- 1Department of Marine Biology, L. H. Charney School of Marine Sciences, University of Haifa, Haifa, Israel
- 2The Fredy & Nadine Herrmann Institute of Earth Sciences, Hebrew University of Jerusalem, Jerusalem, Israel
Mariculture production has increased significantly in recent years due to global rise in human population. However, in addition to providing food, fish farms are also a source of nutrients and antibiotics to the water column. Here, we model the nutrient changes originating from fish cages in the Eastern Mediterranean by utilizing a Lagrangian modeling approach that followed trajectories of the water parcels. The effects of farm size and the farm's distance from the shoreline were included in the model, and biological uptake and sinking of nutrients were incorporated into the analysis. By using computation of back-trajectories examining the origin of the water arriving at strategically important shoreline areas, such as desalination plants, we were able to identify which of the proposed farm locations might be potentially harmful. The results suggest that remotely-located, smaller and spatially distant farms are more preferable to limit the nutrient effects resulting from mariculture activity.
Introduction
Global marine fishing is expanding into previously unexploited areas as the fish yields in existing areas are depleted by overfishing (Jackson et al., 2001; Cheung et al., 2007; Froese et al., 2008; Swartz et al., 2010). This is due to more efficient fishing technology and the rise in human population that causes an increased requirement for food resources (Wackernagel and Rees, 1996). Alongside this, fish consumption is rapidly increasing to meet this demand (Godfray et al., 2010; Campbell and Pauly, 2013). Over recent decades, mariculture has increases dramatically in response to this increased demand. One of the most common types of mariculture is cage culture of finfish. Such mariculture may have ecological and economic benefits, such as compensating for the destruction of wild fisheries and increasing state revenues (Klinger and Naylor, 2012), however, with this development may come associated environmental concerns (Grigorakis and Rigos, 2011; Bouwman et al., 2013; Granada et al., 2015). For example, mariculture in sea cages can cause environmental damage in terms of: loss of natural habitats (Beveridge, 2001; Holmer et al., 2008), inadequate waste treatment (Wu, 1995), antibiotic discharge to the environment (Cabello, 2003), abundant use of marine fish in fishmeal (Sanchez-Jerez et al., 2011), transfer of pathogens from farmed to wild populations (Mladineo, 2007), effect of genetic exchange between wild and farmed populations (Grigorakis, 2010), and competition for resources between native and escaped fish (Karakassis et al., 2000; Holmer, 2010; Grigorakis and Rigos, 2011; Aguado-Gimenez and Ruiz-Fernandez, 2012).
In Israel, 100,000 tons of fish are consumed yearly. At present about 25% of the total (22–24,000 tons) is supplied by local fisheries and onshore aquaculture as well as some offshore facilities; the rest is imported (Ministry of Agriculture of Israel [MOAG], 2015). Onshore aquaculture accounts for 18,000 tons, while both artisanal fisheries and offshore farming in sea cages account for 2–3,000 tons each. Due to the gap between imports and local production, there is increasing pressure to expand the local mariculture industry and develop new farm locations in the Mediterranean coastal zone.
Increasing the number of sites for the purpose of fish farming raises some complex ecological questions regarding environmental impacts, as the fish cages constitute a source of nutrient enrichment (Lupatsch and Kissil, 1998; Holmer et al., 2008). The eastern Mediterranean basin is considered to be in a highly oligotrophic state (Berman et al., 1984; Krom et al., 2014). Following the construction of the Aswan high dam in the 1960s across the Nile river, it has become even more so, since the Nile was an important supply of nutrients to the South East Levantine basin in general and the Israeli coastal zone in particular (Nixon, 2003; Krom et al., 2004). It has been suggested that the anthropogenic nutrient loads, originating from various sources could replace, at least in part, the Nile nutrient supply to the Israeli coastal zone (Powley et al., 2016). Among these sources, mariculture and wastewater treatment facilities can input nutrients directly or indirectly into the coastal zone.
According to MOAG, the Food Conversion Ratio (FCR) of the Ashdod mariculture facility (Figure 1) sea bream (n.b., Sparus aurata) is 1 kg of fish per 1.8–2 kg of dry-food, similar to other sea bream farms in the world (Sadek et al., 2004). The annual production of the site is 14,000 tons y−1, in an area of 14 km2. About 5% of the dry food, the non-assimilated part, is considered a direct nitrogen discharge into the environment, and equates to 2.7 tons per day. Since there has been a proposal to increase the local fish production to 100,000 tons y−1, an impact assessment to examine the effects on environment is required. Increase in production can be through farm size and/or by farm numbers. The potential location of the new farms must also be examined pollution-wise, as the pollution may teach the coast, and specifically strategic sites such as desalination plants. Therefore, the effects of farm size, location and vicinity of farms to public beaches and desalination plants were examined.
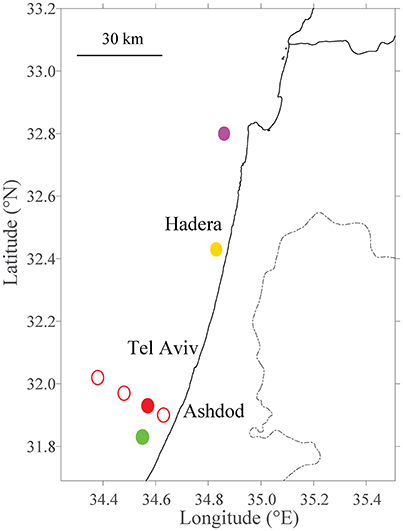
Figure 1. Site selection of fish farms tested within model domain. The existing farm in Ashdod is marked with a filled red circle. Different distances from the shoreline are marked with thin, empty red circles (Section Sensitivity to Distance from the Shoreline). Primary future farm sites (Section Sensitivity to Farm Sizes and Testing Potential Locations for Future Farms) are marked in filled circles in purple (Haifa; north), yellow (Hadera; center) and green (Ashdod; south).
There are several approaches to estimate the potential effects caused by mariculture, including the use of coupled hydrodynamic and water quality models (Wu et al., 1999), or Lagrangian models. For example, Wu et al. (1999) studied the effect of tidal currents on nutrient concentrations surrounding fish farms in the subtropical waters of Hong Kong, and how the effluent affected the local fish community. A similar scenario was examined by Lee et al. (2003), who approached nutrient concentration from tidal flushing with Lagrangian methodology and using numerical tracer experiments. Tracking of particulate matter, especially organic carbon, from fish farms and its effect on sediment and benthos has been examined by several authors (e.g., Cromey et al., 2002; Jusup et al., 2009; Brigolin et al., 2014). An example of such a study concerns the application of a Lagrangian model for simulating the deposition of feces and uneaten feed on the seabed with two individual-based fish population models in Italy (Brigolin et al., 2014). Similar models were tested in the Ligurian Sea (Doglioli et al., 2004), the Adriatic Sea (Jusup et al., 2009), and the North Aegean Sea (Petihakis et al., 2014). Doglioli et al. (2004) nested a Lagrangian particle model within a numerical hydrodynamic model, and estimated the regional dispersion patterns of different inorganic and organic nutrients, fecal matter and feed wastes. To date, no model has been used for the eastern Levantine basin, a densely populated and complex region with high potential and large demand for mariculture. Moreover, unlike previous Lagrangian models, we also consider the effect of biological uptake of the released nutrients by primary producers, a major factor in depleting nutrients (Thingstad et al., 2005; Pitta et al., 2009; Suari and Brenner, 2015).
The goal of the present study is to develop a Lagrangian tracking model, for studying the nutrient plume originated from fish farms. We used the Lagrangian model to track the fate of nutrients originated in fish farms, considering both physical and biological processes. The model follows virtual particles, which are used to represent the nutrients, and considers various hypothetical farm locations and sizes. The particles are advected by currents, sink and then are biologically consumed by phytoplankton. Our objectives are to develop a model, which potentially could be used by decision makers using a Lagrangian tracking model to prioritize farm placement, and their respective carrying capacities, using the Israeli shoreline as our test case. In addition, by looking at back-trajectories (i.e., the source regions of water or pollutants at certain locations), we can attempt to identify the “best” location to ensure minimal water quality damage to shoreline areas adjacent to desalination facilities and public beaches. Utilizing this modeling approach allows to adequately science based plan for growth of the industry, while minimizing major risks to the environment. A similar approach is also applicable to several other marine management-related issues, such as tracking oil plumes after major spill events (Petihakis et al., 2014; Goldman et al., 2015).
Methodology
Numerical simulations of the nutrient trajectories that have originated from the existing fish farm offshore Ashdod and from additional potential sites were performed, and several hypothetical simulations of scenarios were tested. The study site is described in the following subsection. The underlying velocity field derived from a 2D circulation model is described in Section The Velocity Field, and the particle tracking and analysis tool in Subsection Particle Tracking and Dispersion Analysis. The nutrients were initially treated as passive particles, and their uptake was detailed in Section Reduction Half-Life.
Study Site
Our study site is located in the territorial and Exclusive Economical waters of Israel, situated at the eastern Mediterranean Sea, with 190 km of shoreline (Figure 1). The sea-surface circulation of the Levantine Basin is characterized by a cyclonic along-slope current flowing over the continental shelf and slope zones (Rosentraub and Brenner, 2007). This current is prevailing throughout most of the year, although sometimes interrupted by southward episodic flows. The maximum current velocity is usually attained during summer or during winter storming events, with speeds reaching up to 1 m s−1 observed during winter (Rosentraub et al., 2010).
Data from the existing fish farm cages were inputed from offshore of Ashdod, Israel. The open water mariculture site is located ~12 km west of Ashdod, at a depth of 10 m (total water depth is 60 m). At this site, there are 6–10 fish-cages operating at any one time, at a small-scale capacity, farming sea bream (Sparus aurata), where each cage can hold up to 10,000 fish. The cages are designed to operate in an open sea and exposed location, as they are anchored on a single point mooring (SPM) and rotate with currents, and can withstand waves up to 15 m. The system can be submerged during high sea conditions and thus protect the cultivated fish during storms.
The Velocity Field
The circulation field utilized by the Lagrangian simulation was taken from southeastern Levantine Israeli Prediction System (SELIPS) forecast (Goldman et al., 2015). SELIPS is a forecasting system developed and operated routinely by the Israel Oceanographic and Limnological Research institute (IOLR), and can be used for operative purposes (Rosentraub et al., 2010; e.g., Goldman et al., 2012, 2014, 2015). The model's resolution is 0.01° × 0.00833° and we used the velocities at 10 m of water depth, which was the average depth of the fish farm cages. The bathymetry is smooth in the SELIPS model, thus at very shallow water its predictions may be biased. We used the current data from September 2012 to September 2013 that were detailed in Goldman et al. (2015).
Particle Tracking and Dispersion Analysis
Trajectories of nutrient particles originating from mariculture farms were performed to estimate the spatial effect of the anthropogenic eutrophication caused by farm activity. The significance of the effect of distance from shoreline on determining pollutant loading was tested, as well as the effect of farm size. Scenario testing was a key to examining these questions. To simulate the effects of biological uptake and particulate sinking, a nutrient reduction term was added. For areas in which water quality was important, such as desalination facilities and public beaches, water parcels were identified in order to determine the origin of the water origin using back-trajectory simulations.
The MatLab toolbox “PaTATO” (Fredj et al., 2016) that follows trajectories of water parcels was used. A pre-release version of this toolbox was used in Fine et al. (2013), Mundel et al. (2014), and Amitai et al. (in press). Each parcel is transported according to predefined currents, with an additional option to include first-order stochasticity. The trajectory analysis was performed using modeled 2D velocity fields. In our analyses, nutrients originating from the fish-cages were simulated as a parcel of water containing particles in various concentrations, depending on the scenario. The particle trajectories were computed for a period of 15 days. The number of particles from each farm, excluding the large farm in the second scenario (see Section Sensitivity to Farm Sizes and Testing Potential Locations for Future Farms), was set to a concentration of one particle per 0.01 km2, representing 0.27 ton km−2 d−1 of nitrogen released from the farm (totaling of 1,600 particles, per 16 km2). In the second scenario, the comparison of particle distribution between one large farm, with scattered small farms (the existing farm and three future farms, each with 1,600 particles), the number of particles in the large farm was 4 × 1,600. This means that the particle concentration of one particle in 0.002 km2 for the large farm. For the back-trajectory analysis, each location had 1,600 particles (16 km2), which were followed backward for 15 days to observe their origin. Each scenario was run for four different months of the year: January, April, July and October, in order to observe any effect or patterns of seasonality. A first-order stochastic diffusivity of K = 2 m2 s−1 was incorporated in the trajectory calculation to represent sub-grid processes that are not resolved by observational systems or by numerical models. K was based on Okubo (1971), for a grid size of ~O (1 km).
Reduction Half-Life
The concentration of nutrients are reduced in the open water column, due to phytoplankton uptake and the sinking of nutrient-rich particles. Therefore, in addition to treating the nutrients as passive particles, we also tested the effect of uptake and removal. Since estimating nutrient reduction is complex, because many factors affect it like the extant primary productivity and seasonality, the representation of reduction as a function of time was performed using a simple “half-life.” This was computed by: , where N(t) is the number of particles after time t (days), N(0) is the number of particles at the beginning of the simulation, and H is the half-life constant (days). The particles were eliminated randomly by the factor of . Since estimating H is difficult, a range for this parameter was set, and the reduction was calculated twice, for the minimum and maximum estimates of this range. According to Lipschultz (2001), who performed in situ incubations in the Sargasso Sea (BATS) to test the linearity of nitrogen (, , and ) uptake, ~50% reduction of the source label was recorded in 9–10 h. A set of microcosm experiments conducted in the eastern Mediterranean experimented by adding nutrients (both N and P) into the oligotrophic water, and suggested that H was between ~0.9 and 5 days (Kress et al., 2005, calculations provided in Appendix A, Supplementary Table A1). Therefore, H was set to be H = 1 day as the minimum boundary, and H = 5 days as the maximum.
Model Performance
A transect cruise was conducted on December 9, 2015 on the R/V Mediterranean Explorer and we used its samples to evaluate the model. The cruise included 11 sample stations as follows: at the north and south boundaries of the fish farm; at both 500 m north and south of the farm; three locations at 1,000 m north of the farm; and three locations at 5,000 m north of the farm. For control, a remote spot in front of Hertzelia was chosen (station 1). All sample points were in water depth of ~60 m, and described in Table 1, and all stations apart from station 1 as shown in Figures 9A,B.
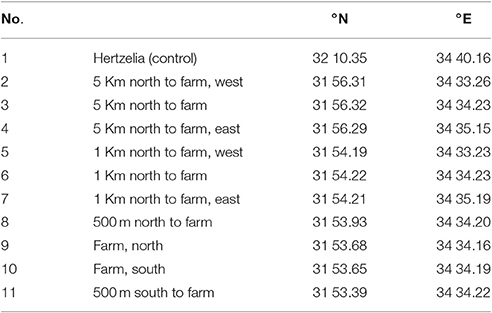
Table 1. Sample points description and locations from north to south (see also Figures 9A,B).
At each station, water samples were taken for inorganic nutrient analysis (NH4 and PO4). Water was collected using Niskin bottles deployed on a CTD/rosette package from 10 m depth. Samples for nutrient analyses were collected unfiltered into 15 ml vial bottles and immediately frozen upright in racks at −20°C. Nutrient content of ammonia and phosphate was determined using the segmented flow Seal Analytical AA3 colorimeter. The limit of detection (twice the standard deviation of the blank) was 0.008 μM for phosphate and 0.005 μM for ammonium.
To compare the Lagrangian particle tracking performance with nutrient concentrations collected during the cruise in the different stations, we conducted a simulation using SELIPS modeled currents from December 2015. This time, the seeded area was matched to the actual size of the farm and not its maximum capacity. Virtual particles were released from the farm's area every hour starting 3 days prior to the cruise date until 10:00 on the day of the cruise. At the time of nutrient sampling at station 9. 31,400 particles were released each hour, and the biological reduction (described in Section Reduction Half-Life) was calculated for each release separately, as each release spent a different time in the water.
Results
In two different scenarios, we followed the particle plumes from the farm locations, and in a third scenario, as back-trajectories from important shoreline locales (a desalination plant and a public beach). All farm locations and distances from shorelines are presented in Figure 1, and their coordinates are given in Table 2.
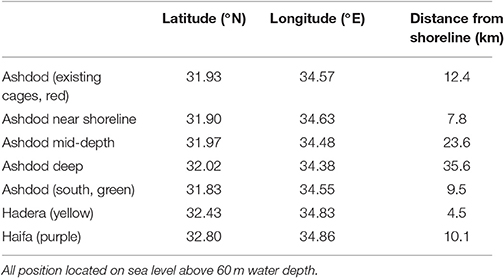
Table 2. Geographic positions of existing and proposed fish farm cages (coordinates of farm's center).
Sensitivity to Distance from the Shoreline
In the first scenario, we investigated the effect of fish farms at different distances from the shoreline. Since currents behave differently dependent on season and as a function of the distance from the shoreline, the location of a fish farm will affect its nutrient distribution. Obviously, there are logistical advantages of having the farms as close to shoreline as possible. On the other hand, the potential damage of pollution is greater from such inshore fish farms. To test the effect of distance from the shoreline, we conducted a set of experiments in which we place farms at several distances from the shoreline, and then track the released pollution according to season. We placed the farms at 7.8 (green circle in Figure 2), 12.4 (existing location, red circle in Figures 1, 2), 23.6 (yellow circle in Figure 2) and 35.6 km (purple circle in Figure 2) from shoreline. These locations are detailed in Table 2 and appear as empty circles in Figure 1). All farms are comparable in size to the existing farm.
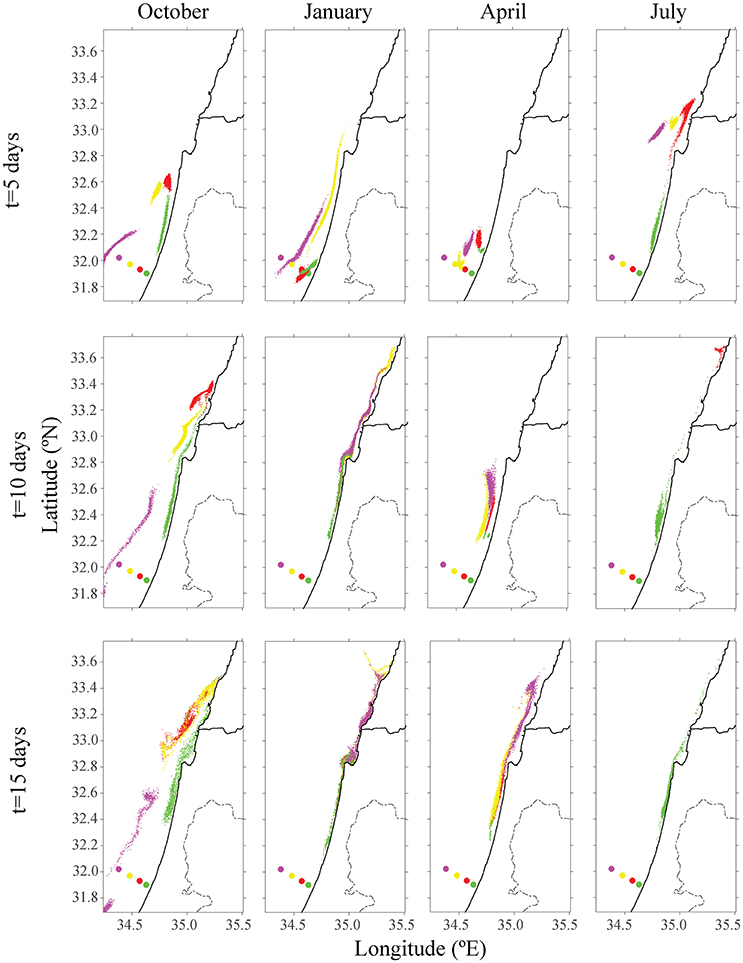
Figure 2. Distribution of particles from the existing offshore fish cage of Ashdod (in red) and proposed sites for different seasons in other colors (purple, yellow, and green). The top row describes the particle's distribution over 5 days; the middle row describes position after 10 days, and the bottom row after 15 days. Each color matches the farm's origin.
The existing cages and close to shore cages (red and green farms in Figure 2) both transport particulate nutrients very close to the shoreline. This pattern is most evident in the first 5 days of every season, and particularly in the first days of October and April. By January, all particles were transported to the shoreline, where particles from the proposed yellow farm (~23 km from shoreline) were transported north. In both July and October, particles from the farm located on the edge of the open sea (purple farm) did not approach the shoreline. In July, the greater current velocity transported the particles further up north, outside of our model's domain. These results were repeated for different positions of the four different locations. For all seasons besides January, there was a clear advantage of placing the farm at least 23 km (or greater) from the shoreline, in terms of water quality at the shoreline. Figure 3 shows the tracers' density after 15 days for each farm.
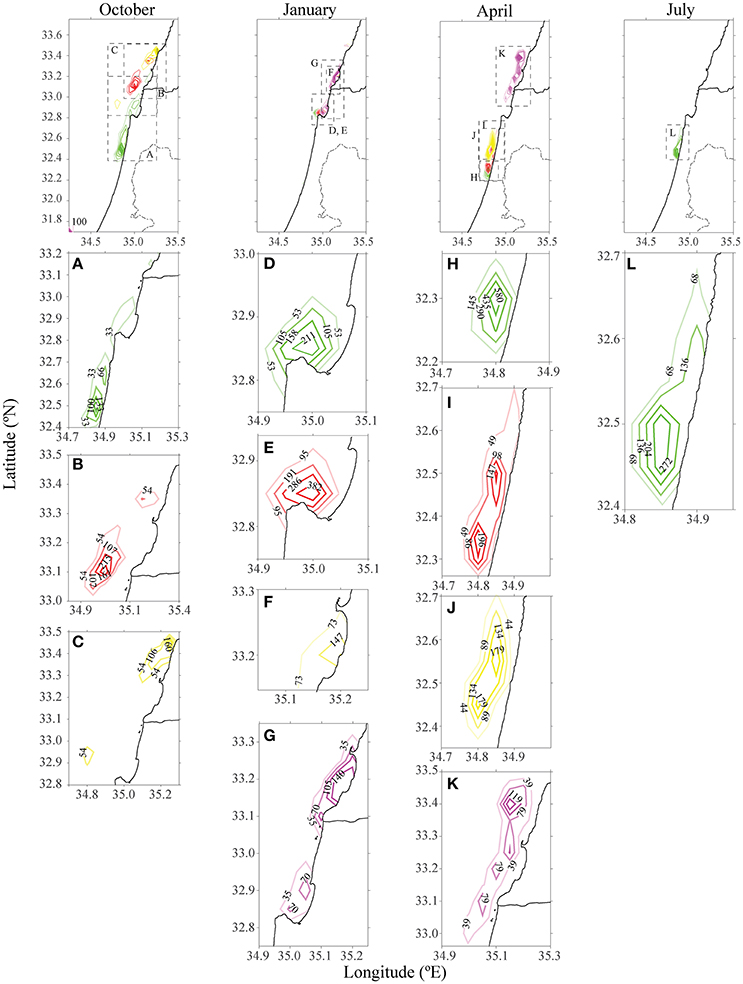
Figure 3. Density of particles from farms in different distances from the shoreline offshore Ashdod in different seasons after 15 days. The existing cages are highlighted in red. Each color matches the farm's origin as in Figure 2. Each column represents a season, and the panels below each season are individual farm's densities, zoomed. For October and July, only farms which had particles present in the model's domain were depicted.
Sensitivity to Farm Sizes and Testing Potential Locations for Future Farms
Another environmental consideration is whether to distribute the farming pressure amongst few small farms or to concentrate activity into one large expansive site, which is likely more economical. In the second scenario, production of four scattered farms (each with 1,600 tracers) was compared to a single farm with four times the production of the existing farm (4 × 1,600 tracers). Farms were placed along the northern, central and southern Israeli shoreline, and within the territorial seas limit, according to the recommendations from the Sustainable Aquaculture in the Mediterranean Sea Report (Ayalon et al., 2015). Then, we performed a comparison between a large farm, which represents a farm with four-fold production as the present one, and four farms scattered along the shoreline, each with a present production (i.e., total production in each case is equal, and is four-fold larger than present). As shown in Figure 4, for all four seasons, the distribution of particles was greater for four farms, with the purple farm scenario reaching up to Lebanon. Although particle distribution for four farms was greater, the concentration was lower (see Figure 5). In both January and July, the stronger currents transported the particles northwards, outside from the model's domain, thus only particles from the southern farms were viewable after 15 days. In April, the particles are relatively close to shoreline, and in October, most of the particles are found in the open sea in the north of Israel, and quite scattered. To test the effect of farm location, four farms were modeled next to one another (Figure 6, middle row). The results suggest that the more scattered the farms, the greater the particle distribution and lower the concentration.
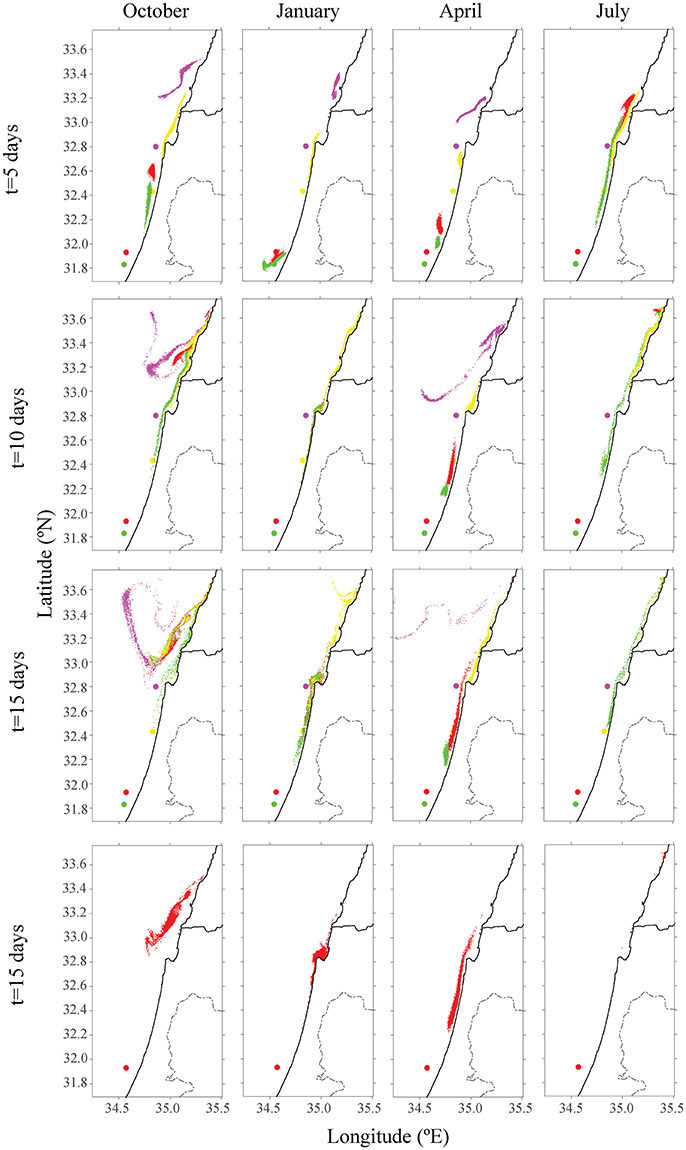
Figure 4. Distribution of particles from different existing and future farm scenarios, at different seasons. The red farm are the existing cages. The top row shows the particle's distribution after 5 days, the second row after 10 days, and the third after 15 days. Each color matches the farm's origin. Bottom row is the distribution after 15 days at the existing cages only, at the same concentration of the four farms.
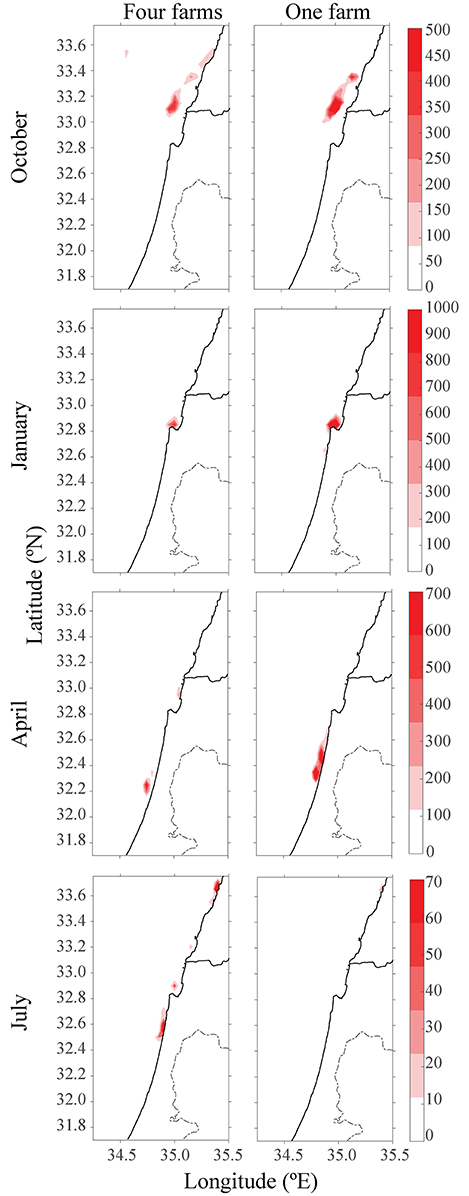
Figure 5. Heat-map of particles concentration after 15 days and from different seasons. Left column represents four scattered farms, and right column represents one large farm with production of four.
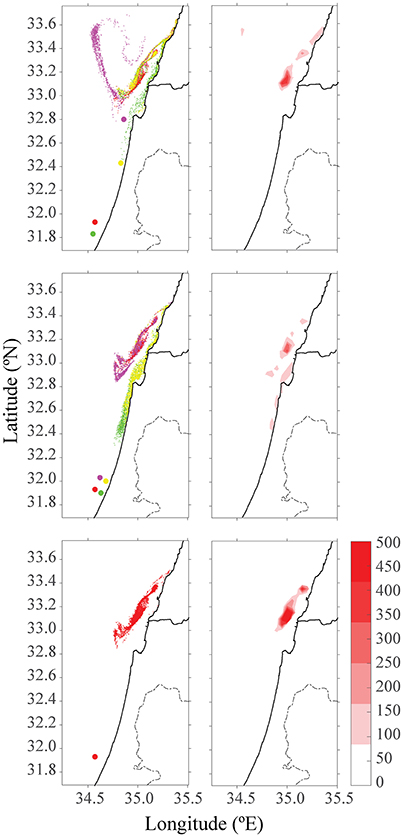
Figure 6. Distribution of particles and color-density maps of their concentrations after 15 days in October. The top row is four different farms in their future locations, the middle row is for four adjacent farms, and the bottom row is for one large farm. The red farm in all three scenarios indicates the existing farm cages in Ashdod.
Adding Nutrient Reduction
After 15 days of tracking, there were no more particles to track down when H = 1 day. In Figure 7, the maximum estimation for the half-life time (H = 5 days) assuming the real half-life time lies between the two values. For simplicity, the scenario with four farms is presented after 15 days. In April, instead of a maximum of 1,400 particles concentration (Figure 5), the highest concentration of particles was 75 particles. In July, most of the particles had breached the model's domain, leaving only 14 particles in the plot.
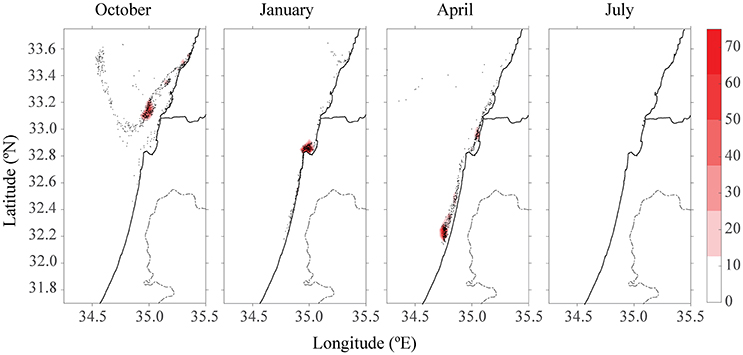
Figure 7. Color density map of particle concentration after 15 days for all four farm scenarios at different seasons for H = 5 days.
Origin of Water And Implications for Water Quality
This mathematical model has the potential to determine strategic farming prioritization. Given the location of certain key industries, such as a power or desalination plant, can we determine which areas should not be farmed, as the pollution originating from it may reach these strategic sites? In the third scenario, a test was conducted to determine how shoreline water quality might be affected by the fish farms. The desalination plant in Hadera, which utilizes seawater to produce drinking water (32.47°N, 34.88°E), and a Tel-Aviv public beach (32.08°N, 34.76°E) were chosen as locations where water quality standards are most important. Back-trajectories analysis from these two locations over a 15-day period suggested that the origin of near-shoreline water is mostly close to the shoreline (Figure 8). In winter (see Figure 8B), the water is rather turbulent, and water from the open sea reaches all the way to the shoreline. Apart from the winter season, the water does not originate from the existing fish cages. By tracking water back trajectories from important infrastructures, one can prioritize areas for future farms, to minimize the effects at these key sites.
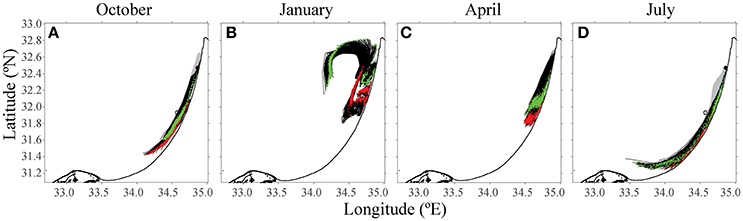
Figure 8. Results from the 15-day back-trajectories analysis to source water origins from the desalination plant and the public beach. The green path indicates particles from Hadera desalination factory target, and the red path follows water sampled from a Tel Aviv public beach. Both the black and gray are the trajectories of the particles till the target. The open circle is the location of the existing fish farm cages offshore Ashdod. (A) October, (B) January, (C) April, and (D) July.
Model-Observation Comparison
While on the day of the cruise the current headed typically northward (040°), there was an uncommon current regime heading southward for 4 days finishing 2 days before the cruise started (oceanographic data from Ashqelon monitoring station supplied by Dr. Eli Biton, IOLR, see Supplementary Table A2).
The phosphate concentrations are similar among all station (black bars, Figure 9C). Much higher concentrations of ammonia were observed further away from the cages (white bars) than around the fish farm. There was only one measurement for each nutrient, hence no statistical analysis. Some of the measured values were below the limit of detection.
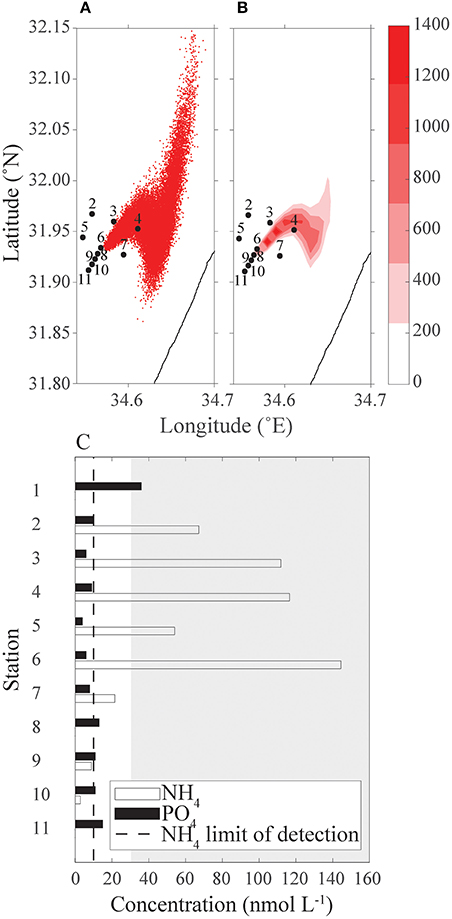
Figure 9. Comparison between measured nutrient concentrations from a cruise to the continuous release of particles simulation starting 3 days before the cruise to that day. (A) Simulation particle distribution; (B) heat map of simulated particles concentration; and (C) ammonium and phosphate concentrations (nmol L−1) measured from the cruise's samples, dashed line is limit of detection, gray-shaded area is the typical East Mediterranean range of NH4 in spring and summer time surface concentration above ground depth >7 m (data from IOLR reports). In both panels (A) and (B), sampling stations 2–11 are marked.
The inorganic nutrient analysis results were compared with the continuous release scenario output (Figure 9) qualitatively, and the comparison suggests that there is a good qualitative fit between the observation and model. In both the scenario output and the cruise measurements, stations 7–11 have a very small concentration of particle/nutrients; station 2 concentration is lower than stations 3 and 4; and the concentration in station 6 is higher than this of station 5 (Figure 9). The nutrient analysis suggests a higher concentration of NH4 than the prediction of the continuous release scenario output. As the continuous release scenario reflects nutrients originated from the fish farm only, the high concentration relative to the scenario's output assumed to be ammonium from a different source, such as the waste discharges from Tel Aviv sewage works. Around the cages the phytoplankton community was higher (unpublished results), resulting in lower concentrations of ammonium.
Discussion
Studying the effects of expanding the mariculture industry on water quality (and thus ecosystem health) is both economically and ecologically important. Agricultural discharge that flows into the sea may cause eutrophication, a process characterized by excessive algal growth or proliferation due to nutrient availability (Anderson et al., 2002). Excess of nutrients such as nitrate (NO3) and phosphate (PO4) may cause environmental imbalances in aquatic ecosystems (Bell et al., 1989). In extreme cases, high concentrations of nitrate may have an impact on human health (Ferreira et al., 2007). Moreover, studies have demonstrated that an increase in chlorophyll a due to eutrophication is usually accompanied by changes in phytoplankton community structure parameters such as total abundance, species richness, and evenness (Tsirtsis et al., 2008; Spatharis and Tsirtsis, 2010).
A shift in phytoplankton communities would force the whole food web structure to adjust, and trophic reorganization to be assumed. Changes in the phytoplankton composition also influence water chemistry (e.g., nutrients, pH, alkalinity, O2) and, consequently, material fluxes to sediments. Therefore, nutrient enrichment may cause basin-wide biogeochemical shifts, as well as massive ecosystem restructuring and may result in a new steady state of primary productivity. This can be observed all the way up the trophic levels to apex predator biomass (Turley, 1999).
Mariculture is a growing industry worldwide, and could provide the necessary food security in light of population increase and while providing relief to pressures of commercial fishing (Goldburg and Naylor, 2005; Godfray et al., 2010; Campbell and Pauly, 2013). In order to develop a sustainable industry that includes an ever-growing component of aquaculture, it is essential to assess via modeling and to inform the policy and implementation. The Lagrangian modeling method, testing several scenarios based on size and distance from the shoreline, was done as part of an effort to try to establish initial guidelines for both scientists and policy makers to insure a sustainable future to this growing industry in the Eastern Mediterranean basin.
According to the introduced model (Figures 2, 4), simulated particles from all the cages have reached the shorelines regardless of the cages' distance. Fish farming activity is constant throughout the year, with only a 20% reduction in fish feeding during wintertime (N. Mozes, MOAG, pers. comm.). However, a persistent plume of nutrients is released from the farms year-round.
When comparing one large farm with four smaller scattered farms, it was noted that although the distribution of particles was greater for the four small farms, the concentration was lower (see Figures 4, 5), suggesting several smaller farms would be the better solution in terms of water quality. This conclusion is supported when comparing the results of four adjacent farms compared with four scattered ones (Figure 5); the distribution and concentration of particles is similar to the large farm, yet greater and less dense, respectively.
Adding nutrient reduction is an important step toward a more realistic simulation, since nutrients are being consumed by phytoplankton and may sink. Wu (1995) writes that despite the high pollution loadings, results from various studies show that some 23% of C, 21% of N and 53% of P of feed input into the culture system are accumulated in the bottom sediments with significant impact normally confined to within 1 km of the farm. Our study suggests that 0–12.5% of these nutrients are staying in the environment for a period of 15 days (Figure 7) but our range is higher than the one measured by Wu (0.2–3%, Wu, 1995). Without considering reduction, there are some particles, which disperse to Lebanon even from the southernmost farms. The eastern Mediterranean is ultra-oligotrophic (Berman et al., 1984) and any addition of nutrients, either as fertilizer or by river input, is rapidly consumed (Krom et al., 2005; Pitta et al., 2009; Suari and Brenner, 2015). According to Suari and Brenner (2015), nutrients are being both advected and biologically consumed in such a way that the biological uptake is so fast that it cannot be detected by measurements. This study model is very sensitive, thus, it should be tested experimentally through novel methods, such as isotopically marking (Lipschultz, 2001) and tracking the particles via SF6, an inert, human-made, gas tracer, that is sparingly soluble, easy to measure at extremely low concentrations, and both biologically and chemically inert (Cole and Caraco, 1998).
We chose two strategic locations along the Israeli shoreline to demonstrate how to visualize the trajectories of nutrient plumes and inform decision makers. Potable water is of utmost importance for public health, and so the water desalination plant in Hadera was chosen. A beach in Tel Aviv, the most trafficked tourist and citizen public space was chosen as our second scenario. This analysis revealed that the origin of the water, for two of the months, was not coming from the farms in Ashdod. Similar tools can be used to examine potential locations for expanding the industry and their effect on these strategic, shoreline locations.
Caveats and Limitations
The goal of this paper is to demonstrate the usefulness of the Lagrangian tracking model for studying the pollution plume originated by fish farms and aiding decision makers, rather than produce realistic simulations. We stress that the results are based on the SELIPS currents field from September 2012 to September 2013. Other years may have produced different results, thus, predicting trajectories may be more appropriate when calculating an average of currents on a decadal scale. The trajectories in the analysis are based on particles leaving the farm for one specific day, whereas nutrients are actually put in continuously from the farms. Over a period of several days, the trajectories would vary and probably cover a larger area with accumulation of nutrients. Nevertheless, we evaluated the model performance using available data from 2015, as described in details in Sections Reduction Half-Life and Model-Observation Comparison.
Detecting changes in Chl a content of the water column in the vicinity of fish farms implying ghost nutrient discharged from fish farming activity, yet, studies (La Rosa et al., 2002; e.g., Pitta et al., 1999, 2005) have failed to detect it despite the large amount of continuous nutrient wastes discharged into the marine environment. Microbial grazing plays a key role in regulating phytoplankton biomass, keeping Chl a at very low levels and effectively transferring nutrients up the food web (Thingstad et al., 2005; Pitta et al., 2009). Lack of a detectable response of phytoplankton to nutrient enrichment has been attributed also to the rapid dilution of nutrients, since marine sites used for fish farming are selected for their relatively strong local current regime to ensure efficient water renewal (Gowen et al., 1983). Evaluating this model is, therefore, difficult. However, the SELIPS currents and PaTATO tool-box have been used by other models proving their accuracy, for example, Goldman et al. (2012, 2014, 2015), and Rosentraub et al. (2010).
Conclusion
Despite the above-mentioned limitations, this study demonstrates the potential in using a Lagrangian modeling approach to answer certain water-quality and nutrient distribution queries that arise from industry development. Furthermore, this tool is also suitable toward addressing other marine management related problems involving particle tracing and water movements (e.g., pollution, oil spills, larval connectivity, etc.).
Author Contributions
MG and HG designed the research, MG performed field sampling, and computer analyses. All authors revised and wrote the manuscript.
Funding
This study was conducted as part of the report for Sustainable Aquaculture in the Mediterranean Sea (Neaman Institute for National Policy Research). HG was supported by a grant from the Ministry of Science and Technology.
Conflict of Interest Statement
The authors declare that the research was conducted in the absence of any commercial or financial relationships that could be construed as a potential conflict of interest.
Acknowledgments
We wish to thank Ron Goldman and Dr. Eli Biton from Israel Oceanographic and Limnological Research (IOLR) for supplying currents data from the SELIPS model, Dr. Avi Gozolchiani and Yael Amitai from the Hebrew University of Jerusalem (HUJI) for advice and technical support with PaTATO toolbox, Eng. Noam Mozes from MOAG for fish farm data and advice on mariculture in Israel, Dr. Eyal Rahav from IOLR for his kind assistance in calculating nutrient half-lives and other useful remarks, Dr. Dalit Roth-Rosenberg from University of Haifa for helping with field data collection, Dr. Michael Krom and Anat Tzemel from University of Haifa for nutrient analyses. However, the content of this manuscript may not necessarily represent their personal opinion of the matters discussed. We also thank Leigh A. Kroeger for scientific editing.
Supplementary Material
The Supplementary Material for this article can be found online at: http://journal.frontiersin.org/article/10.3389/fmars.2017.00134/full#supplementary-material
References
Aguado-Gimenez, F., and Ruiz-Fernandez, J. M. (2012). Influence of an experimental fish farm on the spatio-temporal dynamics of a Mediterranean maerl algae community. Mar. Environ. Res. 74, 47–55. doi: 10.1016/j.marenvres.2011.12.003
Amitai, Y., Ashkenazy, Y., and Gildor, H. (in press). Multiple equilibria overturning variability of the Aegean-Adriatic Seas. Glob. Planet. Change doi: 10.1016/j.gloplacha.2016.05.004
Anderson, D., Glibert, P., and Burkholder, J. (2002). Harmful algal blooms and eutrophication: nutrient sources, composition, and consequences. Estuaries 25, 704–726. doi: 10.1007/BF02804901
Ayalon, O., Trop, T., Eshet, T., Liebes, I., Kerem, E., Zerbib, M., et al. (2015). Sustainable Aquaculture in the Mediterranean Sea. p. 142. Samuel Neaman Institute for National Policy Research. Hebrew with English abstract.
Bell, P., Greenfield, P., Hawker, D., and Connell, D. (1989). The impact of waste discharges on coral-reef regions. Water Sci. Technol. 21, 121–130.
Berman, T., Townsend, D., Elsayed, S., Trees, C., and Azov, Y. (1984). Optical transparency, chlorophyll and primary productivity in the Eastern Mediterranean near the Israeli coast. Oceanol. Acta 7, 367–327.
Beveridge, M. (2001). “Aquaculture and wildlife interactions,” in Environmental Impact Assessment of Mediterranean Aquaculture Farms, eds A. Uriarte and B. Basurco (Zaragoza: CIHEAM), 57–66.
Bouwman, L., Beusen, A., Glibert, P. M., Overbeek, C., Pawlowski, M., Herrera, J., et al. (2013). Mariculture: significant and expanding cause of coastal nutrient enrichment. Environ. Res. Lett. 8:044026. doi: 10.1088/1748-9326/8/4/044026
Brigolin, D., Meccia, V., Venier, C., Tomassetti, P., Porrello, S., and Pastres, R. (2014). Modelling biogeochemical fluxes across a Mediterranean fish cage farm. Aquacult. Environ. Interact. 5, 71–88. doi: 10.3354/aei00093
Cabello, F. (2003). Antibiotics and aquaculture. An analysis of their potential impact upon the environment, human and animal health in Chile. Fundacion Terram. Anal. Pol. Publ. 17, 1–16. doi: 10.5772/62049
Campbell, B., and Pauly, D. (2013). Mariculture: a global analysis of production trends since 1950. Mar. Policy 39, 94–100. doi: 10.1016/j.marpol.2012.10.009
Cheung, W., Watson, R., Morato, T., Pitcher, T., and Pauly, D. (2007). Intrinsic vulnerability in the global fish catch. Mar. Ecol. Prog. Ser. 333, 1–12. doi: 10.3354/meps333001
Cole, J., and Caraco, N. (1998). Atmospheric exchange of carbon dioxide in a low-wind oligotrophic lake measured by the addition of SF6. Limnol. Oceanogr. 43, 647–656. doi: 10.4319/lo.1998.43.4.0647
Cromey, C., Nickell, T., Black, K., Provost, P., and Griffiths, C. (2002). Validation of a fish farm waste resuspension model by use of a particulate tracer discharged from a point source in a coastal environment. Estuaries 25, 916–929. doi: 10.1007/BF02691340
Doglioli, A., Magaldi, M., Vezzulli, L., and Tucci, S. (2004). Development of a numerical model to study the dispersion of wastes coming from a marine fish farm in the Ligurian Sea (Western Mediterranean). Aquaculture 231, 215–235. doi: 10.1016/j.aquaculture.2003.09.030
Ferreira, J. G., Bricker, S. B., and Simas, T. C. (2007). Application and sensitivity testing of a eutrophication assessment method on coastal systems in the United States and European Union. J. Environ. Manag. 82, 433–445. doi: 10.1016/j.jenvman.2006.01.003
Fine, M., Gildor, H., and Genin, A. (2013). A coral reef refuge in the Red Sea. Glob. Change Biol. 19, 3640–3647. doi: 10.1111/gcb.12356
Fredj, E., Carlson, D., Amitai, Y., Gozolchiani, A., and Gildor, H. (2016). The particle tracking and analysis toolbox (PaTATO) for Matlab. Limnol. Oceanogr. Methods 14, 586–599. doi: 10.1002/lom3.10114
Froese, R., Stern-Pirlot, A., and Kesner-Reyes, K. (2008). Out of new stocks in 2020: a comment on “not all fisheries will be collapsed in 2048.” Mar. Policy 33, 180–181. doi: 10.1016/j.marpol.2008.03.009
Godfray, H. C. J., Beddington, J. R., Crute, I. R., Haddad, L., Lawrence, D., Muir, J. F., et al. (2010). Food security: the challenge of feeding 9 billion people. Science 327, 812–818. doi: 10.1126/science.1185383
Goldburg, R., and Naylor, R. (2005). Future seascapes, fishing, and fish farming. Front. Ecol. Environ. 3, 21–28. doi: 10.1890/1540-9295(2005)003[0021:FSFAFF]2.0.CO;2
Goldman, R., Biton, E., Brokovich, E., Kark, S., and Levin, N. (2015). Oil spill contamination probability in the southeastern Levantine basin. Mar. Pollut. Bull. 91, 347–356. doi: 10.1016/j.marpolbul.2014.10.050
Goldman, R., Efrati, S., Lehahn, Y., Gertman, I., and Heifetz, E. (2014). “Comparison of remotely sensed chlorophyll and Lagrangian coherent structures for velocity field validation,” in EGU General Assembly Conference Abstracts (Vienna) 16, 10332.
Goldman, R., Gertman, I., Rosentraub, Z., Ozer, T., and Hasson, N. (2012). “Operational forecasting of sea current in the south east Mediterranean Sea,” in The 9th Annual Conference of the Israeli Association of Aquatic Studies (IAAS). (in Hebrew) (Jordan Valley: Kinneret Academic Center).
Gowen, R., Tett, P., and Jones, K. (1983). The hydrography and phytoplankton ecology of Loch Ardbhair: a small sea-loch on the west coast of Scotland. J. Exp. Mar. Biol. Ecol. 71, 1–16. doi: 10.1016/0022-0981(83)90100-4
Granada, L., Sousa, N., Lopes, S., and Lemos, M. (2015). Is integrated multitrophic aquaculture the solution to the sectors' major challenges? – a review. Rev. Aquacult. 6, 1–18. doi: 10.1111/raq.12093
Grigorakis, K. (2010). Ethical issues in aquaculture production. J. Agric. Environ. Ethics 23, 345–370. doi: 10.1007/s10806-009-9210-5
Grigorakis, K., and Rigos, G. (2011). Aquaculture effects on environmental and public welfare - The case of Mediterranean mariculture. Chemosphere 85, 899–919. doi: 10.1016/j.chemosphere.2011.07.015
Holmer, M. (2010). Environmental issues of fish farming in offshore waters: perspectives, concerns and research needs. Aquacult. Environ. Int. 1, 57–70. doi: 10.3354/aei00007
Holmer, M., Argyrou, M., Dalsgaard, T., Danovaro, R., Diaz-Almela, E., Duarte, C. M., et al. (2008). Effects of fish farm waste on Posidonia oceanica meadows: synthesis and provision of monitoring and management tools. Mar. Pollut. Bull. 56, 1618–1629. doi: 10.1016/j.marpolbul.2008.05.020
Jackson, J. B. C., Kirby, M. X., Berger, W. H., Bjorndal, K. A., Botsford, L. W., Bourque, B. J., et al. (2001). Historical overfishing and the recent collapse of coastal ecosystems. Science 293, 629–638. doi: 10.1126/science.1059199
Jusup, M., Klanjscek, J., Petricioli, D., and Legovic, T. (2009). Predicting aquaculture-derived benthic organic enrichment: model validation. Ecol. Model. 220, 2470–2414. doi: 10.1016/j.ecolmodel.2009.06.033
Karakassis, I., Tsapakis, M., Hatziyanni, E., Papadopoulou, K. N., and Plaiti, W. (2000). Impact of cage farming of fish on the seabed in three Mediterranean coastal areas. ICES J. Mar. Sci. 57, 1462–1471. doi: 10.1006/jmsc.2000.0925
Klinger, D., and Naylor, R. (2012). Searching for solutions in aquaculture: charting a sustainable course. Annu. Rev. Environ. Resour. 37, 247–276. doi: 10.1146/annurev-environ-021111-161531
Kress, N., Thingstad, T. F., Pitta, P., Psarra, S., Tanaka, T., Zohary, T., et al. (2005). Effect of P and N addition to oligotrophic Eastern Mediterranean waters influenced by near-shore waters: a microcosm experiment. Deep Sea Res. 52, 3054–3073. doi: 10.1016/j.dsr2.2005.08.013
Krom, M., Herut, B., and Mantoura, R. (2004). Nutrient budget for the Eastern Mediterranean: implications for phosphorus limitation. Limnol. Oceanogr. 49, 1582–1592. doi: 10.4319/lo.2004.49.5.1582
Krom, M., Kress, N., Berman-Frank, I., and Rahav, E. (2014). “Past, present and future patterns in the nutrient chemistry of the Eastern Mediterranean,” in The Mediterranean Sea: Its History and Present Challenges, eds S. Goffredo, H. Baader, and Z. Dubinsky (New York, NY: Springer), 49–68.
Krom, M., Thingstad, T. F., Brenner, S., Carbo, P., Drakopoulos, P., Fileman, T. W., et al. (2005). Summary and overview of the CYCLOPS P addition Lagrangian experiment in the Eastern Mediterranean. Deep Sea Res. 52, 3090–3108. doi: 10.1016/j.dsr2.2005.08.018
La Rosa, T., Mirto, S., Favaloro, E., Savona, B., Sara, G., Danovaro, R., et al. (2002). Impact on the water column biogeochemistry of a Mediterranean mussel and fish farm. Water Res. 36, 713–721. doi: 10.1016/S0043-1354(01)00274-3
Lee, J., Choi, K., and Arega, F. (2003). Environmental management of marine fish culture in Hong Kong. Mar. Pollut. Bull. 47, 202–210. doi: 10.1016/S0025-326X(02)00410-1
Lipschultz, F. (2001). A time-series assessment of the nitrogen cycle at BATS. Deep Sea Res. 48, 1897–1924. doi: 10.1016/S0967-0645(00)00168-5
Lupatsch, I., and Kissil, G. W. (1998). Predicting aquaculture waste from gilthead seabream (Sparus aurata) culture using a nutritional approach. Aquat. Living Resour. 11, 265–268. doi: 10.1016/S0990-7440(98)80010-7
Mladineo, I. (2007). “Disease interactions and pathogen exchange between farmed and wild fish population,” in Review of Disease Interactions and Pathogen Exchange Between Farmed and Wild Finfish and Shellfish in Europe, eds R. Raynard, T. Wahli, I. Vatsos, and S. Mortensen (Oslo: DIP-net, Work Package), 309–314.
Mundel, R., Fredj, E., Gildor, H., and Rom-Kedar, V. (2014). New Lagrangian diagnostics for characterizing fluid flow mixing. Phys. Fluids 26, 126602. doi: 10.1063/1.4903239
Nixon, S. (2003). Replacing the Nile: are anthropogenic nutrients providing the fertility once brought to the Mediterranean by a great river? Ambio 32, 30–39. doi: 10.1579/0044-7447-32.1.30
Okubo, A. (1971). Oceanic diffusion diagrams. Deep Sea Res. 18, 789–802. doi: 10.1016/0011-7471(71)90046-5
Petihakis, G., Theodorou, A., Tsiaras, K., Pollani, A., Prospathopoulos, A., and Triantafyllou, G. (2014). A tool for ecosystem-based management applied to Pagasitikos Gulf. Fish Aquac. J. 5:092. doi: 10.4172/2150-3508.1000092
Pitta, P., Apostolaki, E., Giannoulaki, M., and Karakassis, I. (2005). Mesoscale changes in the water column in response to fish farming zones in three coastal areas in the Eastern Mediterranean Sea. Estuar. Coast. Shelf Sci. 65, 501–512. doi: 10.1016/j.ecss.2005.06.021
Pitta, P., Karakassis, I., Tsapakis, M., and Zivanovic, S. (1999). Natural vs. mariculture induced variability in nutrients and plankton in the eastern Mediterranean. Hydrobiologia 391, 179–192. doi: 10.1023/A:1003501832069
Pitta, P., Tsapakis, M., Apostolaki, E. T., Tsagaraki, T., Holmer, M., and Karakassis, I. (2009). Ghost nutrients from fish farms are transferred up the food web by phytoplankton grazers. Mar. Ecol. Prog. Ser. 374, 1–6. doi: 10.3354/meps07763
Powley, H. R., Dürr, H. H., Lima, A. T., Krom, M. D., and Van Cappellen, P. (2016). Direct discharges of domestic wastewater are a major source of phosphorus and nitrogen to the Mediterranean Sea. Environ. Sci. Technol. 50, 8722–8730. doi: 10.1021/acs.est.6b01742
Rosentraub, Z., Anis, A., and Goldman, R. (2010). Wintertime cross shelf circulation and shelf/slope interaction off the central Israeli coast. Rapp. Comm. Int. Mer. Medit. 39:171. doi: 10.1029/2006JC003775
Rosentraub, Z., and Brenner, S. (2007). Circulation over the southeastern continental shelf and slope of the Mediterranean Sea: direct current measurements, winds, and numerical model simulations. J. Geophys. Res. 112, C11001. doi: 10.1029/2006JC003775
Sadek, S., Osman, M., and Mansour, M. (2004). Growth, survival and feed conversion rates of sea bream (Sparus aurata) cultured in earthen brackish water ponds fed different feed types. Aquacult. Int. 12, 409–421. doi: 10.1023/B:AQUI.0000042131.29346.93
Sanchez-Jerez, P., Fernandez-Jover, D., Uglem, I., Arechavala-Lopez, P., Dempster, T., Bayle-Sempere, J. T., et al. (2011). “Coastal fish farms as fish aggregation devices (FADs),” in Artificial Reefs in Fishery Management, eds S. A. Bortone, F. Pereira Brandini, G. Fabi, and S. Otake (Boca Raton, FL: CRC Press; Taylor & Francis Group), 187–208.
Spatharis, S., and Tsirtsis, G. (2010). Ecological quality scales based on phytoplankton for the implementation of water framework directive in the Eastern Mediterranean. Ecol. Indic. 10, 840–847. doi: 10.1016/j.ecolind.2010.01.005
Suari, Y., and Brenner, S. (2015). Decadal biogeochemical history of the south east Levantine basin: simulations of the river Nile regimes. J. Mar. Syst. 148, 112–121. doi: 10.1016/j.jmarsys.2015.02.004
Swartz, W., Sala, E., Tracey, S., Watson, R., and Pauly, D. (2010). The spatial expansion and ecological footprint of fisheries (1950 to Present). PLoS ONE 5:E15143. doi: 10.1371/journal.pone.0015143
Thingstad, T. F., Krom, M. D., Mantoura, R. F. C., Flaten, G. A. F., Groom, S., Herut, B., et al. (2005). Nature of phosphorus limitation in the ultraoligotrophic eastern Mediterranean. Science 309, 1068–1071. doi: 10.1126/science.1112632
Tsirtsis, G., Spatharis, S., and Karydis, M. (2008). Application of the lognormal equation to assess phytoplankton community structural changes induced by marine eutrophication. Hydrobiologia 605, 89–98. doi: 10.1007/s10750-008-9307-2
Turley, C. (1999). The changing Mediterranean Sea - a sensitive ecosystem? Prog. Oceanogr. 44, 387–400. doi: 10.1016/S0079-6611(99)00033-6
Wackernagel, M., and Rees, W. (1996). Our Ecological Footprint. Gabriola Island, BC: New Society Publishers.
Wu, R. (1995). The environmental impact of marine fish culture: towards a sustainable future. Mar. Pollut. Bull. 31, 159–166. doi: 10.1016/0025-326X(95)00100-2
Keywords: levantine basin, lagrangian simulation, anthropogenic eutrophication, mariculture, coastal management
Citation: Grossowicz M, Tchernov D and Gildor H (2017) A Quantitative Management Tool Reflecting Impact of Nutrient Enrichment from Mariculture in the Levantine Basin. Front. Mar. Sci. 4:134. doi: 10.3389/fmars.2017.00134
Received: 23 October 2016; Accepted: 21 April 2017;
Published: 10 May 2017.
Edited by:
Hans Uwe Dahms, Kaohsiung Medical University, TaiwanReviewed by:
Xiaoshou Liu, Ocean University of China, ChinaRathinam Arthur James, Bharathidasan University, India
Copyright © 2017 Grossowicz, Tchernov and Gildor. This is an open-access article distributed under the terms of the Creative Commons Attribution License (CC BY). The use, distribution or reproduction in other forums is permitted, provided the original author(s) or licensor are credited and that the original publication in this journal is cited, in accordance with accepted academic practice. No use, distribution or reproduction is permitted which does not comply with these terms.
*Correspondence: Hezi Gildor, hezi.gildor@mail.huji.ac.il
†Present Address: Michal Grossowicz, Yigal Allon Kinneret Limnological Laboratory, Israel Oceanographic and Limnological Research, Migdal, Israel