stanford ChangeLabs, unpublished). While some of these authors cited real-world examples of scaling success throughout their papers (Kern et al., 2001; Rosenberg, 2011; World Health Organization ExpandNet, 2011; Management Systems International, 2012; Ardoin et al., 2013 UNDP Small Grants Programme), the discussion of which factors were most important to ensure success were largely theoretical. Furthermore, we found no empirical evaluations of the association of these factors with scaling success.
The compiled list of 128 putative scaling factors was processed by eliminating redundancies, combining similar factors, and sorting factors into categories based on the part of the scaling process they are relevant for. The categories were then organized into a roughly sequential order that corresponds with the steps an organization might take when designing, implementing, and then scaling a new innovation. This processing resulted in a list of 69 putative “principles for scaling” that fall into 10 categories, which are listed with explanations below. The 69 Putative Principles, organized by category, can be found in Table 1.
Categories:
1. Innovation Design: Principles in this category describe aspects of the innovation itself (e.g., new management technique, policy, or regulatory instrument) that may facilitate scaling success.
2. Pilot Project and Pilot Site: Principles in this category describe attributes of the pilot project and pilot site that may significantly influence scaling success.
3. Resource Team: The “resource team” refers to the organization/group that brings the innovation to the new site(s) during the scaling process. Principles in this category describe resource team characteristics that may be important for scaling success.
4. User Organization: The “user organization” refers to the individuals being targeted with the innovation at each new site. Principles in this category describe user organization characteristics that may impact efforts to scale.
5. Overarching Environment: Principles in this category include large-scale, contextual factors that may ensure scaling efforts are sustainable.
6. Scaling Strategy: There are many different methods through which a project can be brought to scale. This category contains principles describing efforts to deliberately consider these options.
7. Funding: Principles in this category describe efforts to dedicate resources and capacity to scaling.
8. Project Design: Clearly aspects of project design will be important for ensuring project success in one site. Principles in this category, however, describe those aspects of project design that may be especially important for ensuring scaling success.
9. Marketing and Dissemination: Principles in this category describe explicit efforts to promote and encourage uptake of an innovation at new target sites.
10. Monitoring and Evaluation (M&E): Principles in this category refer to dedicated efforts to monitor and evaluate the progress of the scaling effort itself (not just the progress at each project site) so that problems can be identified and methods can be adjusted.
Definitions and sources for these 69 principles can be found in Supporting Information 6: PRISMA Checklist. We aimed to identify which of these putative scaling principles are associated with scaling success.
Evaluation and Scoring of Case Studies
To evaluate the role of the putative scaling principles in scaling success, we returned to the literature to collect case studies of project scaling efforts across a variety of disciplines. We did so by searching Google and Google Scholar using combinations of terms from the following two groups: (1) “scale,” “scaling,” “scaling up,” “scale up,” “scaling out,” “scale out,” or “to scale;” and (2) “case study,” “innovation,” “intervention,” “implementation,” “pilot,” or “pilot project.” We included cases where an innovation or behavior change intervention had been successfully implemented at a pilot scale. Included cases also had to contain enough information on the scaling effort to make conclusive determinations about the presence or absence of at least one of the putative principles, and to judge scaling success. Our focus was on cases of environmental protection and resource conservation, but given that these were quite limited, we also included many cases from the public health and international development sectors. We excluded cases that described corporate expansions or the passage and spread of regulations or policies, as these types of efforts, where “scaling” happens by fiat, powered by corporate or governmental authority, were deemed to be fundamentally different from attempts to spread an idea or innovation through appeal to autonomous groups or geographies, as is commonly the goal of environmental scaling efforts.
This search revealed 74 abstracts, of which four were duplicate cases (where different authors wrote about the same case study), and three had to be excluded because we could not access the full text articles or papers. In addition to this online search, 11 case studies were found through other methods, including conversations with colleagues and reports in the mass media (see Supporting Information 1: Stepwise Principles, Table 1 for all cases and sources). This resulted in a set of 56 case studies, which are listed with sources in Table 2 (see Supporting Information 1: Stepwise Principles, Table 1 for case study summaries). A flow chart that summarizes the number of case studies screened, assessed for eligibility, and included in the analysis, with reasons for exclusions, is presented as Figure 1.
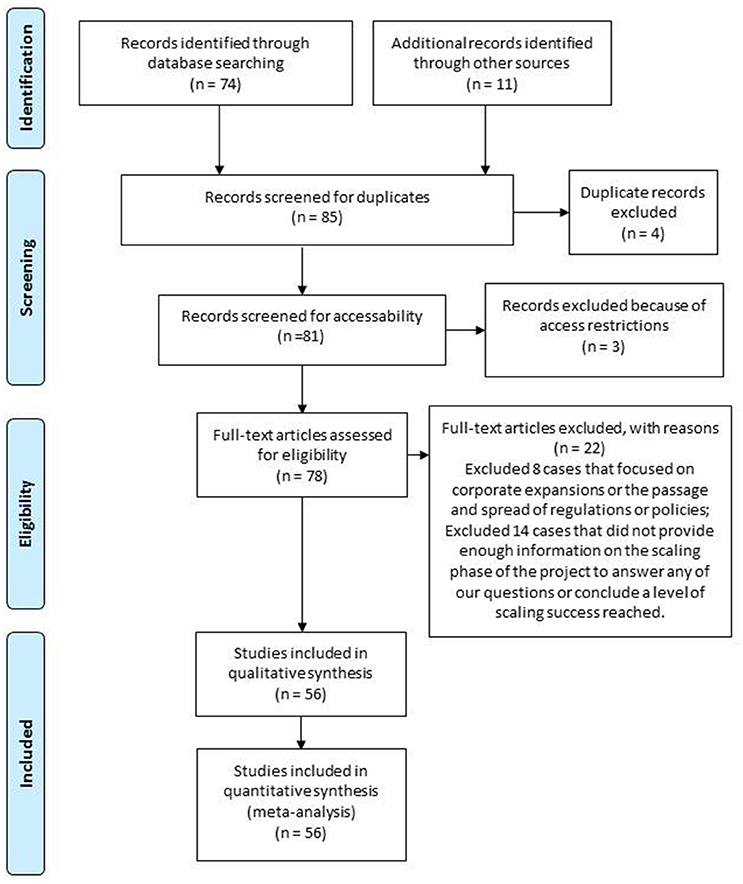
Figure 1. Preferred reporting items for systematic reviews and meta-analyses (PRISMA) flow chart (Moher et al., 2009) summarizing the number of case studies screened, assessed for eligibility, and included in the analysis, with reasons for exclusions (Supporting Information 5: Correlation Tests R Code).
We assigned each of these case studies a “success score” (Table 2). Here, success is defined in terms of the amount of instigating-organization (or “resource team”) involvement necessary to keep the scaling process/new project sites sustainable. Thus, our success scores, which ranged between 1 and 4, represented the following classifications of success: 1 = a successful pilot project that completely failed to catch on at additional sites; 2 = a successful pilot that was able to scale to any extent (to just a few new sites or to many), but that did not become self-replicating (such that it was still necessary for the resource team to be heavily involved at all new project sites); 3 = a successful pilot that was able to scale to any extent, and that became self-replicating, such that the resource team is no longer involved (outside of occasional support) in the selection and implementation of new project sites; and 4 = a successful pilot that was able to scale, became self-replicating, and is now the mainstream or predominant approach to solving the targeted problem in the targeted area, based on author statements (following the methods of Rogers (2010), where “adoption” of an innovation is defined as “full use of an innovation as the best course of action available”, p. 177). By focusing on the distinction between self-replicating and non-self-replicating projects we avoided the need to make subjective judgments about the degree to which one project had scaled in comparison to another project targeted to a different audience, over a different spatial area, and under completely different conditions.
We then recorded whether any of the 69 putative principles (Table 1) was “present” (noted as present by the author or authors of the case study materials), “absent” (explicitly noted to be absent by the author or authors), or “unknown” (not mentioned by the author or authors) in each case study. We chose this conservative method of scoring the presence or absence of principles because, we are, to our knowledge, the first to compile a comprehensive list of putative scaling principles from across disciplines. Thus, it is likely that many of the authors of the case studies we examined were not directly considering, and may even be unaware of, some or all of the factors on our list. Furthermore, there is a dearth of literature explicitly aimed at detailing project scaling efforts, and thus many of the case studies focus on the qualities and merits of a specific project or innovation, with information on the results of a scaling effort provided in limited detail. Therefore, it seemed unreasonable to assume that a principle was absent from a scaling case simply because it was not mentioned by an author; instead we recorded these instances as “unknowns.”
Robustness Scores
We also assumed that if an author does discuss the presence or absence of a specific factor, that factor was likely to have been especially relevant in that particular case. In other words, if an author not aware of the full list of scaling principles, or not explicitly writing about scaling, links a scaling principle to some degree of scaling success, we consider that principle to be more robust than principles that are not explicitly linked to scaling by authors.
Based on this assumption, we created a “robustness” scoring system, which we applied to each instance where a scaling factor was scored as “present” in a given case, in order to assess the relative importance of scaling principles. Our robustness scoring metric is as follows: 1 = the factor is present in the case study, but there is no evidence or reason to believe that it was especially relevant for the scaling effort; 2 = the factor is present in the case study, and the researcher scoring the case believes that it likely facilitated the scaling effort; 3 = the factor is present in the case study and the author of the case study explicitly states that it was important to the scaling effort; or 4 = the factor is present in the case study, the author states that it was important for scaling, and this assertion is supported by evidence (e.g., survey data that shows that members of the user organization found that factor especially compelling). These robustness scores can be thought of as an “index of inferred importance.” For every factor that received a “present” score, which was translated to a “1” for analysis, we also assigned a robustness score, and then used these values to weight the raw data during some of our analyses (see below). Factors that received “absent” scores (“0”s) or “unknown” scores (“NA”s) were not assigned robustness scores. This allowed us to evaluate the relative importance of each factor identified in the case study, according to the authors of the case studies and the researchers scoring them.
Analysis
Our literature review revealed that there is a strong publication bias toward scaling efforts that are at least marginally successful, and away from scaling failures. Out of 56 case studies evaluated, we found only one that could be classified as a complete scaling failure. We were thus unable to identify principles that are associated with “failure” or that can make the difference between success and failure. We can, however, evaluate the relationship between scaling principles and varying degrees of success.
We were also limited by a small sample size (n = 7) for the group of “fully successful” cases (those with success scores of “4,” indicating self-replicating scaling, and that the innovation has become mainstream). To overcome these small sample sizes, we created two larger groups, one containing all cases where the scaling effort has become self-replicating and a second containing all cases where intensive resource team involvement is still necessary to ensure project uptake at new sites. Thus, we grouped the cases with success scores of either “1” or “2” together into a “less successful” category (n = 27), and those cases that scored either a “3” or a “4” together into a “more successful” category (n = 29). We were then able to evaluate the number and type of principles in each of these two groups, and identify patterns that might explain why the more successful cases were able to become self-replicating and the less successful cases were not.
Fisher's Exact Tests
In order to determine if any of the 69 principles were significantly correlated with higher degrees of scaling success, we carried out two tailed Fisher's exact tests on each principle as it presented in the 56 case studies. We did this by creating 2 × 2 contingency tables using a nested design comparing the number of cases in the lower success group to the number in the higher success group (columns), and the sum of the “present” or “absent” scores for a given principle to the sum of all the other scores (both “absent” or “present,” respectively, and “unknown”) for that same principle (rows). The Fisher's exact test examines the null hypothesis that the proportion of cases falling into the two success groups is independent of the presence/absence scores. In other words, it determines the probability of finding the differences in success scores (numbers of cases in the less successful and more successful columns) between the cases with and without the given principle purely by chance. See Table 3 for an example.
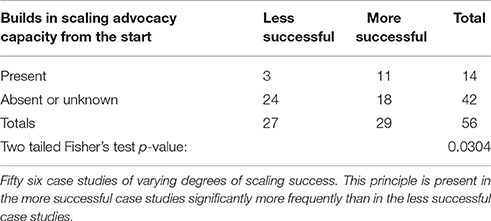
Table 3. Fisher's exact test contingency table for presence score frequency of “Builds in scaling advocacy capacity from the start”.
We chose the Fisher's exact test instead of the more common Chi Squared test because our small sample size (total n = 56, more successful n = 29, less successful n = 27) resulted in many of the values in the test contingency table cells falling below 10, and some falling below 5 (as in the example above). With small sample sizes the Fisher's exact test is more appropriate (McDonald, 2014).
We completed four main sets of Fisher's exact test analyses in order to examine the different relationships between four case study groups. We first examined the relationship between the less successful (success scores of 1 or 2; n = 27) and the more successful (success scores of 3 or 4; n = 29) groups of cases. Next, we compared the less successful cases with the “mostly successful” cases (cases that achieved self-replication, but where the innovation had not (yet) become the predominant solution to the given problem; success = 3; n = 22). Third, we compared the mostly successful cases with the cases where scaling efforts were “fully successful” (success = 4; n = 7). Finally, we compared the less successful cases with the fully successful cases. The results of these latter two analyses must be considered carefully given the small sample size of the fully successful group. See Supporting Information 1: Stepwise Principles for full analysis.
Within these four sets of analyses, we examined the principle frequency data in three different ways. We first assessed the un-weighted presence score frequencies of each principle to determine which principles are significantly associated with higher degrees of success given the basic “presence/absence” data we collected (Table 3). Next, we assessed these same presence frequencies, but weighted by our robustness scores to indicate relative importance. Because the robustness weights alter the frequency sums, the marginal totals in our contingency tables no longer reflect the total number of cases in each group. These weights were only applied to the “present” scores, not to the “absent” scores or the “unknowns” (which were still summed). See Table 4 for an example.
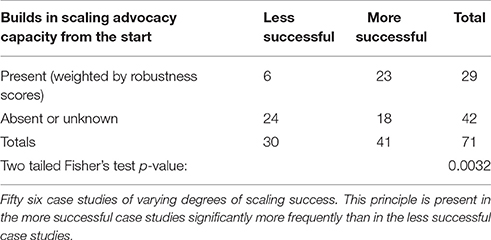
Table 4. Fisher's exact test contingency table for presence score frequency of “Builds in scaling advocacy capacity from the start,” with presence scores weighted by robustness values.
Finally, we assessed the absence score frequencies, identifying principles for which absence is significantly associated with lower degrees of success. To do this we again applied two-tailed Fisher's exact tests to each principle, this time comparing the frequency “absent” scores with the frequency of “present or unknown” scores for each principle in each of the groups of case studies. Cases in which authors noted that one of the principles was absent were considerably less common than instances where authors noted the presence of a principle. The frequencies evaluated below are thus very small, and all results should be interpreted with caution. See Table 5 for an example.
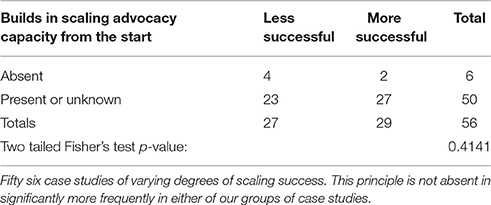
Table 5. Fisher's exact test contingency table for absence score frequency of “Builds in scaling advocacy capacity from the start”.
For ease of comprehension, we have created a figure to graphically represent all 12 of the assessments we conducted (Figure 2), and assigned each one a number and letter (see Box 1) which will be referenced throughout the results section.
Box 1 Analysis code key.
First set of analyses: Examining the relationship of the more successful (success scores of 3 or 4) cases to the less successful cases (success scores of 1 or 2).
1a – Unweighted principle presence frequency in more successful case studies compared with presence frequency in less successful case studies.
1b – Principle presence frequency in more successful case studies compared with presence frequency in less successful case studies; Presence scores weighted by robustness to indicate relative importance.
1c – Principle absence frequency in less successful case studies compared with absence frequency in more successful case studies. (Significance signifies that absence of given principle is harmful.)
Second set of analyses: Examining the relationship of the “mostly” successful cases (success scores of 3) to the less successful cases (success scores of 1 or 2).
2a – Unweighted principle presence frequency in mostly successful case studies compared with presence frequency in less successful case studies.
2b – Principle presence frequency in mostly successful case studies compared with presence frequency in less successful case studies; Presence scores weighted by robustness to indicate relative importance.
2c – Principle absence frequency in less successful case studies compared with absence frequency in mostly successful case studies. (Significance signifies that absence of given principle is harmful.) *No significant principles found.*
Third set of analyses: Examining the relationship of the fully successful cases (success scores of 4) to the mostly successful cases (success scores of 3).
3a – Unweighted principle presence frequency in fully successful case studies compared with presence frequency in mostly successful case studies.
3b – Principle presence frequency in fully successful case studies compared with presence frequency in mostly successful case studies; Presence scores weighted by robustness to indicate relative importance.
3c – Principle absence frequency in mostly successful case studies compared with absence frequency in fully successful case studies. (Significance signifies that absence of given principle is harmful.)
Fourth set of analyses: Examining the relationship of the fully successful cases (success scores of 4) to the less successful cases (success scores of 1 or 2).
4a – Unweighted principle presence frequency in fully successful case studies compared with presence frequency in less successful case studies.
4b – Principle presence frequency in fully successful case studies compared with presence frequency in less successful case studies; Presence scores weighted by robustness to indicate relative importance.
4c – Principle absence frequency in less successful case studies compared with absence frequency in mostly successful case studies. (Significance signifies that absence of given principle is harmful.) *No significant principles found.*
Regression Tree Analysis of Interactions and Surrogates
Fisher's exact tests indicate which individual principles are most associated with different degrees of scaling success. However, it is likely that the 69 principles examined here interact with each other, and that these interactions may also impact scaling success. We wanted to examine our data for two types of potential interactions: (1) synergies i.e., whether any of the factors interact with each other such that they must occur together to increase the likelihood of success; and (2) surrogates, i.e., whether any of the factors affect the data in the same way as other factors, such that they can in essence “stand in” or act as alternates for each other, implying a project might only need to focus on ensuring the presence of one or the other to improve the chances of success.
Because of our limited sample size (56 cases), and because there were more principles than cases (69 vs. 56, respectively), we were limited in the types of analyses we could conduct to identify correlations. Specifically, we could not apply a standard multivariate regression or a principal component analysis because our data set violates the assumptions of these methods (Osborne and Costello, 2004). Instead, we applied a regression tree analysis, which is suitable for small sample sizes, and which can help to answer both of the above questions (see Supporting Information 2: Case Studies and Analyses for R code). We selected a precautionary “minsplit” value of 10, which directs the code not to create a split in the tree unless at least 10 of the 56 cases contain the given principle. This minsplit value is one quarter of the maximum frequency across our principles (40), and just over half of the average and median frequencies (18.9 and 19, respectively). We also “pruned” our tree to minimize the relative error returned in an effort to avoid overfitting the tree (Kabacoff, 2014). This pruning process did not change the regression tree returned by the original code.
The regression tree analysis as coded in R identifies which principles explain the largest amount of variance in success scores. It also explores the data to determine whether any of the principles can act as “surrogates” for each other. A perfect surrogate is one that would split the data in exactly the same way as the primary factor in the regression tree analysis. In other words, surrogate factors can be thought of as potential substitutes for each other, with their presence and absence resulting in roughly the same impact on scaling success. If the model determines that any principles are sufficient surrogates for each other, it will consider them together when determining where to create splits or “branches” in the tree.
We applied the regression tree analysis only to our unweighted data set, as the robustness weights are an ex post facto construct designed to capture additional information about the relative importance of each factor to a given case study, and not an inherent quality of the principles themselves, or their relationships to each other. In other words, the robustness weights capture how important a given case study author thought a factor was to that case. This information could be seen as a confound in the regression tree analysis, which determines, based on presence/ absence data, which factors explain the largest amount of variance in case study success scores. Finally, we used the results of the regression tree to guide application of a series of Mann-Whitney-Wilcoxon tests (appropriate for data sets with non-normal distributions) to explore whether the principle groupings identified by the tree are statistically significantly associated with higher or lower success scores. This analysis did not reveal any significant results, and is thus discussed only in Supporting Information 3: Non-Statistical Analyses.
Results
Fisher's Exact Results
The significant results of the twelve Fisher's exact tests we completed are presented in the two tables below (Tables 6, 7). The full results from each of our analyses (both significant and non-significant) can be found in Supporting Information 3: Non-Statistical Analyses.
In the tables below, we organize the principles that were identified as significant in any of these 12 analyses into two groups: (1) those principles which were found to be significantly more frequent in at least one analysis in the cases in which interventions became self-replicating, suggesting that they may contribute to scaling success as we have defined it (23 principles; Table 6), and (2) principles which were found to be significantly more frequent in the cases with lower success scores in at least one analysis (19 principles; Table 7). P-values in regular typeface, are significant at the 0.05 level, while p-values in bold typeface, are significant at the 0.01 level.
Table 6 shows those principles which are significantly associated with the more successful case studies in at least one of our 12 Fisher's exact test analyses. P-values in regular typeface, are significant at the 0.05 level, while p-values in bold typeface, are significant at the 0.01 level. Columns 1a through 2b contain significant p-values from Fisher's exact test analyses comparing our more successful (success = 3 or 4; n = 29) group of case studies to our less successful (success = 1 or 2; n = 27) group. Columns 3a through 4b contain significant p-values from Fisher's exact tests comparing cases in the mostly successful (success = 3; n = 22) group to cases in the fully successful (success = 4; n = 7) group. See Box 1 for analysis code key.
Table 7 shows those principles which are significantly associated with the less successful case studies in at least one of our 12 analyses, and that are thus not sufficient to ensure scaling efforts become self-replicating. P-values in regular typeface, are significant at the 0.05 level, while p-values in bold typeface, are significant at the 0.01 level. Columns 1a through 2b contain significant p-values from Fisher's exact test analyses comparing our more successful (success = 3 or 4; n = 29) group of case studies to our less successful (success = 1 or 2; n = 27) group. Columns 3a through 4b contain significant p-values from Fisher's exact tests comparing cases in the mostly successful (success = 3; n = 22) group to cases in the fully successful (success = 4; n = 7) group. See Box 1 for analysis code key.
Examination of these two tables reveals significant principles from all ten of the sequential scaling process categories (Innovation Design, Pilot Project and Site, Resource Team, User Organization, Overarching Environment, Scaling Strategy, Funding, Project Design, Marketing and Dissemination, Monitoring and Evaluation). Analyses 1a, 1b, and 1c revealed 14 principles to be statistically significantly correlated with higher degrees of scaling success (cases in the more successful group), and three principles to be statistically significantly correlated with lower degrees of scaling success (cases in the less successful group). Analyses 2a and 2b revealed 14 principles to be statistically significantly more frequent in the “mostly” successful cases, or those which have achieved self-replication, but have not yet become mainstream in the given area, and five principles to be statistically significantly correlated with cases in the less successful group. Analyses 3a, 3b, and 3c revealed eight principles to appear statistically significantly more frequently in cases in the “fully successful” group—where the given innovation has become mainstream, or the predominant solution for addressing the given problem, and six principles to be statistically significantly more frequent in the mostly successful cases, which have achieved self-replication, but not become mainstream solutions. Finally, analyses 4a and 4b revealed just three principles that appear significantly more frequently in the fully successful cases, and ten principles that appear more frequently in the less successful cases to a significant degree. This disparity is likely due to the very small number of cases in the fully successful group as compared with the much larger number in the less successful group. Analyses 2c and 4c, both of which explored principle absence scores, did not reveal any statistically significant results. This may be due to the relatively small number of instances of principle absence recorded across the case studies.
There is a significant amount of overlap between cases revealed to be statistically significant in the ten analyses discussed above, resulting in a combined list of 23 principles that appear to be correlated with higher degrees of scaling success, and 19 principles that appear to be correlated with lower degrees of success. The assessments of principle presence when not weighted by robustness scores (analyses 1a, 2a, 3a, and 4a) revealed notably fewer statistically significant principles than did the analyses of presence weighted by robustness (analyses 1b, 2b, 3b, and 4b). Analyses examining absence of principles (1c, 2c, 3c, and 4c) revealed the fewest statistically significant principles of all of our analyses.
Regression Tree Results
Application of the regression tree analysis in R revealed the following results (Figure 3; see Supporting Information 3: Non-Statistical Analyses for full results and Supporting Information 2: Case Studies and Analyses for R code):
Our regression tree analysis revealed surrogate factors (potential substitutes) for only the first node in our tree: the provision of long-term support to users. This factor has three surrogates:
• Project Design Turns Users Into Partners (75% agreeance with long-term support)
• User Organization has a Wide Reach (65% agreeance with long-term support)
• Project Design Empowers People with Necessary Skills (which also has a 65% agreeance with long-term support)
The regression tree R code replaces missing values (in our case, the instances where the presence or absence of a principle in a given case study was “unknown”) with corresponding values from that factor's surrogate(s) (Chambers and Hastie, 1992; Therneau et al., 1997). Thus, if principles A and B are surrogates for each other, and principle A is determined to explain a large enough proportion of the success score variance to create a split in the tree, the model will include cases that contain either principle A or principle B in the branch of the tree assigned to the presence of principle A.
Thus, in the first split shown in Figure 3 above, we can infer that the 10 cases fall on the left side branch are cases where neither the provision of long-term support nor any of its surrogates are present. The 46 cases that fall on the right side branch are cases where this first principle or any of its surrogates are present, and it is these 46 cases that become the pool of cases on which the rest of the tree is based.
Examination of these results reveals that the single factor that explains the largest amount of variance in success scores among our 56 cases is the provision of long-term support to users of the innovation. Cases where this factor (along with all of its surrogates) is absent have a mean success score of only 2.2, indicating that these projects did not, on average, become self-replicating. Within those cases that do include either the provision of long-term support, a project design that turns users into partners or empowers people with the necessary skills, or a user organization with a wide reach, however, mobilizing project resources from both within and outside the system (second level of the tree) becomes the factor that explains the largest percent of success score variance. Among cases that have long-term support systems (or one of its surrogates), those that do not mobilize resources from within and outside the system still only receive average success scores of 2 (not self-replicating), while cases that have both of these factors receive higher average success scores (2.8), indicating that many do become self-replicating. Together these two factors explain roughly 17.5% of success score variance. The third important factor identified through the regression tree analysis is the capacity of the “user organization” (or the group of individuals in the new site being targeted with the new innovation) to implement the project or take up the innovation. Cases that have all three factors receive higher success scores (2.86) than those that have the first two, but not this third factor (2.25).
After these first three splits, the regression tree results become more surprising. The next two factors that are identified as important for explaining success score variance (innovations that are “platforms” (meaning they have the potential to solve multiple problems), and innovations that provide rapid feedback to users) are inversely related to higher degrees of success, such that their absence appears to be associated with greater scaling success. Within cases that have the first three factors (long-term support (or surrogate), funding from within and outside the system, and a user organization with sufficient innovation capacity), those for which the innovation is not a platform receive the highest average success scores in our whole tree (3.67), indicating that most become self-replicating and some go on to achieve full scaling success (as we have defined it). However, those cases that do have innovations that serve as platforms (along with the first three factors in the tree) still receive relatively high average success scores (2.79), signifying that most become self-replicating. Similarly, the fifth level in our regression tree reveals that within cases that have the preceding four factors, the absence of an innovation provides rapid feedback is associated with higher degrees of success (3.33), but the 28 cases where this factor is present (along with the preceding four) still receive average success scores in the range that indicates most become self-replicating (2.67). Inclusion of these five factors in the model (the provision of long-term support, mobilization of resources from within and outside the system, user organization capacity, innovations that are platforms, and innovations that provide rapid feedback) together accounts for approximately 37% of success score variance.
The final factor revealed as important for explaining success score variance by the regression tree analysis is whether or not the pilot site represents realistic conditions relative to those that can be expected at future target sites. Among cases that have all five of the factors discussed above, this sixth factor may determine whether or not the innovation will become self-replicating or not. For cases that provide long-term support to users, mobilize resources from within and outside the system, ensure the user organization has sufficient capacity, and where the innovation is a platform solution that provides rapid feedback, ensuring the pilot site is representative of future sites results in an average success score of 2.72 (most became self-replicating). Failing to do so results in an average score of 2.3 (not self-replicating). Inclusion of this sixth factor in the grouping brings the explanatory power of the model up to 38%.
Discussion and Caveats
Overarching Lessons
The results of the empirical analyses of case studies conducted here suggest three lessons that should be considered when planning a project that is intended to scale. First, scaling is not an isolated event that begins at the end of the pilot phase. Principles from all ten components of a typical scaling trajectory (see above, Putative Scaling Principles section) were revealed as important for scaling success in at least one of our analyses (Table 6), which implies that successful scaling requires consideration of the scaling implications of every component, and at each step, of the project. This stands in contrast to much of the literature on scaling, in which only one, or just a few of the stages of project design and implementation are considered [e.g., aspects of the innovation itself or features of the “target audience” or “user group” Rogers, 1962; Granovetter, 1978; Moore, 2002; Butler et al., 2013; internal organizational characteristics Sutton and Rao, 2014; or external systemic features (Kern et al., 2001)].
The strong association of principles related to innovation design (innovations designed to be congruent with local sociocultural patterns, to integrate aspects of existing scaled infrastructure, and facilitate a paradigm shift), and characteristics of the resource team (unifying vision, members who were part of the innovation design, and members who are part of the user organization), the user organization (user organization takes ownership), and the pilot project (built-in scaling advocacy capacity and consensus on scaling expectations) and pilot site (realistic conditions relative to future sites) with scaling success suggest that these early-stage factors can be very important for scaling. This finding is consistent with conclusions in several other reports and publications (Gershon, 2009; World Health Organization ExpandNet, 2011; Management Systems International, 2012; Sutton and Rao, 2014; Stanford ChangeLabs, unpublished).
Likewise, the fact that greater scaling success is associated with certain aspects of project design and implementation (empowering users with necessary skills, providing long term support to users, and taking advantage of economies of scale) and fundraising (mobilizing resources from within and outside the system, and fundraising for scaling from the very start of the project) highlight the value of considering scaling goals throughout the life span of the project. Successful projects that train users to be able to implement innovations on their own, and that also provide long-term support systems to ensure users do not revert to old behaviors when problems arise, tend to reach higher degrees of scaling success (Moore, 2002; Rogers, 2010; World Health Organization ExpandNet, 2011; Butler et al., 2013; UNDP Small Grants Programme). Identifying and utilizing opportunities for economies of scale can make this process smoother, faster, and more affordable (World Health Organization ExpandNet, 2011; Management Systems International, 2012). However, bringing a project to scale can be expensive, both in terms of financial and human capital, and the failure to adequately provide resources for scaling starting from an early phase in the project can hinder scaling success. Projects that include scaling goals in their fundraising efforts and seek support from multiple sources, both within and outside of project systems, are more likely to achieve better outcomes (Gershon, 2009; World Health Organization ExpandNet, 2011; Management Systems International, 2012; UNDP Small Grants Programme).
The second overarching lesson revealed by this analysis is that there are significant benefits associated with active management of external factors (managing supply and demand, building a constituency, and understanding ongoing environmental dynamics), indicating that successful scaling may require direct manipulation of the surrounding context and active removal of barriers. For example, if the given innovation creates excess supply, working to generate additional demand can improve scaling success by preventing market fluctuations from halting growth or spread. The reverse relationship is also true—if a new innovation or idea increases demand for something, working with producers to generate or increase supply can remove an important barrier to scaling (Stanford ChangeLabs, unpublished). Developing an understanding of the systemic and environmental influences that could be facilitating or hindering scaling efforts may allow managers to take advantage of opportunities when they arise and to avoid problems that may undermine success (World Health Organization ExpandNet, 2011). Building a constituency of supporters, both within and outside the system, may improve uptake of the innovation and increase capacity to manage barriers (Gershon, 2009; World Health Organization ExpandNet, 2011; Management Systems International, 2012; UNDP Small Grants Programme).
Finally, we found that principles pertaining to the careful consideration of a scaling strategy (evaluation of trade-offs between duplication and customization and conscious decisions about scaling pace), marketing and dissemination efforts (marketing the innovation to niche markets with targeted, customized messages), and of the evaluation of the progress of the scaling effort itself (as opposed to monitoring and evaluation of the impact of the innovation alone) are associated with scaling success. This indicates that taking a project to scale requires paying explicit and targeted attention to the scaling strategy, and cannot be expected to develop naturally or automatically from a successful pilot project. Organizations that make and follow through on conscious decisions about the mechanisms and methods through which they want to achieve scale (e.g., choosing between exact replication and customization, deciding on a target scaling pace) appear to enjoy greater scaling success (Kern et al., 2001; Rogers, 2010; World Health Organization ExpandNet, 2011; Management Systems International, 2012; Ardoin et al., 2013; Sutton and Rao, 2014). Organizations that engage in efforts to understand and create targeted marketing materials for the different groups who they hope will adopt their methods are more likely to see their innovations become mainstream (Kemp et al., 1998; Moore, 2002; Rogers, 2010; World Health Organization ExpandNet, 2011; Management Systems International, 2012; Ardoin et al., 2013; Butler et al., 2013). Organizations that monitor and evaluate the progress of their scaling efforts based on indicators that have been agreed upon ahead of time (e.g., the extent to which essential features of the innovation are being implemented (e.g., training, management, facility construction); the extent of community participation in and support for the innovation; the number of sites implementing the innovation) are able to adapt their scaling efforts as necessary, and these efforts result in higher degrees of success (Gershon, 2009; World Health Organization ExpandNet, 2011; Management Systems International, 2012).
Table 7 above shows those principles which are significantly associated with the less successful case studies in at least one of the twelve Fisher's exact test analyses. Because we believe there is a strong bias against publishing accounts of failure, our lower success cases cannot be considered “scaling failures,” and thus the principles in this latter group are not necessarily associated with failure; rather, they are associated with lower degrees of success. Although the principles in this latter group may be necessary for some degree of scaling success, they do not appear to be sufficient for self-replication. It is also worth noting here that the difference between a “mostly” successful case and a “fully” successful case may be entirely dependent on the moment in time when the case study was written. This temporal dependence of our case study success scores is an important caveat that should be weighed when interpreting the results of this analysis. Because of the nature of these case studies, which chronicle efforts to implement an innovation and then to scale that innovation out and/ or up, most of the reports on the progress or status are necessarily only snapshots in time, as opposed to official “end points.” We therefore cannot be sure that any given case in the less successful group will not eventually become self-replicating, or (perhaps more likely) that any of the innovations in the mostly successful group will not eventually go on to become mainstream in their respective regions if given enough time. These changes could conceivably move in the other direction as well, although it may be less likely for a project that's become self-replicating to revert back to a state where significant resource organization intervention is needed for it to persist. It would be interesting to reexamine these same fifty six case studies at some future date to explore if the degrees of success achieved, as well as the scaling principles present, have changed.
Another important lesson can be gleaned from examination of the principles in Table 7, above: Following many of the accepted tenants of intervention design, i.e., creating an innovation that is credible, testable, and provides rapid feedback (Rogers, 1962; Moore, 2002; World Health Organization and ExpandNet, 2009) or focusing on local leadership (Rogers and Shoemaker, 1971; World Health Organization and ExpandNet, 2009), may not be sufficient to ensure scaling success. It is also interesting that a number of principles that were identified as beneficial for scaling success (Table 6) are also significantly associated with the less successful case studies (Table 7). This may be due to the fact that the statistical analysis that was applied—the Fisher's exact tests—explore each principle in isolation, which allows different relationships to be revealed for the same principle when examined through different lenses (e.g., comparing the less successful cases with the more successful cases vs. comparing the mostly successful cases with the fully successful cases). This implies that there may be relationships between these principles and other system factors not evaluated here that are also important for scaling success. A single principle may be beneficial in one case, but not a powerful enough driver of scaling to overcome barriers to scaling in another case.
Factor Correlations
The results of our regression tree analysis indicate that some of the 69 principles interact with each other in ways that may impact success. Specifically, we identified a group of nine principles that together explain nearly 40% of the variance in success scores among our cases. The first four of these are a group of surrogates that affect the data in roughly the same way as each other:
• The provision of long term support to users (identified as the single most important principle for explaining success score variance).
• Project design that turns users into partners.
• User organization with a wide reach.
• Project designed to empower people with necessary skills.
These principles can essentially be thought of as alternates, implying a project might only need to focus on ensuring the presence of one of these factors to improve the chances of success.
The regression tree revealed five other principles that are associated with scaling success in the cases we examined:
• Mobilization of resources from within and outside the system.
• User organization that has the necessary capacity to implement the innovation.
• Innovation is a platform to solve multiple problems.
• Innovation provides rapid feedback.
• Pilot site has realistic conditions relative to future sites.
The inclusion of these principles (one of the first four, plus the latter five) explains nearly 40% of the variance in success scores among our 56 cases.
Furthermore, cases that contain one of the first four principles, plus the remaining five achieve higher average success scores (scores that are closer to 3—self-replicating, than to 2—not self-replicating) than cases that do not contain this configuration of factors. The presence of the principles included in the first three levels of the regression tree (provision of long-term support or one of its surrogates (resources mobilized from within and outside the system, and user organization capacity) progressively increases the explanatory power of the model and average scaling success scores increase as each of these principles are included. Cases that have all three of these principles receive an average success score of 2.86, while those that do not contain all three have lower success scores on average (2.25).
Within cases that have the principles included in the first three levels of the regression tree, the analysis indicates that the absence of the fourth and fifth principles (innovations that are platforms to solve multiple problems and that provide rapid feedback) could be key to moving from a “mostly successful” case—where the innovation has become self-replicating—to a “fully successful” case—where the innovation has become the predominant solution to the targeted problem. These unexpected results must be considered cautiously, given the very small number of cases fitting these exact criteria (having long-term support systems (or one of its surrogates), resources from within and outside the system, and a user organization with sufficient capacity, but with an innovation that is not a platform and does not provide rapid feedback).
The final branch in our regression tree analysis indicates that cases that include the principles indicated by the first three levels of the tree, but where the innovation is a platform solution and does provide rapid feedback, can still achieve high success scores associated with self-replication. For these cases, the key principle in explaining success score variance becomes the selection of a pilot site that is realistic relative to future sites. Without this final factor, average success scores are only slightly higher than those cases that do not have the provision of long-term support or one of its surrogates (average scores are 2.3 and 2.2, respectively). Thus, we can conclude that these nine factors identified by the regression tree analysis interact with each other in important ways that influence the degree of scaling success that may be achieved.
This finding supports the conclusions drawn from our Fisher's exact tests: scaling must be considered at all project phases, from efforts to secure project funding, through pilot site and user organization selection, to the provision of long-term support systems. It is not difficult to understand how a project with ample funding to scale an innovation that is a platform solution to multiple problems and provides rapid feedback, that is sited and targeted to maximize current and future user uptake, and that includes the provision of long-term user support systems to ensure that challenges that arise post-pilot do not undermine scaling success would become self-replicating.
Why Were Some Principles Not as Important?
The statistically significant principles discussed above are only a small subset of the full list of principles cited in the literature as important for scaling up (Table 1; Supporting Information 6: PRISMA Checklist). There are a variety of reasons why the rest of the principles on this list were not significantly associated with successful scaling in our analyses. First, the small sample size limits the ability of statistical tests to detect differences in the distribution of principles in the case studies. While we chose statistical tests designed for small sample sizes (the Fisher's exact test, regression tree, and Mann-Whitney-Wilcoxon test), there is no denying that an increased number of cases in each of our four success categories would increase the robustness of this analysis. This is an important caveat to our results. Second, many items have very high frequencies in both the less successful and the more successful groups of case studies (see Supporting Information 4: Full Results, Figures S3, S4), hence, while these principles are not statistically correlated with greater success, this does not mean that they are not important for scaling. They may be necessary, but not sufficient to ensure scaling success. In the below list of principles that occur in at least half of the more successful cases, all items in bold also occur in at least half of the less successful cases:
• Mobilize Resources from Within and Outside the system.
• Innovation has Relative Advantage Over Other Options.
• Chose a Method for Achieving Scale (Expansion, Replication, or Collaboration).
• Chose a Type of Scaling (Horizontally or Vertically).
• Scaled based on Lessons from the Pilot.
• Innovation Solves Multiple Problems (a Platform, not just a Solution).
• User Organization has Innovation Capacity.
• Project Design Empowers People with Necessary Skills.
• Project is Designed to have Clear (Ideally Quantifiable) Results.
• Evaluate Scaling Progress using Agreed Upon Indicators.
• Innovation is Relevant (Initial demand exists).
• Innovation Alignment with local policy and program priorities.
• Resource Team has Necessary Skills.
• User Organization takes Ownership of the Innovation and Process.
• Project Design Makes Users into Partners.
• User Organization has Innovation Demand.
• User Organization has Wide Reach.
Another possible reason why some principles from the initial list are not significantly associated with successful scaling is that authors may simply be failing to write about these factors. As previously mentioned, instances where authors directly discuss the scaling process and results in case studies are scarce. Some factors which are important for scaling may therefore be omitted from case study write-ups simply because the scaling process is not discussed in enough detail, or because authors are unaware that they may be of interest. We suspect this is the case for principles such as “Design for process learning” (Table 1; Supporting Information 6: PRISMA Checklist), which is likely both important and ubiquitous in scaling efforts.
Furthermore, the limited accounts of scaling efforts that do get published are inconsistent as to which principles are included. Some principles, especially those that were introduced into the theory many decades ago and are more well-known, receive much more attention than others by authors across all case studies, regardless of the degree of success reached. For example, the relative advantage of the given innovation over the other existing options is frequently a focal topic of authors describing implementation and scaling efforts. Other principles, such as the identification and targeting of “niche markets” or the use of multiple channels to tell compelling stories when promoting a new innovation, were only mentioned in a few of our fifty six case studies. This disparity skews the data toward “front-end” principles that pertain to aspects of innovation or project design and user organization selection, which have a longer history of discussion in the literature.
Some items that did not occur with statistically significant frequency in any of our cases may actually be missing from many project scaling efforts because project managers are unaware of their importance. This possibility implies that all scaling efforts could potentially be improved through incorporation of additional principles. We suspect that this might be the case for principles such as “Marketing and dissemination efforts leverage the principles of behavior change,” which are based on relatively new research (i.e., Butler et al., 2013; Stanford ChangeLabs, unpublished), and may not have been incorporated into project design processes on a large scale yet. We hope that our analysis will serve to highlight some of these factors, and potentially increase their use in future scaling efforts.
Finally, some of the principles which authors have theorized will facilitate scaling efforts may simply not be relevant for scaling success. Because no rigorous (e.g., before-after/control-impact) empirical evaluations of the factors that are important for scaling have been conducted, many authors have extrapolated from individual successful scaling cases, resulting in the identification of factors thought to be important for scaling which may not actually be the case. Furthermore, there has been considerably more exploration into factors that will facilitate the spread of an innovation within a pilot community, or to a target audience (e.g., Granovetter, 1978; Moore, 2002; Rogers, 2010; Butler et al., 2013), than into factors that will facilitate spread between sites or communities. It could be that some of the factors which have proven useful in spreading an innovation from person to person are not actually that useful for passing an innovation from one community to another. This could explain the low number of principles derived from the literature on spreading innovations between individuals that are significantly associated with scaling success.
Conclusion
This empirical analysis of the association of a variety of factors with scaling success suggests that to be successful, scaling efforts must be deliberate. Our results suggest that while the design of the intervention is important, a comprehensive strategy for scaling that includes pilot project design, pilot project siting, a resource team devoted to scaling, funding for scaling, marketing and dissemination tactics, careful monitoring and evaluation of scaling progress, and the provision of long-term support to users may be equally important. Our results reveal a number of opportunities to improve scaling outcomes at various times throughout the lifespan of the project. The innovation itself can be designed to take advantage of existing scaled infrastructure and to mesh well with local sociocultural patterns, and if possible, to motivate an entirely new paradigm, or way of addressing a given problem. The resource team and user organization can be carefully selected with a focus on each member's role in the scaling process and on each group's attitudes and vision. Pilot sites can be selected that have realistic conditions that reflect those likely to be encountered at future sites, and pilot projects can be designed with scaling in mind, so that useful lessons can be extracted and implemented in future sites. Agreeing on the scope, pace, and strategy of the hopeful scaling effort at the outset, and by all members of the project team, is likely to lead to greater success. External and internal system factors can be monitored and managed to create conditions that are conducive to scaling, fundraising efforts can include scaling goals, and active efforts to “market” the new intervention to the targeted audiences can be carried out. Furthermore, projects that consider the long-term needs of their users, not just at pilot sites, but at all future potential sites, building in support systems to ensure project components do not deteriorate over time will probably see better scaling outcomes. Finally, efforts to monitor and evaluate the progress of the scaling effort itself (not just the innovation impacts) can be implemented in order to identify gaps or places where progress seems slow and can be targeted with reforms.
In addition, our analysis of correlations between principles revealed that cases that include a certain set of principles achieve somewhat higher degrees of success than cases that do not. This principle set consists of: the provision of long-term support systems for users (or one of its surrogates: a project design that turns users into partners, a user organization with a wide reach, and a project designed to empower people with necessary skills); resources mobilized from within and outside the system; user organizations that have the capacity to implement the new innovation; innovations that are platform solutions to multiple problems and that provide rapid feedback; and pilot sites that have realistic conditions relative to future sites.
This study also highlights the value of empirical analysis for informing decision making. Many of principles that authors from various disciplines have cited as beneficial to project scaling for decades are not significantly associated with scaling success in the case studies we examined. These items, such as “user organization has strong local leadership,” and “user organization has wide reach” are generally accepted as being important for scaling and sometimes serve as criteria for designing projects. However, our research indicates that cases with these factors in place will not necessarily reach high degrees of scaling success. These science-based results can help to focus and guide management decisions and improve scaling outcomes for projects at all stages.
Areas for Future Research
The most obvious extension of this research would be to conduct a similar set of analyses with a much larger set of cases, and preferably with cases wherein more of the 69 putative principles are discussed. In addition, an in-depth evaluation of the presence or absence of each of these principles in individual case studies would reduce the uncertainty inherent in any meta-analysis of cases by elucidating how various factors influence scaling success, and could potentially provide lessons for managers on how to ensure the presence of a given principle in their own project design. Finally, an evaluation of the principles that are beneficial to efforts to scale projects explicitly in the environmental sector would be helpful in determining which principles are generalizable across sectors, and which are not.
Author Contributions
WB conducted the initial literature review that resulted in the extensive list of putative scaling “principles,” which were then assessed against case studies of varying degrees of scaling success. She also assisted with this case study review phase of the research, and conducted all statistical analyses on the resulting data. AT and CW also assisted with case study analysis and extraction of data. RF assisted first with the theoretical framing of the research question, and later with the interpretation of the results of the statistical analyses. Finally, WB drafted the manuscript, which was then reviewed and edited by all authors. All authors have reviewed this final version of the manuscript and are in support of manuscript submission at this time.
Conflict of Interest Statement
The authors declare that the research was conducted in the absence of any commercial or financial relationships that could be construed as a potential conflict of interest.
Acknowledgments
The authors would like to express their gratitude to Banny Banerjee, Jason Bade, and Theo Gibbs of Stanford University as well as Nya Van Leuvan of Root Solutions for their assistance with the compilation of the putative scaling principles. We would also like to thank Doctors Kendra Karr, Ryan P. Kelly, and Allison Horst as well as Mr. Kenneth Qin for their assistance with the statistical analyses and interpretation of results. Finally, we are grateful to the Walton Family Foundation and the Gordon and Betty Moore Foundation for providing funding to the Environmental Defense Fund that made this research possible. The views expressed in this publication are solely those of the authors and have not been reviewed by the funding sources or affiliated institutions.
Supplementary Material
The Supplementary Material for this article can be found online at: http://journal.frontiersin.org/article/10.3389/fmars.2016.00278/full#supplementary-material
References
Airamé, S., Dugan, J. E., Lafferty, K. D., Leslie, H., McArdle, D. A., and Warner, R. R. (2003). Applying ecological criteria to marine reserve design: a case study from the California Channel Islands. Ecol. Appl. 13, 170–184. doi: 10.1890/1051-0761(2003)013[0170:AECTMR
Ardoin, N., Heimlich, J., Braus, J., and Merrick, C. (2013). Influencing Conservation Action: What Research Says About Environmental Literacy, Behavior, and Conservation Results. National Audubon Society in partnership with the U.S. Fish and Wildlife Service, the Environmental Education and Training Partnership (EETAP), the North American Association for Environmental Education (New York, NY: NAAEE), TogetherGreen.
Arslan, A., McCarthy, N., Lipper, L., Asfaw, S., and Cattaneo, A. (2013). Adoption and Intensity of Adoption of Conservation Farming Practices in Zambia. Rome, IL: Agricultural Development Economics Division Food and Agriculture Organization of the United Nations.
Butler, P., Green, K., and Galvin, D. (2013). The Principles of Pride: The Science Behind the Mascots. Arlington, VA: Rare. Available online at: http://staging.rareconservation.fayze2.com/sites/default/files/Principles%20of%20Pride%202013%20lo%20res.pdf (Accessed December 29, 2015).
Catacutan, D. C., and Cramb, R. A. (2004). “Scaling up soil conservation programs: the case of landcare in the Philippines,” in 13th International Soil Conservation Organization Conference (Brisbane, QLD). Available online at: http://www.worldagroforestry.org/sea/Publications/files/paper/PP0103-04.pdf (Accessed December 29, 2015).
Chambers, J. M., and Hastie, T. J. (1992). “R: handle missing values in objects,” in Statistical Models in S (Wadsworth and Brooks/Cole). Available online at: https://stat.ethz.ch/R-manual/R-patched/library/stats/html/na.fail.html (Accessed November 20, 2016).
Chandy, L., and Linn, J. F. (2011). Taking Development Activities to Scale in Fragile and low Capacity Environments. Brookings Institution, Global Economy and Development. Available online at: http://www.brookings.edu/~/media/research/files/papers/2011/9/development%20activities%20chandy%20linn/scaling%20up%20fragile%20states.pdf (Accessed December 29, 2015).
Coastal Resources Center (2015). Developing Community-Based Approaches to Coastal Management in North Sulawesi Province. Available online at: http://www.crc.uri.edu/activities_page/developing-community-based-approaches-to-coastal-management-in-north-sulawesi-province/ (Accessed December 30, 2015).
Cramb, R. A. (2005). Social capital and soil conservation: evidence from the Philippines*. Aust. J. Agric. Resour. Econ. 49, 211–226. doi: 10.1111/j.1467-8489.2005.00286.x
Cramb, R. A., and Culasero, Z. (2003). Landcare and livelihoods: the promotion and adoption of conservation farming systems in the Philippine uplands. Int. J. Agric. Sustain. 1, 141–154. doi: 10.3763/ijas.2003.0113
Edgar, G. J., Stuart-Smith, R. D., Willis, T. J., Kininmonth, S., Baker, S. C., Banks, S., et al. (2014). Global conservation outcomes depend on marine protected areas with five key features. Nature 506, 216–220. doi: 10.1038/nature13022
Fuller, M. C., Kunkel, C., Zimring, M., Hoffman, I., Soroye, K. L., and Goldman, C. (2010). Driving Demand for Home Energy Improvements: Motivating Residential Customers to invest in Comprehensive Upgrades that Eliminate Energy Waste, Avoid High Utility Bills, and Spur the Economy. Environmental Energy Technologies Division, Lawrence Berkeley National Laboratory. Available online at: http://escholarship.org/uc/item/2010405t.pdf (Accessed December 29, 2015).
Gasco, M., Wright, C., Patruleasa, M., and Hedgecock, D. (2006). Romania: Scaling Up Integrated Family Planning Services: A Case Study. Arlington, VA: DELIVER, for the US Agency for International Development.
Gershon, D. (2009). Social Change 2.0: A Blueprint for Reinventing Our World. Pennsylvania State University, PA; High Point.
Granovetter, M. (1978). Threshold models of collective behavior. Am. J. Sociol. 83, 1420–1443. doi: 10.1086/226707
Haggblade, S., and Tembo, G. (2003). Development, Diffusion, and Impact of Conservation Farming in Zambia. Lusaka: Food Security Research Project. Available online at: http://www.fsg.afre.msu.edu/zambia/wp8zambia_all.pdf (Accessed December 29, 2015).
Hartmann, A., Kharas, H., Kohl, R., Linn, J., Massler, B., and Sourang, C. (2013). Scaling Up Programs for the Rurla Poor. Washington, DC: Global Economy and Development at Brookings.
Heal, G., and Rising, J. (2014). “Global benefits of marine protected areas,” in NBER Working Paper Series (19982).
Joffe-Walt, C. (2014). The Town Where Everyone Talks About Death. NPR Morning Edition. Available online at: http://www.npr.org/sections/money/2014/03/05/286126451/living-wills-are-the-talk-of-the-town-in-la-crosse-wis (Accessed December 30, 2015).
Kabacoff, R. I. (2014). Tree-Based Models. Quick-R: Accessing the Power of R. Available online at: http://www.statmethods.net/advstats/cart.html (Accessed November 16, 2016).
Kemp, R., Schot, J., and Hoogma, R. (1998). Regime shifts to sustainability through processes of niche formation: the approach of strategic niche management. Technol. Anal. Strat. Manag. 10, 175–195. doi: 10.1080/09537329808524310
Kern, K., Jörgens, H., and Jänicke, M. (2001). The Diffusion of Environmental Policy Innovations: A Contribution to the Globalisation of Environmental Policy. Wissenschaftszentrum Berlin für Sozialforschung. Available online at: http://papers.ssrn.com/sol3/papers.cfm?abstract_id=653583 (Accessed December 29, 2015).
Lester, S., Halpern, B., Grorud-Colvert, K., Lubchenco, J., Ruttenberg, B., Gaines, S., et al. (2009). Biological effects within no-take marine reserves: a global synthesis. Mar. Ecol. Prog. Ser. 384, 33–46. doi: 10.3354/meps08029
Management Systems International (2012). Scaling Up - From Vision to Large-Scale Change - Framework and Toolkit. Washington DC: Management Systems International.
Mandel, B. (2014). Cool Roofs Offer a Salve for Hot Cities — and the Climate, too. grist.org. Available online at: http://grist.org/climate-energy/cool-roofs-offer-a-salve-for-hot-cities-and-the-climate-too/
McDonald, J. (2014). “Small numbers in chi-square and G–tests,” in Handbook of Biological Statistics (Baltimore, MD: Sparky House Publishing), 86–89. Available online at: http://www.biostathandbook.com/small.html (Accessed December 3, 2015).
Mckenzie-Mohr, D. (2013). Fostering Sustainable Behavior: An introduction to Community-Based Social Marketing. New Society Publishers. Available online at: http://www.cbsm.com/pages/guide/preface/ (Accessed February 4, 2016).
Middleton, T., Roman, M. A., Ellis-Jones, J., Garforth, C., and Goldey, P. (2002). Lessons Learnt on Scaling-up from Case Studies in Bolivia. IDG/DFID. Available online at: http://r4d.dfid.gov.uk/PDF/Outputs/NatResSys/R7866Rep.pdf (Accessed December 29, 2015).
Moher, D., Liberati, A., Tetzlaff, J., Altman, D. G., and The PRISMA Group (2009). Preferred reporting items for systematic reviews and meta-analyses: the PRISMA statement. PLoS Med. 6:e1000097. doi: 10.1371/journal.pmed1000097
Moore, G. A. (2002). Crossing the Chasm: Marketing and Selling Technology Project. New York, NY: Harper Collins.
Mora, C. (2006). Coral reefs and the global network of marine protected areas. Science 312, 1750–1751. doi: 10.1126/science.1125295
Osborne, J. W., and Costello, A. B. (2004). Sample size and subject to item ratio in principal components analysis. Pract. Assess. Res. Eval. 9:8. doi: 10.4172/2155-6180.1000106
Pathmanathan, I., Liljestrand, J., Martins, J. M., Rajapaksa, L. C., Lissner, C., de Silva, A., et al. (2003). Investing in Maternal Health: Learning from Malaysia and Sri Lanka. Washington, DC: The World Bank.
Patlis, J., Tangkilisan, N., Karwur, D., Ering, M. E., Tulungen, J. J., Titahelu, et al. (2003). Case Study Developing a District Law in Minahasa on Community-Based Integrated Coastal Management: USAID Koleksi Dokumen Proyek Pesisir 1997–2003. Rhode Island: Coastal Resources Center, University of Rhode Island, Narragansett.
Peace Corps Master Farmer Program (2013). Peace Corps' Master Farmer Program Boosts Food Security and Economic Growth in Senegal. Available online at: http://www.peacecorps.gov/media/forpress/press/2253/
Porras, I., Barton, D. N., Miranda, M., and Chacon-Cascante, A. (2013). Learning from 20 years of Payments for Ecosystem Services in Costa Rica. London: International Institute for Environment and Development.
Prochaska, J. O., and DiClemente, C. C. (1982). Transtheoretical Therapy: toward a more integrative model of change. Psychotherapy 19, 276–288. doi: 10.1037/h0088437
Raes, L., and Mohebalian, P. (2014). The Socio Bosque Program for Rainforest and páramo Conservation, Ecuador. Geneva: The Economics of Ecosystems & Biodiversity. Available online at: http://www.teebweb.org/
Rogers, E. M., and Shoemaker, F. F. (1971). Communication of Innovations; A Cross-Cultural Approach. Available online at: http://eric.ed.gov/?id=ED065999 (Accessed December 29, 2015).
Rosenberg, T. (2011). Join the Club: How Peer Pressure Can Transform the World. New York, NY: Icon Books Ltd.
SEARCH (2016). Replicating the Home-Based Newborn Care in India: New Evidence from 12 Sites and Implications for National Policy (2006). In Report of the convention - SEARCH: Society for Education Action and Re SEARCH in Community Health.
Simmons, R., Fajans, P., and Ghiron, L. (eds.) (2007). Scaling up Health Service Delivery: From Pilot Innovations to Policies and Programmes. Geneva: World Health Organization.
Siry, H. Y. (2011). In search of appropriate approaches to coastal zone management in Indonesia. Ocean Coast. Manag. 54, 469–477. doi: 10.1016/j.ocecoaman.2011.03.009
Snetro-Plewman, G., Tapia, M., Uccellani, V., Brasington, A., and McNulty, M. (2007). Taking Community Empowerment to Scale: Lessons from Three Successful Experiences. Baltimore, MD: Johns Hopkins Bloomberg School of Public Health/Center for Communication Programs.
Sprinkles Global Health Initiative/About Us – History (2015). Sprinkles Global Health Initiative [Internet]. Available online at: http://www.sghi.org/about_us/history.html
Sutton, R. I., and Rao, H. (2014). Scaling Up Excellence: Getting to More Without Settling for Less. Stanford, CA: Crown Publishing Group.
Therneau, T. M., Atkinson, E. J., and others (1997). An Introduction to Recursive Partitioning Using the RPART Routines. Technical Report 61. Available online at: http://www.mayo.edu/research/documents/rpartminipdf/doc-10027257 (Accessed November 20, 2016).
Thiam, S., Thwing, J., Diallo, I., Fall, F. B., Diouf, M. B., Perry, R., et al. (2012). Scale-up of home-based management of malaria based on rapid diagnostic tests and artemisinin-based combination therapy in a resource-poor country: results in Senegal. Malar J. 11:334. doi: 10.1186/1475-2875-11-334
UNDP Small Grants Programme (SGP). Building on Cornerstones: Replication Scaling-Up Strategy for Community Based Innovative Projects of the UNDP GEF SGP India Programme - A Draft Note. Available online at: http://www.sgpindia.org/
USAID (2011). Scaling Up Community-Based Distribution of Injectable Contraception: Case Studies from Madagascar and Uganda. USAID, fhi360, and Progress in Family Planning.
World Health Organization ExpandNet (2009). Practical Guidance for Scaling Up Health Service Innovations. Geneva: World Health Organization.
World Health Organization ExpandNet (2011). Beginning with the End in Mind: Planning Pilot Projects and Other Programmatic Research for Successful Scaling Up. World Health Organization. Available online at: http://apps.who.int/iris/handle/10665/44708 (Accessed December 29, 2015).
Keywords: scaling, expanded impact, scaling success, conservation initiatives, pilot project design, targeted marketing, economies of scale, barrier removal
Citation: Battista W, Tourgee A, Wu C and Fujita R (2017) How to Achieve Conservation Outcomes at Scale: An Evaluation of Scaling Principles. Front. Mar. Sci. 3:278. doi: 10.3389/fmars.2016.00278
Received: 18 June 2016; Accepted: 13 December 2016;
Published: 04 January 2017.
Edited by:
E. Christien Michael Parsons, George Mason University, USAReviewed by:
Leslie Cornick, Alaska Pacific University, USAEdward Jeremy Hind-Ozan, Manchester Metropolitan University, UK
Copyright © 2017 Battista, Tourgee, Wu and Fujita. This is an open-access article distributed under the terms of the Creative Commons Attribution License (CC BY). The use, distribution or reproduction in other forums is permitted, provided the original author(s) or licensor are credited and that the original publication in this journal is cited, in accordance with accepted academic practice. No use, distribution or reproduction is permitted which does not comply with these terms.
*Correspondence: Willow Battista, d2lsbG93YmVAZ21haWwuY29t