- 1Marine Information Resources Corporation, Ellicott City, MD, United States
- 2Southeastern Universities Research Association, Washington, DC, United States
- 3Australian Institute of Marine Science, Townsville, QLD, Australia
- 4Social Science Research Center, Mississippi State University, Starkville, MS, United States
- 5LETG UMR CNRS UBO 6554, Université de Bretagne Occidentale, Plouzané, France
- 6Virginia Institute of Marine Science, Gloucester Point, VA, United States
- 7LGO UMR CNRS UBO 6538, Université de Bretagne Occidentale, Plouzané, France
- 8Cerema, Eau, Mer et Fleuves, Margny-lès-Compiègne, France
- 9Taylor Engineering Research Institute, University of North Florida, Jacksonville, FL, United States
- 10Florida Institute of Technology, Melbourne, FL, United States
Impacts from natural and anthropogenic coastal hazards are substantial and increasing significantly with climate change. Coasts and coastal communities are increasingly at risk. In addition to short-term events, long-term changes, including rising sea levels, increasing storm intensity, and consequent severe compound flooding events are degrading coastal ecosystems and threatening coastal dwellers. Consequently, people living near the coast require environmental intelligence in the form of reliable short-term and long-term predictions in order to anticipate, prepare for, adapt to, resist, and recover from hazards. Risk-informed decision making is crucial, but for the resulting information to be actionable, it must be effectively and promptly communicated to planners, decision makers and emergency managers in readily understood terms and formats. The information, critical to forecasts of extreme weather and flooding, as well as long-term projections of future risks, must involve synergistic interplay between observations and models. In addition to serving data for assimilation into models, the observations are also essential for objective validation of models via hind casts. Linked observing and modeling programs that involve stakeholder input and integrate engineering, environmental, and community vulnerability are needed to evaluate conditions prior to and following severe storm events, to update baselines, and to plan for future changes over the long term. In contrast to most deep-sea phenomena, coastal vulnerabilities are locally and regionally specific and prioritization of the most important observational data and model predictions must rely heavily on input from local and regional communities and decision makers. Innovative technologies and nature-based solutions are already helping to reduce vulnerability from coastal hazards in some localities but more focus on local circumstances, as opposed to global solutions, is needed. Agile and spatially distributed response capabilities will assist operational organizations in predicting, preparing for and mitigating potential community-wide disasters. This white paper outlines the rationale, synthesizes recent literature and summarizes some data-driven approaches to coastal resilience.
Introduction and Goals of This White Paper
As pointed out by Wright and Nichols (2019): “A high priority vision for future coastal science should be to enhance resilience of coastal communities by anticipating and mitigating hazards to human health, safety and welfare and reducing economic harm to coastal industries such as tourism, fisheries and shipping.” Accomplishing this objective will require anticipating future hazards to human and ecosystem health, safety and welfare. To adapt to accelerating coastal change, we must predict future conditions with increasing accuracy and precision, providing improved predictions on global, regional, and local spatial scales and on decadal, annual, and event time scales. Meeting future challenges requires collaborations across the broadest range of disciplines and coastal landscapes to integrate and assess observational data and model output. This will require a trans-institutional collaborative environment and active engagement with local communities and NGO’s, especially those in resource poor regions. Effective prediction of conditions at a specific coastal site requires a hierarchy of coupled multi-disciplinary models in combination with spatially distributed observations to provide predictions downscaled from the global to the local level. Improved knowledge of natural and social processes and their interactions will result in the development of actionable information to reduce coastal vulnerability. Because of the diversity and complexity of coasts and coastal communities, observing and predicting strategies must be tailored to local circumstances and driven in large measure by input from local stakeholders and policy makers.
The primary goal of this white paper is to motivate development and outline the possible functions of a virtual coastal environment within which a cyber-connected network of stakeholders and scientists from different disciplines can interconnect and share needs, insights, observational data, model codes, model output, objective evaluations of models and forecasts and, ultimately, communicate results to diverse end users including local communities. Some specific objectives related to OceanObs’19 are to advocate for greatly expanded collaboration, outline some of needs for the future and offer a summary review of recent literature related to coastal resilience. Global collaborations must include academic, government, and industry colleagues supported by multi-agency investments and international partnerships. Local and regional collaborations must also involve community stakeholders and policy makers. To become an integral part of future operational systems, the virtual environment must rely on linked observation and modeling systems capable of meeting both existing and expected future critical needs for coastal resilience. We must address today’s coastal problems within an integrated framework that complements existing operational systems and assists in identifying future modeling requirements. In this white paper, we offer a brief synthesis of some diverse perspectives and strategies recognizing that solutions to coastal threats must be found at local and regional levels. There is no “one size fits all” global model for coastal resilience.
Observing and Predicting to Enable Resilience
According to a National Academies report on disaster resilience (National Academies, 2012): “Resilience is the ability to prepare and plan for, absorb, recover from, and more successfully adapt to adverse events.” This point was reiterated in a more recent National Academies report on community resilience focused on the Gulf of Mexico coast (National Academies of Sciences, Engineering, and Medicine, 2019). Resilience involves the ability to adapt to changing environmental, economic, and social stressors. It does not imply constancy. It is the capacity to change and adapt while retaining effective viability. There are other definitions for coastal resilience, but we suggest one that focuses on enabling a community or environment to “bounce back” after a hazardous event such as a hurricane that causes flooding or to progressively adapt to long-term environmental changes in hazards and risks. In many cases, adaptation may involve adjusting infrastructure, relocating housing, or altering socio-economic behavior. Of paramount importance is the notion of preparing for natural hazards (Wright et al., 2016).
Numerous authors have described how physical, biological, and anthropogenic processes shape and impact the coast (Carter, 1988; Komar and McDougal, 1988; Paskoff, 1993, 1998; Camfield and Morang, 1996; Doornkamp, 1998; Bernatchez and Fraser, 2012; Ranasinghe and Jongejan, 2018). The European Eurosion project has shown that human influence, particularly urbanization, in the coastal zone has increasingly exacerbated coastal erosion (Salman et al., 2004). Some other examples are reviewed in a recent book on future coastal change (Wright and Nichols, 2019). The overall sensitivity of a coast to physical and biological forces determines the impacts of extreme conditions and human activities. The ability of a coast to adjust to different conditions is one component of resilience. The ensuing land-use planning considerations can increase or decrease the level of resilience depending on how well informed the decision makers are. Spatially distributed observations, on land and in the coastal ocean and nearshore are essential to adaptive coastal management. These observations need to account for the locally specific coastal dynamics and hazards as well as the different stakeholders, management and societal considerations. Rates of recovery of coastal realms following extreme events vary dramatically depending on local configuration and built infrastructure. Equilibrium responses to anthropogenic impacts can require several decades. Plans must take account of environmental factors, locally available resources, costs and benefits, and the most urgent needs of the local communities.
Risk depends on the combination of hazards, impacts, and vulnerabilities. A knowledge of impacts, such as coastal erosion and flooding, is needed at a range of time scales. This is especially important since the rates and demographics of coastal urbanization have shown significant temporal variability, and this has seriously challenged coastal management. Only through the integration of nearshore monitoring, modeling and adaptive management can plans be effectively implemented to increase resilience by relocating high-value assets and vulnerable people to less threatened areas or by redistributing resources needed for flood or erosion mitigation. The strategies required to resettle, or otherwise protect, threatened, or displaced communities and help them adapt to changed or new environments is a major challenge for governments, particularly in regions such as the Bay of Bengal (Wright, 2019).
To be effective and trusted, models and data must involve objective testing to ensure accuracy and coupling across multiple disciplines. An essential element of effective future coastal forecasting systems, in addition to observations and models, must be advanced data management, dissemination and visualization systems that enable modelers to readily access observational data for assimilation and objective model validation. Forecasting systems should provide planners, emergency managers, and the general public with clear and readily understandable information to assess impending threats. Some of this is now technically possible by rapidly evolving high-performance computing technology and the Internet of Things (IoT), which connects an ever-growing number of smart devices for real time monitoring and tracking of many physical events.
Rationale and Identification of the Need
Systemic vulnerability is a key multidimensional concept in developing strategies for long-term coastal management. Based on an interdisciplinary approach to risk, one may assess “the fragility of a system as a whole” (d’Ercole and Pigeon, 1999). Vulnerability characterizes a society (or individual) subject to risks related to situational factors (e.g., hazards) and structural factors determined by the socio-economic, cultural, functional, and institutional context of a place and time. As illustrated in Figure 1, systemic vulnerability generally has four major components (Meur-Férec, 2008; Hénaff and Philippe, 2014): hazards; stakeholder involvement (people and property exposed to hazards); management (public policies of prevention and crisis management, defense works, and infrastructure); and representation (Meur-Férec et al., 2003–2004; Meur-Férec and Morel, 2004; Meur-Férec, 2006). Coastal observatories that apply a systemic approach to evaluating and monitoring the four components of vulnerability will be able to provide stakeholders with additional tools and methodologies to adapt to coastal risks. Enhanced long-term nearshore observations of physical and social processes will improve societies’ understanding of and adaptation to high-impact, low-frequency natural hazards and climate change.
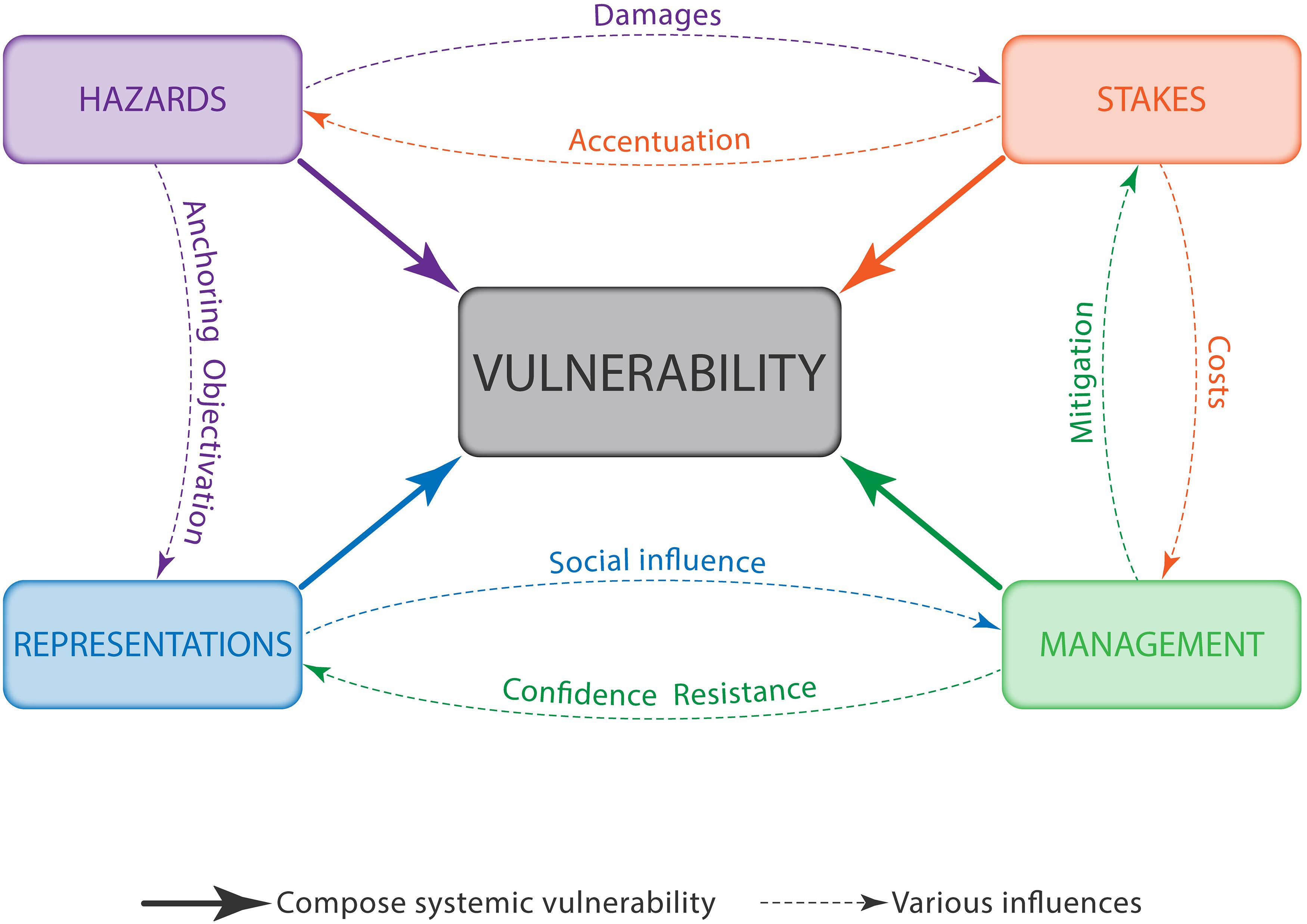
Figure 1. Factors contributing to and interacting with vulnerability (Michel-Guillou and Meur-Féréc, 2017).
Assessment of the reliability and accuracy of predictive models requires continuous objective monitoring of what is actually happening and how those “happenings” impact resilience. Models, sensor arrays, data management systems, communication protocols, and collaboration strategies should be considered together and not “stove piped” into isolated subsystems. Similarly, the large pool of local community resources, such as citizen science projects, should be fully utilized to ensure representation in model systems of areas that may lack the resources to collect the required baseline and event data. There is an immediate need for increased observations of processes during extreme storms when waves, flooding, sediment transport, and morphological changes are large. Citizen scientists, whether members of the general public or dedicated volunteers, can contribute essential data in data sparse regions.
Sensors and models alone do not address the underlying issue of damaging long-term changes and extreme events acting on coastal communities of varying vulnerabilities. The nature and severity of the vulnerabilities of coastal communities must be assessed before the risks can be evaluated. In this context, observing systems and complementary models provide essential tools for problem-solving. Fully integrated physical and social data sets will help operators to test and improve coastal process models that can be applied to improve resilience. However, research is needed to investigate the statistical issues in synthesizing environmental and social science data that are collected at various locations, frequencies, and accuracies.
Predicting Socio-Economic Vulnerabilities and Inundation Threats to Urban Coasts
Vulnerability Assessments
Worldwide there are 23 megacities with populations of over 10 million people. Of these, 16 are in the coastal zone (Blackburn and Pelling, 2014; Pelling and Blackburn, 2014). Hallegatte et al. (2013) and Dawson (2017) describe the dire plight of the growing number of people living in flood prone coastal cities and urban slums. Wright (2019) reviews recent literature on societal factors and changes that can impact community resilience including income, age and health, minority status, housing, and psychology. Some examples include works by Gunderson and Holling (2002), Norris et al. (2008), Cutter et al. (2010, 2014), and Van Zandt et al. (2012) who have evolved the concept of Baseline Resilience Indicators for Communities as empirical metrics for gaging the resilience of communities to disasters. Guillard-Gonçalves et al. (2014) developed a regionally specific “Social Vulnerability Index.” Flanagan et al. (2011) developed a social vulnerability index for disaster management that considers 15 different factors obtained from census data. Existing cyber tools for quickly assessing the social vulnerability of coastal urban communities to rising water levels are the “Surging Seas” risk zone maps produced by Climate Central. Figure 2 shows an example for New York City, NY, and Newark, NJ. Comprehensive, distributed data on elevations are crucial to accurate assessments of inundation probabilities associated with rising sea levels as well as event-scale storm surges and compound flooding (Gesch, 2018). It must be pointed out that the most vulnerable communities are not in affluent Western nations but in developing countries that have limited resources to protect or recover from disasters. Within the near future as many as 2 billion people worldwide could be in dire jeopardy. A very urgent challenge for international scientific organizations to find ways to make necessary observing, modeling and data dissemination technologies accessible to severely impoverished and threatened communities such as those that surround the Bay of Bengal (e.g., India, Bangladesh, Myanmar).
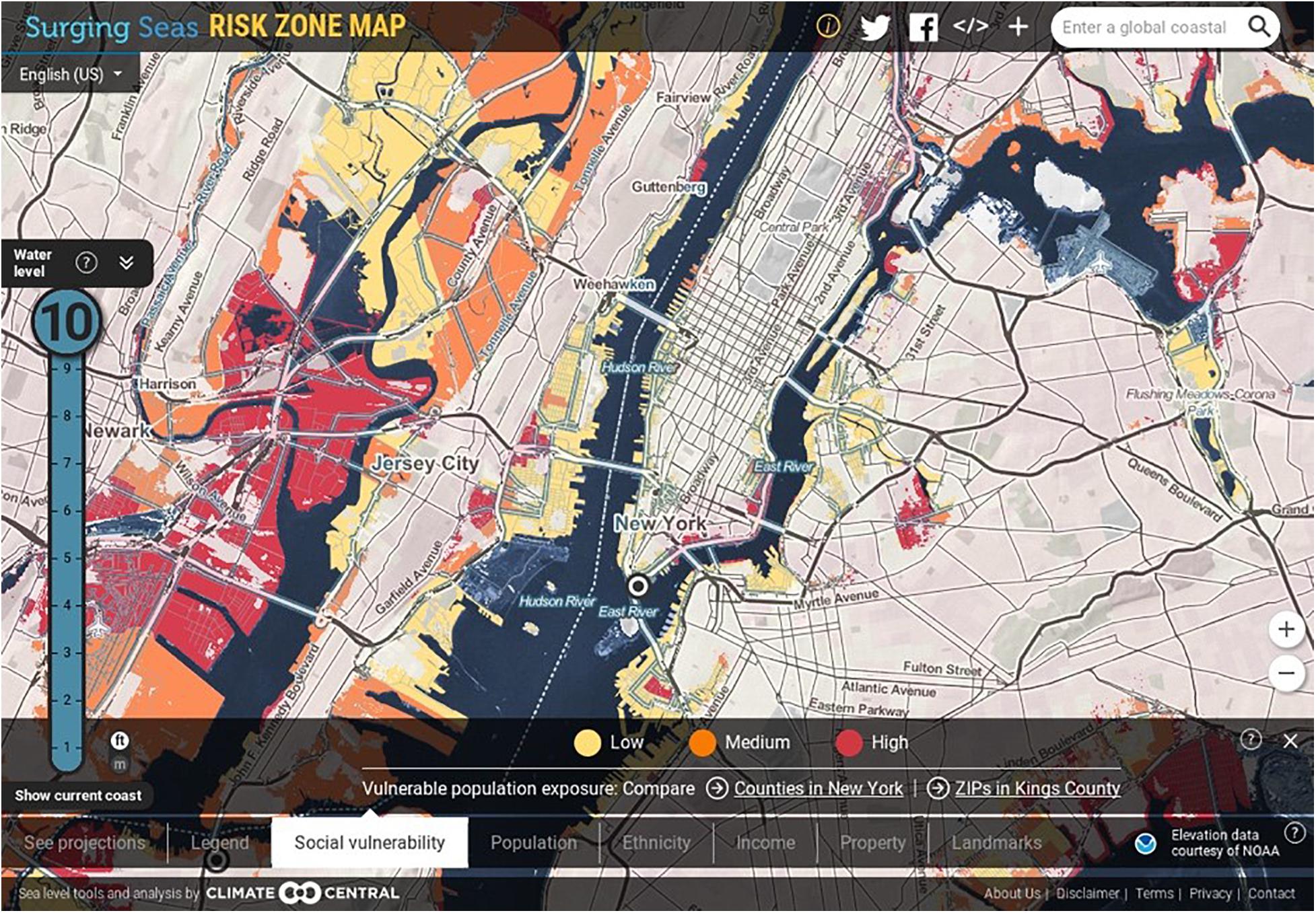
Figure 2. Predicted social vulnerability of communities centered around New York City and Newark, New Jersey to a 10-foot storm surge or sea level rise. From “Surging Seas” risk zone maps produced by Climate Central. Orange, medium vulnerability; red, high vulnerability. Note the high level of vulnerability of several New Jersey communities.
For the specific case of the United States, The US Army Corps of Engineers (USACE), Engineer Research and Development Center (ERDC) offers a tiered set of coastal resilience metrics that integrate engineering, environmental, and community resilience (Rosati et al., 2015). This approach factors parameters such as need, time, space, and available funding. Some cyber tools and websites that support decision making and emergency response at local and regional levels include the National Oceanic and Atmospheric Administration (NOAA) U.S. Climate Resilience Toolkit.1 The local approaches advocated in the toolkit involve: (1) identifying the local climate related threats; (2) assessing risks and vulnerabilities; (3) exploring options; and (4) prioritizing actions allowed by available resources. The online toolkits for addressing vulnerabilities include the following interactive sites:
• Climate Change Vulnerability Assessment Tool for Coastal Habitats (CCVATCH)
• Coastal County Snapshots
• Coastal Flood Exposure Mapper
• Coastal Change Hazards Portal
• FEMA Flood Map Service Center
• Sea Level Rise and Coastal Flooding Impacts Viewer
• Surging Seas—Sea Level Rise Analysis by Climate Central
Next Generation Coastal Data Acquisition
Ocean observing systems provide environmental intelligence to a variety of users including weather forecasters, commercial and recreational mariners, emergency responders, coastal zone managers, researchers, educators, and more. Fully integrated observing systems consist of platforms, sensors, and technologies to transmit the data to users. Networks operated by NOAA range in size from PORTS® (Physical Oceanographic Real-Time System) to the U.S. IOOS® (Integrated Ocean Observing System) to the NDBC (National Data Buoy Center). Innovative new sensors may be integrated into ocean observing systems to improve our understanding of oceanographic phenomena. Affordable and miniaturized new sensors offer wider and more densely distributed portrayals of coastal processes and hazards at temporal and spatial scales that support decision making. Sensors, particularly water level sensors, widely distributed on land and along streets and highways provide real-time maps of spatially varying water levels during inundation events (Gesch, 2018).
Examples of new in situ sensor-based approaches to quantifying the problems of coastal flooding is offered by the Virginia StormSense Program and the Sea Level Rise Report Card site2 maintained by the Virginia Institute of Marine Science of the College of William & Mary. Storm Sense, involves propagation of cost-effective water level sensors powered through the IoT, and has expanded the available offerings of ingestible data streams at the disposal of today’s cities. StormSense is an IoT-enabled inundation forecasting research initiative working to enhance flood preparedness for flooding resulting from storm surge, rain, and tides. In this study, the results from 28 new water level sensors installed in summer 2017 helped establish the regional resilience monitoring network as recommended by Virginia’s Intergovernmental Pilot Project. To accomplish this, the VA Commonwealth Center for Recurrent Flooding Resiliency’s automated Tidewatch tidal forecast system (36-h lead time) is being used as a starting point to integrate the extant (NOAA) and new (USGS and StormSense) water level observing sensors throughout the Hampton Roads region of Virginia. The StormSense network employs ultrasonic and radar remote sensing technologies alongside web cameras with flood-edge detection capabilities. These new sensors recorded high water levels during Hurricanes Jose and Maria, and the November king tide in 2017. Observations from these events were used validate the inundation predictions of a street-level hydrodynamic inundation model. By the end of 2019, StormSense intends to automate flood control gates, communicate risk via chat-bots, and guide traffic patterns via route guidance apps to keep citizens out of the flood path.
The marine science community is making a great effort to address the estimation of parameters over large areas through in situ sensors, remote sensing, and numerical modeling. Instrumentation for recording atmospheric and oceanographic processes is continually evolving and, in many cases, becoming less expensive allowing for more widespread distribution of monitoring resources. Regional coastal observations within United States waters are currently supported by the U.S. IOOS, under the auspices of NOAA. Included under IOOS are arrays of in situ environmental sensors as well as the U.S. National High Frequency Radar Network. Radar is especially useful to extract both current and waves and supports operations such as search and rescue and oil spill cleanup. Although improvements in the spatial and temporal resolution of data for coastal applications are still needed, new generations of satellite and lower altitude remote observing systems are permitting high-resolution images to be obtained around the clock (Cazenave et al., 2017).
The French OSIRISC (Vers un Observatoire Intégré des Risques côtiers d’érosion-submersion) project (Hénaff, 2017; Marcel et al., 2018; Figure 3), involves the monitoring of coastal systemic vulnerability to erosion and flooding risks within an integrated observatory based on relatively simple and inexpensive data acquisition devices. A key element is the collection of data with relevant spatial representativeness and temporal frequency according to the processes being monitored. These simple indicators allow managers and citizens to participate in the data acquisition process. For example, erosion distance in the hazard component has been determined through spatially registered and oriented photography relying on landscape landmarks and the use of a measuring tape, high technology instruments using a laser pointer device for distance and angle measurements, or tachometer, differential GPS, photogrammetry, and laser scanners.
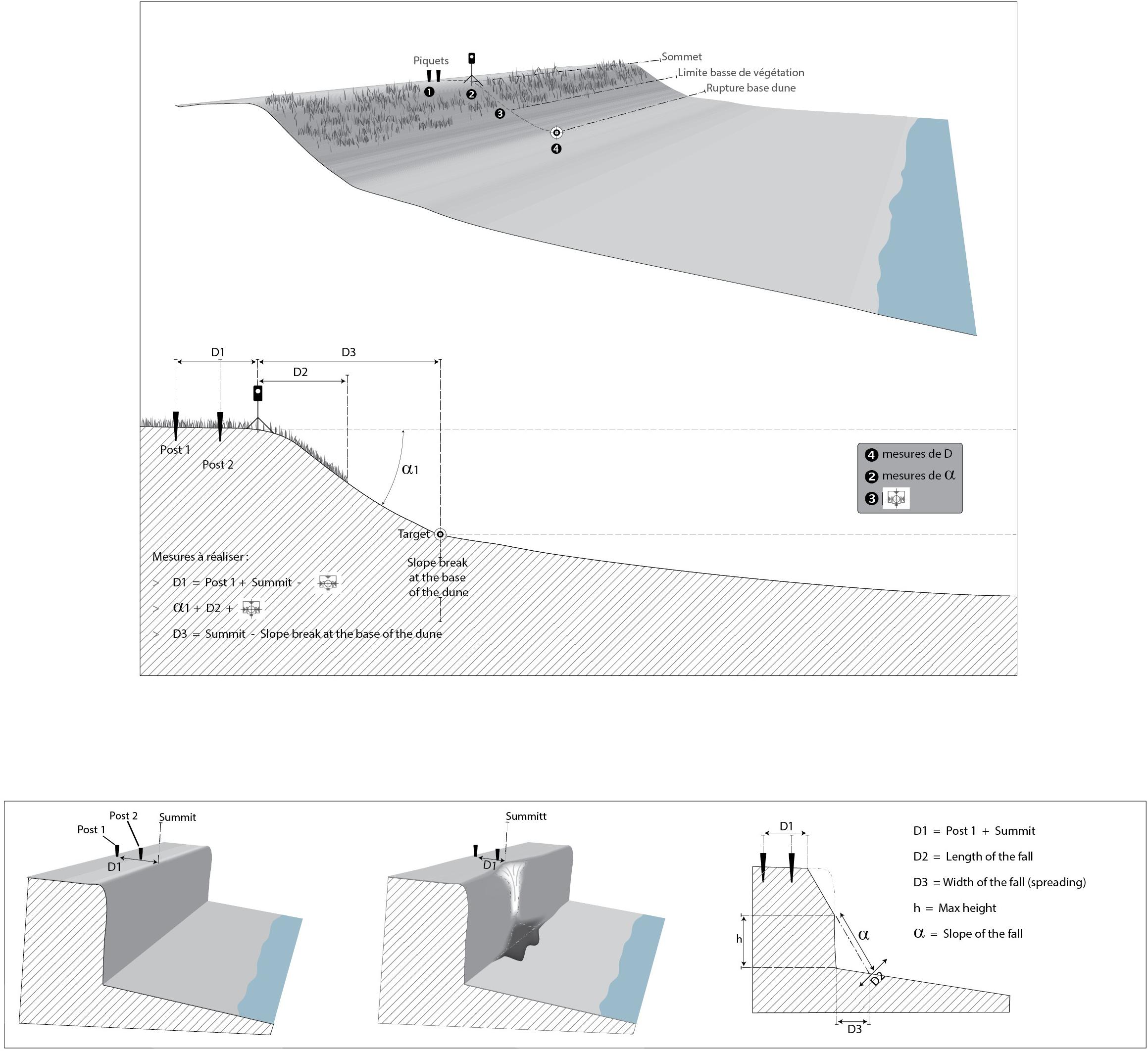
Figure 3. Example of measurements carried out in the OSIRISC project (Hénaff, 2017; Marcel et al., 2018).
Remote sensing technologies provide increasingly accessible information for integrated coastal resilience. Recent advances in airborne and satellite-based radar and laser altimeters have the ability to map coastal topography and its changes at high spatial and temporal resolutions (Salisbury et al., 2017). Drones with high technology sensors are used to map the coastal zone following extreme weather. Guillot et al. (2018) describe flight plans and photogrammetric techniques that were used to study recovery of True Vert beach along the Gironde coast in France from severe storms during winter 2013/2014. Kovacs et al. (2018) have compared several multispectral satellites to classify submerged aquatic vegetation (SAV) in Moreton Bay located along the east coast of Queensland, Australia. This research is important since resilience indicators include the extent of SAV beds and the distribution of juvenile fish that depend on the SAV beds. Engineering and emergency managers may use different types of imagery to characterize the resilience of a built-up areas. Parente and Pepe (2018) describe the retrieval of bathymetry in turbid water near the port city of Lisbon by using radiometric band ratios from WorldView-3 imagery. Electro-optical and Synthetic Aperture Radar are important resources to provide updated maritime domain awareness near ports and harbors. Bannister and Neyland (2015) described the benefit of using regularly updated satellite images to improve maritime operations such as search and rescue. Scientists and engineers apply techniques such as change detection analysis with time series of images to characterize the environment to support planning. Bachmann et al. (2019) illustrated the use of hyperspectral imagery to improve coastal characterization and these approaches provide ocean observing systems with nearshore Digital Elevation Models and features from the land such as biomass and the water such as turbidity.
Bathymetric retrievals from hyperspectral imagery could be used to provide updated information for assimilation into nearshore models before, during, and after storms. Radar imagery continues to be an essential tool to mapping the changing polar regions in support of national ice centers, icebreaking, and those involved in climate studies. Community planners and resource managers are also using lidar data to fill critical information gaps as they devise plans to cope with rising seas, increased coastal flooding, and storm surges. Marine scientists, engineers, coastal zone managers, and other practitioners will increasingly depend on imagery to monitor critical facilities, the response of ecosystems such as a marsh or submerged aquatic vegetation beds to sea level rise, and to plan for resilient coastal communities.
Storm and flood waters are not the only issues of concern. Pathogens and toxins may adversely impact human health and ecosystems and require improvements in their measurement and modeling. Included are Harmful Algal Blooms, across a range of temporal and spatial scales. Some technological advancements have been made since the Fukushima Daiichi nuclear disaster that followed the Tōhoku earthquake on March 11, 2011. Effective ocean observing systems will need to exploit leading-edge sensor technologies for the detection of harmful substances and organisms in the ocean that range in size from radionuclides and microbes to bull sharks and ghost nets. Analytical tools including models may be applied to provide improved environmental information to emergency response, public health, and natural resource managers. Information may include potential threats to infrastructure such as power, sewerage and water, transport and communications systems. Observations and models are key to improving the mapping of dangerous marine organisms, chemical pollutants, or algal toxins that may help decision makers who must disseminate advisories or close shellfish beds or beaches to protect and prevent human illness. The resulting information archived by observing systems and models, needs innovative algorithms that improve contextual reasoning capabilities by recognizing anomalous situations and helping observing system users adapt to anomalies such as extreme weather. The development of Artificial Intelligence (AI) systems may provide tools for the large scale analysis of time series data (Shang et al., 2014). Coastal resilience including the provision of actionable information from AI will involve disparate data from thousands of data sets and computation of non-linear relationships between variables.
Marine scientists and engineers are developing new sensors that are deployable in networked monitoring systems to better observe changes occurring in coastal oceans (e.g., sea level rise, inundation, erosion, sea states, hypoxia, harmful algal blooms). For example, the planned France-based Kineis constellation of 20 nanosatellites will provide connectivity for ocean observing, fishing, maritime security, and more. The Partnership for Observation of the Global Oceans continues to contribute to sensor advances as evidenced by the expanding network of Argo floats that gather temperature, salinity, oxygen, and current velocity data. Important innovations are in development to improve the monitoring of carbon storage and biogeochemical processes, including the development of sensors to measure seawater carbon content, acidity, the concentration of nutrients such as nitrates and phosphorous and even genomic data.
Next Generation Numerical Models
The synthesis of ocean observations combined with accurate models is essential to addressing ecologic, economic, and community resilience. Coastal systems, structures, people and loss of habitat cannot be quantified without a combination of both of these tools. The breadth of scales in the dynamics of coastal processes makes it essential that both observational and modeling capabilities be improved and tightly linked. Today’s numerical models for air-sea interaction, coastal circulation, waves and surges affecting the coast are adequate for many operational applications. However, the essential model tuning often relies on very sparse sets of objective measurements, particularly during extreme events. Improved validation is critically needed to improve model performance in coastal areas worldwide. Predictions and observations of coastal risk in relation to climate change are the subjects a recent special issue of the Journal of Marine Science and Engineering (Ranasinghe and Jongejan, 2018).
The probability of severe storm surge and pluvial flooding jointly occurring in United States coastal cities has increased significantly over the past century (Wahl et al., 2015). In cases where rivers are nearby, fluvial flooding further exacerbates the severity of inundation. Reed et al. (2015) conclude that the risk of compound flooding in New York City is increasing. Urban coasts commonly include large low-lying and flood-prone areas often occupied by low-income and vulnerable residents. The most recent example of compound flooding of low-lying coastal towns was hurricane Florence which made landfall on the United States coasts of the Carolinas in September 2018. Around the same time, “Super Typhoon” Manghut brought extensive flooding to Guangdong Province, China. Much of the damage in both cases was related to precipitation and runoff into elevated coastal water levels. Mutual interactions between hydrometeorological and ocean models must be advanced in the near future.
The constraints and impacts of urban infrastructure and changing land use patterns must be included in models. The coupling of hydrology models to coastal ocean models is an area of active research and considered by some agencies as a Grand Challenge. To address this challenge, there is an urgent need for multi-institutional models combined with objective test metrics, and innovative forecast products related to flooding of urban coasts at a hierarchy of time and space scales. The goal should be to: Improve Understanding and Forecasts of Future Compound Inundation Events Affecting Urban Coasts and Related Socio-economic Vulnerabilities. This will initially involve identifying and assessing the relative impacts of urban flooding under different regimes and designing innovative models to predict future threats. This should be accompanied by effective communication with local, regional and state planners and policy makers concerning specific needs and challenges. NOAA’s U.S. IOOS Program Office has sponsored programs such as the Coastal and Ocean Modeling Testbed (COMT; Luettich et al., 2013, 2017) to transition advances by the modeling research community to the community at large, especially partners such as U.S. IOOS Regional Associations, NOAA Center for Operational Oceanographic Products and Services, U.S. Geological Survey (Verdi et al., 2017), Environmental Protection Agency, and U.S. Army Corps of Engineers.
These collaborative efforts have helped to improve emergency preparedness on a synoptic forecast scale. However, additional collaboration will be required to develop coupled models of hydrologic and ocean flooding in order to understand the probabilities and effects of events such as hurricanes Harvey (2017) in the Houston area, Irma (2017) in Jacksonville, Florida, Florence (2018) along the coast of the Carolinas and Michael (2018) on the Florida panhandle. The outcomes of the observing and modeling communities working together via a common platform provide not just better models, but improved observations as the modelers define what observations they need to improve the model performance.
Evolving Strategies for Improving Coastal Resilience
On a synoptic forecast scale, pre-event preparations typically involve actions such as evacuation operations, pre-positioning materials, and the operation of water-control and transportation infrastructure. At this time scale, models and data assimilation play important roles in maximizing the utility of available resources and minimizing loss of life. However, there is often little that can be done on this time scale to prevent or reduce damages to the built environment and the natural environment. Thus, in addition to improving modeling and sensor technology, new strategies for rapidly disseminating data for adaptive management purposes must be developed. This will be particularly important at the local level. The preparation of the populations to coastal hazards through outreach is particularly important. To be effective, education of the populations exposed to the danger must be prepared well in advance of events. While reminders of past events such as flooding are important, it is also necessary to convince stakeholders that future threats may be more severe than those they remember. Understanding the sensibility of communities in the face of coastal hazards is crucial when designing risk management plans.
Most oceanographic data assimilation related to resilience is currently associated with forecast-scale operations. Although this can be extremely valuable to decision-making and for overall guidance at event time scales, the application of coastal observations for improving objective model validation in long-term planning scales has not received the same level of attention. Models cannot replace measurements without introducing large uncertainties and biases into critical decisions. Most models today contain numerous parametric approximations and empirical coefficients. Careful tuning of coefficients is often performed after a devastating event in forensics studies, but it has been found that these coefficients must be varied significantly to obtain optimal performance at different sites and for different events. There is a need for more focus on high-quality, event-based measurements as well as long-term data sets. Many deficiencies in today’s models have been identified with respect to the physics of nearshore processes. On time scales relevant to long-term planning, potential landscape and ecosystem evolution remains speculative, adding uncertainty to predictions of change in both environmental and anthropocentric factors such as economic and community health. Predictions of waves, surge, and pollutant transport are better than models of coastal evolution.
The overall balance of effort expended on fixed measurements should place more emphasis on event-based sampling. This will require the development of an integrated suite of instruments that could function over a substantial range of spatial and temporal scales in estuarine, riverine, and open-coast environments as well as on flooded lands and roads. Such instrumentation suites would have to be deployable within 24 h and contain sufficient quality and number of sensors within an integrated telemetering and self-recording system (an event-based infosphere) to provide needed data to validate the physics and numerical approximations imbedded in models. The potential contribution of such measurements to improved modeling systems and predictions for resilience cannot be overstated. As noted in section “Next Generation Coastal Data Acquisition” observing future environmental changes in coastal urban areas will require new sensors along with new data pathways and workflows that enable a range of users to collect data and that allow data to be centrally processed for quality control and utility. Some of the phenomena to be observed must include the following:
• Storm surge
• Hydrology
• Pluvial flooding
• Compound flooding
• Water quality
• Socio-economic behavior and vulnerability
• Pathogens and pollutants
• Urbanization and urban renewal trends
• Urban and coastal management principles and practices
Obtaining the needed observations from coasts and coastal communities in developing countries where the threats and vulnerabilities are most acute is a serious challenge but one that must be overcome with the help of international organizations. Some potential strategies are discussed in the following section “Data Intensive Infrastructure for Rapid Dissemination of Information.” Coastal slums in impoverished coastal megacities require global assistance to become even modestly resilient through improved forecasts.
Data Intensive Infrastructure for Rapid Dissemination of Information
Digital connectivity is transforming the way humans deal with problems of collective action. Big data-driven applications can improve emergency management efforts during weather-related disasters in coastal areas by integrating human-generated sources with meteorological and oceanographic big data sources. Integrating social and physical sciences is challenging, given the multiplicity of approaches and subjects of study; however, the acceleration of climate change is increasingly demanding creative and integrated solutions to minimize the hazards of weather-related events exacerbated by climate change. This requires multidisciplinary collaboration, multi-sector partnerships, and the expansion of geographic coverage.
Most emergency management “lessons learned” and “best practices” examine biophysical outcomes of disasters rather than the cultural aspects (Weichselgartner, 2001). Given the expansion of digital connectivity and the increased computational capacity for collecting, storing, and analyzing millions of bits of online data related to human activity, combined social media and biophysical data will improve knowledge about collective action during these weather-related emergencies and to enhance emergency response efforts (e.g., coordination efforts, dissemination of information, identification of critically affected areas). While information about emergency and disaster situations has always been transmitted via different types of media networks, the explosion of human-generated online content has led to a shift in how these networks operate and how crisis-related information is disseminated (Ripberger et al., 2014). Understanding disaster-related communications spread across online social networks is key to assisting emergency management agencies in improving disaster response and coordinating messaging (Hossmann et al., 2011; Kongthon et al., 2012; Gomez-Rodriguez et al., 2013; Saleem et al., 2014; Edwards et al., 2015; Kogan et al., 2015; Rudat and Buder, 2015).
Recent interdisciplinary research has demonstrated the utility of new sources of big data for understanding social processes and generating insights that were previously impossible (Boyd and Crawford, 2012, p. 663). Despite the importance of human-generated big data such as social media, during emergency management situations, there remains a gap between the ability of social and computational scientists to analyze these patterns ex post facto and the ability of emergency management agencies to make use of this data in real-time to prepare for, respond to, and recover from emergency events. Algorithms are needed to provide the users of ocean observation systems with improved realizations of unfolding events. Rule-based and statistical approaches to data analytics include situational learning. The Southeastern Universities Research Association (SURA) and partner organizations such as the Florida Institute of Technology, Louisiana State University, Mississippi State University, and University of North Florida are working on contextual adaptation to enhance coastal resilience. The first step requires a big data needs assessment among interested parties, followed by capacity building to access and analyze big data sources.
One of the biggest impediments to emergency managers and other public officials making use of social media data is its relative inaccessibility and relevance to a particular decision-making issue. While use of social media platforms is free, the ability to scrape and analyze raw data generated by or within these platforms is quite costly. Unlike some other platforms, Twitter makes a subset of its data freely available via its Application Programming Interface (API). However, using the Twitter API requires technical insights that many emergency management agencies are unlikely to have. And while a number of scholars have developed dashboards or other systems for collecting and storing large amounts of this data for social research (Stefanidis et al., 2013; Felt, 2016; Yang et al., 2016; Poorthuis and Zook, 2017), the Twitter Terms of Service prevents these data from being shared. These limitations create a divide between data producers and those who want to access and analyze data. University developed tools are addressing these limitations by building capacity among interested parties. For example, the Social Science Research Center at Mississippi State University has made a major investment in techniques for accessing and analyzing social media data. The result of this effort is a web-based software application known as the Social Media Tracking and Analysis System that can access Twitter messages worldwide.
It is important to understand the variety of ways that social media are used by individuals during disaster situations and leverage this understanding to better develop and target interventions during emergencies. By optimizing methods for analyzing social media data in the context of emergency management, it is possible to identify approaches most capable of yielding actionable insights in emergency situations. In particular, the interest is in drawing on multiple aspects of Twitter data, analyzing the qualitative content of each tweet, the social networks that each tweet diffuses through, and the particular spaces and places where these tweets are created.
The massive volume of digital information available from social media platforms necessitates automated approaches to analyzing the actual content of these messages (Hopkins and King, 2010). Although interpreting big data is challenging (Shah et al., 2015), understanding the content of large bodies of human-generated messages can provide important information for social scientists, government officials, and first responders during disasters and emergencies. Content analysis of social media can be especially important for understanding the communication environment and providing situational awareness before, during, and after a disaster or emergency. In fact, the use of content analysis to identify information gaps, rumors, and misinformation is a recommended strategy for crisis emergency and risk communication (Centers for Disease Control and Prevention, 2014). Houston et al. (2015) have conceptualized a variety of uses of social media data during a disaster (from preparedness to post-disaster reconnection), and the value of content analyzing Twitter data emerging from disasters has been illustrated in a number of case studies (e.g., Muralidharan et al., 2011; Spence et al., 2015; Takahashi et al., 2015).
In addition to the qualitative content of tweets, a critical aspect is the relational nature. The networked structure of social media makes it possible to think of it as a mechanism to improve dissemination of information in the context of disaster and emergency response (Magsino, 2009; Jones and Faas, 2016). The goal of network analysis is to map or describe the array of relationships between a set of objects, or nodes (Kadushin, 2012). Given the expansion of internet-based social networks and increased computational capacity for data collection and analysis of these platforms, Social Network Analysis (SNA) allows us to obtain useful information from the large number of relationships within this data. In the context of disaster and emergency response this analysis can improve information propagation throughout a given social network. This can facilitate quicker relays of information, or combat misinformation before it spreads.
Using geographic information systems and spatial analytical techniques, spatially referenced big data can inform responders as to where disasters have occurred and where people might need assistance (Crooks et al., 2013; Kent and Capello, 2013). When combined with the network and content analyses, the spatial analysis of human-generated big data can reveal whether differences in social networks, information diffusion and messaging are shaped by spatial proximity or by some other form of place-based process (Starbird and Palen, 2010; Shelton et al., 2014; Kogan et al., 2015). Because disasters and emergency response situations are intensely place-based events, the addition of a spatial analysis component is key to understanding how these events are experienced and managed by everyday people and governmental agencies, which may not always be in sync, especially when underrepresented or marginalized groups are heavily affected (Crutcher and Zook, 2009; Mulder et al., 2016).
Strategies for Coastal Risk Assessment and Adaptive Management
In order to enhance coastal risk assessment and adaptive management, we advocate the establishment of partnerships that transcend geographic and disciplinary boundaries. Partnerships that include representatives from state and federal agencies, non-profits, conservation groups, and the business, health, and industry sectors provide an effective constituency for the needed information. These data provide the basis to develop a conceptual framework for coastal risk assessment in a structured process that is based on the specification of geographic patterns of hazards overlaid by patterns of vulnerability (life-loss, damages, critical infrastructure, social hubs, etc.). In this context, the risks can be quantified to meet the needs of a variety of stakeholders who must make data-driven decisions. The value of accurate estimation and the consequences of inaccuracies in the hazard and risk estimations make a strong case for a unified approach to this issue. A good example of this is the dramatic rise in losses and casualties due to natural disasters such as storm-surge-induced flooding, seismic hazards and tsunami incidence along many coasts over the past few decades that has prompted global concern on impacts and mitigation strategies (Wright and Nichols, 2019). Marine scientists analyze and forecast coastal changes such as regionally varying sea level rise (e.g., Thompson et al., 2014, 2016). Government officials have already started to plan for sea-level rise by completing coastal hazard assessments and developing maps showing areas which are expected to be affected over the next 50–100 years. In some cases, these planning guides support activities such as restricting development in areas prone to coastal erosion, moving structures away from the coast, and discouraging the construction of shore protection.
Government organizations including ocean and meteorological agencies (e.g., Australian Bureau of Meteorology, METEO France, and NOAA), local universities, businesses, and citizens have provided discoverable data from networked sensors that are included as big data resources which can support decision makers. These data come in various forms including historical archives from national data centers, in situ data from the neighbor’s weather station to ocean observing systems, handheld to satellite imagery, and numerical model output. Users need to sift through data from local, national, and globally available datasets that can help address environmental issues, ranging from recurrent flooding to sea level rise. Local university researchers are already applying new technologies such as unmanned vehicles to fill data gaps that may mask important processes, providing algorithms as evidenced by the COMT, and are defining levels of uncertainty in the data that are available for analysis (Luettich et al., 2017). Private sector companies are also applying big data for targeted solutions and predictive power such as apps that provide weather data to commercial and recreational fishermen. Crowd-sourcing and citizen science like the Hawaiian and Pacific Islands King Tide Project and Geofeedia – a social media intelligence platform that associates social media posts with geographic locations – are increasingly popular tools for creating information where there previously was none. Open hardware and software are expanding to offer widely distributed, inexpensive tools to enable crowd-sourced data collection and analysis.
Coastal risk assessment and adaptive management can employ big data to resolve spatially and temporally variable phenomena that impact coastal communities. Environmental phenomena such as flash floods manifest themselves quickly whereas sea level rise is slow. Big data include historical information, in situ data, imagery, and model output. Next generation tools will need to aggregate these vast amounts of data to aid decisions. Baseline information will be key to identifying the magnitude of current changes in coastal processes. Government funded infrastructure such as the National Ecological Observatory Network, Long-term Ecological Research stations, and the U.S. IOOS should collaborate to improve our understanding about issues such as sea level rise, recurring flooding, land use change, harmful algal blooms, hypoxia, and invasive species impacts. These efforts require the sharing of data through open automated resources at both national and international levels. Through this collaboration, next generation models, and data analytics can be applied in an iterative decision-making process. Research is still needed on contextual explanatory models that are reflective of real-world situations. For example, through “on the fly” skill assessments, operational users can select the best model to use for their particular applications.
Communicating Knowledge and Predictions to Decision Makers and the Public
Technology solutions and information sciences must be refocused to provide cities, localities, city planners, port engineers, and emergency responders with both specific actionable information that meets the specific needs of that decision maker and anticipates global changes. This information is based on structured and unstructured data, but to be actionable it must be communicated to decision makers in non-technical language and readily understood graphics. Disparate sources of data are gathered from data centers (historical information), IoT sensors (in situ data from weather stations, buoys, drifters), aircraft and satellites (remotely sensed imagery), and environmental prediction centers (numerical models). All are essential to providing situational awareness for decision making. Trying to make sense of the coastal zone is a complex challenge. University and institutional researchers have developed a plethora of solutions, tools, and services to help fuse the disparate data to predict events such as inundation with greater certainty.
Understanding of information requirements on different time scales is crucial to future success since the stakeholder’s operations are impacted differently at these scales. Typical needs can be partitioned into four contiguous scales: pre-storm preparation; during-storm information and reaction, post-storm recovery and long-term planning. Successful applications require an understanding of each stakeholder’s mission, objectives, and definition of success before implementing a technological approach. Stakeholders are diverse, not only in terms of their requirements but in their capacity to participate. For example, communities in developing areas may not have access to data or the resources required to act on the information provided. Stakeholder’s requirements are constantly evolving and applications should as well.
Processes that include artificial intelligence, via Deep Learning approaches, may meet some future requirements to support forecasting and response to extreme events. Fused data, where multiple data streams are merged to give local context (for example stream heights contextualized by local levee heights), are more usable by first responders. This type of value-added information can increase the speed and effectiveness of response. AI systems that can interconnect engineering, environmental, and community systems and provide contextualized data as information, enhances the effectiveness of decision-makers and first responders. There is considerable value in having strong relationships between researchers working on AI, such as through a local university or research agency, and local first responders. In this way, research can be linked to response and expertise transferred to state and local programs.
Large datasets culled from data centers, ocean observing systems, model centers, and imagery libraries can easily grow to a size beyond the analytical capability of common software tools and single researchers. To fully utilize the potential of big data requires dedicated resources and expertise. Margaret Davidson, the former director of today’s NOAA Office for Coastal Management, recommended the establishment of a collaborative virtual community focusing on data intensive computing to integrate physical and social sciences and improve coastal resilience – a facility that she called a “collaboratorium.” This virtual infrastructure for data intensive analysis would integrate diverse teams of scientists and also leverage cloud-based infrastructure, storage, networks, high performance computing, heterogeneous multi-provider services integration, data centric service models, and security for trusted infrastructure and data processing and storage. The collaboratorium would be designed to collect and process high-volume, high-velocity, high-variety data to develop effective and innovative forms of data. Collaborators will share information processing (analytics) for enhanced process control, insight, and decision making. This idea of dedicated centers or environments, where resources, expertise, data and robust use cases, can be brought together to transition research-based systems into operational or semi-operational systems.
The French National Research Infrastructure (FNRI) Data and Services Center for Earth System Modeling offers an example of a data management and dissemination system.
This system is intended to serve primarily the French research community. However, the products also have international aims (satellite missions, global observing networks, partnership for development). The FNRI products are defined and elaborated under the guidance of experts to ensure that they agree with the highest scientific and technical standards. Data series and products from observing networks, in situ campaigns and satellite missions, will be qualified, described, and interoperable. The FNRI also provides tools for data discovery, visualization, extraction, and processing, as well as computing resources. To encourage sharing information, good practices and to contribute to the scientific and technical training of the user community, the FNRI has developed collaborative platforms for marine data (e.g., ODATIS).3 Data and information distributed by the FNRI are important for the implementation of public policies. Developments using these data have important socio-economic impacts on domains such as natural hazards, climate change, and natural resources. The data centers contributing to the FNRI are spread over different regions where they participate in the development of Subject Matter Experts who can exploit their data to generate environmental intelligence.
Creating a Collaborative Environment to Enhance Coastal Resilience
There is a clear societal need for increased collaboration that leads to improved understanding and mitigation of extreme events. This will require “the science of collaboration” among observational groups, modelers, stakeholders, and others to meet critical future needs in coastal areas. Wright and Thom (2019) describe some of the challenges of promoting effective coastal resilience collaboration as well as some of the approaches that may help overcome those challenges. Especially important is the communication of research results to stakeholders from the community. In addition to traditional modes of collaboration, the Southeastern Universities Research Association (SURA) has implemented a collaborative virtual environment to complete big science projects such as the Southeastern Coastal Ocean Observing and Predicting Program (SCOOP) and the Coastal and Ocean Modeling Testbed (COMT) supported by the NOAA IOOS Office. Both projects required the accomplishment of multidisciplinary and integrated tasks completed by university, government, and industry participants that were spread throughout the United States and Canada. Typical components used to share information and integrate results in a collaborative virtual environment were distributed databases, open source models, webinars, and other types of collaborative software. Important software applications were components of the COMT cyberinfrastructure that were designed to promote data sharing of seminal datasets. In its recent report on community resilience pertaining specifically to the Gulf (of Mexico) Research Program (GRP), the National Academies of Sciences, Engineering, and Medicine, (2019, p. 83) recommends: “The GRP should create a resilience learning collaborative for stakeholders to exchange information, approaches, challenges, and successes in their respective and collective work to advance community resilience in the Gulf region. The collaborative participants should include government (local, state, federal levels), industry, academia, and other organizations engaged in addressing community resilience.”
Another prominent example of a collaborative program is the French OSIRISC project: Toward an Integrated Observatory of Coastal Risk and erosion and submersion. The French researchers follow a systemic approach to vulnerability in order to better understand hazards, improve management, and mitigate the associated impacts on populations and other stakes. This interdisciplinary, systemic approach has demonstrated success in addressing the coastal risks of erosion and floods. The evolution of coastal vulnerability over time – whether due to anthropogenic or natural forcing – is readily observable in the present context of climate change and sustained growth of the exposed stakes on coastal territories. Whereas the monitoring of coastal hazards is benefiting from new sensors and data centers, methodologies and tools that allow the assessment of trends, and the management of individual and social representations of risk are still limited.
To address the need for a coherent coastal vulnerability evaluation framework for the benefits of both academics and stakeholders, the OSIRISC project (2017–2019) offers a methodology dedicated to the interdisciplinary observation of the coastal risks of erosion and flooding. OSIRISC focuses specifically on:
(1) The elaboration of indicators describing these components.
(2) The integration of such indicators into a web-based interface with high spatial resolution.
(3) An iterative testing process bringing together coastal risk experts (researchers, stakeholders, land use managers) at the French regional level, in Brittany.
Key to realizing the promise of virtual collaboration is the assignment of collaboration leaders. These individuals facilitate interaction among participants regardless of location and organization to mine the collective wisdom of a widely dispersed group of researchers. Collaboration leaders need to focus on methods that get virtual teams to bond in order to create meaningful dialogue, share successes, and avoid misunderstandings. Collaboration leadership was important to establishing unwritten agreements related to communications and decision-making and building virtual trust on SURA’s big science projects. Trust among the various partners grew out of SCOOP and expanded with COMT. One key element facilitating collaboration involved the planning and execution of the annual COMT PI and Partners meeting. Such meetings facilitate strong collaborations across research communities and federal agencies.
Creating a new generation of virtual collaborative environments encourages innovation and allows diverse perspectives to be leveraged and to connect interdisciplinary researchers. Collaborative demonstrations with stakeholders in parallel with research and development are essential to prepare, resist, recover, and adapt to extreme events and long-term change. By building team confidence in the abilities of its members and establishing specific strategies for knowledge sharing, the diversity of perspectives on collaborative teams can be more readily accessed. Leadership must ensure that virtual collaboration stays focused and that project goals are accomplished in a timely and cost-effective manner. Furthermore, community support and acceptance are essential. Hence, next generation observatories for coastal resilience and adaptation should invest in outreach to and nurturing of citizen scientists.
Vision for Future Support of Coastal Resilience Initiatives
The global coastal science community should develop a vision for 2029 for observations and systems that promote and enhance coastal resilience and adaptation and meld the following enhancements into a framework for delivering new outcomes:
(1) Increased collection of coastal observation data through use of satellites, traditional observing programs, smart-city projects such as the StormSense network in Hampton Roads, Virginia, and cheap sensor or mobile-phone-based citizen science projects via community, NGO’s or other groups;
(2) Implementation of new sensors including satellite constellations such as Kinesis, cheaper water level sensors, sensors for pollutants and contaminants and for biological responses such as Harmful Algal Blooms, organized as sensor webs using IoT principles for data collection and analysis;
(3) Use of social media both as a valuable input data source and as a means of delivering targeted information about alerts, risks and responses;
(4) A better understanding of how humans react to and utilize emergency response data and information to ensure that the social science around public level emergency response is understood so that new tools and approaches provide outcomes not just information;
(5) Development of better models through collaborative environments, such as COMT, where models can be developed, validated, assessed and operationalized;
(6) Use of AI as a fundamental tool for bringing together a range of disparate data of varying levels of quality and type for processing for input into models and to constrain outputs to actionable information;
(7) A method of collecting on ground data during events, via trained first responders or equivalent, to better force models and ensure that model outputs are relevant and of use;
(8) Virtual collaborative environments, aka “collaboratoriums,” that bring together the latest advances in computing, in data science, in observational science, in social science, environmental science, engineering and policy to address particular end-user use-cases with the goal of undertaking the research and development required to deliver new types of information, tools and understandings.
(9) An objective evaluation of models at all locations following events to identify issues that may compromise predictions. Comparisons should be done by a collaborative group that collects the observations independent of operational groups that produce the model results.
(10) Improved use of derived information and development of enhanced technologies for post-event recovery.
(11) It is critical for additional information to be collected during extreme events. The sparse estimates of waves and water levels, often after the events, does not allow a rigorous evaluation of model physics and numerical methods.
Figure 4 shows how the various components of a collaborative system might interconnect to provide a single framework to promote and enhance coastal resilience. Future plans should leverage resilience efforts that are focused on threats common to a geographic region through sustained educational and outreach programs that help develop stakeholders from the private, public, and university sectors. A seminar series is essential to promote the inclusion of powerful partnerships extending from local to regional scales that leverage big data resources and viable data analytics. Develop a website that connects coastal resilience resources to facilitate progress in a field that is already developing globally. The website should feature digital scholarship developed by natural and social scientists, especially results from grand challenges such as the coupling of inundation and social “urban planning” models. This website will provide a foundation for progress and the development of fully integrated partnerships that include government, university, and industry members. There should be provision for limited objective experiments that allow partnership assessment and evaluation of approaches that best achieve integrated environmental, engineering, and community resilience results at the local level. These experiments would apply data that includes historical information, in situ observations, imagery, and numerical models. Based on local successes, they would provide an opportunity for the planning and execution of regional-scale research experiments where science-based results can be demonstrated to benefit a large geographic region, one that might include observational networks such as the National Science Foundation (NSF) Long-term Ecological Research Network, NOAA’s U.S. IOOS, and the NSF Ocean Observing Initiative. Importantly, rapidly deployable in situ observations should be collected to characterize extreme events. A capstone demonstration should be conducted that allows for the objective assessment of research results by operational users from local emergency responders and federal stakeholders.
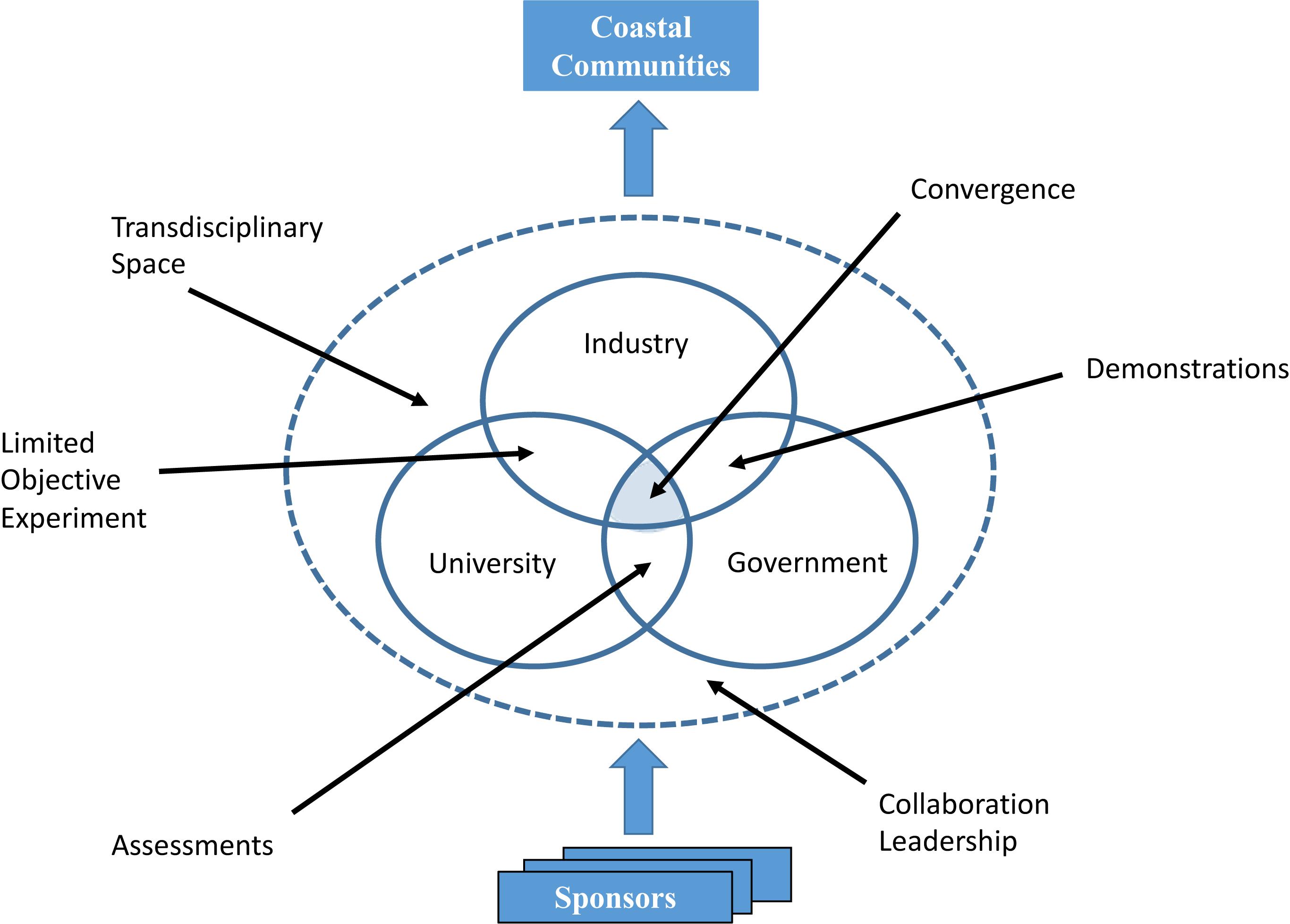
Figure 4. Fully integrated projects should include stakeholders from government, academia, and industry. Collaboration leadership brings together the environmental scientists, engineers, and community to develop the theoretical foundations of coastal resilience through integrated research and training activities. Arrows indicate the flow of progress toward developing more resilient communities.
Recommendations
Observing systems, coupled with models and information dissemination subsystems, can provide the environmental intelligence to allow coastal communities to adapt to long-term coastal degradation and short-term catastrophic events. Many of the required components already exist or are in development. The main need is for networks to connect observers, modelers, managers and first responders so that solutions can flow across disciplinary and stakeholder boundaries. This already includes connecting observing and modeling communities. For new developments in understanding and predicting to enhance the resilience of coastal communities in the future, more attention needs be devoted to education of, and outreach to, the affected communities and their leaders.
Some challenges that still need to be addressed include the following:
• New observing systems, including satellites, sensors, and collection mechanisms, such as community driven data collection, should be developed using the frameworks from the IoT, to allow for large scale collection and analysis of data including fundamental base-line data and maps.
• To facilitate the development of new models and information systems, including AI approaches to big data, there is a need for better integration of data during extreme events to deliver outcomes suitable for decision makers and first responders.
• Development of “collaboratoriums,” will allow observing, modeling, informatics, management, and response communities to connect. Results will foster better strategies and systems, validate and operationalize models and facilitate coordination among communities, nationally and internationally.
• Collaboration leaders must be equipped to help science groups and teams meet the challenges of collaborating across organizations, regions, and nations.
• Information products and prediction, including base-line data sets, infrastructure and expertise, must be made available to developing or resource poor countries that, in many cases, have much of the high-risk coastline and host many of the 16 coastal based mega-cities.
The constraining issues are not primarily technical but organizational. Coastal resilience is important to all countries at some level and so the task is to take best practice from organizations around the world and deliver outcomes that enhance our ability to maintain and sustain our coastal communities. Coastal communities are at the forefront of climate change impacts including short term events and longer-term trends. Community resilience requires new approaches to the collection of observing data and new methods for data dissemination. The challenge is to develop collaborations and projects that bring all of the parties together to ensure that coastal communities can implement resilient strategies. Regionally specific coastal resilience seminars and workshops can identify optimal areas to plan transdisciplinary experiments. Such workshops allow stakeholders and science teams, to share views for selected projects while deepening the researcher’s grasp of issues and priorities.
Author Contributions
CN initiated the preliminary draft, added materials along the way, and made edits for the entire white paper. LW facilitated the collaborative writing, ensured citations of the recent work, and made substantial edits. AC and GM contributed to the big data sections. LC coordinated the input from AH, PL, and NLD contributions on the Université de Bretagne Occidentale resilience research, Eurosion, and edits. SB contributed to the AIMS material and made excellent edits that enhanced the final manuscript. JDL and SK provided results and text concerning the Storm sense program. AH, PL, NLD, and LC conducted research and provided text on the French programs. DR made important changes related to the modeling and nearshore processes. GZ helped with the organization and added nearshore and coast type perspectives.
Funding
The OSIRISC project was supported by grants from the Fondation de France under grant agreement No. 1539.
Conflict of Interest Statement
The authors declare that the research was conducted in the absence of any commercial or financial relationships that could be construed as a potential conflict of interest.
Acknowledgments
This synthesis was an outcome of ongoing support by the Southeastern Universities Research Association for the Coastal Resilience Program and support by the host institutions of all coauthors.
Footnotes
- ^ https://toolkit.climate.gov
- ^ http://www.vims.edu/research/products/slrc/compare/east_coast/index.php
- ^ https://www.odatis-ocean.fr/en/
References
Bachmann, C. M., Rehman, S. E., Lapszynski, C. S., Badura, G. P., Vodacek, A., Hoffman, M. J., et al. (2019). A low-rate video approach to hyperspectral imaging of dynamic scenes. J. Imaging 5:6. doi: 10.3390/jimaging501000
Bannister, N. P., and Neyland, D. L. (2015). Maritime domain awareness with commercially accessible electro-optical sensors in space. Int. J. Remote Sens. 36, 211–243. doi: 10.1080/01431161.2014.990647
Bernatchez, P., and Fraser, C. (2012). Evolution of Coastal defense structures and consequences for beach width trends, Québec, Canada. J. Coast. Res. 28, 1550–1566. doi: 10.2112/jcoastres-d-10-00189.1
Blackburn, S., and Pelling, M. (2014). Coastal megacities: risks and opportunities. IGBP Glob. Chang. Magaz. 82, 8–11.
Boyd, D., and Crawford, K. (2012). Critical questions for big data: provocations for a cultural, technological, and scholarly phenomenon. Inform. Commun. Soc. 15, 662–679. doi: 10.1080/1369118x.2012.678878
Camfield, F. E., and Morang, A. (1996). Defining and interpreting shoreline change. Ocean Coast. Manag. 32, 129–151. doi: 10.1016/s0964-5691(96)00059-2
Carter, R. W. G. (1988). An Introduction to the Physcial, Ecological, and Cultural Systems of Coastlines. London: Academic Press Limited.
Cazenave, A., Cozannet, G., Le Benveniste, J., Woodworth, P. L., and Champollion, N. (2017). Monitoring Coastal Zone Changes From Space. Lisbon: EOS.
Centers for Disease Control and Prevention (2014). Crisis and Emergency Risk Communication. Washington, DC: U.S. Department of Health and Human Services.
Crooks, A., Croitoru, A., Stefanidis, A., and Radzikowski, J. (2013). Earthquake: twitter as a distributed sensor system. Trans. GIS 17, 124–147. doi: 10.1111/j.1467-9671.2012.01359.x
Crutcher, M., and Zook, M. (2009). Placemarks and waterlines: racialized cyberscapes in post-Katrina google earth. Geoforum 40, 523–534. doi: 10.1016/j.geoforum.2009.01.003
Cutter, S. L., Ash, K., and Emrich, C. (2014). The geographies of community disaster resilience. Glob. Environ. Chang. 29, 65–77. doi: 10.5055/jem.2014.0165
Cutter, S. L., Burton, C. G., and Emrich, C. T. (2010). Disaster resilience indicators for benchmarking baseline conditions. J. Homeland Secur. Emerg. Manag. 7:51. doi: 10.2202/1547-7355.1732
Dawson, A. (2017). Extreme Cities: The Perils and Promise of Urban Life in the Age of Climate Change. New York, NY: Verso, 378.
d’Ercole, R., and Pigeon, P. (1999). L’expertise internationale des risques dits naturels: intérêt géographique/Geographical relevance of natural risk assessment on an international scale. Ann. Géogr. 608, 339–357. doi: 10.3406/geo.1999.21777
Doornkamp, J. C. (1998). Coastal flooding, global warming and environmental management. J. Environ. Manag. 52, 327–333. doi: 10.1006/jema.1998.0188
Edwards, J. F., Mohanty, S., and Fitzpatrick, P. (2015). Assessment of Social Media Usage During Sever Weather Events and the Development of a Twitter-Based Model for Improved Communication of Storm-related Information. Starkville, MS: NOAA.
Felt, M. (2016). Social media and the social sciences: how researchers employ big data analytics. Big Data Soc. 3, 1–15.
Flanagan, B. E., Gregory, E. W., Hallisey, E. J., Heitgerd, J. L., and Lewis, B. (2011). A social vulnerability index for disaster management. J. Homeland Secur. Emerg. Manag. 8:3. doi: 10.2202/1547-7355.1792
Gesch, D. B. (2018). Best practices for elevation-based assessments of sea-level rise and coastal flooding exposure. Front. Earth Sci. 6:230. doi: 10.3389/feart.2018.00230
Gomez-Rodriguez, M., Leskovec, J., and Schölkopf, B. (2013). “Structure and dynamics of information pathways in online media,” in Proceedings of the Sixth ACM International Conference on Web Search and Data Mining, (New York, NY: ACM), 23–32.
Guillard-Gonçalves, C., Cutter, S. L., Emrich, C. T., and Zêzere, J. L. (2014). Application of social vulnerability index (SoVI) and delineation of natural risk zones in greater lisbon. Portugal. J. Risk Res. 18, 651–674. doi: 10.1080/13669877.2014.910689
Guillot, B., Castelle, B., Marieu, V., Bujan, S., and Rosebery, D. (2018). “UAV Monitoring of 3 year foredune partial recovery from a severe winter: Truc Vert beach,” in Proceedings from the International Coastal Symposium (ICS) 2018, eds J.-S. Shim, I. Chun, and H. S. Lim Busan, 276–280. doi: 10.2112/si85-056.1
Gunderson, L. H., and Holling, C. S. (2002). Panarchy: Understanding Transformations in Human and Natural Systems. Washington, DC: Island Press.
Hallegatte, S., Green, C., Nicholls, R. J., and Corfee-Morlot, J. (2013). Future flood losses in major coastal cities. Nat. Clim. Chang. 3, 802–806. doi: 10.1111/risa.13285
Hénaff, A. (2017). Vers un Observatoire Intégré des Risques Côtiers d’érosion- Submersion. Journées du Littoral de la Fondation de France. Paris: UICP.
Hénaff, A., and Philippe, M. (2014). Gestion des Risques d’érosion et de Submersion Marines. Guide Méthodologique. Available at: http://www.risques-cotiers.fr/fr/boite-a-outils/guide_cocorisco (accessed November, 2014).
Hopkins, D. J., and King, G. (2010). A method of automated nonparametric content analysis for social science. Am. J. Polit. Sci. 54, 229–247. doi: 10.1111/j.1540-5907.2009.00428.x
Hossmann, T., Legendre, F., Carta, P., Gunningberg, P., and Rohner, C. (2011). “Twitter in disaster mode: Opportunistic communication and distribution of sensor data in emergencies,” in Proceedings of the 3rd Extreme Conference on Communication: The Amazon Expedition, (New York, NY: ACM).
Houston, J. B., Hawthorne, J., Perreault, M. F., Park, E. H., Goldstein Hode, M., Halliwell, M. R., et al. (2015). Social media and disasters: a functional framework for social media use in disaster planning, response, and research. Disasters 39, 1–22. doi: 10.1111/disa.12092
Jones, E. C., and Faas, A. J. (eds). (2016). Social Network Analysis of Disaster Response, Recovery, and Adaptation. Oxford: Elsevier.
Kadushin, C. (2012). Understanding social networks: Theories, concepts, and findings. Oxford: Oxford University Press.
Kent, J. D., and Capello, H. T. Jr. (2013). Spatial patterns and demographic indicators of effective social media content during the Horsethief Canyon fire of 2012. Cartogr. Geogr. Inform. Sci. 40, 78–89. doi: 10.1080/15230406.2013.776727
Kogan, M., Palen, L., and Anderson, K. M. (2015). “Think local, retweet global: Retweeting by the geographically-vulnerable during Hurricane Sandy,” in Proceedings of the 18th ACM Conference on Computer Supported Cooperative Work & Social Computing, (New York, NY: ACM), 981–993.
Komar, P. D., and McDougal, W. G. (1988). Coastal erosion and engineering structures: the Oregon experience. J. Coast. Res. Spec. Issue 4, 77–92.
Kongthon, A., Haruechaiyasak, C., Pailai, J., and Kongyoung, S. (2012). “The role of Twitter during a natural disaster: Case study of 2011 Thai Flood,” in Proceedings of PICMET’12Technology Management for Emerging Technologies (PICMET), (Piscataway, NJ: IEEE), 2227–2232.
Kovacs, E., Roelfsema, C., Lyons, M., Zhao, S., and Phinn, S. (2018). Seagrass habitat mapping: how do Landsat 8 OLI, Sentinel-2, ZY-3A, and Worldview-3 perform? Remote Sens. Lett. 9, 686–695. doi: 10.1080/2150704X.2018.1468101
Luettich, R. A. Jr., Wright, L. D., Nichols, C. R., Baltes, R., Friedrichs, M. A. M., Kurapov, A., et al. (2017). A Test Bed for Coastal and Ocean Modeling. Lisbon: EOS.
Luettich, R. A., Wright, L. D., and Signell, R. (2013). Introduction to special section on the U.S. IOOS Coastal and Ocean modeling testbed. J. Geophys. Res. Oceans 118, 6319–6328. doi: 10.1002/2013JC008939
Magsino, S. L. (2009). Applications of Social Network Analysis for Building Community Disaster Resilience. Washington, DC: National Academy of Sciences.
Marcel, O., Le Berre, I., Rouan, M., Philippe, M., and Hénaff, A. (2018). Une Application WEB- SIG de Suivi de la Vulnérabilité Systémique des Littoraux (Projet OSIRISC). Plouzané: Ifremer.
Meur-Férec, C., Deboudt, P., Deldrève, V., Flanquart, H., Hellequin, A.-P., Herbert, V., et al. (2003–2004). La Vulnérabilité des Territoires Côtiers: Évaluation, Enjeux et POLITIQUES Publiques. France: Programme National Environnement Côtier (PNEC).
Meur-Férec, C. (2006). De la Dynamique Naturelle à la Gestion Intégrée de L’espace Littoral: Unitinéraire de Géographe. Géographie. Nantes: Université de Nantes.
Meur-Férec, C. (2008). De la Dynamique Naturelle à la Gestion Intégrée de L’espace Littoral: Unitinéraire de Géographe. Paris: Edilivre, 250.
Meur-Férec, C., and Morel, V. (2004). L’érosion sur la frange côtière: un exemple de gestion des risques. Nat. Sci. Soc. 12, 263–273. doi: 10.1051/nss:2004038
Michel-Guillou, E., and Meur-Féréc, C. (2017). Representations of coastal risk (erosion and marine flooding) among inhabitants of at-risk municipalities. J. Risk Res. 20, 776–799. doi: 10.1080/13669877.2015.1119181
Mulder, F., Ferguson, J., Groenewegen, P., Boersma, K., and Wolbers, J. (2016). Questioning big data: Crowdsourcing crisis data towards an inclusive humanitarian response. Big Data Soc. 3:2053951716662054.
Muralidharan, S., Rasmussen, L., Patterson, D., and Shin, J. H. (2011). Hope for Haiti: an analysis of facebook and twitter usage during the earthquake relief efforts. Public Relat. Rev. 37, 175–177. doi: 10.1016/j.pubrev.2011.01.010
National Academies (2012). Disaster Resilience: A National Imperative. Report of Committee on Increasing National Resilience to Hazards and Disasters. Washington DC: National Academies Press, 260.
National Academies of Sciences, Engineering, and Medicine (2019). Building and Measuring Community Resilience: Actions for Communities and the Gulf Research Program. Washington, DC: The National Academies Press.
Norris, F., Stevens, S., Pfefferbaum, B., Wyche, K., and Pfefferbaum, R. (2008). Community resilience as a metaphor, theory, set of capacities, and strategy for disaster readiness. Am. J. Commun. Psychol. 41, 127–150. doi: 10.1007/s10464-007-9156-6
Parente, C., and Pepe, M. (2018). Bathymetry from worldView-3 satellite data using radiometric band ratio. Acta Polytech. 58, 109–117. doi: 10.14311/AP.2018.58.0109
Paskoff, R. (1998). Les Littoraux, Impacts des Aménagements Sur Leur Évolution. Paris: Armand Colin.
Pelling, M., and Blackburn, S. (eds). (2014). Megacities and the Coast: Risk, Resilience and Transformation. New York, NY: Routledge, 248.
Poorthuis, A., and Zook, M. (2017). Making big data small: strategies to expand Urban and Geographical research using social media. J. Urban Technol. 24, 115–135. doi: 10.1080/10630732.2017.1335153
Ranasinghe, R., and Jongejan, R. (eds). (2018). Climate Change, Coasts and Coastal Risk. Basel: MDPI.
Reed, A. J., Mann, M. E., Emanuel, K. A., Lin, N., Horton, B. P., Kemp, A. C., et al. (2015). Increased threat of tropical cyclones and coastal flooding to New York City during the anthropogenic era. Proc. Natl. Acad. Sci. U.S.A. 112, 12610–12615. doi: 10.1073/pnas.1513127112
Ripberger, J. T., Jenkins-Smith, H. C., Silva, C. L., Carlson, D. E., and Henderson, M. (2014). Social media and severe weather: do tweets provide a valid indicator of public attention to severe weather risk communication? Weather Clim. Soc. 6, 520–530. doi: 10.1175/wcas-d-13-00028.1
Rosati, J. D., Touzinsky, K. F., and Lillycrop, W. J. (2015). Quantifying coastal system resilience for the US Army Corps of Engineers. Environ. Syst. Decis. 35, 196–208. doi: 10.1007/s10669-015-9548-3
Rudat, A., and Buder, J. (2015). Making retweeting social: The influence of content and context information on sharing news in twitter. Comp. Hum. Behav. 46, 75–84. doi: 10.1016/j.chb.2015.01.005
Saleem, H. M., Xu, Y., and Ruths, D. (2014). Novel situational information in mass emergencies: what does twitter provide? Proc. Eng. 78, 155–164. doi: 10.1016/j.proeng.2014.07.052
Salisbury, J., Davis, C., Erb, A., Hu, C., Gatebe, C., Jordan, C., et al. (2017). Coastal observations from a new vantage point. EOS Earth Space Sci. News 98, 20–25.
Salman, A., Lombardo, S., and Doody, P. (2004). Living with Coastal Erosion in Europe: Sediment and Space for Sustainability. Part IV—A Guide to Coastal Erosion Management Practices in Europe: Lessons Learned. Hague: Eurosion, Directorate General Environment European Commission.
Shah, D. V., Cappella, J. N., and Neuman, W. R. (2015). Big data, digital media, and computational social science: Possibilities and perils. Ann. Am. Acad. Polit. Soc. Sci. 659, 6–13. doi: 10.1177/0002716215572084
Shang, C., Yang, F., Huang, D., and Lyu, W. (2014). Data-driven soft sensor development based on deep learning technique. J. Process Control 242:233.
Shelton, T., Poorthuis, A., Graham, M., and Zook, M. (2014). Mapping the data shadows of Hurricane Sandy: uncovering the sociospatial dimensions of ‘big data’. Geoforum 52, 167–179. doi: 10.1016/j.geoforum.2014.01.006
Spence, P. R., Lachlan, K. A., Lin, X., and del Greco, M. (2015). Variability in twitter content across the stages of a natural disaster: implications for crisis communication. Commun. Q. 63, 171–186. doi: 10.1080/01463373.2015.1012219
Starbird, K., and Palen, L. (2010). “Pass it on?: Retweeting in mass emergency,” in Proceedings of the International Community on Information Systems for Crisis Response and Management, Seattle, 1–10.
Stefanidis, A., Crooks, A., and Radzikowski, J. (2013). Harvesting ambient geospatial information from social media feeds. GeoJournal 78, 319–338. doi: 10.1007/s10708-011-9438-2
Takahashi, B., Tandoc, E. C., and Carmichael, C. (2015). Communicating on Twitter during a disaster: an analysis of tweets during Typhoon Haiyan in the Philippines. Comp. Hum. Behav. 50, 392–398. doi: 10.1016/j.chb.2015.04.020
Thompson, P. R., Merrifield, M. A., Wells, J. R., and Chang, C. M. (2014). Wind-driven coastal Sea Level Variability in the Northeast Pacific. J. Clim. 27, 4733–4751. doi: 10.1175/JCLI-D-13-00225.1
Thompson, P. R., Piecuch, C. G., Merrifield, M. A., McCreary, J. P., and Firing, E. (2016). Forcing of recent decadal variability in the Equatorial and North Indian Ocean. J. Geophys. Res. Oceans 121, 6762–6778. doi: 10.1002/2016JC012132
Van Zandt, S., Peacock, W. G., Henry, D. W., Grover, H., Highfield, W. E., and Brody, S. D. (2012). Mapping social vulnerability to enhance housing and neighborhood resilience. Hous. Policy Debate 22, 29–55. doi: 10.1080/10511482.2011.624528
Verdi, R. J., Lotspeich, R. R., Robbins, J. C., Busciolano, R. J., Mullaney, J. R., Massey, A. J., et al. (2017). The surge, wave, and tide hydrodynamics (SWaTH) network of the U.S. Geological Survey—Past and future implementation of storm-response monitoring,data collection, and data delivery. U.S. Geol. Surv. Circ. 1431:35. doi: 10.3133/cir1431
Wahl, T., Jain, S., Bender, J., Meyers, S. D., and Luther, M. E. (2015). Increasing risk of compound flooding from storm surge and rainfall for major US cities. Nat. Clim. Change 5, 1093–1097. doi: 10.1038/nclimate2736
Weichselgartner, J. (2001). Disaster mitigation: the concept of vulnerability revisited. Disast. Preven. Manag. Int. J. 10, 85–95. doi: 10.1098/rsta.2014.0373
Wright, L. D. (2019). “Future Societal Vulnerability, Risk and Adaptability,” in Tomorrow’s Coasts: Complex and Impermanent. Coastal Research Library, eds L. Wright and C. Nichols (Cham: Springer), 293–304. doi: 10.1007/978-3-319-75453-6_18
Wright, L. D., and Nichols, C. R. (eds) (2019). Tomorrow’s Coasts: Complex and Impermanent. Coastal Research Library Series. Cham: Springer.
Wright, L. D., Nichols, C. R., Cosby, A. G., and D’Elia, C. F. (2016). Collaboration to Enhance Coastal Resilience. Lisbon: Eos.
Wright, L. D., and Thom, B. G. (2019). “Promoting Resilience of Tomorrow’s Impermanent Coasts,” in Tomorrow’s Coasts: Complex and Impermanent. Coastal Research Library, eds L. Wright and C. Nichols (Cham: Springer), 341–353. doi: 10.1007/978-3-319-75453-6_21
Keywords: coastal observations, numerical models, coastal flooding, big data, collaboration, community vulnerability, climate change, urban coasts
Citation: Nichols CR, Wright LD, Bainbridge SJ, Cosby A, Hénaff A, Loftis JD, Cocquempot L, Katragadda S, Mendez GR, Letortu P, Le Dantec N, Resio D and Zarillo G (2019) Collaborative Science to Enhance Coastal Resilience and Adaptation. Front. Mar. Sci. 6:404. doi: 10.3389/fmars.2019.00404
Received: 30 October 2018; Accepted: 28 June 2019;
Published: 12 July 2019.
Edited by:
Laura Lorenzoni, University of South Florida, United StatesReviewed by:
Lisa Clough, National Science Foundation, United StatesCristina Carollo, Independent Researcher, Cape Charles, VA, United States
Copyright © 2019 Nichols, Wright, Bainbridge, Cosby, Hénaff, Loftis, Cocquempot, Katragadda, Mendez, Letortu, Le Dantec, Resio and Zarillo. This is an open-access article distributed under the terms of the Creative Commons Attribution License (CC BY). The use, distribution or reproduction in other forums is permitted, provided the original author(s) and the copyright owner(s) are credited and that the original publication in this journal is cited, in accordance with accepted academic practice. No use, distribution or reproduction is permitted which does not comply with these terms.
*Correspondence: Lynn D. Wright, d3JpZ2h0QHN1cmEub3Jn