- 1Smithsonian Tropical Research Institute, Balboa, Panama
- 2Tobii Technology AB, Danderyd, Sweden
- 3Centro de Ciencias del Mar y Limnología, Universidad de Panamá, Panama City, Panama
Sea turtles migrate from breeding grounds to foraging areas through the territorial waters of several countries. Olive ridleys lack clear migratory routes, which makes it a challenge for conservation. This study aimed to evaluate if the migratory behavior of olive ridleys nesting on the Pacific Coast of Panama is correlated to environmental conditions along the migration and to identify common foraging areas. Thirty-four olive ridley turtles were tagged with satellite transmitters along the Pacific coast of Panama and tracked for up to 9 months. A Hidden Markov Model was used to identify behavioral states (foraging and migrating) and their correlation to chlorophyll a concentration, sea surface temperature (SST), eddy kinetic energy (EKE), and primary productivity. Turtle tracks overlapped with eddies and industrial fishing areas. The probability of foraging rather than migrating was positively correlated to SST, productivity and chlorophyll levels and negatively correlated to EKE. Turtles spent an average 30% of their time migrating and 70% foraging. Only 8.4% of the turtle locations occurred within an eddy, and 82.7% of the locations overlapped with previously described industrial fishing areas. Although this sea turtle species seems to migrate freely, without clear migratory corridors, turtles may have preferred foraging destinations that they travel to through different migratory routes, such as the gulfs of Tehuantepec, Fonseca, and Guayaquil. Turtles traveled up to 6,684 km through nine countries, most of them foraging in oceanic waters in Salvador, Costa Rica, and Panama, which highlights the importance of collaborative conservation strategies throughout foraging and nesting areas. The creation of a regional management unit (RMU), including Costa Rica, Panama, and Colombia, is discussed based on the observed seasonal overlap with the use of multiple fishing gears in coastal and oceanic fishing areas.
Introduction
Understanding the role played by environmental factors on animal behavior is key to developing effective conservation strategies, especially for endangered migratory species with migratory routes that are geographically unrestricted. The influence of oceanic variables such as currents or sea surface temperature (SST) on the migratory patterns and foraging behavior of marine species may be better understood by combining the results of satellite tracking with proximate environmental data (Gaspar et al., 2006; Revelles et al., 2007; Godley et al., 2008; Schick et al., 2013). For the present study, olive ridley turtles (Lepidochelys olivacea) were tagged with satellite transmitters to monitor their movements within the Eastern Tropical Pacific (ETP) and evaluate any correlation between their foraging or migratory behavior and local environmental conditions, as well as the spatial and temporal overlap with industrial fishing areas.
Olive ridleys are considered the most abundant sea turtle species, inhabiting all tropical and subtropical waters (Godfrey and Godley, 2008). This species has a generalist diet (Bjorndal, 1985; McMahon et al., 2007), and mainly feeds on pelagic organisms through its extensive distribution (Polovina et al., 2006). In the Tropical Eastern Pacific this species nest from northwestern Mexico to northern Peru (Cliffton et al., 1995; Kelez et al., 2009) and has an estimated population size of 1.39 million turtles (Eguchi et al., 2007).
The nesting season for the olive ridley in the ETP occurs between July and December (Caldwell and Casebeer, 1964; Cornelius, 1982; Pitman, 1990; Eckrich and Owens, 1995; Plotkin, 2007) and may take the form of group nesting (known as Arribadas) where hundreds of individuals nest at the same location over a period of several days (Eckrich and Owens, 1995; Bernardo and Plotkin, 2007). Olive ridleys are observed to be highly vagile; moving continuously throughout novel marine areas covering hundreds of kilometers (Luschi et al., 2003; Polovina et al., 2004; Spotila, 2004; Plotkin, 2010). This migratory behavior has been termed “nomadism” and is considered to be a unique advantage to be able to adapt to a highly dynamic environment that, at times, becomes unfavorable (Seminoff et al., 2008). During migrations, routes between individuals do not generally overlap in space or time and thus may lack migratory corridors, making group analysis of tracks a particular challenge (Plotkin, 2010). This nomadic nature also presents a challenge in conservation efforts as foraging areas may vary significantly between populations and individuals. Not all olive ridley populations, however, are oceanic nomads; some groups have been observed to habitually occupy neritic waters near nesting sites, as observed in North West Australia (McMahon et al., 2007; Whiting et al., 2007), French Guiana (Plot et al., 2015), and Oman (Rees et al., 2012). This behavior may be explained by nearby food resource abundance, favorable temperatures, or other oceanic conditions (Plot et al., 2015; Chambault et al., 2016, 2017) but nevertheless demonstrates significant behavioral plasticity for the species throughout their global range.
Through their nomadic migrations these turtles can encounter different local environmental conditions, which can determine the travel direction, traveling speed, diving behavior and residence time (Chambault et al., 2016, 2017, 2019). Mesoscale eddies, which drive ocean productivity in the open ocean, transport heat, salts and nutrients (Revelles et al., 2007), are known to aggregate different sea turtle species, both in cold-core cyclonic eddies, and in warm-core anticyclonic eddies (Polovina et al., 2006; Mansfield et al., 2014; Chambault et al., 2016, 2019).
As many other marine species, olive ridleys are subject to incidental fishing, by large and small-scale fisheries (Cheng and Chen, 1997; Pandav et al., 1998; Lewison et al., 2004; Pinedo and Polacheck, 2004), in which they are caught on fishing nets (Montero et al., 2016) and in pelagic long lines fishing gears (Ramirez and Ania, 2000). Incidental catch can occur when turtles directly feed on bait, by entanglement on fishing lines or by getting incidentally caught on fishing nets (Pinedo and Polacheck, 2004). Therefore, the probability of an incidental bycatch increases when fishing areas overlap with the turtles high-use areas (Montero et al., 2016). In the Eastern Pacific region, bycatch is one of the highest on the planet and is associated to multiple fishing gear that include gillnets, long-liners and trawling (Wallace et al., 2010, 2013).
The ETP hosts several major converging current systems. Nearby mountain ranges subject to high winds can give rise to localized coastal upwelling throughout the region (D’Croz and O’Dea, 2007). Thus, regions of low temperature and high productivity (and vice versa) occur during different times of the year (Fiedler, 2002; Fiedler and Talley, 2006; Saba et al., 2008). These changes in water temperature and productivity are demonstrated during the El Niño Southern Oscillation, in which pressure gradients over the Pacific Ocean give rise to complex and intense wind–current interactions, highly influencing marine conditions within the ETP. As an oceanic realm with such contrasted oceanographic condition, resident turtles must adapt to a highly dynamic habitat, and at the same time, overlap with industrial and artisanal fisheries, which also take advantage of the high productive waters. Therefore, there is a need to determine the correlations between olive ridley movements and relevant oceanographic conditions to increase our understanding of the behavioral ecology of marine organisms that occupy dynamic habitats and to aid conservation strategies for this protected species.
Materials and Methods
Satellite Tagging
A group of 34 solitary adult olive ridley turtles were temporarily captured from the Pacific coast of Panama for the purpose of satellite tagging from August 2009 to May 2011 on nesting beaches and the surrounding waters off the Las Perlas Archipelago in the Gulf of Panama and near Coiba Island in the Gulf of Chiriqui (Table 1). Turtles were tagged with SPOT5 AM-S244A satellite tags from Wildlife Computers Inc. (Redmond, WA, United States), which provided the location (latitude and longitude) of the turtle for variable time frames after release.
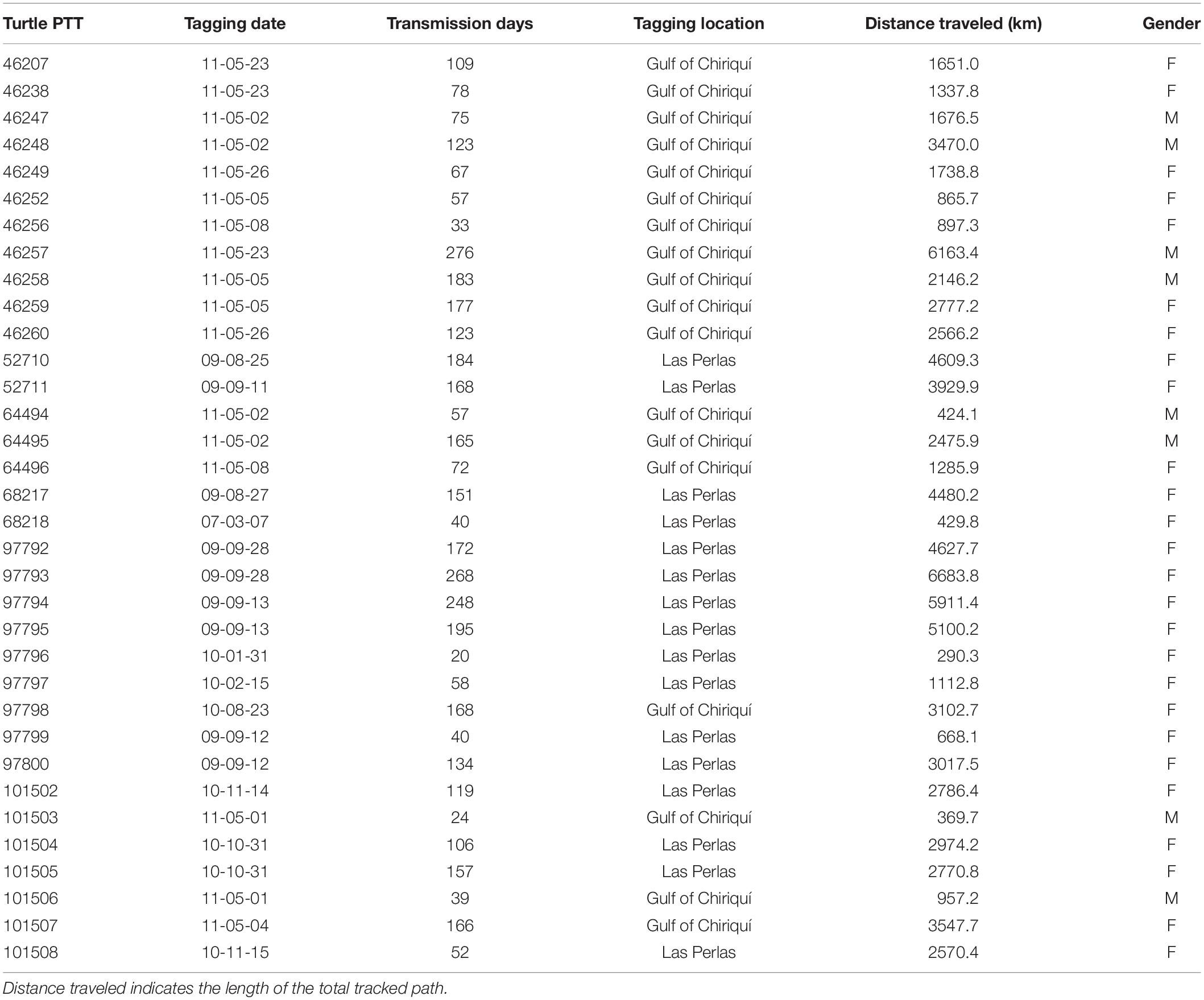
Table 1. Summary of tracked olive ridley turtles tagged off the Pacific Coast of Panama, tagging location, and tracking length.
Upon capture, curved carapace length was measured and the area for device attachment was lightly sanded to remove encrusted organisms. Epoxy glue was applied to the shell to which the tracking device was attached and once set it was coated in anti-fouling paint. Flipper tags were applied to turtles when not already present. Tracking devices were set to transmit hourly with data collected via the Argos satellite system and imported into Seaturtle.org’s Satellite Tracking and Analysis Tool (Coyne and Godley, 2005) for preliminary filtering. To ensure high quality, all locations with an accuracy classified as “Z” (unknown location) and dry were removed. Any values that implied speeds >5 km/h were discarded in accordance with established procedures (Rees et al., 2012). The Animal Care and Use Committee of the Smithsonian Tropical Research Institute approved tagging procedures.
Track Correction
We used a Bayesian State-Space model for animal movements to correct the tracks based on Argos satellite tracking data error. This model is a first difference correlated random walk model that simultaneously deals with estimation error and observations that occurred irregularly in time (Jonsen et al., 2005). The model was run using the function “fit_ssm” in the package bsam (Jonsen, 2016) in R version 3.4.4 (R Core Team, 2018). It was set to have four time steps per day (tstep = 0.25), 5000 samples during the adaptation and update phase, a thinning of 10 to minimize within-chain sample autocorrelation, and a span of 0.2 for the degree of smoothing.
Behavioral States Identification and Correlation With Environmental Variables
Each location along the corrected tracks was matched to six environmental variables: SST, chlorophyll (Chl), productivity (Prod), marine currents (Curr; U and V vectors), and the presence of eddies at the time and location of each transmission. The eddy kinetic energy (EKE) was calculated for each location using the U and V vectors extracted from the V and U-“Currents, Geostrophic, Aviso, Global (1 Day Composite)” database at a 0.25 decimal degree daily resolution1. Daily SST was extracted from the “Multi-scale Ultra-high Resolution (MUR) SST analysis fv04.1, Global, 0.011 Degree, Daily, Analyzed SST” data at a 0.01 decimal degree resolution2. Monthly composite Chlorophyll-a concentration level (mg/m3) data was extracted from the “Chlorophyll-a, Aqua MODIS, NPP, L3SMI, Global, Science Quality (Monthly Composite)” data set at a 0.0417 decimal degree spatial resolution3. Daily net primary productivity of Carbon (mg C/m2) was extracted from “Primary Productivity, Aqua MODIS, NPP, Global, 1-Day, EXPERIMENTAL” data set at a 0.0147 degree spatial resolution4. These data sets were obtained and matched to each turtle location and date by using the function “xtracto” in the R package xtractomatic (Mendelssohn, 2018).
We used the Mesoscale Eddy Trajectory Atlas Product from AVISO Satellite Altimetry Data5 to identify the presence of eddies during each transmission day and location. A circular buffer zone was created around each eddy center; the buffer diameter was equivalent to the diameter of each eddy on a given day (r2). We overlaid the eddy spatial layer with the turtle location layer and identified turtle locations within eddies buffer zones, we filtered out spatial matches that didn’t have a temporal match. These analyses were done with the Spatial analyst tool in ArcGIS (v10.6).
After all turtle locations were matched to real-time environmental conditions, we used a Hidden Markov Model (HMM) to identify different behavioral states (foraging and migrating) along corrected tracks. We used the environmental variables as model covariates to find any potential correlation between behavioral states and SST, Chl, Prod, Curr, and EKE. We fitted the model using the function “fitHMM” in the R package moveHMM (Michelot et al., 2016). We set the initial values to two states: 5 ± 5 and 50 ± 20 km for the step mean and pi to 0 for the turning angle and included the environmental variables as covariates. The presence of eddies was not included in the model owing to the small sample size. A kernel density plot was used to identify high- and intermediate-use foraging areas. The analysis was performed only using locations that the HMM model identified to be in the foraging behavioral state. Kernel density was plotted using bilinear interpolation and classified in two categories: High-use foraging areas were defined as areas where 50% of foraging transmissions occurred, intermediate-use areas were where 75% of the foraging transmissions occurred. The analysis was carried out in ArcGIS (v10.6).
Turtle Tracks Overlapping With Industrial Fishing Areas
Fishing effort was obtained from Global Fishing Watch (GFM)6 (Kroodsma et al., 2018). The time frame of Kroodsma’s data (2012–2016) did not overlap our study period (2009–2012). Therefore, we calculated a trimestral mean for our study area and used it as a reference mean for fishing effort, the first trimester corresponding to the north hemisphere’s winter, the second to spring, the third to summer and the fourth to fall. We used ArcMap v10.6 (ESRI) and the Spatial Analyst tool to create kernel density plots and identify areas with low, intermediate, and high fishing effort along sea turtle tracks. We calculated a mean fishing effort for each trimester of the year and extracted the interpolated data to each turtle coordinate location during the corresponding trimester. Fishing effort was divided into three categories: low: from the smallest values excluding 0 to the first quartile (25% of effort); intermediate: from the first to third quartiles (50% of effort); and high: above the third quartile (75% of effort).
Results
General Findings
Olive ridley turtles (n = 34) were tagged on the Pacific coast of Panama between 23 August 2009 and 22 Mach 2011 (except for one in May 2007) (Table 1) and were observed to travel substantial distances in numerous directions from their starting location (Figure 1A and Table 1). Females (n = 26) and males (n = 8) traveled distance was not significantly different (Mann–Whitney Z score p < 0.05) with an overall average of 2,629.9 ± 0.8 km in 121 ± 4 days representing an average speed of 21.8 km/day or 0.9 km/h. Turtles traveled through nine different countries and in international waters, with most locations occurring within the Panamanian Economic Exclusive Zone (60%) and Costa Rica (19.3%) (Table 2).
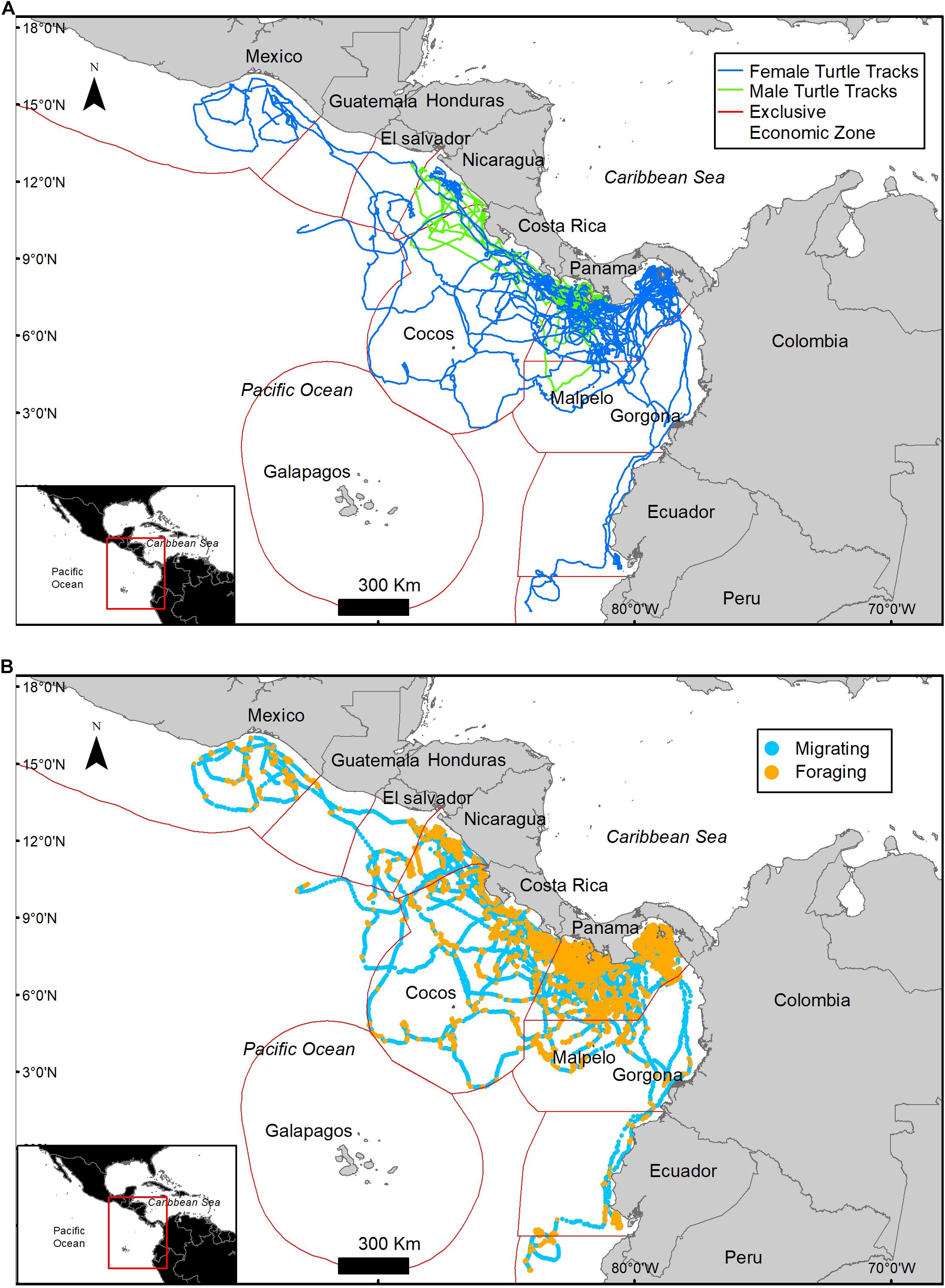
Figure 1. Migratory tracks of 34 olive ridley turtles tagged off the Pacific Coast of Panama. Turtles traveled over the Exclusive Economic Zones of nine countries and in international waters (A). Behavioral states identified by the HMM model, foraging (orange) and migrating (blue) (B).
Four turtles (PTTs: 68218, 101503, 46256, and 64494) were not included in the bsam model because their model did not converge. The initial database, which included all turtles, had 10,566 Argos locations distributed among error classes, as follows (in descending order): B (27.4%), 0 (22.2%), A (22.1%), 1 (17.4%), 2 (8.6%), and 3 (2.4%). After track correction the database increased to 16,024 coordinate locations.
Behavioral States Identification and Correlation With Environmental Variables
The HMM described two behavioral states: foraging (state 1) as having a step distance (distance between time intervals) of 3.9 ± 2.9 km and a turning angle of −0.02 ± 0.1rad and migrating (state 2) having a step distance of 9.3 ± 5.9 km and a turning angle of 0.001 ± 13.2rad. The probability of switching between behavioral states (from migrating to foraging or vice versa) was correlated with local environmental conditions under stationary long-term distribution (Table 3). The probability of a turtle being in state 1 (foraging) increased as SST and Chl increased, and the probability of being in state 2 (migrating) decreased as temperature and Chl decreased (Figures 2A,B). The effect of the EKE was the opposite, with state 1 being more probable with low EKE and state 2 more probable with high EKE (Figure 2C). Tracked turtles spent most of their time in water with Chl levels of 0.75 ± 0.02 mg/m–3, EKE 0.02 ± 0.00 cm2/s2, Prod 1038.38 ± 24.41 mg C/m2, and SST 27.82 ± 0.01°C. However, there was a significant difference in Chl levels between behavioral states; turtles foraged in waters with significantly higher Chl (0.91 ± 0.04 mg/m–3) than when they were migrating (0.41 ± 0.01 mg/m–3) (p < 0.005, Figure 3). Turtles spent an average of 30 ± 17% of their time in state 2 (migrating) and 70 ± 17% in state 1 (foraging) (Figure 1B). All results are presented as mean ± SE.
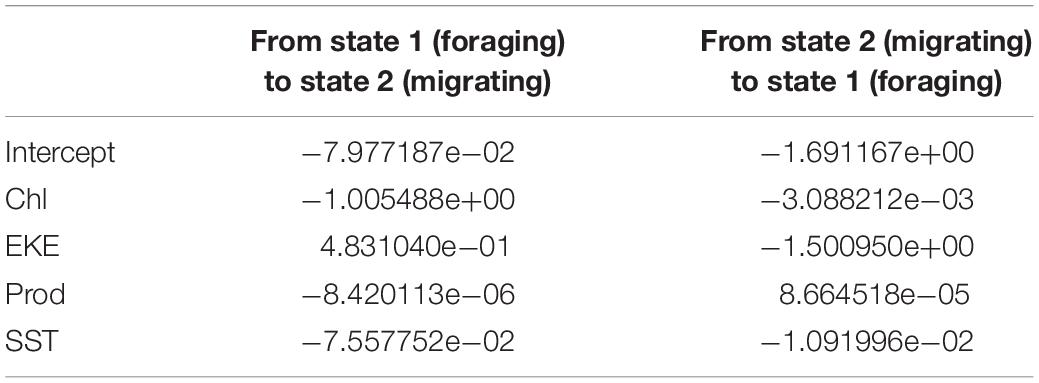
Table 3. Model coefficient of correlation between each environmental variable (model covariate) to the probabilities of switching between behavioral states (1: foraging and 2: migration).
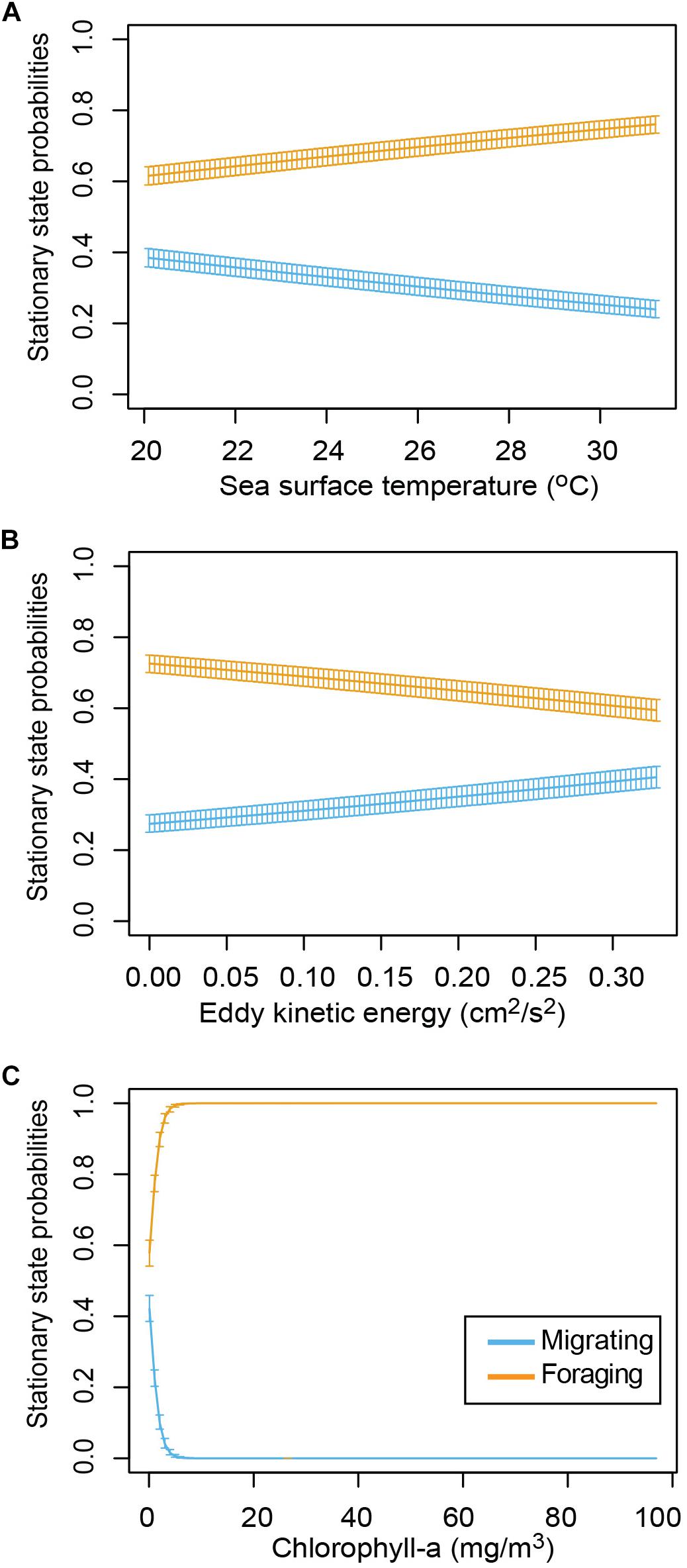
Figure 2. Long-term probabilities (stationary matrix of Hidden Markov Model) of turtles being in each behavioral state at different values of the covariates (95% confidence intervals). (A) Sea surface temperature, (B) eddy kinetic energy, and (C) chlorophyll-a during each behavioral state.
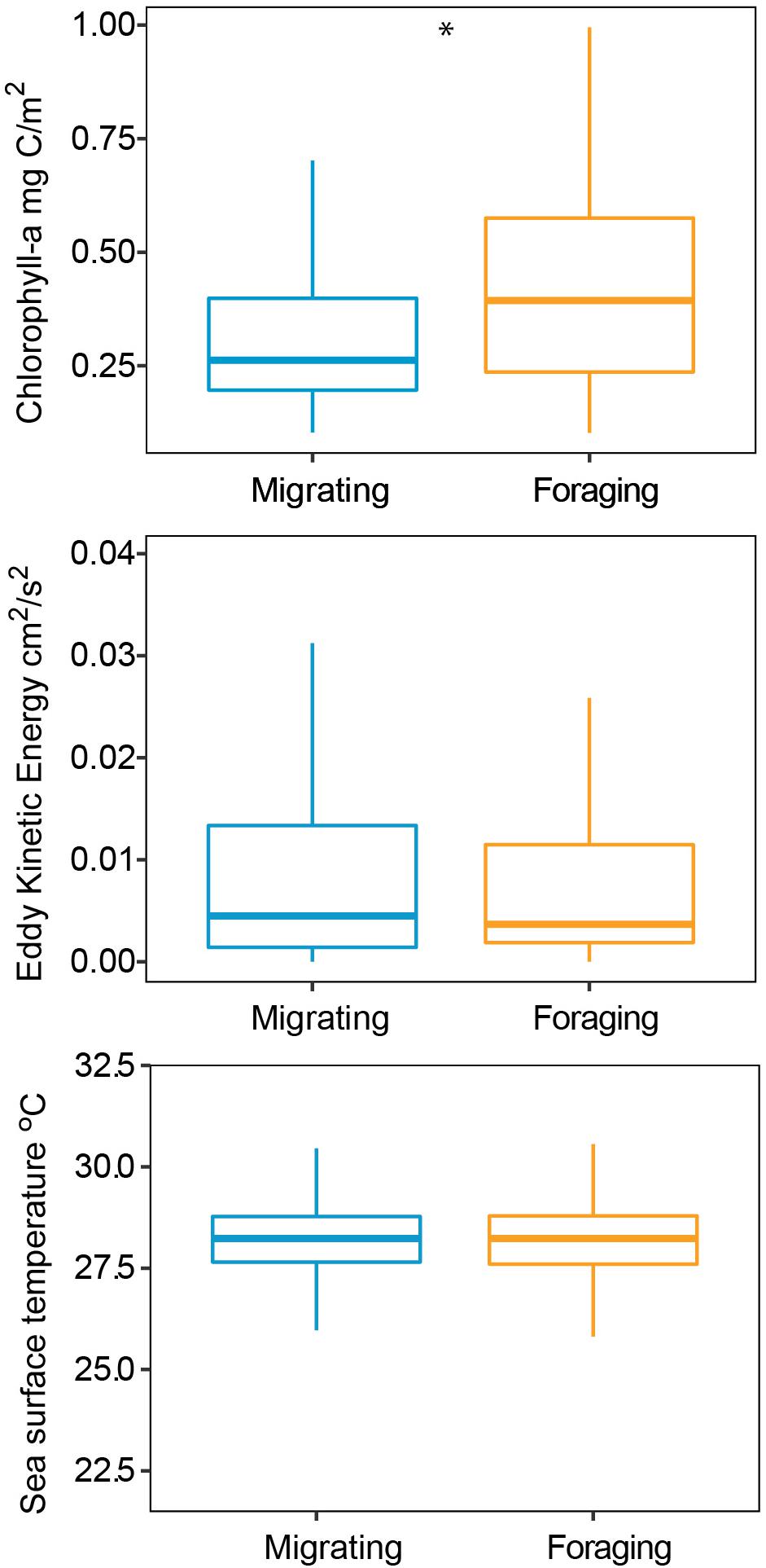
Figure 3. Environmental conditions during migration and foraging states modeled by a Hidden Markov Model, turtles preferred to forage when chlorophyll levels were higher. Star indicates a significant difference.
A total of 765 eddies occurred in the study area during the study period, 51% of these eddies were cyclonic and 49% were anti-cyclonic, with an average radius of 133.6 ± 7.3 km. Only 8.4% of the turtle locations occurred within an eddy (Figure 4). When migrating an average of 11 ± 12.6% of the locations were within an eddy and an average of 6.3 ± 9% were within an eddy when foraging (Table 4). Turtle tracks overlapped temporally and spatially with cyclonic and anti-cyclonic eddies: 4.4% of the locations within eddies were in anti-cyclonic eddies and 4% in cyclonic eddies (Table 4 and Figure 4). High-use foraging areas identified by a kernel density analysis (where 50% of the locations in state 2 occurred) were primarily located in the territorial waters of Panama, Costa Rica, Nicaragua, and southern Mexico (Figure 5).
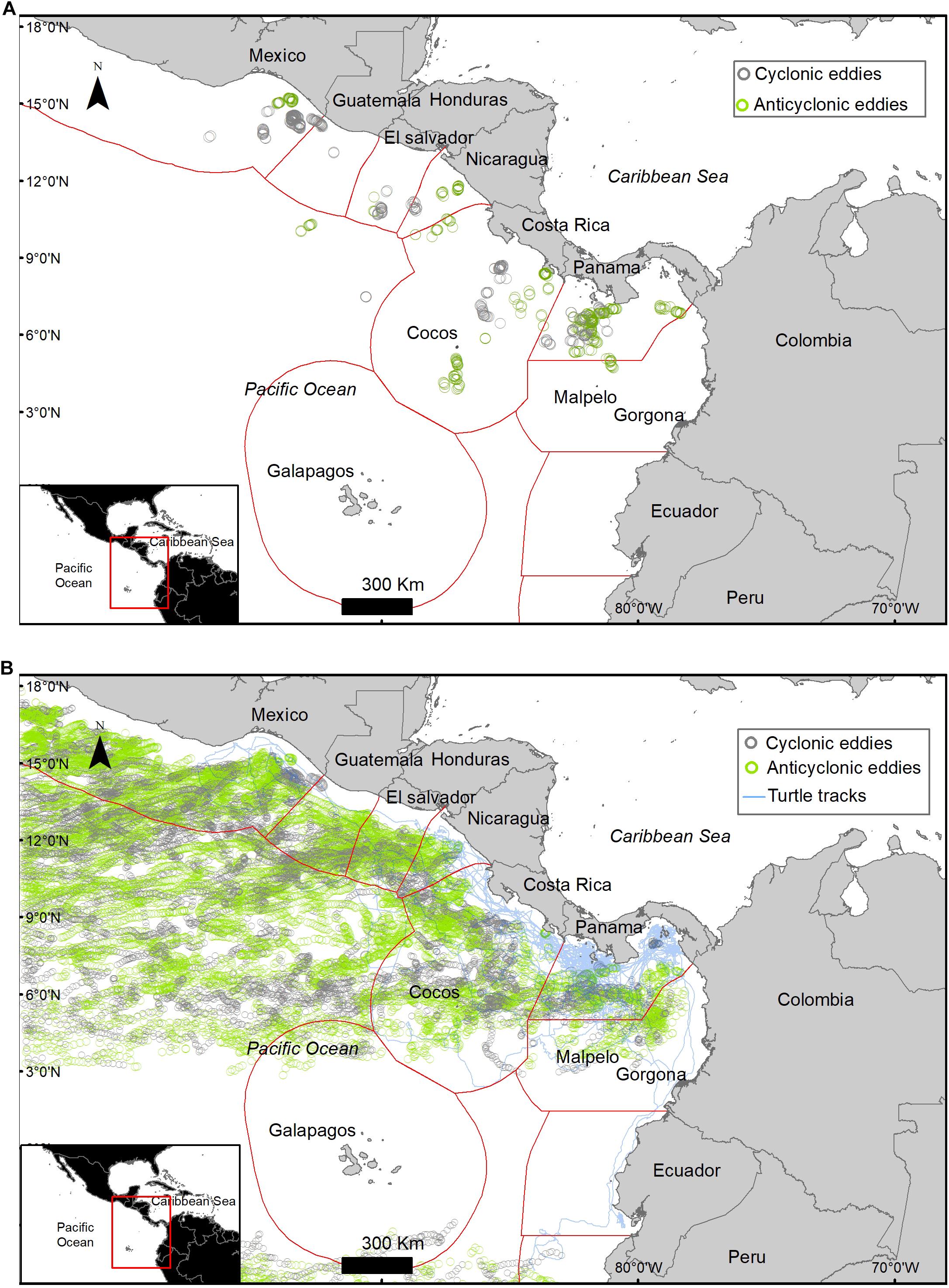
Figure 4. (A) Turtle locations that overlapped spatially and temporally within the radius of eddies (8.4% of the locations). (B) Daily location of eddies that occurred during the length of the study at the study area (n = 765), a single eddy can be plotted multiple times as it moves through space in time; cyclonic eddy (gray) and anticyclone eddy (green).
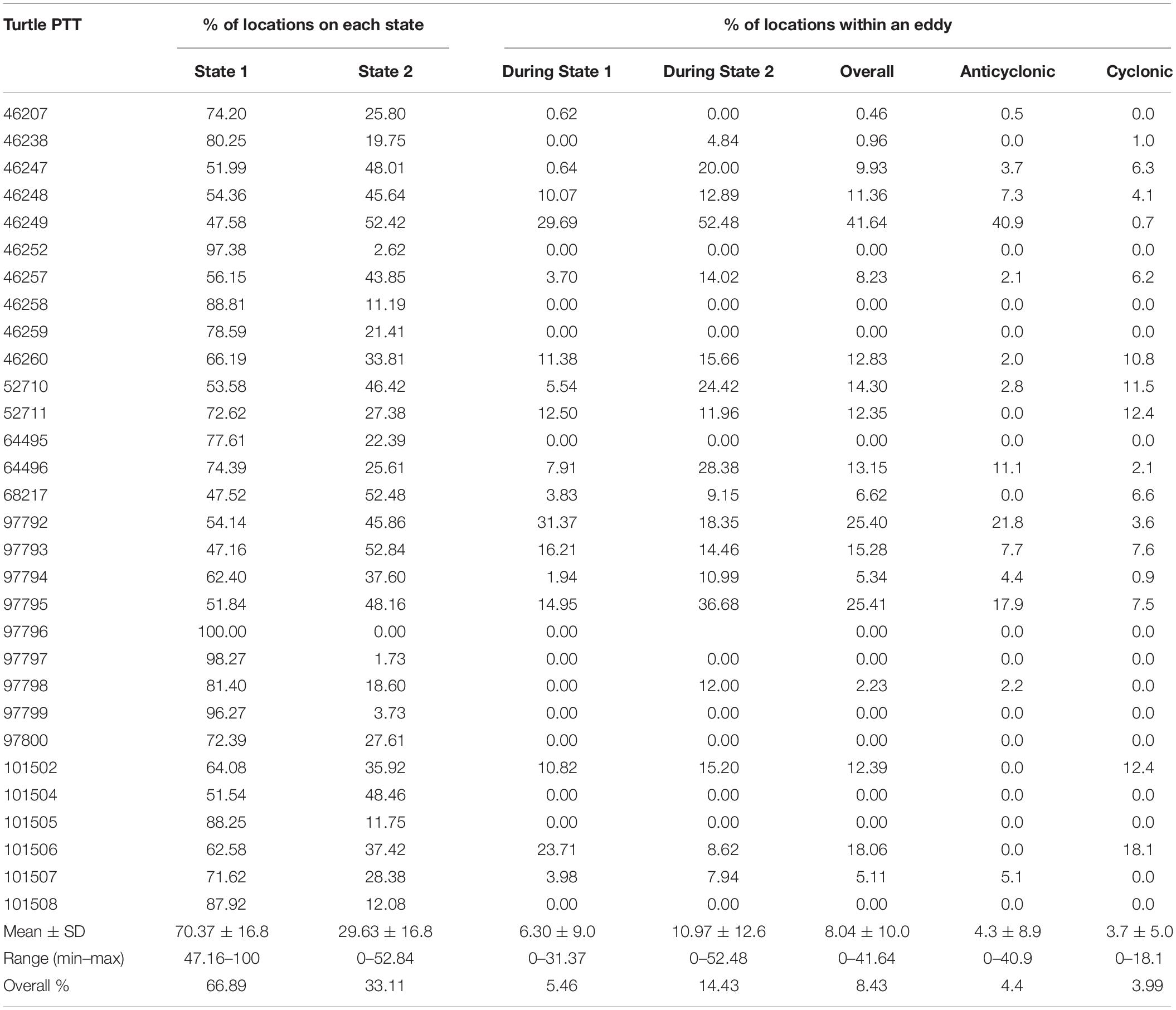
Table 4. Percentage of turtle transmissions on each behavioral state (1: foraging and 2: migration) and the percentage of locations within an eddy during each behavioral state, overall and in anti-cyclonic and cyclonic eddies.
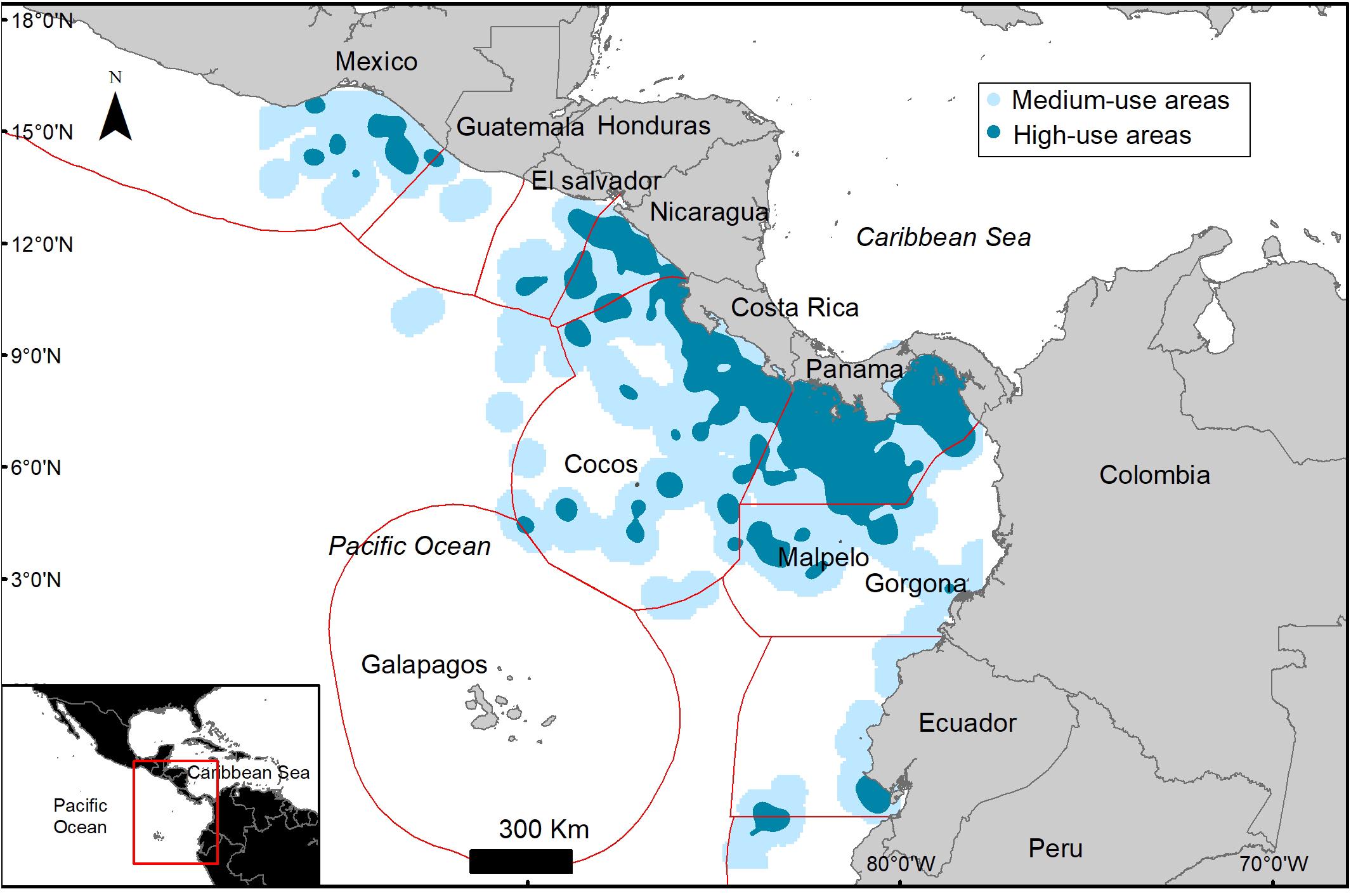
Figure 5. Kernel density of high (dark blue) and medium (light blue) use foraging areas used by Lepidochelys olivacea turtles tagged in Pacific Panama.
Turtle Tracks Overlap With Industrial Fishing Areas
Turtle tracks overlapped with the industrial fishing areas identified by Kroodsma et al. (2018) with 82.7% of turtle locations occurring within industrial fishing areas; 25% of them in areas with low fishing effort, 50% with intermediate, and 25% with high fishing effort. However, the extent of the overlap between turtles and industrial fisheries was dependent upon season (χ2, p < 0.001), with more turtle locations in areas with high fishing effort during fall (Figures 6, 7A). Purse-seine fisheries were the most common industrial fishing type in the study area during all seasons (79.4 ± 5.6%), followed by long liners (11.9 ± 1.4%), and squid jiggers (6.1 ± 4.9%), while industrial trawling represented 1.7% ± 0.1 of the fishing. Squid jiggers, however, were outside the area traveled by these turtles.
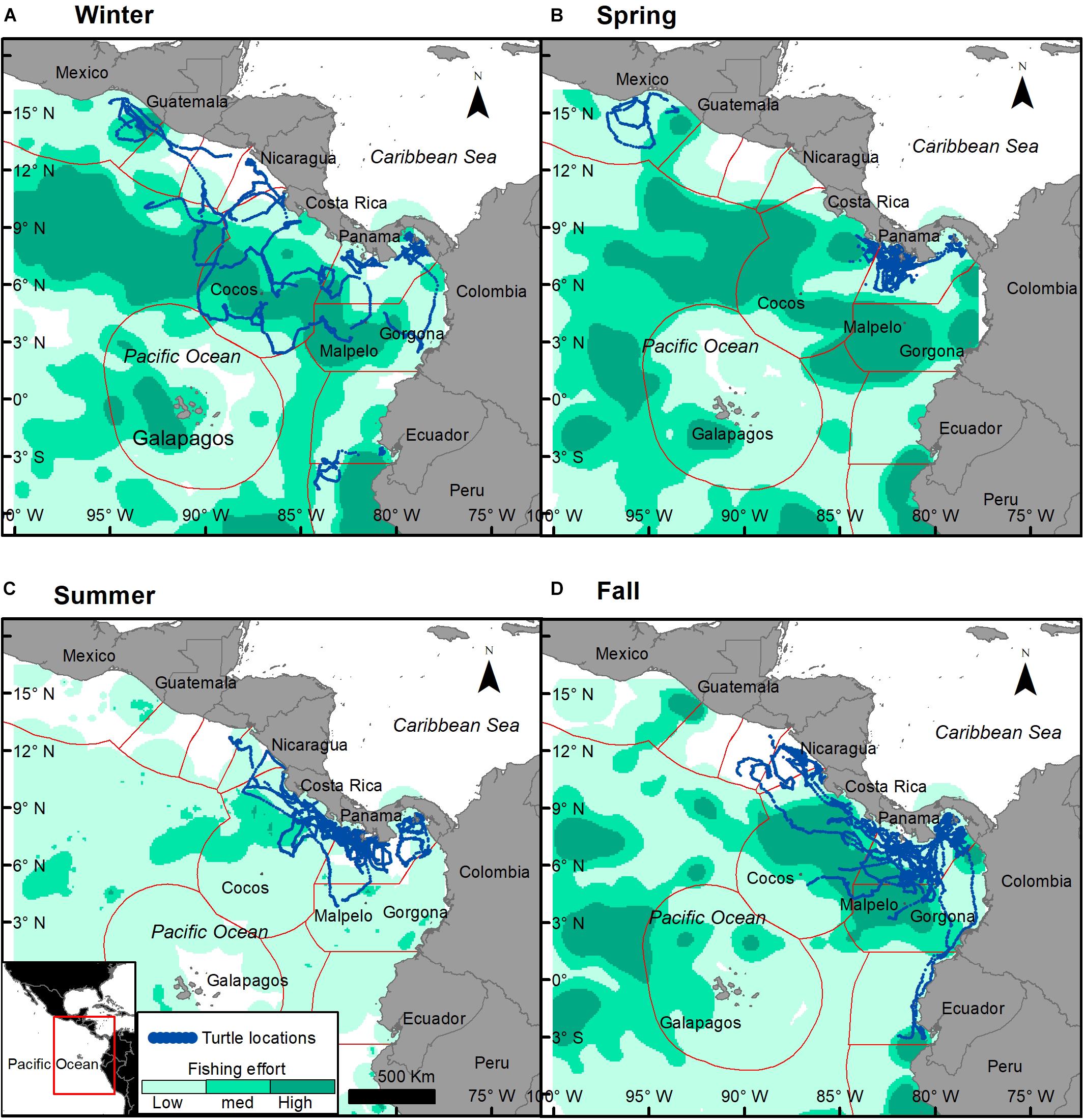
Figure 6. Turtle tracks (blue dots) overlapping industrial fishing areas during each season with low fishing effort (light green), medium (cyan), and high (dark green). Fishing effort seasonal mean was calculated based on Kroodsma et al. (2018). (A) Winter, (B) spring, (C) summer, and (D) fall.
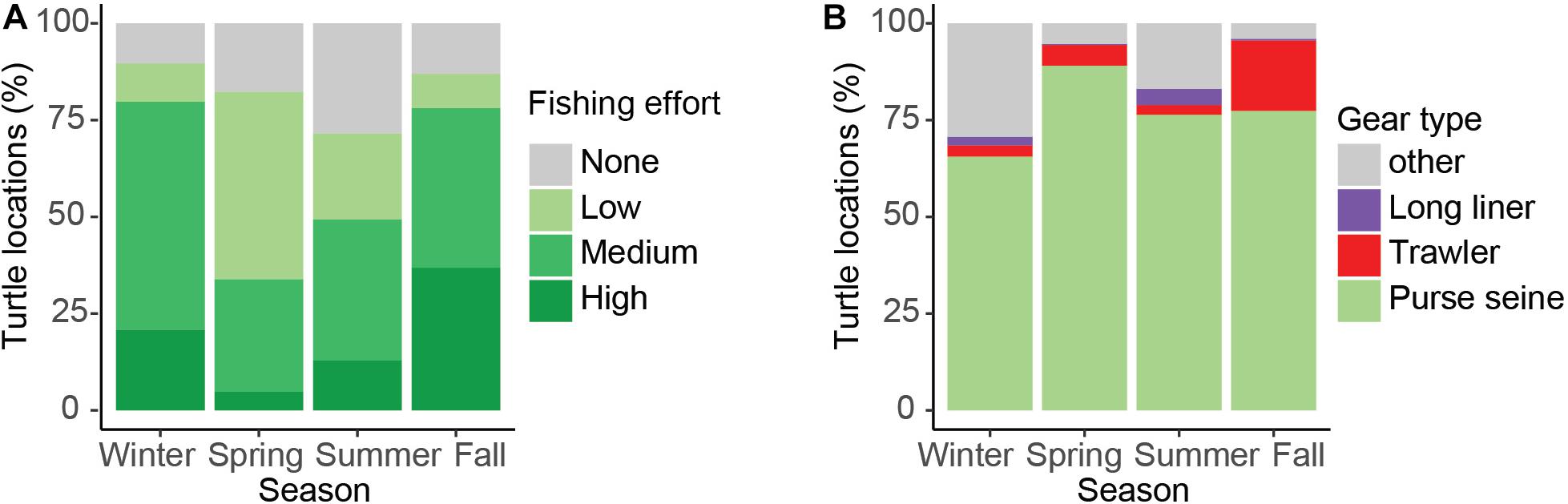
Figure 7. Percentage of turtle locations that overlapped with low, medium, and high-use industrial fishing areas (A), and the proportional overlap with different fishing gear types (B).
On average, 24.4% of turtle locations overlapped with more than one fishing gear within industrial fishing areas, varying up to 36% in summer and a minimum of 11.7% in spring. Figure 8 shows the spatial and seasonal distribution of the three most important fishing gears (excluding the jigger) and clearly indicates that purse-seine fishing covers the largest fishing area in the region. Turtle movements coincided and overlapped with purse-seiners throughout the Eastern Pacific region by an average of 77%, varying between 65 and 89% depending on the season (Figure 7B). This overlap seems more intense during the summer and fall within the Costa Rican EEZ and the southwest and northwest of Panama and Colombia, respectively (Figure 8). Trawling seems to mainly affect Costa Rica throughout the year both the coastal zone and the EEZ, but intensely during spring with a maximum overlap of 18% (Figure 8). The distribution of longline fishing does not seem to affect the region widely, with a maximum overlap of 4.2%, except in the Gulf of Tehuantepec during winter and the northwest of the Gulf of Panama during summer and winter, in addition to international waters (Figures 7B, 8). Both gulfs are affected by intense seasonal upwelling in winter, which may explain an increase in the presence of turtles and fishing vessels during this season.
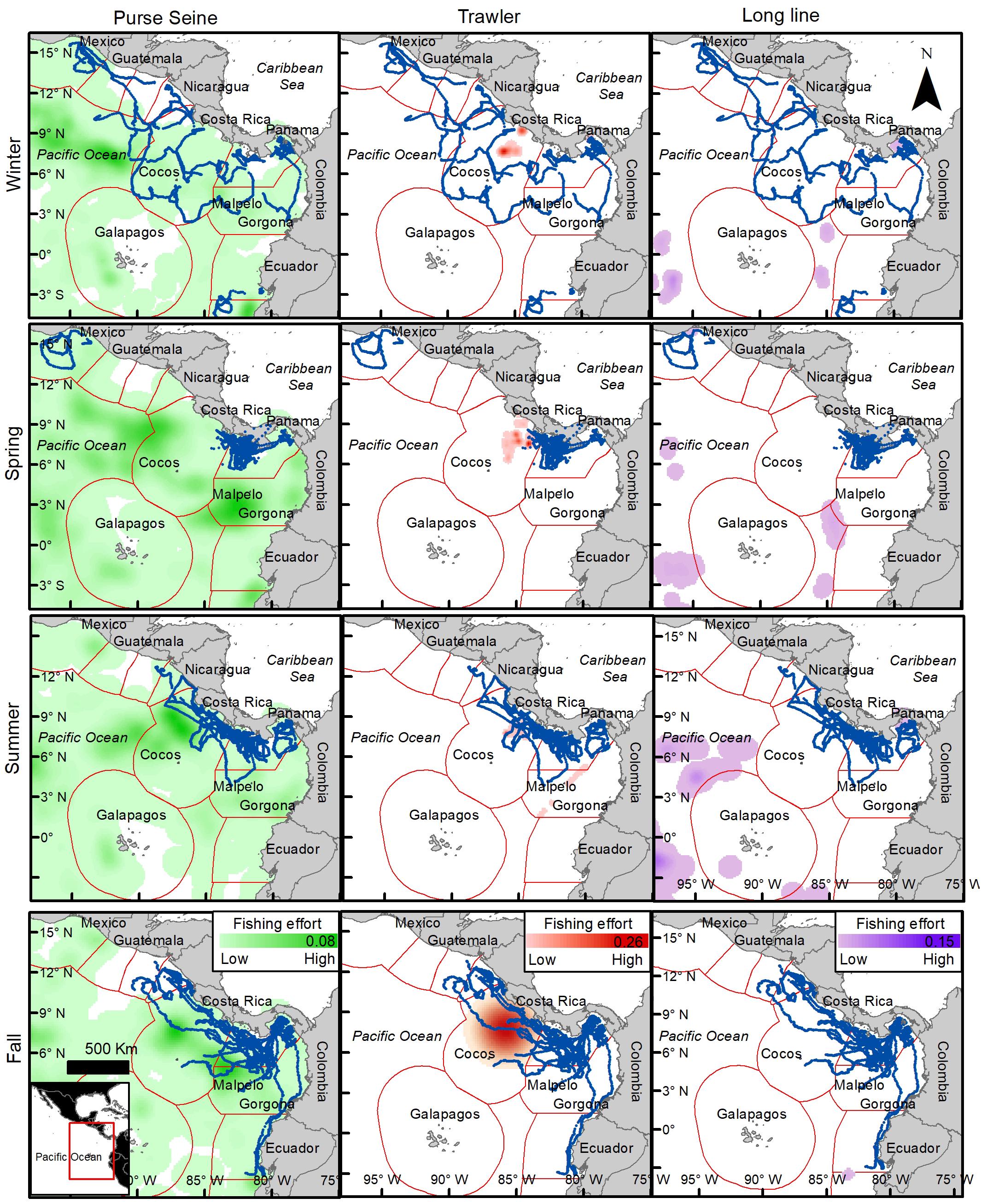
Figure 8. Turtle tracks (blue dots) overlapping industrial fishing areas divided by fishing gears (Purse-Seine, Trawler, Long liner) and seasons. Intensity the background color indicates fishing effort, maximum value indicated in scale bar (number of fishing hours per k2). Based on Kroodsma et al. (2018).
Regional Foraging Patterns
Although tagged turtles migrated in multiple directions toward North and South America and toward oceanic islands, some individuals headed toward common foraging areas under different circumstances by using alternative routes at different times and crossing over the Exclusive Economic Zones of nine countries and international waters. Common foraging areas and stop-overs, included the Gulf of Guayaquil and Pampanal de Bolivar (Ecuador), Gorgona Island (Colombia), Gulf of Fonseca (Salvador and Honduras border), and the Gulf of Tehuantepec (Mexico).
Two turtles tagged in the Las Perlas Archipelago (101504 and 97800) in October 2010 and September 2009 migrated to the foraging area at the Gulf of Guayaquil (southern Ecuador), an area known for its high Prod and high frequency of commercial fishing boats (Félix et al., 2017). Both turtles took ∼1 month (20 and 31 days, respectively) to get to Ecuador’s waters, however, they used two different routes to get there, turtle 101504 used a more oceanic route than turtle 97800, having stopovers in Gorgona Island (Colombia) and in Pampanal de Bolivar (Ecuador) (Figure 9A) In the same way, turtles 9778 (tagged on August 2010) and 46248 (tagged on May 2011) tagged at the Gulf of Chiriqui (Panama) migrated to the Gulf of Fonseca (Salvador and Honduras border) by taking different routs, at different times, but with the same destination (Figure 9B).
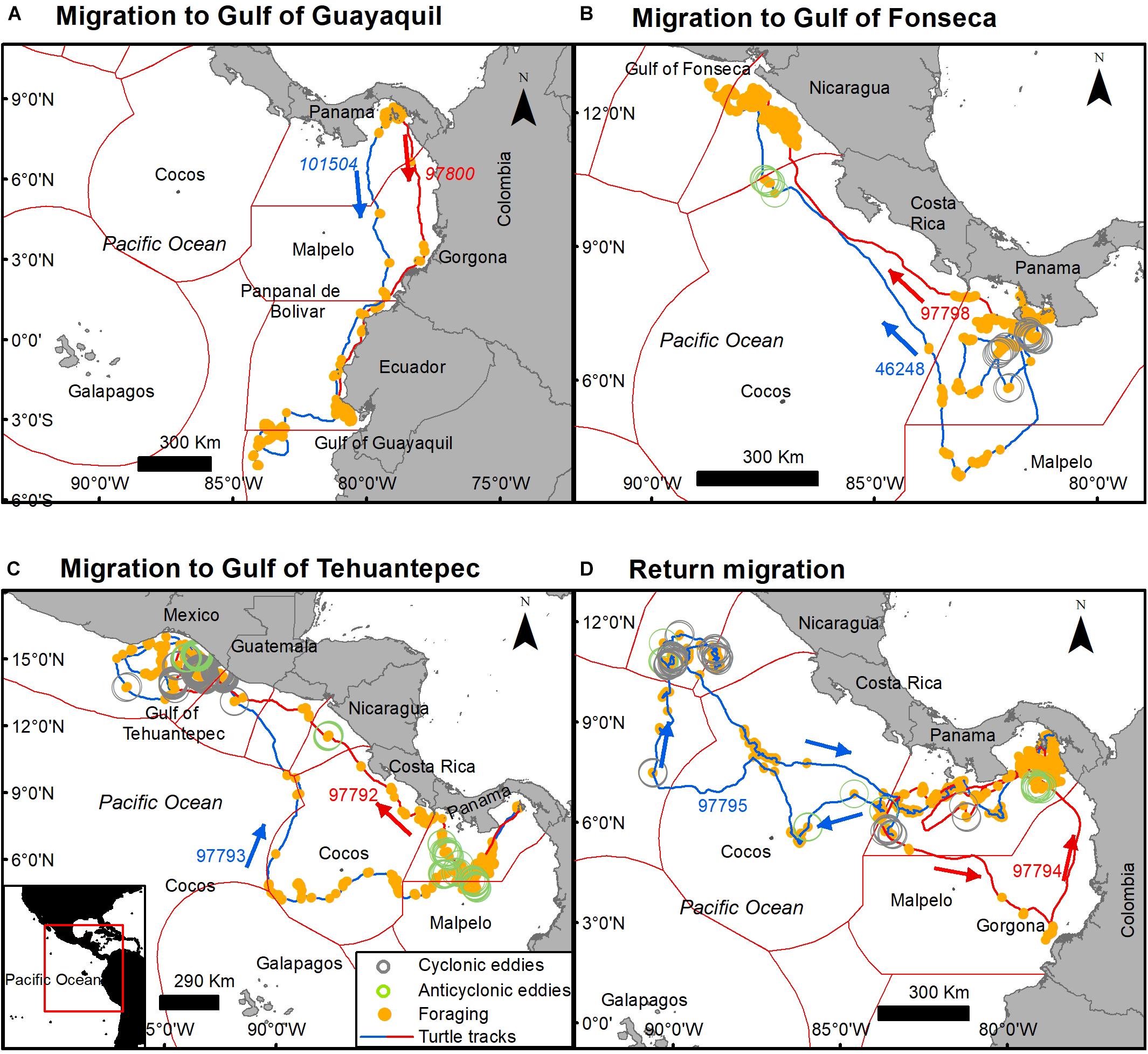
Figure 9. Common destination and foraging sites of tagged olive ridley turtles tagged off the Pacific coast of Panama. (A) Migration south to the Gulf of Guayaquil (Ecuador), (B) migration north to the Gulf of Fonseca (Honduras and El Salvador border), (C) migration north to the Gulf of Tehuantepec (México), and (D) long oceanic northern and southern migrations that returned to nesting areas. Orange dots indicate transmission in state 1 (foraging), arrows indicate the direction of the movement. Open circles indicate spatial and temporal overlap with cyclonic eddies (gray) and anticyclone eddies (green).
Two other turtles (97793 and 97792) migrated from the Las Perlas Archipelago to the Gulf of Tehuantepec (Mexico). Both of these turtles were tagged in September 2009 and had a similar southwestern migration until they encountered an anticyclonic eddy, started to forage, and their migratory routes diverged. Turtle 97792 headed northward with several foraging stops relatively close to the shore, and turtle 97793 continued its migration through oceanic waters. Both turtles arrived at the Gulf of Tehuantepec (Mexico) where their foraging overlapped with cyclonic and anti-cyclonic eddies (Figure 9C).
Two turtles had long and distinct oceanic migrations that returned to foraging grounds near their nesting area (tagging area). Both turtles were tagged simultaneously in Las Perlas Archipelago and migrated south until their routes diverged in parallel 6°N. Turtle 97795 headed northward to parallel 11°N where it foraged for 1 month in oceanic waters with high Prod and Chl concentrations and frequent eddies, and started a return until we lost the transmission. Turtle 97794 migrated south through oceanic waters and arrived to Sanquianga National Park (Colombia, south of Gorgona Island) where it foraged for 1 week, it then migrated northward back to Panama, arriving at the nesting area (tagging area) 6 months later (Figure 9D).
Discussion
Olive ridley turtles tracked in this study migrated up to 6,684 km from the Pacific coast of Panama to different destinations without a clear migratory corridor, passing over the Economic Exclusive Zones of nine countries an over international waters and using five stop-overs and common foraging locations in the Tropical Eastern Pacific. Their plastic behavior was related to local environmental conditions, which has been described for the same species in French Guiana (Chambault et al., 2016) and for other sea turtle species that do have specific migratory corridors, such as green turtles migrating along the South American northeastern coast (Baudouin et al., 2015).
We identified two modeled behavioral states (migrating and foraging) and based on the HMM, the probability of changing from one state to another one was correlated with local environmental conditions. Individual movements were driven by oceanic conditions and resource availability and not by spatially explicit migratory corridors. Turtles switched from a directional migratory movement to foraging when they encountered Chl-rich and productive waters, where they spent most of their time (70%) overlapping with industrial fishing areas. Some turtles, however, coincided in some directional movements to forage in nutrient-rich destinations: the Gulf of Guayaquil (Ecuador), the Gulf of Tehuantepec (Mexico), and the Gulf of Fonseca (Salvador and Honduras border). During their migration turtles encountered a low number of eddies (only 8% of the locations), this strong mismatched could be likely due to turtle’s behavior (oriented swimming outside these high-velocity structures) and low occurrence of coastal eddies.
Turtles tagged in this study (at the TEP) had more oceanic and disperse migrations compared to turtles tagged in French Guiana, which had a distinct coastal migratory route (Chambault et al., 2016). However, the average traveling distance (2,629 km) and the average traveling speed (0.9 km/h) found in this study lays within the ranged found in French Guiana (412–3651 km for traveling distance at a speed of 0.9–2.8 km/h) (Chambault et al., 2016). Distance traveled and traveling speed was not different between male and female turtles, however, in this study only eight out or 34 tagged turtles were males, therefore, further studies with a more symmetrical sample size should support this result. Although the turtles tracked in this study traveled through coastal and oceanic waters they stayed in waters around 28°C, comparable to turtles tracked in Australia and French Guiana (McMahon et al., 2007; Chambault et al., 2016).
Turtles that migrated south to the Gulf of Guayaquil in Ecuador and north to the Gulf of Fonseca in Costa Rica did not overlap temporally during their migration (tagged in different years) but arrived at the same foraging destination via different migratory routes. The two turtles that migrated to the Gulf of Tehuantepec in Mexico did overlap temporally but also took different migratory routes to arrive at the same destination. These results support the previously described nomadic behavior of this species. Our study further indicated that, although these turtles move freely in the oceans driven by resource availability, changing directional migratory behavior to foraging when resources were available (statistically confirmed herein) or changing direction in response to eddies, they do have a foraging destination to get to. They also have a remarkable ability to navigate back to the nesting area after long and improvised migration from foraging areas, as other migratory species do (Milner-Gulland et al., 2011).
Although migratory routes were scattered and different between tagged turtles, important foraging areas could be identified primarily in the territorial waters of Panama, Costa Rica, Nicaragua, and Mexico. Their overlap with high-Productive areas (e.g., Costa Rica Dome and Gulf of Guayaquil) commonly used by fishers is a matter of concern for sea turtle populations, which are threatened both in coastal waters, owing to habitat lost on nesting beaches, and offshore, owing to by-catch by fishing vessels. During the present study, data on artisanal fisheries or fishing boats not equipped with AIS devises where not included in the analysis due to lack of available reliable data. Montero et al. (2016) determined that the probability of incidental catch per unit effort by ETP purse-seine fisheries, which accounts for the majority of the fishing vessels in the study area, increases in temperatures (SST) of 26–30°C, with higher incidence occurring from June to December, in bycatch hotspots from North and south of the equator between 0–10°N; 0–10°S and from 120 to 140°W; and along the Colombian coast and surrounding regions. This indicates that turtles migrating off the Pacific coast of Panama, such as the ones tagged in the present study, are particularly vulnerable since they overlap temporally and spatially with these high by-catch areas and seasons.
Owing to their nomadic migrations and the lack of clearly defined migratory corridors, protection of this species should focus on good fishing practices and turtle-safe fishing gear particularly through Central America, and should be added to special management programs in foraging destinations such as the gulfs of Guayaquil (Ecuador), Fonseca (Salvador and Honduras), and Tehuantepec (Mexico) and, of course, the protection of nesting habitats. Education of how to deal with incidentally caught turtles should also be implemented, since adequate handling of lightly hooked turtles incidentally caught by long liners reduces their probability of dying upon release (Swimmer et al., 2006).
This scenario shows that bycatch of turtles in the region affects all species and the scale of the problem is very difficult to analyze in order to implement adequate management and mitigation measures. In the United States for example, data from more than a decade on multiple turtle species demonstrated that implementing management or mitigation measures can have a significant change in the impact of the fisheries, with a reduction of by-catch and mortality in 60 and 94%, respectively (Finkbeiner et al., 2011). Additionally, the authors make potential management recommendations, which include increasing the presence of observers on fishing vessels, the modifications to fishing gear, the establishment of temporary closures in critical areas, and relevant to our study, consider the different countries whose Exclusive Economic Zones overlap, so that the resource must be managed across political borders. This management plan can be designed by identifying priority areas as previously suggested (Wallace et al., 2013), and improved by using satellite monitoring of the species to obtain an enhance spatial resolution, similar to ours and other studies (Maxwell et al., 2011).
Although the nomadic nature of olive ridley turtles in the Eastern Pacific region suggests that climate change may not affect their distribution (sensu Plotkin, 2010), fishing continues to be the biggest threat. Figures 6, 7 showed the movements of olive ridley turtles within the countries of Central America and Costa Rica in particular, demonstrating the overlap with fisheries within the discrete EEZs during the first and last trimesters of the year. Indeed, the need for cross-border management can be demonstrated by an example close to Panama, where bycatch of olive ridley turtles within the Costa Rica’s EEZ was estimated in almost 700,000 individuals between 1999 and 2010, based on observer analysis on board the long-liners fleet (Dapp et al., 2013). Wallace et al. (2013), suggested that type of gear and species-specific information can be considered for reducing bycatch and to create or improve existing regional management units (RMUs). Regulating the use of a particular gear could be argued necessary to reduce fishing pressure and bycatch for this species but requires well-planned political initiatives at least in some countries within the region. Our results, suggest that Colombia, Panama and Costa Rica are of particular concern due to the high level of fishing gears overlapping coastal and oceanic waters throughout the seasons and across the entire range of olive ridley turtles. Consequently, an RMU including only those three countries can be initially considered and perhaps political feasible to manage than a larger regional one (e.g., Tropical Eastern Pacific). Proposing further conservation and management measures is beyond the scope of this study, because it requires access to country-based VMS or AIS tracking databases not included in the GFW database used here. If the suggested specific RMU can be established, a priority could be to gather or update all existing information including fishing licenses for national and international vessels, size of local industrial and industrial fleets, and more important, the access to local satellite tracking systems that have been operational for several years and are not shared with GFW. The present spatial analysis, the first for this region and for this species showing the distribution of the different fisheries within the EEZ, can be used as an initial tool for improving current management and mitigation measures (sensu Pikesley et al., 2013; Wallace et al., 2013), that can include but not be limited to the creation of protected seaways or corridors void of fisheries across the region.
Previous studies of olive ridley turtles within the ETP (Luschi et al., 2003; Polovina et al., 2004; Plotkin, 2010) have indicated an absence of common foraging areas with turtles generally distributed throughout the pelagic marine region performing wandering movements as an oceanic nomad. Whilst many of the turtles tagged within the study indeed followed this established pattern, others instead demonstrated different types of foraging behaviors with common use of a number of localized areas such as the gulfs of Tehuantepec, Fonseca and Guayaquil. Although fidelity to specific foraging areas has been observed in other oceanic regions (Whiting et al., 2007; Da Silva et al., 2011), the foraging behavior observed in the present study for olive ridleys of the ETP is novel. This may indicate a higher behavioral plasticity for this population than previously thought. As noted previously (Plotkin, 2010), such an ability to adapt to environmental changes in the marine ecosystem suggests that ETP olive ridleys may be less vulnerable to the effects of climate change than other sea turtle species in the area.
Further studies on olive ridley movements in relation to oceanographic conditions would support our results and be beneficial to furthering our understanding, especially because the behaviors described in this study were identified by a model based on coordinate locations alone, with no other behavioral data, such as diving behavior (e.g., Chambault et al., 2016). In the current study, no thermocline information was included, which can be highly dynamic in the region, and provide further clues to their foraging behavior (Hochscheid et al., 2010). Perhaps most importantly, the ability to monitor turtle movements over longer time periods to determine the time spent in certain areas and whether there exists fidelity between individuals to specific sites, both nesting and foraging. A large variety of tracking durations have been demonstrated from other studies of olive ridleys with averages ranging from 77 days (Whiting et al., 2007) to 113 days (Da Silva et al., 2011) and 236 days (Plotkin, 2010). Further research is dependent upon the technological development of the transmitters and the improvement of anchoring systems.
As the oceanic climate continues to change due to human activities, the influence it exerts on sea turtle movements is unpredictable. Thus, we strongly recommend the continual monitoring of their movements and behaviors to ensure the efficacy of current protective legislation both regionally and internationally, especially in foraging areas identified by the present study, the Gulf of Guayaquil, Pampanal de Bolivar (Ecuador), Gorgona Island (Colombia), Gulf of Fonseca (Salvador and Honduras border), and the Gulf of Tehuantepec (Mexico).
Data Availability Statement
Tracking data obtained in this study will be made available by the authors to any qualified researcher upon request.
Ethics Statement
The animal study was reviewed and approved by the Smithsonian Tropical Research Institute.
Author Contributions
HG led the research project and tagged the turtles. CG performed the statistical analyses, models, and figures. HG, CG, and GR drafted the manuscript.
Funding
This research was partially sponsored by the International Community Foundation, INGEMAR, S.A., Grupo Eleta, Conservation International, and the Smithsonian Tropical Research Institute. CG and HG were partially funded by the Sistema Nacional de Investigadores (SNI) of the Secretaria Nacional de Ciencia y Tecnología SENACYT of Panama.
Conflict of Interest
GR was employed by the company Tobii Technology AB.
The remaining authors declare that the research was conducted in the absence of any commercial or financial relationships that could be construed as a potential conflict of interest.
Acknowledgments
We thank the Government of Panama for providing the research permits. We thank Carlos Guevara, Betzi Perez, Javier Pinzon, Marietta Marroquin, and Marcos Limchin for field assistance, and Gabriela Etchelecu from Fundación MarViva and crew members for partially providing the vessel support during tagging. CG and HG were partially funded by the Sistema Nacional de Investigadores (SNI) of the Secretaría Nacional de Ciencia y Tecnología (SENACYT) of Panama. We also thank the Global Fishing Watch program for allowing access to use the database and Editage (www.editage.com) for English language editing.
Footnotes
- ^ https://catalog.data.gov/dataset/currents-geostrophic-aviso-0-25-degrees-global-1992-2012-1-day-composite
- ^ https://catalog.data.gov/dataset/multi-scale-ultra-high-resolution-mur-sst-analysis-fv04-0-global-0-011-2002-present-daily-depre
- ^ https://coastwatch.pfeg.noaa.gov/erddap/griddap/erdMH1chlamday.html
- ^ https://catalog.data.gov/dataset/primary-productivity-aqua-modis-npp-global-2003-present-experimental-1-day-composite
- ^ https://www.aviso.altimetry.fr/en/data/products/value-added-products/global-mesoscale-eddy-trajectory-product.html
- ^ https://globalfishingwatch.org
References
Baudouin, M., de Thoisy, B., Chambault, P., Berzins, R., Entraygues, M., Kelle, L., et al. (2015). Identification of key marine areas for conservation based on satellite tracking of post-nesting migrating green turtles (Chelonia mydas). Biol. Conserv. 184, 36–41. doi: 10.1016/j.biocon.2014.12.021
Bernardo, J., and Plotkin, P. T. (2007). “An evolutionary perspective on the arribada phenomenon and reproductive behavioral polymorphism of olive ridley sea turtles (Lepidochelys olivacea),” in Biology and conservation of Ridley sea turtles, ed. P. T. Plotkin, (Baltimore, MA: Johns Hopkins University Press), 59–87.
Caldwell, D. K., and Casebeer, R. S. (1964). A note on the nesting of the eastern Pacific ridley sea turtle, Lepidochelys olivacea. Herpetologica 20, 213–213.
Chambault, P., Baudena, A., Bjorndal, K. A., Santos, M. A., Bolten, A. B., and Vandeperre, F. (2019). Swirling in the ocean: immature loggerhead turtles seasonally target old anticyclonic eddies at the fringe of the North Atlantic gyre. Progr. Oceanogr. 175, 345–358. doi: 10.1016/j.pocean.2019.05.005
Chambault, P., de Thoisy, B., Heerah, K., Conchon, A., Barrioz, S., Dos Reis, V., et al. (2016). The influence of oceanographic features on the foraging behavior of the olive ridley sea turtle Lepidochelys olivacea along the Guiana coast. Progr. Oceanogr. 142, 58–71. doi: 10.1016/j.pocean.2016.01.006
Chambault, P., Giraudou, L., de Thoisy, B., Bonola, M., Kelle, L., Dos Reis, V., et al. (2017). Habitat use and diving behaviour of gravid olive ridley sea turtles under riverine conditions in French Guiana. J. Marine Syst. 165, 115–123. doi: 10.1016/j.jmarsys.2016.10.005
Cheng, I. J., and Chen, T. H. (1997). The incidental capture of 5 species of sea turtles by coastal setnet fisheries in the Eastern waters of Taiwan. Biol. Conserv. 82, 235–239. doi: 10.1016/s0006-3207(97)00027-x
Cliffton, K., Cornejo, D. O., and Felger, R. S. (1995). “Sea turtles of the Pacific coast of Mexico,” in Biology and Conservation of Sea Turtles, ed. K. A. Bjorndal, (Washington, DC: Smithsonian Institution Press), 199–209.
Cornelius, S. E. (1982). “Status of sea turtles along the Pacific coast of Middle America,” in Biology and Conservation of Sea Turtles, ed. K. A. Bjorndal, (Washington, DC: Smithsonian Institution Press), 211–219.
Coyne, M., and Godley, B. (2005). Satellite Tracking and Analysis Tool(STAT): an integrated system for archiving, analyzing and mapping animal tracking data. Mar. Ecol. Prog. Ser. 301, 1–7. doi: 10.3354/meps301001
D’Croz, L., and O’Dea, A. (2007). Variability in upwelling along the Pacific shelf of Panama and implications for the distribution of nutrients and chlorophyll. Estuar. Coast Shelf. 73, 325–340. doi: 10.1016/j.ecss.2007.01.013
Da Silva, A. C., Dos Santos, E. A., Oliveira, F. L. C., Weber, M. I., Batista, J. A., Serafini, T. Z., et al. (2011). Satellite-tracking reveals multiple foraging strategies and threats for olive ridley turtles in Brazil. Mar. Ecol. Prog. Ser. 443, 237–247. doi: 10.3354/meps09427
Dapp, D., Arauz, R., Spotila, J. R., and O’Connor, M. P. (2013). Impact of Costa Rican longline fishery on its bycatch of sharks, stingrays, bony fish and olive ridley turtles (Lepidochelys olivacea). J. Exp. Mar. Biol. Ecol. 448, 228–239. doi: 10.1016/j.jembe.2013.07.014
Eckrich, C. E., and Owens, D. W. (1995). Solitary versus arribada nesting in the olive ridley sea turtles (Lepidochelys olivacea): a test of the predator-satiation hypothesis. Herpetologica 349:354.
Eguchi, T., Gerrodette, T., Pitman, R. L., Seminoff, J. A., and Dutton, P. H. (2007). At-sea density and abundance estimates of the olive ridley turtle Lepidochelys olivacea in the eastern tropical Pacific. Endanger Species Res. 3, 191–203. doi: 10.3354/esr003191
Félix, F., Calderón, A., Vintimilla, M., and Bayas-Rea, R. A. (2017). Decreasing population trend in coastal bottlenose dolphin (Tursiops truncatus) from the Gulf of Guayaquil, Ecuador. Aquat. Conserv. 27, 856–866. doi: 10.1002/aqc.2763
Fiedler, P. C. (2002). The annual cycle and biological effects of the Costa Rica Dome. Deep. Sea. Res. 49, 321–338. doi: 10.1016/s0967-0637(01)00057-7
Fiedler, P. C., and Talley, L. D. (2006). Hydrography of the eastern tropical Pacific: a review. Prog. Oceanogr. 69, 143–180. doi: 10.1016/j.pocean.2006.03.008
Finkbeiner, E. M., Wallace, B. P., Moore, J. E., Lewison, R. L., Crowder, L. B., and Read, A. J. (2011). Cumulative estimates of sea turtle bycatch and mortality in USA fisheries between 1990 and 2007. Biol. Conserv. 144, 2719–2727. doi: 10.1016/j.biocon.2011.07.033
Gaspar, P., Georges, J. Y., Fossette, S., Lenoble, A., Ferraroli, S., and Le Maho, Y. (2006). Marine animal behaviour: neglecting ocean currents can lead us up the wrong track. Proc. R. Soc. Lond. Biol. 273, 2697–2702. doi: 10.1098/rspb.2006.3623
Godfrey, M., and Godley, B. J. (2008). Seeing past the red: flawed IUCN global listings for sea turtles. Endanger Species Res. 6, 155–159. doi: 10.3354/esr006155
Godley, B., Blumenthal, J., Broderick, A., Coyne, M., Godfrey, M., Hawkes, L., et al. (2008). Satellite tracking of sea turtles: where have we been and where do we go next. Endanger Species Res. 4, 3–22. doi: 10.3354/esr00060
Hochscheid, S., Bentivegna, F., Hamza, A., and Hays, G. (2010). When surfacers do not dive: multiple significance of extended surface times in marine turtles. J. Exp. Biol. 213, 1328–1337. doi: 10.1242/jeb.037184
Jonsen, I. (2016). Joint estimation over multiple individuals improves behavioural state inference from animal movement data. Sci. Rep.U.K. 6:2062. doi: 10.1038/srep20625
Jonsen, I. D., Flemming, J. M., and Myers, R. A. (2005). Robust state-space modeling of animal movement data. Ecology 86, 2874–2880. doi: 10.1890/04-1852
Kelez, S., Velez-Zuazo, X., Angulo, F., and Manrique, C. (2009). Olive ridley Lepidochelys olivacea nesting in Peru: the southernmost records in the Eastern Pacific. Mar. Turtle Newslett. 126, 5–9.
Kroodsma, D. A., Mayorga, J., Hochberg, T., Miller, N. A., Boerder, K., Ferretti, F., et al. (2018). Tracking the global footprint of fisheries. Science 359, 904–908. doi: 10.1126/science.aao5646
Lewison, R. L., Crowder, L. B., Read, A. J., and Freeman, S. A. (2004). Understanding impacts of fisheries bycatch on marine megafauna. Trends Ecol. Evol. 19, 598–604. doi: 10.1111/mec.13726
Luschi, P., Hays, G. C., and Papi, F. (2003). A review of long-distance movements by marine turtles, and the possible role of ocean currents. Oikos 103, 293–302. doi: 10.1034/j.1600-0706.2003.12123.x
Mansfield, K. L., Wyneken, J., Porter, W. P., and Luo, J. (2014). First satellite tracks of neonate sea turtles redefine the ‘lost years’ oceanic Niche. Proc. R. Soc. Lond. B 281, 20133039. doi: 10.1098/rspb.2013.3039
Maxwell, S. M., Breed, G. A., Nickel, B. A., Makanga-Bahouna, J., Pemo-Makaya, E., Parnell, R. J., et al. (2011). Using satellite tracking to optimize protection of long-lived marine species: olive ridley sea turtle conservation in Central Africa. PLoS One 6:e19905. doi: 10.1371/journal.pone.0019905
McMahon, C., Bradshaw, C., and Hays, G. (2007). Satellite tracking reveals unusual diving characteristics for a marine reptile the olive ridley turtle (Lepidochelys olivacea). Mar. Ecol. Prog. Ser. 329, 239–252. doi: 10.3354/meps329239
Mendelssohn, R. (2018). xtractomatic: Accessing Environmental Data from ERD’s ERDDAP Server, R package version 3.4.2. Available at: https://CRAN.R-project.org/package=xtractomatic
Michelot, T., Langrock, R., and Patterson, T. A. (2016). moveHMM: an R package for the statistical modelling of animal movement data using hidden Markov models. Methods Ecol. Evol. 7, 1308–1315. doi: 10.1111/2041-210x.12578
Milner-Gulland, E. J., Fryxell, J. M., and Sinclair, A. R. (2011). Animal Migration: A Síntesis. New York: Oxford University Press.
Montero, J. T., Martinez-Rincon, R. O., Heppell, S. S., Hall, M., and Ewal, M. (2016). Characterizing environmental and spatial variables associated with the incidental catch of olive Ridley (Lepidochelys olivacea) in the Eastern Tropical Pacific purse-seine fishery. Fish. Oceanogr. 25, 1–14. doi: 10.1111/fog.12130
Pandav, B., Choudhury, B. C., and Shanker, K. (1998). The olive ridley sea turtle (Lepidochelys olivacea) in Orissa: an urgent call for an intensive and integrated conservation programme. Curr. Sci. 75, 1323–1328.
Pikesley, S. K., Maxwell, S. M., Pendoley, K., Costa, D. P., Coyne, M. S., Formia, A., et al. (2013). On the front line: integrated habitat mapping for olive ridley sea turtles in the southeast Atlantic. Divers. Distrib. 19, 1518–1530. doi: 10.1111/ddi.12118
Pinedo, M. C., and Polacheck, T. (2004). Sea turtle by-catch in pelagic longline sets off southern Brazil. Biol. Conserv. 119, 335–339. doi: 10.1016/j.biocon.2003.11.016
Pitman, R. L. (1990). “Pelagic distribution and biology of sea turtles in the eastern tropical Pacific,” in Proceedings of the Tenth Annual Workshop on Sea Turtles Biology and Conservation US Dep Commerce (NOAA Technical Memo), NMFS-SEC-278, eds E. H. Richardson, J. A. Richardson, and M. Donnell, (Silver Spring: NOAA).
Plot, V., de Thoisy, B., and Georges, J. Y. (2015). Dispersal and dive patterns during the post-nesting migration of olive ridley turtles from French Guiana. Endanger Species Res. 26, 221–234. doi: 10.3354/esr00625
Plotkin, P. T. (2007). Biology and Conservation of Ridley Sea Turtles. Baltimore, MA: Johns Hopkins University Press.
Plotkin, P. T. (2010). Nomadic behaviour of the highly migratory olive ridley sea turtle Lepidochelys olivacea in the eastern tropical Pacific Ocean. Endanger Species Res. 13, 33–40. doi: 10.3354/esr00314
Polovina, J. J., Balazs, G. H., Howell, E. A., Parker, D. M., Seki, M. P., and Dutton, P. H. (2004). Forage and migration habitat of loggerhead (Caretta caretta) and olive ridley (Lepidochelys olivacea) sea turtles in the central North Pacific Ocean. Fish. Oceanogr. 13, 36–51. doi: 10.1046/j.1365-2419.2003.00270.x
Polovina, J., Uchida, I., Balazs, G., Howell, E. A., Parker, D., and Dutton, P. (2006). The Kuroshio extension bifurcation region: a pelagic hotspot for juvenile loggerhead sea turtles. Deep Sea Res. Part II 53, 326–339. doi: 10.1016/j.dsr2.2006.01.006
R Core Team (2018). R: A Language and Environment for Statistical Computing. Vienna: R Foundation for Statistical Computing.
Ramirez, P. A., and Ania, L. V. (2000). “Incidence of marine turtles in the Mexican long-line tuna fishery in the Gulf of Mexico,” in Proceedings of the Eighteenth Annual Workshop on Sea Turtle Biology and Conservation, NOAA Technical Memorandum. NMFS-SEFSC-436:110, (Mexico).
Rees, A. F., Al-Kiyumi, A., Broderick, A. C., Papathanasopoulou, N., and Godley, B. J. (2012). Conservation related insights into the behaviour of the olive ridley sea turtle Lepidochelys olivacea nesting in Oman. Mar. Ecol. Prog. Ser. 450, 195–205. doi: 10.3354/meps09527
Revelles, M., Isern-Fontanet, J., Cardona, L., San Félix, M., Carreras, C., and Aguilar, A. (2007). Mesoscale eddies, surface circulation and the scale of habitat selection by immature loggerhead sea turtles. J. Exp. Mar. Biol. Ecol. 347, 41–57. doi: 10.1016/j.jembe.2007.03.013
Saba, V. S., Shillinger, G. L., Swithenbank, A. M., Block, B. A., Spotila, J. R., Musick, J. A., et al. (2008). An oceanographic context for the foraging ecology of eastern Pacific leatherback turtles: consequences of ENSO. Deep. Sea. Res. 55, 646–660. doi: 10.1016/j.dsr.2008.02.006
Schick, R. S., Roberts, J. J., Eckert, S. A., Halpin, P. N., Bailey, H., Chai, F., et al. (2013). Pelagic movements of Pacific leatherback turtles (Dermochelys coriacea) highlight the role of prey and ocean currents. Mov. Ecol. 1:11.
Seminoff, J. A., Zárate, P., Coyne, M., Foley, D. G., Parker, D., Lyon, B. N., et al. (2008). Post-nesting migrations of Galápagos green turtles Chelonia mydas in relation to oceanographic conditions: integrating satellite telemetry with remotely sensed ocean data. Endanger Species Res. 4, 57–72. doi: 10.3354/esr00066
Spotila, J. R. (2004). Sea Turtles: A Complete Guide to Their Biology, Behavior, and Conservation. Baltimore, MA: Johns Hopkins University Press.
Swimmer, Y., Arauz, R., McCracken, M., McNaughton, L., Ballestero, J., Musyl, M., et al. (2006). Diving behavior and delayed mortality of olive ridley sea turtles Lepidochelys olivacea after their release from longline fishing gear. Mar. Ecol. Prog. Ser. 323, 253–261. doi: 10.3354/meps323253
Wallace, B. P., Kot, C. Y., DiMatteo, A. D., Lee, T., Crowder, L. B., and Lewison, R. L. (2013). Impacts of fisheries bycatch on marine turtle populations worldwide: toward conservation and research priorities. Ecosphere 4, 1–49.
Wallace, B. P., Lewison, R. L., McDonald, S., McDonald, R. T., Bjorkland, R. K., Kelez, S., et al. (2010). Global patterns of marine turtle bycatch. Conserv. Lett. 3, 131–142. doi: 10.1073/pnas.1318960111
Keywords: behavioral ecology, Lepidochelys olivacea, Hidden Markov Model, migration, Tropical Eastern Pacific, satellite tagging
Citation: Guzman HM, Rogers G and Gomez CG (2019) Behavioral States Related to Environmental Conditions and Fisheries During Olive Ridley Turtle Migration From Pacific Panama. Front. Mar. Sci. 6:770. doi: 10.3389/fmars.2019.00770
Received: 30 July 2019; Accepted: 28 November 2019;
Published: 11 December 2019.
Edited by:
Rob Harcourt, Macquarie University, AustraliaReviewed by:
Philippine Chambault, Institut Français de Recherche pour l’Exploitation de la Mer (IFREMER), FranceDonna Jill Shaver, National Park Service, United States
Copyright © 2019 Guzman, Rogers and Gomez. This is an open-access article distributed under the terms of the Creative Commons Attribution License (CC BY). The use, distribution or reproduction in other forums is permitted, provided the original author(s) and the copyright owner(s) are credited and that the original publication in this journal is cited, in accordance with accepted academic practice. No use, distribution or reproduction is permitted which does not comply with these terms.
*Correspondence: Catalina G. Gomez, Y2F0YWxpbmFnb21lei51cEBnbWFpbC5jb20=