- 1NOAA Fisheries, Office of Protected Resources, Silver Spring, MD, United States
- 2Smultea Environmental Sciences, Washington Grove, MD, United States
- 3ECS Federal, LLC, Fairfax, VA, United States
- 4NOAA Fisheries, Office of Science and Technology, Silver Spring, MD, United States
- 5Marine Mammal Commission, Bethesda, MD, United States
- 6NOAA Fisheries, Pacific Islands Fisheries Science Center, Seattle, WA, United States
- 7Woods Hole Oceanographic Institution, Woods Hole, MA, United States
- 8Southwest Fisheries Science Center, National Marine Fisheries Service, National Oceanic and Atmospheric Administration, Moss Landing, CA, United States
- 9NOAA Fisheries, Marine Mammal Laboratory, Alaska Fisheries Science Center, Seattle, WA, United States
- 10Ocean Associates, Inc., Arlington, VA, United States
- 11Ocean Sciences Department, University of California, Santa Cruz, Santa Cruz, CA, United States
- 12British Antarctic Survey, Natural Environment Research Council, Cambridge, United Kingdom
- 13Moss Landing Marine Laboratories, Moss Landing, CA, United States
- 14NOAA Fisheries, Northeast Fisheries Science Center, Woods Hole, MA, United States
- 15CSIRO Marine and Atmospheric Research, Hobart, TAS, Australia
- 16Centre for Marine Socioecology, University of Tasmania, Hobart, TAS, Australia
- 17Institute of Marine Research, Bergen, Norway
- 18Applied Physics Laboratory, University of Washington, Seattle, WA, United States
- 19Southwest Fisheries Science Center, National Marine Fisheries Service, National Oceanic and Atmospheric Administration, Santa Cruz, CA, United States
- 20Alaska Department of Fish and Game, Fairbanks, AK, United States
- 21Department of Applied Mathematics and Statistics, University of California, Santa Cruz, Santa Cruz, CA, United States
- 22NOAA Fisheries, Alaska Fisheries Science Center, Seattle, WA, United States
- 23Geophysical Fluid Dynamics Laboratory, NOAA Oceanic and Atmospheric Research, Princeton, NJ, United States
- 24Farallon Institute, Petaluma, CA, United States
- 25Monterey Bay Aquarium, Monterey, CA, United States
- 26Duke University, Nicholas School of the Environment, Durham, NC, United States
- 27NOAA Fisheries, Northwest Fisheries Science Center, Seattle, WA, United States
Climate-related shifts in marine mammal range and distribution have been observed in some populations; however, the nature and magnitude of future responses are uncertain in novel environments projected under climate change. This poses a challenge for agencies charged with management and conservation of these species. Specialized diets, restricted ranges, or reliance on specific substrates or sites (e.g., for pupping) make many marine mammal populations particularly vulnerable to climate change. High-latitude, predominantly ice-obligate, species have experienced some of the largest changes in habitat and distribution and these are expected to continue. Efforts to predict and project marine mammal distributions to date have emphasized data-driven statistical habitat models. These have proven successful for short time-scale (e.g., seasonal) management activities, but confidence that such relationships will hold for multi-decade projections and novel environments is limited. Recent advances in mechanistic modeling of marine mammals (i.e., models that rely on robust physiological and ecological principles expected to hold under climate change) may address this limitation. The success of such approaches rests on continued advances in marine mammal ecology, behavior, and physiology together with improved regional climate projections. The broad scope of this challenge suggests initial priorities be placed on vulnerable species or populations (those already experiencing declines or projected to undergo ecological shifts resulting from climate changes that are consistent across climate projections) and species or populations for which ample data already exist (with the hope that these may inform climate change sensitivities in less well observed species or populations elsewhere). The sustained monitoring networks, novel observations, and modeling advances required to more confidently project marine mammal distributions in a changing climate will ultimately benefit management decisions across time-scales, further promoting the resilience of marine mammal populations.
Introduction
Marine mammals are exposed to a variety of threats and habitat perturbations from human activities (e.g., by-catch incidental to commercial fisheries, industrial noise, ship-strike) on regional and global scales. Human activities can lead to changes in marine mammal distribution, such as extirpation due to whaling or hunting [e.g., southern right whales (Eubalaena australis) in New Zealand Patenaude et al., 1998; Carroll et al., 2014] and temporary abandonment of portions of a range [e.g., gray whales (Eschrichtius robustus) in Laguna Guerrero Negro, Baja California, Mexico Bryant et al., 1984]. Alteration of oceanographic conditions and processes due to anthropogenic global climate change are expected to profoundly influence ecosystems (i.e., ocean warming, acidification, and deoxygenation) (Burrows et al., 2011; IPCC, 2014) and, in turn, marine mammal distributions in the foreseeable future (Learmonth et al., 2006; Schumann et al., 2013; Laidre et al., 2015). While the full nature and scope of climate-driven impacts on marine mammals are unclear, changes in population ranges and regional abundance are expected (Learmonth et al., 2006).
Shifting ranges of various marine species have been observed across all ocean regions (Poloczanska et al., 2016). Integration of long time series and modeling studies have demonstrated climate change-associated distributional changes in numerous marine fish and invertebrate populations (e.g., Perry et al., 2005; Nye et al., 2009; Pinsky et al., 2013; Poloczanska et al., 2013; Walsh et al., 2015), including commercially important North Atlantic Ocean species in multi-billion dollar fishing industries (NMFS, 2016). Additional shifts in these taxa, as well as the potential for accelerated rates of change, are expected as warming continues (Hazen et al., 2013; Lynch et al., 2015; Hare et al., 2016).
Shifts have included important prey items for marine mammals. Rising temperatures in the Gulf of Maine, for example, have prompted Calanus finmarchicus, a major food source for herring, mackerel, and North Atlantic right whales (Eubalaena glacialis), to prematurely exit winter dormancy, denying key predators a lipid rich food source in spring and summer (Runge et al., 2015). Such changes in the quality, timing, and abundance of key zooplankton and forage fish species may confound efficient transfer of marine primary and secondary production to higher trophic levels with corresponding cascading implications for food webs (Peterson, 2009; Lauria et al., 2012; Sydeman et al., 2015), including those involving marine mammals (Trathan et al., 2007; Moore and Huntington, 2008).
Range shifts associated with climate change have been observed in some marine mammal populations (e.g., Kovacs et al., 2011; Clarke et al., 2013; Hamilton et al., 2015). Substantive shifts in the distribution of marine mammals and other large marine vertebrates have been predicted (e.g., IWC, 2010; Gilles et al., 2011; Becker et al., 2012; Hezel et al., 2012; Keller et al., 2012; Gregr et al., 2013; Hazen et al., 2013; Mannocci et al., 2014). As such shifts occur in novel environments expected under climate change, protective measures for marine mammals will need to be adapted. However, capabilities available to managers to anticipate and react to such changes are currently limited.
Marine Mammal Management–Authorities and Mandates
Conservation of marine mammals and their habitats is generally the responsibility of federal, state, and provincial governments, in some cases working with co-management partners. Anthropogenic threats to marine mammal populations are largely managed in the United States through policies and conservation activities developed under the Marine Mammal Protection Act (MMPA) and Endangered Species Act (ESA) and in other nations through similar statutes (e.g., Canada's Species at Risk Act; Australia's Environment Protection and Biodiversity Conservation Act). Under these statutes, agencies are required to develop programs to assess and permit various ocean-use activities (e.g., oil and gas development and production, renewable energy facility siting, and development), minimize threats to marine mammal habitat, engage in endangered species recovery planning, and assess global climate change as it impacts the ecology and health of marine mammal populations. In addition, marine mammals can be large consumers of commercially important fish species, and any shifts in their distribution may therefore be of concern to fisheries management programs that also will need to adapt to such changes. Moreover, marine mammals are integral parts of their ecosystems—as both predators and prey—and ecosystem-based fishery management will need to take into account any changes in marine mammal distribution, abundance and predator-prey relationships. Agencies involved in marine mammal management and conservation, as well as those engaged in managing subsistence harvests, will increasingly need means to anticipate shifts in marine mammal distribution and abundance resulting from climate change (e.g., Laidre et al., 2015).
While most agencies have broad authority to reduce anthropogenic threats (e.g., those arising from commercial, industrial, or military activities), agencies charged with management do not currently have the ability to regulate greenhouse gases, the primary driver of global climate change (IPCC, 2014). Thus, limited resources, regulatory inertia, and lack of public consensus constrain many agencies' abilities to react with appropriate protective measures to climate-driven changes in marine mammal distribution. In addition, as centers of abundance or areas of aggregation shift when existing habitats undergo change, existing threat-reduction measures may become obsolete or require modification as marine mammal distributions shift. For example, the location of a renewable energy or other industrial facility may be selected based on an assessment that its effects on marine mammal populations are anticipated to be relatively low. However, if the composition and regional abundance of those populations change, the facility may have greater overall impact than originally expected, and permitting of the facility may need to be re-assessed with a substantial investment of time and resources and lost opportunities to protect key species. Marine Protected Areas (including for marine mammals; Hoyt, 2011) and critical habitats are also established based on the existing occurrence of marine taxa; but these areas would have little conservation value if key species undergo shifts in distribution. An ability to track and anticipate such changes would aid in the protection of those species.
In rapidly changing ecosystems, including those in high-latitudes (Forcada et al., 2006; Laidre et al., 2008, 2015; Ragen et al., 2008), or in instances where populations are already in decline (e.g., Shelden et al., 2015), managers face a heightened need to respond with appropriate protective measures. Near- and long-term conservation planning would be enhanced by increased capacity to anticipate marine mammal response to changes in habitats brought about by climate change. Long-term planning may be particularly important given the long lifespans, long maturation periods, and low recruitment rates of many marine mammal species and will be important for those with complex social structures.
Conservation and planning actions occur on various spatial scales and temporal planning horizons (Figure 1), motivating marine mammal distribution and abundance predictions across a similar range of space and time-scales. These may include nowcasts and short-term (<1 week) forecasts (Hazen et al., 2016), to seasonal predictions up to 6 months in the future, to multi-year forecasts, increasing to decadal or century-scale projections. In the United States for example, endangered species recovery plans are updated on 5-year cycles, while permitting for industrial activities, such as the siting or construction of offshore renewable energy facilities and oil and gas exploration and development activities, tends to be on the order of decades (BOEM, 2016; Figure 1). On even longer time scales, designation of endangered status often necessitates evaluation of species persistence on the order of a century (e.g., Angliss et al., 2002). Conversely, measures developed to reduce the risk of whales being struck by ships (e.g., Silber et al., 2012) or limit marine mammal exposure to military operations (e.g., NOAA, 2015) may be established in finite areas and be implemented only for seasons to a few years (NOAA, 2013). While the emphasis here is meeting the challenge of projecting marine mammal distributions in a changing climate on multi-decadal to century time-scales, we note that decisions across multiple time-scales can contribute to the long-term resilience of marine mammal populations. We will thus discuss and contrast approaches across time-scales.
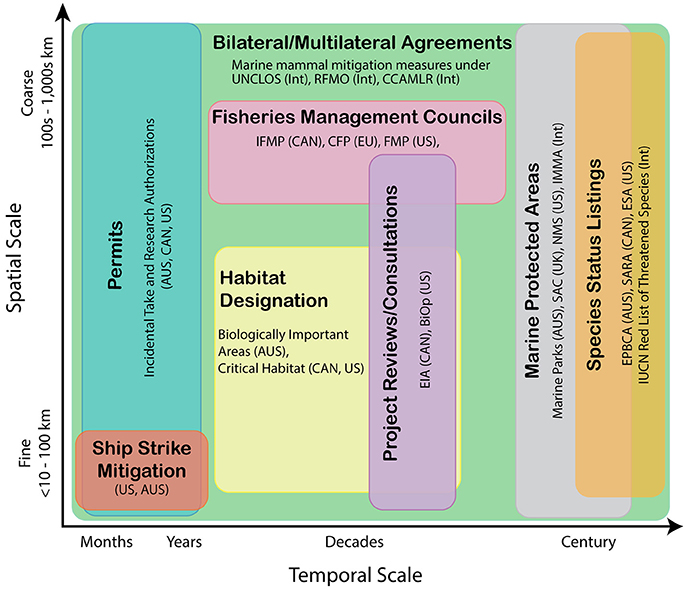
Figure 1. Various examples of national and international management activities mapped conceptually along generalized temporal and spatial (both granularity and extent) scales. Management and research objectives on appropriate spatial/temporal scales will dictate model type selection and data requirements. AUS, Australia; BiOp, Biological Opinions; CFP, Common Fisheries Policy; CCAMLR, Commission for the Conservation of Antarctic Marine Living Resources; ESA, Endangered Species Act; EIA, Environmental Impact Assessments; EPBCA, Environment Protection and Biodiversity Conservation Act; EU, European Union; FMP, Fishery Management Plans; IFMP, Integrated Fisheries Management Plans; IMMA, Important Marine Mammal Areas; Int, International; IUCN, International Union for Conservation of Nature; NMS, National Marine Sanctuaries; RFMO, Regional Fishery Management Organizations; SAC, Special Areas of Conservation; SARA, Species at Risk Act; UK, United Kingdom; UNCLOS, United Nations Convention of the Law of the Sea.
Marine Mammal Ecology and Climate Change
Marine mammals have unique ecologies with complex life cycles that make predicting responses to climate change more difficult and, in some cases, make the species especially vulnerable to climate change impacts. Perhaps most notable amongst these traits in the context of climate change is endothermy, which provides for a broader range of temperature tolerance in marine mammals relative to fish. This could be a mechanism that adds resilience in marine mammal populations, but also makes for less predictable responses relative to fish or invertebrates that show isotherm-following behaviors (Pinsky et al., 2013).
Endothermy also elevates the importance of predicting changes in food resources relative to warming signals. While progress has been made in projecting large-scale prey resource changes (e.g., Stock et al., 2014; Lefort et al., 2015), marine mammal species such as blue whales (Balaenoptera musculus) show tight coupling to smaller scale oceanographic features (Fiedler et al., 1998; Moore et al., 2002; Croll et al., 2005) associated with high euphausiid (krill) abundance (Santora et al., 2011). Similar relations have been exhibited by bowhead whales (Balaena mysticetus) across the Arctic (Laidre et al., 2007; Citta et al., 2015; George et al., 2015) and North Atlantic right whales (Baumgartner et al., 2003; Baumgartner and Mate, 2005). Such aggregations remain poorly resolved in even high-resolution models and may exhibit climate change responses that differ from large-scale changes.
Other common traits are long lifespan, low birth rate, and long generation time. These traits are not conducive to rapid evolutionary adaptation (Trathan et al., 2007), making behavioral flexibility critical for adaptation to changing climatologies. Fortunately, some marine mammals have exhibited substantial behavioral and prey selection flexibility (Palacios et al., 2013). For example, humpback whales (Megaptera novaeangliae) remained abundant and were suspected to adapt foraging behavior to new prey species when climate conditions were unfavorable to common target prey species (Benson et al., 2002; Fleming et al., 2016). Failure to account for such flexibility could lead to unrealistically pessimistic projections.
Alternatively, many marine mammal species exhibit strong dependence on habitat types or oceanographic features that may be highly vulnerable to climate change, or high fidelity to particular sites or migration routes. For example, ringed seals (Pusa hispida) rely on sea ice habitat for pupping and sufficient snow to hide young from predators (Smith and Stirling, 1975; Laidre et al., 2008; Kovacs et al., 2011; NOAA, 2012). Hawaiian monk seals (Neomonachus schauinslandi) may lose haul-out sites to sea level rise; thus, understanding the climate-related threats to this species might require a different type of model (e.g., dynamic shoreline evolution) for forecasting its distribution (Baker et al., 2006). Failure to account for unique vulnerabilities arising from such life-cycle strategies may result in unrealistically optimistic projections.
Lastly, some marine mammals are near the top of marine food webs, introducing a potentially strong dependence on integrated food web effects (Doney et al., 2012; Sydeman et al., 2015). Recent studies suggest robust amplification of productivity changes at higher trophic levels (Chust et al., 2014; Stock et al., 2014, 2017; Lefort et al., 2015). This potential sensitivity is further heightened by mid-trophic level fishing (Forcada et al., 2012), motivating work to analyze marine mammal responses in a food web context.
Current Approaches for Predicting and Projecting Marine Mammal Occurrence and Distribution
Two components are essential for predicting marine mammal occurrence and distribution. The first is a model capable of estimating marine mammal distribution and/or abundance given a set of physical and/or ecological variables (e.g., ocean temperature, food resources). The second is the capability to project the states of the relevant physical and ecological variables at the time and space scales of interest. Models are designed to address a variety of research questions (Guisan and Thuiller, 2005), and a range of model types have been used for the first component (Gregr et al., 2013; Palacios et al., 2013). Early efforts employed simple visual mapping to describe marine mammal seasonal occurrence. For example, Winn et al. (1986) used North Atlantic right whale sighting records to develop a simple conceptual model of the species' seasonal distribution.
Statistical habitat models use environmental data and species occurrence data to generate predictions of the distribution of the species. The environmental predictors chosen for these models usually pertain to biological mechanisms (e.g., temperature is an important physiological constraint; chlorophyll-a has often proved to be a meaningful proxy for ocean productivity and food resources), which helps to avoid spurious correlations. For example, Baumgartner and Mate (2005) used water depth, depth gradient, bottom hydrographic properties, sea surface temperature (SST), chlorophyll concentration, and other features to characterize North Atlantic right whale habitat. Becker et al. (2016) developed predictive habitat-based models of cetacean density and distribution for a number of species in the California Current System using a variety of dynamic environmental variables, including temperature, salinity, sea surface height, and mixed layer depth. Models used to predict distribution patterns, given observed environmental variables, may also be used to project a species' distribution using expected future values of the environmental variables (Becker et al., 2012).
In Panels 1 and 2, we highlight two examples of such statistical models in detail. Case study 1 describes “nowcasts” (i.e., estimates of the current ocean state) and seasonal predictions for 11 marine mammal species in the California Current based on habitat-based density models (Becker et al., 2016). Case study 2 applies similar empirically-driven approaches to assess potential challenges for ringed seal pup survival related to sea-ice and snowpack changes projected over the next century due to global warming (Hezel et al., 2012).
There are several limitations associated with statistical correlative models when used in a predictive context (Elith and Graham, 2009; Monahan, 2009; Webber et al., 2011). First, the relationships inferred from field data may not adequately describe the critical factors determining species distributions. Existing data on species distributions reflect the realized rather than potential habitat (i.e., the area where the intrinsic rate of population growth is >0; Araujo and Guisan, 2006; Soberon and Nakamura, 2009). This realized habitat implicitly reflects interactions with predators, prey species, and barriers to movement and comprises a level of structural detail not generally included in sets of climate predictors or even present in climate change projections. Predictions and projections based on realized niches thus presume that the impacts of these implicit factors act to maintain present realized habitat boundaries.
A second limitation is that, although the mechanisms by which environmental conditions affect biology and species distributions are considered, these relationships are empirically-defined from available data on past conditions and may not represent future conditions. Stationarity of these relationships is assumed when such models are used in a predictive context. This is clearly reasonable when predicting for conditions that have analogs in the historical record, but becomes less reliable for responses to extreme events or for the novel conditions expected under climate change (Williams et al., 2007; Hothorn et al., 2011).
Relatively recent advances in observational data collection and access to large marine environmental databases (e.g., the National Oceanographic Data Center; NCEI, 2017 or the Southwest Fisheries Science Center's ERDDAP site, SWFSC, 2017) provide an improved foundation for statistical habitat models, but would not address structural uncertainties in models that arise from incomplete understanding of species interactions and physiological thresholds. This level of knowledge would instead require a shift from reliance on correlations between marine mammals and their environment toward models that more clearly establish functional relationships with the physical and biological underpinnings of habitat utilization (Gregr et al., 2013; Cribb et al., 2015). These relationships enable development of process-based models that yield robust predictions of species distributions rooted in ecological understanding (Palacios et al., 2013). While such models have been traditionally developed for a broad range of zooplankton and higher trophic level species, including forage fish (e.g., Ito et al., 2015; Rose et al., 2015), migratory predators (e.g., Lehodey et al., 2008) and other commercially and ecologically important species (e.g., Cury et al., 2008; Fiechter et al., 2015), marine mammal case studies are also starting to appear. For example, California sea lion (Zalophus californianus) foraging patterns and feeding success were simulated using sub-models for biogeochemical processes, regional ocean circulation, and forage fish abundance (Fiechter et al., 2016). The parameterization of more mechanistic models is challenging, but will also benefit from data collection advances referenced above. In data-poor situations, mechanistic models informed by general principles can help define the scope of potential marine mammal responses by testing the implications of general principles drawn from theory or data-rich regions (Friedrichs et al., 2007). While such exercises inevitably lead to large uncertainty bounds, they can help prioritize future observations on quantities or processes within existing uncertainty bounds (Plaganyi et al., 2011).
Predictions and projections for future states of drivers of marine resource abundance and distribution are generally derived from global climate and earth system models (Stock et al., 2011; Hobday et al., 2016; Tommasi et al., 2017a). Several characteristics of global climate and earth system models have shaped their application in projecting marine mammal distribution and abundance under climate change (Stock et al., 2011). The coarse resolution of global climate models (often 1–2°, or 100–200 km ocean grids in past assessments of the Intergovernmental Panel on Climate Change, IPCC) can generate significant coastal biases (Scales et al., 2017). Large inter-model differences in regional climate change projections limit confidence in scenarios for the future states of drivers of marine resource distribution and abundance (Hawkins and Sutton, 2009; Frölicher et al., 2016). Limited resolution of marine food webs in most earth system models may under-estimate the potential amplification of climate change effects for higher trophic levels, particularly at regional scales (Chust et al., 2014; Stock et al., 2014, 2017; Lefort et al., 2015). As a result of these limitations, confidence in predicting the magnitude and direction of climate change trends that are capable of altering marine resource distributions is generally greatest at ocean-basin spatial scales and multi-decadal to century time-scales. In the absence of refined resolution via downscaling or high-resolution global models (see below), regional inferences must focus on the magnitude and direction of changes due to large-scale greenhouse gas accumulation, and include the caveat that unresolved regional responses may significantly modify these trends (e.g., Hare et al., 2012; Lynch et al., 2015).
Global climate model configurations similar to those used for long-term climate change projections are also applied to short-term predictions on seasonal to multi-annual scales that have proven valuable for a range of marine resource applications (Hobday et al., 2016; Tommasi et al., 2017a). Analysis to date has suggested that skillful seasonal and, in some cases, multi-annual sea surface temperature predictions are possible for many regions despite coarse model resolution (Stock et al., 2015; Tommasi et al., 2017b). Seasonal to multi-annual predictions of ocean productivity, oxygen and other potential factors important to marine resource distributions and abundance have provided some cause for optimism (Séférian et al., 2014; Gehlen et al., 2015), but have not been assessed with the same rigor as ocean temperatures, in part due to the sparsity of data with which to robustly characterize the model skill. The use of short-term forecasts in dynamic management approaches (Maxwell et al., 2015; Dunn et al., 2016; Tommasi et al., 2017b) may also contribute to building long-term resilience (Hobday et al., 2016; Tommasi et al., 2017a).
Improving Marine Mammal Distribution Projections
Uncertainty in projected marine mammal distributions ultimately reflects the integration of uncertainties in climate, food resources and marine mammal ecology, physiology and behavior (Cheung et al., 2016; Payne et al., 2016). Each of these components is likely to make a significant contribution to the combined uncertainty. The complexity of marine mammal ecology, physiology and behavior (see “Marine Mammal Ecology and Climate Change”), however, suggests that marine mammal responses to changing habitat and prey distributions may be a dominant uncertainty source for climate change projections. This suggests the importance of: (a) better understanding marine mammal behavior in order to improve the ecological and mechanistic underpinning of statistical habitat models; and (b) continued development and application of mechanistic population models as critical steps to increasing confidence in marine mammal projections under climate change. Confidence in physical climate change projections rests in large part on the foundation of climate models on robust physical principles that are expected to hold as climate changes (Randall et al., 2007). Solidifying the ecological and physiological principles upon which marine resource projections are based is equally critical (Stock et al., 2011).
Key requirements for credible mechanistic models are observations that provide the understanding and constraints necessary to build and validate such models. To support modeling efforts, research plans are needed that extend beyond the collection and dissemination of marine mammal occurrence and environmental correlate data, particularly where critical management needs exist or where correlative modeling studies are already underway. For example, improved understanding of how prey resources drive marine mammal distribution as well as the underlying physical and biological features that dictate prey occurrence (Fiedler et al., 1998; Croll et al., 2005; Santora et al., 2013; Schroeder et al., 2014) will likely lead to advances in marine mammal distribution modeling.
Monitoring of marine mammals and their habitats has become increasingly sophisticated, yet despite the broad distribution of many species well outside continental shelves, most monitoring programs are largely focused in coastal and nearshore areas, where most anthropogenic activities are concentrated. As a result, validating models and detecting changes in distribution and regional abundance of many species may be difficult. Monitoring networks are needed that incorporate additional assets outside areas where marine mammals are traditionally studied. Research vessel surveys allow the collection of broad-scale data on marine mammals and their environment, but such surveys are costly and often limited by ship-time availability. Technologies such as passive acoustics, satellite telemetry, remote sensing, and autonomous underwater vehicles (AUVs) are also well-suited for collecting data on marine mammal occurrence and important physical and oceanographic data and may provide cost-effective alternatives, especially in remote areas. As such, long-term planning for data collection and monitoring is needed, accompanied by fiscal planning for access to appropriate platforms for gathering priority data.
Another critical challenge in projecting marine mammal responses to climate change is uncertainty in regional climate and earth system projections (Hawkins and Sutton, 2009; Frölicher et al., 2016). Improved resolution of regional climate processes is a focal point of current climate research (Xie et al., 2015). Refined resolution in global climate models has allowed them to better resolve coastal processes and, in some cases, to reduce regional model biases (Saba et al., 2016). Dynamical downscaling offers a second means of improving coastal process resolution (e.g., Hermann et al., 2016; Holt et al., 2016), but the underlying global climate models are still subject to biases that propagate through regional model boundaries (e.g., Meier et al., 2006). Lastly, climate variability, which present-day models suggest has limited predictability beyond a year for all but a few regions of the ocean (Meehl et al., 2014), will continue to contribute uncertainty to future climate states (e.g., Deser et al., 2012). These results suggest that some gains in the accuracy and precision of regional climate change projections are likely, but progress will be difficult. Future marine mammal projections at regional scales must thus prioritize using a range of climate projections to ensure the best possible estimate of the range of potential marine mammal responses.
To be effective, future modeling efforts must involve researchers from diverse disciplines, including climate science, ecology, physical oceanography, marine mammal biology, and marine resource management. These multidisciplinary research efforts will be essential to identify how ongoing marine mammal modeling studies might be refined, better characterized to reduce the uncertainties in projections on different time and spatial scales, and build their value in informing pressing management decisions—both relating to marine mammals directly as well as for other interacting ecosystem components. Thus, marine mammal populations of highest concern, settings where protective measures are needed most, and the temporal and spatial scales for management actions need to be identified. In addition, biological and climate change modelers must provide feedback on the limits of modeling efforts and describe likely levels of uncertainty in model outputs.
Priority Actions and Exemplar Species
The broad scope of the challenges identified above (“Current Approaches for Predicting and Projecting Marine Mammal Occurrence and Distribution”) and the limited resources available to meet these challenges suggests initial prioritization of species/populations that represent a high management need and represent a high suitability to be modeled. Species that represent high management need are: (a) depleted or currently undergoing declines in abundance; (b) exposed to multiple (and perhaps compounding) anthropogenic threats; or (c) occur in high latitudes or other regions undergoing rapid change. Species with a high modeling suitability may: (a) already have multiple aspects of their ecology quantified; or (b) occur in ecosystems modeled by previous or ongoing efforts. In contrast, it may be of relatively limited value to devote resources to populations that are abundant and increasing in number [e.g., sperm whales (Physeter macrocephalus)] or of relatively low management priority, or for which modeling suitability may be limited (Figure 2).
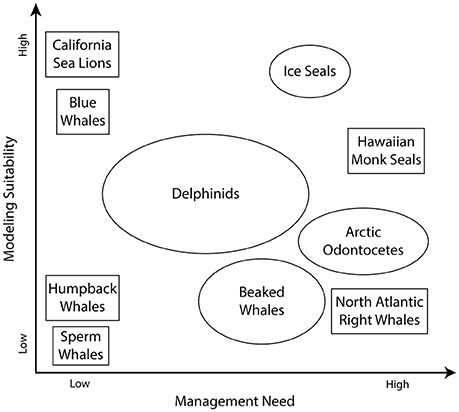
Figure 2. The range of increasing modeling suitability and management need for some illustrative marine mammal populations. Species and their relative positions are provided for illustration purposes and may not indicate an official agency prioritization.
Some marine mammal populations with limited ranges are exhibiting slow population growth or undergoing declines in abundance, such as the Cook Inlet beluga whale (Delphinapterus leucas) and vaquita (Phocoena sinus) (NMFS, 2016; Jaramillo-Legorreta et al., 2017). Note that for these species, fisheries bycatch and other factors may be the proximal cause for the severe depletion or extinction. However, given their highly-limited ranges, vulnerability to climate change is heightened as habitat and ecosystem perturbations become added stressors. Other species or populations with limited ranges, specialized diets, or similarly limiting ecological features may also be particularly vulnerable to habitat perturbations or large-scale ecological shifts. These populations, in addition to those exposed to the effects of various human activities such as bycatch in fisheries, underwater industrial noise, or competition for commercially valuable fish species, are high-priority candidates for refining forecast modeling studies. Ongoing efforts are focused on developing approaches to identify which protected marine vertebrates (i.e., pinnipeds, cetaceans, and turtles) are likely to be vulnerable to climate change and the attributes that make them vulnerable (Link et al., 2015), using an approach similar to that used for commercial fisheries (Pecl et al., 2014; Morrison et al., 2015; Hare et al., 2016). Such vulnerability assessments can inform decisions regarding priorities for assessing future impacts on marine mammals.
High-latitude marine systems are among the regions responding most rapidly to climate change (e.g., Doney et al., 2012; Hobday and Pecl, 2014; Thomas et al., 2016). As a result, marine mammal populations in these regions are likely to undergo range expansions/contractions or changes in local abundance sooner and perhaps more profoundly than in other regions, particularly those at lower latitudes (e.g., Laidre et al., 2008; Moore and Huntington, 2008; Gilg et al., 2012). These changes may lead to novel species interactions (Doney et al., 2012). Shifts in occurrence or availability of key prey species may also be expected in these high-latitude areas (McBride et al., 2014; Thomas et al., 2016). Ice-obligate species that use ice as a platform for raising young (e.g., ringed seal pupping lairs) (NOAA, 2012) or for hunting (e.g., for polar bears, Ursus maritimus), or whose prey species are closely or directly linked to ice [e.g., Antarctic fur seals (Arctocephalus gazella) (Forcada et al., 2008)] may be particularly vulnerable to declines in the extent of seasonal and multi-year ice. While some forecasting work has already been done for polar bears, ice seals, and walruses (Odobenus rosmarus) in the context of endangered species determinations under the ESA (Jay et al., 2011; Regehr et al., 2016), additional work is required to develop and refine models for species residing or occurring seasonally in high latitudes.
Forecast uncertainties will be less in cases where the species' ecology is relatively well understood and plentiful occurrence and environmental data exist than for data-poor populations. Thus, modeling populations about which much is already known is of particular importance because such studies may help elucidate potential climate change sensitivities that can strategically guide conservation and management efforts for less well observed regions.
Given the level of effort that has been devoted to characterizing their marine mammal populations and physical and biological components, the California Current and Bering Sea ecosystems are particularly important areas for such modeling efforts (Moore, 2008; Friday et al., 2013; Redfern et al., 2013; Dransfield et al., 2014; Becker et al., 2016). Continuation of these studies should be encouraged because ample environmental and species distribution data already exist, linkages between species occurrence and their environmental drivers have been explored, and these systems have been extensively modeled. Modeling studies of multiple trophic levels, species complexes, and well-understood systems (predator-prey relationships in particular) are a means to capitalize on interdisciplinary work already underway, represent cost-effective ways to maximize use of limited analytical resources, and provide opportunities for model validation with application to other taxa. They also help foster collaborative work with other modeling studies (e.g., fisheries, ecosystem) in the region studied. This will be most effective in regions where species interactions and relations to the physical environment are already well known. Thus, candidates for exploratory study of species complexes might include biogeographic provinces such as along shelf breaks, and portions of Bering Sea, Gulf Stream, and Antarctic ecosystems.
Therefore, key candidate marine mammal populations for future modeling studies are those particularly vulnerable to climate change, with high management priorities, and/or for which ecological data already exist. Among these are ice seal species [e.g., ringed seals and bearded seals (Erignathus barbatus)], Arctic odontocetes [beluga, narwhal (Monodon monoceros), and killer whales (Orcinus orca)], bowhead whales, North Pacific right whales (Eubalaena japonica), North Atlantic right whales, and cetacean species occurring in the Bering Sea [e.g., humpback and gray whales or California Current ecosystems [gray, fin (Balaenoptera physalus), and blue whales]; Figure 2].
Conclusions
Climate change presents unprecedented challenges for managers responsible for developing and implementing conservation measures for marine mammals. As marine mammals respond to climate change, protective measures must be developed that are responsive to provide adequate protection, which requires the ability to anticipate changes in occurrence, distribution, phenology, and relative abundance of populations. A range of models, varying in levels of complexity and with varying informational and computational requirements, have been developed to project climate change impacts on marine organisms. These models can be adjusted to appropriate temporal and spatial scales and use new and existing species-related data (e.g., life history, distributional ecology, behavioral responses, and population dynamics) to be more effective under projected climate conditions. Ongoing modeling studies can be strengthened by improving predictive capacities, increasing data available for marine mammals and their habitats, particularly in areas poorly-sampled in the past.
Marine resource managers and modelers need to work together to identify priority management needs and the strengths and weaknesses inherent in modeling studies. Agencies should engage in long-term fiscal planning to equip scientists and managers with tools and resources needed to increase data collection, enhance ongoing studies, and refine current models, particularly as they pertain to appropriate temporal and spatial scales relevant to conservation/management actions in settings where needs are greatest. Given the global scale and complexity of both climate change impacts and marine mammal distributions, multidisciplinary collaborations are necessary to develop new, and improve existing, models to better address conservation of living marine resources in an uncertain future.
Author Contributions
GS and ML: Lead authors and workshop co-convenor. PT: Author, workshop co-convenor. JB, MB, EB, PB, DD, JeF, JaF, KF, RG, JH, AH, DH, KL, NM, LQ, JS, KS, PS, CS, WS, KV, and RW: workshop participants and contributing authors. All authors made substantial contributions to the workshop content and discussion of ideas, drafting and revising the manuscript, providing final approval of submitted version and agree to be accountable for the work.
Funding
The U.S. National Oceanic and Atmospheric Administration and U.S. Marine Mammal Commission provided support for workshop travel and logistics funding.
Conflict of Interest Statement
The authors declare that the research was conducted in the absence of any commercial or financial relationships that could be construed as a potential conflict of interest.
Acknowledgments
The concepts and findings reported here were the product of a workshop convened on 12–14 January 2016 in Santa Cruz, CA, USA. Its goals were to: identify approaches to assess future changes in distribution and abundance of marine mammal species, metrics relevant to assessing physical changes and biological responses of marine mammals, exemplar species/population(s) for assessing approaches or metrics, and next steps for performing these studies. The workshop was jointly funded and co-convened by NOAA's Offices of Protected Resources and Science & Technology, and the U.S. Marine Mammal Commission. We are grateful to the workshop Steering Committee: MB, PB, RG, NM, GS, PT, KV, and RW.
References
Angliss, R., Silber, G., and Merrick, R. (2002). Report of a Workshop on Developing Recovery Criteria for Large Whale Species. U.S. Department of Commerce. NOAA Technical Memorandum NMFS-F/OPR-21.p. 32.
Araujo, M. B., and Guisan, A. (2006). Five (or so) challenges for species distribution modelling. J. Biogeogr. 33, 1677–1688. doi: 10.1111/j.1365-2699.2006.01584.x
Baker, J., Littnan, C., and Johnston, D. (2006). Potential effects of sea-level rise on the terrestrial habitats of endangered and endemic megafauna in the Northwestern Hawaiian Islands. Endanger. Species Res. 4, 1–10. doi: 10.3354/esr002021
Barlow, J., Ferguson, M. C., Becker, E., Redfern, J. V., Forney, K. A., Vilchis, I. L., et al. (2009). Predictive Modeling of Cetacean Densities in the Eastern Pacific Ocean. U.S. Department of Commerce. NOAA Technical Memorandum NMFS-SWFSC-444.p. 206.
Baumgartner, M. F., and Mate, B. R. (2005). Summer and fall habitat of North Atlantic right whales (Eubalaena glacialis) inferred from satellite telemetry. Can. J. Fish. Aquat. Sci. 62, 527–543. doi: 10.1139/f04-238
Baumgartner, M. F., Cole, T. V. N., Clapham, P. J., and Mate, B. R. (2003). North Atlantic right whale habitat in the lower Bay of Fundy and on the SW Scotian Shelf during 1999-2001. Mar. Ecol. Prog. Ser. 264, 137–154. doi: 10.3354/meps264137
Becker, E., Forney, K., Fiedler, P., Barlow, J., Chivers, S. J., Edwards, C. A., et al. (2016). Moving towards dynamic ocean management: how well do modeled ocean products predict species distributions? Remote Sens. 8:149. doi: 10.3390/rs8020149
Becker, E. A., Foley, D. G., Forney, K. A., Barlow, J., Redfern, J. V., and Gentemann, C. L. (2012). Forecasting cetacean abundance patterns to enhance management decisions. Endanger. Species Res. 16, 97–112. doi: 10.3354/esr00390
Becker, E. A., Forney, K. A., Ferguson, M. C., Foley, D. G., Smith, R. C., Barlow, J., et al. (2010). Comparing California Current cetacean-habitat models developed using in situ and remotely sensed sea surface temperature data. Mar. Ecol. Prog. Ser. 413, 163–183. doi: 10.3354/meps08696
Becker, E. A., Forney, K. A., Foley, D. G., Smith, R. C., Moore, T. J., and Barlow, J. (2014). Predicting seasonal density patterns of California cetaceans based on habitat models. Endanger. Species Res. 23, 1–22. doi: 10.3354/esr00548
Benson, S. R., Croll, D. A., Marinovic, B. B., Chavezc, F. P., and Harvey, J. T. (2002). Changes in the cetacean assemblage of a coastal upwelling ecosystem during El Nino 1997-98 and La Nina 1999. Prog. Oceanogr. 54, 279–291. doi: 10.1016/S0079-6611(02)00054-X
Bryant, P. J., Lafferty, C. M., and Lafferty, S. K. (1984). “Reoccupation of Laguna Guerrero Negro, Baja California, Mexico, by gray whales,” in The Gray Whale: Eschrichtius robustus, eds M. L. Jones, S. L. Swartz, and S. Leatherwood (Orlando, FL: Academic Press), 375–387.
Bureau of Ocean Energy Management (BOEM) (2016). Notice of Availability (NOA) of and Request for Comments on the 2017–2022 Outer Continental Shelf (OCS) Oil and Gas Leasing Proposed Program. Fed. Reg. 81, 14881–14885. Available online at: https://www.gpo.gov/fdsys/pkg/FR-2016-03-18/pdf/2016-06109.pdf
Burrows, M. T., Schoeman, D. S., Buckley, L. B., Moore, P., Poloczanska, E. S., Brander, K. M., et al. (2011). The pace of shifting climate in marine and terrestrial ecosystems. Science 334, 652–655. doi: 10.1126/science.1210288
Carroll, E. L., Rayment, W. J., Alexander, A. M., Baker, C. S., Patenaude, N. J., Steel, D., et al. (2014). Reestablishment of former wintering grounds by New Zealand southern right whales. Mar. Mamm. Sci. 30, 206–220. doi: 10.1111/mms.12031
Cheung, W. W. L., Frölicher, T. L., Asch, R. G., Jones, M. C., Pinsky, M. L., Reygondeau, G., et al. (2016). Building confidence in projections of the responses of living marine resources to climate change. ICES J. Mar. Sci. 73, 1283–1296. doi: 10.1093/icesjms/fsv250
Chust, G., Allen, J. I., Bopp, L., Schrum, C., Holt, J., Tsiaras, K., et al. (2014). Biomass changes and trophic amplification of plankton in a warmer ocean. Glob. Chang. Biol. 20, 2124–2139. doi: 10.1111/gcb.12562
Citta, J. J., Quakenbush, L. T., Okkonen, S. R., Druckenmiller, M. L., Maslowski, W., Clement-Kinney, J., et al. (2015). Ecological characteristics of core-use areas used by Bering-Chukchi-Beaufort (BCB) bowhead whales, 2006-2012. Prog. Oceanogr. 136, 201–222. doi: 10.1016/j.pocean.2014.08.012
Clarke, J., Stafford, K., Moore, S. E., Rone, B., Aerts, L., and Crance, J. (2013). Subarctic cetaceans in the southern Chukchi Sea: evidence of recovery or response to a changing ecosystem. Oceanography 26, 136–151. doi: 10.5670/oceanog.2013.81
Cribb, N., Miller, C., and Seuront, L. (2015). Towards a standardized approach of cetacean habitat: past achievements and future directions. Open J. Mar. Sci. 5, 335–357. doi: 10.4236/ojms.2015.53028
Croll, D. A., Marinovic, B., Benson, S., Chavez, F. P., Black, N., Ternullo, R., et al. (2005). From wind to whales: trophic links in a coastal upwelling system. Mar. Ecol. Prog. Ser. 289, 117–130. doi: 10.3354/meps289117
Cury, P. M., Shin, Y. J., Planque, B., Durant, J. M., Fromentin, J. M., Kramer-Schadt, S., et al. (2008). Ecosystem oceanography for global change in fisheries. Trends Ecol. Evol. (Amst). 23, 338–346. doi: 10.1016/j.tree.2008.02.005
Deser, C., Knutti, R., Solomon, S., and Phillips, A. S. (2012). Communication of the role of natural variability in future North American climate. Nat. Clim. Change 2, 775–779. doi: 10.1038/nclimate1562
Doney, S. C., Ruckelshaus, M., Duffy, J. E., Barry, J. P., Chan, F., English, C. A., et al. (2012). Climate change impacts on marine ecosystems. Ann. Rev. Mar. Sci. 4, 11–37. doi: 10.1146/annurev-marine-041911-111611
Dransfield, A., Hines, E., McGowan, J., Holzman, B., Nur, N., Elliott, M., et al. (2014). Where the whales are: using habitat modeling to support changes in shipping regulations within National Marine Sanctuaries in Central California. Endanger. Species Res. 26, 39–57. doi: 10.3354/esr00627
Dunn, D. C., Maxwell, S. M., Boustany, A. M., and Halpin, P. N. (2016). Dynamic ocean management increases the efficiency and efficacy of fisheries management. Proc. Natl. Acad. Sci. U.S.A. 113, 668–673. doi: 10.1073/pnas.1513626113
Elith, J., and Graham, C. H. (2009). Do they? How do they? WHY do they differ? On finding reasons for differing performances of species distribution models. Ecography 32, 66–77. doi: 10.1111/j.1600-0587.2008.05505.x
Ferguson, M. C., Barlow, J., Fiedler, P., Reilly, S. B., and Gerrodette, T. (2006). Spatial models of delphinid (family Delphinidae) encounter rate and group size in the eastern tropical Pacific Ocean. Ecol. Model. 193, 645–662. doi: 10.1016/j.ecolmodel.2005.10.034
Fiechter, J., Huckstadt, L. A., Rose, K. A., and Costa, D. P. (2016). A fully coupled ecosystem model to predict the foraging ecology of apex predators in the California Current. Mar. Ecol. Prog. Ser. 556, 273–285. doi: 10.3354/meps11849
Fiechter, J., Rose, K. A., Curchitser, E. N., and Hedstromd, K. S. (2015). The role of environmental controls in determining sardine and anchovy population cycles in the California Current: Analysis of an end-to-end model. Prog. Oceanogr. 138, 381–398. doi: 10.1016/j.pocean.2014.11.013
Fiedler, P. C., Reilly, S. B., Hewitt, R. P., Demer, D., Philbrick, V. A., Smith, S., et al. (1998). Blue whale habitat and prey in the California Channel Islands. Deep Sea Res. II. 45, 1781–1801. doi: 10.1016/S0967-0645(98)80017-9
Fleming, A. H., Clark, C. T., Calambokidis, J., and Barlow, J. (2016). Humpback whale diets respond to variance in ocean climate and ecosystem conditions in the California Current. Glob. Chang. Biol. 22, 1214–1224. doi: 10.1111/gcb.13171
Forcada, J., Trathan, P. N., Boveng, P. L., Boyd, I. L., Burns, J. M., Costa, D. P., et al. (2012). Responses of Antarctic pack-ice seals to environmental change and increasing krill fishing. Biol. Conserv. 149, 40–50. doi: 10.1016/j.biocon.2012.02.002
Forcada, J., Trathan, P. N., and Murphy, E. J. (2008). Life history buffering in Antarctic mammals and birds against changing patterns of climate and environmental variation. Glob. Chang. Biol. 14, 2473–2488. doi: 10.1111/j.1365-2486.2008.01678.x
Forcada, J., Trathan, P. N., Reid, K., Murphy, E. J., and Croxall, J. P. (2006). Contrasting population changes in sympatric penguin species in association with climate warming. Glob. Chang. Biol. 12, 411–423. doi: 10.1111/j.1365-2486.2006.01108.x
Forney, K. A., Becker, E. A., Foley, D. G., Barlow, J., and Oleson, E. M. (2015). Habitat-based models of cetacean density and distribution in the central North Pacific. Endanger. Species Res. 27, 1–20. doi: 10.3354/esr00632
Forney, K. A., Ferguson, M. C., Becker, E. A., Fiedler, P. C., Redfern, J. V., Barlow, J., et al. (2012). Habitat-based spatial models of cetacean density in the eastern Pacific Ocean. Endanger. Species Res. 16, 113–133. doi: 10.3354/esr00393
Friday, N. A., Zerbini, A. N., Waite, J. M., Moore, S. E., and Clapham, P. J. (2013). Cetacean distribution and abundance in relation to oceanographic domains on the eastern Bering Sea shelf, June and July of 2002, 2008, and 2010. Deep Sea Res. II 94, 244–256. doi: 10.1016/j.dsr2.2013.03.011
Friedrichs, M. A. M., Dusenberry, J. A., Anderson, L. A., Armstrong, R. A., Chai, F., Christian, J. R., et al. (2007). Assessment of skill and portability in regional marine biogeochemical models: role of multiple planktonic groups. J. Geophys. Res. Oceans 112:C08001. doi: 10.1029/2006JC003852
Frölicher, T. L., Rodgers, K. B., Stock, C. A., and Cheung, W. W. L. (2016). Sources of uncertainties in 21st century projections of potential ocean ecosystem stressors. Global Biogeochem. Cycles 30, 1224–1243. doi: 10.1002/2015GB005338
Gehlen, M., Barciela, R., Bertino, L., Brasseur, P., Butenschön, M., Chai, F., et al. (2015). Building the capacity for forecasting marine biogeochemistry and ecosystems: recent advances and future developments. J. Oper. Oceanogr. 8, S168–S187. doi: 10.1080/1755876X.2015.1022350
George, J. C., Druckenmiller, M. L., Laidre, K. L., Suydam, R., and Person, B. (2015). Bowhead whale body condition and links to summer sea ice and upwelling in the Beaufort Sea. Prog. Oceanogr. 136, 250–262. doi: 10.1016/j.pocean.2015.05.001
Gilg, O., Kovacs, K. M., Aars, J., Fort, J., Gauthier, G., Grémillet, D., et al. (2012). Climate change and the ecology and evolution of Arctic vertebrates. Ann. N.Y. Acad. Sci. 1249, 166–190. doi: 10.1111/j.1749-6632.2011.06412.x
Gilles, A., Adler, S., Kaschner, K., Scheidat, M., and Siebert, U. (2011). Modelling harbour porpoise seasonal density as a function of the German Bight environment: implications for management. Endanger. Species Res. 14, 157–169. doi: 10.3354/esr00344
Gregr, E. J., Baumgartner, M. F., Laidre, K. L., and Palacios, D. M. (2013). Marine mammal habitat models come of age: the emergence of ecological and management relevance. Endanger. Species Res. 22, 205–212. doi: 10.3354/esr00476
Guisan, A., and Thuiller, W. (2005). Predicting species distribution: offering more than simple habitat models. Ecol. Lett. 8, 993–1009. doi: 10.1111/j.1461-0248.2005.00792.x
Hamilton, C. D., Lydersen, C., Ims, R. A., and Kovacs, K. M. (2015). Predictions replaced by facts: a keystone species' behavioural responses to declining arctic sea-ice. Biol. Lett. 11:20150803. doi: 10.1098/rsbl.2015.0803
Hamilton, T. A., Redfern, J. V., Barlow, J., Ballance, L. T., Gerrodette, T., Holt, R. S., et al. (2009). Atlas of Cetacean Sightings from Southwest Fisheries Science Center Cetacean Ecosystem Surveys: 1986–2005. U.S. Department of Commerce. NOAA Technical Memorandum NMFS-SWFSC-440. p. 70.
Hare, J. A., Manderson, J. P., Nye, J. A., Alexander, M. A., Auster, P. J., Borggaard, D. L., et al. (2012). Cusk (Brosme brosme) and climate change: assessing the threat to a candidate marine fish species under the US Endangered Species Act. ICES J. Mar. Sci. 69, 1753–1768. doi: 10.1093/icesjms/fss160
Hare, J. A., Morrison, W. E., Nelson, M. W., Stachura, M. M., Teeters, E. J., Griffis, R. B., et al. (2016). A vulnerability assessment of fish and invertebrates to climate change on the Northeast, U.S. Continental Shelf. PLoS ONE 11:e0146756. doi: 10.1371/journal.pone.0146756
Hawkins, E., and Sutton, R. (2009). The potential to narrow uncertainty in regional climate predictions. B. Am. Meteorol. Soc. 90:1095. doi: 10.1175/2009BAMS2607.1
Hazen, E. L., Jorgensen, S., Rykaczewski, R. R., Bograd, S. J., Foley, D. G., Jonsen, I. D., et al. (2013). Predicted habitat shifts of Pacific top predators in a changing climate. Nat. Clim. Change 3, 234–238. doi: 10.1038/nclimate1686
Hazen, E. L., Palacios, D. M., Forney, K. A., Howell, E. A., Becker, E., Hoover, A. L., et al. (2016). WhaleWatch: a dynamic management tool for predicting blue whale density in the California Current. J. Appl. Ecol. 54, 1415–1428. doi: 10.1111/1365-2664.12820
Hermann, A. J., Gibson, G. A., Bond, N. A., Curchitser, E. N., Hedstrom, K., Cheng, W., et al. (2016). Projected future biophysical states of the Bering Sea. Deep Sea Res. II 134, 30–47. doi: 10.1016/j.dsr2.2015.11.001
Hezel, P. J., Zhang, X., Bitz, C. M., Kelly, B. P., and Massonnet, F. (2012). Projected decline in spring snow depth on Arctic sea ice caused by progressively later autumn open ocean freeze-up this century. Geophys. Res. Lett. 39:L17505. doi: 10.1029/2012GL052794
Hobday, A. J., and Pecl, G. T. (2014). Identification of global marine hotspots: sentinels for change and vanguards for adaptation action. Rev. Fish Biol. Fish. 24, 415–425. doi: 10.1007/s11160-013-9326-6
Hobday, A. J., Spillman, C. M., Eveson, J. P., and Hartog, J. R. (2016). Seasonal forecasting for decision support in marine fisheries and aquaculture. Fish. Oceanogr. 25, 45–56. doi: 10.1111/fog.12083
Holt, J., Schrum, C., Cannaby, H., Daewel, U., Allen, I., Artioli, Y., et al. (2016). Potential impacts of climate change on the primary production of regional seas: a comparative analysis of five European seas. Prog. Oceanogr. 140, 91–115. doi: 10.1016/j.pocean.2015.11.004
Hothorn, T., Muller, J., Schroder, B., Kneib, T., and Brandl, R. (2011). Decomposing environmental, spatial, and spatiotemporal components of species distributions. Ecol. Monogr. 81, 329–347. doi: 10.1890/10-0602.1
Hoyt, E. (2011). Marine Protected Areas for Whales, Dolphins and Porpoises: A World Handbook for Cetacean Habitat Conservation and Planning. London: Earthscan/Routledge and Taylor & Francis.
Intergovernmental Panel on Climate Change (IPCC) (2014). “Climate change 2014: synthesis report,” in Contribution of Working Groups I, II and III to the Fifth Assessment Report of the Intergovernmental Panel on Climate Change, eds R. K. Pachauri, M. R. Allen, V. R. Barros, J. Broome, W. Cramer, R. Christ, et al. (Geneva: Intergovernmental Panel on Climate Change), p. 151.
International Whaling Commission (IWC) (2010). Report of the workshop on cetaceans and climate change. J. Cetacean Res. Manag. Supp. 11, 451–480. Available online at: https://archive.iwc.int/pages/view.php?ref=295&k=&search=%21collection29&offset=0&order_by=relevance&sort=DESC&archive=0
Ito, S. I., Rose, K. A., Megrey, B. A., Schweigert, J., Hay, D., Werner, F. E., et al. (2015). Geographic variation in Pacific herring growth in response to regime shifts in the North Pacific Ocean. Prog. Oceanogr. 138, 331–347. doi: 10.1016/j.pocean.2015.05.022
Jaramillo-Legorreta, A., Cardenas-Hinojosa, G., Nieto-Garcia, E., Rojas-Bracho, L., Ver Hoef, J., Moore, J., et al. (2017). Passive acoustic monitoring of the decline of Mexico's critically endangered vaquita. Conserv. Biol. 31, 183–191. doi: 10.1111/cobi.12789
Jay, C. V., Marcot, B. G., and Douglas, D. C. (2011). Projected status of the Pacific walrus (Odobenus rosmarus divergens) in the twenty-first century. Polar Biol. 34, 1065–1084. doi: 10.1007/s00300-011-0967-4
Keller, C. A., Garrison, L., Baumstark, R., Ward-Geiger, L. I., and Hines, E. (2012). Application of a habitat model to define calving habitat of the North Atlantic right whale in the southeastern United States. Endanger. Species Res. 18, 73–87. doi: 10.3354/esr00413
Kovacs, K. M., Lydersen, C., Overland, J. E., and Moore, S. E. (2011). Impacts of changing sea-ice conditions on Arctic marine mammals. Mar. Biodiv. 41, 181–194. doi: 10.1007/s12526-010-0061-0
Laidre, K. L., Heide-Jorgensen, M. P., and Nielsen, T. G. (2007). Role of the bowhead whale as a predator in West Greenland. Mar. Ecol. Prog. Ser. 346, 285–297. doi: 10.3354/meps06995
Laidre, K. L., Stern, H., Kovacs, K. M., Lowry, L., Moore, S. E., Regehr, E. V., et al. (2015). Arctic marine mammal population status, sea ice habitat loss, and conservation recommendations for the 21st century. Conserv. Biol. 29, 724–737. doi: 10.1111/cobi.12474
Laidre, K. L., Stirling, I., Lowry, L. F., Wiig, O., Heide-Jørgensen, M. P., Ferguson, S. H., et al. (2008). Quantifying the sensitivity of Arctic marine mammals to climate-induced habitat change. Ecol. Appl. 18, S97–S125. doi: 10.1890/06-0546.1
Lauria, V., Attrill, M. J., Pinnegar, J. K., Brown, A., Edwards, M., and Votier, S. C. (2012). Influence of climate change and trophic coupling across four trophic levels in the Celtic Sea. PLoS ONE 7:e47408. doi: 10.1371/journal.pone.0047408
Learmonth, J. A., MacLeod, C. D., Santos, M. B., Crick, H. Q. P., Pierce, G. J., Learmonth, J. A., et al. (2006). Potential effects of climate change on marine mammals. Oceanogr. Mar. Biol. 44, 431–464. doi: 10.1201/9781420006391.ch8
Lefort, S., Aumont, O., Bopp, L., Arsouze, T., Gehlen, M., Maury, O., et al. (2015). Spatial and body-size dependent response of marine pelagic communities to projected global climate change. Glob. Chang. Biol. 21, 154–164. doi: 10.1111/gcb.12679
Lehodey, P., Senina, I., and Murtugudde, R. (2008). A spatial ecosystem and populations dynamics model (SEAPODYM) - Modeling of tuna and tuna-like populations. Prog. Oceanogr. 78, 304–318. doi: 10.1016/j.pocean.2008.06.004
Link, J. S., Griffis, R., and Busch, S. (2015). NOAA Fisheries Climate Science Strategy. U.S. Department of Commerce. NOAA Technical Memorandum NMFS-F/SPO-155.p. 70.
Lynch, P. D., Nye, J. A., Hare, J. A., Stock, C. A., Alexander, M. A., Scott, J. D., et al. (2015). Projected ocean warming creates a conservation challenge for river herring populations. ICES J. Mar. Sci. 72, 374–387. doi: 10.1093/icesjms/fsu134
Mannocci, L., Catalogna, M., Doremus, G., Laran, S., Lehodey, P., Massart, W., et al. (2014). Predicting cetacean and seabird habitats across a productivity gradient in the South Pacific gyre. Prog. Oceanogr. 120, 383–398. doi: 10.1016/j.pocean.2013.11.005
Maxwell, S. M., Hazen, E. L., Lewison, R. L., Dunn, D. C., Bailey, H., Bograd, S. J., et al. (2015). Dynamic ocean management: defining and conceptualizing real-time management of the ocean. Mar. Policy 58, 42–50. doi: 10.1016/j.marpol.2015.03.014
McBride, M. M., Dalpadado, P., Drinkwater, K. F., Godø, O. R., Hobday, A. J., Hollowed, A. B., et al. (2014). Krill, climate, and contrasting future scenarios for Arctic and Antarctic fisheries. ICES J. Mar. Sci. 71, 1934–1955. doi: 10.1093/icesjms/fsu002
Meehl, G. A., Goddard, L., Boer, G., Burgman, R., Branstator, G., Cassou, C., et al. (2014). Decadal climate prediction: an update from the trenches. B. Am. Meteorol. Soc. 95, 243–267. doi: 10.1175/BAMS-D-12-00241.1
Meier, H. E. M., Kjellstrom, E., and Graham, L. P. (2006). Estimating uncertainties of projected Baltic Sea salinity in the late 21st century. Geophys. Res. Lett. 33:L15705. doi: 10.1029/2006GL026488
Monahan, W. B. (2009). A mechanistic niche model for measuring species' distributional responses to seasonal temperature gradients. PLoS ONE 4:e7921. doi: 10.1371/journal.pone.0007921
Moore, S. E. (2008). Marine mammals as ecosystem sentinels. J. Mammal. 89, 534–540. doi: 10.1644/07-MAMM-S-312R1.1
Moore, S. E., and Huntington, H. P. (2008). Arctic marine mammals and climate change: impacts and resilience. Ecol. Appl. 18, s157–s165. doi: 10.1890/06-0571.1
Moore, S. E., Watkins, W. A., Daher, M. A., Davies, J. R., and Dahlheim, M. E. (2002). Blue whale habitat associations in the Northwest Pacific: analysis of remotely-sensed data using a Geographic Information System. Oceanography 15, 20–25. doi: 10.5670/oceanog.2002.11
Morrison, W. E., Nelson, M. W., Howard, J. F., Teeters, E. J., Hare, J. A., Griffis, R. B., et al. (2015). Methodology for Assessing the Vulnerability of Marine Fish and Shellfish Species to a Changing Climate. U.S. Department of Commerce. NOAA Technical Memorandum NMFS-OSF-3. p. 48.
National Centers for Environmental Information (NCEI) (2017). Available online at: https://www.nodc.noaa.gov/access/allproducts.html
National Marine Fisheries Service (NMFS) (2016). Recovery Plan for the Cook Inlet Beluga Whale (Delphinapterus leucas). Juneau, AK: National Marine Fisheries Service ARRD.
National Oceanic and Atmospheric Administration (NOAA) (2012). Final rule: Endangered and threatened species; Threatened status for the Arctic, Okhotsk, and Baltic subspecies of the ringed seal and endangered status for the Ladoga subspecies of the ringed seal. Fed. Reg. 77, 76705–76738. Available online at: https://www.gpo.gov/fdsys/pkg/FR-2012-12-28/pdf/2012-31066.pdf
National Oceanic and Atmospheric Administration (NOAA) (2013). Endangered fish and wildlife; final rule to remove the sunset provision of the final rule implementing vessel speed restrictions to reduce the threat of ship collisions with North Atlantic right whales. Fed. Reg. 78, 73726–73736. Available online at: https://www.gpo.gov/fdsys/pkg/FR-2013-12-09/pdf/2013-29355.pdf
National Oceanic and Atmospheric Administration (NOAA) (2015). Final rule: takes of marine mammals incidental to specified activities; U.S. navy training and testing activities in the northwest training and testing study area. Fed. Reg. 80, 73555–73629. Available online at: https://www.gpo.gov/fdsys/pkg/FR-2015-08-03/pdf/2015-18633.pdf
Nye, J. A., Link, J. S., Hare, J. A., and Overholtz, W. J. (2009). Changing spatial distribution of fish stocks in relation to climate and population size on the Northeast United States continental shelf. Mar. Ecol. Prog. Ser. 393, 111–129. doi: 10.3354/meps08220
Palacios, D. M., Baumgartner, M. F., Laidre, K. L., and Gregr, E. J. (2013). Beyond correlation: integrating environmentally and behaviourally mediated processes in models of marine mammal distributions. Endanger. Species Res. 22, 191–203. doi: 10.3354/esr00558
Patenaude, N. J., Baker, C. S., and Gales, N. J. (1998). Observations of southern right whales on New Zealand's subantarctic wintering grounds. Mar. Mammal Sci. 14, 350–355. doi: 10.1111/j.1748-7692.1998.tb00726.x
Payne, J. L., Bush, A. M., Heim, N. A., Knope, M. L., and McCauley, D. J. (2016). Ecological selectivity of the emerging mass extinction in the oceans. Science 353, 1284–1286. doi: 10.1126/science.aaf2416
Pecl, G. T., Ward, T. M., Doubleday, Z. A., Clarke, S., Day, J., Dixon, C., et al. (2014). Rapid assessment of fisheries species sensitivity to climate change. Clim. Change 127, 505–520. doi: 10.1007/s10584-014-1284-z
Perry, A. L., Low, P. J., Ellis, J. R., and Reynolds, J. D. (2005). Climate change and distribution shifts in marine fishes. Science 308, 1912–1915. doi: 10.1126/science.1111322
Peterson, W. T. (2009). Copepod species richness as an indicator of long-term changes in the coastal ecosystem of the northern California Current. CalCOFI Rep. 50, 73–81. Available online at: http://calcofi.org/publications/calcofireports/v50/73-81_Peterson.pdf
Pinsky, M. L., Worm, B., Fogarty, M. J., Sarmiento, J. L., and Levin, S. A. (2013). Marine taxa track local climate velocities. Science 341, 1239–1242. doi: 10.1126/science.1239352
Plaganyi, E. E., Bell, J. D., Bustamante, R. H., Dambacher, J. M., Dennis, D. M., Dichmont, C. M., et al. (2011). Modelling climate-change effects on Australian and Pacific aquatic ecosystems: a review of analytical tools and management implications. Mar. Freshw. Res. 62, 1132–1147. doi: 10.1071/MF10279
Poloczanska, E. S., Burrows, M. T., Brown, C. J., Molinos, J. G., Halpern, B. S., Hoegh-Guldberg, O., et al. (2016). Responses of marine organisms to climate change across oceans. Front. Mar. Sci. 3:62. doi: 10.3389/fmars.2016.00062
Poloczanska, E. S., Brown, C. J., Sydeman, W. J., Kiessling, W., Schoeman, D. S., Moore, P. J., et al. (2013). Global imprint of climate change on marine life. Nat. Clim. Change 3, 919–925. doi: 10.1038/nclimate1958
Ragen, T. J., Huntington, H. P., and Hovelsrud, G. K. (2008). Conservation of Arctic marine mammals faced with climate change. Ecol. Appl. 18, S166–S174. doi: 10.1890/06-0734.1
Randall, D., Wood, R., Bony, S., Colman, T., Fichefet, J., Fyfe, V., et al. (2007). “Climate models and their evaluation,” in Climate Change 2007: The Physical Science Basis Contribution of Working Group I to the Fourth Assessment Report of the Intergovernmental Panel on Climate Change (Cambridge: Cambridge University Press).
Redfern, J. V., Barlow, J., Ballance, L. T., Gerrodette, T., and Becker, E. A. (2008). Absence of scale dependence in dolphin-habitat models for the eastern tropical Pacific Ocean. Mar. Ecol. Prog. Ser. 363, 1–14. doi: 10.3354/meps07495
Redfern, J. V., McKenna, M. F., Moore, T. J., Calambokidis, J., Deangelis, M. L., Becker, E. A., et al. (2013). Assessing the risk of ships striking large whales in marine spatial planning. Conserv. Biol. 27, 292–302. doi: 10.1111/cobi.12029
Regehr, E. V., Laidre, K. L., Akçakaya, H. R., Amstrup, S. C., Atwood, T. C., Lunn, N. J., et al. (2016). Conservation status of polar bears (Ursus maritimus) in relation to projected sea-ice declines. Biol. Lett. 12:20160556. doi: 10.1098/rsbl.2016.0556
Rose, K. A., Sable, S., DeAngelis, D. L., Yurek, S., Trexler, J. C., Graf, W. L., et al. (2015). Proposed best modeling practices for assessing the effects of ecosystem restoration on fish. Ecol. Model. 300, 12–29. doi: 10.1016/j.ecolmodel.2014.12.020
Runge, J. A., Ji, R. B., Thompson, C. R. S., Record, N. R., Chen, C., Vandemark, D. C., et al. (2015). Persistence of Calanus finmarchicus in the western Gulf of Maine during recent extreme warming. J. Plankton Res. 37, 221–232. doi: 10.1093/plankt/fbu098
Saba, V. S., Griffies, S. M., Anderson, W. G., Winton, M., Alexander, M. A., Delworth, T. L., et al. (2016). Enhanced warming of the Northwest Atlantic Ocean under climate change. J. Geophys. Res. Oceans 121, 118–132. doi: 10.1002/2015JC011346
Santora, J. A., Sydeman, W. J., Messie, M., Chai, F., Chao, Y., Thompson, S. A., et al. (2013). Triple check: Observations verify structural realism of an ocean ecosystem model. Geophys. Res. Lett. 40, 1367–1372. doi: 10.1002/grl.50312
Santora, J. A., Sydeman, W. J., Schroeder, I. D., Wells, B. K., and Field, J. C. (2011). Mesoscale structure and oceanographic determinants of krill hotspots in the California current: implications for trophic transfer and conservation. Prog. Oceanogr. 91, 397–409. doi: 10.1016/j.pocean.2011.04.002
Scales, K. L., Hazen, E. L., Jacox, M. G., Edwards, C. A., Boustany, A. M., Oliver, M. J., et al. (2017). Scale of inference: on the sensitivity of habitat models for wide-ranging marine predators to the resolution of environmental data. Ecography 40, 210–220. doi: 10.1111/ecog.02272
Schroeder, I. D., Santora, J. A., Moore, A. M., Edwards, C. A., Fiechter, J., Hazen, E. L., et al. (2014). Application of a data-assimilative regional ocean modeling system for assessing California Current System ocean conditions, krill, and juvenile rockfish interannual variability. Geophys. Res. Lett. 41, 5942–5950. doi: 10.1002/2014GL061045
Schumann, N., Gales, N. J., Harcourt, R. G., and Arnould, J. P. Y. (2013). Impacts of climate change on Australian marine mammals. Aus. J. Zool. 61, 146–159. doi: 10.1071/ZO12131
Séférian, R., Bopp, L., Gehlen, M., Swingedouw, D., Mignot, J., Guilyardi, E., et al. (2014). Multiyear predictability of tropical marine productivity. Proc. Natl. Acad. Sci. U.S.A. 111, 11646–11651. doi: 10.1073/pnas.1315855111
Shelden, K., Sims, C., Vate Brattstrom, L., Goetz, K. T., and Hobbs, R. C. (2015). Aerial Surveys of Beluga Whales (Delphinapterus leucas) in Cook Inlet, Alaska, June 2014. AFSC Processed Report 2015–03, Alaska Fisheries Science Center, Seattle, WA.
Silber, G. K., Vanderlaan, A. S. M., Tejedor Arceredillo, A., Johnson, L., Taggart, C. T., Brown, M. W., et al. (2012). The role of the International Maritime Organization in reducing vessel threat to whales: process, options, action and effectiveness. Mar. Policy 36, 1221–1233. doi: 10.1016/j.marpol.2012.03.008
Smith, T. G., and Stirling, I. (1975). Breeding habitat of ringed seal (Phoca hispida) - birth lair and associated structures. Can. J. Zool. 53, 1297–1305. doi: 10.1139/z75-155
Soberón, J., and Nakamura, M. (2009). Niches and distributional areas: concepts, methods, and assumptions. Proc. Natl. Acad. Sci. U.S.A. 106, 19644–19650. doi: 10.1073/pnas.0901637106
Stock, C. A., Alexander, M. A., Bond, N. A., Brander, K. M., Cheung, W. W. L., Curchitser, E. N., et al. (2011). On the use of IPCC-class models to assess the impact of climate on Living Marine Resources. Prog. Oceanogr. 88, 1–27. doi: 10.1016/j.pocean.2010.09.001
Stock, C. A., Dunne, J. P., and John, J. G. (2014). Drivers of trophic amplification of ocean productivity trends in a changing climate. Biogeosciences 11, 7125–7135. doi: 10.5194/bg-11-7125-2014
Stock, C. A., John, J. G., Rykaczewski, R. R., Asch, R. G., Cheung, W. W. L., Dunne, J. P., et al. (2017). Reconciling fisheries catch and ocean productivity. Proc. Natl. Acad. Sci. U.S.A. 114, E1441–E1449. doi: 10.1073/pnas.1610238114
Stock, C. A., Pegion, K., Vecchi, G. A., Alexander, M. A., Tommasi, D., Bond, N. A., et al. (2015). Seasonal sea surface temperature anomaly prediction for coastal ecosystems. Prog. Oceanogr. 137, 219–236. doi: 10.1016/j.pocean.2015.06.007
Southwest Fisheries Science Center (SWFSC) (2017). ERDDAP. Available online at: http://coastwatch.pfeg.noaa.gov/erddap/index.html
Sydeman, W. J., Poloczanska, E., Reed, T. E., and Thompson, S. A. (2015). Climate change and marine vertebrates. Science 350, 772–777. doi: 10.1126/science.aac9874
Thomas, P. O., Reeves, R. R., and Brownell, R. L. (2016). Status of the world's baleen whales. Mar. Mammal Sci. 32, 682–734. doi: 10.1111/mms.12281
Tommasi, D., Stock, C. A., Hobday, A. J., Methot, R., Kaplan, I. C., Holsman, K., et al. (2017a). Managing living marine resources in a dynamic environment: the role of seasonal to decadal climate forecasts. Prog. Oceanogr. 152, 15–49. doi: 10.1016/j.pocean.2016.12.011
Tommasi, D., Stock, C. A., Pegion, K., Vecchi, G. A., Methot, R. D., Alexander, M. A., et al. (2017b). Improved management of small pelagic fisheries through seasonal climate prediction. Ecol. Appl. 27, 378–388. doi: 10.1002/eap.1458
Trathan, P. N., Forcada, J., and Murphy, E. J. (2007). Environmental forcing and Southern Ocean marine predator populations: effects of climate change and variability. Philos. T.R. Soc. B. 362, 2351–2365. doi: 10.1098/rstb.2006.1953
Walsh, H. J., Richardson, D. E., Marancik, K. E., and Hare, J. A. (2015). Long-Term Changes in the Distributions of Larval and Adult Fish in the Northeast US Shelf Ecosystem. PLoS ONE 10:e0137382. doi: 10.1371/journal.pone.0137382
Webber, B. L., Yates, C. J., Le Maitre, D. C., Scott, J. K., Kriticos, D. J., Ota, N., et al. (2011). Modelling horses for novel climate courses: insights from projecting potential distributions of native and alien Australian acacias with correlative and mechanistic models. Div. Distrib. 17, 978–1000. doi: 10.1111/j.1472-4642.2011.00811.x
Williams, J. W., Jackson, S. T., and Kutzbach, J. E. (2007). Projected distributions of novel and disappearing climates by 2100 AD. Proc. Natl. Acad. Sci. U.S.A. 104, 5738–5742. doi: 10.1073/pnas.0606292104
Winn, H., Price, C., and Sorensen, P. (1986). The Distributional Biology of the Right Whale (Eubalaena glacialis) in the Western North Atlantic. Report of the International Whaling Commission (special issue).
Xie, S. P., Deser, C., Vecchi, G. A., Collins, M., Delworth, T. L., Hall, A., et al. (2015). Towards predictive understanding of regional climate change. Nat. Clim. Change 5, 921–930. doi: 10.1038/nclimate2689
Appendix: Panel
Case Study 1. Modeling Cetacean Density in the Pacific Ocean
Predictive habitat-based density models were developed for 11 marine mammal species or species assemblages in the California Current Ecosystem, eastern tropical Pacific, and central Pacific Ocean (Figure A1). Models used a generalized additive modeling framework at ecosystem-dependent scales (2–120 km) and extensive line-transect survey data (e.g., Ferguson et al., 2006; Redfern et al., 2008; Forney et al., 2012; Becker et al., 2016). This approach has enabled comparisons of modeling framework effectiveness, evaluation of predictor variables at differing spatial and temporal resolutions, development of methods to characterize uncertainty in model predictions, and model validation (Barlow et al., 2009; Becker et al., 2010, 2014; Forney et al., 2015).
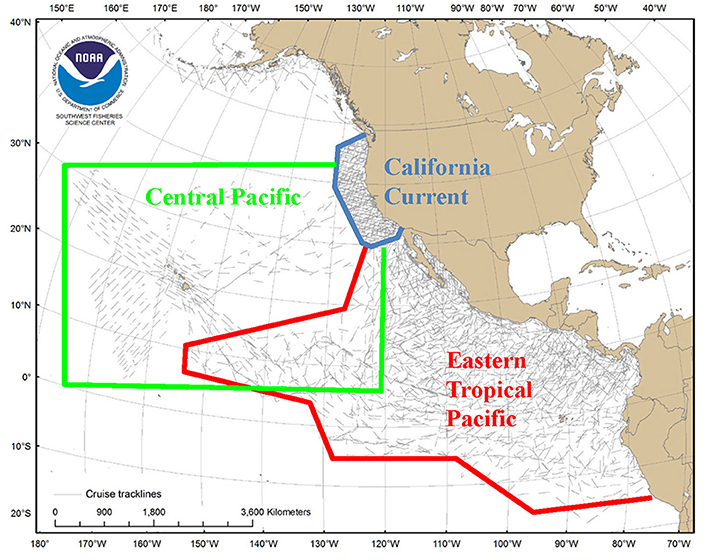
Figure A1. (Case study 1) Transect coverage for surveys conducted by SWFSC between 1986 and 2006 in three broad study areas in the eastern North Pacific. Modified from Hamilton et al. (2009).
Dynamic environmental covariates included sea surface temperature (SST), salinity (SSS) and height (SSH), chlorophyll concentration (CHL),thermocline depth and strength, and mixed layer depth (MLD) collected in situ during transect surveys and sensed remotely. In addition, dynamic variables (including SST, SSS, MLD, and SSH) from ocean models have been used as potential predictors in habitat models (Becker et al., 2016). Habitat predictors from ocean circulation models all served as proxies for unmeasured underlying ecological processes linking cetaceans to their prey, and they provide opportunities for dynamic predictions.
Models have successfully captured variability in cetacean density and distribution at seasonal and interannual time scales (e.g., Figure A2, Forney et al., 2012; Becker et al., 2014, 2016), but they only reflect historical data and the variation therein and do not take into account current or future conditions. Becker et al. (2012) demonstrated that advanced satellite data and forecasts from ocean models allow “nowcasts” of marine mammal distributions on time scales of days to weeks and forecasts on time scales of 3–4 months. Ocean circulation models provide robust predictive models of cetacean distributions (Becker et al., 2016), showing promise for future predictions of marine mammal distributions in a changing climate. However, these models rely on proxy variables, and future forecasts can fail if the proxy relationships change; likewise, modeling marine mammal distribution in one area may not be readily transferable (i.e., may perform poorly) to other locations. Future steps require additional model validation, particularly at different spatial resolutions and longer temporal scales.
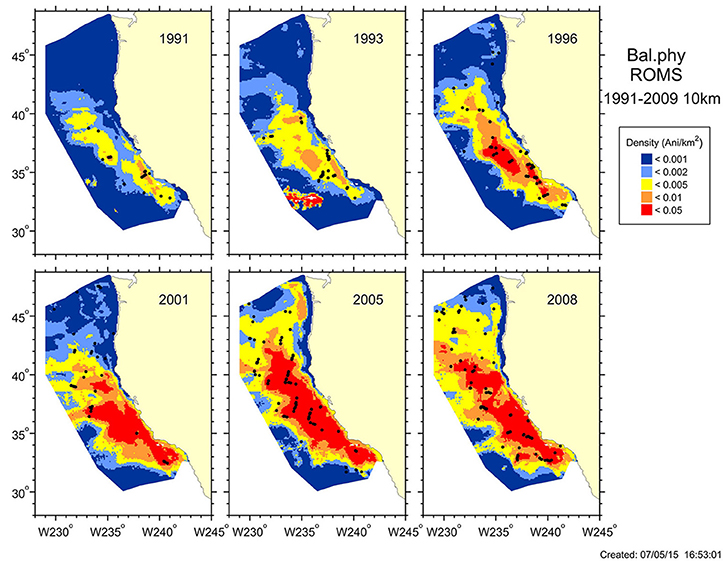
Figure A2. (Case study 1) Model-based estimates of fin whale summer/fall density (animals/km–2) and distribution for six different years, 1991–2009, in the California Current Ecosystem. Black dots show actual sighting locations during ship surveys conducted in each year. (Details provided in Becker et al., 2016).
Case Study 2. Projecting Ringed Seal Distributions
In the context of a biological review regarding the listing of the ringed seal as threatened or endangered under the ESA, ringed seal distributions were projected through the twenty-first century using a highly-simplified definition of habitat required by the species for the critical life history functions of whelping, nursing, and weaning pups. These functions occur in spring, a period when the pups are highly vulnerable to predation and hypothermia if there is insufficient snow cover for mothers to construct and maintain lairs on top of the ice. Studies of lair construction indicated that accumulated snow depths of at least 20 cm are required for drifts to form that are sufficiently deep (50–65 cm) for adequate birth lairs (NOAA, 2012). Therefore, ringed seal habitat and breeding distribution were assumed to be those areas of the Arctic where at least 20 cm of snow depth could be expected in the month of April. Output from global climate models predicts that although precipitation in the Arctic is expected to increase, much of it will fall as rain. Delayed autumn ice formation will mean that some of the snow that does fall will fall into open water rather than accumulating on the ice surface. Consequently, the area with snow depths above 20 cm in April was projected to decline under a broad range of plausible greenhouse gas emission scenarios, with the greatest decline up to 70% by the end of the twenty-first century under one emissions scenario (Hezel et al., 2012). This would result in a substantial loss of ringed seal reproductive habitat.
One strength of this approach is that it is built on climate projections that are, qualitatively at least, broadly agreed to represent the best available science and project robust large-scale trends in Arctic sea ice. A significant limitation of this method for ringed seal habitat projection is that the species' habitat has been reduced to just two dimensions (sea ice extent and snow accumulation), a drastic oversimplification. Another limitation is that regional and finer scale variability may not be adequately reflected in the coarse resolution of the global circulation models used for the climate projections (Stock et al., 2011).
Keywords: marine mammal distribution and abundance, climate-change, marine ecosystems, predicting and forecasting, Marine mammal conservation
Citation: Silber GK, Lettrich MD, Thomas PO, Baker JD, Baumgartner M, Becker EA, Boveng P, Dick DM, Fiechter J, Forcada J, Forney KA, Griffis RB, Hare JA, Hobday AJ, Howell D, Laidre KL, Mantua N, Quakenbush L, Santora JA, Stafford KM, Spencer P, Stock C, Sydeman W, Van Houtan K and Waples RS (2017) Projecting Marine Mammal Distribution in a Changing Climate. Front. Mar. Sci. 4:413. doi: 10.3389/fmars.2017.00413
Received: 06 December 2016; Accepted: 01 December 2017;
Published: 20 December 2017.
Edited by:
Gretchen E. Hofmann, University of California, Santa Barbara, United StatesReviewed by:
Edward Gregr, University of British Columbia, CanadaE. Christien Michael Parsons, George Mason University, United States
Copyright © 2017 Silber, Lettrich, Thomas, Baker, Baumgartner, Becker, Boveng, Dick, Fiechter, Forcada, Forney, Griffis, Hare, Hobday, Howell, Laidre, Mantua, Quakenbush, Santora, Stafford, Spencer, Stock, Sydeman, Van Houtan and Waples. This is an open-access article distributed under the terms of the Creative Commons Attribution License (CC BY). The use, distribution or reproduction in other forums is permitted, provided the original author(s) or licensor are credited and that the original publication in this journal is cited, in accordance with accepted academic practice. No use, distribution or reproduction is permitted which does not comply with these terms.
*Correspondence: Gregory K. Silber, Z3JlZ3NpbGJlcjJAZ21haWwuY29t
Matthew D. Lettrich, bWF0dGhldy5sZXR0cmljaEBub2FhLmdvdg==