- 1SINTEF Ocean, Trondheim, Norway
- 2SalMar Farming AS, Kverva, Norway
- 3Åkerblå AS, Sistranda, Norway
A spatially explicit coupled hydrodynamic-mass transport model system was used to simulate dispersal of particulate organic matter from Atlantic salmon (Salmo salar) farming in central Norway. Model setups of 32 m horizontal resolution were run for periods of up to 650 days for 3 sites of different oceanographic characteristics: one fjord location, one medium-exposed location influenced by fjord water, and one coastal location. Records on feed used for each cage at each location were converted to feces released based on a published mass balance model. The results from the simulations were compared with scores from corresponding mandatory benthic surveys (MOM-B) of the sediment layer beneath the farms. The correspondence between simulated and measured thickness of the sediment layer was good, and improved with the inclusion of resuspension processes. At all sites the distribution of organic matter in the bottom layer was non-homogeneous, with significant temporal variation and transport and settling of matter up to at least 0.5 km away from one of the farms. Our results indicate that the monitoring practice used in Norway until now, with a few sediment grab samples taken mainly within the fish farm, may not adequately determine the areal impacts of all salmon farming operations. The patchy distribution of organic matter and the correspondence between simulation and survey results is attributed to the use of full 3D current fields of a high spatiotemporal resolution and a good model for resuspension processes that some previous model studies have failed to properly account for.
Introduction
Global aquaculture production increased by 6.1% annually from 2002 to 2012 (FAO, 2012). It is predicted that in the future an increasing fraction of human food consumed will come from the oceans in general and aquaculture in particular (Olsen, 2011). For example, Norwegian aquaculture production (mainly Atlantic salmon Salmo salar and rainbow trout Salmo trutta) increased from 5 × 105 to 1.25 × 106 t from 2003 to 2012 (Statistics Norway, www.ssb.no) and it has been suggested that by 2050 this production may be increased by a factor of 3–5 T (Olafsen et al., 2012). One of the main limiting factors for further growth of the mariculture sector is the availability of good locations (Hersoug, 2013). In Norway the number of concessions has decreased in recent years (Gullestad et al., 2011; Bannister et al., 2014). It is therefore likely that with the present cultivation technology the average production at each location will further increase, with an ensuing increase in the local release of particulate and dissolved organic matter leading to a potentially higher impact on the pelagic and benthic (Mazzola et al., 2000; Carroll et al., 2003; Kalantzi and Karakassis, 2006).
In Norway, the impacts of mariculture on the benthic system are monitored through the MOM survey (Ervik et al., 1997; Hansen et al., 2001; Stigebrandt et al., 2004), which is specified in the Norwegian standard NS9410. This survey includes sampling of the sediment for pH, redox potential, sediment type, presence of gas bubbles, color, smell, consistency, and the presence of mud. Sediment samples are also analyzed for benthic fauna where the species type and number of specimens are recorded. Carefully choosing the right locations and the proper production volume for each location might minimize observable effects on the benthic system and shorten fallowing time. Dynamic model tools capable of predicting the main variables in the management systems being used (e.g., MOM survey or its equivalents) may therefore be useful in the process of siting farms and in planning sampling campaigns. Such model tools may also be used to guide ecosystem based and adaptive management schemes, and may be readily expanded to include further variables and processes should they be required in the future.
In the present study a dispersion model (DREAM) with high resolution 3D data from a hydrodynamic model (SINMOD) is applied to simulating the dispersion and sedimentation of particulate wastes from three fish farms in central Norway (Figures 1, 2). There are two major current systems in this coastal area: the relatively fresh Norwegian Coastal Current (NCC) and the more saline Norwegian Atlantic Current (NAC) (Sætre, 2007). The larger fjord systems along the coastline are forced by freshwater runoff from land resulting in a surface outflow of brackish water that eventually adds to the NCC. Wind induced currents, tides and the rough topography including steep bathymetric gradients, banks, islands, skerries, and narrow straits creates a dynamic and complex physical environment. Exposed fish farms are often located near islands or skerries providing shelter from rough seas, but there is also great spatiotemporal variability of currents in fjords. In the present study farm locations with three different oceanographic characteristics were chosen: a fjord location, a semi-exposed location with moderate current speeds, and an exposed location with high current speeds and a dynamic environment. The main purpose of this study is to evaluate the spatially explicit model system's ability to resolve farm waste deposit thickness as reported in the MOM-reports. Resuspension and erosion processes are included. Finally, we present a metric for the resuspension, dispersion, and spatial heterogeneity of fish farm wastes that may be used to characterize a farming site.
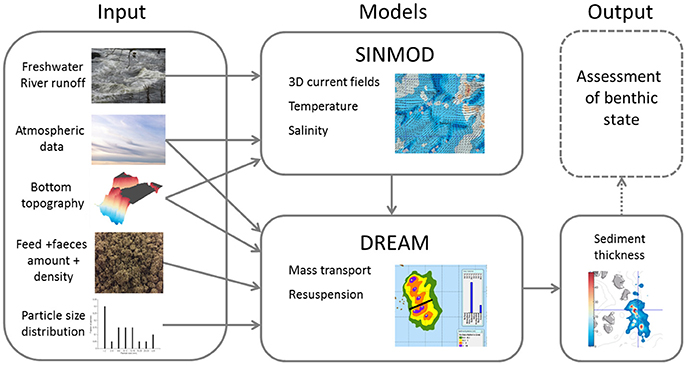
Figure 1. Schematic for the coupling of the model systems SINMOD and DREAM, the input data used and the output. The model system provides explicit information on the mud thickness. The ultimate assessment of benthic state has to be made based on the simulation results (dashed lines).
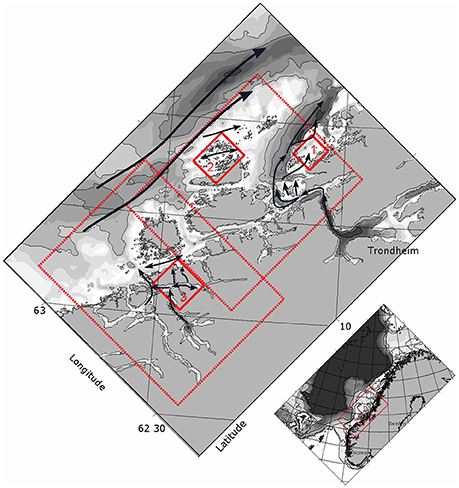
Figure 2. Overview of the study areas in the coastal region of central Norway and the SINMOD model domains used to generate water current fields for the DREAM simulations. Excerpt from the 800 m resolution model domain (indicated by solid red lines in the small panel) with 160 m (dashed red lines) and 32 m (solid red lines) model domains indicated. The three locations used in this study are location 1 (Tristeinen, red T, 63.87°N, 9.62°E), location 2 (Rataren, two cage arrays, red R, at 63.781°N, 8.517°E, and 63.783°N, 8.523°E) and location 3 (Korsneset, red K, 63.143°N, 8.225°E). The coordinates refer to the location of the middle of the cage arrays at the time when the MOM surveys were carried out.
The results of the model framework are useful for managing the aquaculture industry and in production planning at single locations, e.g., by estimating the potential for increasing production or the need for biomass reduction at a site. The farm layout and orientation of cage arrays may also be evaluated using the tools presented here. A number of studies and models have been published internationally focusing on the same general subject matter. The present study adds to the existing literature by including three dimensional current fields in high spatiotemporal resolution for a full production cycle and resuspension processes in the simulations, as well as by contrasting three sites of different oceanographic characteristics by a novel location specific metric. Results from such simulations, in high resolution and covering significant time periods have not previously been published.
Materials and Methods
We apply a coupled hydrodynamics-mass transport model (Figure 1) to the study of the dispersal and deposition of particulate wastes from 3 fish farming locations in central Norway operated by SalMar Farming AS: location 1 (Tristeinen, semi-exposed location), location 2 (Rataren, coastal, exposed location, 2 cage arrays: I and II from west to east), and location 3 (Korsneset, fjord location; Figure 2). The dominating natural sediment types were sand and silts (location 1) and sand and gravel (locations 2 and 3).
Description of the Model System
SINMOD
SINMOD is a coupled 3 dimensional hydrodynamic-ecological model system (Støle-Hansen and Slagstad, 1991; Slagstad and McClimans, 2005; Wassmann et al., 2006). The hydrodynamic model is based on the primitive Navier–Stokes equations solved by a finite difference scheme. The model uses z-coordinates, i.e., each model depth level has a fixed thickness, except for the surface level and the one closest to the bottom. Vertical mixing in SINMOD is handled using a Richardson scheme (Sundfjord et al., 2008).
Boundary conditions were generated in a 4 step nesting procedure, running models of increasingly finer grids from 20,000 to 32 m horizontal resolution. The 20,000 and 4,000 m model domains encompass the Arctic Ocean and Nordic seas (Ellingsen et al., 2009), while the 800 m model domain covers the Norwegian coast from 61°53′N, 4°46′E–66°22′N, 12°8′E (Broch et al., 2013). SINMOD has been shown to successfully resolve the circulation dynamics of the Norwegian shelf off Northern Norway (Slagstad et al., 1999; Skarðhamar and Svendsen, 2005; Anon, 2011) and has previously been applied to studying e.g., dispersal patterns of cod eggs and larvae in fjord environments (Uglem et al., 2012) and the dispersal of dissolved nutrients from salmon farming (Broch et al., 2013). Two 160 m resolution model domains were used to generate boundary conditions for the 3 model domains of 32 m resolution (Figure 2).
The 32 m model domains for locations 1, 2, and 3 used vertical layers of thickness ranging from 0.5 m near the surface to 25 m from 100 m depth. The surface layer had a thickness of 2 m for locations 2 and 3, and 3 m for location 1. The thickness of the uppermost layer in SINMOD is determined by surface elevation.
The 20,000 m model domain was forced by tidal components M2, S2, K1, and N2 at the open boundaries, with data taken from the TPXO 6.2 model of global ocean tides (http://www.coas.oregonstate.edu/research/po/research/tide/global.html).
Atmospheric forcing was applied using data from ECMWF ERA-Interim (Dee et al., 2011; for location 3) and the Norwegian Meteorological Institute (www.met.no; for locations 1 and 2). Freshwater discharges from rivers and diffuse discharges from land were provided by NVE (Norwegian Water Resources and Energy Directorate, www.nve.no). Bathymetric data were supplied by The Norwegian Mapping Authority (www.kartverket.no) and supplemented with high-resolution bathymetry for the fish farm locations and their immediate surroundings recorded by AquaCulture Engineering (ACE) and Havbrukstjenesten AS using OLEX Automatic seabed charting (Olex AS, Trondheim) and a multibeam echosounder.
The simulation time steps were 8, 10, and 6 s for the setups for location 1, location 2, and location 3, respectively, depending on standard numerical stability criteria.
DREAM
DREAM (Dose-related Risk and Effects Assessment Model) is a 3 dimensional multi-component Lagrangian particle tracking model (Rye et al., 1998, 2006; Reed and Hetland, 2002). Based on pre-computed current and wind fields (Figure 1), DREAM simulates the transport and fate of particulate matter and chemical compounds in the water column, and the build-up of mud on the sea floor. The particulate matter can be released over time from any number of spatial locations, at the sea surface and in the water column at a specified depth. The model also includes calculation of waves from the wind field and fetch based on the JONSWAP equations (Rye et al., 2006) as well as sea bed erosion processes.
DREAM uses a distribution of size classes for each component of particulate material releases, resulting in different settling velocities depending on the relative density and particle diameters. Coarse particles thus settle closer to the release point than finer particles.
In the present context we wish to determine where the waste accumulates on the sea bed and in what quantities. DREAM has a grid-based sea bed compartment where mass accumulates in different grain size classes (defined by the size classes of the discharge). Erosion from the sea bed may occur and contribute significantly to the distribution of waste over time. This is accounted for by a resuspension sub model. This sub model calculates the frictional stress on the sea bed in each model grid cell from the combined action of currents and waves. Whenever this stress exceeds the critical Shield's parameter (Shields, 1936), resuspension of deposited matter occurs, which is distributed vertically near the sea bed according to a cell-specific calculated turbulent mixing height (Zyserman and Fredsøe, 1994; Black and Vincent, 2001). The Stokes-based sinking speed of the resuspended particles determines whether they settle back to the sea bed in the same time step (based on the distance they settle in that time interval compared to the mixing height), or if they remain in suspension. For the latter case, the mass is transformed back to the Lagrangian particle representation used elsewhere in the model. More details can be found in Rye et al. (2006).
Turbulent vertical mixing in the DREAM model is computed using a semi-empirical formula taking into account surface waves (Ichiye, 1967).
Model Simulations
Fish Farm Production Data and Periods and Input Data for Simulations
Feed data were reported by SalMar Farming AS. The feed data were converted into waste released from each cage by assuming a feed factor (dry weight feed/wet weight of fish produced) of 1.1 and a release of 0.272 t of particulate matter per tone of salmon biomass produced, including about 5% of uneaten feed (Cromey et al., 2002a; Reid et al., 2009; Wang et al., 2012), c.f. Figure 3. The relative frequency distribution of feed and fecal particle sizes were based on Buryniuk et al. (2006) (Figure 4). The waste particle density was assumed to be 1.033 t m−3.
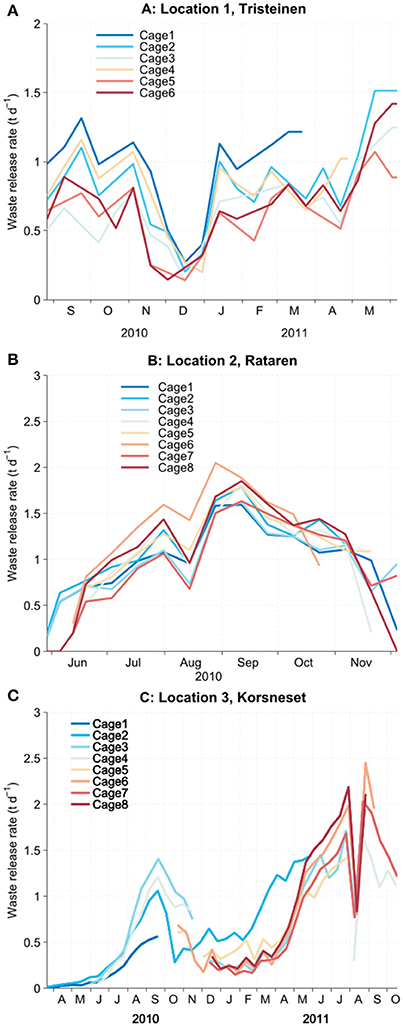
Figure 3. Daily release rates of feed and feces wastes from each of the cages at locations 1 (A), location 2 (B), and location 3 (C). Averages for 14 day periods were used in the simulations and are plotted in the graphs.
SINMOD Simulations
SINMOD was run for the relevant production period for each location/model setup (Table 1, Figure 2). At location 1, a period of maximum feed use was considered. At location 2, the simulation was run from when fish was moved to the farm from another location until slaughter. For location 3 the model was run from the start of a production cycle until the mandatory MOM survey.
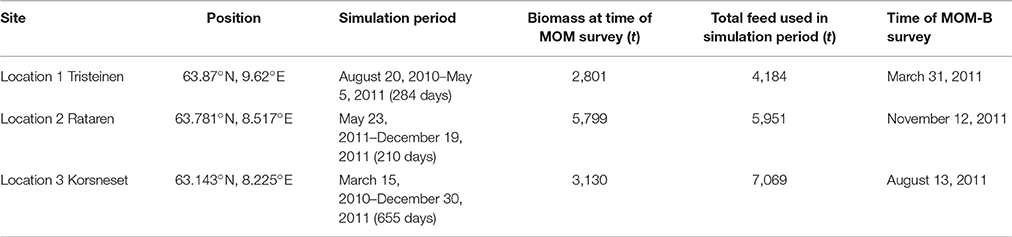
Table 1. Overview of model parameters and assumptions, relevant production data, and sampling dates (MOM-B surveys).
The 3 dimensional water current velocity fields were stored every 0.5 h and used to run DREAM.
DREAM Sensitivity Tests
Initial sensitivity studies with a base case of a 10 day release from one cage were performed. One input parameter was changed compared to the base case for each simulation. The input parameters that were studied were: the number of release points per cage, the resuspension model turned on/off, particle size distribution, and the resolution of the hydrodynamic model (160 × 160 vs. 32 × 32 m spatial resolution).
DREAM Simulations (The Main Simulations)
The DREAM model was set up to simulate the release from one production cycle at three different locations. One release point per net cage was set up at 5 m depth and the release was assumed to be constant for periods of 2 weeks before changing. Two simulations for each location were run, one without resuspension of mud particles, and one with the resuspension model activated. A total of 30,000 Lagrangian particles (“numerical particles”), each representing a varying number of actual waste particles, were used in each simulation, distributed evenly among the cages and over time. The total mass and number of actual waste particles represented by each Lagrangian particle thus varied with the release rate of feces. The relative frequency distribution of particles was kept fixed throughout each simulation. The spatial resolution of the sediment grid was 25 × 25 m for locations 1 and 3 and 10 × 10 m for location 2.
No information on the sediment state in the period before the start of the simulations was available, and the mud thickness was initialized to zeros at the beginning of each simulation. Therefore the “simulated mud thickness” throughout this article refers to the organic matter released from the fish farms only.
Resuspension Index
A “resuspension index” quantifying the contribution of resuspension processes to the sediment distribution was calculated for all the three farming locations, as follows. Let Sresusp and S denote the 2D fields of the distribution of the sediments with and without resuspension, respectively. The influence of resuspension processes at a site may be quantified as the spatially integrated difference between Sresusp and S relative to the spatially integrated field S, thus:
where the integrals are taken over the model domain Ω Formally speaking RI is the fraction of the L2-norms of the scalar fields Sresusp − S and S. Then 0 ≤ RI ≤ 1. If RI = 0 resuspension has no impact on the mud distribution, while if RI = 1, the entire distribution field has been shifted by resuspension (Figure 8). In theory all matter may be resuspended and still give RI = 0, but if so resuspension does not alter the distribution of the mud anyway.
MOM Surveys
The MOM survey is described in Norwegian Standard no 9410:2007 (available through www.standard.no; Ervik et al., 1997; Hansen et al., 2001). The survey is based on three groups of sediment variables: assessment of the benthic fauna, chemical assessment (pH and redox potential), sensory assessment (gas, color, smell, consistency), grab sample volume and mud thickness (including sediments of natural origin and/or already present). Each variable is scored based on the degree of influence by organic matter. In particular, the mud thickness is given a score of 0 (thickness <20 mm), 1 (20 mm ≤ thickness <80 mm), or 2 (thickness ≥ 80 mm). Finally, the sample itself is given a total score ranging from 1 (best condition) to 4 (worst condition). See Table 1 for the timing of the MOM surveys used here.
Samples are taken at 10 points selected based on the site's local topography and hydrographic conditions, previous surveys conducted at the site and the aim of the survey. The 10 points are distributed over the entire site, but are not to be located on ridges or raised areas. The site area is the area from under the cage array to the outer edges of the anchors. If a survey was previously conducted at the site then a number of the previously chosen points must be re-sampled in the current survey. The samples are taken with a Van Veen grab, with a minimum of two attempts made if there is not enough mud collected in the first grab. Samples are emptied over a sieve and the state of the mud is examined before the sample is washed and the benthic fauna registered.
The MOM score for each sample is calculated as a weighted average of the scores for each of the chemical and sensory variables. The MOM score for the location is then calculated from the average of the sample scores. See Hansen et al. (2001) for the details.
The MOM survey is carried out during periods of intensive production. If the survey results in a score of either 1 (very good) or 2 (good), a new generation of fish may be transferred to the site after a two month fallow period. If the survey results in a score of either 3 (bad) or 4 (very bad), a mandatory fallow period of 2 months is followed by a new MOM survey to determine whether a prolonged fallow period is required or whether the sea bed under the site is recovered enough that a new generation of fish may be transferred to the site.
Measurements of Water Currents
Current data were recorded at location 2 from January 29th to February 19th, 2010 using a SD 6,000 rotor current meter from Sensordata AS. Current speeds were collected at two positions, north and south of the cage arrays, while current directions were recorded south of the farm only (Figure 5). At the northern position measurements were taken at 5 m depth and at the bottom, while at the southern position the measurements were taken at 5 and 15 m depths.
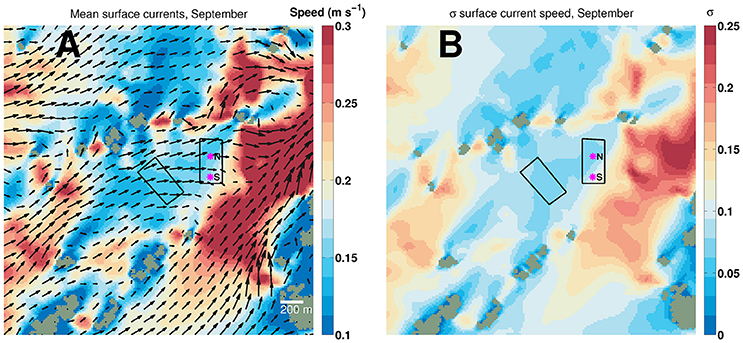
Figure 5. Simulated mean surface currents at location 2 for September 2011. (A) The colors indicate mean current speeds, while the black arrows indicate the direction. (B) Temporal standard deviation. The black rectangles in both panels indicate the position of the fish farm cage arrays. The short side of the cage arrays is 200 m long, also indicated by the white segment in (A). Note that these panels display only a minor part of the complete domain for the hydrodynamic model (Figure 2).
Results
Simulated Current Data from SINMOD
Simulated mean current speeds (January 29–February 26, 2010) south of location 2 were 14.4 and 8.6 cm s−1 at 5 and 15 m depths, respectively. The corresponding mean values from the current velocity recordings were 17 and 10.5 cm s−1. North of the farm, the mean simulated values at 5 m depth and at the bottom (43 m depth) were 12.1 and 7.7 cm s−1, while the measured values were 11.7 and 8.1 cm s−1, respectively.
The measured current velocities at the southern station (Figure 5) indicate main current directions northeastward (5 and 15 m depth) and going south (5 m depth) and south-southwest (15 m; Figures 6A,B). The simulation results were generally similar, but with a tendency to a more westerly direction at 5 m depth. At 5 m depth, the simulated current directions were more spread than indicated by the measurements. At 15 m depth, the model showed a tendency for water movement more in the north-easterly direction than the measurements.
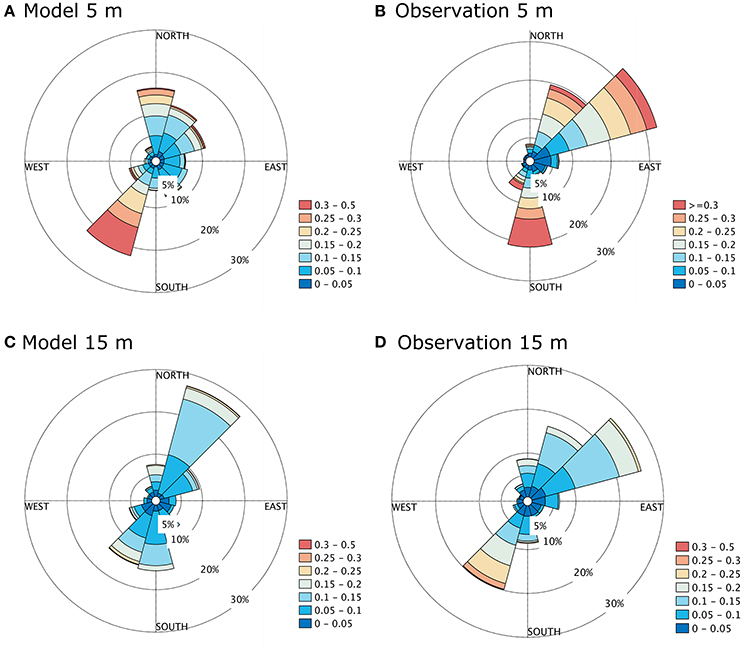
Figure 6. Current rose distribution plots of simulated (A,C) and measured (B,D) water velocities at the south end of array II at location 2 (labeled “S” in Figure 5). The upper (A,B) panels show velocities for 5 m depth. The lower panels show velocities for 15 m depth. The position of the S station was 64.7813°N, 8.5264°E. The measurements and corresponding SINMOD simulation were made for the period 29 January to 26 February, 2010.
There was substantial spatial and temporal variability in the simulated surface water currents in the area immediately surrounding the cage arrays at location 2, illustrated in Figure 5 by mean surface currents for September 2011 (left) and temporal standard deviation (right). The mean surface current speed within and immediately outside the cage arrays varied from 0.14 to 0.2 m s−1, while west and south-west of the cage arrays there were strong currents (mean ~ 0.28 m s−1) going in a north-easterly direction (Figure 5). At the station south of the farm the simulated current direction was more in an easterly direction than the observed currents (Figure 6).
Results from the DREAM Sensitivity Simulations
The sensitivity tests showed that by increasing the number of release points per net cage, the maximum simulated mud thickness decreased. This happened because the matter was distributed over several points covering a larger area within the cage, thus distributing the matter over a greater area on the bottom. The resuspension model led to increased erosion. Using a finer particle size distribution (e.g., shifting the size distribution toward smaller sizes), more matter was suspended in the water column for a longer period, resulting in less sedimentation close to the fish farm. The spatial resolution of the current model grid (32 vs. 160 m) showed increased mud build up and a smaller impacted area for the 32 m current grid compared with the 160 m grid.
Results from the DREAM Simulations
In the simulations without resuspension the greatest deposits were found around the release points (Figures 7D–F), with some tendency to “smearing,” illustrating the tidal influence on the local simulation patterns, in particular at location 2. At all three locations the inclusion of resuspension processes in the simulations lead to a more irregularly shaped deposit region and a less homogeneous distribution of the deposits (Figures 7A–C, 8A–C). The deposits were more concentrated in the resuspension than in the non-resuspension simulations, with more instances of higher mud thickness, as seem from comparing the changes in mean, median, and excess kurtosis of the mud thickness in Table 2.
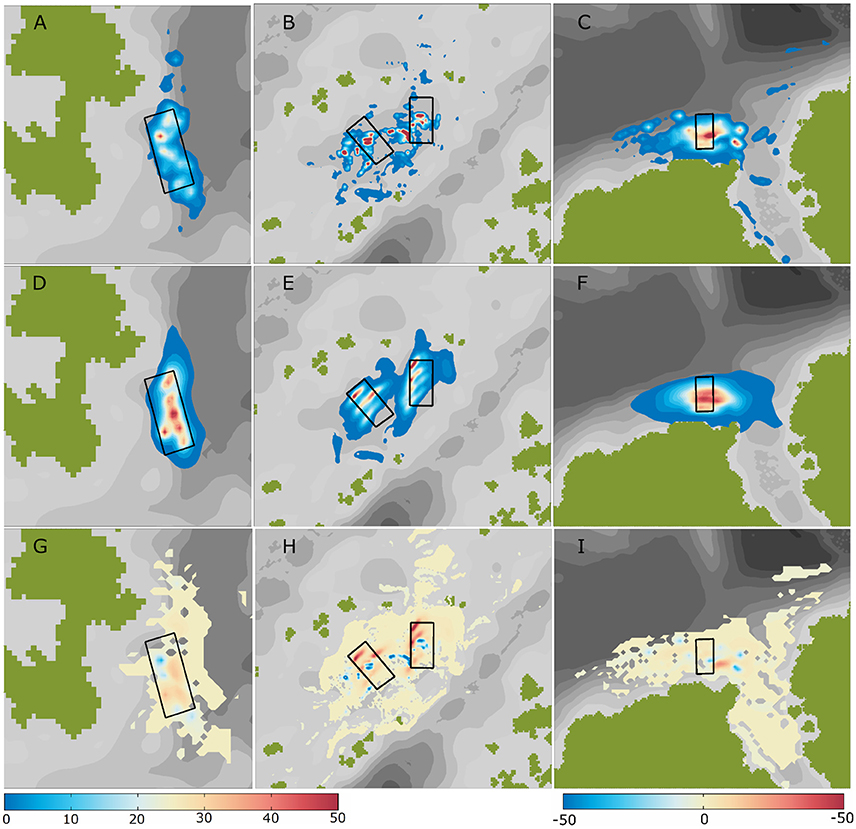
Figure 7. Simulated mud thickness (mm) at the time of the MOM surveys (Table 1) at locations 1–3 (top to bottom panels). (A–C) Results from the simulations with resuspension processes. (D–F) Results from the simulations without resuspension processes. (G–I) Plots of the differences in simulated mud thickness between the simulations without and with resuspension. The cage arrays are 200 m wide. The colors indicated mud thickness (mm) (left bar, A–F) and differences in mud thickness (mm) (right bar, G–I). The shade of gray indicate bottom depth.
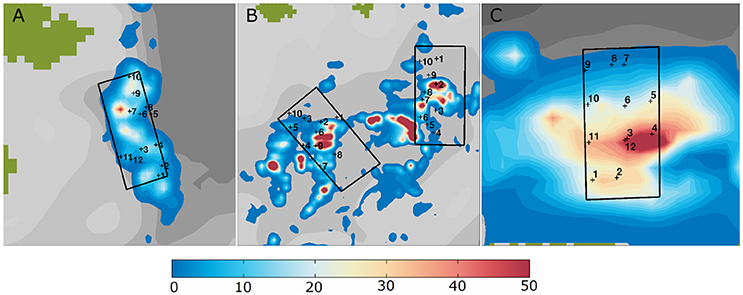
Figure 8. Magnification of the corresponding panels in Figures 7A–C, from the simulations with resuspension at location 1 (A), location 2 (B), and location 3 (C). The numbers in the plot correspond to the MOM samples presented in Figure 10. The colors indicate mud thickness (mm). The shade of gray indicate bottom depth.
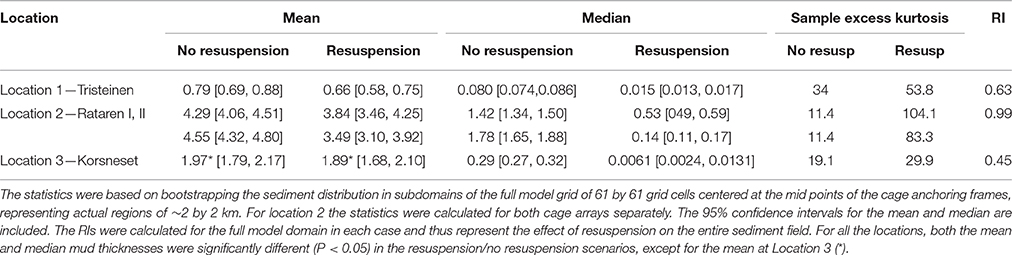
Table 2. Statistics (spatial mean, median, and sample excess kurtosis) for the simulated mud thickness distribution at the time of the MOM surveys (Table 1; Figures 8, 9), for the simulations with and without resuspension, and the RI values for locations 1–3.
At both locations 2 and 3 patches of simulated mud thickness high enough to potentially deteriorate the benthic states occurred well outside the cage arrays (Figures 7B,C, 8B,C, 9) in the simulations with resuspension. For example, at location 2 there was a patch of simulated mud thickness ~50 mm at a distance of ~150 m southwest of the westernmost cage array (Figure 8B). At location 3, there were patches of 30–50 mm simulated mud thickness more than 200 m to the east of the cage array, while there were patches of 10–20 mm thickness more than 400 m to the west of the cage array. This tendency was not that pronounced in the simulations without resuspension (Figure 7E). At location 1, the inclusion of resuspension lead to higher simulated mud thickness within the cage array, but not that much outside of it (Figures 7A, 8A).
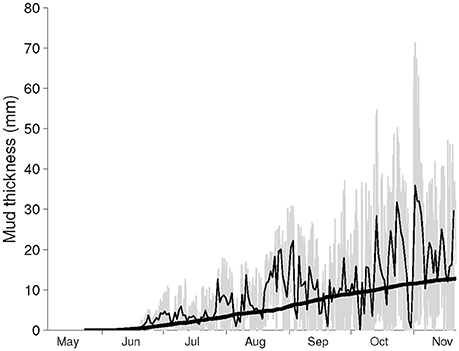
Figure 9. Example time series for the simulated mud thickness at one point within the cage arrays at location 2. Gray line: values of mud thickness with resuspension plotted at 6 h intervals. Thin black line: daily running means of the mud thickness with resuspension. Heavy black line: daily values for simulated mud thickness without resuspension.
At all the locations the inclusion of resuspension led to a decrease in the mean and median mud mass within a 2 by 2 km region surrounding the cages (Table 2). These changes were all significant at the 0.05 level, except for a non-significant decrease in the mean at location 3. At location 1, 16% less of the released mass remained within the 2 by 2 km region when resuspension was included, while for the regions around the two cages at location 2, the corresponding figures were 11 and 23%.
Including resuspension lead to different temporal development of the simulated mud thickness, as illustrated by a time series for simulated mud thickness in a single model grid cell (Figure 9). Without resuspension, there was a gradual build-up of sediments. With resuspension, the mud thickness varied dramatically within hours.
The RIs for location 1, location 2, and location 3 were 0.63, 0.99, and 0.45, respectively, at the time of the MOM surveys (Figure 7, Table 2). Thus, the simulation results for location 2 were the most influenced by the resuspension process, while those for location 3 were least influenced.
In terms of areas covered by mud layers of different thickness, the simulation results with resuspension gave very different results for the three locations. At location 1, 5,519 m2 of bottom was covered by mud of 20–80 mm thickness, while 0 area was covered by mud above 80 mm thickness. At location 2, 23,409 m2 was covered by mud layers of thickness between 20 and 80 mm and 3,401 m2 by mud thicker than 80 mm. Finally, at location 3, 46,162 m2 was covered by mud layers 20–80 mm thick and 0 m2 was covered by mud thicker than 80 mm.
Comparison of the Results from the MOM Survey and DREAM Model
Temporal averages of simulated mud thickness both with and without resuspension were compared with the corresponding mud thickness intervals from the MOM-B survey (Figures 10A–D). When resuspension was not considered, the simulated values for mud thickness were in the same intervals as the MOM samples for 27 out of the 44 samples (Figure 10) at the relevant dates. When resuspension was taken into account, this figure improved to 34 out of 44. At the second cage array at location 2, all simulated values matched the MOM-intervals when resuspension was included (Figure 10C).
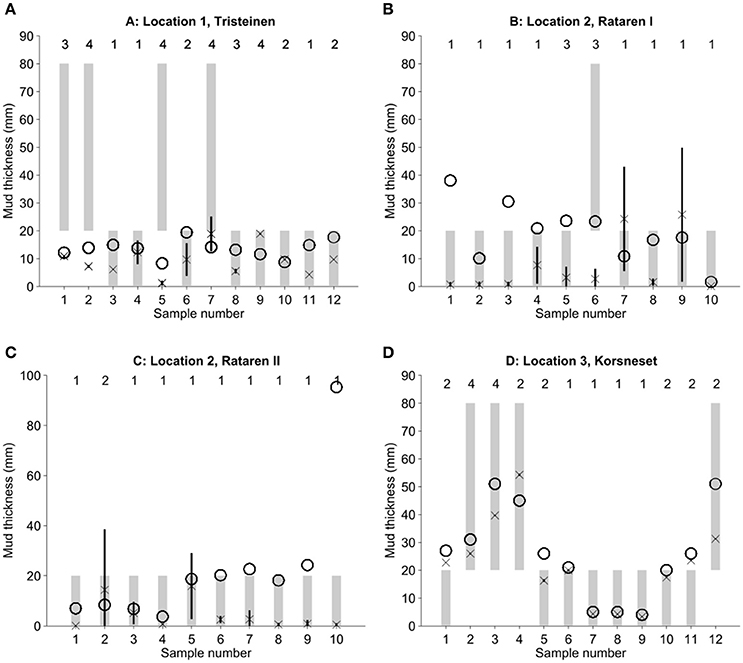
Figure 10. Comparison of simulated and observed mud thickness at each of the four farms. (A) Location 1 (Tristeinen). (B) Location 2 (Rataren), western array I. (C) Location 2, eastern array II. (D) Location 3 (Korsneset). The locations for sediment sampling for each of the four farms are shown in Figure 8. The sample numbers in Figure 8 and the present figure correspond (below abscissa). The gray bars indicate the recorded interval of mud thickness from the MOM surveys (Table 1). Time-averages (n = 3, time step 12 h for location 1, 6 h for location 2, and 24 h for location 3) of simulated values, with resuspension, at the corresponding points are represented by ×s, while the thin black lines represent standard deviations. The final MOM scores (including chemical assessments) of the samples are plotted above the axes. The circles represent mud thicknesses simulated without resuspension.
At location 1 the simulated mud thickness at the sample positions was generally low, and lower than the mud thickness reported in the MOM surveys at sample points 1, 2, 5, and 7 (Figures 8A, 10A) when resuspension was not included. At sample points 4, 6, and 7 there was substantial temporal variation in the mud thickness when resuspension was included. At sample point 7, the simulated mud thickness changed from one MOM state to another with time (Figure 1A).
In general, the inclusion of resuspension lead to lower simulated mud thickness at all sample positions at location 2 (both arrays). Most notably, the simulated mud thickness at sample point 1/array I and 10/array II fell from 40 (resp. 95) mm to almost 0 from the simulation without to the one with resuspension (Figures 10B,C). Sample point 10 at the second array was just beneath a fish cage (Figure 8B). At location 2 the temporal variation in the simulated mud thickness with resuspension was very large at some points and negligible at others (Figures 10B,C). The simulated mud thickness of samples 7 and 9 (array I) moved in and out of the interval indicated by the MOM survey with time when resuspension was included (Figure 10B).
At location 3 there was very little temporal variability in the simulated mud thickness, both with and without resuspension. For all but one of the sample positions at location 3 the simulated mud thickness decreased when resuspension was included. At sample positions 1, 5, 6, 10, and 12 the simulated mud thickness was closer to the mud thickness estimated by the MOM survey with resuspension than without (Figure 10D).
The simulated mud thickness when the resuspension module was activated exhibited sharp spatial gradients (Figures 7, 8). Notably, at location 1 (stations 2 and 7), the simulated mud thickness entered the lowest thickness category in the MOM-system, while both were close to patches of significantly higher mud layer thickness (>20 mm).
Discussion
The existing literature on the fate and dispersal of particulate matter from fish farming is considerable (e.g., Mazzola et al., 2000; Carroll et al., 2003; Reid et al., 2009; Brager et al., 2014; Martinez-Garcia et al., 2015). The combined near-and far-field aspects of transport of organic matter has been somewhat missing. Law et al. (2014) estimate, very roughly based on settling velocities, that floc particles from finfish aquaculture may travel as far as 2 km away from finfish farms. A recent simulation study presents similar values (Bannister et al., 2016). The present study indicates that transport as far as, or further away than, ~2 km may be significant (Table 2).
Several modeling studies have been published, mainly addressing the DEPOMOD (Cromey et al., 2002a, 2009; Keeley et al., 2013; Chang et al., 2014; Bannister et al., 2016). Some of these simulation studies reveal a more homogeneous distribution of the sediments/organic matter than the present results. There are at least two reasons for this.
Firstly, we have used simulated 3D current velocity fields for a substantial fraction of a production cycle (6–22 months) in very high spatial (32 m) and temporal (0.5 h time intervals) resolution to run the particle transport model. In contrast, previous studies have been run with data from point measurements of currents, for a limited time period (Chang et al., 2014), naturally giving a more homogeneous current field, and hence a more homogeneous transport of matter (Keeley et al., 2013; Chang et al., 2014).
Secondly, resuspension processes included in the present simulations consistently improved the correspondence between the mud thickness estimated by grab sampling and the model (Figure 10). Though resuspension processes have been considered previously (Cromey et al., 2002b), this is the first study based on a highly spatiotemporally resolved model system to properly account for this process on such a large scale. The correspondence between the simulated mud thickness and the MOM measurements were consistently better when resuspension was included.
A highly resolved current field in combination with a reasonable resuspension model account well for heterogeneity in the distribution and potential aggregation of organic matter in fish farm near field and beyond (Figure 11). The importance of resuspension was most striking at location 2 (Rataren, the coastal site; Figure 10, Table 2), and in particular at sample point 10/array II. The “resuspension index,” RI, is a conceptually simple metric for the potential importance of resuspension, and it is only location dependent in the sense that it is independent of the total production and feeding volumes. It complements the other statistical information in Table 2. Combined with information on the temporal statistics of the (simulated) bottom layer water current speed (Figure 12), the RI provides some insight into the possible spatiotemporal dynamics of organic matter at fish farming sites, and may help inform the planning process for surveys and sampling campaigns.
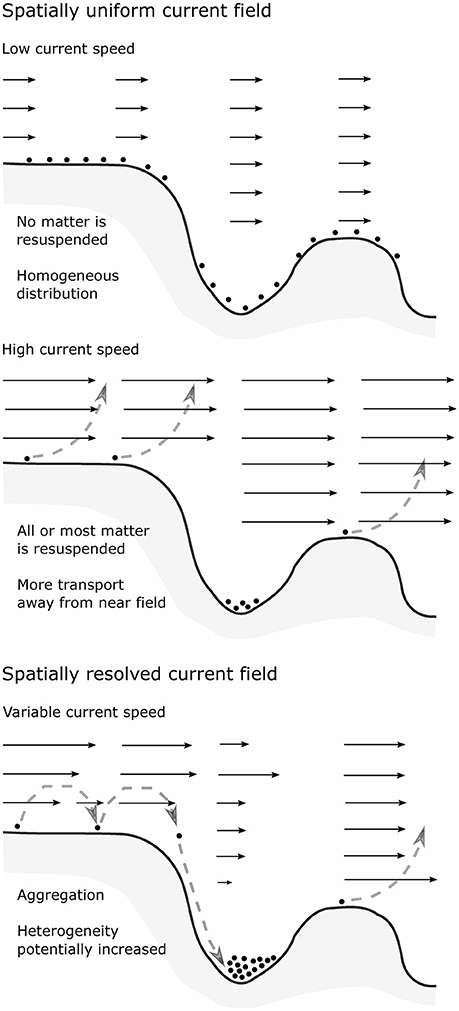
Figure 11. Conceptual illustration of how increased spatiotemporal resolution of the water current fields lead to changes in the simulated transport of particulate organic matter. If resuspension processes are included, a uniform water field may lead to consistent over or under estimation of the concentration of organic matter depending on the current speed. A high resolution variable current field may lead to resuspension in some places and aggregation of matter in others.
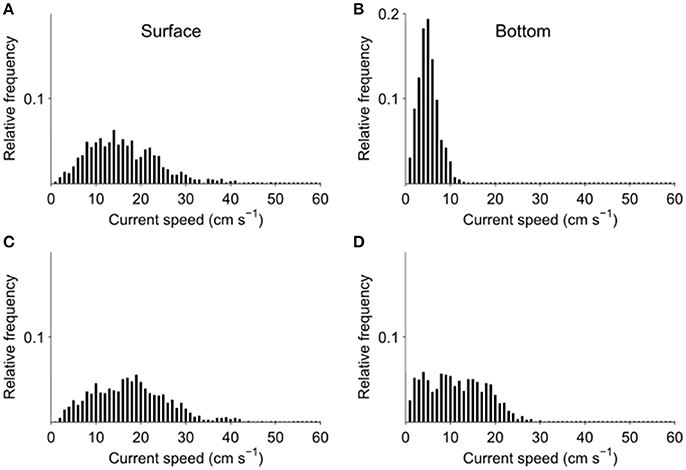
Figure 12. Statistical distribution, for the entire simulation period, of simulated water current speeds at 5 m depth (A,C) and the bottom (B,D) for sample points 2 (A,B) and 9 (C,D) at location 2 (eastern cage array II; Figure 8).
Model Sensitivity, Uncertainty, Limitations, and Need for Further Validation
Particle Size and Density Distribution
Fecal particle density, size, and settling velocity distributions are parameters of importance in studies and simulations of dispersal of wastes from aquaculture (Cromey et al., 2002a; Reid et al., 2009; Bannister et al., 2016). DREAM calculates sinking and settling velocities from the sizes and densities of the particles. Hence the selected particle size distribution and particle densities impact on the endpoint (mud thickness) in the present study. Information on in situ particle size distributions of fish farm wastes is scarce (Reid et al., 2009). Law et al. (2014) make a case for including small particles of diameters <1 mm in depositional models. Their results indicate a correspondence between fine particle sizes and low settling velocities and hence suggest a potential for far-field transport. In the present simulations it was assumed that 30% of the released fecal particles had a diameter of <2 mm (Figure 4), and the particle density of the Lagrangian particles (numerical waste particles) used here [see Sections Fish Farm Production Data and Periods and Input Data for Simulations and DREAM Simulations (the Main Simulations)] is similar to modeled values for flocs (Law et al., 2014) and consistent with measured values (Ogunkoya et al., 2006). Thus, the properties of the Lagrangian particles used in the present simulations cover a relevant range.
Since feed loss is assumed to account for only about 3–5% of the released matter (Reid et al., 2009), the assumption that uneaten feed particles and pellets had the same density and size distribution as fecal particles has little importance for the conclusions.
Simulated and Observed Mud Thickness
Although, the mud thickness of the grab samples is but one of several variables contributing to the total MOM score and the final assessment of benthic state, mud thickness was a reasonable proxy of the benthic state in the samples presented here. The mud thickness and the MOM score were significantly correlated (Pearson correlation coefficient R = 0.72, P = 0.015) when all 44 MOM samples were combined.
Of the three locations, the worst correspondence between simulated and observed mud thickness was found at location 1 (Figures 7, 8, 10). No water current measurements for the relevant period was available for location 1, so it is not possible to pin point underestimations of current speed as the cause of the mismatch between simulation and data. However, modification of the water currents by the farm structures may be of importance (Wu et al., 2014), and this has not been included in the present simulations. SINMOD is currently being extended to include such processes.
Biodegradation processes were not included in the present simulations, and therefore one would expect the model to overestimate the amounts of organic matter in the bottom layer. Again, reduced flow through might have reduced current speeds and possibly increased the amounts of deposits. For example, the indication of overestimation at stations 7 and 9 at location 2 (Figure 10B) is consistent with the model slightly underestimating current speeds there (Figure 6). Depending on the bathymetry, reduced surface currents might increase bottom currents, however (Klebert et al., 2015), and the interplay between a number of processes and input data is what eventually determines the amounts of bottom deposits.
The model indicates a high spatial variability in the simulated mud thickness at several sample stations (Figures 7, 8, 10). This means that a good spatial match between measurements and simulation results can be hard to achieve with 10–12 grab samples covering an entire cage array of about 10 ha. At location 1 there is a position with high simulated mud thickness close to sample 7 (Figure 10A) where the model underestimated deposits. St 6 at location 2 is another example.
Natural background levels of sediments have not been considered in the model simulations, but may have had an impact on the mud thickness as reported in the MOM surveys. Initialization of the DREAM model with a background level of sediments would increase the simulated sediment levels presented here.
There is further great temporal variation in the simulated sediment distribution field. This temporal variation cannot be validated based on the data available here. On the other hand it cannot be ruled out that the grab samples for the MOM survey may have been taken at a time with little organic matter actually lying on the bottom mud thickness. It is thus possible that single grab samples is not always a good way to assess the impact of fish farm derived near and on the bottom, since a lot of this matter may be in suspension more often than lying on the bottom. The matter is still present, however, and should be considered in an assessment of the benthic state, and in overall impacts on the biota.
Although, DREAM takes into consideration seabed erosion and resuspension, the composition and type of sediment matters (Law et al., 2016). Refining the description of these processes is a point of potential improvement.
Further Validation
Although, the model system is able to resolve important variables measured in the present management regime, a more thorough validation should be performed. More frequent and denser spatial sampling must be considered in order to fully validate this simulation tool. Furthermore, more spatially resolved current data should be collected alongside the sediment sampling and sediment trap sampling in order to evaluate more thoroughly the relative contributions of the environmental (e.g., water currents) and production (e.g., fecal size distribution and density) variables on the distribution of deposits. Finally, far field dispersal should be validated/investigated. The simulation results presented here indicate that significant amounts of particulate matter may be transported several km away from the release point (Table 2).
Implications for Surveys of Benthic State, Management, and Operational Planning
The simulation results indicate several patches of high mud thickness/high concentrations of organic matter within the farm not covered by any of the points where grab samples were taken, and also several patches up to 0.5 km from the farm (Figures 7, 8). The temporal variation, in particular at the coastal location, was considerable, with the possibility that the state at a point changed from one category to another in a matter of hours [e.g., samples 7 and 9 at location 2 (array I), Figure 10B]. This suggests that a complete assessment of the benthic state under a fish farm must be based on more than a few grab samples taken at large time intervals. Sampling must be dense both in time and space, though spatially discrete sampling has its obvious limitations (Jansen et al., 2016). Alternatively, instruments capable of continuously monitoring essential parameters, either directly or by proxy, could be used. Model tools such as the present one should be included in sample planning as far as possible. The models used must be capable of reproducing high resolution water current fields, since otherwise important information may be lost.
The present model system may inform site selection and operational planning. With a high resolution hydrodynamic model it is possible to differentiate between the releases from different cages. Therefore, the system can be used not only for finding a general location for placing the cage, but also in the process of orienting and positioning the cages and the farm. The optimal timing for using different cages in the farm for different generations and sizes of fish for minimization of the benthic impacts may be estimated. This could also be used to plan optimal fallowing periods and regimes. The model system may further be applied to study the dispersal potential of various chemical compounds used in salmon production (e.g., lice-treatment compounds) and the dispersal of fouling particles from the process of cleaning cages.
While the focus of this study has been on three farms in a specific Norwegian region, the results are of general interest for knowledge based management of the aquaculture industry because they highlight the potential importance of the physical dynamics in assessing the benthic state of the region around an aquaculture operation. The results further indicate that discrete sampling of the bottom sediments at a few points within a cage or aquaculture system may not fully account for the actual benthic state. The model systems used are generic in the sense that they may be established for any region anywhere given appropriate forcing data like bottom topography and atmospheric data in addition to the farm production data. The system is applicable to other fish or aquaculture species with appropriate re-parametrization. SINMOD's ecosystem module (Wassmann et al., 2006; Broch et al., 2013) has a number of biogeochemical variables that may be coupled with the dispersal model. For example, SINMOD may simulate oxygen concentrations and hence the potential for deteriorating water quality in situations with a high influx of particulate matter and high temperatures. This will be the focus of future work.
Author Contributions
OB, IE, RN, RD, GS, EB, and JR all contributed to the planning and design of the study. OB and IE did hydrodynamic modeling using SINMOD. RN and RD performed the DREAM setup and simulations. GS and EB acquired and prepared the bathymetry and production data. JR did hydrodynamic measurements and performed the MOM surveys. All authors contributed to analysis of results and the writing of the paper.
Conflict of Interest Statement
The authors declare that the research was conducted in the absence of any commercial or financial relationships that could be construed as a potential conflict of interest.
At the time of writing of this article, EB was employed by SalMar Farming AS. SalMar Farming AS did not contribute financial support to the research or writing of the paper.
Acknowledgments
This research was funded by the Research Council of Norway through the CREATE Centre for Research driven Innovation. We thank SalMar Farming AS for providing feed and production data; Rune Olden (manager at Tristeinen), Christer Johansen (manager at Rataren), Kjell Ove Betten (manager at Korsneset), Guttorm Lange (ACE—AquaCulture Engineering) for technical and practical information and assistance. Computational resources were partially supplied through NOTUR. We thank the HPC centre at UiT—The Arctic University for expert human support.
References
Anon (2011). LOfoten and VEsterålen CURrents (LOVECUR). Comparison of Hindcasts with MEasurements. Camden, ME: Forristall Ocean Engineering, Inc.
Bannister, R. J., Johnsen, I. A., Hansen, P. K., Kutti, T., and Asplin, L. (2016). Near- and far-field dispersal modelling of organic waste from Atlantic salmon aquaculture in fjord systems. ICES J. Mar. Sci. 73, 2408–2419. doi: 10.1093/icesjms/fsw027
Bannister, R. J., Valdemarsen, T., Hansen, P. K., Holmer, M., and Ervik, A. (2014). Changes in benthic sediment conditions under an Atlantic salmon farm at a deep, well-flushed coastal site. Aquacult. Environ. Interact. 5, 29–47. doi: 10.3354/aei00092
Black, K. P., and Vincent, C. E. (2001). High-resolution field measurements and numerical modelling of intra-wave sediment suspension on plane beds under shoaling waves. Coast. Eng. 42, 173–197. doi: 10.1016/S0378-3839(00)00058-2
Brager, L. M., Cranford, P. J., Grant, J., and Robinson, S. M. C. (2014). Spatial distribution of suspended particulate wastes at open-water Atlantic salmon and sablefish aquaculture farms in Canada. Aquacult. Environ. Interact. 6, 135–149. doi: 10.3354/aei00120
Broch, O. J., Ellingsen, I. H., Forbord, S., Wang, X., Volent, Z., Alver, M. O., et al. (2013). Modelling the cultivation and bioremediation potential of the kelp Saccharina latissima in close proximity to an exposed salmon farm in Norway. Aquacult. Environ. Interact. 4, 187–206. doi: 10.3354/aei00080
Buryniuk, M., Petrell, R. J., Baldwin, S., and Lo, K. V. (2006). Accumulation and natural disintegration of solid wastes caught on a screen suspended below a fish farm cage. Aquacult. Eng. 35, 78–90. doi: 10.1016/j.aquaeng.2005.08.008
Carroll, M. L., Cochrane, S., Fieler, R., Velvin, R., and White, P. (2003). Organic enrichment of sediments from salmon farming in Norway: environmental factors, management practices, and monitoring techniques. Aquaculture 226, 165–180. doi: 10.1016/S0044-8486(03)00475-7
Chang, B. D., Page, F. H., Losier, R. J., and McCurdy, E. P. (2014). Organic enrichment at salmon farms in the Bay of Fundy, Canada: DEPOMOD predictions versus observed sediment sulfide concentrations. Aquacult. Environ. Interact. 5, 185–208. doi: 10.3354/aei00104
Cromey, C. J., Nickell, T. D., and Black, K. D. (2002a). DEPOMOD-modelling the deposition and biological effects of waste solids from marine cage farms. Aquaculture 214, 211–239. doi: 10.1016/S0044-8486(02)00368-X
Cromey, C. J., Nickell, T. D., Black, K. D., Provost, P. G., and Griffiths, C. R. (2002b). Validation of a fish farm waste resuspension model by use of a particulate tracer discharged from a point source in a coastal environment. Estuaries 25, 916–929. doi: 10.1007/BF02691340
Cromey, C. J., Nickell, T. D., Treasurer, J., Black, K. D., and Inall, M. (2009). Modelling the impact of cod (Gadus Morhua L.) farming in the marine environment-CODMOD Aquaculture 289, 42–53. doi: 10.1016/j.aquaculture.2008.12.020
Dee, D. P., Uppala, S. M., Simmons, A. J., Berrisford, P., Poli, P., Kobayashi, S., et al. (2011). The ERA-interim reanalysis: configuration and performance of the data assimilation system. Q. J. R. Meteorol. Soc. 137, 553–597. doi: 10.1002/qj.828
Ellingsen, I., Slagstad, D., and Sundfjord, A. (2009). Modification of water masses in the Barents Sea and its coupling to ice dynamics: a model study. Ocean Dyn. 59, 1095–1108. doi: 10.1007/s10236-009-0230-5
Ervik, A., Hansen, P. K., Aure, J., Stigebrandt, A., Johannessen, P., Jahnsen, T., et al. (1997). Regulating the local environmental impact of intensive marine fish farming, I. The concept of the MOM system (modelling-ongrowing fish farms-monitoring). Aquaculture 158, 85–94. doi: 10.1016/S0044-8486(97)00186-5
FAO (2012). FAO Yearbook. Fishery and Aquaculture Statistics. Rome. Available online at: http://www.fao.org/3/478cfa2b-90f0-4902-a836-94a5dddd6730/i3740t.pdf
Gullestad, P., Bjørgo, S., Eithun, I., Ervik, A., Gudding, R., Hansen, H., et al. (2011). Effektiv Bærekraftig Arealbruk I Havbruksnæringen - Areal Til Begjær. Rapport Fra Ekspertutvalg Til Fiskeri Og Kystdepartementet, Bergen.
Hansen, P. K., Ervik, A., Schaanning, M., Johannessen, P., Aure, J., Jahnsen, T., et al. (2001). Regulating the local environmental impact of intensive, marine fish farming: II. The monitoring programme of the MOM system (modelling–ongrowing fish farms–monitoring). Aquaculture 194, 75–92. doi: 10.1016/S0044-8486(00)00520-2
Hersoug, B. (2013). The Battle for Space - The Position of Norwegian Aquaculture in Integrated Coastal Zone Planning, 159–168. Available online at: http://www.scopus.com/inward/record.url?eid=2-s2.0-84889306648&partnerID=40&md5=20c41b04d8e40b3de676a189726f1ed5
Ichiye, T. (1967). Upper ocean boundary-layer flow determined by dye diffusion. Phys. Fluids 10, S270. doi: 10.1063/1.1762467
Jansen, H. M., Reid, G. K., Bannister, R. J., Husa, H., Robinson, S. M. C., Cooper, J. A., et al. (2016). Discrete water quality sampling at open-water aquaculture sites: limitations and strategies. Aquacult. Environ. Interact. 8, 463–480. doi: 10.3354/aei00192
Kalantzi, I., and Karakassis, I. (2006). Benthic impacts of fish farming: meta-analysis of community and geochemical data. Mar. Pollut. Bull. 52, 484–493. doi: 10.1016/j.marpolbul.2005.09.034
Keeley, N. B., Cromey, C. J., Goodwin, E. O., Gibbs, M. T., and Macleod, C. M. (2013). Predictive depositional modelling (DEPOMOD) of the interactive effect of current flow and resuspension on ecological impacts beneath salmon farms. Aquacult. Environ. Interact. 3, 275–291. doi: 10.3354/aei00068
Klebert, P., Patursson, Ø., Endresen, P. C., Rundtorp, P., Birkevold, J., and Rasmussen, H. W. (2015). Three-dimensional deformation of a large circular flexible sea cage in high currents: field experiment and modeling. Ocean Eng. 104, 511–520. doi: 10.1016/j.oceaneng.2015.04.045
Law, B. A., Hill, P. S., Maier, I., Milligan, T. G., and Page, F. (2014). Size, settling velocity and density of small suspended particles at an active salmon aquaculture site. Aquacult. Environ. Interact. 6, 29–42. doi: 10.3354/aei00116
Law, B. A., Hill, P. S., Milligan, T. G., and Zions, V. (2016). Erodibility of aquaculture waste from different bottom substrates. Aquacult. Environ. Interact. 8, 575–584. doi: 10.3354/aei00199
Martinez-Garcia, E., Carlsson, M. S., Sanchez-Jerez, P., Sánchez-Lizaso, J. L., Sanz-Lazaro, C., and Holmer, M. (2015). Effect of sediment grain size and bioturbation on decomposition of organic matter from aquaculture. Biogeochemistry 125, 133–148. doi: 10.1007/s10533-015-0119-y
Mazzola, A., Mirto, S., La Rosa, T., Fabiano, M., and Danovaro, R. (2000). Fish-farming effects on benthic community structure in coastal sediments: analysis of meiofaunal recovery. ICES J. Mar. Sci. 57, 1454–1461. doi: 10.1006/jmsc.2000.0904
Ogunkoya, A. E., Page, G. I., Adewolu, M. A., and Bureau, D. P. (2006). Dietary incorporation of soybean meal and exogenous enzyme cocktail can affect physical characteristics of faecal material egested by rainbow trout (Oncorhynchus mykiss). Aquaculture 254, 466–475. doi: 10.1016/j.aquaculture.2005.10.032
Olafsen, T., Winther, U., Olsen, Y., and Skjermo, J., and Det Kongelige Norske (2012). Videksabers Selskab (DKNVS) og Norges Teknkiske Vitenskapsakademi (NTVA), Verdiskapning Basert På Produktive Hav I 2050 (Det Kongelige Norske Videksabers Selskab (DKNVS) og Norges Teknkiske Vitenskapsakademi. NTVA.
Olsen, Y. (2011). Resources for fish feed in future mariculture. Aquacult. Environ. Interact. 1, 187–200. doi: 10.3354/aei00019
Reed, M., and Hetland, B. (2002). “DREAM: a dose-related exposure assessment model technical description of physical-chemical fates components,” in Proceedings of SPE International Conference on Health, Safety and Environment in Oil and Gas Exploration and Production (Society of Petroleum Engineers). Available online at: http://www.onepetro.org/mslib/servlet/onepetropreview?id=00073856&soc=SPE (Accessed December 10, 2012).
Reid, G. K., Liutkus, M., Robinson, S. M. C., Chopin, T. R., Blair, T., Lander, T., et al. (2009). A review of the biophysical properties of salmonid faeces: implications for aquaculture waste dispersal models and integrated multi-trophic aquaculture. Aquacult. Res. 40, 257–273. doi: 10.1111/j.1365-2109.2008.02065.x
Rye, H., Johansen, O., Durgut, I., Ditlevsen, M. K., and Ditlevsen, K. (2006). Restitution of an Impacted Sediment. Available online at: http://www.sintef.no/project/ERMS/Reports/ERMSreportno21_Restitution_SINTEF.pdf
Rye, H., Reed, M., and Ekrol, N. (1998). The PARTRACK model for calculation of the spreading and deposition of drilling mud, chemicals and drill cuttings. Environ. Modell. Softw. 13, 431–441. doi: 10.1016/S1364-8152(98)00048-6
Shields, A. (1936). Application of similarity principles and turbulence research to bed-load movement. Mitt. Preuss. Versuchsanst. Wasserbau Schiffbau 26, 47.
Skarðhamar, J., and Svendsen, H. (2005). Circulation and shelf–ocean interaction off North Norway. Continen. Shelf Res. 25, 1541–1560. doi: 10.1016/j.csr.2005.04.007
Slagstad, D., and McClimans, T. A. (2005). Modeling the ecosystem dynamics of the barents sea including the marginal ice zone: physical, I., and chemical oceanography. J. Mar. Syst. 58, 1–18. doi: 10.1016/j.jmarsys.2005.05.005
Slagstad, D., Tande, K., and Wassman, P. (1999). Modelled carbon fluxes as validated by field dta on the North Norwegian shelf during the productive period in 1994. Sarsia 84, 303–317. doi: 10.1080/00364827.1999.10420434
Stigebrandt, A., Aure, J., Ervik, A., and Hansen, P. K. (2004). Regulating the local environmental impact of intensive marine fish farming: III. A model for estimation of the holding capacity in the modelling–ongrowing fish farm–monitoring system. Aquaculture 234, 239–261. doi: 10.1016/j.aquaculture.2003.11.029
Støle-Hansen, K., and Slagstad, D. (1991). Simulation of currents, ice melting, and vertical mixing in the barents sea using a 3-D Baroclinic Model. Polar Res. 10, 12. doi: 10.3402/polar.v10i1.6725
Sundfjord, A., Ellingsen, I., Slagstad, D., and Svendsen, H. (2008). Vertical mixing in the marginal ice zone of the northern Barents Sea—results from numerical model experiments. Deep Sea Res. II 55, 2154–2168. doi: 10.1016/j.dsr2.2008.05.027
Uglem, I., Knutsen, Ø., Kjesbu, O. S., Hansen, Ø. J., Mork, J., Bjørn, P. A., et al. (2012). Extent and ecological importance of escape through spawning in sea-cages for Atlantic Cod. Aquacult. Environ. Interact. 3, 33–49. doi: 10.3354/aei00049
Wang, X., Olsen, L. M., Reitan, K. I., and Olsen, Y. (2012). Discharge of nutrient wastes from salmon farms: environmental effects, and potential for integrated multi-trophic aquaculture. Aquacult. Environ. Interact. 2, 267–283. doi: 10.3354/aei00044
Wassmann, P., Slagstad, D., Riser, C. W., and Reigstad, M. (2006). Modelling the ecosystem dynamics of the barents sea including the marginal ice zone: II. Carbon flux and interannual variability. J. Mar. Syst. 59, 1–24. doi: 10.1016/j.jmarsys.2005.05.006
Wu, Y., Chaffey, J., Law, B., Greenberg, D. A., Drozdowski, A., Page, F., et al. (2014). A three-dimensional hydrodynamic model for aquaculture: a case study in the Bay of Fundy. Aquacult. Environ. Interact. 5, 235–248. doi: 10.3354/aei00108
Keywords: aquaculture effects, hydrodynamic modeling, fish farm wastes, environmental effects, depositional model, aquaculture dispersal model
Citation: Broch OJ, Daae RL, Ellingsen IH, Nepstad R, Bendiksen EÅ, Reed JL and Senneset G (2017) Spatiotemporal Dispersal and Deposition of Fish Farm Wastes: A Model Study from Central Norway. Front. Mar. Sci. 4:199. doi: 10.3389/fmars.2017.00199
Received: 21 February 2017; Accepted: 09 June 2017;
Published: 28 June 2017.
Edited by:
Simone Libralato, National Institute of Oceanography and Experimental Geophysics, ItalyReviewed by:
Gianluca Sarà, University of Palermo, ArgentinaArthur Capet, University of Liège, Belgium
Matteo Sinerchia, Consiglio Nazionale Delle Ricerche (CNR), Italy
Copyright © 2017 Broch, Daae, Ellingsen, Nepstad, Bendiksen, Reed and Senneset. This is an open-access article distributed under the terms of the Creative Commons Attribution License (CC BY). The use, distribution or reproduction in other forums is permitted, provided the original author(s) or licensor are credited and that the original publication in this journal is cited, in accordance with accepted academic practice. No use, distribution or reproduction is permitted which does not comply with these terms.
*Correspondence: Ole J. Broch, b2xlLmphY29iLmJyb2NoQHNpbnRlZi5ubw==