- 1Department of Marine Sciences, University of the Aegean, Mytilene, Greece
- 2Institute for Applied Data Analytics, Universiti Brunei Darussalam, Bandar Seri Begawan, Brunei
Uncertainty can be considered as an attribute of (or reciprocally lack of) information (Zadeh, 2005). Nevertheless, this vital attribute is usually missing from marine invasion science studies (Figure 1), despite the fact that methods such as Monte Carlo simulations, sensitivity analysis, Bayesian uncertainty assessment, and Latin hypercube sampling appear more frequently in the ecological literature (e.g., Harwood and Stokes, 2003; Moustakas and Evans, 2015; Aiello-Lammens and Akçakaya, 2017). Most studies of marine invasions are plagued by uncertainties, which in most cases are totally ignored or if acknowledged are not properly addressed or quantified. Herein we provide some examples of uncertainties in invasion science, aiming to highlight the existing gaps and stress the need for the development and implementation of frameworks, methods and tools that will assist invasion biologists to adequately tackle uncertainty.
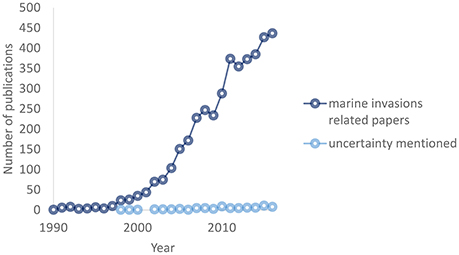
Figure 1. Time series of publications registered in Scopus, including in the title or abstract or keywords at least one the words “biological invasions” or “non-indigenous species” or “alien species” or “invasive species,” and at least one of the words “marine” or “sea” (dark blue markers). If in addition one of the words “uncertainty” or “confidence” is included (light blue markers) the number of publications drops to 2% of the initial number (91/4415).
Uncertainty in Alien Status
Although there are various definitions of alien species (Falk-Petersen et al., 2006), the most widely accepted one is that of species, which, by human agency, have managed to overcome physical barriers and colonize new regions beyond their natural range. In a rapidly changing world, it is often difficult to attribute a new arrival to a region to human activities. Species' natural ranges are inherently dynamic, shaped by natural variability, and thus sometimes it is impossible to determine the relative importance of natural and anthropogenic factors in distributional changes. Furthermore, assessing the alien or native status of rare species or of very old invasions is often impossible due to the lack of historical data. For example, shipping has been acting as an invasion pathway for many millennia making it difficult to evaluate the biogeographic status of species introduced to new marine regions hundreds or thousands years ago, some of which may have gradually become cosmopolitan. The term “cryptogenic” has been used by invasion biologists (Carlton, 1996) to indicate species that could be either native or alien but for which uncertainty is high. Assessing the native/alien status of species is important both for ecological science and management, hence a standardized framework with robust definitions, transparent criteria for classification and proper uncertainty assessment is urgently needed.
Uncertainty in Alien Species Inventories
National or regional inventories of alien species are a valuable tool for both invasion science and management, especially for prioritizing pathways, developing prevention or control strategies, and assessing (through trend analyses) of the effectiveness of management measures. However, alien species inventories suffer from a number of uncertainties e.g. in species identification (taxonomic uncertainty), incomprehensive search of data sources, low spatial resolution, poor documentation of data and knowledge, and inadequate native range information (McGeoch et al., 2012). This has resulted in regular revisions of such inventories, excluding species previously included (e.g., Zenetos et al., 2017), and even in scientific disputes among research groups (e.g., Galil, 2009, 2012; Zenetos, 2010; Zenetos et al., 2017). To avoid misuse of inventories, misunderstandings, mistrust and unnecessary conflicts, proper account of the level of confidence and inherent uncertainties should be included in all species inventories. The uncertainty in inventories is likely to increase with the use of citizen science (ElQadi et al., 2017) as well as with mining (geo-referenced) social media (Daume, 2016). While these techniques are likely to increase the total amount of data availability, the inclusion of non-experts in the process of data collection as well as the automated way of classification is likely to increase uncertainty as well as to introduce noise and spurious correlations in the dataset (Moustakas, 2017). In addition, as more datasets become publicly accessible it is likely that datasets collected under different protocols or for different purposes may be merged together and thus introduce additive uncertainties (Evans and Moustakas, 2016).
Uncertainty in Pathway Assessment
Assessment of the pathways of introduction of alien species (i.e., any means that allows the entry or spread of an alien species into a new region) is fundamental to biological invasion risk assessments, management of invasive species, monitoring and surveillance of new introductions (Essl et al., 2015). However, assigning each marine alien introduction to a specific pathway is subject to substantial uncertainty (Zenetos et al., 2012). Apart from intentional introductions, in most other cases more than one possible pathway can be inferred based on the human activities in or near the locality of first record. However, such inferences are largely based on expert judgement and suffer from lack of evidence. For example, the invasive crab Percnon gibbesi was recorded for the first time in the Mediterranean Sea in 1999 in many different locations, and its introduction has been attributed to a number of different possible pathways by different authors: shipping, aquarium trade or larval drift by the Atlantic surface current entering the Mediterranean (see (Katsanevakis et al., 2011) and references therein); in the latter case the species should not be considered as an alien, because its introduction was not mediated by humans but happened through natural processes. Many other possible pathways that are commonly neglected or understudied, e.g., aquarium trade (Padilla and Williams, 2004; Strecker et al., 2011) or marine litter (Barnes, 2002), could have had much higher importance than currently acknowledged. It is vital that such uncertainties in pathway assessments are transparent by providing an estimate of the related confidence and highlight possibilities for alternative pathways (Zenetos et al., 2012; Essl et al., 2015).
Uncertainty in Impact and Risk Assessments
Impact assessments depend on uncertain data such as the actual distribution of alien species, the vulnerability of ecosystems and their services, heterogeneity of biophysical processes, and spatiotemporal variability in the magnitude of impacts. Assessments of cumulative impacts of many invasive species to marine ecosystems suffer from additional uncertainties related to insufficient data, sensitivity weights, type of responses of ecosystems to invasive species, type of multiple species effects (additive or with synergistic or antagonistic interactions), and resolution of spatial data (Katsanevakis et al., 2016). Such uncertainties can substantially affect impact assessments and jeopardize their quality and consequently their value for management. Important gaps exist in properly addressing uncertainty in impact assessments, which, if at all, are restricted in qualitative accounts based largely on expert judgement (Blackburn et al., 2014). The International Pest Risk Mapping Workgroup has identified “improving representations of uncertainty” among the most important actions to improve pest risk assessment procedures (Venette et al., 2010). Research effort is needed to further develop proper tools that will allow addressing uncertainty in impact and risk assessments in an adequate and transparent way.
Uncertainty in Field Surveys
Monitoring of marine aliens species is based on field surveys, commonly conducted by visual surveys through SCUBA diving or the use of sampling and fishing gears. One important source of error in field surveys of marine species is imperfect detectability or imperfect catchability, i.e., the fact that not all individuals or all target species in a study area can be detected by observers (divers) or caught by sampling devices or fishing gears (Katsanevakis et al., 2012). Although many methods have been developed that properly account for imperfect detectability/catchability, such as distance sampling, mark-recapture, repeated presence-absence surveys for occupancy estimation (Issaris et al., 2012; Katsanevakis et al., 2012), the number of marine field surveys that apply such methods remains very limited. Failure to properly account for detectability leads to underestimation of the population state variable (e.g., abundance, population density, or occupancy), or even total failure to detect an alien species and thus underestimation of alien species richness in community studies. This is further intensified by the inability to properly identify alien species in situ in visual surveys. To improve the performance of field surveys and reduce uncertainties it is imperative to select an adequate method properly accounting for detectability, and apply field protocols and tools (sampling devices, photos, video) that will reduce identification uncertainties.
Uncertainty in Distribution Modeling
Species distribution models (SDM) have been extensively used to predict the potential present or future distribution of marine alien species, based on a limited set of observations and a set of environmental variables that presumably describe their niches (Peterson, 2003). Uncertainty arising due to the applied modeling technique, spatial resolutions, scales, data availability, climate change and subsequent biological responses, model selection and evaluation methods can be substantially high (Beale and Lennon, 2012). SDMs are based in the (silent) presupposition that there exists information in the species distribution that can be used for estimating the species' niche, though there is no certainty that all niche axes have limits within the spatial extent of the dataset (Beale and Lennon, 2012). In addition, a critical form of uncertainty in SDMs is identifying the dimensions of explanatory variables (Beale et al., 2010); omitting informative variables produces poor model predictions while including uninformative variables may result in correlations with informative variables and thus result in reduced parameter estimation accuracy (Beale et al., 2010). Improved assessment of errors and uncertainties is among the prevailing challenges facing SDM research (Guisan and Thuiller, 2005).
Concluding Remarks
Quantifying or at least acknowledging uncertainty is an elementary exercise in science, as without uncertainty assessment it is hard to envisage future improvements (see e.g., Benali et al., 2017). In addition, biological invasions and their impacts is a very timely topic receiving high public attention. If scientists are perceived by the public either to overstate their findings in order to receive high visibility or to downplay the uncertainty of their findings, society is likely to lose confidence in the outputs of invasion science.
It is evident from the above non-exhaustive list that invasion science is challenged by various sources of uncertainty. Such uncertainties, if ignored, render many types of analyses and results doubtful and of limited practical use to policy makers and marine managers. To further advance invasion science in the marine environment we need (1) to acknowledge the need for appropriate assessments of uncertainty in all aspects of biological invasions research, (2) put research effort in improving/developing methods and tools for uncertainty analyses, (3) report uncertainties and their effects on research outcomes in all scientific outputs and technical reports.
Author Contributions
All authors listed have made a substantial, direct and intellectual contribution to the work, and approved it for publication.
Conflict of Interest Statement
The authors declare that the research was conducted in the absence of any commercial or financial relationships that could be construed as a potential conflict of interest.
References
Aiello-Lammens, M. E., and Akçakaya, H. R. (2017). Using global sensitivity analysis of demographic models for ecological impact assessment. Conserv. Biol. 31, 116–125. doi: 10.1111/cobi.12726
Barnes, D. K. A. (2002). Invasions by marine life on plastic debris. Nature 416, 808–809. doi: 10.1038/416808a
Beale, C. M., and Lennon, J. J. (2012). Incorporating uncertainty in predictive species distribution modelling. Philos. T. Roy. Soc. B 367, 247–258. doi: 10.1098/rstb.2011.0178
Beale, C. M., Lennon, J. J., Yearsley, J. M., Brewer, M. J., and Elston, D. A. (2010). Regression analysis of spatial data. Ecol. Lett. 13, 246–264. doi: 10.1111/j.1461-0248.2009.01422.x
Benali, A., Sa, A. C. L., Ervilha, A. R., Trigo, R. M., Fernandes, P. M., and Pereira, J. M. C. (2017). Fire spread predictions: sweeping uncertainty under the rug. Sci. Total Environ. 592, 187–196. doi: 10.1016/j.scitotenv.2017.03.106
Blackburn, T. M., Essl, F., Evans, T., Hulme, P. E., Jeschke, J. M., Kühn, I., et al. (2014). A unified classification of alien species based on the magnitude of their environmental impacts. PLoS Biol. 12:e1001850. doi: 10.1371/journal.pbio.1001850
Carlton, J. T. (1996). Biological invasions and cryptogenic species. Ecology 77, 1653–1655. doi: 10.2307/2265767
Daume, S. (2016). Mining Twitter to monitor invasive alien species — An analytical framework and sample information topologies. Ecol. Inform. 31, 70–82. doi: 10.1016/j.ecoinf.2015.11.014
ElQadi, M. M., Dorin, A., Dyer, A., Burd, M., Bukovac, Z., and Shrestha, M. (2017). Mapping species distributions with social media geo-tagged images: case studies of bees and flowering plants in Australia. Ecol. Inform. 39, 23–31. doi: 10.1016/j.ecoinf.2017.02.006
Essl, F., Bacher, S., Blackburn, T., Booy, O., Brundu, G., Brunel, S., et al. (2015). Crossing frontiers in tackling pathways of biological invasions. BioScience 65, 769–782. doi: 10.1093/biosci/biv082
Evans, M. R., and Moustakas, A. (2016). A comparison between data requirements and availability for calibrating predictive ecological models for lowland UK woodlands: learning new tricks from old trees. Ecol. Evol. 6, 4812–4822. doi: 10.1002/ece3.2217
Falk-Petersen, J., Bohn, T., and Sandlund, O. T. (2006). On the numerous concepts in invasion biology. Biol. Invasions 8, 1409–1424. doi: 10.1007/s10530-005-0710-6
Galil, B. S. (2009). Taking stock: inventory of alien species in the Mediterranean Sea. Biol. Invasions 11, 359–372. doi: 10.1007/s10530-008-9253-y
Galil, B. S. (2012). Truth and consequences: the bioinvasion of the Mediterranean Sea. Integr. Zool. 7, 299–311. doi: 10.1111/j.1749-4877.2012.00307.x
Guisan, A., and Thuiller, W. (2005). Predicting species distribution: offering more than simple habitat models. Ecol. Lett. 8, 993–1009. doi: 10.1111/j.1461-0248.2005.00792.x
Harwood, J., and Stokes, K. (2003). Coping with uncertainty in ecological advice: lessons from fisheries. Trends Ecol. Evol. 18, 617–622. doi: 10.1016/j.tree.2003.08.001
Issaris, Y., Katsanevakis, S., Salomidi, M., Tsiamis, K., Katsiaras, N., and Verriopoulos, G. (2012). Occupancy estimation of marine demersal species: dealing with imperfect detectability. Mar. Ecol. Prog. Ser. 453, 95–106. doi: 10.3354/meps09668
Katsanevakis, S., Poursanidis, D., Yokes, B., Mačić, V., Beqiraj, S., Kashta, L., et al. (2011). Twelve years after the first report of the crab Percnon gibbesi (H. Milne Edwards, 1853) in the Mediterranean: current distribution and invasion rates. J. Biol. Res. 16, 224–236.
Katsanevakis, S., Tempera, F., and Teixeira, H. (2016). Mapping the impact of alien species on marine ecosystems: the Mediterranean Sea case study. Divers. Distrib. 22, 694–707. doi: 10.1111/ddi.12429
Katsanevakis, S., Weber, A., Pipitone, C., Leopold, M., Cronin, M., Scheidat, M., et al. (2012). Monitoring marine populations and communities: review of methods and tools dealing with imperfect detectability. Aquat. Biol. 16, 31–52. doi: 10.3354/ab00426
McGeoch, M. A., Spear, D., Kleynhans, E. J., and Marais, E. (2012). Uncertainty in invasive species listing. Ecol. Appl. 22, 959–971. doi: 10.1890/11-1252.1
Moustakas, A. (2017). Spatio-temporal data mining in ecological and veterinary epidemiology. Stoch. Environ. Res. Risk Assess. 31, 829–834. doi: 10.1007/s00477-016-1374-8
Moustakas, A., and Evans, M. (2015). Coupling models of cattle and farms with models of badgers for predicting the dynamics of bovine tuberculosis (TB). Stoch. Environ. Res. Risk Assess. 29, 623–635. doi: 10.1007/s00477-014-1016-y
Padilla, D. K., and Williams, S. L. (2004). Beyond ballast water: aquarium and ornamental trades as sources of invasive species in aquatic ecosystems. Front. Ecol. Environ. 2, 131–138. doi: 10.1890/1540-9295(2004)002[0131:BBWAAO]2.0.CO;2
Peterson, A. T. (2003). Predicting the geography of species' invasions via ecological niche modeling. Q. Rev. Biol. 78, 419–433. doi: 10.1086/378926
Strecker, A. L., Campbell, P. M., and Olden, J. D. (2011). The aquarium trade as an invasion pathway in the Pacific Northwest. Fisheries 36, 74–85. doi: 10.1577/03632415.2011.10389070
Venette, R. C., Kriticos, D. J., Magarey, R., Koch, F., Baker, R. H. A., Worner, S. P., et al. (2010). Pest risk maps for invasive alien species: a roadmap for improvement. Bioscience 80, 349–362. doi: 10.1525/bio.2010.60.5.5
Zadeh, L. A. (2005). Toward a generalized theory of uncertainty (GTU)—an outline. Inform. Sci. 172, 1–40. doi: 10.1016/j.ins.2005.01.017
Zenetos, A. (2010). Trend in aliens species in the Mediterranean. An answer to Galil, 2009 ≪Taking stock: inventory of alien species in the Mediterranean Sea≫. Biol. Invasions 12, 3379–3381. doi: 10.1007/s10530-009-9679-x
Zenetos, A., Gofas, S., Morri, C., Rosso, A., Violanti, D., et al. (2012). Alien species in the Mediterranean Sea by 2012. A contribution to the application of European Union's Marine Strategy Framework Directive (MSFD). Part 2. Introduction trends and pathways. Medit. Mar. Sci. 13, 328–352. doi: 10.12681/mms.327
Keywords: alien species, invasive species, marine environment, uncertainty analysis, biological invasions
Citation: Katsanevakis S and Moustakas A (2018) Uncertainty in Marine Invasion Science. Front. Mar. Sci. 5:38. doi: 10.3389/fmars.2018.00038
Received: 28 September 2017; Accepted: 25 January 2018;
Published: 09 February 2018.
Edited by:
Juergen Pilz, Alpen-Adria-Universität Klagenfurt, AustriaReviewed by:
Laura Uusitalo, Finnish Environment Institute (SYKE), FinlandCopyright © 2018 Katsanevakis and Moustakas. This is an open-access article distributed under the terms of the Creative Commons Attribution License (CC BY). The use, distribution or reproduction in other forums is permitted, provided the original author(s) and the copyright owner are credited and that the original publication in this journal is cited, in accordance with accepted academic practice. No use, distribution or reproduction is permitted which does not comply with these terms.
*Correspondence: Stelios Katsanevakis, c3RlbGlvc0BrYXRzYW5ldmFraXMuY29t