- 1National Oceanography Centre, Southampton, United Kingdom
- 2Met Office Hadley Centre, Exeter, United Kingdom
- 3Department of Atmospheric Sciences, University of Washington, Seattle, WA, United States
- 4Shirshov Institute of Oceanology, Russian Academy of Sciences, Moscow, Russia
- 5National Oceanic and Atmospheric Administration, National Centers for Environmental Information, Asheville, NC, United States
- 6Center for Ocean-Atmospheric Prediction Studies, Florida State University, Tallahassee, FL, United States
Observations of conditions at the ocean surface have been made for centuries, contributing to some of the longest instrumental records of climate change. Most prominent is the climate data record (CDR) of sea surface temperature (SST), which is itself essential to the majority of activities in climate science and climate service provision. A much wider range of surface marine observations is available however, providing a rich source of data on past climate. We present a general error model describing the characteristics of observations used for the construction of climate records, illustrating the importance of multi-variate records with rich metadata for reducing uncertainty in CDRs. We describe the data and metadata requirements for the construction of stable, multi-century marine CDRs for variables important for describing the changing climate: SST, mean sea level pressure, air temperature, humidity, winds, clouds, and waves. Available sources of surface marine data are reviewed in the context of the error model. We outline the need for a range of complementary observations, including very high quality observations at a limited number of locations and also observations that sample more broadly but with greater uncertainty. We describe how high-resolution modern records, particularly those of high-quality, can help to improve the quality of observations throughout the historical record. We recommend the extension of internationally-coordinated data management and curation to observation types that do not have a primary focus of the construction of climate records. Also recommended is reprocessing the existing surface marine climate archive to improve and quantify data and metadata quality and homogeneity. We also recommend the expansion of observations from research vessels and high quality moorings, routine observations from ships and from data and metadata rescue. Other priorities include: field evaluation of sensors; resources for the process of establishing user requirements and determining whether requirements are being met; and research to estimate uncertainty, quantify biases and to improve methods of construction of CDRs. The requirements developed in this paper encompass specific actions involving a variety of stakeholders, including funding agencies, scientists, data managers, observing network operators, satellite agencies, and international co-ordination bodies.
Introduction
Observations of environmental conditions near the ocean surface have been made from ships for centuries, and more recently from a wider range of observing platforms, including satellites. This paper will introduce the different types of measurements that have been made and describe the methods used to assemble the observations to generate records that can be used to characterize the changing conditions over the oceans. The most well-known long term marine climate record is that of sea surface temperature (SST), but there are also observations of air temperature, pressure, humidity, wind, clouds, waves, and weather conditions that have been used to generate climate records.
Most in situ surface marine climate records are based on the International Comprehensive Ocean-Atmosphere Data Set (ICOADS, Freeman et al., 2019), presently on Release 3.0 (Freeman et al., 2017). ICOADS is the most complete archive of its kind presently available and forms the basis for our discussion.
The requirements for data and metadata to construct long term climate records are hard to summarize in a simple form. Requirements for climate monitoring are collated by the Global Climate Observing System (GCOS) as part of the Observing Systems Capability Analysis and Review Tool (OSCAR) requirements database. The World Meteorological Organization (WMO) operate a “Rolling Review of Requirements (RRR)” in which user requirements for observations are compared with the capabilities of present and planned observing systems. For climate monitoring the outcomes of this review are published as reports on observing system status (GCOS, 2015) and requirements (GCOS, 2016). OSCAR considers a range of different application areas, other areas related to climate include climate science, applications and services, but climate monitoring is the most relevant to the construction of long-term climate records. The OSCAR/RRR process presents requirements for each variable as a desired accuracy at a chosen space and time resolution, plus a temporal stability. This is well-suited to measurements derived from satellites, but problematic for marine in situ measurements from mobile platforms (Berry and Kent, 2017). This paper therefore discusses the wide range of considerations underlying the development of accurate and stable long-term records in the context of an error model. Examples of the types of information that feed into the error model and therefore have an impact on the observing requirements are the availability of metadata describing measurement methods and protocols, and requirements for ancillary observations, for example for bias estimation or height adjustment. There are a range of other organizations concerned with the development of user requirements for surface marine data, for example the Copernicus Climate Change Service, the European Space Agency Climate Change Initiative, and groups focused on particular variables such as SST (the Group for High Resolution SST) and winds (the International Ocean Vector Winds Science Team). Where relevant to climate monitoring and the development of long-term climate records requirements from such groups feed into the WMO RRR.
The GCOS defines three different types of observation networks: reference, baseline, and global (GCOS, 2016). These give a hierarchy of high quality traceable measurements at limited locations (reference network), through good quality measurements made more widely (baseline), to less accurate but widespread measurements to capture the important scales of variability (global). Such a suite of measurements can be combined to give the quality and coverage to provide stable, long term, climate records. The task is to assess the accuracy and sampling required for the different networks and how well the available historical observations map onto the requirements. Only then will it be possible to specify requirements for the continuation of long term records with the future observing system, and for prioritization of data and metadata recovery to improve the historical record.
Scope and Terminology
Here we consider the construction of climate data records (CDRs) of physical parameters observed near the ocean surface. These include the GCOS Essential Climate Variables (ECVs, Bojinski et al., 2014; GCOS, 2016) and Global Ocean Observing System (GCOS, 2018) Essential Ocean Variables (EOVs). Hereafter, we will refer to this combination of ECVs and EOVs simply as ECVs. Specifically we consider SST, marine air temperature (MAT), humidity, wind speed and direction, atmospheric sea level pressure (SLP) and also sea state and cloud parameters. Our criterion in this paper for considering a CDR to be long is greater than about 50 years, longer than the recent period with extensive satellite observations, and ideally centennial or longer.
This scope presently excludes the consideration of records derived from satellite data only as these have a maximum record length of about 40 years and are the subject of other papers in this issue (e.g., Ardhuin et al., 2019; Bourassa et al., 2019; O’Carroll et al., 2019). We note that many of the themes contained in this paper are relevant to both in situ and satellite data. It is critically important that high-quality satellite ECV records are maintained, evaluated and characterized with uncertainty estimates to create stand-alone CDRs, to enable the construction of long records jointly with in situ observations, to provide estimates of ECV variability and also data for evaluation. The Committee for Earth Observation Satellites (CEOSs) and the Coordination Group for Meteorological Satellite (CGMS) Joint Working Group on Climate provide coordination for activities related to the construction of CDR from satellites.
It is helpful to define some terminology for this paper.
Long term: about 50 years or ideally longer.
Large-scale: ranges from several degrees latitude/longitude to global scale.
Data: a collection of individual observations or measurements including information on date, time, and location.
Platform: any type of structure from which observations are made. Platforms providing records longer than 50 years include various types of ship, and fixed platforms such as rigs or coastal stations. Platforms providing shorter records include moored and drifting buoys, satellites, autonomous profilers, and surface vehicles.
Metadata: information describing characteristics of the platform, instrument, environmental conditions, observing protocols or data management that are helpful for interpreting the observations and estimating their uncertainty.
Climate data record: a time series of measurements of sufficient length, consistency, and continuity to determine climate variability and change and ideally accompanied by estimates of uncertainty and its correlation structure.
Gridded analysis: a timeseries of fields on a regular spatial grid, sometimes in-filled, typically at monthly or daily resolution, constructed from CDRs and ideally accompanied by estimates of uncertainty in the gridded values and the correlation structure of the uncertainty.
Data product: usually a gridded analysis, CDR or collection of data enhanced with derived variables or metadata.
Introduction to the Generation of Climate Data Records (CDRs) and Data Products
Figure 1 shows in schematic form the process involved in constructing a long-term dataset. In summary this consists of the following steps:
Step 1 – understand what is required by different end users, prioritize
Step 2 – gather together available data and metadata, or digitize new data
Step 3 – determine the structure of the error model for each observation type and source and estimate contributing uncertainties and their dependencies
Step 4 – clean up and bias adjust observations, propagating uncertainties and assessing uncertainty in the adjustments
Step 5 – compare measurements from different components of the observing system in the context of their estimated uncertainties and user requirements, revisit steps 2–5 (and possibly 1) if needed
Step 6 – produce data products tailored for particular applications
Step 7 – evaluate products by comparison with withheld observations or independent reference data if available, and with other similar data products if available
Step 8 – disseminate products with appropriate metadata, uncertainty estimates and documentation.
As shown in Figure 1 this should be an iterative process, as changes to any part of the system will affect successive parts, and all can be refined by iteration.
Structure of This Paper
A general error model is first introduced along with an overview of approaches to uncertainty estimation and the generation of gridded and gap-filled data products (Section “An Error Model and Its Application”). The section “Overview of Available Data and Observational Metadata” describes the main types of platforms providing data and ancillary information relevant for the construction of surface marine CDRs, how each contributes to CDR construction and how the available observations might be improved and extended. The next section considers “User Needs for Data and Data Products” followed by an overview of approaches to the construction of CDRs, including summaries of specific issues for each ECV (Section “Considerations When Creating Internally-Consistent Records for Marine Surface ECVs”). The final sections look to the future and provide reflections and recommendations.
An Error Model and Its Application
Introduction to an Error Model for Individual Observations
The observed value of measurand (O) at location co-ordinates (x, y), date and time (t) and observing height or depth (h), hereafter location (x, y, t, h) is an approximation to the true value (T) at a desired nearby location (x′, y′, t′, h′). The definition of “nearby” will depend on the application, for example on the size of output grid or distance from a reference observation. The observed value will contain systematic and random errors (e.g., JCGM, 2008), and is an approximation for T. It may be possible to estimate the component due to systematic errors (B). Similarly, it may be possible to estimate the component due to differences in location or spatiotemporal representativeness (L). In each case we wish to correct the observations for bias or location, leaving residual errors εb and εl respectively. The local random errors, after any adjustment, can be represented by εo.
The systematic errors (B, hereafter bias) may depend on location, the general measurement method (m), the specific instrument used (i), the platform (p), the ambient environmental conditions at the time of the observation (a), and how the data were recorded, transmitted, exchanged, stored and archived (d) or other factors. The differences due to location (L) will depend on the gradients in the field of interest and on the distance. Examples of such location adjustments are to adjust observations to a common reference level (such as the adjustment of marine air temperature measurements to a common reference level, Kent et al., 2013), or time (such as the adjustment of satellite observations of SST to a common reference time to remove inhomogeneity in retrievals due to changing overpass times relative to local time of day, Merchant et al., 2014). Following correction for location differences and systematic observational errors our best estimate is that all error terms (εo, εb, εl) will have zero mean across a large number of observations, but there may be structural relationships between subsets of errors that might be, for example, correlated across x, y, t, m, i, p, a, or d.
With the residual difference between our observed and approximated value given by:
Where the overbar indicates an estimated value of the systematic () and location errors (). Estimates of T and gradients in T will have further dependencies, for example on x, y, t, h, or a.
Typically we also need estimates of the expected error variances() and their covariance structure. The random errors are usually considered independent and the covariance structure between observations takes the form of a diagonal covariance matrix. The covariance structures for the other error terms are more complicated. For example, the residual bias and location errors may be considered to be fully correlated across observations sharing particular characteristics (e.g., same m, i, p, a or d or nearby) and uncorrelated otherwise (JCGM, 2008).
The general error model informs data requirements. The most obvious requirement is for observations (O) of the parameter of interest at known dates, times, and locations. Next is for information on the methods and instruments used in order to accurately estimate any bias correction () and any auxiliary information required to calculate the location adjustment (), for example atmospheric stability for the air temperature height adjustment. The number of observations required will depend on the objective (e.g., location or region, spatiotemporal resolution, and desired accuracy), and on the uncertainty in the observations (and its correlation structure). These requirements will be discussed further for specific variables in Section “User Needs for Data and Data Products.” In addition to estimates of the adjustments required for biases and location differences we also need estimates of the expected variance of the residual errors, these are addressed in the next section.
Approach to Uncertainty Estimation
Historical data vary in quality and have a variety of errors. Understanding these errors, the degree to which they can be corrected, and the residual uncertainty associated with the adjusted measurements is essential for making use of the data. Quantification of uncertainties is likely to reveal complex dependencies of (e.g., on x, y, t, h, m, i, d, p, a).
There are two primary ways of estimating the uncertainty in the observations, either through the direct observation and estimation of the probability density functions for the different errors (Type A) or through the use of assumed probability density functions based on other evidence (Type B) (JCGM, 2008). Instruments calibrated to international (and national or community) standards will conform with the Type A approach prior to deployment. However, even if international standards are followed and an instrument installed on a ship is accurate, siting of the instrument and misreporting can still lead to significant errors (e.g., Beggs et al., 2012). Drifting buoys are rarely recovered, so their calibration chain is effectively broken upon deployment. Moored buoys, such as those in the TAO array, are periodically recalibrated, but detailed calibration information is not presently delivered alongside the observations.
Typically, other solutions following the Type B approach are required. For example, one solution for detecting drifts in the calibration of sensors on drifting buoys is to fit each drifter with multiple sensors (Reverdin et al., 2010; Poli et al., 2018). Another solution is to compare coincident or nearly coincident measurements from different instruments such as using variograms (Kent and Berry, 2005). Triple co-locations (e.g., Stoffelen, 1998; O’Carroll et al., 2008) can be used in situations where errors can be considered to be independent. Application of such techniques requires redundancy in observing systems and consideration of the correlation structure of the errors. Comparisons between nearly coincident measurements are also important for quality control and bias estimation.
Quality control systems such as the in situ SST quality monitor (iQuam) Atkinson et al. (2013) and Xu and Ignatov (2016) use comparisons between in situ measurements and a reference field derived from a satellite-based analysis to quantify biases and standard deviations for individual platforms, which are effectively estimates of uncertainties. Others (for example Kent et al., 1993; Stoffelen, 1998; Ingleby, 2010) have used weather forecast fields as a basis for estimating measurement errors and uncertainties. More modern statistical infilling techniques can also be used to estimate and assess errors and uncertainties (see, e.g., Kent et al., 2017) but it is challenging to implement methods with complex dependencies globally and with high data volumes, for example, as associated with satellite data.
The Construction of Gridded and Gap-Filled Records
One of the main requirements for long-term CDRs is the creation of datasets containing summary statistics, such as the mean, on a regular grid. These gridded products tend to be more usable than the individual observations for many applications and are widely used. The simplest gridded products are based on the arithmetic mean of all observations within a grid box passing quality control. Examples include the ICOADS summaries (Freeman et al., 2017) and HadSST3 (Kennedy et al., 2011a,b). Such products are typically easy to use and maybe relatively easy to produce, but suffer from a variety of problems such as unresolved biases, incomplete coverage and inhomogeneous variance in time and space owing to the vastly different sampling density in different times and places. Gridbox uncertainty estimation should account for all important components of the appropriate error model for the observation types used, and will typically require observational and platform metadata to correctly represent the expected error covariances. Kennedy et al. (2011a) showed large increases in the uncertainty in the global average SST associated with measurement error when covarying errors were taken into account.
Simple gridded products often form the basis of more sophisticated data treatments. A number of data sets interpolate or fill in gaps in the data, mostly for SST (e.g., ERSSTv5, Huang et al., 2017; COBE-SST2, Hirahara et al., 2014; HadISST, Rayner et al., 2003; Kaplan SST, Kaplan et al., 1998), but also for pressure (HadSLP2, Allan and Ansell, 2006). For filling of large-scale gaps as is required particularly in the 19th century (Figure 2A), these reconstruction methods typically use patterns (e.g., principal components or empirical orthogonal functions) derived from modern well-sampled data to reconstruct sparsely observed past climate states. The advantage of having globally or regionally complete fields is obvious and such analyses are widely used. A number of generic difficulties are expected and seen in such analyses including loss of variance, under-estimation of trends, over-fitting, and under-estimation of uncertainty. This highlights the difficulty of representing the broad spectrum of possible climate states from the relatively small and noisy sample of observed historical variability. In addition, these methods do not typically take into account the full range of error structures seen in the data and typically assume that errors are uncorrelated. Smaller-scale gaps and smoothing of data can also use techniques based on describing the expected local structure of variability such as optimal interpolation (e.g., Reynolds et al., 2002) or using a statistical model of the mid-scale variability (Karspeck et al., 2012).
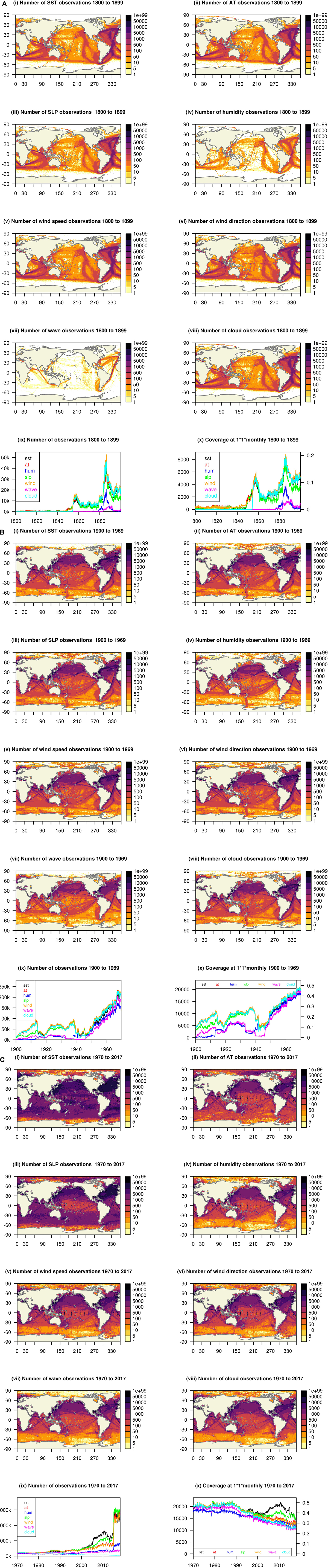
Figure 2. (A) Total number of observations on a 1∘ latitude-longitude grid for selected ECVs from ICOADS Release 3.0 over the period, 1800–1899. The lower panels show (ix) the total number of monthly observations and (x) the number of 1∘ monthly areas sampled (left axis) and fraction of ocean 1∘ areas (right axis). No QC or other data selection has been applied. (B) As 2a but for 1900–1969. (C) As 2a but for 1970–2017.
One aspect of gap-filling that has not yet been widely used is exploitation of co-variability between different variables (except in the context of dynamical reanalysis production which exploits physical relationships between variables via assimilation into a weather forecasting model). Examples where this is likely to be useful include: joint analysis of winds and pressure; pressure or atmospheric circulation and temperature, humidity or cloud cover; and of SST, MAT, and humidity.
Overview of Available Data and Observational Metadata
The Different Types of Observing Platforms
Figure 2 summarizes data availability based on the ICOADS Release 3.0 from each of the in situ observation types considered in Section “The Different Types of Observing Platforms.” Figure 2A shows sampling by ECV for 1800 to 1899, a period dominated by ship observations (Figure 3). Reports typically contain several ECVs with humidity and sea state reported less frequently than SST, MAT, SLP or winds. Numbers of observations and coverage generally increase over time, with peaks associated with the start of international co-ordination (Maury, 1854) and the ingest of a large collection of US logbooks. Observation numbers and coverage both increase over 1900 to 1969 (Figure 2B) with decreases associated with the two world wars. There is an increasing contribution from meteorological observations associated with oceanographic measurements (Boyer et al., 2013). Humidity and waves remain less well-sampled than the other variables, their coverage depending on the sources of data in this period. Since 1970 the range of platform types diversifies (Figure 3) but while the number of observations increases, the coverage is lower by 2017 than in 1970 for all ECVs (Figures 2C, 3). This is due to a declining contribution by ships since the 1990s, partially compensated for SST and SLP by an increase in coverage from drifting buoys (noting that ICOADS is currently missing some drifting buoy observations from 2016 onwards).
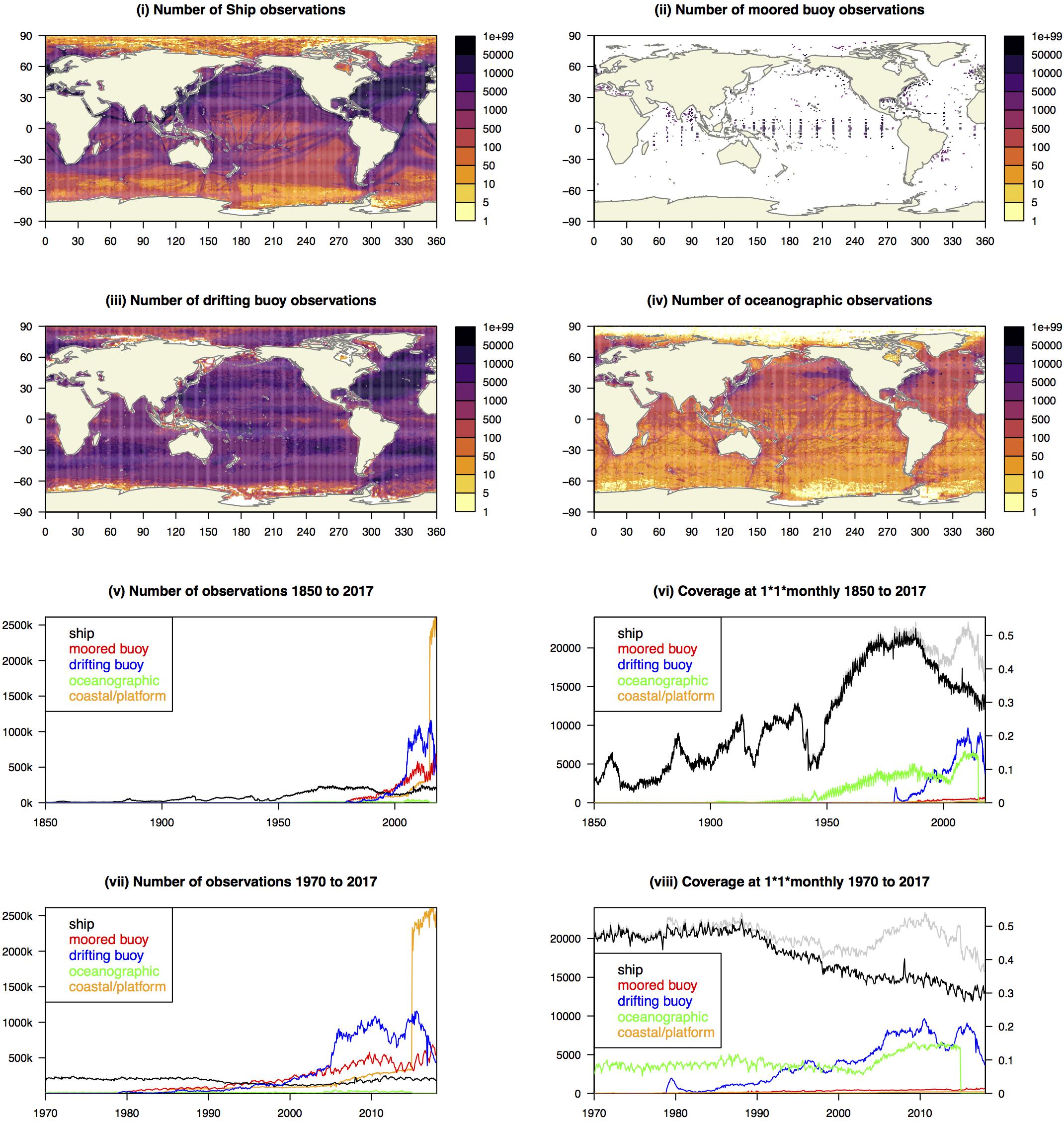
Figure 3. Number of observations between 1850 and 2017 (as Figure 2) but by ICOADS platform type (PT): ships (PT 0-5); moored buoys (PT 6), drifting buoys (PT 7), oceanographic observations (PT 10-12, 19-21), and coastal and other platforms (9, 13-16). Note that some missing PT have been estimated based on data sources. The gray line in panels vi and viii shows the coverage across all platforms. Panels vii and viii show the same information as v and vi but starting in 1970. Oceanographic data are ingested into ICOADS in delayed mode, data for 2015 onwards will be ingested in the next ICOADS release.
Non-specialist Observing Ships
The creation of long records depends on combining many different types of observations. Many ship observations were originally made for ship operations or navigation, or in support of numerical weather prediction (NWP). Some observations arise from programs that did aim to understand marine climate variability, but typically to chart long term mean conditions (climatology) such as Maury (1854) or the WMO Marine Climatological Summaries Scheme (MCSS, WMO, 2012). The amount of information we have for such vessels varies dramatically with the data source. For observations digitized recently the value of multi-variate records with rich metadata is recognized and typically an attempt is made to recover all relevant information. However, in the past data and metadata are often lost through the many format conversions that observations may have been subjected to.
The earliest observations come from digitization of observations from individual voyages of discovery (Woodruff et al., 2005). The earliest systematic observations come from logbooks of the East India Company covering the period 1789 to 1834 (Freeman et al., 2017) and then from international co-ordination of marine observing by Maury (1854). This international co-ordination is now under the WMO Voluntary Observing Ships (VOSs) scheme (WMO, 2012). Presently data from VOS are collected in near real time (NRT) in support of NWP, via the WMO Global Telecommunications System (GTS). Archives of GTS-derived reports are kept by several National Weather Services (NWSs) but there is no systematic international system that ensures completeness and quality of these records that become the mainstay of the climate observing system.
Some VOS observations are also made available in delayed mode with additional parameters and improved quality control through dedicated WMO Global Assembly Centers (GDACs) in the United Kingdom and Germany. Over the years ICOADS has acquired major collections of surface marine observations from prior to the VOS scheme that were not collected by Maury, notable examples are from the United States, Japan (Kobe collection), Norway, and Australia (Manabe, 1999; Worley et al., 2005).
Specialist Observing Ships
Observations from specialist vessels obviously have the potential for providing higher quality observations than non-specialist ships. However, in order for such observations to be most useful for the construction of long term records the data must be clearly identifiable and accompanied by extensive platform and observational metadata. ICOADS contains observations from research vessels (RVs), ocean weather ships (OWSs), and light vessels (LVs) that have the potential to be used in a variety of ways either as a source of observations in sometimes sparse ocean regions, or as high quality data for evaluation (e.g., Smith et al., 2001).
International Comprehensive Ocean-Atmosphere Data Set mainly contains observations from RVs operating as VOS and using a separate set of instruments from those used for research applications. In some cases, particularly in the US, VOS observations from RVs may come from the research instruments. Additionally, some RV data in ICOADS are from delayed-mode data sources that are focused on collection and quality evaluation of measurements from research instrumentation (e.g., Smith et al., 2018). There is the potential for some confusion as to the source of any observations identified as coming from RVs by their identifiers (callsign or ship name, hereafter IDs). In some cases, the same RV may provide VOS and delayed mode observations from two different instrument systems. A similar situation exists for OWS, which can be found in ICOADS in several different source data collections. Some OWS observations are clearly identified as such, either through being identified with a specialist source, or through the ICOADS “platform type” identifier (PT). However some ICOADS data sources contain mixtures of OWS and other data types, not always clearly identified, some duplicating observations in dedicated OWS sources likely to be of higher quality and completeness, and some that may be unique.
Data from RVs are typically managed at the national level with no dedicated international data management or archival system (Smith et al., 2019). Some nations do have dedicated data management systems for RVs including the US (Smith et al., 2018) and Australia [Integrated Marine Observing System (IMOS)], full international integration of such national RV data systems would enable the construction of high quality datasets for evaluation of a wide range of data and data products.
Data from specialist observing ships could have huge value for the development of long-term datasets, but the lack of an integrated international management system for RV data means that observations are not well-utilized for uncertainty estimation and quality evaluation.
Moored Buoys
Moored buoy observations have contributed to the global observing system since the 1970s (Figures 2C, 3) and are found in ICOADS in data sources deriving from both NRT and delayed mode archives. The two largest sources of moored buoy observations are the Global Tropical Moored Buoy Array (GTMBA) providing measurements in the Pacific, Atlantic and Indian Oceans (McPhaden et al., 1998) and the coastal network of buoys that make observations in support of NWP (DBCP, 2016). GTMBA data are curated by the NOAA Pacific Marine Environmental Laboratory (PMEL) who provide consolidated access to data from the full tropical array1 (accessed 26 March 2019). ICOADS ingested the GTMBA archive as of February 2016 for Release 3.0 which included data collected up to the end of 2014 (Freeman et al., 2017). The PMEL archive is updated when improved information such as from post-calibration becomes available, but updates and changes to the archive are not prominently publicized.
Observations from national coastal networks have not been a priority for historical curation, and no definitive archive for the data and metadata exist. Whilst potentially valuable, a combination of mixed data quality with many moorings sited in highly-variable coastal locations has meant that these moorings have not been widely exploited for climate applications (e.g., Wentz et al., 2017).
The OceanSITES aim is to collect, deliver and promote the use of high-quality data from long-term, high-frequency observations at fixed locations in the open ocean. Its scope is wider than considered here, including also biogeochemical and subsurface observations. OceanSITES funds a Technical Coordinator and IT staff who are based at the JCOMMOPS Project Office (OceanSITES, 2016). OceanSITES collates timeseries data from many different providers, and makes it available in a consistent format (OceanSITES, 2015) via two Data Assembly Centres. Several of the GTMBA moorings are designated as OceanSITES as are long-term moorings from the Woods Hole Oceanographic Institution.
Other Moored and Fixed Platforms
Observations made in coastal regions and from fixed platforms such as oil rigs can also contribute to the marine climate record (Figure 3). Land-based coastal observations are often excluded from CDRs and other data products as unrepresentative of open ocean conditions, but have a role to play for example for evaluation of datasets (see Hanawa et al., 2000; Kent et al., 2017; Cowtan et al., 2018 in the context of SST).
Surface Drifters
Surface drifters predominantly report SST and SLP (Figures 2C, 3), providing critical data for NWP and reanalysis (Centurioni, 2018). The development of the surface drifter observing system has been facilitated by clear user requirements (e.g., Zhang et al., 2006) and assessment of the impact of the data (e.g., Ingleby and Isaksen, 2018). However the reliance on surface drifters has led to a decline in observations of important ECVs such as MAT, humidity, wind, and cloud (Kent et al., 2006, also Figure 2C). Some drifting buoys have reported MAT, but their quality has not yet been evaluated. There is the potential for correlated sampling uncertainty as drifters may get trapped in eddies and will follow currents. They cannot provide good sampling in regions of divergence or areas of upwelling. Lack of multivariate sampling can be problematic for analysis, for example Morak-Bozzo et al. (2016) associated model output with drifting buoy measurements to characterize the dependencies of their diurnal cycles.
Oceanographic or Profile Measurements for Temperature
Historically, typically from 1950 to 2000s, ocean temperature was measured by reversing thermometers (attached to hydrographic bottles), conductivity–temperature–depth (CTD), mechanical bathythermographs (MBTs), and expendable bathythermographs (XBTs). Globally, there were 5000–20,000 measurements within 5 m of the ocean surface each month from 1950 to 2000s (Figure 4A), which covers 5–20% area of the ocean on 2∘ × 2∘ grids (Figure 4B). Argo floats provide highly accurate temperature measurements but observations are sparse due to the typical 10 day sampling cycle. Argo data are only available for regions where the ocean is deeper than ∼2 km.
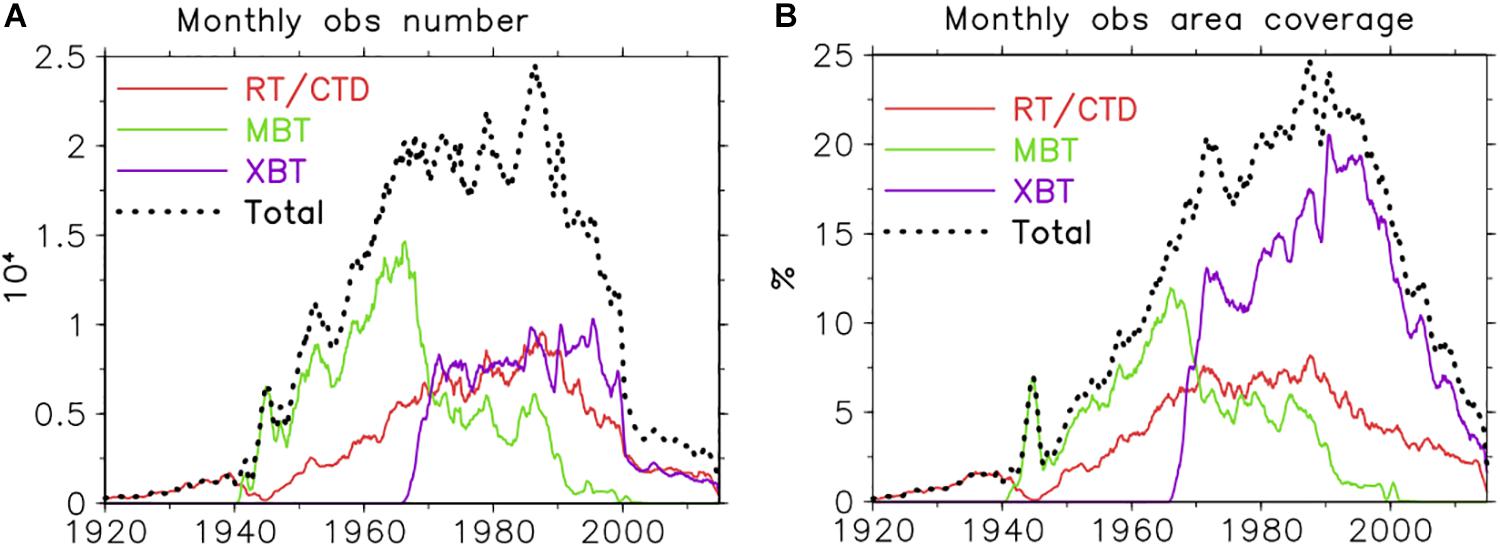
Figure 4. (A) Number and (B) area coverage of monthly measurements from RT/CTD (solid red), MBT (solid green), XBT (solid purple), and total (dotted black) in the global oceans.
These measurements were commonly used to estimate ocean heat content (e.g., Levitus et al., 2009), but XBT are problematic for SST analysis due to biases caused by the shock of entry into the water. Recent studies have however (e.g., Gouretski et al., 2012; Hausfather et al., 2017; Berry et al., 2018; Huang et al., 2018) have used these measurements to evaluate SST data products.
Satellites
Data from satellites have become an important resource for climate. Their record length is presently not yet sufficient on their own to meet our definition of a long term climate record. Increasingly CDRs will be constructed from satellite observations without blending with in situ observations, and the same considerations for stability and uncertainty will apply. In situ data are also required for calibration and validation of satellite climate records (e.g., Belmonte Rivas et al., 2007; Berry et al., 2018). As with in situ networks, satellite missions have not always been designed with climate applications foremost, so typically substantial work has to go into constructing stable records (Hollmann et al., 2013; Verhoef et al., 2017). However there are now efforts to establish and maintain traceability of global fiducial reference measurements, including for satellite-derived surface temperature (Snook, 2016).
Sea surface temperature is one ECV where satellite data has been most used for the construction of CDR, notably in the HadISST dataset (Rayner et al., 2003). Substantial effort has been put into the construction of stable and accurate SST records (Merchant et al., 2012, 2014). Other variables where satellites will play an important part in the construction of long-term CDR are for clouds and radiation (e.g., Loeb et al., 2012), winds (e.g., Verhoef et al., 2017), and waves (e.g., Young et al., 2011; Ardhuin et al., 2019). However, to date only SST has extended the satellite record back in time using in situ observations. Air temperature and humidity are hard to derive from space (e.g., Andersson et al., 2011; Prytherch et al., 2015) but doing so would be valuable for estimation of air-sea exchanges (Weller, 2018; Cronin et al., 2019).
The satellite community are important users of in situ-measured surface ECVs (e.g., Belmonte Rivas et al., 2007; Stoffelen et al., 2015; Jackson and Wick, 2016; Kinzel et al., 2016; Berry et al., 2018; Liman et al., 2018; Thorne et al., 2018), and recommendations that aim to improve the quantity, quality, and consistency of in situ data will be of huge benefit for satellite calibration and evaluation.
Selection of Data Sources
Selection of in situ Observations for the CDR
The construction of long records inevitably means that a range of data sources needs to be considered and that there will be compromises on data quality to increase sampling. Most modern in situ-based surface marine climate records are built using the ICOADS (Freeman et al., 2017). ICOADS collates surface marine data from a range of different observing platforms, keeping the majority of available parameters and metadata together. Obvious requirements are measurements or visual estimates of the parameter of interest, along with information about the date and time of the observation and its location. Observations in ICOADS may have substantial uncertainty in their locations, dates, and times. Some data sources have positions recorded to the nearest degree, and some positions, dates and times have gross errors (Carella et al., 2017). Increasingly information on the identity of the measurement platform is used, for quality control, but also as part of bias adjustment and the construction of error covariances in gridded analyses. Observational metadata giving measurement methods, heights or depths of sensors is also valuable, but can be estimated (e.g., Kennedy et al., 2011a; Kent et al., 2013; Carella et al., 2018). It is also becoming more common to use information on the ambient environmental conditions as input to bias adjustment schemes and uncertainty estimation, which may further require estimates of ship speed and course, winds, cloud, temperature, humidity, pressure, wave conditions, and coded weather information. The availability of a full suite of estimates of environmental conditions also permits multivariate quality control.
Despite ICOADS providing all the information in a common format, the content and quality of records from different sources varies markedly. Much of the data provided in ICOADS (then COADS) Release 1 came from data that had been initially recorded in ships logbooks, then stored on punchcards and later transitioned to reel-to-reel tapes (Woodruff et al., 1987). Each format change and re-archival inevitably results in some degree of lost information, and the introduction of transcription errors. The present ICOADS format [International Marine Meteorological Archive (IMMA) format version 1] (Smith et al., 2016) allows the preservation of the entire record, but at cost of considerable complexity. Recently ingested data, such as that from the GTS or data recovery (Allan et al., 2011) therefore can retain a more complete record of the original data and observational metadata.
The amount of information we have for such vessels varies dramatically with the data source, summarized in ICOADS by the DCK (derived from a “deck” of punch cards) and SID (source identifier) indicators. Presently the information required to select observations from ICOADS based on an objective quantification of the quality of the data and metadata needed to construct CDRs is not available. A reprocessing of ICOADS to improve its compatibility with WMO Integrated Global Observing System (WIGOS) data and metadata standards would substantially improve this deficit.
Observations for Bias and Location Adjustment and Uncertainty Estimation
Methods for the estimation of data uncertainty such as the calculation of variograms (e.g., Kent and Berry, 2005) are based only on the variability of observations themselves, although these may be calculated for subsets of data, for example by measurement method. Bias estimation may use measures of internal consistency between subsets of measurements made using different methods (e.g., Folland and Parker, 1995; Kent and Kaplan, 2006; Kennedy et al., 2011a; Hirahara et al., 2014; Carella et al., 2018), between different platforms, or based on other characteristics (Chan et al., 2019), requiring metadata to identify appropriate subsets.
High quality observations are important for the detection and evaluation of biases (Hausfather et al., 2017; Berry et al., 2018). Specially designed datasets are also useful, often containing enhanced metadata (Kent et al., 1993; Berry and Kent, 2005), and/or co-located observations from different methods (James and Fox, 1972; Berry et al., 2004). Comparison of data products of similar ECVs that are likely to have largely independent errors (for example SST and MAT) can be used for bias adjustment (Huang et al., 2017) or evaluation (Cowtan et al., 2018).
Nearby observations are needed for some types of QC (see section “Quality Control”) but can also be used to diagnose relative data biases (e.g., Thomas et al., 2005; Chan and Huybers, 2019).
Observations of ambient environmental conditions which may be based on measurements or climatology are needed for estimation of B, L and their uncertainties, and may also feed into estimation of other components of uncertainty. Coded weather information is particularly useful for identifying ambient conditions, for example whether or not it is raining.
Observations for Variability
Estimates of local ECV variability and gradients are needed for quantification of location uncertainty, as input to QC and for implementation of local gap-filling and smoothing algorithms. Estimates of large-scale variability are needed to provide patterns of expected modes of variability for reconstructions. Estimates of temporal variability are often based on in situ observations, moored buoys are particularly good for quantification of high-frequency temporal variability, and for understanding co-variability among different ECVs. Many ECVs show diurnal variability, both real and spurious. Real diurnal variations are often not considered, but ideally variations in local sampling time should be assessed as part of L. Satellite observations can be valuable for the estimation of spatial variability, but for variables such as MAT and humidity can only be assessed from VOS observations at present (Figures 2C, 3).
Observations for Evaluation
Ideally evaluation would use high-quality independent data widely distributed and spanning the entire period of record. These data would also be independent of those used for evaluation of biases and uncertainty. Examples where such evaluation has been possible are largely limited to SST using observations from Argo or other near surface measurements from oceanographic profiles (e.g., Hausfather et al., 2017; Huang et al., 2018), drifting buoys (e.g., Berry et al., 2018), moored buoys (e.g., Merchant et al., 2012). Evaluation for marine surface CDRs often relies on co-evaluation of different data products, which can provide important information on structural uncertainty (Kennedy, 2014; Kennedy et al., 2019) and be used to evaluate uncertainty estimates (Kent et al., 2017). Examples include: evaluation of SST using MAT from ships (Kent et al., 2013); coastal SST (Hanawa et al., 2000); or coastal air temperatures (Cowtan et al., 2018). Folland (2005) used a climate model to evaluate SST bias adjustments. Triple colocation (Stoffelen, 1998) is often used for evaluation of satellite winds, but has typically not been applied to historical in situ observations due to lack of collocated observations with independent errors.
An alternative, is to define subsets of data for evaluation that are excluded from the construction of data products. Near surface temperatures from Argo have often been excluded from analyses for use in validation (e.g., Martin et al., 2012) and the evaluation of air temperatures over land has also taken this approach by creating a separate version withholding a high quality subset (Hausfather et al., 2016).
When comparing different types of observation, or different gridded products, it is important to account for mismatches in spatiotemporal scales, known as representativeness. An example is when comparing in situ temporal average measurements at a single point with satellite measurements that are an almost instantaneous average across a spatial footprint. In order to estimate the expected uncertainty due to representativeness it is necessary to quantify the differences in scales and the variability of the ECV field across those scales.
Lack of independent high-quality observations is a barrier to the evaluation of long CDRs. As record lengths increase for timeseries stations such as OceanSITES and the GTMBA, and for satellite CDRs it will be possible to extend evaluation of in situ CDRs to other variables. The continuation and extension of OceanSITES will enable much evaluation of a wider range of ECVs with more independent data, at least for the modern period. RV observations are underutilized for evaluation, mainly because their data management is not internationally coordinated into quality-evaluated archives (Smith et al., 2019). Some potentially high-quality sources of data for evaluation, including from RVs, OWS and coastal stations, may require data rescue (see section “Data and Metadata Rescue”) or reprocessing (see section “Reprocessing of Existing Archives”).
Enhancing the Observational Archive
Quality Control
Initial checking can identify reports with incorrect values for date, time and position, unphysical values for elements or incorrectly coded parameters or metadata. If these errors are systematic, it may be possible to re-translate the available observation source, or to provide feedback to the data provider and obtain a revised version. More often than not such problems are discovered too late for such remedial action (e.g., the original data may have been lost, or staff may no longer be in post) and the data source may be excluded from the analysis, but some values might be correctable.
Basic checks can identify unusable data or impossible values such as non-existent dates or locations, or observations made over land. Any data failing checks should be flagged rather than discarded as changes to tests may be required in the future, and analysis of reasons for data failing checks can be used in the refinement of QC. Simple tests for each variable include ensuring there is a measured value which is reasonable given typical conditions, usually tested against a monthly or daily climatology with allowances for a combination of the expected real variability in the parameter and observational uncertainty. Further checks may be possible, for example, cloud and weather codes can be checked for consistency (Hahn et al., 1988) and relative humidity should be close to saturation when it is raining or foggy.
More sophisticated checks may then be implemented such as evaluation of the tracks of individual ships or drifting buoys. Tests applied by Rayner et al. (2006) include limits on the inferred speed of the ship, consistency between actual and reported heading, consistency between interpolated locations and reported locations. Other tests that can be performed on individual ships include: checks for repeated values, repeated super-saturation, and counts of observations from the platform (ships which make very small numbers of reports are typically less reliable). More extensive checks can also be performed at this level (e.g., Atkinson et al., 2013; Xu and Ignatov, 2016) by comparing measurements from an individual ship or buoy to a reference field and making decisions based on metrics such as the average bias or standard deviation relative to the reference data set.
A further step may be comparison of nearby observations (Rayner et al., 2006). Typically, a single observation is a compared to a mean of its neighbors. These “buddy checks” are of varying degrees of sophistication and effectiveness depending on the expected density and quality of the neighbors.
At each stage in the process, data which pass or fail individual checks are flagged and an overall decision is made based on some combination of these checks.
Following quality evaluation and flagging particular data sources or platforms that are identified as being of consistently low quality can be explicitly excluded from further analysis. Depending on the volume and characteristics of the observations that are flagged as erroneous or suspect, it may be advantageous to reevaluate parameters and limits within the QC process (Figure 1).
Enhancing Metadata
The amount of metadata that appears in ICOADS varies dramatically with data source (DCK and SID). Some sources have no platform IDs or observational metadata, some sources have IDs and rich metadata, particularly those that have been more recently digitized or processed. Most data sources have associated documentation, and a systematic review of this information is likely to substantially enhance ICOADS metadata availability. Examples of untapped metadata include mappings of ship numbers to ship names for some Japanese and Australian sources and documentation describing the transcription of data for punchcards. External documentary sources have also been used to infer metadata such as measurement methods (Kennedy et al., 2011b). Metadata from a WMO catalog has been associated with individual observations (Kent et al., 2007) based on IDs, and also used indirectly to infer metadata based on the recruiting country (Kent et al., 2010; Kennedy et al., 2011b). However to take full advantage of new information an improved data system and more flexible data models will be required.
Availability of IDs makes track checking possible (see section “Quality Control”) which can identify mispositioned observations. Recent masking of ship callsigns (Woodruff et al., 2011) in response to ship operators concerns about security and commercial interests, has led to a degradation of data in ICOADS and other archives. If no ID information is available then it is not possible to fully apply QC, to easily identify mispositioned or duplicate reports, to appropriately propagate uncertainty and to associate external metadata (Kent et al., 2007). Coded IDs have been adopted by some operators, which avoid some of these issues, but it may still not be possible to associate existing external metadata, and may preclude association of information that becomes available in the future.
It is possible to extend metadata using the characteristics of the data themselves. Examples include: the clustering of reports likely to be made on the same platform by ship tracking (Carella et al., 2017); the inference of data units or reporting precision from the distribution of reported values (Rhines et al., 2015); or the assignment of observing methods based on the data characteristics (Carella et al., 2018). Ideally such indirect methods of deducing metadata should be supported by full descriptions including observing instructions, information on instruments, their locations and installation and also documenting each stage of report coding and recoding.
There is useful information available from a wide range of other sources that have not yet been systematically integrated with marine observations. Where ship names are available it is often possible to determine a wealth of information about the ship, including pictures and quantitative information on size and tonnage from the internet. It may also be possible to infer ship names for reports with a numerical ID based on ports of departure and arrival from online sources. Many other sources of information exist that might provide relevant metadata, for example databases held by Lloyds Register of Shipping or metadata transmitted with Automatic Identification System (AIS) vessel tracking.
Merging Different Sources and Archives
If data from different sources are to be combined and analyzed together then clear metadata is required to indicate the data sources and typically effort will be needed to harmonize data formats, particularly metadata and data flags. Documentation of all steps is essential to avoid misinterpretation.
The identification of any reports that may derive from the same original observation may be required. Examples include where observations may become available in delayed mode in enhanced formats or with additional checks. In this case, any NRT version of the same report should be identified and is usually flagged as inferior (although in some cases inadequacies in the archival process may have degraded the data). This may be straightforward, but in some cases, especially where corruption has occurred, different versions of the same observation can only be identified with uncertainty. For example, prior to ICOADS Release 2.5 (Woodruff et al., 2011) reports identified as inferior duplicates are only available in depreciated data formats, so it is not easy to test the efficacy of the duplicate identification procedures applied. Another issue arises where newly-digitized data sources should replace older versions, but it is not always possible to clearly identify previous digitizations of the same source material.
Ideally the identification of data likely to be derived from the same original observations should allow for a specified tolerance for the permissible degree of difference between candidate reports. This should be based where possible on known recording, conversion, and data management practices (ideally from metadata flags). Where this is not possible the classification of expected differences can be built up from a comparison of the data sources themselves. This classification of expected differences should feed into uncertainty estimation and be used when selecting the preferred version of observations thought to be duplicates.
Data for ICOADS Releases prior to 2.5 (Woodruff et al., 2011) for sources that have been given low priority for selection in the ICOADS “dupelim” duplicate elimination processing and are not available in the present archive should be prioritized for recovery and reprocessing. This will provide improved information on likely differences between data sources and also information on typical errors/miscoding.
The Need for Expert Data System Design and Management
The modern observing system is diverse and each data type requires careful management, documentation, evaluation and quality control. Observations collected primarily for applications other than climate monitoring, typically for NWP, form the majority of the surface marine climate observing system. It is therefore critical that the needs of climate monitoring are considered in observing system design, and that observations needed for climate monitoring have dedicated data management systems and centers, whatever their origin. Whilst single variable repositories are used for some applications, observations made on the same platform should primarily be managed together and with adequate resources to allow expert evaluation, QC, and bias estimation.
User Needs for Data and Data Products
Understanding the Needs of Different Applications
Needs for observational climate records have in recent years been discussed in a number of contexts, for example under the ESA Climate Change Initiative, the Copernicus Climate Change Service and the 5th International Conference on Reanalyses.
In general, these experiences yield a requirement for:
(1) More data, i.e., existing observing arrays should be maintained, and sparsely observed regions should be better monitored and the potential of past measurements needs to be unlocked via digitisation and wider data sharing;
(2) More research to be undertaken into creating consistent records so that multi-decadal records can be used for the evaluation of decadal re-forecasts, for example;
(3) More research to be undertaken into quantifying uncertainty components and their covariance structures; and
(4) Better statistical modeling techniques to create analyses and allow better representation of the full data distribution to allow, for example, provision of observational constraints on future climate projections.
As the need for higher resolution information (in space and time) grows, there needs to be continued development of long-term products from in situ and satellite which are consistent to support the optimal combination of these different data types. This growing requirement for higher resolution information places high demands on the historical and current observing systems.
To support services with frequent updates, such as seasonal forecasting, and in short delay, such as short-delay event attribution, short-delay updates to monitoring and SST and sea ice boundary forcing data sets (and their underpinning data bases, like ICOADS) need to be developed which are consistent with the long-term record (e.g., Schwab et al., 2015). ECV products need to be developed with consistent coverage through time (in-filled) and good uncertainty estimates.
The adequacy of observing systems for variables that are not yet associated with requirements for operational services has declined in recent years (Figure 2C, e.g., Kent et al., 2006; Berry and Kent, 2017). An example is air temperature where a need has been identified for long term records for comparison with climate model output (Richardson et al., 2018). Observations of air temperature from VOS are required to extend coverage to enable the continued production of global air temperature analyses. To resolve this sampling deficit it will be necessary to relate observation requirements for gridded datasets to requirements for numbers and sampling strategies for VOS and other observing networks.
In the following, we explore two specific experiences: gathering of user requirements for sea-surface temperature and the needs for observations of dynamical reanalysis development.
Gathering User Requirements for Marine Climate Data
The development of user requirements covering a broad range of applications is increasingly important, and it is necessary to ensure that a narrow specification of requirements for a particular application does not lead to a degradation for other applications. An example of such a broad approach to gathering user requirements is that of the European Space Agency (ESA) Climate Change Initiative (CCI) SST project which aims to improve SST satellite data records to meet the requirements of the climate research community. A User Requirements exercise undertaken by the project (Good and Rayner, 2010) and then repeated 5 years later (Rayner, 2017) gathered the needs of the climate research community for observed SST information in general (not just for satellite SST products) via six methods:
(1) a literature review of relevant documents from bodies such as the GCOS;
(2) review of lessons learned information provided by other projects;
(3) a questionnaire, which asked about;
(a) currently available SST data; and
(b) future needs for SST data, 5 years from now;
(4) discussion sessions;
(5) review of user requirements found in other related projects; and
(6) a user workshop on uncertainties2.
Current and future users of SST data were invited to enter their requirements into an online questionnaire and over 100 people responded on each occasion from all over the world. Respondents’ work spanned the full range of climate applications, together with air quality modeling, fisheries, atmospheric chemistry, agricultural research, etc.
In general, different applications require different levels of data and the SST CCI User Requirements Document (URD) supports this conclusion. At least half of the users of SST information surveyed each time stated a need for information which has been made complete using a statistical infilling technique. However, it is also important to provide both individual observations and gridded, but not infilled, information for the other types of application such as data assimilation and climate change detection and attribution respectively. Furthermore, data from different instruments should be combined where this will allow weaknesses in individual datasets to be overcome. For example, there is a requirement for SSTs retrieved from infrared and microwave satellite instruments to be combined to reduce biases and data gaps in particular regions. By making available single-sensor records, sensor-series datasets, and multiple-sensor analyses and extending records back before the satellite era using in situ measurements, the needs of different users can be met.
A large majority of survey respondents needs global information, but different applications require different resolutions of information, both spatially and temporally. The full range of options from <1 km to >1 degree latitude-longitude and <3-hourly to monthly were required, which indicates that the climate observing system needs to be able to support some very exacting requirements for some climate applications and with a sizeable minority needing information about diurnal variability. The most common response in terms of length of record needed was >30 years, but as an objective >100 years would be ideal for many applications with compatibility between satellite and in situ data being extremely important. Analysis of decadal variability and the study of climate extremes, detection of long-term trends and the study of long-term changes associated with coral systems, fish growth or genetic changes all need long records. These potential users want to be able to use data before the satellite era but also want to take advantage of satellite-derived products, so it is important that the two are consistent.
The concept of interim CDRs, i.e., short-delay updates to CDRs consistent with the long-term record, is relevant to many users. For some, a delay of less than a day is needed until data receipt. Some climate monitoring applications ideally need data within just a few days, but some can tolerate longer delays. This continuing need for climate quality data can be addressed by ensuring that the data record is extendable in the future when new instrumentation is available, but also requires conversion of data processing systems from research to operational, supported by the convergence of climate and operational requirements.
Typically, biases in SST data are not tolerated above about 0.1∘C and precision is required to be 0.1∘C or better. Strict stability requirements of better than 0.05∘C/decade are stated. Users require these statistics to be demonstrated over spatial scales of ∼100 km, which is not typically possible everywhere and certainly not unless large high-quality data subsets are reserved for evaluation.
The SST CCI User Workshop on Uncertainty3 identified a significant interest within the user community of the provision of uncertainty information via an ensemble (a set of plausible realizations of each SST field which span the uncertainties in the data). Respondents to a second questionnaire were asked if SST uncertainty information were to be represented by an ensemble, how many ensemble members would they need for their application? Over half of respondents indicated they would require more than 10 ensemble members, and a similar number also felt they would benefit from the provision of uncertainty information via a parameterized error covariance matrix. These responses indicate a movement amongst the SST user community toward much more consideration of observational uncertainties.
Users also felt that confidence in uncertainty estimates needs to be stated and that uncertainty characteristics should be verified by comparison against independent observations. As with the evaluation of bias, precision and drift over time, this places strict requirements on the availability of independent, high quality reference data.
Observational Needs for Reanalysis
Many climate users access information about the climate system from dynamical reanalyses. Historical marine observations are key data sources for the production of climate-quality reanalyses. At the Fifth International Conference on Reanalysis (Buizza et al., 2018), the session on observations discussed the needs for observations of reanalysis production and the processing required to ensure a climate-quality outcome. These processing steps (data assembly, data rescue, quality control, bias correction, and data assimilation feedback analysis) and stated needs echo, in many cases, the processing and the SST needs discussed above, and demonstrate that they are generalizable to other variables.
Work on observations for reanalyses requires a sustained, well-supported effort involving cooperation with reanalysis producers; operational services are inadequate to support this work alone. Operationally-produced reanalyses are susceptible to changes in observing practice or transmission practice, such as the recent general move to the use of BUFR format for message transmission. Operationally-sustained production of underlying key data sets, consistent with the climate record, is essential to avoid discontinuities that affect downstream services.
Ongoing data rescue and recalibration, both of satellite and in situ data sources are very important for improving the quality of reanalyses and extending their quality back in time. In particular, data rescue is crucial for preserving data currently on fragile tape or paper. Reprocessing and making observational [both ECV and parameters used to generate Fundamental Climate Data Records (FCDRs) such as radiance (GCOS, 2011)] series more consistent allows more of the information to be included in a reanalysis and allows our reanalyses to better reflect the observations. This requires a sustained program (Brönnimann et al., 2018a, 2018b).
The fundamental observational record needs to be carefully preserved for future reanalyses and for every other activity we may need it for now and in the future. This needs ongoing, adequate support. Previous experience demonstrates that once observational holdings become fragmented, it requires a great deal of effort to correctly reconstruct them.
Development of future assimilation systems needs to consider how best to use observations and information on their uncertainties and this effort needs to be supported. End-to-end propagation of uncertainties through observation processing is required to enable this.
The reanalysis community need to clearly state their ongoing requirements for the observing system, considering also the known downstream needs of applications. For example: a subset of the observations used are particularly key and reduce uncertainty so much that they could be considered akin to reference series – these should be defined and particularly cared for; resolution of boundary/constraining SST fields is key to reproducing precipitation over frontal zones and reducing tropical biases – microwave SST data should be continued and its resolution increased; and funding for new satellite missions should be continued, since that provides for future innovation.
How Do User Needs Impact on the Construction of CDRs?
Producers of CDR and other climate data products have responded to user requests for more frequent updates, particularly important for climate monitoring. There is inevitably a tension between the speed with which products are made available and their quality. Observations may not be available in time for cut-off deadlines, improvements in data quality from delayed mode processing are not available, and assumptions must be made that data quality is similar to that seen previously. For those products aiming to meet the needs of users with requirements for fast data delivery, updates are typically made a few days after the end of every month. Except where particular problems are apparent, revision of these fast delivery products is usually every few years. This is a compromise between having relatively stable versions, and ensuring the highest quality.
Other trade-offs that may occur between different user needs are between quality, resolution and completeness. Infilled datasets are needed for some applications but uncertainty is bound to increase in unsampled regions. Need for increased coverage, or higher resolution, may require that lower quality data sources are utilized, perhaps without the desired metadata. In all these cases careful consideration of the terms in the error model will guide data selection, and provide estimates of data uncertainty that can guide user choice.
Considerations When Creating Internally-Consistent Records for Marine Surface ECVs
Air Temperature
Observations of MAT have been made on ships since about 1750, but observation numbers increased with availability of measurements from the East India Company in the late 18th Century (Freeman et al., 2017) (Figure 2A). Early in the record measurements of MAT are more common than SST, which only become consistently observed following Maury (1854). Measurements are made with thermometers with a transition between mercury thermometers to electronic sensors over time (Kent et al., 2007). The most important factors for MAT data quality are thought to be the location, ventilation, and degree of sheltering from the sun (Kent et al., 1993).
Marine air temperature observations need to be adjusted to a common reference height (Kent et al., 2013), requiring information on the actual observing height (h) and an estimate of the stability of near surface atmosphere which depends on ambient conditions (a). h may be available from Publication 47 (Kent et al., 2007), but only from around the 1970s, prior to this some rather broad generalizations must be made (Kent et al., 2013). A further source of uncertainty is the difference in measurement heights and depths that occurs with a change in ship loading. Elements of a required are wind speed, air-sea temperature difference, and ideally the humidity. Present adjustments applied to ICOADS use climatological estimates of a and its uncertainty. For high-quality data sources it is likely that using observed values would be an improvement, but this will require further research to quantify uncertainties for each data source and their impact on height adjustment. Improvements are likely to be possible for MAT without high-quality a through, for example, further constraining the likely climatological distribution of atmospheric stability with additional information such as wind direction.
The most prevalent source of bias (B) in MAT is due to the daytime influence of spurious heating by the ship infrastructure (Berry et al., 2004). This effect will be larger for sensors that are installed close to the deck as is required for manual reading of thermometers installed in Stevenson Screens (Berry and Kent, 2005). The move to remote reading electronic thermometers enables sensors to be installed in well-exposed locations should reduce the magnitude of B, but this has not yet been evaluated across ICOADS. Additional metadata, for measurement method (m), instrument (i), ship size and type and height above deck may in the future provide valuable information for more refined estimates of B and εb that may depend on proximity to the heat source and the ventilation of the sensor (for example an estimate of the ambient air flow and i, m). An estimate of (Berry et al., 2004) has been implemented with ICOADS from 1973 (Berry and Kent, 2011), but existing longer records minimize B by selecting night-MAT only (Rayner et al., 2003; Kent et al., 2013), restricting the record to start after 1850. Other B specific to particular data sources, periods or regions are treated ad hoc (Rayner et al., 2003; Kent et al., 2013).
Humidity
Humidity observation records exist intermittently from the late 1800s, reaching reasonable monthly coverage (∼30–40% ocean area) from around 1960 onwards (Figure 2). Likely, many more observations exist but these have not typically been prioritized in data rescue efforts. Ships are the most prolific, and arguably most reliable, platform providing humidity observations given that they are manned and sensors are located further from sea level, and therefore from sea spray contamination, than moored buoys. However, since the 1990s moored buoys have steadily increased in number, rapidly from around 2010 to now almost double the number of ship observations (Figures 2C, 3).
Humidity has been typically measured using paired wet-bulb and dry-bulb thermometers (psychrometers) in either a hand held sling or within a ventilated screen. Humidity is then often converted and reported as dew point temperature, any such conversions should be documented (d). Since the 1990s, available metadata (40–60% of ships) shows that capacitance and electric sensors have steadily increased in number to approximately 40% of the ships with metadata combined. These typically measure relative humidity which is then converted and reported as dew point temperature.
Sources of error for humidity observations depend on m. For wet-bulb thermometers the wick surrounding the thermometer can dry out or become contaminated with sea spray. Relative humidity sensors are also prone to contamination and can drift relatively rapidly, particularly in humid environments (Ingleby, 2010). While psychrometers tend to have better accuracy in moist environments, relative humidity sensors perform better in drier environments. Field evaluations of relative humidity sensors in the marine environment, extending the Ingleby et al. (2013) study of the United Kingdom land station network, are urgently required. If not well-ventilated, through active whirling or artificial ventilation, sensors will be biased humid. Adjustments have been applied for naturally ventilated screens (Berry and Kent, 2009, 2011), but require knowledge of m or i. Errors in MAT due to daytime heating of ship structures may not directly lead to biased humidity measurements (Kent and Taylor, 1996), but both sources of bias are likely to be larger for poorly exposed or ventilated sensors. As for MAT, humidity observations need to be adjusted to a common reference level requiring h and a. Sources of B for humidity largely follow those for MAT but are more complex owing to the derived and non-linear nature of humidity.
Sea-Surface Temperature
Sea surface temperature provides the marine component of global surface temperature, combined with air temperatures measured over land (Hartmann et al., 2013) and is the marine variable that receives the most attention because many activities in climate science and service provision rely upon it. Its uncertainties and bias adjustment have been recently reviewed (Kennedy, 2014; Kent et al., 2017). A wide range of different types of SST data are available, from satellites (e.g., Merchant et al., 2014), and in situ from ships, drifting buoys, moored buoys, platforms and coastal stations and the upper parts of temperature profiles from XBTs, CTDs, and Argo floats. There are several long-term global SST data sets (HadSST3, Kennedy et al., 2011a,b; HadISST, Rayner et al., 2003; ERSSTv5, Huang et al., 2017; COBE-SST2, Hirahara et al., 2014). This redundancy enables an estimation of structural uncertainty (Kennedy, 2014), demonstration that differences in B for different datasets exceeds their joint uncertainty (Kent et al., 2017) and that significant biases likely remain at the scale of individual ocean basins (Davis et al., 2018).
There is a need to reconcile near-surface and sub-surface measurements of temperature. The near surface layer is complicated (Kawai and Wada, 2007) and sampling just below the surface is worse than that close to the surface (Figures 2, 4). Despite this upper measurements from temperature profiles have been used to evaluate SST products (e.g., Gouretski et al., 2012; Hausfather et al., 2017; Berry et al., 2018; Huang et al., 2018).
Estimation of B for SST is relatively mature (Kent et al., 2017) and ideally requires knowledge of p, m, d, and a. The need for improved estimates of m and p for SST has driven improvements in metadata estimation (see section “Enhancing Metadata”). Improved estimates of B will need further improvements to m and p, better documentation of past data management (d) and ambient conditions (a), facilitated by a reprocessing of ICOADS to improve duplicate identification and uncertainty estimation. Measurements from sensors attached to the hulls of ships are yet to be evaluated for uncertainty.
Availability of data for evaluation is better than for most ECVs, but improvements through data rescue and reprocessing would still be valuable (Kent et al., 2017).
Wind Speed and Direction
Wind speed observations based on the Beaufort scale and derived either from descriptions on the sails carried, or of the sea state, are available from the 18th century (Figure 2A). The transition from these visual observations to measurements by anemometers (Kent and Taylor, 1997) resulted in a spurious trend in mean wind speeds (Cardone et al., 1990; Thomas et al., 2008). This is compounded by an overall increase in h over time, and biases due the disturbance of air flow around ships (Moat et al., 2006). True wind speed and direction (relative to the earth or ocean surface rather than to the frame of reference of the ship) needs to be calculated from measured or estimated relative wind speed and direction and the ship motion. The procedure is different for measured and visually estimated winds and is not always performed correctly (Smith et al., 1999). There are also stronger diurnal variations in visual wind estimates from ships than in those measured with anemometers, probably due to the difficulty of observing sea state when it is dark (Thomas et al., 2008). To minimize inhomogeneity Tokinaga and Xie (2011) generated a long-term wind product based only on visual wind estimates and anemometers with known heights.
Measurements from buoys also contain uncertainties: any averaging applied needs to be documented, including whether scalar or vector averaging was used (Thomas et al., 2005), and measurements made close to the sea surface may be affected by waves. The moored buoy network provides calibration for satellite winds (Wentz et al., 2017) and helps to anchor wind products such as the Cross-Calibrated Multi-Platform (CCMP) winds (Atlas et al., 2011). Comparisons between in situ satellite winds should account for geophysical differences between the records such as the effects of surface currents (Plagge et al., 2012; Rodriguez et al., 2019).
Atmospheric Pressure
An extensive review of historical atmospheric pressure observations was conducted by Allan and Ansell (2006) and Ansell et al. (2006). Pressure observations need adjustment to sea level (L) requiring information on h and temperature. Mercury barometers need adjustment for local gravity and thermal expansion (requiring m, x, y and temperature) and also information on whether these adjustments have already been applied and if so how (d). Diurnal variations are significant (Ansell et al., 2006).
Lack of clarity on past data management remains a substantial problem for the use of pressure observations, with examples of adjustments having been applied twice, incorrectly, or not at all. A comprehensive review of available documentation, reprocessing of ICOADS original data sources and comparison between those sources would be particularly beneficial for the historical pressure record, including for reanalysis.
Waves and Sea State
Visual observations of sea state have been made for centuries (Figure 2), observing practice was defined in the 1850s (Maury, 1854) and constitute the longest record of wind waves. Visual observations can identify several wave systems when a well-trained observer estimates a number of sea state characteristics along with atmospheric parameters. The observer’s estimation is dependent on surrounding parameters (weather conditions, place of observation, type of vessel, stationary point, etc.). Even though the approach is essentially qualitative, it is possible to integrate all of these factors into a comprehensive observation. VOS data provide basic wave characteristics, such as wind sea, first and second swell heights, periods, and the directions of propagation of those wave components. Reported parameters enable the estimation of significant wave height, dominant period, wave age, steepness and wavelength (Gulev et al., 2003; Grigorieva et al., 2017) and have been used in extreme waves analysis (Grigorieva and Gulev, 2008), and for the assessment of long-term tendencies in wave parameters (Gulev and Grigorieva, 2004, 2006).
Observational practice has not changed, however, significant changes to the coding system occurred in 1950 and it is important to account for this when developing consistent climate records (Gulev et al., 2003; Grigorieva and Badulin, 2016; Grigorieva et al., 2017). Evaluation of the internal consistency of the wave record over time is critical for the identification of coding changes and their impact. Fitting of Weibull distributions to available data can produce more reliable gridbox values. Using these methods a century long record of wave parameters has been developed, that has not yet been fully exploited for applications such as comparison with satellite-based wave records or with model output.
Cloud Type, Cloud Cover, and Coded Weather Information
Visual cloud observations from human surface observers (Figure 2) contain a great deal of information about the atmosphere, interacting with radiation and modifying weather. The structure, height, and shape of clouds, which can be quantified by weather observers at the surface, can be used to assess the atmospheric state (Norris, 1998). The cloud record has been used to study how cloud cover interacts with the SST and changing meteorological variables such as lower tropospheric stability as well as to examine long-term trends in ocean cloudiness whilst revealing some spurious long-term variations (Eastman et al., 2011).
Visual cloud observations board ships throughout the oceans have been recorded using the same methodology and format, since the 1950s, 20 years before satellites. The Extended Edited Cloud Reports Archive (EECRA, Hahn et al., 1988; Hahn and Warren, 2009) contains millions of visual cloud reports from 1954 through 2008, based on observations in the synoptic code of the WMO that have been assessed for internal consistency and QCd. The EECRA provides a measure of lowest-level cloud cover and total cloud cover along with a cloud type at three levels: high, middle, and low. At least 10 cloud types are defined at each level and selected meteorological information is also included in the record. EECRA methodology has been applied to ICOADS reports from 1950 to 2014 to provide an enhanced cloud record, but this has not yet been used to derive gridded estimates. VOS reports contain coded weather information that can be used to provide context of the environmental conditions that may be used in uncertainty or bias estimation. Precipitation data products have been produced using such codes (Petty, 1995; Josey et al., 1999).
Future Improvements
Ensuring the Future Record
Reference Observations
OceanSITES provides the most consistent source of surface marine data presently available with unified access and common data formats. Whilst it does not strictly conform to the definition of a reference network used for observations over land (Thorne et al., 2017b) the maintenance of a high quality array of moored observations is critical for the maintenance of long-term records. The number of OceanSITES currently providing most of the ECVs in the scope of this paper is about 20, but an extension of similar size has been recommended in support of air-sea fluxes (Cronin et al., 2019), sampling regions thought to be particularly important for understanding the mechanisms of air-sea interaction and providing validation observations for satellites. Such an extension to approximately 40 sites would be extremely valuable for the construction of CDRs.
Select RVs have the potential, based on their level of technical personnel onboard and typically research-quality instrumentation, to act as mobile reference stations, provided a high-level of quality assessment can be achieved (Smith et al., 2019). This would be extremely valuable to link up fixed point high-quality OceanSITES, particularly if the same RVs are used to service OceanSites, and provide more distributed data.
Argo provides a reference network for SST, but suffers from large sampling uncertainty due to the relatively short time spent at the surface. Surface drifters with higher quality sensors have been deployed to quantify sensor drift (Reverdin et al., 2010; Poli et al., 2018) and if deployed systematically in the future might provide a reference network for SST. Satellite missions such as the ATSR-series (Merchant et al., 2012) that are designed with stable orbits and sampling strategies such as dual view to enable the removal of artifacts such as aerosol contamination should be maintained and extended to provide stable long-term global records.
Baseline Observations
Baseline observations should be of good quality, widespread, and provide observations to link the reference and global networks. RVs that do not meet the criteria for reference observations are an obvious choice, but presently their data and metadata are not collected and managed systematically, and there is no globally integrated data management system (Smith et al., 2019). Moored buoys other than OceanSITES are also candidates for contribution to baseline networks, but as for RVs there is no integrated data and metadata management system. Enhanced VOS, once evaluated, may also provide baseline observations.
Global Observations
Global networks are those data types that may not reach accuracy requirements for baseline networks but provide widespread measurements to capture the important scales of variability. Standard VOS form such a network, and data from AIS may in the future add to the global network of ship observations. Autonomous observations from Argo and drifting buoys contribute to the reference and baseline networks, but data from emerging autonomous vehicles will initially contribute to a global network. Observations from satellites have the potential to extend sampling to data sparse regions and can provide observations to quantify variability. Long term records blending satellite and in situ data to extend records prior to the satellite era should be developed for ECVs beyond the presently-available SST. New technology, especially from autonomous vehicles will also provide valuable observations for long term climate records. Such data can improve our knowledge of ECV variability over the oceans which will contribute to improved CDRs throughout the record and allow the extension of reconstruction methods to a wider range of ECVs. It also needs to be recognized that single variable observations are not as useful as multivariate ones which typically provide more of the ancillary information needed to quantify B, L and quantify uncertainty and its correlation structure.
Construction of long term records means that new observing technologies need to be continually incorporated into the record. This is made substantially easier when the changes are well-managed, there is co-located overlap between old and new types of observations, and all observations are described by appropriate metadata. It is critical that we retain a network of high quality observations, and evaluations of existing and new technologies are designed to exploit these high quality data.
Data and Metadata Rescue
The digitization of marine observations has dramatically extended the climate record (e.g., Freeman et al., 2017). Most data and metadata rescue activities are presently overseen by the Atmospheric Circulation Reconstructions over the Earth (ACRE) initiative (Allan et al., 2011). International co-ordination of data rescue is needed for several reasons, most obviously to avoid duplication of effort but also because those countries or organizations with the resources or requirements for data digitization may not be the same as those with holdings of undigitized data. The first step is the identification and cataloging of suitable material, typically ships logbooks, observing instructions, or other documentation. This may involve visits to libraries, archives or other institutions. If resources are identified then the material will be imaged, the resulting images digitized, the data or metadata evaluated, QC applied and data made available for ingestion into climate archives such as ICOADS. The prioritization of resources for imaging will depend on the likely quality and quantity of data, the parameters available, the period and region covered, the match to user requirements and the level of risk for deterioration of the original material. Best practice is that as much information as possible should be digitized from each image along with all of the relevant metadata and ancillary data.
In the past all data digitization was performed by manual keying. Often the output data format was severely restricted, such as that for punchcards. In these cases it is particularly valuable to have documentation describing the procedures used. Sometimes the more complete original sources were then destroyed, but there may be examples where it may be possible to recover data from original sources. Automatic techniques for digitization do not yet work well with most data sources, but this likely to improve in the future, for example with tailored post-processing of output from standard optical character recognition algorithms. Recently crowdsourcing has been used with good success (Brohan et al., 2009; Burt and Hawkins, 2019). Crowdsourcing can be very effective, but requires substantial preparation of material so is most suitable for large volumes of data in similar formats. It is also necessary to maintain the enthusiasm of volunteers, and to demonstrate the value of their contributions.
Much recent digitization has been driven by requirements for reanalysis (e.g., Allan et al., 2011; Brönnimann et al., 2018a,b), and records containing pressure observations are most valuable for this application, especially in data sparse regions or periods. However there are many other applications that would also benefit from data recovery. Of particular value for the construction of long-term records are high-quality independent validation data, especially those that are expected to be consistent through periods of rapid changes in the observing system, for example during the World Wars.
Much marine data rescue has focused on old ships logbooks and metadata such as observing instructions, but there are other data sources that need to be integrated into international archives. Some RV operators have data archives on archaic media or in proprietary formats that could be lost and should be cataloged and prioritized for rescue. Many national services hold collections of GTS data that might provide additional observations, or if duplicating existing archives, information about uncertainty. Early satellite data also exist that need painstaking rescue from at-risk media.
Reprocessing of Existing Archives
The surface marine climate community has long benefited from the ICOADS integrated archive, the value of which is widely recognized (Thorne et al., 2017a). However compromises made during past data management are now reducing the potential for application of advanced methods for estimation of bias and location adjustments, thereby degrading gridded analyses and limiting further improvement. ICOADS has retained original data sources and the available documentation, so it is possible to re-ingest these data, without constraints on formats or data volumes and in a way more compatible with international standards (see section “An Integrated Data System”).
Advantages of reprocessing selected ICOADS original data sources include:
• Recovery of observations thought to be duplicates and excluded from further analysis. This will allow the development of improved approaches to duplicate identification, and the quantification of differences between versions of the same measurements due to past data management.
• Improved identification of data from sources likely to be useful for evaluation, such as OWS or RVs.
• Recovery of data and metadata that were not retained in past data formats.
• Extension of metadata through a comprehensive review of documentation.
A further advantage would come from a reprocessing of GTS accessions to better identify reports that should be replaced with higher quality data from delayed mode sources.
An Integrated Data System
As noted above, the observing system is made up of a diverse range of platforms, observing systems and data streams, each with their own issues. In order to efficiently use the observations in the generation of CDRs a number of basic requirements need to be met:
• Observations from identifiable sources at the platform level
• Instrument metadata (methods, error characteristics, etc.) associated with the observations
• Open data sources for reproducibility
• Consistent conventions and format used to represent the observations (and metadata)
• Timely access to the observations (and metadata)
• Redundancy of NRT data streams and archive access.
These requirements are beginning to be addressed for surface ocean data through a number of initiatives. For example, within JCOMM a database containing the instrumental and platform metadata for all observing platforms contributing to the JCOMM programs (e.g., VOS, DBCP, etc.) has been developed and populated. Similarly, a Marine Climate Data System is under development to improve the timely flow of delayed mode and real time data as well as promoting best practices and standards. For the VOS the JCOMM metadata database has been built based on the WMO Publication 47 metadata and, in part, been driven by development of the WIGOS and metadata requirements from the WIGOS Metadata Standard. Integrated data management and archival systems are needed for all components of the GOOS and GCOS providing ECVs for the construction of CDRs. WIGOS, if used fully, provides an appropriate framework for the provision of data and metadata together. Whilst the developments of the JCOMM and WMO systems has focused on operational data flows other systems are being developed for climate data. An exemplar is the C3S Climate Data Store part of the European Union funded Copernicus Climate Change Service.
Reflections and Recommendations
Reflections
The construction of CDRs for all ECVs has been made more difficult by the lack of availability of good platform and observational metadata. This has in the past been compounded by a focus on rather narrow user requirements, for example for NWP, reanalysis or satellite bias adjustment. This has led to a narrowing of the range of ECVs that are typically measured together, and in some cases to truncated data formats and lack of metadata. Each set of observations has a non-trivial error model and this is linked with everything done to the data. Understanding, documenting, and encoding in metadata everything relevant about the platform, instruments, observing protocols, coding and recoding helps to reduce the uncertainty in CDRs and enables reconciliation between different measurement types (GCOS, 2016).
New data systems under development, for example at JCOMM and for the C3S Climate Data Store, should enable the relevant information to be captured and provided to users. But having the ability to record metadata is only part of the story. Data providers need also to be diligent in ensuring that metadata are reported, and archival formats need to preserve it.
Compromises are inevitable when the needs of CDR construction interact with the needs of real users, particularly in the area of timeliness vs. quality. The impact of any compromise will be minimized if the data system understands these potentially conflicting needs from the start.
The decline in the number of VOS reports has had a severe and detrimental effect on the sampling for several ECVs, in particular MAT, humidity, winds, clouds, and waves. The resulting decline in the number of multivariate records also has an impact on ability to bias adjust and quantify uncertainty those ECVs that are reported.
Rescue through digitization of data and metadata are key to the extension and improvements of CDRs. In many cases the priorities for CDR construction are similar to those for applications such as reanalysis which target data sparse regions and periods. But other requirements for CDRs include recovery of metadata and documentation, high quality and consistent timeseries, data with measurements of the same ECV made by different methods, and might prioritize reports that do not contain pressure measurements.
User requirements for GCOS are typically expressed in terms of a required accuracy at particular spatiotemporal resolutions4. Requirements for CDRs are often described in terms of quite strict stability of anomalies over large space and time scales. An example is a need for SST data sets which are global and demonstrably stable to much better than 0.1∘C decade−1, ideally with deviations less than 0.01∘C decade−1. This has been achieved for satellite SST (Merchant et al., 2012) but requires complementary and independent data for evaluation. SST and winds are the only record for which the community is able to demonstrate compliance with stringent user requirements, and typically only for relatively short periods and limited regions (e.g., Verhoef et al., 2017; Berry et al., 2018). SST is the only ECV where there are multiple long term data products with uncertainty estimates which enables us to also evaluate uncertainty estimates and state that uncertainties are underestimated (Kent et al., 2017; Davis et al., 2018).
Author Contributions
EK and NR wrote the first draft of the manuscript. All authors provided text and comments and approved the final draft.
Funding
EK and DB were supported by the NERC under the grants NE/R015953/1 and NE/J020788/1. NR, JK, and KW were supported by the Met Office Hadley Centre Climate Programme funded by the BEIS and Defra. VG was supported by the Russian Ministry of Science and Education under the Project 14.W03.31.0006.
Conflict of Interest Statement
The authors declare that the research was conducted in the absence of any commercial or financial relationships that could be construed as a potential conflict of interest.
Footnotes
- ^ https://www.pmel.noaa.gov/tao/drupal/disdel/
- ^ http://www.esa-sst-cci.org/PUG/pdf/CombinedSSTUserWorkshopReport.pdf
- ^ http://www.esa-sst-cci.org/PUG/workshop.htm
- ^ http://www.wmo-sat.info/oscar/observingrequirements
References
Allan, R., and Ansell, T. (2006). A new globally complete monthly historical gridded mean sea level pressure dataset (HadSLP2): 1850–2004. J. Clim. 19, 5816–5842. doi: 10.1175/JCLI3937.1
Allan, R., Brohan, P., Compo, G. P., Stone, R., Luterbacher, J., and Brönnimann, S. (2011). The international atmospheric circulation reconstructions over the earth (ACRE) initiative. Bull. Am. Meteorol. Soc. 92, 1421–1425. doi: 10.1175/2011BAMS3218.1
Andersson, A., Klepp, C., Fennig, K., Bakan, S., Grassl, H., and Schulz, J. (2011). Evaluation of HOAPS-3 ocean surface freshwater flux components. J. Appl. Meteorol. Climatol. 50, 379–398. doi: 10.1175/2010JAMC2341.1
Ansell, T. J., Jones, P. D., Allan, R. J., Lister, D., Parker, D. E., Brunet, M., et al. (2006). Daily mean sea level pressure reconstructions for the european–north atlantic region for the period 1850–2003. J. Clim. 19, 2717–2742. doi: 10.1175/JCLI3775.1
Ardhuin, F., Stopa, J. E., Chapron, B., Collard, F., Jensen, R. E., Johannessen, J., et al. (2019). Observing sea states. Front. Mar. Sci. 6:124. doi: 10.3389/fmars.2019.00124
Atkinson, C. P., Rayner, N. A., Roberts-Jones, J., and Smith, R. O. (2013). Assessing the quality of sea surface temperature observations from drifting buoys and ships on a platform-by-platform basis: assessing buoy and ship SST observations. J. Geophys. Res. Oceans 118, 3507–3529. doi: 10.1002/jgrc.20257
Atlas, R., Hoffman, R. N., Ardizzone, J., Leidner, S. M., Jusem, J. C., Smith, D. K., et al. (2011). A cross-calibrated, multiplatform ocean surface wind velocity product for meteorological and oceanographic applications. Bull. Am. Meteorol. Soc. 92, 157–174. doi: 10.1175/2010BAMS2946.1
Beggs, H. M., Verein, R., Paltoglou, G., Kippo, H., and Underwood, M. (2012). Enhancing ship of opportunity sea surface temperature observations in the Australian region. J. Oper. Oceanogr. 5, 59–73. doi: 10.1080/1755876X.2012.11020132
Belmonte Rivas, M., Stoffelen, A., Verspeek, J., Verhoef, A., Neyt, X., and Anderson, C. (2007). Cone metrics: a new tool for the intercomparison of scatterometer records. IEEE J. Sel. Top. Appl. Earth Obs. Remote Sens. 10, 2195–2204. doi: 10.1109/jstars.2017.2647842
Berry, D., Corlett, G., Embury, O., and Merchant, C. (2018). Stability assessment of the (A)ATSR sea surface temperature climate dataset from the european space agency climate change initiative. Remote Sens. 10:126. doi: 10.3390/rs10010126
Berry, D. I., and Kent, E. C. (2005). The effect of instrument exposure on marine air temperatures: an assessment using VOSClim data. Inter. J. Clim. 25, 1007–1022. doi: 10.1002/joc.1178
Berry, D. I., and Kent, E. C. (2009). A new air–sea interaction gridded dataset from ICOADS with uncertainty estimates. Bull. Am. Meteorol. Soc. 90, 645–656. doi: 10.1175/2008BAMS2639.1
Berry, D. I., and Kent, E. C. (2011). Air-Sea fluxes from ICOADS: the construction of a new gridded dataset with uncertainty estimates. Inter. J. Clim. 31, 987–1001. doi: 10.1002/joc.2059
Berry, D. I., and Kent, E. C. (2017). Assessing the health of the in situ global surface marine climate observing system. Inter. J. Clim. 37, 2248–2259. doi: 10.1002/joc.4914
Berry, D. I., Kent, E. C., and Taylor, P. K. (2004). An analytical model of heating errors in marine air temperatures from ships. J. Atmos. Ocean. Technol. 21, 1198–1215. doi: 10.1175/1520-0426(2004)021<1198:aamohe>2.0.co;2
Bojinski, S., Verstraete, M., Peterson, T. C., Richter, C., Simmons, A., and Zemp, M. (2014). The concept of essential climate variables in support of climate research, applications, and policy. Bull. Am. Meteorol. Soc. 95, 1431–1443. doi: 10.1175/BAMS-D-13-00047.1
Bourassa, M. A., Meissner, T., Cerovecki, I., Chang, P., Xiaolong, D., De Chiara, G., et al. (2019). Remotely sensed winds and wind stresses for marine forecasting and ocean modeling. Front. Mar. Sci. submitted.
Boyer, T. P., Antonov, J. I, Baranova, O. K., Coleman, C., Garcia, H. E., Grodsky, A., et al. (2013). World Ocean Database 2013. Silver Spring, MD: NOAA Printing Officce.
Brohan, P., Allan, R., Freeman, J. E., Waple, A. M., Wheeler, D., Wilkinson, C., et al. (2009). Marine observations of old weather. Bull. Am. Meteorol. Soc. 90, 219–230. doi: 10.1175/2008BAMS2522.1
Brönnimann, S., Allan, R., Atkinson, C., Buizza, R., Bulygina, O., Dahlgren, P., et al. (2018a). Observations for reanalyses. Bull. Am. Meteorol. Soc. 99, 1851–1866. doi: 10.1175/BAMS-D-17-0229.1
Brönnimann, S., Brugnara, Y., Allan, R. J., Brunet, M., Compo, G. P., Crouthamel, R. I., et al. (2018b). A roadmap to climate data rescue services. Geosci. Data J. 5, 28–39. doi: 10.1002/gdj3.56
Buizza, R., Poli, P., Rixen, M., Alonso-Balmaseda, M., Bosilovich, M. G., Brönnimann, S., et al. (2018). Advancing global and regional reanalyses. Bull. Am. Meteor. Soc. 99, ES139–ES144. doi: 10.1175/BAMS-D-17-0312.1
Burt, S., and Hawkins, E. (2019). Near-zero humidities on Ben Nevis, Scotland, revealed by pioneering nineteenth-century observers and modern volunteers. Inter. J. Climatol. doi: 10.1002/joc.6084 [Epub ahead of print].
Cardone, V. J., Greenwood, J. G., and Cane, M. A. (1990). On trends in historical marine wind data. J. Clim. 3, 113–127. doi: 10.1175/1520-0442(1990)003<0113:otihmw>2.0.co;2
Carella, G., Kennedy, J. J., Berry, D. I., Hirahara, S., Merchant, C. J., Morak-Bozzo, S., et al. (2018). Estimating sea surface temperature measurement methods using characteristic differences in the diurnal cycle. Geophys. Res. Lett. 45, 363–371. doi: 10.1002/2017GL076475
Carella, G., Kent, E. C., and Berry, D. I. (2017). A probabilistic approach to ship voyage reconstruction in ICOADS. Inter. J. Climatol. 37, 2233–2247. doi: 10.1002/joc.4492
Centurioni, L. R. (2018). “Drifter Technology and Impacts for Sea Surface Temperature, Sea-Level Pressure, and Ocean Circulation Studies,” in Observing the Oceans in Real Time, eds R. Venkatesan, A. Tandon, E. D’Asaro, and M. A. Atmanand (Cham: Springer International Publishing), 37–57. doi: 10.1007/978-3-319-66493-4_3
Chan, D., and Huybers, P. (2019). Systematic differences in bucket sea surface temperature measurements amongst nations identified using a linear-mixed-effect method. J. Clim. 32, 2569-2589. doi: 10.1175/JCLI-D-18-0562.1
Chan, D., Kent, E. C., Berry, D. I., and Huybers, P. (2019). Correcting datasets leads to more homogeneous early-twentieth-century sea surface warming. Nature 571, 393–397. doi: 10.1038/s41586-019-1349-2
Cowtan, K., Rohde, R., and Hausfather, Z. (2018). Evaluating biases in sea surface temperature records using coastal weather stations. Q. J. R. Meteorol. Soc. 144, 670–681. doi: 10.1002/qj.3235
Cronin, M. F., Gentemann, C. L., Edson, J. B., Ueki, I., Bourassa, M., Brown, S., et al. (2019). Air-sea fluxes with a focus on heat and momentum. Front. Mar. Sci. doi: 10.3389/fmars.2019.00430
Davis, L. L. B., Thompson, D. W. J., Kennedy, J. J., and Kent, E. C. (2018). The importance of unresolved biases in 20th century sea-surface temperature observations. Bull. Am. Meteorol. Soc. 100, 621–629. doi: 10.1175/BAMS-D-18-0104.1
DBCP (2016). Data Buoy Co-Operation Panel, A Retropective, 2016’. Available at: https://www.wmo.int/pages/prog/amp/mmop/documents/dbcp/Dbcp56/DBCP%20TD%20No%2056.pdf (accessed November 2015).
Eastman, R., Warren, S. G., and Hahn, C. J. (2011). Variations in cloud cover and cloud types over the ocean from surface observations, 1954–2008. J. Clim. 24, 5914–5934. doi: 10.1175/2011JCLI3972.1
Folland, C. (2005). Assessing bias corrections in historical sea surface temperature using a climate model. Inter. J. Climatol. 25, 895–911. doi: 10.1002/joc.1171
Folland, C. K., and Parker, D. E. (1995). Correction of instrumental biases in historical sea surface temperature data. Q. J. R. Meteorol. Soc. 121, 319–367. doi: 10.1002/qj.49712152206
Freeman, E., Kent, E. C., Brohan, P., Cram, T., Gates, L., Huang, B., et al. (2019). The international comprehensive ocean-atmosphere data set – meeting users needs and future priorities. Front. Mar. Sci. doi: 10.3389/fmars.2019.00435
Freeman, E., Woodruff, S. D., Worley, S. J., Lubker, S. J., Kent, E. C., and Angel, W. E. (2017). ICOADS Release 3.0: a major update to the historical marine climate record. Inter. J. Climatol. 37, 2211–2232. doi: 10.1002/joc.4775
GCOS (2011). ’Systematic Observation Requirements for Satellite-Based Data Products for Climate. Available at: https://library.wmo.int/doc_num.php?explnum_id=3710 (accessed December 2011).
GCOS (2015). Status of the Global Observing System for Climate. Available at: https://library.wmo.int/pmb_ged/gcos_195_en.pdf (accessed October 2015).
GCOS (2016). The Global Observing System for Climate: Implementation Needs. Available at: https://unfccc.int/sites/default/files/gcos_ip_10oct2016.pdf (accessed July 2019).
GCOS (2018). Essential Ocean Variables. Available at: http://www.goosocean.org/index.php?option=com_content&view=article&id=14&Itemid=114 (accessed July 2019).
Good, S., and Rayner, N. A. (2010). User Requirements Document, CCI Phase 1 (SST), Document Ref SST_CCI-URD-UKMO-001. Available at: http://www.esa-sst-cci.org/sites/default/files/Documents/public/SST_cci%20URD%20Issue%202%20(2010%2011%2030) (accessed July 12, 2019).
Gouretski, V., Kennedy, J., Boyer, T., and Köhl, A. (2012). Consistent near-surface ocean warming since 1900 in two largely independent observing networks. Geophys. Res. Lett. 39:L19606. doi: 10.1029/2012GL052975
Grigorieva, V. G., and Badulin, S. I. (2016). Wind wave characteristics based on visual Observations and satellite altimetry. Oceanology 56, 19–24. doi: 10.1134/S0001437016010045
Grigorieva, V., and Gulev, S. (2008). “Extreme waves in visual wave observations by VOS,” in Proceedings of the Rogue Waves 2008 Workshop, October 13–15, Brest, 41–49. Available at: http://www.ifremer.fr/web-com/stw2008/rw/full/Grigorieva.pdf (accessed July 12, 2019).
Grigorieva, V. G., Gulev, S. K., and Gavrikov, A. V. (2017). Global historical archive of wind waves based on voluntary observing ship data. Oceanology 57, 229–231. doi: 10.1134/S0001437017020060
Gulev, S. K., and Grigorieva, V. (2004). Last century changes in ocean wind wave height from global visual wave data. Geophys. Res. Lett. 31:L24302. doi: 10.1029/2004GL021040
Gulev, S. K., and Grigorieva, V. (2006). Variability of the winter wind waves and swell in the north atlantic and north pacific as revealed by the voluntary observing ship data. J. Clim. 19, 5667–5685. doi: 10.1175/JCLI3936.1
Gulev, S. K., Grigorieva, V., Sterl, A., and Woolf, D. (2003). Assessment of the reliability of wave observations from voluntary observing ships: insights from the validation of a global wind wave climatology based on voluntary observing ship data. J. Geophys. Res. 108, 3236–3257. doi: 10.1029/2002JC001437
Hahn, C. J., and Warren, S. G. (2009). Extended Edited Cloud Reports from Ships and Land Stations over the Globe, 1952–1996 (2009 Update)’. Carbon Dioxide Information Analysis Center Numerical Data Package NDP-026C. Available at: https://cdiac.ess-dive.lbl.gov/epubs/ndp/ndp026c/ndp026c.html (accessed April, 2009).
Hahn, C. J., Warren, S. G., London, J., Jenne, R. L., and Chervin, R. M. (1988). Climatological Data for Clouds Over the Globe from Surface Observations (1988) (NDP-026). Carbon Dioxide Information Analysis Center (CDIAC), Oak Ridge National Laboratory (ORNL), Oak Ridge, TN (United States). doi: 10.3334/CDIAC/CLI.NDP026
Hanawa, K., Yasunaka, S., Manabe, T., and Iwasaka, N. (2000). Examination of correction to historical sst data using long-term coastal sst data taken around Japan. J. Meteorol. Soc. Japan Ser. II 78, 187–195. doi: 10.2151/jmsj1965.78.2_187
Hartmann, D. L., Klein Tank, A. M. G., Rusticucci, M., Alexander, L. V., Brönnimann, S., Charabi, Y., et al. (2013). “Observations: atmosphere and surface,” in Climate Change 2013: The Physical Science Basis. Contribution of Working Group I to the Fifth Assessment Report of the Intergovernmental Panel on Climate Change, eds T. F. Stocker, D. Qin, G.-K. Plattner, M. Tignor, S. K. Allen, J. Boschung, et al. (Cambridge: Cambridge University Press).
Hausfather, Z., Cowtan, K., Clarke, D. C., Jacobs, P., Richardson, M., and Rohde, R. (2017). Assessing recent warming using instrumentally homogeneous sea surface temperature records. Sci. Adv. 3:e1601207. doi: 10.1126/sciadv.1601207
Hausfather, Z., Cowtan, K., Menne, M. J., and Williams, C. N. (2016). Evaluating the impact of U.S. historical climatology network homogenization using the U.S. climate reference network. Geophys. Res. Lett. 43, 1695–1701. doi: 10.1002/2015GL067640
Hirahara, S., Ishii, M., and Fukuda, Y. (2014). Centennial-Scale sea surface temperature analysis and its uncertainty. J. Clim. 27, 57–75. doi: 10.1175/JCLI-D-12-00837.1
Hollmann, R., Merchant, C. J., Saunders, R., Downy, C., Buchwitz, M., and Cazenave, A. (2013). The ESA climate change initiative: satellite data records for essential climate variables. Bull. Am. Meteorol. Soc. 94, 1541–1552. doi: 10.1175/BAMS-D-11-00254.1
Huang, B., Angel, W., Boyer, T., Cheng, L., Chepurin, G., Freeman, E., et al. (2018). Evaluating SST analyses with independent ocean profile observations. J. Clim. 31, 5015–5030. doi: 10.1175/JCLI-D-17-0824.1
Huang, B., Thorne, P. W., Banzon, V. F., Boyer, T., Chepurin, G., Lawrimore, J. H., et al. (2017). Extended reconstructed sea surface temperature, version 5 (ersstv5): upgrades, validations, and intercomparisons. J. Clim. 30, 8179–8205. doi: 10.1175/JCLI-D-16-0836.1
Ingleby, B. (2010). Factors affecting ship and buoy data quality: a data assimilation perspective. J. Atmos. Ocean. Technol. 27, 1476–1489. doi: 10.1175/2010JTECHA1421.1
Ingleby, B., and Isaksen, L. (2018). Drifting buoy pressures: impact on NWP. Atmos. Sci. Lett. 19:e822. doi: 10.1002/asl.822
Ingleby, B., Moore, D., Sloan, C., and Dunn, R. (2013). Evolution and accuracy of surface humidity reports. J. Atmos. Ocean. Technol. 30, 2025–2043. doi: 10.1175/JTECH-D-12-00232.1
Jackson, D. L., and Wick, G. A. (2016). “Development of a 28-year (1987-2014) climatology of single and multi-sensor satellite-based retrievals of near-surface humidity and temperature,” in Proceedings of the 20th Conference on Air-Sea Interactions, (Madison, WI: American Meteorological Society).
James, R. W., and Fox, P. T. (1972). “Comparative sea surface temperature measurements,” in World Meteorological Organization, Reports on Marine Science Affairs, Report No. 5. (Geneva: World Meteorological Organization No. 336).
JCGM (2008). Evaluation of Measurement Data – Guide to the Expression of Uncertainty in Measurement (GUM)’. Available at: http://www.bipm.org/utils/common/documents/jcgm/JCGM_100_2008_E.pdf (accessed September 2008).
Josey, S. A., Kent, E. C., and Taylor, P. K. (1999). New insights into the ocean heat budget closure problem from analysis of the SOC air–sea flux climatology. J. Clim. 12, 2856–2880. doi: 10.1175/1520-0442(1999)012<2856:niitoh>2.0.co;2
Kaplan, A., Cane, M. A., Kushnir, Y., Clement, A. C., Blumenthal, M. B., and Rajagopalan, B. (1998). Analyses of global sea surface temperature 1856-1991. J. Geophys. Res. Oceans 103, 18567–18589. doi: 10.1029/97JC01736
Karspeck, A. R., Kaplan, A., and Sain, S. R. (2012). Bayesian modelling and ensemble reconstruction of mid-scale spatial variability in North Atlantic sea-surface temperatures for 1850-2008. Q. J. R. Meteorol. Soc. 138, 234–248. doi: 10.1002/qj.900
Kawai, Y., and Wada, A. (2007). Diurnal sea surface temperature variation and its impact on the atmosphere and ocean: a review. J. Oceanogr. 63, 721–744. doi: 10.1007/s10872-007-0063-0
Kennedy, J. J. (2014). A review of uncertainty in in situ measurements and data sets of sea surface temperature. Rev. Geophys. 52, 1–32. doi: 10.1002/2013RG000434
Kennedy, J. J., Rayner, N. A., Atkinson, C. P., and Killick, R. E. (2019). An ensemble data set of sea-surface temperature change from 1850: the Met Office Hadley Centre HadSST.4.0.0.0 data set. J. Geophys. Res. Atmos. 124. doi: 10.1029/2018JD029867
Kennedy, J. J., Rayner, N. A., Smith, R. O., Parker, D. E., and Saunby, M. (2011a). Reassessing biases and other uncertainties in sea surface temperature observations measured in situ since 1850: 1. measurement and sampling uncertainties. J. Geophys. Res. 116:D14103. doi: 10.1029/2010JD015218
Kennedy, J. J., Rayner, N. A., Smith, R. O., Parker, D. E., and Saunby, M. (2011b). Reassessing biases and other uncertainties in sea surface temperature observations measured in situ since 1850: 2. biases and homogenization. J. Geophys. Res. 116:D14104. doi: 10.1029/2010JD015220
Kent, E. C., and Berry, D. I. (2005). Quantifying random measurement errors in Voluntary Observing Ships’ meteorological observations. Inter. J. Climatol. 25, 843–856. doi: 10.1002/joc.1167
Kent, E. C., Berry, D. I., Woodruff, S. D., and Taylor, P. K. (2006). Voluntary Observing Ships: a Vital Observing System in Decline. CLIVAR Exchanges 11(3): 20– 21. Available at: http://www.clivar.org/sites/default/files/documents/Exchanges38.pdf (accessed 25 March 2019).
Kent, E. C., and Kaplan, A. (2006). Toward estimating climatic trends in SST. Part III: systematic biases. J. Atmos. Ocean. Technol. 23, 487–500. doi: 10.1175/JTECH1845.1
Kent, E. C., Kennedy, J. J., Berry, D. I., and Smith, R. O. (2010). Effects of instrumentation changes on sea surface temperature measured in situ. Wiley Interdiscip. Rev. Clim. Change 1, 718–728. doi: 10.1002/wcc.55
Kent, E. C., Kennedy, J. J., Smith, T. M., Hirahara, S., Huang, B., and Kaplan, A. (2017). A call for new approaches to quantifying biases in observations of sea surface temperature. Bull. Am. Meteorol. Soc. 98, 1601–1616. doi: 10.1175/BAMS-D-15-00251.1
Kent, E. C., Rayner, N. A., Berry, D. I., Saunby, M., Moat, B. I., Kennedy, J. J., et al. (2013). Global analysis of night marine air temperature and its uncertainty since 1880: the HadNMAT2 data set. J. Geophys. Res. Atmos. 118, 1281–1298. doi: 10.1002/jgrd.50152
Kent, E. C., and Taylor, P. K. (1996). Accuracy of humidity measurement on ships: consideration of solar radiation effects. J. Atmos. Ocean. Technol. 13, 1317–1321. doi: 10.1175/1520-0426(1996)013<1317:aohmos>2.0.co;2
Kent, E. C., and Taylor, P. K. (1997). Choice of a beaufort equivalent scale. J. Atmos. Ocean. Technol. 14, 228–242. doi: 10.1175/1520-0426(1997)014<0228:coabes>2.0.co;2
Kent, E. C., Taylor, P. K., Truscott, B. S., and Hopkins, J. S. (1993). The accuracy of voluntary observing ships’ meteorological observations-results of the VSOP-NA. J. Atmos. Ocean. Technol. 10, 591–608. doi: 10.1175/1520-0426(1993)010<0591:taovos>2.0.co;2
Kent, E. C., Woodruff, S. D., and Berry, D. I. (2007). Metadata from WMO publication no. 47 and an assessment of voluntary observing ship observation heights in ICOADS. J. Atmos. Ocean. Technol. 24, 214–234. doi: 10.1175/JTECH1949.1
Kinzel, J., Fennig, K., Schröder, M., Andersson, A., Bumke, K., and Hollmann, R. (2016). Decomposition of random errors inherent to HOAPS-3.2 near-surface humidity estimates using multiple triple collocation analysis. J. Atmos. Ocean. Technol. 33, 1455–1471. doi: 10.1175/JTECH-D-15-0122.1
Levitus, S., Antonov, J. I., Boyer, T. P., Locarnini, R. A., Garcia, H. E., and Mishonov, A. V. (2009). Global ocean heat content 1955-2008 in light of recently revealed instrumentation problems. Geophys. Res. Lett. 36:L07608. doi: 10.1029/2008GL037155
Liman, J., Schröder, M., Fennig, K., Andersson, A., and Hollmann, R. (2018). Uncertainty characterization of HOAPS 3.3 latent heat-flux-related parameters. Atmos. Meas. Tech. 11, 1793–1815. doi: 10.5194/amt-11-1793-2018
Loeb, N. G., Lyman, J. M., Johnson, G. C., Allan, R. P., Doelling, D. R., Wong, T., et al. (2012). Observed changes in top-of-the-atmosphere radiation and upper-ocean heating consistent within uncertainty. Nat. Geosci. 5:110. doi: 10.1038/ngeo1375
Manabe, T. (1999). The digitized kobe collection, phase I: historical surface marine meteorological observationsin the archive of the japan meteorological agency. Bull. Am. Meteorol. Soc. 80, 2703–2715. doi: 10.1175/1520-0477(1999)080<2703:tdkcpi>2.0.co;2
Martin, M., Dash, P., Ignatov, A., Banzon, V., Beggs, H., and Brasnett, B. (2012). Group for high resolution sea surface temperature (GHRSST) analysis fields inter-comparisons. Part 1: a GHRSST multi-product ensemble (GMPE). Deep Sea Res. Part II Top. Stud. Oceanogr. 7, 21–30. doi: 10.1016/j.dsr2.2012.04.013
Maury, M. F. (1854). “Maritime Conference held at Brussels for devising a uniform system of meteorological observations at sea, August and September, 1853,” in Proceedind of the Maritime Conference Explanations and Sailing Directions to Accompany the Wind and Current Charts, 6th Edn, (Philadelphia, PA: Biddle), 54–96.
McPhaden, M. J., Busalacchi, A. J., Cheney, R., Donguy, J. R., Gage, K. S., Halpern, D., et al. (1998). The tropical ocean-global atmosphere observing system: a decade of progress. J. Geophys. Res.: Oceans 103, 14169–14240. doi: 10.1029/97JC02906
Merchant, C. J., Embury, O., Rayner, N. A., Berry, D. I., Corlett, G. K., Lean, K., et al. (2012). A 20 year independent record of sea surface temperature for climate from Along-Track scanning radiometers. J. Geophys. Res. Oceans 117:C12013. doi: 10.1029/2012JC008400
Merchant, C. J., Embury, O., Roberts-Jones, J., Fiedler, E., Bulgin, C. E., Corlett, G. K., et al. (2014). Sea surface temperature datasets for climate applications from Phase 1 of the European Space Agency Climate Change Initiative (SST CCI). Geosci. Data J. 1, 179–191. doi: 10.1002/gdj3.20
Moat, B. I., Yelland, M. J., and Molland, A. F. (2006). Quantifying the airflow distortion over merchant ships. Part II: application of the model results. J. Atmos. Ocean. Technol. 23, 351–360. doi: 10.1175/JTECH1859.1
Morak-Bozzo, S., Merchant, C. J., Kent, E. C., Berry, D. I., and Carella, G. (2016). Climatological diurnal variability in sea surface temperature characterized from drifting buoy data. Geosci. Data J. 3, 20–28. doi: 10.1002/gdj3.35
Norris, J. R. (1998). Low cloud type over the ocean from surface observations. part I: relationship to surface meteorology and the vertical distribution of temperature and moisture. J. Clim. 11, 369–382. doi: 10.1175/1520-0442(1998)011<0369:lctoto>2.0.co;2
O’Carroll, A. G., Armstrong, E. M., Beggs, H. M., Bouali, M., Casey, K. S., Corlett, G. K., et al. (2019). Observational needs of sea surface temperature. Front. Mar. Sci. doi: 10.3389/fmars.2019.00420
O’Carroll, A. G., Eyre, J. R., and Saunders, R. W. (2008). Three Way error analysis between AATSR, AMSR-E, and in situ sea surface temperature observations. J. Atmos. Ocean. Technol. 25, 1197–1207. doi: 10.1175/2007JTECHO542.1
OceanSITES (2015). OceanSITES Data Format Reference Manual, NetCDF Conventions and Reference Tables Version 1.3. Available at: http://www.oceansites.org/docs/oceansites_data_format_reference_manual.pdf (accessed July, 2017).
OceanSITES (2016). Organization and Governance. Available at: http://www.oceansites.org/documents/Organization_and_Governance_17Mar2016.pdf (accessed Mar, 2016).
Petty, G. W. (1995). Frequencies and characteristics of global oceanic precipitation from shipboard present-weather reports. Bull. Am. Meteorol. Soc. 76, 1593–1616. doi: 10.1175/1520-0477(1995)076<1593:facogo>2.0.co;2
Plagge, A. M., Vandemark, D., and Chapron, B. (2012). Examining the impact of surface currents on satellite scatterometer and altimeter ocean winds. J. Atmos. Ocean. Technol. 29, 1776–1793. doi: 10.1175/JTECH-D-12-00017.1
Poli, P., Lucas, M., O’Carroll, A., Le Menn, M., David, A., Corlett, G. K., et al. (2018). The copernicus surface velocity platform drifter with barometer and reference sensor for temperature (SVP-BRST): genesis, design, and initial results. Ocean Sci. Discuss. 15, 199–214. doi: 10.5194/os-2018-109
Prytherch, J., Kent, E. C., Fangohr, S., and Berry, D. I. (2015). A comparison of SSM/I-derived global marine surface-specific humidity datasets. Inter. J. Climatol. 35, 2359–2381. doi: 10.1002/joc.4150
Rayner, N. A. (2017). User Requirements Document, CCI Phase 1 (SST), Document ref SST_CCI-URD-UKMO-201. Available at: http://www.esa-sst-cci.org/PUG/pdf/SST_CCI-URD-UKMO-201-Issue_2.1-signed.pdf (accessed July 12, 2019).
Rayner, N. A., Brohan, P., Parker, D. E., Folland, C. K., Kennedy, J. J., Vanicek, M., et al. (2006). Improved analyses of changes and uncertainties in sea surface temperature measured in situ since the mid-nineteenth century: the HadSST2 dzataset. J. Clim. 19, 446–469. doi: 10.1175/JCLI3637.1
Rayner, N. A., Parker, D. E., Horton, E. B., Folland, C. K., Alexander, L. V., Rowell, D. P., et al. (2003). Global analyses of sea surface temperature, sea ice, and night marine air temperature since the late nineteenth century. J. Geophys. Res. 108, 4407–4437. doi: 10.1029/2002JD002670
Reverdin, G., Boutin, J., Martin, N., Lourenco, A., Bouruet-Aubertot, P., Lavin, A., et al. (2010). Temperature measurements from surface drifters. J. Atmos. Ocean. Technol. 27, 1403–1409. doi: 10.1175/2010JTECHO741.1
Reynolds, R. W., Rayner, N. A., Smith, T. M., Stokes, D. C., and Wang, W. (2002). An improved in situ and satellite SST analysis for climate. J. Clim. 15, 1609–1625. doi: 10.1175/1520-0442(2002)015<1609:aiisas>2.0.co;2
Rhines, A., Tingley, M. P., McKinnon, K. A., and Huybers, P. (2015). Decoding the precision of historical temperature observations: decoding observational precision. Q. J. R. Meteorol. Soc. 141, 2923–2933. doi: 10.1002/qj.2612
Richardson, M., Cowtan, K., and Millar, R. J. (2018). Global temperature definition affects achievement of long-term climate goals. Environ. Res. Lett. 13:054004. doi: 10.1088/1748-9326/aab305
Rodriguez, R., Bourassa, M. A., Chelton, D., Farrar, J. T., Long, D., Perkovic-Martin, D., et al. (2019). The winds and currents mission concept. Front. Mar. Sci. doi: 10.3389/fmars.2019.00438
Schwab, M., Meinke, I., Vanderlinden, J.-P., and Touili, N. (2015). Empirical Working Paper: Analysing Stakeholders’ Needs and Understanding, European Climate and Weather Events: Interpretation and Attribution Deliverable D4.2. Available at: https://eucleia.eu/wp-content/uploads/2018/07/d4-2_final.pdf (accessed July 12, 2019).
Smith, S. R., Briggs, K., Bourassa, M. A., Elya, J., and Paver, C. R. (2018). Shipboard automated meteorological and oceanographic system data archive: 2005-2017. Geosci. Data J. 5, 73–86. doi: 10.1002/gdj3.59
Smith, S. R., Alory, G., Andersson, A., Asher, W., Baker, A., Berry, D. I., et al. (2019). Ship-based contributions to global ocean, weather, and climate observing systems. Front. Mar. Sci. doi: 10.3389/fmars.2019.00434
Smith, S. R., Bourassa, M. A., and Sharp, R. J. (1999). Establishing more truth in true winds. J. Atmos. Ocean. Technol. 16, 939–952. doi: 10.1175/1520-0426(1999)016<0939:emtitw>2.0.co;2
Smith, S. R., Freeman, J. E., Lubker, S. J., Woodruff, S. D., Worley, S. J., and Angel, W. E. (2016). The International Maritime Meteorological Archive (IMMA) Format. Available at: http://icoads.noaa.gov/e-doc/imma/R3.0-imma1.pdf (accessed July 2016).
Smith, S. R., Legler, D. M., and Verzone, K. V. (2001). Quantifying uncertainties in ncep reanalyses using high-quality research vessel observations. J. Clim. 14, 4062–4072. doi: 10.1175/1520-0442(2001)014<4062:quinru>2.0.co;2
Snook, I. B. (2016). Fiducial Reference Measurements for Validation of Surface Temperature from Satellites (FRM4STS), Project Brochure’. OFE-D-30-V1-Iss-1. Available at: http://www.frm4sts.org/wp-content/uploads/sites/3/2017/12/D30-FRM4STS-project-brochure-with-cover-16Nov16-signed.pdf (accessed October 2016).
Stoffelen, A. (1998). Toward the true near-surface wind speed: error modeling and calibration using triple collocation. J. Geophys. Res. Oceans 103, 7755–7766. doi: 10.1029/97JC03180
Stoffelen, A., Jur Vogelzang, J., and Wenming, L. (2015). On Buoys, Scatterometers and Reanalyses for Globally Representative Winds. Available at: https://www.nwpsaf.eu/publications/tech_reports/nwpsaf-kn-tr-024.pdf (accessed August 2015).
Thomas, B. R., Kent, E. C., and Swail, V. R. (2005). Methods to homogenize wind speeds from ships and buoys. Inter. J. Climatol. 25, 979–995. doi: 10.1002/joc.1176
Thomas, B. R., Kent, E. C., Swail, V. R., and Berry, D. I. (2008). Trends in ship wind speeds adjusted for observation method and height. Inter. J. Climatol. 28, 747–763. doi: 10.1002/joc.1570
Thorne, P. W., Allan, R. J., Ashcroft, L., Brohan, P., Dunn, R. J. H., and Menne, M. J. (2017a). Toward an integrated set of surface meteorological observations for climate science and applications. Bull. Am. Meteorol. Soc. 98, 2689–2702. doi: 10.1175/BAMS-D-16-0165.1
Thorne, P. W., Diamond, H. J., Goodison, B., Harrigan, S., Hausfather, Z., and Ingleby, N. B. (2018). Towards a global land surface climate fiducial reference measurements network. Inter. J. Climatol. 38, 2760–2774. doi: 10.1002/joc.5458
Thorne, P. W., Madonna, F., Schulz, J., Oakley, T., Ingleby, B., Rosoldi, M., et al. (2017b). Making better sense of the mosaic of environmental measurement networks: a system-of-systems approach and quantitative assessment. Geosci. Instrum. Method. Data Sys. 6, 453–472. doi: 10.5194/gi-6-453-2017
Tokinaga, H., and Xie, S.-P. (2011). Wave and anemometer-based sea surface wind (WASWind) for climate change analysis. J. Clim. 24, 267–285. doi: 10.1175/2010JCLI3789.1
Verhoef, A., Vogelzang, J., Verspeek, J., and Stoffelen, A. (2017). Long term scatterometer wind climate data records. IEEE J. Sel. Top. Appl. Earth Obs. Remote Sens. 10, 2186–2194. doi: 10.1109/JSTARS.2016.2615873
Weller, R. A. (2018). “Observing Surface Meteorology and Air-Sea Fluxes,” in ., eds R. Venkatesan, A. Tandon, E. D’Asaro, and M. A. Atmanand (Cham: Springer International Publishing), 17–35. doi: 10.1007/978-3-319-66493-4_2
Wentz, F. J., Ricciardulli, L., Rodriguez, E., Stiles, B. W., Bourassa, M. A., and Long, D. G. (2017). Evaluating and extending the ocean wind climate data record. IEEE J. Sel. Top. Appl. Earth Obs. Remote Sens. 10, 2165–2185. doi: 10.1109/JSTARS.2016.2643641
WMO (2012). Manual on Marine Meteorological Services, Volume I – Global Aspects’. Available at: https://library.wmo.int/pmb_ged/wmo_558_en-v1.pdf (accessed July 12, 2019).
Woodruff, S. D., Diaz, H. F., Worley, S. J., Reynolds, R. W., and Lubker, S. J. (2005). Early ship observational data and icoads. Clim. Change 73, 169–194. doi: 10.1007/s10584-005-3456-3
Woodruff, S. D., Slutz, R. J., Jenne, R. L., and Steurer, P. M. (1987). A comprehensive ocean-atmosphere data set. Bull. Am. Meteorol. Soc. 68, 1239–1250. doi: 10.1175/1520-0477(1987)068<1239:acoads>2.0.co;2
Woodruff, S. D., Worley, S. J., Lubker, S. J., Ji, Z., Eric Freeman, J., Berry, D. I., et al. (2011). ICOADS Release 2.5: extensions and enhancements to the surface marine meteorological archive. Inter. J. Climatol. 31, 951–967. doi: 10.1002/joc.2103
Worley, S. J., Woodruff, S. D., Reynolds, R. W., Lubker, S. J., and Lott, N. (2005). ICOADS release 2.1 data and products. Inter. J. Climatol. 25, 823–842. doi: 10.1002/joc.1166
Xu, F., and Ignatov, A. (2016). Error characterization in I Quam SSTs using triple collocations with satellite measurements. Geophys. Res. Lett. 43:834. doi: 10.1002/2016GL070287
Young, I. R., Zieger, S., and Babanin, A. V. (2011). Global trends in wind speed and wave height. Science 332, 451–455. doi: 10.1126/science.1197219
Keywords: marine, climate, measurement, uncertainty, ocean, atmosphere, ship, buoy
Citation: Kent EC, Rayner NA, Berry DI, Eastman R, Grigorieva VG, Huang B, Kennedy JJ, Smith SR and Willett KM (2019) Observing Requirements for Long-Term Climate Records at the Ocean Surface. Front. Mar. Sci. 6:441. doi: 10.3389/fmars.2019.00441
Received: 15 November 2018; Accepted: 05 July 2019;
Published: 30 July 2019.
Edited by:
Amos Tiereyangn Kabo-Bah, University of Energy and Natural Resources, GhanaReviewed by:
Zeke J. Hausfather, University of California, Berkeley, United StatesMatthew Mayernik, National Center for Atmospheric Research (UCAR), United States
Copyright © 2019 Kent, Rayner, Berry, Eastman, Grigorieva, Huang, Kennedy, Smith and Willett. This is an open-access article distributed under the terms of the Creative Commons Attribution License (CC BY). The use, distribution or reproduction in other forums is permitted, provided the original author(s) and the copyright owner(s) are credited and that the original publication in this journal is cited, in accordance with accepted academic practice. No use, distribution or reproduction is permitted which does not comply with these terms.
*Correspondence: Elizabeth C. Kent, eck@noc.ac.uk