- 1Istituto Nazionale di Oceanografia e di Geofisica Sperimentale, Trieste, Italy
- 2Stazione Zoologica Anton Dohrn Napoli, Naples, Italy
- 3National Institute of Aquatic Resources, Technical University of Denmark, Charlottenlund, Denmark
- 4Hellenic Centre for Marine Research, Institute of Marine Biological Resources and Inland Waters, Heraklion, Greece
- 5National Oceanography Centre, Southampton, United Kingdom
- 6MARUM, Center for Marine Environmental Sciences, University of Bremen, Bremen, Germany
In the field of ocean observing, the term of “observatory” is often used without a unique meaning. A clear and unified definition of observatory is needed in order to facilitate the communication in a multidisciplinary community, to capitalize on future technological innovations and to support the observatory design based on societal needs. In this paper, we present a general framework to define the next generation Marine OBservatory (MOB), its capabilities and functionalities in an operational context. The MOB consists of four interconnected components or “gears” (observation infrastructure, cyberinfrastructure, support capacity, and knowledge generation engine) that are constantly and adaptively interacting with each other. Therefore, a MOB is a complex infrastructure focused on a specific geographic area with the primary scope to generate knowledge via data synthesis and thereby addressing scientific, societal, or economic challenges. Long-term sustainability is a key MOB feature that should be guaranteed through an appropriate governance. MOBs should be open to innovations and good practices to reduce operational costs and to allow their development in quality and quantity. A deeper biological understanding of the marine ecosystem should be reached with the proliferation of MOBs, thus contributing to effective conservation of ecosystems and management of human activities in the oceans. We provide an actionable model for the upgrade and development of sustained marine observatories producing knowledge to support science-based economic and societal decisions.
Introduction
Until now, due to the technological limitations, our observing capacity has been governed to a large extent by the principle we “observe what we can" rather than “we observe what we need." As a result, the Global Ocean Observing System defined the Essential Ocean Variables (EOVs, Lindstrom et al., 2012) to prioritize parameters for new technological developments. The technology involved with acquiring, transmitting, and disseminating marine data is advancing at fast pace. Experts in the marine community need to sustain this momentum by testing, calibrating, and standardizing new sensors measuring EOVs. At the same time, we must learn to handle the ever-increasing volume of data and knowledge. Conversely, the available data and knowledge remain insufficient to provide an adequate response to the requirements of environmental legislation (e.g., Crise et al., 2015) and ocean governance (Fritz, 2016). All this has been reflected in the G7 Science Ministers’ Turin Communiqué (G7 Future of the Seas and Oceans Working Group, 2017) that recommendedthe continuation of existing observations that are augmented by new technologies in an integrated, coordinated, and consistent way. Furthermore, the UN has recently proclaimed a Decade of Ocean Science (UN General Assembly, 2015). All the above requires a revised approach to ocean observing.
The traditional ways to collect repeated in situ ocean observations in a limited geographical area can be grouped in two categories:
(1) Manual Observations: Observations carried out by marine biological/zoological stations, for instance now part of Long-Term Ecological Research (LTER) and Group on Earth Observations Biodiversity Observation Network (GEO BON) or through repeated cruise observations (e.g., Hawaii Ocean Time-series, Bermuda Atlantic Time-series Study, DYnamique des Flux Atmosphériques en MEDiterranée);
(2) Autonomous Observations: Automated stations with limited physical/biogeochemical capabilities [(e.g., OceanSITES, European Multidisciplinary Seafloor and water column Observatory (EMSO)].
These traditional observing strategies, often vessel-dependent, have over time been progressively enriched with a complementary observing infrastructure based on networks of automated, real-time sensors and platforms. The new marine infrastructure is becoming attractive because of its benefits, in the form of improved cost-efficiencies, deployment, and operational flexibility, to marine science and to science-based ocean management (Tintoré et al., 2013). Conversely it still requires large sustained funding plans and dedicated personnel. In most cases autonomous observations will not replace traditional observations, and therefore the additional costs required must be justified to regional and international funding bodies in terms of the value added (Witze, 2013).
The term “observatory,” although of common use in astronomical research, appeared for the first time in the marine sector in Okada (1921). Since then, “observatory” has been combined with different adjectives, describing the location [coastal (Goff et al., 2005), deep-sea (Soltwedel et al., 2005), sea floor (Favali and Beranzoli, 2006), borehole (Suyehiro et al., 2002)], the predominant scientific focus [ecological (Strandell et al., 2007), ecosystem (Schofield et al., 2002), sea-ice (Druckenmiller et al., 2009)], the mode [operational, (Cruzado et al., 2010), integrated (Oliver et al., 2013)], and the data transmission [cabled, (Barnes et al., 2008)]. The North Atlantic Observatory (e.g., Chafik et al., 2014) is comprised of repeated observations collected by a Greenland supply vessel.
To help reconcile these different views this paper will clarify the concept of Marine OBservatory (MOB) while identifying functionalities of and perspectives for the next generation of MOBs. A definition of such a framework is deemed relevant for three good reasons:
• To create a common background for strategy and vision documents and reduce possible misunderstanding caused by diverse uses of the term in heterogeneous communities;
• To identify present functionalities and potential future technological developments of marine observatories;
• To facilitate the dialog among economic, societal, and scientific stakeholders on the services of marine observatories.
A Novel Definition of Marine Observatory (Mob)
The tautological definition of observatory as “A building or place given over to or equipped for observation of natural phenomena” (Merriam-Webster Dictionary, 2002) underpins the central role of the observation concept, which is not synonymous with experimentation and monitoring. We believe these three profoundly different activities should be combined into a novel definition of “Observatory.” Observations should detect the expected and unexpected events with adequate temporal and spatial resolution. This implies that the adopted measurement and/or sampling strategy should be flexible enough to adapt to the specific site variability. Unlike observing, monitoring has the primary aim to characterize and detect change in the state and quality of the marine environment to fulfill regulatory/statutory obligations. Monitoring may require action such as alert and warning according to predefined criteria. Finally, experiments take place in a controlled environment with verifiable protocols and include an action of known intensity to measure the reaction. The sampling strategy and the use of acquired data are critical elements to determine if you are “observing,” “monitoring” or “experimenting.”
Here, we propose the following definition of MOB: An integrated observing, monitoring, and experimenting infrastructure which aims to collect high-resolution data in a restricted geographical region. This region spans surface, water column, seafloor, and/or sub-seafloor. MOB is linked to shore by a wireless or cable connection in real or near-real time supported by samples and other data collected in a delayed mode. A MOB is composed of three “hard gears” namely observation infrastructure, cyberinfrastructure, support capacity and one “soft gear” knowledge generation engine (Figure 1). Such a MOB can be integrated in larger networks and in Ocean Observing Systems from regional to global scale collecting high-resolution data.
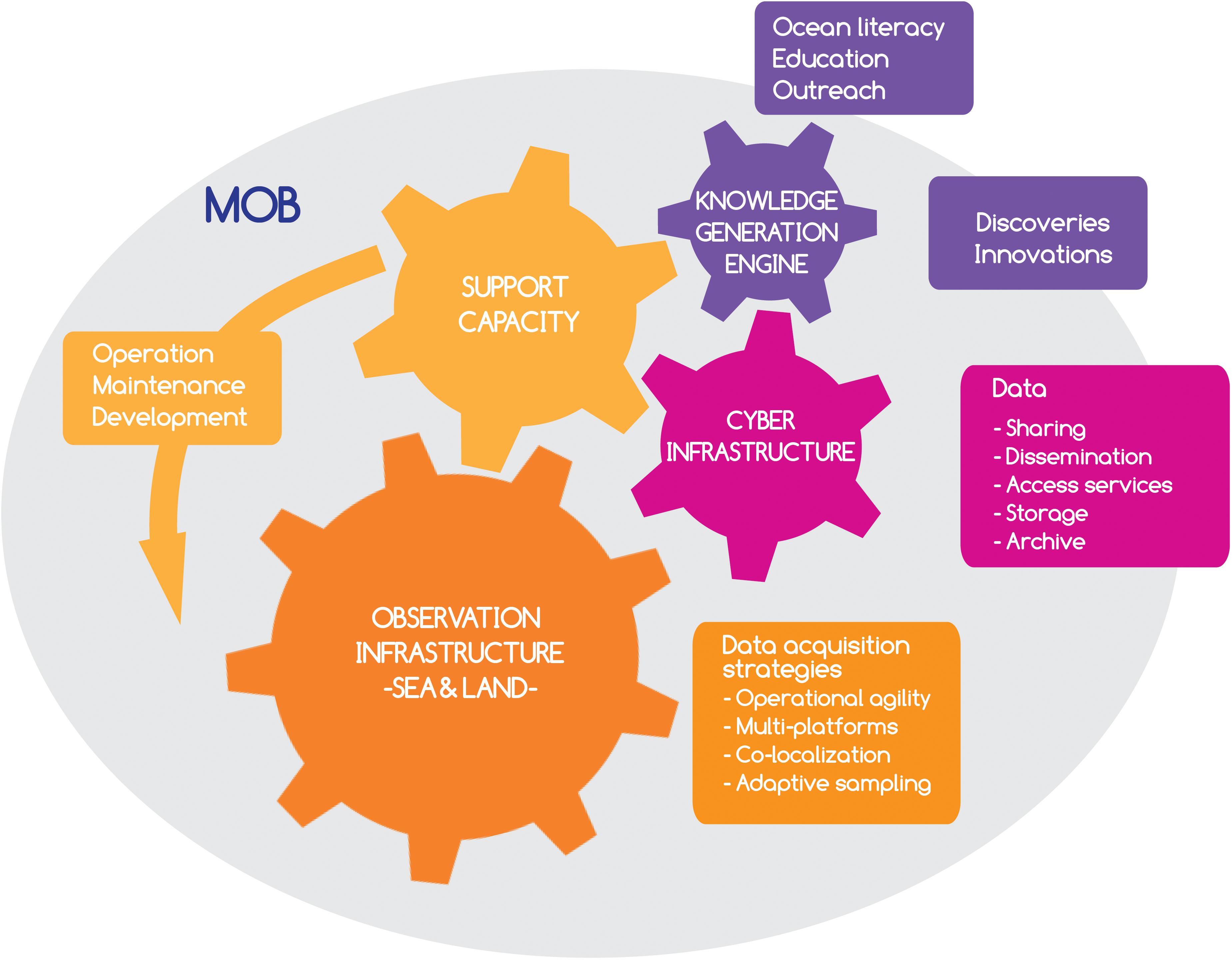
FIGURE 1. The next generation Marine OBservatory structure (MOB). The oval represents MOB with its four fundamental components: three hard gears (Observation infrastructure, Cyberinfrastructure, and human Support capacity) and one soft gear (Knowledge generation engine). Color-coded boxes represent functionalities and capabilities of the associated gears.
At sea, the observation infrastructure refers to a long-term in situ infrastructure operating in a predefined site composed of a number of automated platforms that provide also metadata and operational status. Each platform (e.g., buoy) is a vehicle used to carry a specified set of instruments (e.g., CTD). It also provides infrastructure for instrument power, data storage, and telemetry. Each instrument consists of a set of heterogeneous sensors (e.g., for measuring sea temperature and conductivity) enabling the characterization of the state and evolution of biological, chemical, and physical properties. This structural definition is fully consistent with the functional definitions based on Sensor Web Enablement standards applied to the marine sector (Bermudez et al., 2009). Research vessels and/or additional autonomous platforms (e.g., gliders, AUVs), when operating in the MOB area, are considered to be an integral part of the MOB observation infrastructure. In brief, the observation infrastructure integrates lagrangian and eulerian, remote and direct, transdisciplinary observations collected within the MOB site and timely delivered. The activities of the in situ component are supported on land by specialized laboratories and hardware infrastructures for data analysis, maintenance activities and technological updates. A MOB is thus able to generate a variety of real-time and delayed mode quantitative and qualitative data. The observation infrastructure is, therefore, intimately linked to the cyberinfrastructure that is the secure information and communication system dedicated to (open) data sharing, provision of data to global dissemination systems, data access services (e.g., Global Earth Observation System of Systems), metadata provision, data and metadata submission to the appropriate storage and archive systems.
The MOB human support capacities are essential to operate, maintain and develop both the above infrastructures. Furthermore, MOB staff conduct laboratory analyses (e.g., plankton analyses by microscopy or sequencing), data processing (e.g., quality control), and sensor calibration. Last but not least, MOB staff duties include outreach and education promoting ocean literacy for society.
The MOB knowledge generation engine refers to the research team activities that transforms data into information and knowledge both producing discoveries that can generate innovation as well as providing fundamental baselines to understand ocean change. This gear incorporates, when available, complementary knowledge inputs from other sources such as satellite observations, operational marine services (e.g., Copernicus Marine Environment Monitoring Service) and citizen science initiatives.
Recommendations for the Design of Next Generation MOB
The following features would advance MOB technical capabilities and functionalities in a cohesive direction:
• Co-localization and synopticity of observations: multivariate time series should be collected synchronously in the same site or limited region. Observation design can accommodate monitoring and experimenting;
• Multi-platform, adaptive sampling strategy: Intelligent sampling strategies, by changing sampling rates and/or sampling scales or by integrating autonomous mobile platforms such as gliders and AUVs will complement the strength of permanent observing infrastructure. Where possible, spatial coherence of observed parameters will be refined by broader scale satellite observations in the region;
• Enhanced quality assurance and quality control procedures based on advanced methods (i.e., multivariate statistics and/or machine learning algorithms) exploiting the information contained in multiple/multivariate observations and carrying on regular self-assessment of performance;
• Progressive inclusion in the MOB of the automated observations of Biology and Ecosystem EOVs as previously prioritized (Miloslavich et al., 2018);
• Continuous maintenance and development of the infrastructures, including calibration and intercalibration among new and old as well as different sensors and platforms to maintain an internal coherence in the data series over time;
• Open, secure, free and timely data access, complying to international standards and formats; the data provided by MOBs can be (re)used for multiple purposes (e.g., scientific purposes, monitoring, alerting, marine environment management, education) by adopting the Sensor Web Enablement paradigm;
• Access to infrastructure through open call scheme for developing scientific collaborations, testing new technologies coupled with industry and promoting public engagement.
The evolution of the existing observatories calls for integration within a network of MOBs thus extending the impact from local to global scale. The MOBs will enrich the broad picture provided by the Global Ocean Observing System by providing high-resolution observations of fine-scale processes available with a detail not achievable yet at global scale and by acting as pilots for the implementation of innovative technologies (e.g., Biological and Ecosystem EOVs).
Scope of the Next Generation MOB
The long-term impact of scientific evidence provided by MOBs is expected to contribute to science, society, and economy (Figure 2; Ruhl et al., 2011). MOB contribution will generate information and knowledge from data, as conceptualized in the hierarchy of understanding paradigm (Carpenter and Cannady, 2004). The transformation from data to knowledge is essential to engage non-technical end users that generally are not able to use raw data. It is important to implement in the MOB some feedback mechanisms between support technicians and the scientists team (and vice versa) to keep the MOB functioning and efficient. Beyond the MOB, the external users will leverage the knowledge derived from the MOB data to make better informed decisions and guidelines, and to change or enhance operations and policies. Their feedback will influence the MOB knowledge flow and its management, but also help to improve and innovate the core elements. We expect that MOBs should contribute to:
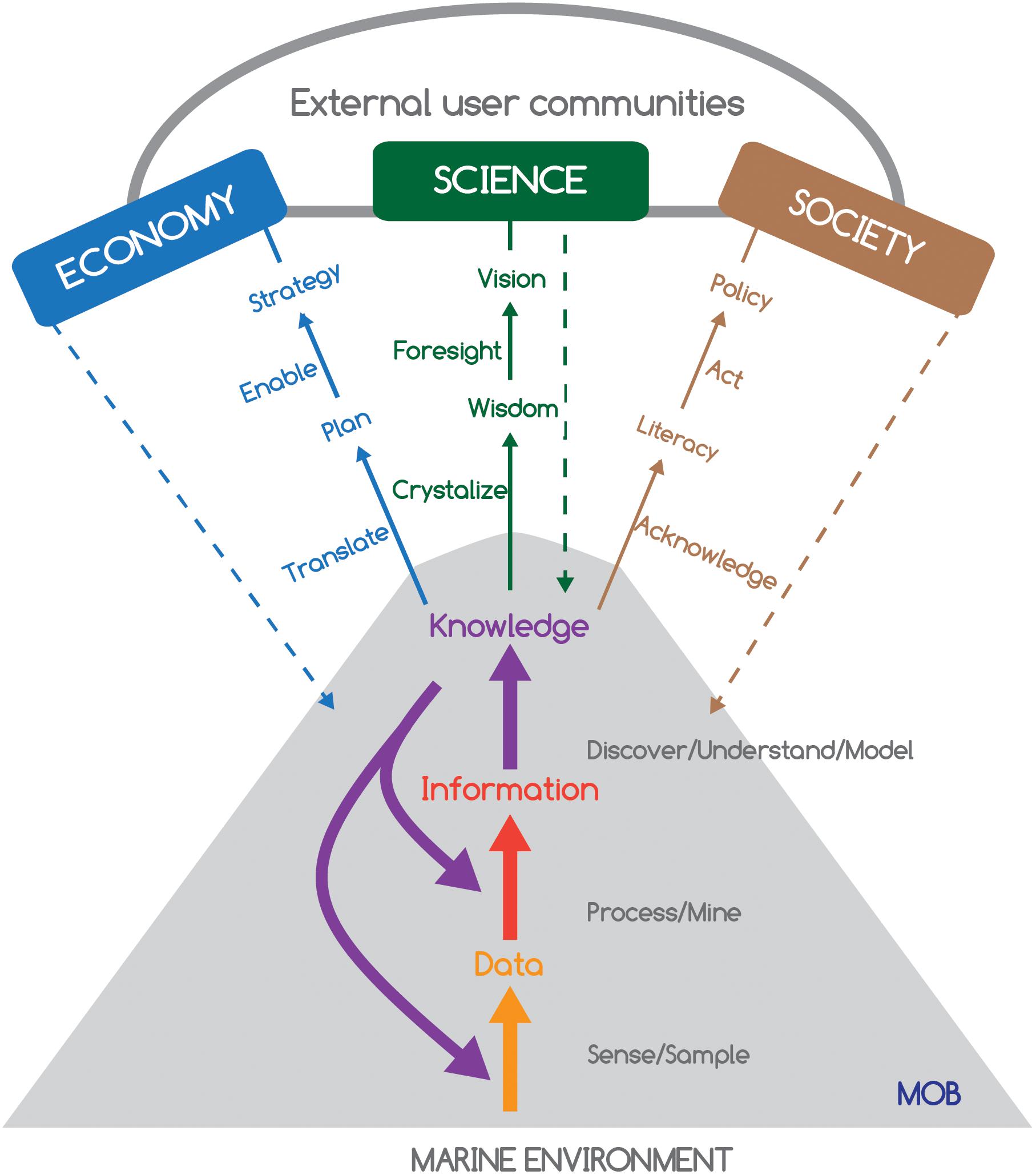
FIGURE 2. MOB-based hierarchy of understanding for science, society, and economy. The MOB generate knowledge by sensing the environment thus creating data that are then processed to become information that is distilled into knowledge. From MOB, user-specific pathways will elaborate and translate knowledge to the external user communities (Economy, Science, and Society). The triangle is the MOB domain, the arrows are processes that link one level of understanding to another, dashed arrows are the feedbacks from the external user communities to the MOB and thick arrows are feedbacks within the MOB. The hierarchy of understanding is modified from Carpenter and Cannady (2004).
Science
• Help to detect climate change impacts on marine ecosystems, enabling differentiation between natural and anthropogenic changes;
• Observe the dynamics of biological communities and their habitats;
• Advance the understanding of the marine ecosystem functioning in a holistic way.
Society
• Provide early warning systems for geohazards, microbial toxin production or other conditions of concern for human welfare;
• Evaluate indicators and/or emerging properties as proxies for the healthy state of the marine environment;
• Ascertain the recovery or degradation of a marine environment by monitoring changes in stressors;
• Contribute to capacity development and international collaboration;
• Encourage public awareness of, and engagement in marine science taking advantage of innovative communication tools, fora and models of interactions.
Economy
• Provide timely and qualified data to operational (re)analysis and forecast services;
• Propel the technological development of marine equipment by providing expertise in requirements, testing and co-design of new instruments;
• Support a permanent demand of high standard marine technologies;
• Engage new players moving across and beyond the traditional marine and maritime sectors (e.g., digital innovation start-ups) with a non-traditional approach.
MOB Sustainability, Dialog With Society and Governance
Unlike terrestrial or atmospheric observatories, the harsh environment of the sea, the higher cost of the equipment, and the remote location often prove to be significant obstacles for sustained observations. To secure funds and political endorsement thus ensuring the long-term operational MOB endurance, we recommend (1) to establish an effective business model that secures a long-term financial support; (2) to capitalize on the infrastructure to fulfill environmental, security, economic, and societal needs connecting scientific community and the societal beneficiaries; (3) to develop international cooperation; (4) to serve end users by providing qualified open data, products and services to support decision making policies; (5) to maintain high standards in scientific studies by connecting and being involved in high priority research programs such as ocean acidification and carbon storage.
A sustained MOB will facilitate the dialog among economic, societal, and scientific stakeholders about what MOB products and services can address. Concrete actions will call for (1) regular meetings with founders, government agencies and stakeholders to inform about MOB capabilities and to receive inputs to better accommodate MOB end user needs, (2) interface with federal or state-based environmental protection plans and activities, (3) share equipment and knowledge within international initiative such as the European Marine Biological Resource Centre and Life-Watch, (4) promote education and science outreach activities in agreement with public authorities, (5) convey the message that MOBs are a key asset to be included in environmental projects. Governance is essential to ensure a sustained MOB vision. An appropriate governance scheme should deal with the financial matters, adopt policies and procedures to sustain MOB operations and developments and make a positive impact of MOB at local/national scale and provide strategic oversight in agreement with the regional and international context. We acknowledge that an effective governance scheme is a challenge given its inherent complexity but we must strive for a constant interactive dialog among the MOB staff, external user communities and governance bodies. To facilitate such a dialog, a possible solution is to include in the MOB advisory board members elected by each territory, and by end user representing the driving economies and the public sector.
Future Directions in Mobs
Innovations in observation infrastructure and cyberinfrastructure will transform the MOBs within the next 20 years. The next generation MOBs will cover a wider range of phenomena with more observations at higher frequency in time and space with reduced operational costs per datum generated. These advancements will make MOBs more convenient and appealing for far-reaching user communities since they adapt and respond to societal and economical challenges. Real-time communication among different platforms and the ability to modify and adapt the observing plan as a response to a sudden change in the environment will be a desirable characteristic for many infrastructures. A critical challenge will be to fully incorporate in a seamless way the new sensors, platforms and techniques (e.g., -omics1 derived) into the existing MOBs both in terms of operation as well as data, information and knowledge delivery. It follows that managing large amount of different data will be a key factor since we are moving from an era of data shortage to one of data adequacy. This data bonanza will allow us to explain much more complex and multifaceted processes (Atkinson et al., 2013). In addition, a vast amount of data produced to serve societal, business or military needs (e.g., monitoring programs, geophysical surveys) is becoming available. This untapped resource should be capitalized and incorporated into the MOB historical/baseline knowledge. The native integration of sensors and platforms in the cyberinfrastructure will require adherence to the Sensor Web Enablement paradigm (Sheth et al., 2008; Pearlman et al., 2016) to ensure data access and interoperability. Furthermore, the cyberinfrastructure will provide a standard interface to the archiving repositories system able to use architecture and artificial intelligence powers to exploit such a vast amount of data. Modelers will be more involved from the early stage of the MOB by supporting the strategic planning and design with targeted Observing Systems Simulation Experiments, during information and knowledge production and throughout the fundamental analyses and the studies of processes and phenomena.
Specifically, we foresee a significant increase in biological observations. Biological observations are necessary to help understand the functioning of marine ecosystems, as well as to determine patterns and trends to support the responsible use of ocean’s resources. Only with sustained biological observations will it be possible to understand our changing oceans and to implement flexible management strategies that will adapt to evolving scenarios. Recent efforts in the identification and prioritization of EOVs for biodiversity and ecosystem observations (Miloslavich et al., 2018) using optical, acoustic, and -omics methodologies have provided the framework necessary to direct advancements to revolutionize our ability to understand ecosystem change. Innovative -omics for assessing marine environmental status (Danovaro et al., 2016) and ocean health via the ocean microbiome (Buttigieg et al., 2018), harmonized and standardized techniques (Field et al., 2008; Kopf et al., 2015) and the modeling approach linking genomics to ecosystems (Stec et al., 2017) demonstrate the feasibility for the implementation of the biological component of MOBs (Davies et al., 2012). Miniaturization (e.g., lab-on-a-chip, (Fukuba et al., 2011), multiplexing (e.g., high-throughput technologies), robotization [e.g., ecogenomic sensors (Scholin, 2013)] improvements in in situ energy harvesting and efficiency will increase the agility and user-friendliness for MOB technologies. Such advancements will allow the extension of MOBs to the open ocean, with an immediate impact on knowledge, management, and marine spatial planning of the global ocean.
Acoustic and optical sensors and animal tagging within observatories have shown to provide real-time monitoring solutions for coastal fisheries and aquaculture (Scholin et al., 2009; Campbell et al., 2010, 2013; Brosnahan et al., 2014), while providing datasets of adequate longevity to evaluate changes in phenology due to climate change (Hunter-Cevera et al., 2016). The next generation MOBs will combine in an unprecedented way -omics with the above technologies to shed light on behavioral processes critical for ecosystem functioning across biological scales.
We envision that collectively MOBs will meet mission-oriented goals within the priority areas identified by society such as ocean-climate influence on humans, ocean and human health, marine ecosystem preservation and sustainable exploitation of marine resources. Such direct societal and economic benefits will act as leverage to boost MOBs development as results of national and international endeavors, facilitating their sustainability and better positioning their vision in the agendas of diverse funding and governance bodies in support of the endangered global ocean.
Author Contributions
AC led the design and development of the manuscript. FM helped design, contributed, and coordinated the paper. JR contributed to the development of the manuscript and provided feedback focused on the Perspective. GP, PM, RB, MRd’A, and DI contributed to the development of the manuscript and provided feedbacks throughout the manuscript.
Funding
AC, MRd’A, and DI were partially supported by RITMARE Flagship Project (CIPE Resolution 2/2011 of 23.03.2011) funded by Italian Ministry of Education and Research. JR was supported by NERC NE/N006496/1 (MARINe-DNA) and Horizon2020 Project ID 633211 (AtlantOS) projects. GP was supported by HORIZON2020 Project ID 654410 (JERICO-NEXT) and ID 731036 (EMSO-LINK) projects. RB was supported by the Werner Siemens-Foundation through the Centre of Innovation for Deep Sea Environmental Monitoring at MARUM at the University of Bremen. PM was partially supported by DTU SENTINEL program and the European Ocean and Fishery Fund (KYSTFISK3, 33113-B-16).
Conflict of Interest Statement
The authors declare that the research was conducted in the absence of any commercial or financial relationships that could be construed as a potential conflict of interest.
Acknowledgments
The authors wish to thank Ms. Valentina Mosetti for the art work and colleagues of NEMO project (2016 MIUR Italian Competitive Research-Progetti premiali) for useful discussions. The authors are particularly indebted with Dr. Henry Ruhl for his precious advice and suggestions.
Footnotes
- ^This neologism informally refers to genomics, proteomics, transcriptomics, metabolomics.
References
Atkinson, M., Baxter, R., Brezany, P., Corcho, O., Galea, M., Parsons, M. (eds). et al. (2013). The Data Bonanza: Improving Knowledge Discovery in Science, Engineering, and Business, Vol. 90. Hoboken, NJ: John Wiley & Sons. doi: 10.1002/9781118540343
Barnes, C. R., Best, M. M., and Zielinski, A. (2008). The Neptune Canada regional cabled ocean observatory. Technology 49, 10–14.
Bermudez, L., Delory, E., O’Reilly, T., and del Rio Fernandez, J. (2009). “Ocean observing systems demystified,” in Proceedings of the OCEANS 2009, MTS/IEEE Biloxi-Marine Technology for Our Future: Global and Local Challenges, (Piscataway, NJ: IEEE).
Brosnahan, M. L., Farzan, S., Keafer, B. A., Sosik, H. M., Olson, R. J., and Anderson, D. M. (2014). Complexities of bloom dynamics in the toxic dinoflagellate Alexandrium fundyense revealed through DNA measurements by imaging flow cytometry coupled with species-specific rRNA probes. Deep Sea Res. Part II 103, 185–198. doi: 10.1016/j.dsr2.2013.05.034
Buttigieg, P. L., Fadeev, E., Bienhold, C., Hehemann, L., Offre, P., and Boetius, A. (2018). Marine microbes in 4D—using time series observation to assess the dynamics of the ocean microbiome and its links to ocean health. Curr. Opin. Microbiol. 43, 169–185. doi: 10.1016/j.mib.2018.01.015
Campbell, L., Henrichs, D. W., Olson, R. J., and Sosik, H. M. (2013). Continuous automated imaging-in-flow cytometry for detection and early warning of Karenia brevis blooms in the Gulf of Mexico. Environ. Sci. Pollut. Res. 20, 6896–6902. doi: 10.1007/s11356-012-1437-4
Campbell, L., Olson, R. J., Sosik, H. M., Abraham, A., Henrichs, D. W., Hyatt, C. J., et al. (2010). First harmful Dinophysis (Dinophyceae, Dinophysiales) bloom in the US is revealed by automated imaging flow cytometry. J. Phycol. 46, 66–75. doi: 10.1111/j.1529-8817.2009.00791.x
Carpenter, S., and Cannady, J. (2004). “Tool for sharing and assessing models of fusion-based space transportation systems,” in Proccedings of the 40th AIAA/ASME/SAE/ASEE Joint Propulsion Conference and Exhibit, New York, NY. doi: 10.2514/6.2004-3535
Chafik, L., Rossby, T., and Schrum, C. (2014). On the spatial structure and temporal variability of poleward transport between Scotland and Greenland. J. Geophys. Res. Oceans 119, 824–841. doi: 10.1002/2013JC009287
Crise, A., Kaberi, H., Ruiz, J., Zatsepin, A., Arashkevich, E., Giani, M., et al. (2015). A MSFD complementary approach for the assessment of pressures, knowledge and data gaps in Southern European Seas: the perseus experience. Mar. Pollut. Bull. 95, 28–39. doi: 10.1016/j.marpolbul.2015.03.024
Cruzado, A., Bahamón Rivera, N., Bernadello, R., Ahumada, M. A., Donis, D., and Cardoso, G. (2010). Operational Observatory of the Catalan Sea (OOCS). Instrumen. Viewp. 8, 75–75. doi: 10.3390/s111211251
Danovaro, R., Carugati, L., Berzano, M., Cahill, A. E., Carvalho, S., Chenuil, A., et al. (2016). Implementing and innovating marine monitoring approaches for assessing marine environmental status. Front. Mar. Sci. 3:213. doi: 10.3389/fmars.2016.00213
Davies, N., Meyer, C., Gilbert, J. A., Amaral-Zettler, L., Deck, J., Bicak, M., et al. (2012). A call for an international network of Genomic Observatories (GOs). Gigascience 1:5. doi: 10.1186/2047-217X-1-5
Druckenmiller, M. L., Eicken, H., Johnson, M. A., Pringle, D. J., and Williams, C. C. (2009). Toward an integrated coastal sea-ice observatory: system components and a case study at Barrow, Alaska. Cold Reg. Sci. Technol. 56, 61–72. doi: 10.1016/j.coldregions.2008.12.003
Favali, P., and Beranzoli, L. (2006). Seafloor observatory science: a review. Ann. Geophys. 49, 515–567.
Field, D., Garrity, G., Gray, T., Morrison, N., Selengut, J., Sterk, P., et al. (2008). The Minimum Information about a Genome Sequence (MIGS) specification. Nat. Biotechnol. 26, 541–547. doi: 10.1038/nbt1360
Fukuba, T., Miyaji, A., Okamoto, T., Yamamoto, T., Kaneda, S., and Fujii, T. (2011). Integrated in situ genetic analyzer for microbiology in extreme environments. RSC Adv. 1, 1567–1573. doi: 10.1039/c1ra00490e
G7 Future of the Seas and Oceans Working Group (2017). Progress Since May 2016 Executive Summary, September, page 5. Available at: http://www.g8.utoronto.ca/science/2017-annex1-seas-oceans.html
Goff, J. A., Mayer, L. A., Traykovski, P., Buynevich, I., Wilkens, R., Raymond, R., et al. (2005). Detailed investigation of sorted bedforms, or “rippled scour depressions,” within the Martha’s vineyard coastal observatory. Cont. Shelf Res. 25, 461–484. doi: 10.1016/j.csr.2004.09.019
Hunter-Cevera, K. R., Neubert, M. G., Olson, R. J., Solow, A. R., Shalapyonok, A., and Sosik, H. M. (2016). Physiological and ecological drivers of early spring blooms of a coastal phytoplankter. Science 354, 326–329. doi: 10.1126/science.aaf8536
Kopf, A., Bicak, M., Kottmann, R., Schnetzer, J., Kostadinov, I., Lehmann, K., et al. (2015). The ocean sampling day consortium. Gigascience 4:27. doi: 10.1186/s13742-015-0066-5
Lindstrom, E., Gunn, J., Fischer, A., McCurdy, A., Glover, L. K., Alverson, K., et al. (2012). “A framework for ocean observing,” in Proceedings of the Ocean Information for Society: Sustaining the Benefits, Realizing the Potential, Venice. doi: 10.5270/OceanObs09-FOO
Merriam-Webster Dictionary (2002). Merriam-Webster Dictionary. Available at: https://www.merriam-webster.com/dictionary/observatory
Miloslavich, P., Bax, N. J., Simmons, S. E., Klein, E., Appeltans, W., Aburto-Oropeza, O., et al. (2018). Essential ocean variables for global sustained observations of biodiversity and ecosystem changes. Glob. Change Biol. 24, 2416–2433. doi: 10.1111/gcb.14108
Okada, T. (1921). Imperial marine observatory at kobe, Japan. J. Geophys. Res. 26, 25–25. doi: 10.1029/TE026i001p00025-01
Oliver, M. J., Breece, M. W., Fox, D. A., Haulsee, D. E., Kohut, J. T., Manderson, J., et al. (2013). Shrinking the haystack: using an AUV in an integrated ocean observatory to map Atlantic Sturgeon in the coastal ocean. Fisheries 38,210–216. doi: 10.1080/03632415.2013.782861
Pearlman, J., Jirka, S., del Rio, J., Delory, E., Frommhold, L., Martinez, S., et al. (2016). “Oceans of Tomorrow sensor interoperability for in-situ ocean monitoring,” in Proceedings of the OCEANS 2016 MTS/IEEE Monterey, (Piscataway, NJ: IEEE). doi: 10.1109/OCEANS.2016.7761404
Ruhl, H. A., André, M., Beranzoli, L., Çağatay, M. N., Colaço, A., Cannat, M., et al. (2011). Societal need for improved understanding of climate change, anthropogenic impacts, and geo-hazard warning drive development of ocean observatories in European Seas. Prog. Oceanogr. 91, 1–33. doi: 10.1016/j.pocean.2011.05.001
Schofield, O., Bergmann, T., Bissett, P., Grassle, J. F., Haidvogel, D. B., Kohut, J., et al. (2002). The long-term ecosystem observatory: an integrated coastal observatory. IEEE J. Ocean. Eng. 27, 146–154. doi: 10.1038/sdata.2015.8
Scholin, C., Doucette, G., Jensen, S., Roman, B., Pargett, D., Marin, R., et al. (2009). Remote detection of marine microbes, small invertebrates, harmful algae and biotoxins using the environmental sample processor (ESP). Oceanography 22, 158–167. doi: 10.5670/oceanog.2009.46
Scholin, C. A. (2013). “Ecogenomic sensors,” in Encyclopedia of Biodiversity, 2nd Edn, Vol. 2, ed. S. A. Levin (Waltham, MA: Academic Press), 690–700. doi: 10.1016/B978-0-12-384719-5.00408-1
Sheth, A., Henson, C., and Sahoo, S. S. (2008). Semantic sensor web. IEEE Internet Comput. 12, 78–83. doi: 10.1109/MIC.2008.87
Soltwedel, T., Bauerfeind, E., Bergmann, M., Budaeva, N., Hoste, E., Jaeckisch, N., et al. (2005). Hausgarten: multidisciplinary investigations at a deep-sea, long-term observatory in the Arctic Ocean. Oceanography 18, 47–61. doi: 10.5670/oceanog.2005.24
Stec, K. F., Caputi, L., Buttigieg, P. L., D’Alelio, D., Ibarbalz, F. M., Sullivan, M. B., et al. (2017). Modelling plankton ecosystems in the meta-omics era. Are we ready? Mar. Genomics 32, 1–17. doi: 10.1016/j.margen.2017.02.006
Strandell, E., Tilak, S., Chou, H. M., Wang, Y. T., Lin, F. P., Arzberger, P., et al. (2007). “Data management at Kenting’s underwater ecological observatory,” in Proceedings of the 3rd International Conference on Intelligent Sensors, Sensor Networks and Information, (Piscataway, NJ: IEEE). doi: 10.1109/ISSNIP.2007.4496931
Suyehiro, K., Araki, E., Shinohara, M., and Kanazawa, T. (2002). Deep sea borehole observatories ready and capturing seismic waves in the western Pacific. Eos. Trans. Am. Geophys. Union 83, 621–625. doi: 10.1029/2002EO000420
Tintoré, J., Vizoso, G., Casas, B., Heslop, E., Pascual, A., Orfila, A., et al. (2013). SOCIB: the Balearic Islands coastal ocean observing and forecasting system responding to science, technology and society needs. Mar. Technol. Soc. J. 47, 101–117. doi: 10.4031/MTSJ.47.1.10
UN General Assembly (2015). Resolution Adopted by the General Assembly on 25 September 2015 n.70/1 Transforming our World: the 2030 Agenda for Sustainable Development. Available at: http://www.un.org/ga/search/view_doc.asp?symbol=A/RES/70/1&Lang=E
Keywords: Marine OBservatory, ocean observing, cyberinfrastructure, long-term sustainability, Essential Ocean Variables, Global Ocean Observing System
Citation: Crise A, Ribera d’Alcalà M, Mariani P, Petihakis G, Robidart J, Iudicone D, Bachmayer R and Malfatti F (2018) A Conceptual Framework for Developing the Next Generation of Marine OBservatories (MOBs) for Science and Society. Front. Mar. Sci. 5:318. doi: 10.3389/fmars.2018.00318
Received: 13 April 2018; Accepted: 21 August 2018;
Published: 07 September 2018.
Edited by:
Juliet Hermes, South African Environmental Observation Network, South AfricaReviewed by:
Thomas George Bornman, Nelson Mandela Metropolitan University, South AfricaNaomi Greenwood, Centre for Environment, Fisheries and Aquaculture Science (CEFAS), United Kingdom
Copyright © 2018 Crise, Ribera d’Alcalà, Mariani, Petihakis, Robidart, Iudicone, Bachmayer and Malfatti. This is an open-access article distributed under the terms of the Creative Commons Attribution License (CC BY). The use, distribution or reproduction in other forums is permitted, provided the original author(s) and the copyright owner(s) are credited and that the original publication in this journal is cited, in accordance with accepted academic practice. No use, distribution or reproduction is permitted which does not comply with these terms.
*Correspondence: Alessandro Crise, acrise@inogs.it