- 1Hellenic Centre for Marine Research, Institute of Marine Biological Resources and Inland Waters, Iraklion, Greece
- 2IFREMER, Sete, France
- 3Institut National des Sciences et Technologies de la Mer, La Goulette, Centre de la Goulette, Port de Pêche la Goulette, La Goulette, Tunisia
- 4Istituto per l'Ambiente Marino Costiero, Consiglio Nazionale delle Ricerche, Campobello di Mazara, Italy
- 5Instituto Espanol de Oceanografia, Centro Oceanografico de Baleares, Palma de Mallorca, Spain
- 6Istituto di Scienze Marine, Consiglio Nazionale delle Ricerche, Ancona, Italy
Our knowledge for the distribution of Atlantic mackerel (Scomber scombrus) in the Mediterranean Sea is limited and fragmented. In the current work habitat suitability modeling was applied to summer acoustic surveys data of Atlantic mackerel juveniles derived from the north part of the Mediterranean (i.e., acoustic data from the Gulf of Lions, pelagic trawls held during acoustic surveys in Spanish Mediterranean waters, south Adriatic Sea, Strait of Sicily, and North Aegean Sea) using generalized additive models (GAMs) along with satellite environmental and bathymetry data. Bathymetry along with sea surface temperature and circulation patterns, expressed through sea level anomaly and the zonal component of the absolute geostrophic velocity, were the environmental variables best to describe nursery grounds. The selected model was used to produce maps presenting the potential nursery grounds of Atlantic mackerel throughout the Mediterranean Sea as a measure of habitat adequacy. However, the assessed potential nursery grounds were generally marked as “occasional,” implying that although there are areas presenting high probability to encounter Atlantic mackerel, this picture can largely vary from year to year stressing the high susceptibility of the species to environmental conditions. In a further step and toward a spatial management perspective, we have estimated and visualized the overlap between Atlantic mackerel and anchovy/ sardine juvenile grounds throughout the basin. Results showed that although the degree of overlapping was generally low, not exceeding 15% in general, this varied at a regional level going up to 30%. The potential of the output of this work for management purposes like the implementation of spatially-explicit management tools is discussed.
Introduction
It is well-known that the most landed small pelagic species in the Mediterranean Sea are anchovy Engraulis encrasicolus, and sardine, Sardina pilchardus (FAO, 2016). As a consequence the majority of pelagic surveys in the Mediterranean used mainly for stock assessment purposes target these two species. However, the ecosystem approach to fisheries entails the need to update our knowledge also for species that might contribute less in the total landings or have lower economic value but play an important ecological role in the ecosystem. This is the case of Atlantic mackerel (Scomber scombrus) in the Mediterranean Sea. Purse seiners is the main gear targeting Atlantic mackerel in the basin with midwater pelagic trawls also operating in the Adriatic Sea, the Strait of Sicily and the French coastal waters. Genetic analysis has revealed a single panmictic population in the northern-central Adriatic Sea, distinct from the population of the southern Adriatic Sea (Papetti et al., 2013). Moreover, midwater trawl landings decreased and the age structure appears shrinked in the north central Adriatic Sea, which might translate a population decline (Meneghesso et al., 2013). However, our knowledge on the status of Atlantic mackerel Mediterranean stocks is totally absent (no stock assessment of mackerel available in GFCM Stock Assessment Working Groups) and info on the species biology in the Mediterranean is very limited. However, the high importance of these stocks in the Mediterranean and the need for stock assessment was recently highlighted (STECF, 2016).
Fine-scale knowledge on the distribution of species and habitats is crucial for effective management and the conservation of marine resources. Ecosystem approach to fisheries impairs the need to develop spatially-explicit management tools, integrating fisheries and ecosystem objectives regionally within one single management scheme. Conservation prioritization exercises require good quality information on the spatial distribution of species and their associated habitats, including different life history stages. Moreover, the increasing number of studies on the effect of climate change on fisheries resources (e.g., Brander, 2010; Pecl et al., 2014; Fortibuoni et al., 2015; Gamito et al., 2015), stresses the need to improve our understanding of past changes in fish distribution to allow reliable future projections. This is even more important for species such as Atlantic mackerel as their spawning habitat in the Atlantic is known to have shifted northward in the last three decades probably in response to global sea warming (Bruge et al., 2016). Habitat heterogeneity could, however, produce more complex patterns than a simple polewards translation in distribution (Hughes et al., 2014) and the environmental complexity of the Mediterranean Sea can be a good example.
Atlantic mackerel is known to be a cold water spawner (Hughes et al., 2014). The Northeast Atlantic mackerel population is known to spawn from January to July along the continental shelf edge from Portuguese to Scottish waters and in the North Sea (Reid et al., 1997, 2001; Villamor et al., 1997; Beare and Reid, 2002). After spawning, this population is known to perform seasonal migrations between feeding and spawning grounds, migrating toward the North Sea and Norwegian Sea to feed. In the Mediterranean, our knowledge on Atlantic mackerel spawning mainly comes from the Adriatic Sea. Atlantic mackerel is reported to spawn from October to April in the eastern part of the Adriatic Sea (Sinovčić, 2001) and from December to April in the western part of the Adriatic Sea (Bottari et al., 2004; Tsikliras et al., 2010; Meneghesso et al., 2013) with a probable peak in January (Gamulin and Hure, 1983; Sinovčić, 2001; Bottari et al., 2004; Meneghesso et al., 2013). As there are no dedicated surveys for this species in the Mediterranean, our knowledge on the distribution grounds, the spawning and nursery habitat of the species mainly relies on past studies of certain areas in the western and central part of the basin. In particular, winter spawning areas have been identified adjacent to the Palagruza Island, offshore of Dugi Otok as well as in the Istrian waters in the Adriatic Sea (Gamulin and Hure, 1983; Sinovčić, 2001). The recent findings of Papetti et al., 2013) suggest that S. scombrus could perform an autumn–winter migration from the northern to the central Adriatic to reach a spawning ground common to different stocks in the northern–central Adriatic. Juvenile grounds have been shown at the coast of Bari in the Adriatic Sea (Bottari et al., 2004) as well as in the Gulf of Gabes (Ghailen et al., 2010) in Tunisian waters and in the area associated to the Ebro river outflow (Sardà et al., 2006) in Spanish Mediterranean waters. In the eastern Mediterranean, information on the distribution grounds is practically absent. In the current paper we aim at pooling local information to assess potential nursery grounds at bigger scale issues and cover to the extent possible our gaps in knowledge.
Quantifying the distribution of a species along environmental gradients (e.g., Guisan and Zimmermann, 2000; Planque et al., 2007; Bellido et al., 2008; Weber and McClatchie, 2010; Giannoulaki et al., 2013) can provide habitat maps presenting geographic areas where environmental variables, in the absence of explicit biotic interactions (such as competition or predation) are considered suitable for the presence of a particular species. This approach has been successfully applied in the Mediterranean to define the potential spawning and nursery grounds of anchovy (Giannoulaki et al., 2013) and sardine (Giannoulaki et al., 2011; Tugores et al., 2011). In the present study, we used data from standardized acoustic surveys that have been carried out routinely in the European Mediterranean areas and applied habitat modeling techniques to identify areas throughout the basin where juvenile mackerels present a high likelihood of long-term persistence, thus defining areas of particular importance for the maintenance of the stocks. Preferential/occasional mackerel habitat areas were defined based on probability, variability and persistence maps (Bellier and Planque, 2007; Giannoulaki et al., 2011, 2013) obtained for the examined periods. This is the first time for Atlantic mackerel that species distribution modeling is applied, linking the species spatial distribution with environmental variables and resulted maps cover the entire Mediterranean Sea.
As the small pelagic fishery is largely a mixed species fishery in the Mediterranean targeting mainly anchovy and sardine, we considered important to compare the assessed nursery grounds of Atlantic mackerel with the respective nurseries of anchovy and sardine. Given the occurrence of minimum landings size or Minimum Conservation Reference Size for these three species according to the EU Regulation for the Mediterranean (European Commission, 2006) and the recent discard ban (EU Regulation, European Commission, 2013) assessing areas where the small pelagic fishery is likely to result into high discard rate of undersized individuals is highly important from a management perspective.
Materials and Methods
Study Areas
In order to look at the distribution along an environmental gradient, we tried to combine data covering to the maximum possible extent Western, Central and Eastern parts of the Mediterranean; exhibiting both contrasting environment and distant locations, i.e., narrow vs. wide continental shelves, warm vs. cooler water and different nutrient inputs from river outflows associated with different productivity levels. Such diversity of conditions should help defining the habitat suitability and avoiding too much extrapolation. Unfortunately, data from the South of the Mediterranean were not available to complement the environmental gradient and some assumptions had to be made to assess the habitat suitability of these areas.
Western Mediterranean Waters: The Spanish Mediterranean Waters and the Gulf of Lions
In the Spanish Mediterranean waters (Figure 1), the continental shelf is generally narrow, being wider in the surroundings of the Ebro River. The circulation is dominated by the inflow of less saline Atlantic waters through the Strait of Gibraltar that generate upwelling and a local enrichment with nutrients and enhanced primary production in the Alboran Sea (Champalbert, 1996). Moreover, the North Current, a cyclonic along-slope front flowing southwards and the outflow of fresh water from large rivers (i.e., the Ebro), characterises this area. The Gulf of Lions (Figure 1) is one of the most productive zones in the western Mediterranean Sea owing to a number of hydrographic features such as the existence of a wide continental shelf, river run-off, and strong vertical mixing during winter. Occasionally coastal upwellings are generated by local wind systems and complex orographic effects (Millot, 1990; Lloret et al., 2001).
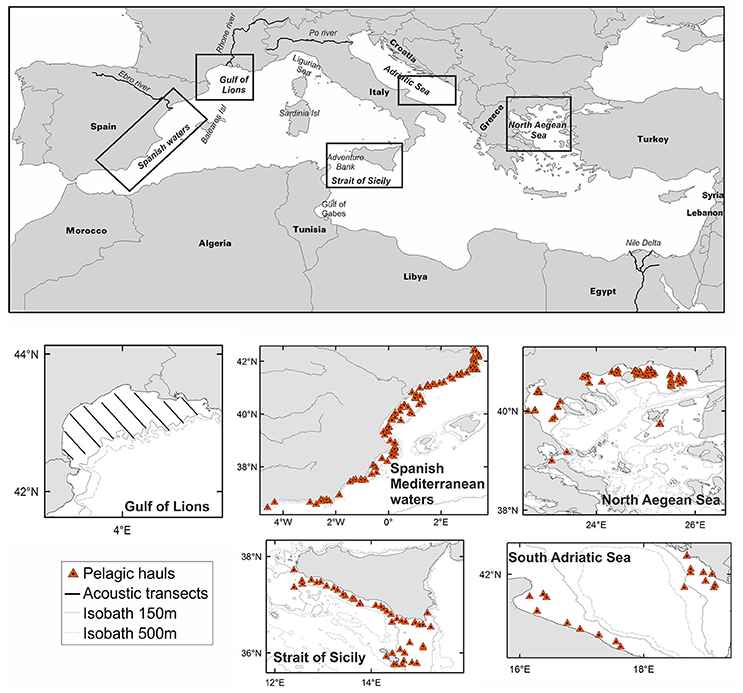
Figure 1. Map of the study areas. Acoustic transects in the Gulf of Lions and the position of pelagic trawls held within acoustic surveys used for analysis in each study area are shown. Toponyms mentioned in the text as well as the position of the main rivers and bathymetry are also indicated.
Central Mediterranean Sea: South Western Adriatic Sea and Strait of Sicily
The south western Adriatic Sea is a sub basin located in the Central Mediterranean (Figure 1), characterized by complex stratification and circulation. In the southern Adriatic the seasonal thermocline extends down to ~75 m with a maximum at 150 m. Homogeneous water properties, forming a different deep water mass, are found below 150 m (Artegiani et al., 1997).
The Strait of Sicily (Figure 1) connects the western and the eastern Mediterranean basins. It has a fairly narrow (15 nmi) continental shelf in the middle of the southern coast but it widens in both its eastern and western parts. The surface circulation is largely determined by the Atlantic-Ionian Stream and its interannual variability has a large impact on upwelling extent and the formation of frontal structures (Cuttitta et al., 2003; Basilone et al., 2013; Bonanno et al., 2014). The upwelling is further reinforced by wind-induced events (Patti et al., 2010).
Eastern Mediterranean Sea: North Aegean Sea
The North Aegean Sea (Figure 1) is characterized by high hydrological complexity mostly related to the Black Sea water (BSW) that enters the Aegean Sea through the Dardanelles strait. The outflow of BSW enhances local productivity and its advection in the Aegean Sea induces high hydrological and biological complexity (Somarakis and Nikolioudakis, 2007). This is further enhanced by the presence of a series of large rivers that outflow into semi-closed gulfs (Giannoulaki et al., 2013).
Mackerel Samplings
As no suitable data were available from the specific summer surveys to estimate length at maturity (L50), this was based on the available literature review from the Atlantic (L50 = 28.6 cm, Froese and Pauly, 2016) and the Adriatic Sea (smallest mature female was 20 cm of fork Length and the smallest male was 20.5 cm, Bottari et al., 2004). However, according to Collette and Nauen, 1983) mackerel may have to wait for ~30 cm to reach maturity in the eastern part of the Mediterranean. Thus within a precautionary approach, individuals with total length <20 cm were selected as juveniles for our analyses.
Acoustic data from standard monitoring stock assessment surveys held in the Gulf of Lions (Western Mediterranean) were used to model the presence of Atlantic mackerel juveniles during summer. No spatially explicit acoustic data were available from other Mediterranean areas as spatially explicit echo is obtained only for anchovy and sardine. Subsequently, spatially explicit catch data from pelagic hauls as collected during standard monitoring stock assessment acoustic surveys in the North Aegean Sea (Eastern Mediterranean), the south western part of the Adriatic Sea, the Strait of Sicily (Central Mediterranean), Spanish Mediterranean Waters (Western Mediterranean) were also used to cover a wider area extent.
Acoustic Sampling
Acoustic sampling was performed in the Gulf of Lions during summer for the period (July 2004–2010) by means of scientific split-beam echosounders (Simrad EK500, Simrad EK60) and frequencies used at 38, 70, 120, and 200 kHz, calibrated following standard techniques (Foote et al., 1987). Data were collected on board the R/V “L'EUROPE” along predetermined parallel transects, perpendicular to the bathymetry 12 nautical miles (nmi, 1.852 km) apart and were recorded at a constant speed of 8 nmi h−1. Minimum sampling depth varied between 10 and 20 m depending on the area. The size of the Elementary Distance Sampling Unit (EDSU) was one nmi. Acoustic data analysis was made using the Movies+ software. Midwater pelagic trawl sampling was carried out in order to identify and verify mackerel echotraces as well as to assess the size distribution and distinguish between juveniles and adults. Mackerel juveniles' echoes discrimination was based on (a) the echogram shape of the schools (b) the output of adjusted algorithm based on frequency response curves and (c) the catch composition of concurrent pelagic trawls (Simmonds and MacLennan, 2005).
Pelagic Hauls Sampling
A set of 238 pelagic hauls held over the period 2003–2010 during summer acoustic surveys was used derived from acoustic surveys held in Spanish Mediterranean waters, the Strait of Sicily, the south western Adriatic and the North Aegean Seas. Details are described in Table 1 as well as in Giannoulaki et al. (2013). In addition, only hauls with adequate percentage of Atlantic mackerel juveniles' were considered as positive (i.e., hauls with Atlantic mackerel juveniles' exceeding 20% of the total catch of Atlantic mackerel in terms of number of individuals). So, at each haul location x, an indicator of Atlantic mackerel juveniles presence I(x) was defined as I(x) = 1, if the percentage of juveniles catch was over 20%, and I(x) = 0 otherwise. Using this criterion, we retained a total of 93 positive Atlantic mackerel juvenile's hauls.
Environmental Data
Satellite environmental data as well as bottom depth were used as explanatory variables to model the habitat of Atlantic mackerel juveniles in the Mediterranean Sea. The area is well-monitored in terms of monthly satellite imagery (summarized in Table 2). Specifically, sea surface temperature (SST in °C), sea surface chlorophyll concentration (CHLA in mg m−3), sea level anomaly (SLA in cm), the meridional component of the absolute geostrophic velocity current (VADT in m s−1, surface northward geostrophic sea water velocity) and zonal component of the absolute geostrophic velocity (UADT in m s−1, surface eastward geostrophic sea water velocity, negative values indicate west direction, positive values indicate east direction) were downloaded from respective databases (see Table 2) and used. These environmental variables are considered important either as a direct influence on the distribution of mackerels (e.g. SST, CHLA, geostrophic velocity) or as proxies for causal factors (Bellido et al., 2001; Basilone et al., 2004, 2006; Giannoulaki et al., 2011, 2013; Tugores et al., 2011). For example, SLA varies with ocean processes such as gyres, meanders, and eddies (Larnicol et al., 2002; Pujol and Larnicol, 2005) which enhance productivity and often function as physical barriers differentiating the distribution of species or species life stages. Negative SLA values are related to upwelling processes whereas positive ones to downwelling processes. Bathymetry, as an indirect factor, was derived from the EMODnet Bathymetry portal (http://www.emodnet-bathymetry.eu). All monthly-averaged satellite images from daily measurements were processed as regular grids under a GIS (Geographic Information Systems) environment using ArcInfo GRID software. Satellite variables were mostly used at their best available resolution provided by the online satellite data distribution archives (Table 2) in order to obtain environmental characteristics for each sampling point at an average spatial resolution of 1.5 km (Valavanis et al., 2008), adequately defining environmental spatial heterogeneity in relation to both the applied EDSU (1.852 km) of acoustic data and the best available resolution of the explanatory environmental variables.
Data Analyses
Habitat Modeling
Generalized additive models (GAMs; Hastie and Tibshirani, 1990) were applied in a presence/absence approach to define the set of environmental factors that describe mackerel juveniles' distribution. The presence/absence approach allowed us to standardize Atlantic mackerel presence from different sources i.e., acoustic sampling and pelagic haul sampling. Furthermore, in order to assess the quality of our models, we had to separate the dataset between a training dataset (data that were used to estimate parameters) and a validation dataset (data that were not used in the model parameterization but used afterwards to assess the quality of the fit). The training dataset was defined in order to ensure (a) merging data from different areas that cover a wider range of environmental conditions (b) merging presence/absence data from acoustic surveys and pelagic hauls we succeeded eliminating any autocorrelation in our training dataset thus avoiding any Type I error in our resulted model and (c) selecting years of data that balanced absence and presence records thus avoiding any prevalence of positive or negative areas that are likely to cause bias to model performance (Fielding and Bell, 1997). As such, GAMs were applied to pooled data from the Gulf of Lions (2006–2010), the North Aegean Sea (2006, 2008) and the Strait of Sicily (2006) to define a “mean” model that we can subsequently use for mapping.
The selection of the GAM smoothing predictors was done using the MGCV library in the R statistical software (R Development Core Team, 2012). In each model fit, a double penalty was applied to the penalized regression solved by MGCV, which allows variables to be solved out of the model entirely (Marra and Wood, 2011), being more robust to identify important features. The degree of smoothing was chosen based on the observed data and the restricted maximum likelihood (REML) estimation that outperforms the generalized cross validation (GCV) smoothing parameter selection, as suggested by Marra and Wood (2011). In order to avoid any bias generated from any possible autocorrelation effect in the spatial structure of our acoustic data, we adjusted to Type I error rate by setting the accepted significance level for each term at the more conservative value of 1%, rather than the usual 5% (Fortin and Dale, 2005). For each case, a final model was built by testing all variables that were considered biologically meaningful starting from a simple initial model with one explanatory variable. The best model was selected based on the minimization of the Akaike's information criterion (AIC) and the level of deviance explained (0–100%; the higher the percentage, the more deviance explained), also taking into account the model's predictive ability. Specifically, as response variable (y), we used the presence / absence of mackerel juveniles. As independent variables (x covariates), we examined the cube root of the bottom depth (to achieve a normal distribution of bottom depth), the natural logarithm of CHLA (to achieve a normal distribution of CHLA), SST, SLA, VADT, and UADT. The binomial error distribution with the logit link function was used and the natural cubic spline smoother (Hastie and Tibshirani, 1990) was applied for GAM fitting. Following the selection of the main effects of the model, all first-order interactions of the main effects were tested using tensor product smoothing as the variables were not on the same scale. To avoid over-fitting the maximum degrees of freedom (measured as number of knots k) allowed to the smoothing functions were limited to the main effects at k = 5. Validation graphs (e.g., residuals vs. fitted values, QQ-plots and residuals vs. the original explanatory variables) were plotted to detect the existence of any pattern and possible model misspecification. Residuals were also checked for autocorrelation. The output of the final selected GAM is presented as plots of the best-fitting smooths. Interaction effects are shown as a perspective plot without error bounds.
Model Validation
In a subsequent step, the final model was tested and evaluated for its predictive performance, in other words the possible error in the predictions. For this purpose we used the validation dataset including data not used for modeling, i.e., (a) acoustic data from the Gulf of Lions in 2003–2005 and pelagic hauls data from (b) North Aegean Sea in 2003, 2005, 2010, (c) the south western Adriatic Sea in 2005, (d) the Strait of Sicily in 2007, 2010 and (e) the Spanish Mediterranean waters in 2009, 2010. The advantage of the specific validation dataset is that it covered to the highest possible extent the entire basin and the prevalence of the positive values was at 0.75 thus reducing the possibility to result into a misleading high overall accuracy using trivial rules when for example the dataset is characterized by low prevalence (Fielding and Bell, 1997). Generally, using the specific pooled dataset we aimed to (a) balance the number of presences and absences as much as possible (b) increase the extent of occurrence/absence data and cover a wide extent of the territory under study and (c) add absences from more environmentally distant locations compared to presences in order to increase the rate of well-predicted absences. We estimated the Receiver Operating Characteristic curve (ROC; Hanley and McNeil, 1982; Guisan and Zimmermann, 2000) and the AUC metric (the area under the ROC), a threshold-independent metric, widely used in the species' distribution modeling literature (Franklin, 2009). As AUC has been criticized as a measure of model performance (Lobo et al., 2008) we have also estimated sensitivity (i.e., the proportion of observed positives that are correctly predicted) and specificity values (i.e., the proportion of observed negatives that are correctly predicted) as a measure of model evaluation (Lobo et al., 2008). We report sensitivity and specificity values in relation to two threshold criteria: (i) the maximization of the specificity-sensitivity sum (MDT) and (ii) the prevalence values (Jiménez-Valverde et al., 2008; Lobo et al., 2008). Moreover, we used a threshold at 0.5 and obtained the matrix of confusion that identified true positive (a), false positive (b), false negative (c) and true negative (d) predicted values. Subsequently, we calculated alternative performance measures including overall prediction success (matching coefficient; Buckland and Elston, 1993), sensitivity, specificity, the odds ratio, Positive predictive power Percentage of predicted absences that were real (PPP), Negative predictive power Percentage of predicted absences that were real (NPP) and Cohen's kappa (Fielding, 1999; Manel et al., 2001). The odds ratio and Cohen's kappa are the two indices that make full use of the information contained in the confusion matrix (Fielding and Bell, 1997). NPP assesses the probability that a case is not correctly predicted. For Cohen kappa, values of 0.0–0.4 are considered to indicate slight to fair model performance, values of 0.4–0.6 moderate, 0.6–0.8 substantial and 0.8–1.0 almost perfect (Manel et al., 2001). Landis and Koch (1977) have suggested the following ranges of agreement for the Kappa statistic: poor K < 0.4; good 0.4 < K < 0.75, and excellent K > 0.75.
Mapping
Based on validation results, the selected model was applied in a predictive mode to provide probability estimates and habitat suitability over a wider grid of mean monthly satellite values at a GIS resolution of 4 km, covering the entire Mediterranean basin (i.e., practically indicating areas where a specific set of values concerning satellite variables occur). Following the technical approach presented in Giannoulaki et al. (2013), annual habitat suitability maps were constructed over the 2004–2010 period at the scale of the Mediterranean. Subsequently, GIS techniques were used to estimate the mean of these annual maps, summarizing the mean average probability estimates at each grid point. The variability map, representing the interannual variability in potential juvenile grounds, was also produced by estimating the standard deviation of the annual maps. Subsequently, preferential and occasional juvenile grounds were defined based on average probability and variability (Bellier and Planque, 2007) and habitat allocation map was created indicating: (i) recurrent/persistent juvenile sites: areas with mean probability >0.5 and standard deviation values <0.12, (ii) occasional juvenile sites: areas with mean probability >0.5 and standard deviation values >0.25 i.e., where mackerel juveniles are present indicating high probability in some years but not in others and (c) rare juvenile sites—areas with low mean and low standard deviation values.
Comparison with Anchovy and Sardine Juveniles' Grounds
In a further step we tried to examine the overlapping between the potential juvenile habitat of Atlantic mackerel with the respective juvenile habitat of anchovy (as estimated in Giannoulaki et al., 2013) and sardine (as estimated by Giannoulaki et al., 2011). The degree of overlapping was estimated based on the following formula using GIS techniques
where 1ik equals one if species i is present on the grid point k, 0 otherwise and 1ijk equals one if both species i and j are present at point k, 0 otherwise.
The degree of overlapping based on the above formula was also estimated per GFCM Geographical Subarea (GSA) level (http://www.fao.org/gfcm/data/map-geographical-subareas/en/).
Results
Habitat Suitability Modeling
The final GAM for pooled data included as main effects: SST, UADT and the interaction of Depth (cubic root transformed) with SLA (Deviance explained = 30.7%, AIC = 1098.73, p << 0.000). Model results (Figure 2) indicated higher probability of finding Atlantic mackerel juveniles present in shallow waters <130 m combined with SLA of −6 to 0 cm (upwelling processes), deeper waters up to 220 m when combined with SLA of 6–8 cm (downwelling processes) as well as SST between 22 and 26°C and UADT values < −0.1 m s−1 and >0.2 m s−1 (Figure 2).
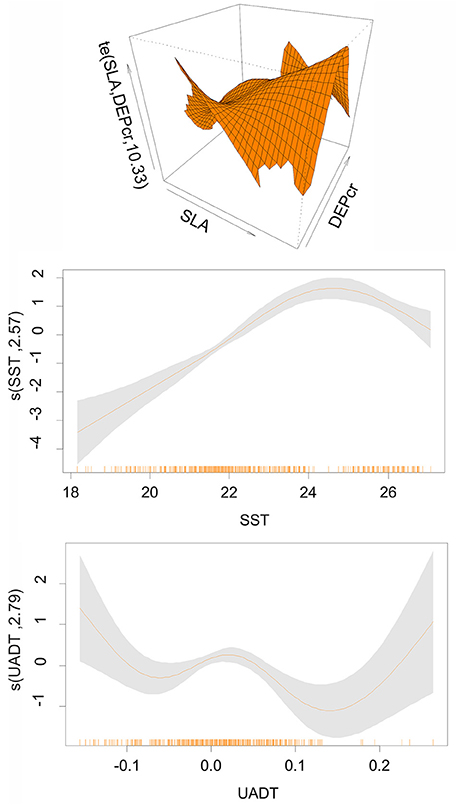
Figure 2. Pooled model: Coefficients of the Generalized Additive Models (GAMs) for Atlantic Mackerel juveniles in summer period (June-July). SST, Sea Surface Temperature (oC); SLA, Sea Level Anomaly (in cm); DEPcr, Bottom Depth (in m); UADT, surface eastward geostrophic sea water velocity in ms−1. The interaction plots are also shown. Thick lines indicate the value of GAMs coefficient. Shadowed areas represent the confidence intervals at p = 0.01. The rug under the single variable effects plots indicates the density of points for different variable values.
The quality of a predictive model is usually judged by its predictive accuracy or otherwise the possible error in its estimates Validation results showed substantial to good model performance based on the Kappa and the Odds ratio values (Table 3). Moreover, the value of the PPP confirms that the model meets our initial objective to assess habitats with high opportunity of occurrence and accurately predict species presence at a rate of 0.6%. Moreover, a value of 0.69 for the AUC means that for 69% of the time a random selection from the positive group will have a score greater than a random selection from the negative class (Fielding and Bell, 1997).
Mapping
The habitat allocation maps as estimated for the study period showed that suitable areas for Atlantic mackerel juveniles are dominated by areas characterized by high probability as well as high variability (occasional habitat, areas characterized by high mean and high standard deviation). From west to east these areas included the continental shelf waters of the Gulf of Lions, the coastal waters of the Baleares, a narrow band along the Moroccan coastline, the coastal waters of the Ligurian Sea and around the island of Sardinia, waters over the banks in the Strait of Sicily, locations in the north eastern part of the Adriatic Sea and along Croatian coastline, the coastal waters of the south eastern Ionian Sea, the continental shelf of North Aegean Sea and the Nile Delta area (Figure 3). Persistent areas were very limited in extent, located in the coastal waters of the Spanish Mediterranean waters, the coastal part of the north eastern Adriatic, the north east part of the Aegean Sea being in proximity with Black sea water input, as well as along a narrow coastal band in the Nile delta region (Figure 3). Moreover, suitable areas were predominantly absent from the Algerian waters, the Libyan waters, the Ionian Sea along the Albanian waters and the coastal part of the Levantine (Syrian and Turkish waters).
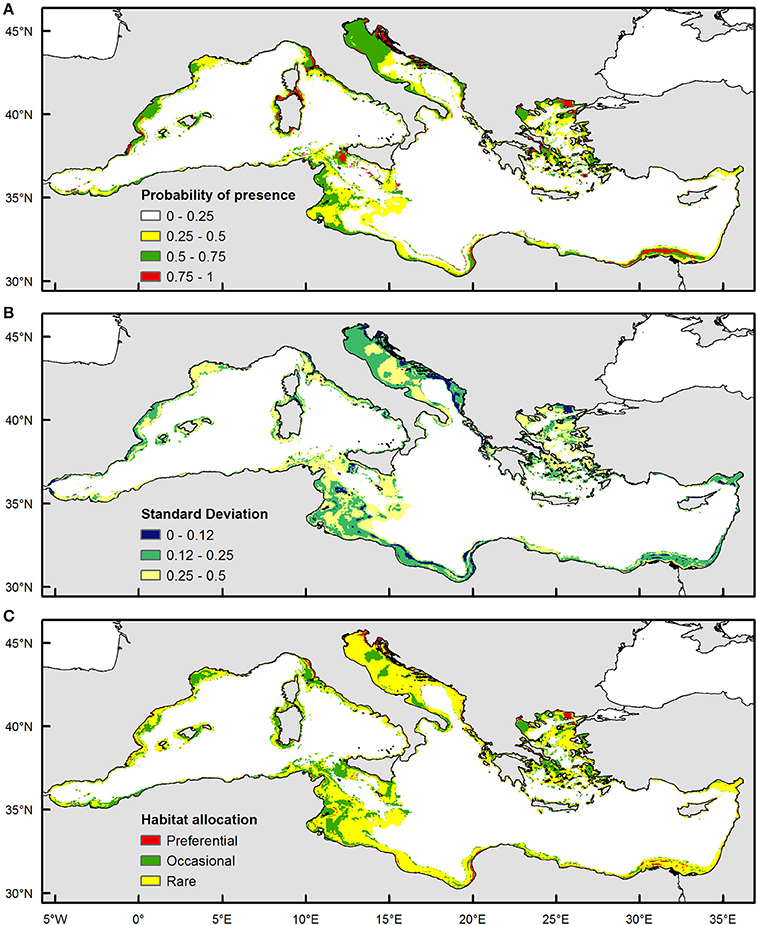
Figure 3. Habitat suitability maps based on pooled model for juvenile Atlantic mackerel in the Mediterranean Sea for June 2003–2010. (A) Mean probability map (B) Standard Deviation Map (C) Habitat allocation map.
Comparison with Anchovy and Sardine Juveniles Potential Habitat
Figure 4 shows the locations characterized as potential juvenile habitat (occasional and persistent) of anchovy / Atlantic mackerel and sardine/Atlantic mackerel, respectively. At first glance the potential juvenile habitat of Atlantic mackerel is much more limited in extent compared to the respective one of anchovy and sardine. The degree of overlapping did not exceed 15% for the entire basin concerning both species. The estimated overlapping index in the western, central and western part of the basin also exhibited values around 15%. However, in the western part of the basin the overlapping was higher with anchovy juvenile grounds whereas in the central and eastern part, higher overlapping was observed with the juvenile grounds of sardine. Certain differences appeared at a regional level with >30% overlapping in the Gulf of Lions (GSA 7) for both species. Looking at the rest of the areas with extended continental shelf, overlap >20% between anchovy and Atlantic mackerel was observed in the Baleares (GSA 5), in the Adventure Bank within the Strait of Sicily (GSA 16) and in the south Adriatic Sea (GSA 18). Regarding sardine and Atlantic mackerel, the >20% overlap in areas with extended continental shelf was observed in the Adventure Bank in the Strait of Sicily (GSA 16), in the south Adriatic Sea (GSA 18), in the Aegean Sea (GSA 22) and in the Egyptian waters (GSA 24).
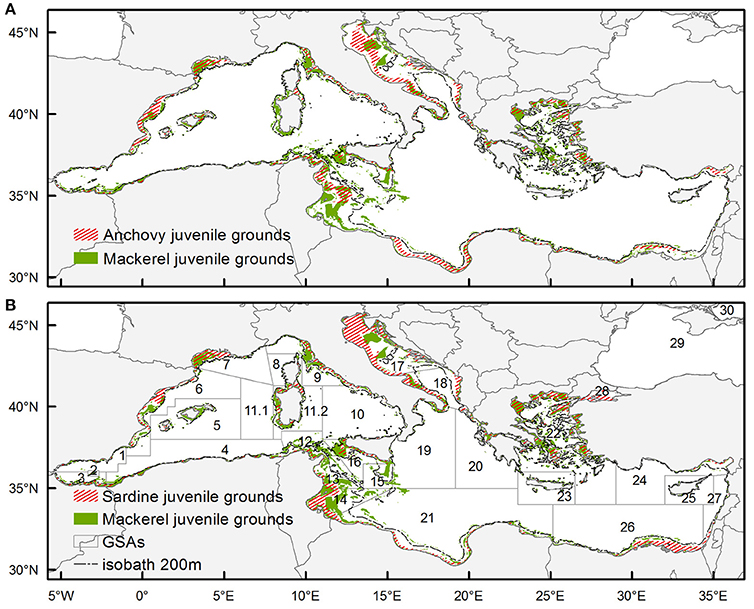
Figure 4. Overlapping of mackerel juveniles' occasional and persistent habitat with (A) anchovy juveniles' occasional and persistent habitat and (B) sardine juveniles occasional and persistent habitat. Anchovy juveniles' habitat is redrawn based on Giannoulaki et al. (2013). Sardine juveniles' habitat is redrawn based on Giannoulaki et al. (2011). GFCM Geographical Subareas (GSAs) (http://www.fao.org/gfcm/data/map-geographical-subareas/en/) are superimposed.
Discussion
Atlantic mackerel population behavior has been an issue for discussion lately (Hughes et al., 2014, 2015) mainly due to the observed poleward shift in the Atlantic catches which is most likely associated to climate changes effects. The Mediterranean region is possibly one of the most vulnerable regions to climate change (Rosenzweig et al., 2007; Giorgi and Lionello, 2008) and has been identified as one of the most prominent “Hot-Spots” in future climate change projections (Giorgi, 2006).
Our approach was to go deeper into the spatial dimension of the species distribution and determine environmental variables that define species spatial distribution and especially species' juvenile grounds. For this purpose we exploited info collected during the summer acoustic surveys carried out in the north part of the Mediterranean since 2000. We need to note that previous info on the spatial distribution of the juvenile grounds of the species was largely missing being available only on limited local scales such as the north-eastern part of the Adriatic Sea.
Bathymetry along with SST and circulation patterns expressed through SLA and UADT (zonal component of the absolute geostrophic velocity) were the variables found to determine species nurseries. Atlantic mackerel juveniles were found most likely to be present in the continental shelf at a depth <130 m combined with upwelling processes as well as in deeper waters up to 220 m when combined with downwelling processes. Areas where zonal velocity values were either < −0.1 m s−1 or > 0.2 m s−1 also appeared favorable. The association with upwelling and downwelling processes might be related to oceanographic regimes related to local retention processes of fish larvae and zooplankton and subsequently food availability. Moreover, Atlantic mackerel is a pelagic species known to undergo seasonal migrations (Reid et al., 2001; Molloy, 2004; Jacobsen, 2008) and the association found with the zonal component of the absolute geostrophic velocity is stressing the migratory nature of the species that uses currents to facilitate transport. The relation between habitat suitability and SST seems to be more complex. Results showed a bell shaped curve for SST and the selection of 22–26°C and the occurrence of optimum temperatures around 24.5°C. Although, our sampling lacks info on temperatures above 26°C, a temperature increase over this value is likely to be related to unfavorable habitat conditions. Our knowledge based on the Atlantic population, verifies spatial shifts in the species spawning habitat in relation to changes in SST within a 20 yrs period (Beare and Reid, 2002). Specifically, a poleward shift in the spawning habitat is recorded suggesting a selection for cooler waters (Hughes et al., 2014). The location of overwintering and the timing direction and speed of migration are also related to SST (e.g., Reid et al., 2001; Jansen and Gislason, 2011, 2013; Hughes et al., 2014).
Validation results of the final selected model based on areas from the western, central and eastern Mediterranean showed substantial to good model performance especially when it comes to assess habitats with high opportunity of occurrence. From West to East, suitable areas included the continental shelf waters of the Gulf of Lions, the Baleares, the Moroccan coastline, the coastal waters of the Ligurian Sea the area around the island of Sardinia, waters over the banks in the Strait of Sicily, the north eastern part of the Adriatic Sea and along Croatian coastline, the coastal waters of the south eastern Ionian Sea, the continental shelf of North Aegean Sea and the Nile Delta area. Persistent areas although highly localized and very limited in extent are confirmed by the scarce available information which indicates the presence of such nurseries along the coast of Bari in the Adriatic Sea (Bottari et al., 2004), in the coastal waters of the Gulf of Gabes (Ghailen et al., 2010) in Tunisia as well as in association to the Ebro river in Spanish Mediterranean waters (Sardà et al., 2006). Moreover, the presence of juvenile grounds is verified offshore the Tunisian waters by the OASIS acoustic surveys results (Hattour, 2000; Ben Abdallah and Gaamour, 2004; Hattour et al., 2004).
We need to single out that the assessed potential nursery grounds were generally marked as “occasional,” implying that although these areas present high probability to encounter Atlantic mackerel this picture can largely vary from year to year. This stresses the high susceptibility of the species to environmental conditions like temperature, circulation patterns and the intensity of currents and further underlines that climate change toward warmer temperatures can alter; possibly reduce the spread and suitable locations of Atlantic mackerel juvenile grounds. The recent example from the North-East Atlantic mackerel population and the poleward shift in its distribution (Hughes et al., 2014) come to support this. In comparison to other European regions the future annual warming rate is likely to be greatest in southern Europe (Hertig and Jacobeit, 2008). Specifically, climate change scenarios forecast temperature increase for the whole Mediterranean area in the period 2071–2100 compared to 1990–2019, ranging mostly between 2 and 4°C, depending on region and season (Giorgi and Lionello, 2008; Hertig and Jacobeit, 2008). Thus, climate change effect on Atlantic mackerel suitable habitat in the Mediterranean becomes more important. Given the very little information we have available from the Mediterranean, the highly variable nature of the habitat and the migratory behavior of the species it becomes appealing to further examine to what extent climate change along with water circulation can define migration routes or be a barrier for stock exchange. Genetic analysis might help toward this perspective.
The latter will also help to define appropriate stock assessment units as the species is currently not assessed in any Mediterranean country but the need for stock assessment has recently been noted (STECF, 2016). Although anchovy and sardine are the main target species for the small pelagic fishery in the Mediterranean, it is well-known that the Mediterranean small pelagic fishery is practically a mixed species fishery and single species management or protection cannot be as effective as ecosystem protection practices. Within this framework we have estimated and visualized the overlap between Atlantic mackerel and anchovy/ sardine juvenile grounds. Results showed that although the degree of overlapping was generally low, not exceeding 15% in general, this varied at a regional level going up to 30%. Although the Mediterranean Regulation has set minimum landing size for all three species, the degree at which the nurseries of all three species coincide with existing fishing grounds might have implications for the management of the fisheries operating in the Gulf of Lions, the Baleares, the North Aegean Sea, the South Adriatic, and over the Banks in the Strait of Sicily. Furthermore, spatial management measures such as fishing restricted areas aiming to protect nurseries should consider as more effective candidates areas serving as nurseries for more than one species. This becomes even more appealing and important for the maintenance of good stock status considering the vulnerability of small pelagics to environmental conditions and climate change. Moreover, some of the areas indicated here like the Gulf of Lions, the Baleares, waters around Sardinia, the North Aegean Sea and the Adventure Bank in the Strait of Sicily are also persistent nurseries for commercially important demersal species such as European hake (Merluccius merluccius), deep water rose shrimp (Parapenaeus longirostris), horned octopus (Eledone cirrhosa), and broadtail short fin squid (Illex coindetii; e.g. Fortibuoni et al., 2010; Garofalo et al., 2011; Colloca et al., 2015). Effective spatial management measures such as MPAs or MPA networks should not rely on the protection of one or two species or species targeted by a single fishery but rather on the protection of areas where the nurseries of the most important small pelagic and demersal resources coincide.
Author Contributions
MG, CS, and MP contributed to the conception or design of the work and conducted the analysis. MI, AB, JB, GB, AD, IL, AV, AM, VV, and LB contributed to the interpretation of data analysis and to drafting the work.
Conflict of Interest Statement
The authors declare that the research was conducted in the absence of any commercial or financial relationships that could be construed as a potential conflict of interest.
Acknowledgments
This study was partly supported by the Commission of the European Union through the MEDISEH project (MAREA PROJECT MEDISEH:Mediterranean Sensitive Habitats, Specific Contract No 2, SI2.600741) and the MEDIAS workshops of the DCF. We thank the captains and crews of the RV Philia, RV L'Europe, IEO RV Cornide de Saavedra, and RV Dallaporta as well as all the scientists for their assistance during the on board surveys.
References
Artegiani, A., Bregant, D., Paschini, E., Pinardi, N., Raicich, F., and Russo, A. (1997). The Adriatic Sea general circulation. Part I: air–sea interactions and water mass structure. J. Phys. Oceanogr. 27, 1492–1514. doi: 10.1175/1520-0485(1997)027<1492:TASGCP>2.0.CO;2
Basilone, G., Bonanno, A., Patti, B., Mazzola, S., Barra, M., Cuttitta, A., et al. (2013). Spawning site selection by European anchovy (Engraulis encrasicolus) in relation to oceanographic conditions in the Strait of Sicily. Fish. Oceanogr. 22, 309–323. doi: 10.1111/fog.12024
Basilone, G., Guisande, C., Patti, B., Mazzola, S., Cuttitta, A., Bonanno, A., et al. (2004). Linking habitat conditions and growth in the European anchovy (Engraulis encrasicolus). Fish. Res. 68, 9–19. doi: 10.1016/j.fishres.2004.02.012
Basilone, G., Guisande, C., Patti, B., Mazzola, S., Cuttitta, A., Bonanno, A., et al. (2006). Effect of habitat conditions on reproduction of the European anchovy (Engraulis encrasicolus) in the Strait of Sicily. Fish. Oceanogr. 15, 271–280. doi: 10.1111/j.1365-2419.2005.00391.x
Beare, D. J., and Reid, D. G. (2002). Investigating spatio-temporal change in spawning activity by Atlantic mackerel between 1977 and 1998 using generalized additive models. ICES J. Mar. Sci. 59, 711–724. doi: 10.1006/jmsc.2002.1207
Bellido, J. M., Brown, A. M., Valavanis, V. D., Giráldez, A., Pierce, G. J., Iglesias, M., et al. (2008). Identifying essential fish habitat for small pelagic species in Spanish Mediterranean waters. Hydrobiologia 612, 171–184. doi: 10.1007/s10750-008-9481-2
Bellido, J. M., Pierce, G. J., and Wang, J. (2001). Modelling intra-annual variation in abundance of squid Loligo forbesi in Scottish waters using generalised additive models. Fish. Res. 52, 23–39. doi: 10.1016/S0165-7836(01)00228-4
Bellier, E., and Planque, B. (2007). Historical fluctuations in spawning location of anchovy (Engraulis encrasicolus) and sardine (Sardina pilchardus) in the Bay of Biscay during 1967 – 73 and 2000 – 2004. Fish. Oceanogr. 16, 1–15. doi: 10.1111/j.1365-2419.2006.00410.x
Ben Abdallah, L., and Gaamour, A. (2004). Répartition Géographique et Estimation de la Biomasse des Petits Pélagiques des Ctes Tunisiennes. FAO, MedSudMed Technical Documents No.5. Mazara del Vallo, Italy.
Bonanno, A., Placenti, F., Basilone, G., Mifsud, R., Genovese, S., Patti, B., et al. (2014). Variability of water mass properties in the strait of sicily in summer period of 1998-2013. Ocean Sci. 10, 759–770. doi: 10.5194/os-10-759-2014
Bottari, T., Rinelli, P., Giordano, D., and Greco, S. (2004). Length-weight relationship and maturity of the Atlantic mackerel Scomber scombrus from the Adriatic Sea (Eastern Mediterranean). Cah. Biol. Mar. 45, 49–53.
Brander, K. (2010). Impacts of climate change on fisheries. J. Mar. Syst. 79, 389–402. doi: 10.1016/j.jmarsys.2008.12.015
Bruge, A., Alvarez, P., Fontán, A., Cotano, U., and Chust, G. (2016). Thermal niche tracking and future distribution of atlantic mackerel spawning in response to ocean warming. Front. Mar. Sci. 3:86. doi: 10.3389/fmars.2016.00086
Buckland, S. T., and Elston, D. A. (1993). Empirical models for the spatial distribution of wildlife. Appl. Ecol. 30, 478–495. doi: 10.2307/2404188
Champalbert, G. (1996). Characteristics of zooplankton standing stock and communities in the Western Mediterranean Sea: relations to hydrology. Sci. Mar. 60, 97–113.
Collette, B. B., and Nauen, C. E. (1983). FAO Species Catalogue, Vol. 2, Scombrids of the World. FAO Fish. Synopsis No. 125 2, 137. Available online at: http://www.fao.org/docrep/009/ac478e/ac478e00.htm
Colloca, F., Garofalo, G., Bitetto, I., Facchini, M. T., Grati, F., Martiradonna, A., et al. (2015). The seascape of demersal fish nursery areas in the North Mediterranean Sea, a First step towards the implementation of spatial planning for trawl fisheries. PLoS ONE 10:e0119590. doi: 10.1371/journal.pone.0119590
Cuttitta, A., Carini, V., Patti, B., Bonanno, A., Basilone, G., Mazzola, S., et al. (2003). Anchovy egg and larval distribution in relation to biological and physical oceanography in the Strait of Sicily. Hydrobiologia 503, 117–120. doi: 10.1023/B:HYDR.0000008473.96144.d3
European Commission (2006). Council Regulation (EC) No 1967/2006 Concerning Management Measures for the Sustainable Exploitation of Fishery Resources in the Mediterranean Sea, Amending Regulation (EEC) No 2847/93 and REPEALING REGULATION (EC) No 1626/94. European Commission, Brussels.
European Commission (2013). Regulation (EU) No 1380/2013 of the European Parliament and of the Council of 11 December 2013 on the Common Fisheries Policy, Amending Council Regulations (EC) No 1954/2003 and (EC) No 1224/2009 and repealing Council Regulations (EC) No 2371/2002 and (EC) No 639/2004 and Council Decision 2004/585/EC. European Commission, Brussels.
FAO (2016). Fisheries and Aquaculture Software. FishStatJ - Software for Fishery Statistical Time Series. Regional Workspace: GFCM (Mediterranean and Black Sea) Capture Production 1970-2014. Available online at: http://www.fao.org/fishery/statistics/software/fishstatj/en (Release date: April 2016).
Fielding, A. (1999). “How should accuracy be measured?,” in Machine Learning Methods for Ecological Applications, ed A. H. Fielding (Boston, MA: Springer), 209–223. doi: 10.1007/978-1-4615-5289-5_8
Fielding, A. H., and Bell, J. F. (1997). A review of methods for the assessment of prediction errors in conservation presence/absence models. Environ. Conserv. 24, 38–49. doi: 10.1017/S0376892997000088
Foote, K. G., Knudsen, H. P., Vestnes, G., MacLennan, D. N., and Simmonds, E. J. (1987). Calibration of acoustic instruments for fish density estimation: a practical guide. ICES Coop. Res. Rep. 1–69. Available online at: https://www.google.gr/url?sa=t&rct=j&q=&esrc=s&source=web&cd=1&ved=0ahUKEwi41M3X0YPVAhUEcBoKHfvpCeUQFgglMAA&url=http%3A%2F%2Fwww.ices.dk%2Fsites%2Fpub%2FPublication%2520Reports%2FCooperative%2520Research%2520Report%2520(CRR)%2Fcrr144%2Fcrr144.pdf&usg=AFQjCNHshtw9EkO-IUaODqx8EXZ7857OuQ&cad=rja
Fortibuoni, T., Aldighieri, F., Giovanardi, O., Pranovi, F., and Zucchetta, M. (2015). Climate impact on Italian fisheries (Mediterranean Sea). Reg. Environ. Change 15, 931–937. doi: 10.1007/s10113-015-0781-6
Fortibuoni, T., Bahri, T., Camilleri, M., Garofalo, G., Gristina, M., and Fiorentino, F. (2010). Nursery and spawning areas of deep-water rose shrimp, Parapenaeus longirostris (Decapoda: Penaeidae), in the strait of sicily (Central Mediterranean Sea). J. Crustacean Biol. 30, 167–114. doi: 10.1651/09-3167.1
Fortin, M. J., and Dale, M. R. T. (2005). Spatial Analysis: A Guide for Ecologists. New York, NY: Cambridge University Press.
Franklin, J. (2009). Mapping Species Distributions. Spatial Inference and Prediction. New York, NY: Cambridge University Press.
Froese, R., and Pauly, D. (2016). FishBase World Wide Web Electronic Publication. Available online at: www.fishbase.org, version 06/2016.
Gamito, R., Teixeira, C. M., Costa, M. J., and Cabral, H. N. (2015). Are regional fisheries' catches changing with climate? Fish. Res. 161, 207–216. doi: 10.1016/j.fishres.2014.07.014
Gamulin, T., and Hure, J. (1983). The spawning and spawning areas of pelagic fishes (Sardina pilchardus, Engraulis engrasicolus, Scomber scombrus, Sardinella aurita and Sprattus sprattus sprattus) in the Adriatic Sea. Acta Adriat. 24, 97–131.
Garofalo, G., Fortibuoni, T., Gristina, M., Sinopoli, M., and Fiorentino, F. (2011). Persistence and co-occurrence of demersal nurseries in the Strait of Sicily (central Mediterranean): implications for fishery management. J. Sea Res. 66, 29–38. doi: 10.1016/j.seares.2011.04.008
Ghailen, H., Abdallah, H., Hassan, A., Mourad, C., Abderrahmen, B., and Othman, J. (2010). Length-weight relationships for 13 fish species from the Gulf of Gabes (Southern Tunisia, Central Mediterranean). Afr. J. Biotechnol. 9, 6177–6181. doi: 10.5897/AJB10.603
Giannoulaki, M., Iglesias, M., Tugores, M. P., Bonanno, A., Patti, B., De Felice, A., et al. (2013). Characterizing the potential habitat of European anchovy Engraulis encrasicolus in the Mediterranean Sea, at different life stages. Fish. Oceanogr. 22, 69–89. doi: 10.1111/fog.12005
Giannoulaki, M., Pyrounaki, M. M., Liorzou, B., Leonori, I., Valavanis, V. D., Tsagarakis, K., et al. (2011). Habitat suitability modelling for sardine juveniles (Sardina pilchardus) in the Mediterranean Sea. Fish. Oceanogr. 20, 367–382. doi: 10.1111/j.1365-2419.2011.00590.x
Giorgi, F., and Lionello, P. (2008). Climate change projections for the Mediterranean region. Glob. Planet. Change 63, 90–104. doi: 10.1016/j.gloplacha.2007.09.005
Guisan, A., and Zimmermann, N. (2000). Predictive habitat distribution models in ecology. Ecol. Modell. 135, 147–186. doi: 10.1016/S0304-3800(00)00354-9
Hanley, J., and McNeil, B. (1982). The meaning and use of the area under a receiver operating characteristic (ROC) curve. Radiology 143, 29–36. doi: 10.1148/radiology.143.1.7063747
Hattour, A. (2000). Contribution a l'etude des Poissons Pelagiques des eaux Tunisiennes. dissertation. Université de Tunis II, Tunis.
Hattour, A., Ben Abdallah, L., and Guennegan, Y. (2004). Abondance relative et estimation de la biomasse des petits pélagiques des eaux tunisiennes. Bull. Inst. Natl. Sci. Technol. Mer Salammbô 31, 5–16.
Hertig, E., and Jacobeit, J. (2008). Downscaling future climate change: temperature scenarios for the Mediterranean area. Glob. Planet. Change 63, 127–131. doi: 10.1016/j.gloplacha.2007.09.003
Hughes, K. M., Dransfeld, L., and Johnson, M. P. (2014). Changes in the spatial distribution of spawning activity by north-east Atlantic mackerel in warming seas: 1977–2010. Mar. Biol. 161, 2563–2576. doi: 10.1007/s00227-014-2528-1
Hughes, K. M., Dransfeld, L., and Johnson, M. P. (2015). Climate and stock influences on the spread and locations of catches in the northeast Atlantic mackerel fishery. Fish. Oceanogr. 24, 540–552. doi: 10.1111/fog.12128
Jacobsen, J. A. (2008). Wide Distribution of Mackerel. Working document to ICES Working Group on Widely Distributed Stocks in September 2008. ICES Headquarters Copenhagen, 691.
Jansen, T., and Gislason, H. (2011). Temperature affects the timing of spawning and migration of North Sea mackerel. Cont. Shelf Res. 31, 64–72. doi: 10.1016/j.csr.2010.11.003
Jansen, T., and Gislason, H. (2013). Population structure of Atlantic Mackerel (Scomber scombrus). PLoS ONE 8:e64744. doi: 10.1371/journal.pone.0064744
Jiménez-Valverde, A., Lobo, J. M., and Hortal, J. (2008). Not as good as they seem: the importance of concepts in species distribution modelling. Divers. Distrib. 14, 885–890. doi: 10.1111/j.1472-4642.2008.00496.x
Landis, J. R., and Koch, G. G. (1977). The measurement of observer agreement for categorical data. Biometrics 33, 159–174. doi: 10.2307/2529310
Larnicol, G., Ayoub, N., and Le Traon, P. (2002). Major changes in Mediterranean Sea level variability from 7 years of TOPEX/Poseidon and ERS-1/2 data. J. Mar. Syst. 33–34, 63–89. doi: 10.1016/S0924-7963(02)00053-2
Lloret, J., Lleonart, J., Solé, I., and Fromentin, J. M. (2001). Fluctuations of landings and environmental conditions in the north-western Mediterranean sea. Fish. Oceanogr. 10, 33–50. doi: 10.1046/j.1365-2419.2001.00151.x
Lobo, J. M., Jiménez-valverde, A., and Real, R. (2008). AUC: a misleading measure of the performance of predictive distribution models. Glob. Ecol. Biogeogr. 17, 145–151. doi: 10.1111/j.1466-8238.2007.00358.x
Manel, S., Williams, H. C., and Ormerod, S. J. (2001). Evaluating presence absence models in ecology; the need to count for prevalence. J. Appl. Ecol. 38, 921–931. doi: 10.1046/j.1365-2664.2001.00647.x
Marra, G., and Wood, S. N. (2011). Practical variable selection for generalized additive models. Comput. Stat. Data Anal. 55, 2372–2387. doi: 10.1016/j.csda.2011.02.004
Meneghesso, C., Riginella, E., La Mesa, M., Donato, F., and Mazzoldi, C. (2013). Life-history traits and population decline of the Atlantic mackerel Scomber scombrus in the Adriatic Sea. J. Fish Biol. 83, 1249–1267. doi: 10.1111/jfb.12223
Millot, C. (1990). The Gulf of Lions' hydrodynamics. Cont. Shelf Res. 10, 885–894. doi: 10.1016/0278-4343(90)90065-T
Molloy, J. (2004). The Irish Mackerel Fishery and the Making of an Industry. Galway: Killybegs Fishermen's Organisation.
Papetti, C., Di Franco, A., Zane, L., Guidetti, P., De Simone V, Spizzotin, M., et al. (2013). Single population and common natal origin for Adriatic Scomber scombrus stocks: evidence from an integrated approach. ICES J. Mar. Sci. 70, 387–398. doi: 10.1093/icesjms/fss201
Patti, B., Guisande, C., Bonanno, A., Basilone, G., Cuttitta, A., and Mazzola, S. (2010). Role of physical forcings and nutrient availability on the control of satellite-based chlorophyll a concentration in the coastal upwelling area of the Sicilian Channel. Sci. Mar. 74, 577–588. doi: 10.3989/scimar.2010.74n3577
Pecl, G. T., Ward, T. M., Doubleday, Z. A., Clarke, S., Day, J., Dixon, C., et al. (2014). Rapid assessment of fisheries species sensitivity to climate change. Clim. Change 127, 505–520. doi: 10.1007/s10584-014-1284-z
Planque, B., Bellier, E., and Lazure, P. (2007). Modelling potential spawning habitat of sardine (Sardina pilchardus) and anchovy (Engraulis encrasicolus) in the Bay of Biscay. Fish. Oceanogr. 16, 16–30. doi: 10.1111/j.1365-2419.2006.00411.x
Pujol, M. I., and Larnicol, G. (2005). Mediterranean sea eddy kinetic energy variability from 11 years of altimetric data. J. Mar. Syst. 58, 121–142. doi: 10.1016/j.jmarsys.2005.07.005
R Development Core Team (2012). R: A Language and Environment for Statistical Computing. Vienna: R Foundation for Statistical Computing. Available online at: http://www.R-project.org
Reid, D. G., Turrell, W. R., Walsh, M., and Corten, A. (1997). Cross-shelf processes north of Scotland in relation to the southerly migration of Western mackerel. ICES J. Mar. Sci. 54, 168–178. doi: 10.1006/jmsc.1996.0202
Reid, D. G., Walsh, M., and Turrell, W. R. (2001). Hydrography and mackerel distribution on the shelf edge west of the Norwegian deeps. Fish. Res. 50, 141–150. doi: 10.1016/S0165-7836(00)00247-2
Rosenzweig, C., Casassa, G., Karoly, D. J., Imeson, A., Liu, C., Menzel, A., et al. (2007). “Assessment of observed changes and responses in natural and managed systems,” in Climate Change 2007: Impacts, Adaptation and Vulnerability. Contribution of Working Group II to the Fourth Assessment Report of the Intergovernmental Panel on Climate Change, eds M. L. Parry, O. F. Canziani, J. P. Palutikof, P. J. van der Linden, and C. E. Hanson (Cambridge, UK: Cambridge University Press), 79–131.
Sardà, F., Bahamon, N., Molí, B., and Sardà-Palomera, F. (2006). The use of a square mesh codend and sorting grids to reduce catches of young fish and improve sustainability in a multispecies bottom trawl fishery in the. Sci. Mar. 70, 347–353. doi: 10.3989/scimar.2006.70n3347
Simmonds, J., and MacLennan, D. (2005). Fisheries Acoustics: Theory and Practice, 2nd Edn. Oxford: Blackwell Science.
Sinovčić, G. (2001). “Small pelagic fish from the Croatian fishing grounds,” in Priority Topics Related to Small Pelagic Fishery Resources of the Adriatic Sea, eds P. Mannini, F. Massa, and N. Milone (Croatia: Split), 53–58. FAO-MiPAF Scientific Cooperation to Support Responsible Fisheries in the Adriatic Sea. GCP/RER/010/ITA/TD-03. AdriaMed Technical Documents, 3.
Somarakis, S., and Nikolioudakis, N. (2007). Oceanographic habitat, growth and mortality of larval anchovy (Engraulis encrasicolus) in the northern Aegean Sea (eastern Mediterranean). Mar. Biol. 152, 1143–1158. doi: 10.1007/s00227-007-0761-6
STECF (2016). Scientific, Technical and Economic Committee for Fisheries (STECF) – Methodology for the Stock Assessments in the Mediterranean Sea (STECF-16-05). Luxembourg.
Tsikliras, A. C., Antonopoulou, E., and Stergiou, K. I. (2010). Spawning period of Mediterranean marine fishes. Rev. Fish Biol. Fish. 20, 499–538. doi: 10.1007/s11160-010-9158-6
Tugores, P., Giannoulaki, M., Iglesias, M., Bonanno, A., Tičina, V., Leonori, I., et al. (2011). Habitat suitability modelling for sardine Sardina pilchardus in a highly diverse ecosystem: the Mediterranean Sea. Mar. Ecol. Prog. Ser. 443, 181–205. doi: 10.3354/meps09366
Valavanis, V. D., Pierce, G. J., Zuur, A. F., Palialexis, A., Saveliev, A., Katara, I., et al. (2008). Modelling of essential fish habitat based on remote sensing, spatial analysis and GIS. Hydrobiologia 612, 5–20. doi: 10.1007/s10750-008-9493-y
Villamor, B., Abaunza, P., Lucio, P., and Porteiro, C. (1997). Distribution and age structure of mackerel (Scomber scombrus L.) and horse mackerel (Trachurus trachurus L.) in the northern coast of Spain, 1989-1994. Sci. Mar. 61, 345–366.
Keywords: habitat suitability modeling, Atlantic mackerel, nursery grounds, Mediterranean Sea, generalized additive models, acoustic surveys
Citation: Giannoulaki M, Pyrounaki MM, Bourdeix J-H, Ben Abdallah L, Bonanno A, Basilone G, Iglesias M, Ventero A, De Felice A, Leonori I, Valavanis VD, Machias A and Saraux C (2017) Habitat Suitability Modeling to Identify the Potential Nursery Grounds of the Atlantic Mackerel and Its Relation to Oceanographic Conditions in the Mediterranean Sea. Front. Mar. Sci. 4:230. doi: 10.3389/fmars.2017.00230
Received: 11 October 2016; Accepted: 07 July 2017;
Published: 26 July 2017.
Edited by:
Çetin Keskin, Istanbul University, TurkeyReviewed by:
Tomaso Fortibuoni, National Institute of Oceanography and Experimental Geophysics, ItalyPeriyadan K. Krishnakumar, King Fahd University of Petroleum and Minerals, Saudi Arabia
Copyright © 2017 Giannoulaki, Pyrounaki, Bourdeix, Ben Abdallah, Bonanno, Basilone, Iglesias, Ventero, De Felice, Leonori, Valavanis, Machias and Saraux. This is an open-access article distributed under the terms of the Creative Commons Attribution License (CC BY). The use, distribution or reproduction in other forums is permitted, provided the original author(s) or licensor are credited and that the original publication in this journal is cited, in accordance with accepted academic practice. No use, distribution or reproduction is permitted which does not comply with these terms.
*Correspondence: Marianna Giannoulaki, marianna@hcmr.gr