- 1Institute of Marine Sciences (ISMAR), Italian National Research Council (CNR), Ancona, Italy
- 2Institute of Earth, Ocean and Atmospheric Science, Rutgers University, New Brunswick, NJ, United States
- 3Program in Interdisciplinary Studies, Institute of Advanced Studies, Princeton, NJ, United States
- 4Earth and Life Science Institute (ELSI), Tokyo Institute of Technology, Tokyo, Japan
- 5Department for Life and Environmental Science (DISVA), Polytechnic University of Marche, Ancona, Italy
- 6Department of Marine Ecology, Royal Netherlands Institute for Sea Research (NIOZ), Texel, Netherlands
The global scale of the biodiversity crisis has stimulated research into the relationship between biodiversity and ecosystem functioning (BEF). Even though the deep sea is the largest biome on Earth, BEF studies in deep-sea benthic ecosystems are scant. Moreover, the small number of recent studies, which mostly focus on meiobenthic nematodes, report conflicting results that range from a very clear positive relationship to none at all. In this BEF study, the deep-sea macrofauna were used as a model to investigate the structural and functional diversity of macrofauna assemblages at three depths (1,200, 1,900, and 3,000 m) in seven open-slope systems from the North-Eastern Atlantic Ocean to the Central-Eastern Mediterranean Sea. The presence and nature of BEF relationships were studied considering two spatial scales, the large and the basin scale, in different environmental settings. Total benthic biomass and macrofaunal predator biomass were used as proxies to assess ecosystem functioning. Ecosystem efficiency was expressed as macrofaunal biomass to biopolymeric carbon content ratio, macrofaunal biomass to prokaryotic biomass ratio, macrofaunal biomass to meiofaunal biomass ratio, and meiofaunal biomass to prokaryotic biomass ratio. On both large and basin spatial scales, some significant relationships between macrofaunal diversity and ecosystem functioning and efficiency were reported. When significant, the nature of BEF relations was positive and exponential or linear supporting the general idea that a higher diversity can enhance ecosystem functioning. Other BEF relationships were explained by the effect of environmental variables. More data from different deep-sea systems are needed, to better elucidate the consequences of biodiversity loss on the ocean floor.
Introduction
The Earth is experiencing a pervasive and uncontrolled loss of species, raising grave concerns about the deterioration of ecosystem functions and services (Hooper et al., 2005). This scenario has stimulated research into biodiversity-ecosystem function (BEF) relationships, in order to clarify how ecosystems work and respond to change, and to establish whether and how biodiversity matters (Loreau, 2010). Ecosystem functioning is a general concept that refers to the overall performance of ecosystems (Jax, 2005). It has been posited as incorporating a variety of ecosystem processes (such as biogeochemical cycles), properties (e.g., pools of organic matter) and goods (e.g., food), individually or in combination (Armstrong et al., 2012). Describing or measuring ecosystem functioning is difficult, particularly given the breadth of the notion, and often a precise definition is not provided at all (Bremner, 2008).
Over the past few decades a large body of work—most of it performed in terrestrial environments or in the laboratory, where manipulative experiments can be performed under controlled conditions (Mora et al., 2011)—has explored BEF relationships (see Cardinale et al., 2011; Tilman et al., 2014 for reviews). BEF studies conducted in terrestrial, freshwater, and marine systems (Tilman et al., 2014) have shown that biodiversity affects ecosystem functioning. Overall, the relationship is positive, especially where heterogeneous systems (e.g., biogenic habitats; Miller et al., 2012) and long timescales are involved (Cardinale et al., 2007). Such a positive effect is often related to the fact that different species play complementary rather than competitive roles (Gamfeldt et al., 2014).
However, not all studies report the same general trend, and conflicting results have been described in small-scale experiments and large-spatial scale observations (Mora et al., 2014; Lefcheck and Duffy, 2015). Experimental studies have usually reported that species diversity exerts a saturating effect, whereas field work has tended to describe a positive effect on ecosystem functioning. In addition, a neutral or a negative effect has been described in several studies, suggesting that a positive effect is not the rule (Lefcheck and Duffy, 2014).
Another important issue when assessing the existence and nature of BEF relationships is the temporal (Yasuhara et al., 2016) and spatial (Venail et al., 2008) scale of the investigation. Accordingly, a major challenge to understanding the effects of biodiversity on ecosystem functioning is to establish whether the patterns observed in the small scale also apply to the larger scale, and vice versa (Biles et al., 2003). Numerous BEF studies have related taxon diversity, primarily taxon richness, to the rate of ecosystem processes, using this diversity measure as a surrogate for functional diversity (Naeem and Wright, 2003). The notion of functional diversity incorporates interactions among organisms and their environment into a concept that can depict ecosystem level structure in marine environments (Bremner et al., 2003). Since functional diversity provides a direct mechanistic link between diversity and ecosystem functioning, a growing amount of research is being devoted to assessing the effect of functional—rather than taxon—diversity on ecosystem functioning (Petchey et al., 2004; Reiss et al., 2009). Numerous independent variables of functional traits, including feeding guilds, reproduction strategy, bioturbation mode and/or body shape (e.g., Ieno et al., 2006; Carvalho et al., 2013; Pape et al., 2013; Piot et al., 2014; Quéiros et al., 2015), and biological traits analysis (e.g., Bremner et al., 2003; Bolam and Eggleton, 2014) have been applied to express functional diversity. Nevertheless, there is currently no universally accepted methodology for selecting the most appropriate traits for a given study (Bolam, 2013), and quite often the selection is heavily affected by the limited biological information that is available for benthic invertebrate taxa (Bremner, 2008).
The deep sea is the most extensive environment on the planet (Ramirez-Llodra et al., 2010), and is a key contributor to ecosystem services and functions, such as ocean carbon cycling (Snelgrove et al., 2014). It is well established that the deep sea is characterized by a relatively high diversity (Grassle and Maciolek, 1992; Rex and Etter, 2010) that varies depending on the habitat being investigated (Levin et al., 2001), spanning from a relatively low diversity with a high level of endemism (e.g., chemosynthetic habitats; Levin et al., 2000) to a rich spatial and faunal diversity, such as that characterizing biogenic habitats (Roberts et al., 2006; Serpetti et al., 2013).
Even though the amount of marine systems investigation has risen steeply over the past few years (Worm et al., 2006; Mora et al., 2011), deep-sea BEF studies (>200 m depth) are quite recent (Danovaro et al., 2008a; Leduc et al., 2013; Narayanaswamy et al., 2013; Pape et al., 2013; Zeppilli et al., 2016). A positive and exponential BEF relationship, reported for deep-sea benthic communities (e.g., Danovaro et al., 2008a; Narayanaswamy et al., 2013), has been explained by the prevalence of mutualistic rather than competitive interactions between organisms (Loreau, 2008). It has been suggested that complementarity relationships between species are predominant in the more diverse systems (like deep-sea systems), with facilitation and resource partitioning leading to overall higher function (Loreau, 2000).
The strength and the nature of BEF relationships differs strongly among habitats (Danovaro, 2012; Lefcheck and Duffy, 2014; Thurber et al., 2014) and can be influenced by environmental factors (e.g., water depth, temperature, input of organic carbon from the surface) that have a major influence on both deep-sea biodiversity (Tittensor et al., 2011) and ecosystem function (Smith et al., 2008). In addition, a positive BEF relationship may only apply to low-diversity assemblages due to increased competition or to greater functional redundancy in more diverse assemblages (Leduc et al., 2013). It is often assumed that a positive relationship between biodiversity and ecosystem function can reach saturation (Loreau, 2008); after saturation has been reached, some species with particular ecological traits have the potential to enhance overall ecosystem functioning (Cardinale et al., 2007).
Most deep-sea BEF investigations have used the meiofauna, particularly nematodes, as model taxa (Danovaro et al., 2008a; Leduc et al., 2013; Pape et al., 2013; Pusceddu et al., 2014b; Yasuhara et al., 2016; Zeppilli et al., 2016), whereas comparatively few studies have examined how microbial and viral components (Danovaro et al., 2008b), or of larger epifauna (Amaro et al., 2010), influence ecosystem functioning. In this study, BEF relationships were explored by focusing on the structural and functional diversity of the macrobenthic fauna. Macrobenthos have recognized ecological roles in key processes such as bioturbation (Meysman et al., 2006; Loreau, 2008; Braeckman et al., 2010), sediment oxygenation, and as a food source for higher trophic levels (Gage and Tyler, 1991), and have often been studied in shallow-water and freshwater BEF investigations (Gamfeldt et al., 2014; Lefcheck and Duffy, 2014). However, to the best of our knowledge, they have never been studied in deep-sea BEF research. Since setting up deep-sea in situ experiments is difficult and costly, the observational—correlative approach (Loreau, 2008) was employed to answer the following questions: (i) Is there a relationship between deep-sea macrofaunal diversity and ecosystem functioning and efficiency? (ii) Does macrofaunal functional diversity have a stronger link to ecosystem functioning than does macrofaunal diversity per se? (iii) Can the spatial scale of the investigation (i.e., large vs. basin scale) and related environmental factors influence BEF relationships?
Materials and Methods
Study Area and Sampling Strategy
Biological and environmental samples were collected during several cruises in the framework of the ESF EuroDEEP BIOFUN project (“Biodiversity and Ecosystem Functioning in Contrasting Southern European Deep-sea Environments: from Viruses to Megafauna”), whose main aim was to explore possible links between biodiversity patterns and ecosystem functioning in relation to environmental conditions, through analyzing organisms ranging from viruses to megafauna.
Sampling was conducted at selected deep-sea sites along a gradient of increasing oligotrophy from west to east—from the Galicia Bank in the Atlantic Ocean to the Levantine Basin—at depths of 1,200, 2,000, and 3,000 m. Sediment samples were collected in 7 open-slope areas: 1 in the North Eastern Atlantic (ATL), 3 in the Western Mediterranean basin (wM1, wM2, and wM3), and 3 in the Central-Eastern Mediterranean basin (c-eM1, c-eM2, and c-eM3) (Figure 1). In each area, 3 stations were sampled at 3 different depth ranges: upper bathyal (1,200 m), mid-bathyal (1,800–1,900 m), and lower bathyal (2,400–3,000 m). Since the sample at the lower bathyal depth (c-eM1) could not be collected, this station was replaced with one at a depth of 2,120 m (Table S1).
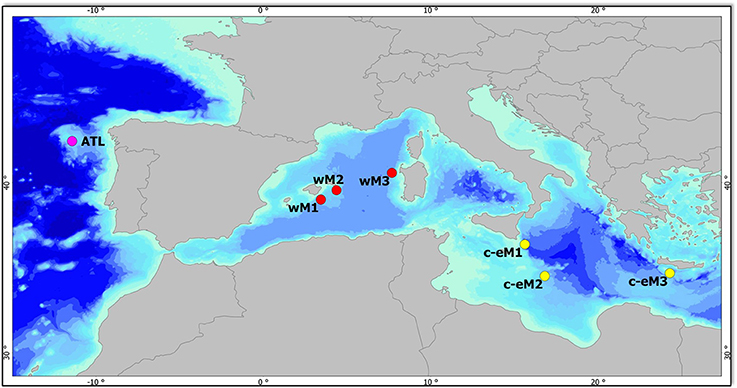
Figure 1. Map of the study area and sampling sites. Purple circle, Galicia Bank—Atlantic Ocean (ATL); red circles, Western Mediterranean basin (wM1, 2, 3), yellow circles, Central-Eastern Mediterranean basin (c-eM1, 2, 3).
The Atlantic sampling area was on the Galicia Bank, a seamount situated on the Iberian margin about 200 km off the Galician coast. The Bank, whose summit is found at a depth of 620 m, is separated from the shallower parts of the continental margin by the Galicia Interior Basin (Pape et al., 2013), whose approximate depth is 3,000 m. Water current velocities on top of the seamount range from 5 to 30 cm s-1 (Pape et al., 2013), which is sufficient to influence organic matter deposition. Such currents result in very low phytopigment and biopolymeric organic carbon concentrations and in the presence of coarse sediments at 1,200 m depth (Table S1). The deep Mediterranean Sea is a highly oligotrophic environment, where nutrient depletion in surface waters combines with high water temperature, promoting the degradation of sinking organic matter (Sardà et al., 2004). The Mediterranean basin is characterized by a trophic gradient between the less productive eastern basin and the more productive western basin (D'Ortenzio and Ribera d'Alcalà, 2009; Giovannelli et al., 2013; Table S1), where the greater nutrient inputs are due to river runoff, the inflow of Atlantic surface water, and the outflow of relatively nutrient-rich Levantine Intermediate Water through the Strait of Gibraltar (Bergamasco and Malanotte-Rizzoli, 2010). The Mediterranean open-slope systems selected for the present study are characterized by a high percentage of silt sediment at all depths. Bottom-water temperature and salinity increase significantly from west to east, with values ranging respectively from 13.1°C and 38.5°C in the wM area to 14.7°C and 38.8°C in the Eastern Mediterranean basin (Baldrighi et al., 2014; Table S1). At each station, sampling involved 3 independent box-corer deployments for macrofauna (entirely sieved) and 3 deployments for meiofauna, microbial components, and environmental variables (n = 3 replicate samples for each parameter). Macrofauna samples were collected with a cylindrical box-corer with an internal diameter of 32 cm at all stations except for ATL, wM2 and c-eM2, where its internal diameter was 50 cm, accounting for a surface area of 803.84 cm2 and 1,962.5 cm2, respectively.
Data of macrofaunal abundance, biomass and diversity as well as data of environmental parameters characterizing the Mediterranean basin from west to east have previously been shown in Baldrighi et al. (2014). Data of meiofauna abundance, structural and functional diversity from the Mediterranean basin have been presented in Baldrighi and Manini (2015) and the relationship with macrofaunal structural and functional diversity has been investigated. In the present study, the same dataset has been used, with the addition of new data from the Atlantic area (i.e., macrofaunal diversity, benthic biomass, environmental features) and ecosystem functioning and efficiency proxies. We adopted a new approach of investigation aimed at finding BEF relations. A complete species list is provided in Table S2.
Processing of Environmental and Biological Samples
For grain size, biochemical composition of organic matter, and microbial component analysis, sediment subsamples from each box-corer were collected using plexiglass cores with an internal diameter of 3.6 cm. The top 1 cm of a sub-sample from each box-corer was collected and frozen at −20°C to analyze chlorophyll-a, phaeopigment, and organic matter content. Replicates of about 1 ml wet sediment were fixed using buffered formaldehyde and stored at +4°C until processing for total prokaryotic abundance and biomass determination (Giovannelli et al., 2013). The top 20 cm was preserved at +4°C for grain size analysis. For meiofauna analysis, sediment from each box-corer was collected using a plexiglass tube with an internal diameter of 3.6 cm and immediately fixed in 4% buffered formalin and Rose Bengal. Once in the laboratory, only the top 5 cm was sieved through a 300-μm and subsequently a 20-μm mesh sieve. Meiofaunal samples were obtained from 6 of the 7 areas (meiofauna data not available from c-eM2 slope area). For macrofauna analysis, the top 20 cm of sediment from each box-corer and the overlying water were sieved through a 300-μm mesh sieve to retain all macrobenthic organisms (here considered sensu lato, including nematodes, copepods, foraminifera, etc.) (Baldrighi and Manini, 2015). The residue left in the sieve was immediately fixed in buffered formalin solution (10%), and stained with Rose Bengal.
For grain size analysis, aliquots of fresh sediment were sieved through a 63-μm mesh. The two fractions (>63 μm, sand; <63 μm, silt and clay) were dried in an oven at 60°C and weighed. Data were expressed as a percentage of the total sediment dry weight (Baldrighi et al., 2014).
Chlorophyll-a and phaeopigments were determined by standard techniques (Danovaro, 2010). The sum of chlorophyll-a and phaeopigment concentrations was designated as chloroplastic pigment equivalents (CPE). Total phytopigment concentrations were converted to carbon (C) equivalents using a conversion factor of 40 (De Jonge, 1980) and expressed as mgC g−1. Biopolymeric organic carbon (BPC) was calculated as the sum of the carbon equivalents of carbohydrates, proteins, and lipids (Fabiano et al., 1995). The protein to carbohydrate (PRT: CHO) ratio was then calculated and used as a descriptor of the nutritional value of sediment organic matter. PRT: CHO ratios >1.0 indicate relatively high quality and high food availability (Pusceddu et al., 2010).
The total prokaryotic number (TPN) was determined by acridine orange staining (Luna et al., 2002) and analyzed using epifluorescence microscopy (magnification, 1,000 x). Total prokaryotic biomass (TPB) was estimated using an ocular micrometer; prokaryotic cells were assigned to different size classes (Fry, 1990) and converted to biovolumes based on the assumption that they had an average carbon content of 310 fgC μm−3 (Fry, 1990). For meiofauna analysis, the fraction remaining on the 20-μm sieve was resuspended and centrifuged using Ludox HS40 (Danovaro, 2010). Meiofaunal biomass was estimated as described by Danovaro (2010). Macrofaunal organisms were sorted under a stereomicroscope and identified to the lowest possible taxonomic level. For each species, the total number was calculated and the wet weight biomass measured and expressed respectively as abundance and biomass per m2. Wet biomass (g wet weight m−2) was converted to ash-free dry weight and organic carbon content using standard conversion factors (Rowe, 1983).
Taxonomic and Functional Diversity of Macrofauna
The diversity and functional diversity of the macrobenthic community were analyzed in each open-slope area (Table S3). Diversity was assessed by 3 measures: richness of macrofaunal higher taxa (no. of taxa), species richness (SR) in each box-corer sample, and expected number of species in each sample of 50 individuals (ES(n)) (Table 1). The SR index and the rarefaction index were both calculated, because they are sensitive to low abundances (Carney, 1997); in particular, the latter measure provides a useful tool to compare species richness among samples with different total abundances or from different surface areas (Soetaert and Heip, 1990). On the other hand, SR incorporates macrofaunal abundance.
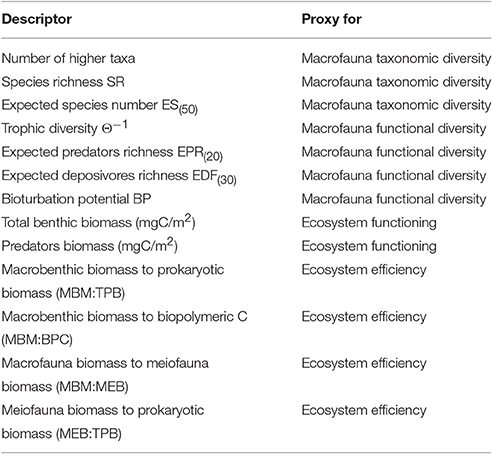
Table 1. List of macrofaunal structural and functional diversity proxies and ecosystem functioning and efficiency proxies used in the present study.
Functional diversity was assessed using 4 indices (Table 1): trophic diversity (Θ−1), expected number of deposit feeders (EDF(30)), expected number of predator species (EPR(20)), and bioturbation potential (BP) (Baldrighi and Manini, 2015; Quéiros et al., 2015). The trophic diversity index was included, because affinity to a given trophic group entails a discrete selection of food sources, hence a different ecological role (Dunne et al., 2002), and feeding mechanisms are held to be central processes structuring marine ecosystems and providing a strong link between species and ecosystem functions (Pearson and Rosenberg, 1987). The number of predator species feeding on macrobenthic organisms was calculated, because the number of species at the top of the benthic food web may reflect a higher functional diversity of the entire benthic assemblage (Ngai and Srivastava, 2006). The number of deposit feeders was included, because they were the most numerous trophic group in our macrofauna samples, and deposit feeding is one of the most effective feeding strategies in environments with generally meager food sources, such as the deep sea (Gage and Tyler, 1991). Bioturbation activity on and in the seafloor, is closely related to the way organisms feed and move (Welsh, 2003) and it is assumed to have an influence on benthic processes (Belly and Snelgrove, 2016). Indeed, we considered the macrofaunal bioturbation activity to be an important functional trait. In the present work, we estimated the macrofaunal bioturbation potential according to the formula reported in Quéiros et al. (2015):
where Bi and Ai are the biomass and abundance of species/taxon i in a sample, Mi is mobility and Ri is sediment reworking. Trait scores of different macrofaunal organisms were assigned following the table of values suggested by Queirós et al. (2013), based on an extensive review of published material, and by Shields and Hughes (2009).
Ecosystem Functioning and Efficiency
Deep-sea ecosystem functioning was estimated as benthic faunal biomass (mgC m−2), considering total benthic biomass (the sum of prokaryotic, meiofaunal, and macrofaunal biomass) and the biomass of the functional group of macrobenthic predators (Table S4). Standing biomass is a metric of ecosystem functioning (e.g., Mora et al., 2011), and a reduction in the size of the predator population may exert effects that go beyond top-down control, thereby affecting cross-system connectivity and ecosystem stability (McCauley et al., 2015). Four indicators were used to assess ecosystem efficiency (Table 1): (i) the ratio of macrofaunal biomass to biopolymeric carbon content (MBM: BPC), a measure of the ability of the system to channel detritus to higher trophic levels (Danovaro, 2012); (ii) the ratio of macrofaunal biomass to prokaryotic biomass (MBM:TPB); (iii) the ratio of macrofaunal biomass to meiofaunal biomass (MBM:MEB); and (iv) the ratio of meiofaunal biomass to prokaryotic biomass (MEB:TPB). Several deep-sea macrobenthic organisms are considered to be deposit feeders because they ingest large amounts of sediment together with detritus, prokaryotes, and meiofauna (Gage and Tyler, 1991). It has been suggested that up to 24% of total bacterial production is grazed by macrofauna, and the meiofauna are assumed to be an important link between smaller (e.g., bacteria) and larger organisms (e.g., macrofauna) (Van Oevelen et al., 2006). The MBM:TPB and MBM:MEB ratios are thus measures of the energy transferred from lower to higher trophic levels based on the hypothesis that macrofauna prey upon microbial and meiofaunal components. The MEB:TPB ratio was included because meiofauna production greatly contributes to total secondary production, particularly in deep-sea systems, where smaller organisms take over in term of standing stock (Rex et al., 2006).
Statistical Analysis
The presence and nature of BEF relationships can be affected by the spatial scale of the investigation and by the environmental factors acting on each scale (Gamfeldt et al., 2014). The presence of a BEF relationship was investigated on two scales: (i) a large spatial scale encompassing the entire dataset, where the data from all 3 research areas, 7 open slopes, and 3 depths were pooled and subjected to statistical analysis; and (ii) a basin scale, where the dataset from each sampling area (ATL, wM, and c-eM) was analyzed separately. The relationships among BEF, biodiversity and ecosystem efficiency were estimated by a linear model (in the form y = a+bx), a power model (y = a+xb), and an exponential model (y = ea+bx). These models are considered to be the best tools to describe BEF relationships in different deep-sea environments (Cardinale et al., 2007; Danovaro et al., 2008a; Lefcheck and Duffy, 2014). Statistical analysis was performed using R-cran software (http://www.R-project.org). Map plots were drawn using Ocean Data View (Schlitzer, 2011). Relationships between variables were tested using linear and non-linear regression. After fitting the three models to the experimental data, the distribution of the residuals, r2, and the Akaike Information Criterion (Akaike, 1974) were used to discriminate the best-fitting model, as appropriate. Model fitting was performed for the large scale, (i.e., the entire dataset), and the basin scale, [i.e., each sampling area (ATL, wM, and c-eM)]. Distance-based multivariate regression analysis with forward selection (DISTLM) (Anderson, 2004) was used to account for the potential effect of environmental variables on the BEF relationship. The effects of depth, longitude, temperature, grain size, and food source quantity and quality were included as covariates in the analyses. In brief, environmental covariates were assessed independently against biodiversity and ecosystem functioning (BEF) variables using a multivariate linear regression model before testing for BEF relationships. P-values for each covariate were obtained with 4,999 permutations of residuals under the reduced model (Anderson, 2001).
In addition, the collinearity between the descriptors of taxonomic and functional diversity was assessed using the Variance Inflation Factor (VIF) test (O'Brien, 2007). Based on the rule of 10, which is commonly used in statistical analysis (O'Brien, 2007), VIF values were greater than 3.0, indicating the lack of a strong correlation between our diversity indices. However, use of a threshold value of 2.50 (which corresponds to an R2 > 0.50), as recommended by some authors (e.g., Quinn and Keough, 2009), identified significant correlations between SR and two descriptors: EPR and EDF.
Results
Relationship between Biodiversity and Functioning on the Large Spatial Scale
The presence of a BEF relationship on the large spatial scale was investigated by testing the effect of different structural and functional macrofauna diversity indices on ecosystem functioning and efficiency proxies (Table 2A). The assessment of collinearity between structural and functional diversity indices with the VIF test using a threshold level of 2.5, indicated that SR exhibited a significant degree of collinearity with EPR(20) and EDF(30) (VIF = 2.7 and 2.8, respectively). This finding was expected because EPR(20) and EDF(30) are both related to the number of species (SR). Analysis of the full dataset (ATL, wM, and c-eM, see Table S5 for total macrofauna abundances) showed that macrofaunal SR was the only diversity index to be positively related to total benthic biomass, and that ES(50) was related to macrobenthic predator biomass (Figure 2A, Table 3). The exponential model was the one that best described these relationships (Tables 2A, 3). The relationships between other diversity indices and benthic biomass were explained by the environmental cofactors (water depth, longitude, food availability, and grain size; Table 4A).

Table 2. Complete list of significant and non-significant BEF relations reported (A) on large spatial scale and (B) on basin spatial scale.
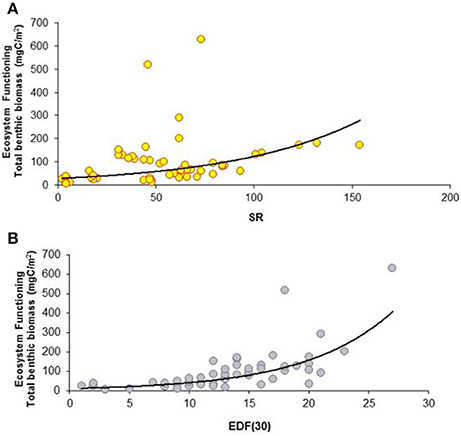
Figure 2. Large spatial-scale relationships between macrofaunal biodiversity and ecosystem functioning and efficiency. (A) Relationship between species richness (SR) and ecosystem functioning expressed as total benthic biomass (mgC/m2). The equation of the fitting line is y = e∧(−1.08+0.13x) (N = 64; R2 = 0.98; P < 0.001). (B) Relationship between functional diversity, expressed as expected richness of deposit feeders (EDF(30)), and ecosystem functioning (total benthic biomass). The equation of the fitting line is y = e∧(2.64−0.16x) (N = 64; R2 = 0.89; P < 0.001).
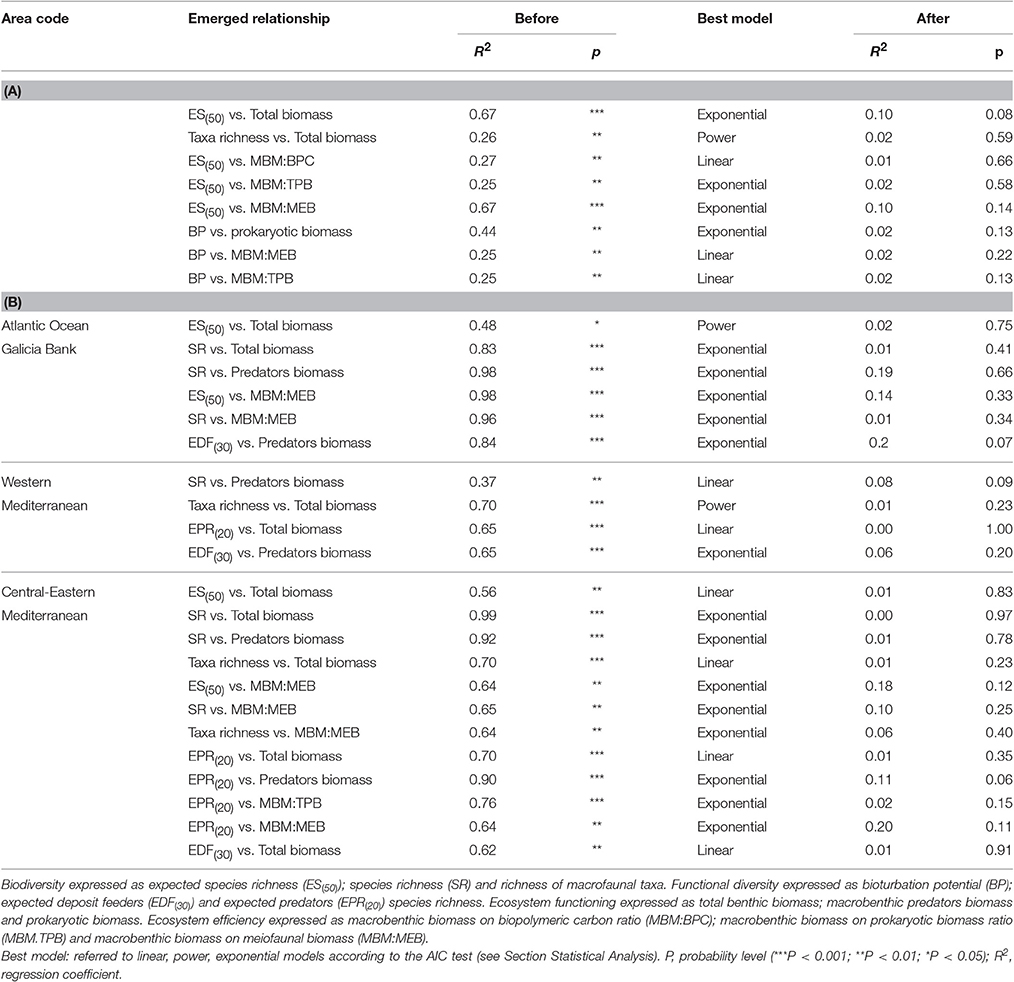
Table 4. Biodiversity and functional diversity effects on ecosystem functioning and efficiency, before and after covariables removal, at (A) large spatial scale and (B) basin- spatial scale.
The energy flow through the biotic ecosystem was quantified using the ratio of macrobenthic biomass to the amount of biopolymeric carbon (MBM:BPC), the ratio of macrofaunal biomass to prokaryotic biomass (MBM:TPB), the ratio of macrofaunal biomass to meiofaunal biomass (MBM: MEB), and the ratio of meiofaunal biomass to prokaryotic biomass (MEB:TPB) as proxies. On the large scale, macrobenthic biodiversity was not significantly related to any of these proxies of ecosystem efficiency (Table 2A), and most of its variability was explained by environmental covariates (Table 4A).
Macrofaunal functional diversity was expressed as trophic diversity, EDF(30), EPR(20), and BP. A BEF relationship was found only when EDF(30) was considered, and it was significant and exponential (Figure 2B, Tables 2A, 3). None of the other functional diversity indices used had any effect on ecosystem functioning, or else the relationships were explained by a covariate effect (Table 4A).
On the large spatial scale, BP was the only functional parameter to be positively and linearly correlated with ecosystem efficiency as measured by the MBM:BPC ratio (Tables 2A, 3). In all the other cases (Table 4A), its effect was overridden by covariate effects.
In summary, 4 of the 42 BEF relationships tested in the study indicated that macrofauna diversity significantly affected ecosystem functioning and efficiency (Table 2A). However, the collinearity between SR and EDF(30) suggests that their number may in fact fall to 3/42.
Basin-Scale BEF Relationships
The relationships identified on the basin scale among macrofaunal structural and functional diversity, and ecosystem functioning and efficiency are reported in Table 2B.
Each slope system yielded different results (Table 5). The number of significant relationships was highest in the w-M basin, where macrofaunal diversity was positively related to ecosystem function and efficiency (Figure 3, Table 5). In the other slope areas, the effect of the environmental variables attenuated the BEF relationships (Table 4B). The nature of the relationships ranged from linear to exponential, according to the proxies that were applied to quantify biodiversity (Table 2B). In regard to macrofaunal functional diversity, significant and exponential relationships were found among EPR(20), EDF(30) and ecosystem functioning in the w-M and ATL area (Figures 4A–C, Table 5). In the c-eM basin, macrofauna diversity did not significantly affect ecosystem functioning and efficiency (Table 2B). Macrofaunal functional diversity only showed an exponential relationship with the MBM:MEB ratio, one of the proxies of ecosystem efficiency, and only in the wM basin (Table 5). A null relationship, that was mostly explained by the effect of environmental factors in the c-eM basin, was found in the ATL area (Table 4B). According to the present findings, the environmental variables governed all the BEF relationships in the c-eM basin and most of the relationships detected in the ATL area (Table 4B). In the wM slope area the environmental effect seemed to be slightly less marked despite the small number of significant relationships out of the number of BEF relationships assessed (Table 2B).
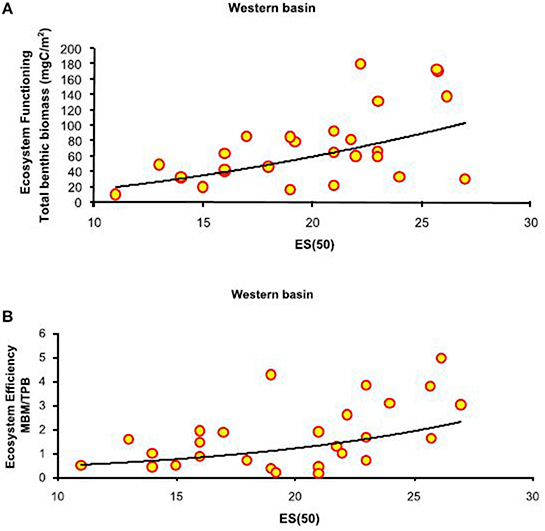
Figure 3. Basin-scale relationships between macrofauna biodiversity and ecosystem functioning and efficiency. (A) Relationship between expected species richness (ES(50)) and ecosystem functioning, expressed as total benthic biomass (mgC/m2). The equation of the fitting line is y = x∧1.43 (N = 27; R2 = 0.32; P < 0.01). (B) Relationship between expected species richness (ES(50)) and ecosystem efficiency, expressed as macrobenthic biomass to prokaryotic biomass (MBM: TPB). The equation of the fitting line is y = e∧(−1.90+0.12x) (N = 27; R2 = 0.33; p < 0.01).
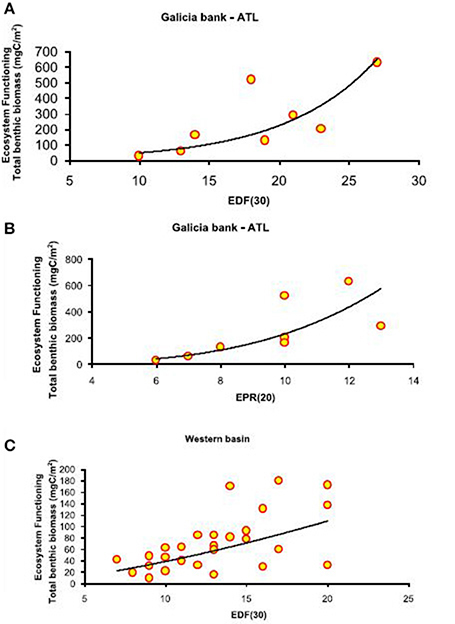
Figure 4. Basin-scale relationships between macrofauna functional diversity and ecosystem functioning. Relationship between functional diversity, expressed as expected richness of deposit feeders (EDF(30)) and expected predator richness (EPR(20)), and ecosystem functioning, expressed as total benthic biomass (mgC/m2). The equations of the fitting line are respectively (A) y = e∧(6.67−4.83x) (N = 9; R2 = 0.98; p < 0.01) and (B) y = x∧2.71 (N = 9; R2 = 0.61; p < 0.05) in the Atlantic Ocean and (C) y = e∧(−1.60+2.82x) (N = 27; R2 = 0.98; p < 0.01) in the Western Mediterranean basin.
Discussion
Does Large Spatial-Scale Sampling Hamper Identification of BEF Relationships?
Continental slopes are ideal sites to investigate BEF relationships, since they account for more than 20% of total marine productivity and for a significant proportion of the organic matter exports to the seafloor (Levin and Sibuet, 2012). Slope sediments host a large quantity of marine biodiversity and are repositories of deep-sea biomass, and their benthic communities are characterized by a high spatial heterogeneity (Coll et al., 2010). The factors commonly invoked as drivers of the spatial distribution and diversity of deep-sea benthic fauna include substrate heterogeneity (Etter and Grassle, 1992), water circulation (Tyler, 2003), oxygen availability (Levin and Sibuet, 2012), productivity and microbial activity (Tyler, 2003), and food resources (Menot et al., 2010). Since the stations sampled in this study lay along a west-east axis encompassing different trophic and oceanographic conditions, environmental factors were expected to influence BEF relationships. The large spatial-scale analysis, spanning the area from the Atlantic Ocean to the Central-Eastern Mediterranean Sea, detected two significant and exponential relationships between macrofaunal diversity and ecosystem functioning. An exponential relationship between BEF has been described for various organism size classes (Mora et al., 2014); positive interspecies interactions between organisms, such as facilitation, have been suggested to sustain such relationships (Danovaro et al., 2008a). However, the diversity indices used in the present study were not all significantly related to the ecosystem functioning measures. In fact, the existence of a BEF relationship appeared to be closely linked to the diversity and ecosystem functioning measures used (Gamfeldt et al., 2014), which are often context-dependent (O'Connor and Donohue, 2013). These findings are in line with other work (Pape et al., 2013; Cusson et al., 2015; Poorter et al., 2015), where not all diversity measures correlated with ecosystem functioning.
On the large spatial scale, macrofaunal diversity—ecosystem efficiency relations were not significant. We chose our ecosystem efficiency proxies following the idea that the positive influence exerted by biodiversity on ecosystem efficiency can lead to an efficient use of available food sources and their conversion to higher biomass values (Naeem et al., 1994). Indeed, the ratio of macrobenthic biomass to biopolymeric carbon has been suggested as a proxy of ecosystem efficiency (Danovaro, 2012). This proxy has been reported to have both a positive relationship with benthic diversity (Danovaro et al., 2008a; Zeppilli et al., 2016) and no relationship at all (Leduc et al., 2013). The ratio of macrobenthic to microbial biomass and of macrofaunal to meiofauna biomass can also be employed as proxies to quantify the energy flow through an ecosystem, i.e., how efficiently it works (Cardinale et al., 2012): the higher the ratio, the more efficient the system. Similarly, the meiofaunal biomass to prokaryotic biomass ratio was used as a further proxy of ecosystem efficiency, because of the efficient carbon recycling and strong contribution to total secondary production provided by the meiofauna (De Mesel et al., 2006). Yet, all these proxies may be a gross oversimplification, because the energy flow through an ecosystem is a complex and commonly indirect flow from the smaller to the larger organisms, and is influenced by numerous biotic interactions (Piot et al., 2014), abiotic variables (Snelgrove et al., 2014; Tilman et al., 2014) and the life history of present organisms (Cusson and Bourget, 2005). This may explain the lack of significant diversity—ecosystem efficiency relationships. Moreover, we hypothesize that including a larger number of sites could lead to potentially different BEF relationships.
Because functional diversity provides a direct, mechanistic link between diversity and ecosystem functioning, numerous studies have found that functional diversity affects ecosystem functioning more strongly than taxon diversity (Reiss et al., 2009; Mineur et al., 2014). The functional diversity of organisms can be expressed in several ways, and different effects on ecosystem functioning can be identified depending on the measure employed (Naeem et al., 1995; Hiddink et al., 2009). Most of the functional diversity indices used in this study rely on the trophic diversity of macrobenthic organisms [trophic diversity index, EDF(30) and EPR(20)], a key functional trait (Dunne et al., 2002; Carvalho et al., 2013). A significant and exponential BEF relationship was detected between EDF(30) and total benthic biomass, and it was also identified between SR and total biomass. This finding may be wholly explained by the collinearity between SR and EDF(30). Unexpectedly, however, while collinearity existed there was no relationship between EPR(20) and benthic biomass. This can probably be attributed to the fact that deposit feeders, which are the most abundant trophic group in the samples collected, may play a far from negligible role in ecosystem functioning. No relationship, but merely a weak positive trend, was detected between EPR(20) and predator biomass; indeed, higher numbers of predator species did not correlate with higher biomass values. Moreover, there was no correlation between predator number (ind m−2) and their biomass (R2 = 0.03, p > 0.05); in particular, the wM slope systems were characterized by a large number of predators and a high EPR(20), whereas their biomass values were lower than those measured in the Atlantic slope area. Mediterranean benthic organisms have been reported to have a generally smaller size than those inhabiting the Atlantic Ocean (Sardà et al., 2004; Tecchio et al., 2011; Pape et al., 2013), and significantly lower macrobenthic biomass values have been described in the Mediterranean basin compared with the Atlantic sector (Tselepides et al., 2000; Coll et al., 2010; Baldrighi et al., 2014). The bioturbation activity of organisms can affect both the abiotic and biotic components of a system (Quéiros et al., 2015), and is one of the functional traits of benthic organisms that may sustain mutualistic interactions on the basis of BEF relationships (Loreau, 2008). On the large spatial scale, bioturbation was positively and linearly correlated with ecosystem efficiency. The biogenic activities (e.g., burrowing, reworking, and displacing of sediment particles) of benthic organisms are fundamental processes with implications for a wide range of ecosystem-related functions, such as organic matter recycling (Thurber et al., 2014). The linear relationship identified in this study indicates that all organisms contribute similarly to ecosystem efficiency (Naeem et al., 1995). This finding seems to support the notion that bioturbation facilitates organic matter recycling and uptake by higher trophic levels (Quéiros et al., 2015).
On the large spatial scale, the significant relationships we identified between macrofauna's structural and functional diversity and ecosystem functioning and efficiency were positive and linear or exponential. This result is in line with previous findings (e.g., Mora et al., 2014; Zeppilli et al., 2016), supporting the idea of a positive influence of deep-sea (macro-) faunal diversity on the functioning of ecosystem. An assumption of this work was that the steep environmental gradients characterizing the Atlantic—Central-Eastern Mediterranean transect would influence large-scale BEF relationships (Cusson et al., 2015). Since the values of BEF measures can be affected by environmental variables, it is conceivable that BEF relationships reflect the covariation of different factors, as found by the present study. Indeed, temperature, food availability, and substrate heterogeneity are among the features that determine the distribution and diversity of deep-sea benthic populations on the broad spatial scale (Levin et al., 2001). Similarly, the lack of significant associations between functional diversity (except for EDF(30)) and ecosystem functioning found in this study may therefore be due to the influence of environmental variables on the majority of macrofaunal functional and ecosystem functioning measures (Cardinale et al., 2000; Hiddink et al., 2009). Belly and Snelgrove (2016) showed how macrofaunal functional diversity and environmental variables can equally explain the majority of variability in some key ecosystem processes. Most deep-sea communities are usually food-limited (Levin et al., 2001), and their reliance on the relatively small input of organic carbon from the surface and the particulate organic carbon flux are held to exert a strong influence on deep-sea biodiversity (Tittensor et al., 2011) and ecosystem function (Smith et al., 2008). Moreover, besides food availability, gradients in other drivers such as sediment heterogeneity, oxygen availability, and hydrodynamic regimes (Levin et al., 2001) are also capable of affecting local diversity by influencing the rates of local processes (Rex and Etter, 2010) and consequently BEF relationships.
Notably, the functional diversity indices employed did not describe ecosystem functioning more exhaustively than the traditional biodiversity indices. A possible explanation for this is that they do not encompass the full array of key macrobenthic functional traits that underpin ecosystem functioning and efficiency processes. Yet another more targeted approach, which focuses on biological traits analysis and has been proposed for the study of functional diversity (Bremner et al., 2003; Bremner, 2008; Bolam and Eggleton, 2014), also entails some limitations since our understanding of benthic functional diversity is still scant (Bolam et al., 2010). Furthermore, identification of the traits that have a direct and indirect functional role in ecosystems is highly problematic (Pakeman, 2011). The limitations are even greater where deep-sea organisms are concerned, given the poor state of current knowledge regarding their diversity and functional diversity (e.g., Smith et al., 2008; Ramirez-Llodra et al., 2010; Levin et al., 2015).
BEF Relationships on the Basin Spatial Scale
It has been suggested that BEF relationships are spatial-scale- and context-dependent (Tylianakis et al., 2008; Zeppilli et al., 2016), and that their nature is related to the system analyzed and the organisms involved (Ieno et al., 2006; Poorter et al., 2015). Analysis of the basin scale data documented a slightly different situation for each of the three slope systems. In the w-M basin, macrofaunal diversity exerted a positive effect on ecosystem function and efficiency, whereas in the other areas the effect of environmental variables explained all (c-eM basin) or nearly all (ATL) BEF relationships. The nature of the relationships identified in the Western Mediterranean basin ranged from linear to exponential, depending on the proxies that were applied to quantify biodiversity. However, irrespective of their nature, some BEF relationships were detected in the western basin but not on the large scale. According to Barnes and Hamylton (2015), the spatial scales on which biodiversity interacts with ecosystem functioning are crucial to understand the significance of BEF relationships. Also in our case, it may be speculated that some BEF relationships identified on the basin scale (the w-M basin) were not detected on the larger scale due to a masking effect exerted by environmental features. Environmental drivers, number of species, and functional diversity affect ecosystem functioning in different ways and with different strength, based on the spatial scale (Cardinale et al., 2007; Poorter et al., 2015). However, a significant relationship between macrofauna diversity and ecosystem functioning was not found in the other two areas (ATL and c-eM basin), as on the large scale. In the deep Mediterranean Sea, the west-east gradient of decreasing surface water productivity is reflected by both a decreasing amount of food reaching the seafloor (D'Ortenzio and Ribera d'Alcalà, 2009), and in a decreasing abundance of deep-sea benthic populations (Coll et al., 2010; see also Table 2). The environmental effects were strong in the c-eM basin, where most relationships were context-dependent. Indeed, environmental conditions, such as food depletion or current regime have been reported to be major factors influencing and structuring benthic populations in the Eastern Mediterranean (Tselepides et al., 2000; Króncke et al., 2003; Tecchio et al., 2011; Giovannelli et al., 2013). Furthermore, Zeppilli et al. (2016) showed that the high sea floor heterogeneity along an open slope system may shape BEF relationships, even on a very small spatial scale. In our study, we considered seven slope systems spread across a wide longitudinal range from the Atlantic Ocean to the east Mediterranean basin. The heterogeneity of the substrate was assessed through the grain size analysis, but we know that granulometric analysis cannot completely catch the seafloor morphologies even on a small spatial scale. Indeed, these seafloor structures are pivotal in shaping the BEF relations, by selecting the species responsible for the effect of ecosystem functioning (Zeppilli et al., 2016). Nonetheless, the presence and shape of BEF relationships can vary when different components (meiofauna, macrofauna, fish) are taken into account (Pusceddu et al., 2014a). This suggests that different environments (i.e., basins) may involve different functional structures of macrobenthic communities. O'Connor and Crowe (2005) concluded that different species play idiosyncratic roles, explaining why no relationship is found between species richness and ecosystem functioning in some cases. Furthermore, the inclusion of more sampling sites in the Atlantic area might lead to different BEF relationships.
Final Remarks
In the present study, some significant relationships between macrofaunal diversity and ecosystem functioning and efficiency were reported. The nature of BEF relations was positive and exponential or linear, as already documented in various systems at different spatial and time scales and encompassing diverse faunal components and systems (e.g., Mora et al., 2014; Pusceddu et al., 2014a; Yasuhara et al., 2016). The role of positive ecological interactions in ecosystem functioning and efficiency provides an explanation for a positive and exponential BEF relation (Cardinale et al., 2002). Many other relationships emerged between macrofaunal BEF and efficiency, and they were influenced by the effect of environmental variables on both large and basin scales. Another aspect that deserves consideration is that the the majority of deep-sea investigations, like the present study, are time-scale limited. Recent experiments (Gravel et al., 2010; Reich et al., 2012) and studies (Yasuhara et al., 2016) have shown that evolutionary and long-term responses may have effects on BEF relationships.
Functional diversity as assessed in the present study did not appear to be more effective in influencing ecosystem processes than is structural diversity per se. The challenge for future investigations is to identify functional traits that affect ecosystem processes in multiple environmental contexts.
It is generally assumed that the vast majority of species in the deep sea are rare (Gaston, 1994). In general, the number of rare species in marine systems seems to increase with within-site and between-site heterogeneity, and to be characterized by habitat-specific species with restricted ranges (Mouillot et al., 2013). Key ecosystem processes may be threatened by the loss of species, many of which may be “rare” and perform specific functions (Mouillot et al., 2013). Some studies have reported that their loss can in fact influence local ecosystem processes and that rare species can contribute significantly to long-term and large-scale ecosystem functioning (Lyons et al., 2005). Altogether, the consequences of losing rare species on ecosystem functioning are still to be explored (Jain et al., 2014). We believed that further research devoted to the investigation of the effect of biodiversity, particularly functional diversity, on ecosystem functioning cannot avoid including the issue of “rarity.”
Author Contributions
EB, EM, and ML designed the research; EB and ML performed the research; EB, GD, and DG analyzed the data; EB, and EM wrote the manuscript; EB, GD, and DG prepared tables and figures; EM, GD, ML, and DG critically reviewed the manuscript.
Funding
This study is part of the ESF EuroDEEP project BIOFUN (CTM2007-28739-E); writing of the manuscript was supported by BALMAS (IPA ADRIATIC project; 1uSTR/0005). DG was supported by a C-DEBI (Center for Dark Energy Biosphere Investigation) postdoctoral fellowship.
Conflict of Interest Statement
The handling Editor declared a shared affiliation, though no other collaboration, with one of the authors GD, and the handling Editor states that the process met the standards of a fair and objective review.
The other authors declare that the research was conducted in the absence of any commercial or financial relationships that could be construed as a potential conflict of interest.
Acknowledgments
The authors are grateful to the crews of ships R/V Pelagia (The Netherlands), R/V Urania (Italy), and R/V Meteor (Germany) for their help during sampling activities. The authors acknowledge all the constructive suggestions of two reviewers that helped us to significantly improve an early version of our manuscript. An early version of this paper was submitted to Biogeosciences and rejected. The rejected copy and interactive discussion are available at http://www.biogeosciences-discuss.net/bg-2016-26/.
Supplementary Material
The Supplementary Material for this article can be found online at: http://journal.frontiersin.org/article/10.3389/fmars.2017.00198/full#supplementary-material
References
Akaike, H. (1974). A new look at the statistical model identification. IEEE Trans. Autom. Contr. 19, 716–723. doi: 10.1109/TAC.1974.1100705
Amaro, T., Bianchelli, S., Billett, D. S. M., Cunha, R. M., Pusceddu, A., and Danovaro, R. (2010). The trophic biology of the holothurian Molpadia musculus: implications for organic matter cycling and ecosystem functioning in a deep submarine canyon. Biogeosciences 7, 2419–2432. doi: 10.5194/bg-7-2419-2010
Anderson, M. J. (2001). Permutation tests for univariate or multivariate analysis of variance and regression. Can. J. Fish. Aquat. Sci. 58, 626–639. doi: 10.1139/f01-004
Anderson, M. J. (2004). Dros. Inf. Serv. TLM version 5: A FORTRAN Computer Program to Calculate a Distance-Based Multivariate Analysis for a Linear Model. Auckland: University of Auckland.
Armstrong, C. W., Foley, N. S., Tinch, R., and van den Hove, S. (2012). Services from the deep: steps towards valuation of deep sea goods and services. Ecol. Ser. 2, 2–13. doi: 10.1016/j.ecoser.2012.07.001
Baldrighi, E., Lavaleye, M., Aliani, S., Conversi, A., and Manini, E. (2014). Large spatial scale variability in bathyal macrobenthos abundance, biomass, α- and β- diversity along the Mediterranean continental margin. PLoS ONE 9:e107261. doi: 10.1371/journal.pone.0107261
Baldrighi, E., and Manini, E. (2015). Deep-sea meiofauna and macrofauna diversity and functional diversity: are they related? Mar. Biodiv. 45, 469–488. doi: 10.1007/s12526-015-0333-9
Barnes, S. R. K., and Hamylton, S. (2015). Uniform functional structure across spatial scales in an intertidal benthic assemblage. Mar. Envir. Res. 106, 89–91. doi: 10.1016/j.marenvres.2015.03.006
Belly, R., and Snelgrove, P. V. R. (2016). Relative contribution of biodiversity and environment to benthic ecosystem functioning. Front. Mar. Sci. 3:242. doi: 10.3389/fmars.2016.00242
Bergamasco, A., and Malanotte-Rizzoli, P. (2010). The circulation of the Mediterranean Sea: a historical review of experimental investigations. Adv. Oceanogr. Limnol. 1, 11–28. doi: 10.4081/aiol.2010.5293
Biles, C. L., Solan, M., Isaksson, I., Paterson, D. M., Emes, C., and Raffaelli, D. G. (2003). Flow modifies the effect of biodiversity on ecosystem functioning: an in situ study of estuarine sediments. J. Exper. Mar. Biol. Ecol. 285/286, 165–177. doi: 10.1016/S0022-0981(02)00525-7
Bolam, S. G. (2013). Relationships between Macroinvertebrate Biological Traits and Sea Bed Functioning in European waters. FP7 BENTHIS Task 1.1a Final Report (25 pp.).
Bolam, S. G., Barrio Froján, C. R. S., and Eggleton, J. D. (2010). Macrofaunal production along the UK continental shelf. J. Sea Res. 64, 166–179. doi: 10.1016/j.seares.2010.02.003
Bolam, S. G., and Eggleton, J. D. (2014). Macrofaunal production and biological traits: spatial relationships along the UK continental shelf. J. Sea Res. 88, 47–58. doi: 10.1016/j.seares.2014.01.001
Braeckman, U., Provoost, P., Gribsholt, B., Van Gasbeke, D., Middelburg, J. J., Soetaert, K., et al. (2010). Role of macrofauna functional traits and density in biogeochemical fluxes and bioturbation. Mar. Ecol. Prog. Ser. 399, 173–186. doi: 10.3354/meps08336
Bremner, J. (2008). Species' traits and ecological functioning in marine conservation and management. J. Exp. Mar. Biol. Ecol. 366, 37–47. doi: 10.1016/j.jembe.2008.07.007
Bremner, J., Rogers, S. I., and Frid, C. L. J. (2003). Assessing functional diversity in marine benthic ecosystems: a comparison of approaches. Mar. Ecol. Prog. Ser. 254, 11–25. doi: 10.3354/meps254011
Cardinale, B. J., Duffy, J. E., Gonzalez, A., Hooper, D. U., Perrings, C., Venail, P., et al. (2012). Biodiversity loss and its impact on humanity. Nature 486, 59–67. doi: 10.1038/nature11148
Cardinale, B. J., Matulich, K. M., Hooper, D. U., Byrnes, J. E., Duffy, E., Gamfeldt, L., et al. (2011). The functional role of producer diversity in ecosystems. Am. J. Bot. 98, 572–592. doi: 10.3732/ajb.1000364
Cardinale, B. J., Nelson, K., and Palmer, M. A. (2000). Linking species diversity to the functioning of ecosystems: on the importance of environmental context. Oikos 91, 175–183. doi: 10.1034/j.1600-0706.2000.910117.x
Cardinale, B. J., Palmer, M. A., and Collins, S. L. (2002). Species diversity enhances ecosystem functioning through interspecific facilitation. Nature 415, 426–429. doi: 10.1038/415426a
Cardinale, B. J., Wright, J. P., Cadotte, M. W., Carroll, I. T., Hector, A., Srivastava, D. S., et al. (2007). Impacts of plant diversity on biomass production increase through time because of species complementarity. Proc. Natl. Acad. Sci. U.S.A. 104, 18123–18128. doi: 10.1073/pnas.0709069104
Carney, R. S. (1997). Basing conservation policies for the deep-sea floor on current-diversity concepts: a consideration of rarity. Biodiv. Conserv. 6, 1463–1485. doi: 10.1023/A:1018310302215
Carvalho, R., Wei, C. L., Rowe, G., and Schulze, A. (2013). Complex depth-related patterns in taxonomic and functional diversity of polychaetes in the Gulf of Mexico. Deep-Sea Res. I. 80, 66–77. doi: 10.1016/j.dsr.2013.07.002
Coll, M., Piroddi, C., Steenbeek, J., Kaschner, K., Ben Rais Lasram, F., Aguzzi, J., et al. (2010). The biodiversity of the mediterranean sea: estimates, patterns, and threats. PLoS ONE 5:e11842. doi: 10.1371/journal.pone.0011842
Cusson, M., and Bourget, E. (2005). Global patterns of macroinvertebrate production in marine benthic habitats. Mar. Ecol. Prog. Ser. 297, 1–14. doi: 10.3354/meps297001
Cusson, M., Crowe, T. P., Araújo, R., Arenas, F., Aspden, R., Bulleri, F., et al. (2015). Relationships between biodiversity and the stability of marine ecosystems: comparisons at a European scale using meta-analysis. J. Sea Res. 98, 5–14. doi: 10.1016/j.seares.2014.08.004
D'Ortenzio, F., and Ribera d'Alcalà, M. (2009). On the trophic regimes of the Mediterranean Sea: a satellite analysis. Biogeosciences 6, 139–148. doi: 10.5194/bg-6-139-2009
Danovaro, R. (2010). Methods for the Study of Deep-Sea Sediments, Their Functioning and Biodiversity. New York, NY: Taylor & Francis Group.
Danovaro, R. (2012). “Extending the approaches of biodiversity and ecosystem functioning to the deep ocean,” in Marine Biodiversity and Ecosystem Functioning: Frameworks, Methodologies and Integration, eds M. Solan, R. J. Aspden, and D. M. Paterson (Oxford: Oxford University Press), 113–124.
Danovaro, R., Dell'Anno, A., Corinaldesi, C., Magagnini, M., Noble, R., Tamburini, C., et al. (2008b). Major viral impact on the functioning of benthic deep-sea ecosystems. Nature 454, 1084–1088. doi: 10.1038/nature07268
Danovaro, R., Gambi, C., Dell'Anno, A., Corinaldesi, C., Fraschetti, S., Vanreusel, A., et al. (2008a). Exponential decline of deep-sea ecosystem functioning linked to benthic biodiversity loss. Curr. Biol. 18, 1–8. doi: 10.1016/j.cub.2007.11.056
De Jonge, V. N. (1980). Fluctuations in the organic carbon to chlorophyll a ratios for estuarine benthic diatom populations. Mar. Ecol. Prog. Ser. 2, 345–353. doi: 10.3354/meps002345
De Mesel, I., Derycke, S., Swings, J., Vincx, M., and Moens, T. (2006). Role of nematodes in decomposition processes: does within trophic group diversity matter? Mar. Ecol.Prog. Ser. 321, 157–166. doi: 10.3354/meps321157
Dunne, J. A., Williams, R. J., and Martinez, N. D. (2002). Network structure and biodiversity loss in food webs: robustness increases with connectance. Ecol. Lett. 5, 558–567. doi: 10.1046/j.1461-0248.2002.00354.x
Etter, R. J., and Grassle, J. F. (1992). Patterns of species diversity in the deep sea as a function of sediment particle size diversity. Nature 360, 576–578. doi: 10.1038/360576a0
Fabiano, M., Danovaro, R., and Fraschetti, S. (1995). A three-year time series of elemental and biochemical composition of organic matter in subtidal sandy sediments of the Ligurian Sea (northwestern Mediterranean). Cont. Shelf Res. 15, 1453–1469. doi: 10.1016/0278-4343(94)00088-5
Fry, J. C. (1990). Determination of Biomass. Methods in Aquatic Bacteriology. New York, NY: John Wiley & Sons.
Gage, J. D., and Tyler, P. A. (1991). Deep-Sea Biology: A Natural History of Organisms at the Deep Sea Floor. Cambridge: Cambridge University Press.
Gamfeldt, L., Lefcheck, J. S., Byrnes, J. E. K., Cardinale, B. J., Duffy, J. E., and Griffin, J. N. (2014). Marine biodiversity and ecosystem functioning: what's known and what's next? OIKOS 124, 252–265. doi: 10.1111/oik.01549
Giovannelli, D., Molari, M., d'Errico, G., Baldrighi, E., Pala, C., and Manini, E. (2013). Large-scale distribution and activity of prokaryotes in deep-sea surface sediments of the Mediterranean sea and the adjacent Atlantic Ocean. PLoS ONE 8:e72996. doi: 10.1371/journal.pone.0072996
Grassle, J. F., and Maciolek, N. J. (1992). Deep-sea species richness: regional and local diversity estimates from quantitative bottom samples. Am. Natural. 139, 313–341. doi: 10.1086/285329
Gravel, D., Bell, T., Barbera, C., Bouvier, T., Pommier, T., Venail, P., et al. (2010). Experimental niche evolution alters the strength of the diversity productivity relationship. Nature 469, 89–92. doi: 10.1038/nature09592
Hiddink, J. G., Wynter Davies, T., Perkins, M., Machairopoulou, M., and Neill, S. P. (2009). Context dependency of relationships between biodiversity and ecosystem functioning is different for multiple ecosystem functions. Oikos 118, 1892–1900. doi: 10.1111/j.1600-0706.2009.17556.x
Hooper, D. U., Chapin, F. S. III, Ewel, J. J., Hector, A., Inchausti, P., Lawton, J. H., et al. (2005). Effects of biodiversity on ecosystem functioning: a consensus of current knowledge. Ecol. Monogr. 75, 3–35. doi: 10.1890/04-0922
Ieno, E. N., Solan, M., Batty, P., and Pierce, G. J. (2006). How biodiversity affects ecosystem functioning: roles of infaunal species richness, identity and density in the marine benthos. Mar. Ecol. Progr. Ser. 31, 263–271. doi: 10.3354/meps311263
Jain, M., Flynn, D. F. B., Prager, C. M., Hart, G. M., DeVan, C. M., Ahrestani, F. S., et al. (2014). The importance of rare species: a trait-based assessment of rare species contributions to functional diversity and possible ecosystem function in tall-grass prairies. Ecol. Evol. 4, 104–112. doi: 10.1002/ece3.915
Jax, K. (2005). Function and “functioning” in ecology: what does it mean? Oikos 111, 641–648. doi: 10.1111/j.1600-0706.2005.13851.x
Króncke, I., Türkay, M., and Fiege, D. (2003). Macrofauna communities in the eastern Mediterranean deep sea. Mar. Ecol. 24, 193–206. doi: 10.1046/j.0173-9565.2003.00825.x
Leduc, D., Rowden, A. A., Pilditch, C. A., Maas, E. W., and Probert, P. K. (2013). Is there a link between deep-sea biodiversity and ecosystem function? Mar. Ecol. 34, 334–344. doi: 10.1111/maec.12019
Lefcheck, J. S., and Duffy, J. E. (2014). Multitrophic functional diversity predicts ecosystem functioning in experimental assemblages of estuarine consumers. PeerJ. PrePrints 2:e540v1. doi: 10.7287/peerj.preprints.540v1
Lefcheck, J. S., and Duffy, J. E. (2015). Multitrophic functional diversity predicts ecosystem functioning in experimental assemblages of estuarine consumers. Ecology 96, 2973–2983. doi: 10.1890/14-1977.1
Levin, L. A., Etter, R. J., Rex, M. A., Gooday, A. J., Smith, C. R., and Pineda, J. (2001). Environmental influences on regional deep-sea species diversity. Annu. Rev. Ecol. Sys. 32, 51–93. doi: 10.1146/annurev.ecolsys.32.081501.114002
Levin, L. A., James, D. W., Martin, C. M., Rathburn, A. E., Harris, L. H., and Michener, R. H. (2000). Do methane seeps support distinct macrofaunal assemblages? Observations on community structure and nutrition from the northern California slope and shelf. Mar. Ecol. Prog. Ser. 208, 21–39. doi: 10.3354/meps208021
Levin, L. A., Liu, K.-K., Emeis, K.-C., Breitburg, D. L., Cloern, J., Deutsch, C., et al. (2015). Comparative biogeochemistry-ecosystem-human interactions on dynamic continental margins. J. Mar. Syst. 141, 3–17. doi: 10.1016/j.jmarsys.2014.04.016
Levin, L. A., and Sibuet, M. (2012). understanding continental margin biodiversity: a new imperative. Ann. Rev. Mar. Sci. 4, 79–112. doi: 10.1146/annurev-marine-120709-142714
Loreau, M. (2000). Biodiversity and ecosystem functioning: recent theoretical advances. Oikos 91, 3–17. doi: 10.1034/j.1600-0706.2000.910101.x
Loreau, M. (2008). Biodiversity and ecosystem functioning: the mystery of the deep sea. Curr. Biol. 18, 126–128. doi: 10.1016/j.cub.2007.11.060
Loreau, M. (2010). Linking biodiversity and ecosystems: towards a unifying ecological theory. Phil. Tran. R. Soc. B. 365, 49–60. doi: 10.1098/rstb.2009.0155
Luna, G. M., Manini, E., and Danovaro, R. (2002). Large fraction of dead and inactive bacteria in coastal marine sediments: comparison of protocols for determination and ecological significance. Appl. Environ. Microbiol. 68, 3509–3513. doi: 10.1128/AEM.68.7.3509-3513.2002
Lyons, K. G., Brigham, C. A., Traut, B. H., and Schwartz, M. W. (2005). Rare species and ecosystem functioning. Conserv. Biol. 19, 1019–1024. doi: 10.1111/j.1523-1739.2005.00106.x
McCauley, D. J., Pinsky, M. L., Palumbi, S. R., Estes, J. A., Joyce, F. H., and Warner, R. R. (2015). Marine defaunation: animal loss in the global ocean. Science 347:1255641. doi: 10.1126/science.1255641
Menot, L., Sibuet, M., Carney, R. S., Levin, L. A., Rowe, G. T., Billett, D. S. M., et al. (2010). “New perceptions of continental margin biodiversity,” in Life in the World's Oceans, ed A. D. McIntyre (Oxford: Wiley-Blackwell), 79–102.
Meysman, F. G. R., Middelburg, J. J., and Heip, C. H. R. (2006). Bioturbation: a fresh look at Darwin's last idea. Trends Ecol. Evol. 21, 687–695. doi: 10.1016/j.tree.2006.08.002
Miller, R. J., Hocevar, J., Stone, R. P., and Fedorov, D. V. (2012). Structure-forming corals and sponges and their use as fish habitat in Bering sea submarine canyons. PLoS ONE 7:e33885. doi: 10.1371/journal.pone.0033885
Mineur, F., Arenas, F., Assis, J., Davies, A. J., Engelen, A. H., Fernandes, F., et al. (2014). European seaweeds under pressure: consequences for communities and ecosystem functioning. J. Sea Res. 98, 91–108 doi: 10.1016/j.seares.2014.11.004
Mora, C., Aburto-Oropeza, O., Ayala Bocos, A., Ayotte, P. M., Banks, S., Bauman, A. G., et al. (2011). Global human footprint on the linkage between biodiversity and ecosystem functioning in reef fishes. PLoS Biol. 9:e1000606. doi: 10.1371/journal.pbio.1000606
Mora, C., Danovaro, R., and Loreau, M. (2014). Alternative hypotheses to explain why biodiversity-ecosystem functioning relationships are concave-up in some natural ecosystems but concave-down in manipulative experiments. Sci. Rep. 4:5427. doi: 10.1038/srep05427
Mouillot, D., Bellwood, D. R., Baraloto, C., Chave, J., Galzin, R., Harmelin-Vivien, R., et al. (2013). Rare species support vulnerable functions in high-diversity ecosystems. PLoS Biol. 11:e1001569. doi: 10.1371/journal.pbio.1001569
Naeem, S., Thompson, L. J., Lawler, S. P., Lawton, J. H., and Woodfin, R. M. (1994). Declining biodiversity can alter the performance of ecosystems. Nature 368, 734–736. doi: 10.1038/368734a0
Naeem, S., Thompson, L. J., Lawler, S. P., Lawton, J. H., and Woodfin, R. M. (1995). Empirical evidence that declining species diversity may alter the performance of terrestrial ecosystems. Philos. T. Roy. Soc. B 347, 249–262. doi: 10.1098/rstb.1995.0025
Naeem, S., and Wright, J. P. (2003). Disentangling biodiversity effects on ecosystem functioning: deriving solutions to a seemingly insurmountable problem. Ecol. Lett. 6, 567–579. doi: 10.1046/j.1461-0248.2003.00471.x
Narayanaswamy, B. E., Coll, M., Danovaro, R., Davidson, K., Ojaveer, H., and Renaud, P. E. (2013). Synthesis of knowledge on marine biodiversity in european seas: from census to sustainable management. PLoS ONE 8:e58909. doi: 10.1371/journal.pone.0058909
Ngai, J. T., and Srivastava, D. S. (2006). Predators accelerate nutrient cycling in a bromeliad ecosystem. Science 314:963. doi: 10.1126/science.1132598
O'Brien, R. M. (2007). A caution regarding rules of thumb for variance inflation factors. Qual. Quan. 41, 673–690. doi: 10.1007/s11135-006-9018-6
O'Connor, N. E., and Crowe, T. P. (2005). Biodiversity loss and ecosystem functioning: distinguishing between number and identity of species. Ecology 86, 1783–1796. doi: 10.1890/04-1172
O'Connor, N. E., and Donohue, I. (2013). Environmental context determines multi-trophic effects of consumer species loss. Glob. Chang. Biol. 19, 431–440. doi: 10.1111/gcb.12061
Pakeman, R. J. (2011). Multivariate identification of plant functional response and effect traits in an agricultural landscape. Ecology. 92, 1353–1365. doi: 10.1890/10-1728.1
Pape, E., Bezerra, T. N., Jones, D. O. B., and Vanreusel, A. (2013). Unravelling the environmental drivers of deep-sea nematode biodiversity and its relation with carbon mineralisation along a longitudinal primary productivity gradient. Biogeosciences 10, 3127–3143. doi: 10.5194/bg-10-3127-2013
Pearson, T. H., and Rosenberg, R. (1987). “Feast and famine: structuring factors in marine benthic communities,” in Organization of Communities Past and Present, eds J. Gee and P. Giller (Oxford: Blackwell Scientific Publications), 373–395.
Petchey, O. L., Hector, A., and Gaston, K. J. (2004). How do different measures of functional diversity perform? Ecology 85, 847–857. doi: 10.1890/03-0226
Piot, A., Nozais, C., and Archambault, P. (2014). Meiofauna affect the macrobenthic biodiversity– ecosystem functioning relationship. Oikos 123, 203–213. doi: 10.1111/j.1600-0706.2013.00631.x
Poorter, L., van der Sande, M. T., Thompson, J., Arets, E. J. M. M., Alarcón, A., Álvarez-Sánchez, J., et al. (2015). Diversity enhances carbon storage in tropical forests. Global Ecol. Biogeogr. 24, 1314–1328. doi: 10.1111/geb.12364
Pusceddu, A., Bianchelli, S., Canals, M., Sanchez-Vidal, A., Durrieu De Madron, X., Heussner, S., et al. (2010). Organic matter in sediments of canyons and open slopes of the Portuguese, Catalan, Southern Adriatic and Cretan Sea margins. Deep Sea Res. Part I Oceanogr. Res. Pap. 57, 441–457. doi: 10.1016/j.dsr.2009.11.008
Pusceddu, A., Bianchelli, S., Martìn, J., Puig, P., Palanques, A., Masqué, P., et al. (2014b). Chronic and intensive bottom trawling impairs deep-sea biodiversity and ecosystem functioning. Proc. Natl. Acad. Sci. U.S.A. 111, 8861–8866. doi: 10.1073/pnas.1405454111
Pusceddu, A., Gambi, C., Corinaldesi, C., Scopa, M., and Danovaro, R. (2014a). Relationships between meiofaunal biodiversity and prokaryotic heterotrophic production in different tropical habitats and oceanic regions. PLoS ONE 9:e91056. doi: 10.1371/journal.pone.0091056
Queirós, A. M., Birchenough, S. N. R., Bremner, J., Godbold, J. A., Parker, R. E., Romero-Ramirez, A., et al. (2013). A bioturbation classification of European marine infaunal invertebrates. Ecol. Evol. 3, 3958–3985. doi: 10.1002/ece3.769
Quéiros, A. M., Stephens, N., Cook, R., Ravaglioli, C., Nunes, J., Dashfield, S., et al. (2015). Can benthic community structure be used to predict the process of bioturbation in real ecosystems? Prog. Oceanogr. 137, 559–569. doi: 10.1016/j.pocean.2015.04.027
Quinn, P. Q., and Keough, M. J. (2009). Experimental Design and Data Analysis for Biologists. Cambridge: Cambridge University Press.
Ramirez-Llodra, E., Brandt, A., Danovaro, R., De Mol, B., Escobar, E., German, C. R., et al. (2010). Deep, diverse and definitely different: unique attributes of the world's largest ecosystem. Biogeosciences 7, 2851–2899. doi: 10.5194/bg-7-2851-2010
Reich, P. B., Tilman, D., Isbell, F., Mueller, K., Hobbie, S. E., Flynn Dan, F. B., et al. (2012). Impacts of biodiversity loss escalate through time as redundancy fades. Science 336, 589–592. doi: 10.1126/science.1217909
Reiss, J., Bridle, J. R., Montoya, J. M., and Woodward, G. (2009). Emerging horizons in biodiversity and ecosystem functioning research. Trends Ecol. Evol. 24, 505–514. doi: 10.1016/j.tree.2009.03.018
Rex, M. A., and Etter, R. J. (2010). Deep-Sea Biodiversity: Pattern and Scale. Cambridge: Harvard University Press.
Rex, M. A., Etter, R. J., Morris, J. S., Crouse, J., McClain, C. N., Johnson, N. A., et al. (2006). Global bathymetric patterns of standing stock and body size in the deep-sea benthos. Mar. Ecol. Prog. Ser. 317, 1–8. doi: 10.3354/meps317001
Roberts, J. M., Wheeler, A. J., and Freiwald, A. (2006). Reefs of the deep: the biology and geology of cold-water coral ecosystems. Science 312, 543–547. doi: 10.1126/science.1119861
Sardà, F., Calafat, A., Flexas, M. M., Tselepides, A., Canals, M., Espino, M., et al. (2004). An introduction to the Mediterranean deep-sea. Sci. Mar. 68, 7–38. doi: 10.3989/scimar.2004.68s37
Schlitzer, R. (2011). Ocean Data View. Available online at: http://odv.awi.de
Serpetti, N., Gontikaki, E., Narayanaswamy, B. E., and Witte, U. (2013). Macrofaunal community inside and outside of the Darwin Mounds Special Area of Conservation, NE Atlantic. Biogeosciences 10, 3705–3714. doi: 10.5194/bg-10-3705-2013
Shields, M. A., and Hughes, D. J. (2009). Large-scale variation in macrofaunal communities along the eastern Nordic seas continental margin: a comparison of four stations with contrasting food supply. Progr. Oceanogr. 82, 125–136. doi: 10.1016/j.pocean.2009.05.001
Smith, C. R., De Leo, F. C., Bernardino, A. F., Sweetman, A. K., and Arbizu, P. M. (2008). Abyssal food limitation, ecosystem structure and climate change. Trends Ecol. Evol. 23, 518–528. doi: 10.1016/j.tree.2008.05.002
Snelgrove, P. V. R., Thrush, S. F., Wall, D. H., and Norkko, A. (2014). Real world biodiversity–ecosystem functioning: a seafloor perspective. Trends Ecol. Evol. 29, 398–405. doi: 10.1016/j.tree.2014.05.002
Soetaert, K., and Heip, C. (1990). Sample-size dependence of diversity indices and the determination of sufficient sample size in a high-diversity deep-sea environment. Mar. Ecol. Prog. Ser. 59, 305–307. doi: 10.3354/meps059305
Tecchio, S., Ramirez-Llodra, E., Sarda, F., Company, J. B., Palomera, I., and Mecho, A. (2011). Drivers of deep Mediterranean megabenthos communities along longitudinal and bathymetric gradients. Mar. Ecol. Prog. Ser. 439, 181–219. doi: 10.3354/meps09333
Thurber, A. R., Sweetman, A. K., Narayanaswamy, B. E., Jones, D. O. B., Ingels, J., and Hansman, L. R. (2014). Ecosystem function and services provided by the deep sea. Biogeosciences 11, 3941–3963. doi: 10.5194/bg-11-3941-2014
Tilman, D., Isbell, F., and Cowles, J. M. (2014). Biodiversity and ecosystem functioning. Annu. Rev. Ecol. Evol. Syst. 45, 71–93. doi: 10.1146/annurev-ecolsys-120213-091917
Tittensor, D. P., Rex, M. A., Stuart, C. T., McClain, C. R., and Smith, C. R. (2011). Species–energy relationships in deep-sea molluscs. Biol. Lett. 7, 718–722. doi: 10.1098/rsbl.2010.1174
Tselepides, A., Papadopoulou, K.-N., Podaras, D., Plaiti, W., and Koutsoubas, D. (2000). Macrobenthic community structure over the continental margin of Crete (South Aegean Sea, NE Mediterranean). Progr. Oceanogr. 46, 401–428. doi: 10.1016/S0079-6611(00)00027-6
Tyler, P. A. (ed.). (2003). “The peripheral deep seas,” in Ecosystems of the World (Amsterdam: Elsevier), 261–293.
Tylianakis, J. M., Rand, T. A., Kahmen, A., Klein, A.-M., Buchmann, N., Perner, J., et al. (2008). Resource heterogeneity moderates the biodiversity-function relationship in real world ecosystems. PLoS Biol. 6:e122. doi: 10.1371/journal.pbio.0060122
Van Oevelen, D., Middelburg, J. J., Soetaert, K., and Moodley, L. (2006). The fate of bacterial carbon in an intertidal sediment: modelling an in situ isotope tracer experiment. Limnol. Oceanogr. 5, 1302–1314. doi: 10.4319/lo.2006.51.3.1302
Venail, P. A., MacLean, R. C., Bouvier, T., Brockhurst, M. A., Hochberg, M. E., and Mouquet, N. (2008). Diversity and productivity peak at intermediate dispersal rate in evolving metacommunities. Nature 452, 210–257. doi: 10.1038/nature06554
Welsh, D. T. (2003). It's a dirty job but someone has to do it: the role of marine benthic macrofauna in organic matter turnover and nutrient recycling to the water column. Chem. Ecol. 19, 321–342. doi: 10.1080/0275754031000155474
Worm, B., Barbier, E. B., Beaumont, N., Duffy, J. E., Folke, C., Halpern, B. S., et al. (2006). Impacts of biodiversity loss on ocean ecosystem services. Science 314, 287–290. doi: 10.1126/science.1132294
Yasuhara, M., Doi, H., Wei, C.-L., Danovaro, R., and Myhre, S. E. (2016). Biodiversity–ecosystem functioning relationships in long-term time series and palaeoecological records: deep sea as a test bed. Philos. Trans. R. Soc. B 371:20150282. doi: 10.1098/rstb.2015.0282
Keywords: deep-sea, biodiversity, ecosystem functioning, macrofaunal, relationship
Citation: Baldrighi E, Giovannelli D, D'Errico G, Lavaleye M and Manini E (2017) Exploring the Relationship between Macrofaunal Biodiversity and Ecosystem Functioning in the Deep Sea. Front. Mar. Sci. 4:198. doi: 10.3389/fmars.2017.00198
Received: 26 September 2016; Accepted: 09 June 2017;
Published: 28 June 2017.
Edited by:
Cinzia Corinaldesi, Università Politecnica delle Marche, ItalyReviewed by:
Jeroen Ingels, Florida State University, United StatesAmerico Montiel, University of Magallanes, Chile
Copyright © 2017 Baldrighi, Giovannelli, D'Errico, Lavaleye and Manini. This is an open-access article distributed under the terms of the Creative Commons Attribution License (CC BY). The use, distribution or reproduction in other forums is permitted, provided the original author(s) or licensor are credited and that the original publication in this journal is cited, in accordance with accepted academic practice. No use, distribution or reproduction is permitted which does not comply with these terms.
*Correspondence: Elisa Baldrighi, ZWxpc2EuYmFsZHJpZ2hpQGFuLmlzbWFyLmNuci5pdA==
Elena Manini, ZS5tYW5pbmlAaXNtYXIuY25yLml0