- 1Department of Maritime and Transport Technology, Delft University of Technology, Delft, Netherlands
- 2Development Centre for Ship Technology and Transport Systems, Duisburg, Germany
The design and development of an offshore port terminal is a complex task that involves distinctive design and decision challenges. In this paper, we propose the implementation of a floating, modular, platform that can act as an additional terminal of a port, with the aim of expanding its current container handling capacity. To this end, we introduce a generic methodology to tackle three aspects of an offshore terminal: terminal layout design, strategic logistics optimization, and operational process coordination. The terminal layout design includes the modular arrangements, handling on and between platform modules by the associated equipment. To select the final layout design concept, we evaluate different alternatives on criteria such as layout complexity, scalability, and the number of moves associated with the modular nature of the platform. Subsequently, the selected concept is given as input to a strategic logistics optimization approach that introduces a mixed-integer linear programming model. The proposed model minimizes the capital, operational, and maintenance costs of the floating modular terminal, i.e., number and size of modules, number and type of equipment, as well as capacities. In parallel, we develop a simulation of the floating terminal’s hinterland connections, where the number and type of required vessels are specified for relevant destinations and transport configurations. At the operational level, we focus on the coordination of handling equipment on the offshore platform by employing a tailored simulation/optimization approach. Our methodology is demonstrated on a case study that considers accommodating the growth of a port in the Hamburg-Le Havre range via the use of a modular, floating, transport, and logistics hub.
Introduction
Global trade is increasing continuously in dimensions like tonnage, number of containers, number and size of vessels, and port size (UNCTAD, 2018). Being one of the major arteries for global trade, contemporary seaports constitute essential transport and logistics hubs, which constantly explore viable solutions to enhance their container handling capacity in order to cope with anticipated growth scenarios. A straightforward way to achieve this is by extending the land allocated to the port area. However, this is not always a feasible approach, especially in the case that the land available onshore is completely occupied by existing facilities and infrastructure (Lamas-Pardo et al., 2015). To overcome the scarcity of land, a promising idea is to extend the port area toward the sea through the construction of offshore, floating, platforms that may act as additional container terminals (Gharehgozli et al., 2019). In principle, all logistics operations of an onshore terminal may also take place on an offshore one. Nevertheless, there are additional design and decision challenges for the efficient implementation of an offshore, transport, and logistics hub.
Typically, contemporary seaports include a number of onshore container terminals. Strategic planning of such terminals spans years to decades, while occurring operations require intricate decisions on timescales as small as seconds. Container terminals are considered as complex systems, in which transport, handling, and storage entities interact with each other, in a setting that includes cargo flows to, from, and in between terminals. This results in a multitude of highly interrelated decision problems that need to be tackled within a port terminal environment, satisfying two important requirements: efficient execution of terminal activities and optimal use of each available piece of equipment, with the aim of minimizing different types of transport costs. In general, the involved decision problems are divided into three categories (Günther and Kim, 2006): terminal layout design, operative planning, and real-time control.
Terminal layout design is a core strategic decision that affects all the other decisions taken by container terminal operators and port authorities (Günther and Kim, 2006). Up-to-now, this challenge has been tackled by traditional approaches, which suggest the construction of terminals with common rectangular layouts, typically situated onshore. Also, terminal operators have already started to consider the design of innovative layout designs, mainly motivated by the scarcity of land (Lamas-Pardo et al., 2015). Such novel design concepts have already been proposed also for offshore container terminals (Ali, 2015). For their application, strategic optimization approaches have been used to evaluate such design layouts, from both a technical and an economic point of view (Baird and Rother, 2013; Maletić et al., 2018). The goal of such approaches is to specify the storage capacity and the suitable equipment in order to minimize capital and operational costs. However, to the best of the authors’ knowledge, none of the already proposed approaches considers the design of an offshore terminal based on a floating platform that consists of multiple modules (i.e., interconnected floaters). Therefore, there exists no study focused on the potential of such a modular transport and logistics hub that expands in capacity and size over time to accommodate growth scenarios (Flikkema and Waals, 2019).
Operative planning is concerned with planning and scheduling decisions for logistics challenges such as berth allocation (Wawrzyniak et al., 2020), crane assignment (Wang et al., 2018) and stowage planning (Bilican et al., 2020). The involved decisions are typically taken within a short-term planning horizon, e.g., within several days or weeks. As container terminals involve highly dynamic and stochastic logistics operations, operative planning approaches (Günther and Kim, 2006) are sometimes not suitable. This is because it is exceedingly difficult to predetermine how stochastic and dynamic logistics operations will be accomplished, days or weeks prior to their execution. Hence, instead of employing operative planning strategies, such operations are planned within even shorter time frames, e.g., within several seconds or minutes, employing real-time control approaches (also called real-time operational process coordination strategies). In a container terminal environment, real-time control approaches are used for a variety of logistics problems such as dispatching jobs to the transport equipment (Cheng et al., 2005; Xin et al., 2014) scheduling, and routing of vehicles that transfer containers from the berth to the storage yard (Qiu et al., 2002) as well as for the generation of schedules of the employed QCs (Abou Kasm and Diabat, 2020) and yard cranes (Galle et al., 2018).
Many research studies have addressed the high complexity of container logistics problems via the use of simulation (Iannone et al., 2016). To evaluate the efficiency of terminal operations, simulation approaches consider different performance indicators to assess the performance of the handling and transport equipment (Dulebenets, 2016; A new simulation model for a comprehensive evaluation of yard truck deployment strategies at marine container terminals, 2016). Hence, simulation is deemed a beneficial tool, not only for the detailed scheduling of crane equipment (He et al., 2015), but also, for the analysis of other operations (Rahimikelarijani et al., 2018). Such analyses can also be performed in real-time, typically applying discrete event simulators (Petering, 2010). Complementary to simulation, optimization methods are used to minimize related container transport costs. In practice, (meta-)heuristic optimization approaches are used to tackle the inherent problems, offering (sub)optimal solutions in low execution times (Sammarra et al., 2007) (Bierwirth and Meisel, 2009). To reap the benefits of both simulation and optimization, their integration into collaborative frameworks has also been proposed to tackle various container terminal problems (Zeng and Yang, 2009).
Even though there is a plethora of research studies devoted to logistics operations that take place on land-based terminals, limited attention has been paid to the challenges that arise using offshore solutions. Existing offshore solutions mainly consider offshore handling equipment (e.g., floating QCs) to reduce terminal congestion (Jordan et al., 2007) or handle a fraction of the import containers, under normal or disruptive conditions (Dulebenets et al., 2015). Additionally, some studies present preliminary investigations based on simplistic design concepts, which do not consider the peculiarities of the considered location (Ashar, 2013). To the best of the authors’ knowledge, there exists no research work that presents both a generic methodology to address essential design and decision problems of a floating, modular, offshore terminal and a case study that provides results of the proposed methods, taking into account the detailed characteristics of a particular area.
In general, offshore terminal design and decision challenges originate by the fact that the offshore case introduces original characteristics that can be grouped into two main categories: physical and logistics. Physical characteristics refer to the fact that various processes on the offshore platform are required to respect specific limitations of its structure and modularity. This category also includes the environmental conditions that prevail in the open sea: wind is more intense offshore than onshore (van den Bos, 2011) and the existence of waves affect significantly the movement of an offshore structure such as a floating platform. Logistics characteristics refer to the existence of an additional offshore node that complicates significantly the coordination of the logistics processes in the surrounding area that is extended from the port area to the hinterland.
Motivated by these unprecedented characteristics, the goal of this study is to present a generic methodology with the aim of addressing three key design and decision aspects of a floating, modular, offshore terminal: terminal layout design, strategic logistics optimization, and operational process coordination. Terminal layout design focuses on the design of the terminal by evaluating and comparing various design alternatives, particularly adapted to the unique features of the offshore case. Given a number of evaluation criteria, the best design concept is determined and given as input to the strategic logistics optimization and the operational process coordination. The objective of the strategic logistics optimization approach lies in determining the capacity of the available equipment in order to minimize capital and operating costs. Finally, the operational process coordination involves the development and evaluation of a suitable simulation/optimization approach to address one of the most important offshore logistics operations: the scheduling of the QC situated offshore. The proposed methodology is demonstrated by presenting results of a specific case to accommodate the growth of a port in the Hamburg Le Havre range via the use of a modular, floating, transport, and logistics hub.
Methodology
The framework for the design of floating terminals tackles the challenges on three levels: strategic, tactical, and operational. These three perspectives are illustrated in Figure 1. Floating terminal layout design on the highest level delivers a design concept that is used as input for strategic logistics optimization; operational process coordination is studied using the same design concept as well as the terminal configuration resulting from the optimization. The specific challenges that need to be addressed for a floating platform are the strict modularity of the design, the special climate conditions offshore that has an impact on both the technical systems as on the logistics planning and operation and the remote location of the platform impacting the connections with other, land-based terminals and hinterland connections.
Floating Terminal Layout Design
This section presents an approach for designing a transshipment and storage concept for regular port services on an offshore, modular floating platform. The proposed approach includes several steps. In the first step, existing floating terminal concepts and land-based solutions for different cargo types are identified. In the second step, the storage module design concept is introduced and the functions necessary for regular port services are defined. Key characteristics for the modular concept are the connectors that keep individual modules together and at the same time allow for sufficient degrees of freedom. In addition, a spacing between the modules is foreseen to respond to movements generated by wave and weather conditions. In the design of storage and handling concepts on the platform these spacings have to be traversed. In the third step, several different design concepts are generated, based on equipment types and possible storage configurations. In the fourth step, the generated handling and storage concepts are evaluated based on a set of criteria, and a single concept is chosen. This selected concept is used as a basis for the strategic logistics optimization and the operational process coordination of the floating platform.
The concept evaluation of storage and the container handling on the platform is further specified here. The defined criteria for the storage concept evaluation include physical characteristics such as the number of different types of modules used within the platform, the number and different types of equipment, the loss of storage space due to the required equipment and the handling performance of each concept. Typically, the storage loss is expressed by Eq. 1. The performance is expressed by the number of moves required to move one container in the overall platform configuration, i.e., from one corner of the platform to the other corner of the platform.
Each concept will be also assessed using criteria related to the handling on and between the modules. Since there is a considerable gap between the modules to allow for individual module motions, specific handling concepts to move containers from module to module had to be designed. The used criteria include layout complexity, the storage efficiency (i.e., the ratio between space required for the equipment and storage space left for containers), the productivity (i.e., how fast or easy the containers can be moved across the platform), and the scalability (i.e., how easily the platform can be modified to fit future growth demands or new layout configurations). To review the different design concepts, a review score table can be used. Considering the set of criteria, a score is applied for each generated concept. Finally, the concept design with the best score is selected and it is given as input to the Strategic logistics optimization and the Operational Process Coordination Strategy (Figure 1).
Strategic Logistics Optimization
In this section, we present a generic strategic logistics optimization methodology that can in principle be applied to any offshore island that serves as an additional terminal of a port. The presented methodology is based on the assumption of increasing global trade, thus a growing demand for future terminal concepts. Such novel terminals are meant to meet expectations of larger vessels and progressing number of traded goods. After having defined the floating terminal’s layout design and the throughput scenarios, a logistics optimization is performed for static as well as for time-dependent scenarios, assuming yearly growths rates. This way, terminal concepts and hinterland connections can be evaluated for their performance in a variety of boundary conditions and demands.
Based on these fundamental boundary conditions, the optimization of terminal design and hinterland logistics is performed. Both consider capital as well as operational expenses. Results stemming from the terminal design optimization such as the number of served vessels at hinterland quay side are approached by the hinterland logistics concept.
Container Terminal Optimization
The objective of the developed routine is to establish a strategic level terminal design concept for regular port services on a floating platform, under the assumption that the platform consists of individual modules. The generic approach for the terminal design consists of three steps:
(1) Creation of a database of all potential equipment that is considered for use in an automated floating terminal.
(2) Literature survey and partner input covering equipment logistics (capital and operational expenses).
(3) Development of an optimization model aiming a minimized demand for terminal logistics operations.
In practice, steps 1 and 2 result in defining an aggregation of all the equipment under consideration for the floating terminal, along with their performance, as well as data related to their costs. As for step 3, next, we provide a detailed presentation of a conceptual and mathematical model that optimizes the configuration of the offshore modular platform.
The optimization approach is formulated as a mixed-integer linear programming (MILP) problem that minimizes terminal logistics on a normalized annual basis. The cost function is minimized respecting certain system constraints. The output of the model is the optimal terminal configuration with specific installed capacities for the chosen equipment in terms of overall costs.
Variables
Room for optimization is given by varying the following magnitudes.
nij Type and number of equipment i used at terminal function j.
mkj Type and number of module k used at terminal function j.
xij Utilization of equipment i used at terminal function j.
ykj Utilization of module k used at terminal function j.
Parameters
The list of parameters below sets the models database and is the foundation of calculated absolute costs.
CT Container throughput per scenario [TEU].
ECi Equipment capacity for equipment i [TEU/y].
ENCij Equipment nominal capacity for equipment i [TEU/y].
cte Container transfer efficiency based on environmental conditions [–].
OPH Operational hours of the terminal [h].
.
SCkj Storage capacity of modules [TEU].
bli Berth length [m].
CCi Capital costs of equipment i [€/y].
CRFi Capital recovery factor of equipment i [–].
CAPi Capital costs of equipment i [€].
r Interest rate of equipment [–]
LTi Lifetime of equipment i [y].
OCi Operational costs of equipment i [€/TEU].
ep Industrial electricity price for the considered country [€/kWh].
elci Electricity consumption of equipment i [kWh/TEU].
sf Storage factor (1 day storage, 3 days storage) [–].
Objective
The optimization performed by the model is a strategic terminal logistics optimization. The objective function follows this literal definition:
Minimize the total capital costs incurred as a result of selected equipment needed:
To render this model applicable to each particular situation, additional constraints that respect the assumed demand scenarios need to be included. Examples of such constraints consider the demand of equipment capacity, demand of storage capacity and also respect the quantity of the available handling equipment (e.g., cranes), and the particular formation of the platform (e.g., the type and the number of the used modules).
Hinterland Logistics Optimization
In this section, we present a hinterland logistics optimization methodology with the aim of identifying, analyzing and optimizing suitable hinterland transport chains on sea and inland waterways from and to the considered offshore platform considering options of SSS, RSS, and IWS. To this end, the transport costs and duration of the considered round trips are computed and compared for different transport chains on a strategic level. This is conducted by developing and applying a simulation model for ship operations, which takes as input specific demand scenarios and relevant hinterland connections.
In general, the proposed hinterland logistics optimization methodology considers three steps:
(1) Analysis of an origin/destination matrix to identify relevant hinterland connections for the proposed floating port location.
(2) Definition of a cost model that includes capital and operational costs for different types of vessels and handling at the hinterland.
(3) Development of a simulation model that includes the cost model and the origin destination matrix as a data base to assess transport chains by costs and number of vessels that realize a scenario and the corresponding cargo flow between the offshore platform and a cargo source or drain in the hinterland.
Overall approach
The proposed approach considers the relevant hinterland connections and existing container cargo flows between a port and the hinterland along with the assumed demand scenarios (Assbrock et al., 2020). To render this approach as general as possible, typical ways of shipping are combined to serve the hinterland transport. For example, SSS and IWS are entrenched. Many vessels exist to serve this transport chains and connect an offshore platform to seaports or to destinations in the hinterland. Also, we opt to addresses less established vessel types that can be employed for the entire connection, as long as they combine the small draft and height with structural strength and propulsion power to cope with both environments, namely the sea and inland waterway conditions. These Sea-Going Inland vessels are also referred to as coasters or estuary vessels. Establishing such vessels for the connection of the offshore platform to cargo sources and drains in the hinterland is considered a promising strategy that saves additional handling at stopovers.
A hinterland connection defines the distances of transport to be completed by the vessels, the portion of TEU per 1 day dwell time by the relevant scenario and the ports for stopover. A scenario provides the required boundary conditions like the amount of TEU that are handled on the offshore hub within a month or a year. Feasible solutions for a hinterland connection under the given circumstances of the dominating scenario are evaluated comparing durations of round trips and costs for vessels in operations and at the terminals. For handling at the offshore hub, input from the terminal optimization, presented in Section “Container Terminal Optimization,” is incorporated.
Relevant hinterland connections
The offshore platform acts as an additional terminal of an arbitrary port on the one hand and on the other as an independent hub with SSS connection to seaports but additional direct connection to cargo sources and drains in the hinterland with entrenched vessels for RSS. Figure 2 illustrates the concept of two connections and gives an example. The connection concepts may define suitable modal splits, e.g., 38% direct hinterland shipping at the port for hinterland connections. These splits are to be projected on the offshore hub to define the appropriate types of logistics principles.
In general, individual hinterland connections have the focus of the proposed strategic analysis. Considering the cargo flow distributions on waterborne container transport of a port the assumed demand scenarios are transferred in container portions for individual cargo sources and drains in the hinterland. Additionally, each connection is structured in at least two segments, the sea and the inland waterway stretch. This is necessary since not all considered vessels are capable to complete the full round trip. All calculations can cover a full round trip between the offshore hub and the hinterland connection. The influence of different velocities over ground in up- and downstream conditions on rivers can be considered.
Concept and cost model
Hinterland connections of the offshore hub can be realized twofold. The concept in focus evaluates the beneficial effect of a direct hinterland connection with established vessels for RSS. The non-stop solution is compared with the connection including one transshipment in a seaport. With the developed model also SSS connections can be addressed. Comparison of solutions for relevant connections is done calculating the duration of round trips between the hub and terminals in the hinterland. With a dedicated cost model that includes capital, maintenance, personnel and fuel costs for each vessel type the most beneficial solution is computed. The applied cost model is based on the following assumptions:
The capital costs consist of a vessel’s lightweight approximation that defines the costs for the hull and fixed rate for machinery and equipment, which is specific for the type of vessel and installed power. For the investment a fixed lending term of 20 years and a rate of interest of 1.5% are assumed. With 2 and 5% of the total investment costs per year, expenditures for maintenance and insurance are covered.
The personnel costs depend on the crew size and assumed salaries for personnel being skilled for either navigation on inland water ways or sea ways. Capital costs and personnel costs are calculated for every operational hour. On a round trip, two different operational modes are distinguished and costs scale only with the corresponding mode. The modes are:
(1) Processing at terminal, which includes hours of vessel coordination in the port, and the loading and unloading at the terminal,
(2) Vessel in operation on a short-sea segment or on an inland waterway segment with consideration of heading up- or downstream.
Fuel costs are only applied for the operational mode under way. Depending on the vessels heading on an inland waterway segment the necessary shaft power is related to the assumed velocity the vessels sails up- or downstream. In practice, a margin of 15% is assumed and applied on the total costs per vessel on a round trip. Considering the individual costs, the resulting costs per move for loading or unloading of one container depending on the assumed demand scenario are computed.
Operational Process Coordination Strategy
In this section, we investigate logistics challenges on an offshore platform that require the efficient coordination of the inherent processes at the operational level. Specifically, we tackle the problem of scheduling STSs (also called QCs) that transfer containers from a vessel to a platform and vice versa.
One of the most important challenges in a typical onshore terminal is to minimize the time required for loading and unloading a vessel, which relates to the problem of scheduling QCs (Kim and Park, 2004; Bierwirth and Meisel, 2009). In the offshore platform case, different operational steps are required to solve the problem because each crane is typically situated on a different module of the platform, hence limitations are imposed on the per crane operation range. Motivated by this, we provide an optimization method that offers high-quality solutions at low computational times. The developed method is a beneficial decision-making tool, which can be used in practice by port operators to solve related problems in real-time. The studied Floating-platform Quay Crane Scheduling Problem (FQCSP) is a modification of the problem introduced in Monaco and Sammarra (2011), concerning to incorporate a spatial constraint into the existing model. The inserted spatial constraint ensures that each crane is situated on a different module of the offshore platform, thereby limiting its access to a specific group of bays.
Optimization Approach
To solve the considered deterministic FQCSP, an optimization method, called Iterated Local Search (ILS) (Lourenço et al., 2019), is developed. The employed algorithm belongs to the class of metaheuristic optimization approaches, which offer high quality solutions at low computational times, hence satisfying the essential project requirement to tackle the logistics platform/port coordination problems in real time. The algorithm includes four main components, defined prior to its execution. These are a method that generates an initial solution, a perturbation mechanism, a local search heuristic and an acceptance criterion. The solution generation method creates an initial solution s0 either randomly or by employing a problem-specific heuristic technique. Perturbation is applied to the current solution s leading to a modified solution s′. Then, the local search heuristic comes into play and generates a local optimum s′′ based on s′. Finally, the acceptance criterion determines which solution will be given as input to the perturbation mechanism in the next cycle of the ILS. The algorithm is executed repeatedly until a predefined termination criterion is satisfied. Below, we will address each one of the main components of the ILS algorithm in more detail.
Initial solution
For the sake of simplicity, let us assume that the task and the bay are identified by the same integer number, e.g., task 1 corresponds to bay 1 and vice versa. To generate the initial solution, the first step involves assigning to each crane the tasks that cannot be allocated to any other available crane due to the constraints imposed by the per crane operational ranges. Next, the remaining tasks, which can be assigned to more than one crane, are distributed uniformly at random to the corresponding cranes.
Perturbation mechanism
Typically, the design of this mechanism is not a straightforward task as the perturbation must guide the search away from the current basin of attraction, but not too far leading to a random restart. For the considered problem, the mechanism is developed on the swap of several tasks between each crane and its adjacent ones. The number of tasks swapped between two cranes, called perturbation step and denoted by pst, plays a significant role in the success of the method. The output of the perturbation mechanism is ignored in case it is an infeasible solution with respect to the constraints of the problem at hand.
Local search heuristics
For the considered problem, two local search heuristics are developed. The first one, called shift heuristic, works on the redistribution of tasks between adjacent cranes. The second one, named swap heuristic, interchanges tasks between cranes located in neighboring bays. Assuming an assignment of tasks per crane and a unidirectional schedule the shift heuristic reassigns each task of a crane to the cranes located in upper and lower bays of the current one, respectively. In the case that any of these cranes is not present, the task is shifted to the existing one. As for the swap heuristic, each task of a crane is inserted to the crane located to an upper day and each task belonging to the latter crane is assigned to the former crane.
Acceptance criterion
The acceptance criterion determines which solution will be forwarded to the perturbation mechanism next time. Two alternative solutions are compared at each ILS cycle: the local optimum s′′ generated by the local search procedure in the current cycle of the algorithm and the local optimum s produced in the previous cycle. Between these, the solution with the higher quality with respect to the value of the objective function is selected as the dominant one.
Simulation/Optimization Approach
We also solve a stochastic version of the FQCSP, assuming stochastic crane productivity rates, due to the presence of offshore wind. To tackle this problem, we combine the ILS algorithm with a Monte Carlo Sampling (MCS) method, hence forming a simulation/optimization framework. The MCS is utilized within the proposed framework to compute the value of the expected makespan (i.e., the time when the last executed task is finished), which is the considered objective function for the stochastic FQCSP. Specifically, the local optimal solution generated by each local search heuristic of the ILS is given as input to the MCS, which is executed until a predetermined stopping criterion is satisfied. This criterion constraints the number of simulations required, considering a desired level of precision. The proposed simulation/optimization framework is applied under the assumption that there is a relation between high-quality solutions of the deterministic and the stochastic version of the considered problem.
Results
Section “Methodology” presented the approaches and methods used to address each respective design challenge for the proposed offshore transport and logistics hub. In this section, the respective results of each discussed approach are presented: the considered throughput scenarios, the chosen concept design, the strategic logistics optimization and finally, the operational process coordination. To this end, a case study is conducted, assuming that the offshore platform is used as an extension of the Port of Antwerp. Therefore, our analysis is performed considering the characteristics of the particular port.
Throughput Scenario
The statistics of the Port of Antwerp provide data for the last 37 years. The mean rate of growth over that period aggregates to 7.72% p.a. This is in line with a prognosis by HWWI (HWWI, 2006) from 2006 that stated 7.9% p.a. However, this rate seemed to be aged, since it was published just before the bank crisis in 2008–2009. Between 2016 and 2017, the number of handled containers in the Port of Antwerp increased by 4.3%. Averaging the last 10 years an annual rate of growth of 4.3% percent seems reasonable. It is proposed to follow the scenario “high” as indicated in Table 1. Corresponding growth rates are visualized for the next 12 years until 2030 in Figure 3. A Gauss distribution with a small standard deviation was applied for that forecast. Table 2 lists the container handlings for the upcoming years based on a growth rate of 4.3% per annum until 2025 and 2.2% from 2025 to 2030. In summary, the forecast for the Port of Antwerp results in about 16.4 Mio. TEU in 2030, which is an absolute increase of about 6.0 Mio. TEU compared to 2017. This prognosis represents one of the scenarios the terminal and hinterland logistics are designed for, for the specific case under consideration in this paper.
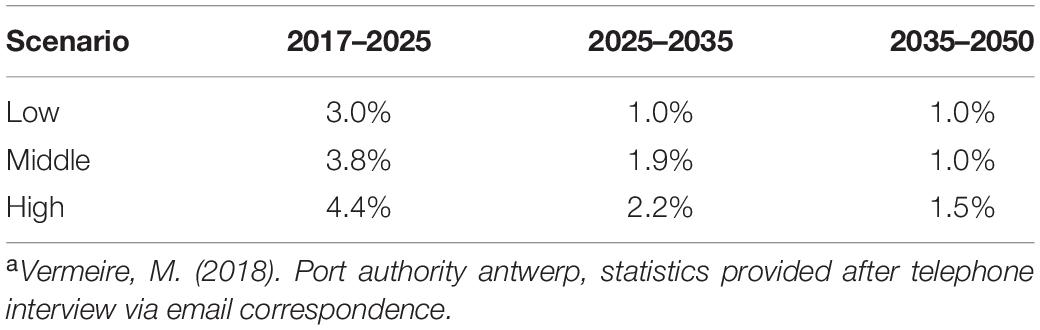
Table 1. Assumed annual growth rates for handled containers for 3 scenarios (low, middle, and high growths)a.
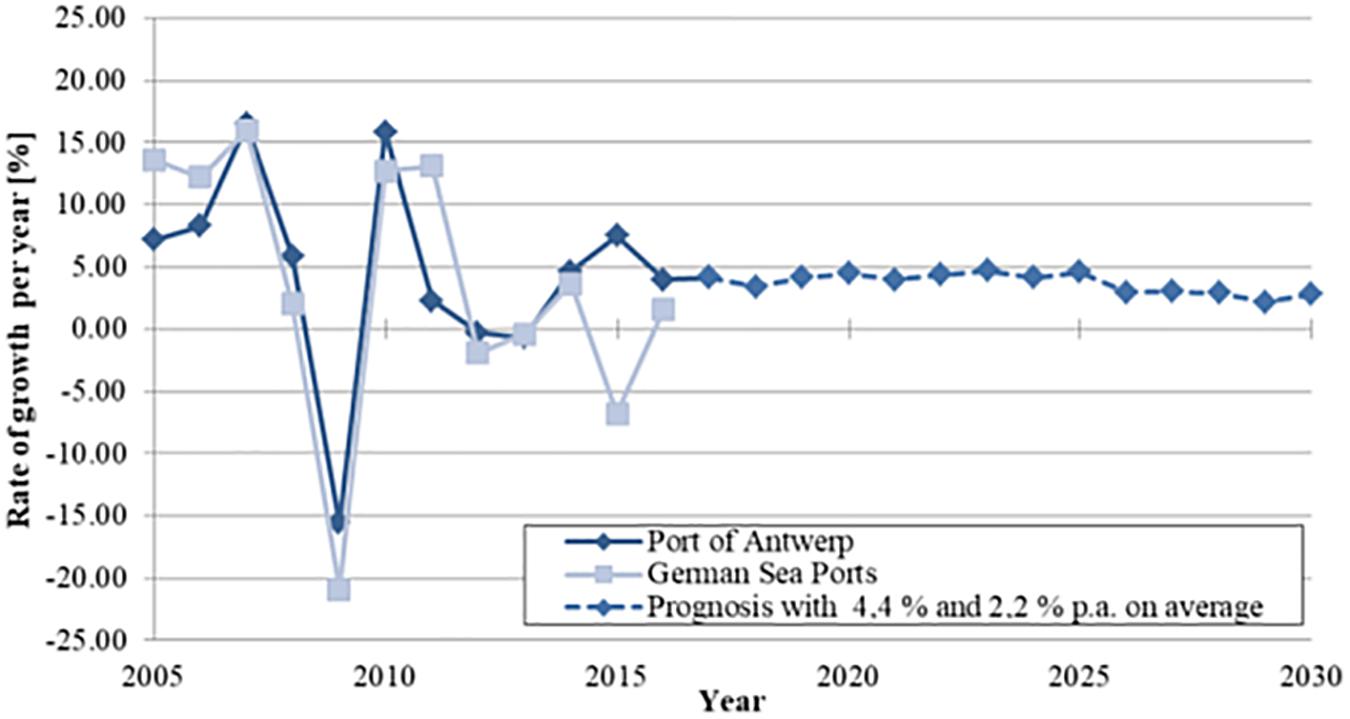
Figure 3. Development of growth rates for handled containers at Port of Antwerp and German sea ports with data from Bundesministerium für Verkehr und digitale Infrastruktur (2017), Havenbedrijf Antwerpen NV (2018).
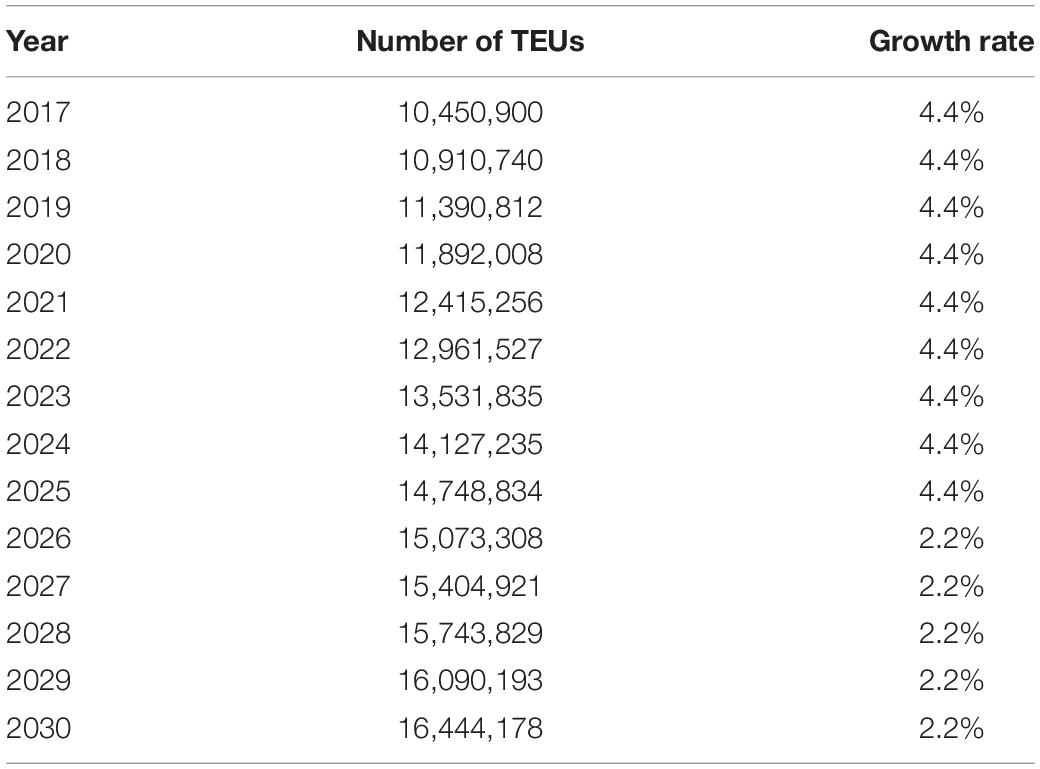
Table 2. Forecast of handled TEU at Port of Antwerp until 2030 (Havenbedrijf Antwerpen NV, 2018).
Design of Transshipment and Storage Concept for Regular Port Services
The concepts must fulfill three different functions which can be realized by different solutions. These are the storage stack layout, the lifting of containers from one module to the neighboring module, and the equipment or layout used to move the containers across the complete platform (multidirectional movements). In total five concepts that can fulfill these functions to varying degrees have been identified (see Table 3) (Gideonse, 2019). To be able to compare and review the concepts regarding the container handling on the platform, the concepts are compared based on their physical characteristics in Table 3. In this table, the number of different types of modules and equipment, the loss of storage space due to the required equipment (see section “Floating Terminal Layout Design,” Eq. 1) and the performance of each concept is presented. The handling performance is expressed as the number of moves that is required to move one container in a platform configuration of three storage modules long and two storage modules wide, from one corner of the platform to the other corner of the platform.
The different concepts are reviewed by means of a review score table, as shown in Table 4. Here the complexity states the complexity of the layout of the equipment, the storage efficiency is the ratio between space required for the equipment and storage space left for containers. The productivity states how fast or easy the containers can be moved across the platform. Scalability states how easily the platform can be modified to fit future demands or new layout configurations. The scores applied are 1 (very bad), 2 (bad), 3 (moderate), 4 (good), and 5 (excellent). Table 5 evaluates the different storage concepts. No weight factors are applied at this stage, because no information is known about the importance of each decision variable. In this table the top row represents the concepts and in the first column the decision variables are indicated.
Concepts 1, 2, and 5 have comparable scores. The use of the second concept with staggered layout is not desirable. In terms of connections between the modules, the motions of the modules due to waves result in load points from a corner of a module onto the middle of the side of a module. This will result in high forces in the coupling points of the modules. The difference between the total score of concept 1 and concept 5 is just one point. Concept 5 has higher complexity in terms of motion due to radial movements of the luffing cranes on the corner points of some storage modules. Also the performance of concept 5 is less than concept 1 because it requires an additional move to transport the container on the predefined path. Therefore, concept 1 is chosen here as a basis for the layout concept of the platform. Concept 1 is not particularly complex, has a high storage efficiency, good performance and is suitable to use in a growth scenario.
Strategic Logistics Optimization
Container Terminal Optimization
The shape and size of the modules used for the floating platform are assumed to be squares of 45 m or optionally 90 m. The scenarios developed in Section “Throughput Scenario,” as well as different dwell times will be used as a sensitivity analysis of the optimization model, in order to assess the different options for the final platform setup. Section “Design of Transshipment and Storage Concept for Regular Port Services” provides the container handling and storage concept that will be used for the optimization modeling – concept 1, gantry crane use only. The equipment under consideration for the floating terminal, along with their performance, as well as data related to their costs can be found in Table 6. While the productivity of container handling equipment is usually measured in moves/hour, it is important to transform this into TEU/hour for consistency in all calculations.
Steady-state throughput
For any amount of throughput, the model provides the optimal equipment and module selection and utilization, in terms of TEU handled per year, which results in the minimization of the annual floating platform logistics. Table 7 presents the equipment selection and utilization as well as the module selection that achieves the minimum annual costs for this static scenario, as this was defined in Sections “Floating Terminal Layout Design” and “Throughput Scenario,” and for the specific parameters mentioned in the table. The term utilization in the context of this work refers to the amount of TEU that the equipment handles within a 1 year period. Double spreader STS cranes are selected for the (un)loading operations of the terminal in order to capitalize on their increased capacity over the single spreader STS cranes. On the contrary, single spreader RMG cranes are mostly used on the storage modules. This might seem counter intuitive to the logistics optimization objective of the modeling, but it is a direct result of one of the main constraints of the model. Namely, the need for storage supersedes the costs related to the storage operations. Since a certain amount of storage modules is required in any case, the model selects modules with single spreaders in order to minimize capital, maintenance and operational costs. The breakdown of the equipment selection and utilization can be seen in Table 7.
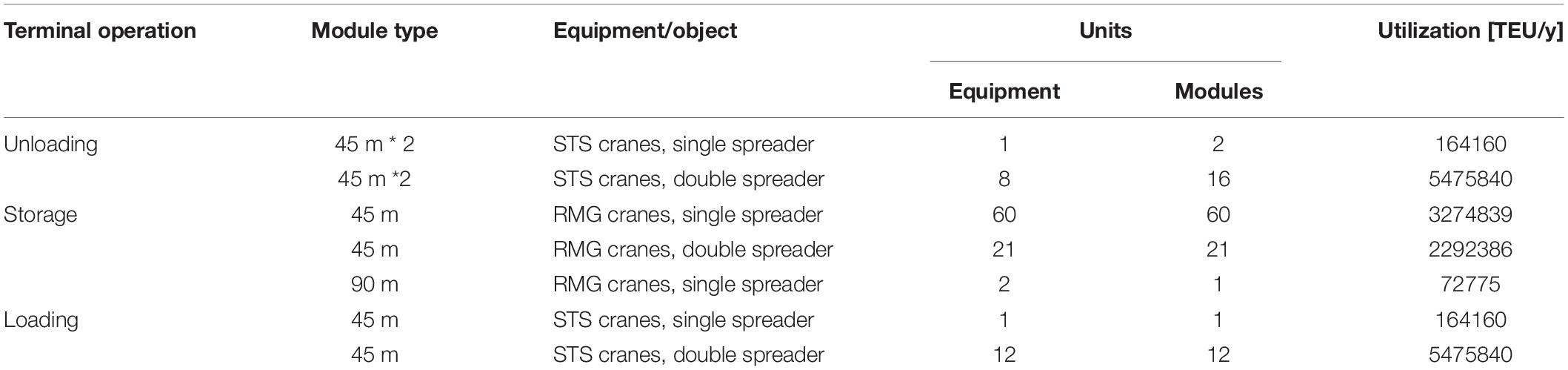
Table 7. Equipment and module selection and utilization, steady-state scenario, 1 day dwell time, 3 containers stacking height.
Figures 4, 5 provide a visualization of the terminal’s costs per operational step, and the percentage of these costs in terms of total costs, respectively. On the top left is the case for 1 day dwell time and 3 container stack height (A), and the bottom right is the case for 3 days dwell time and 5 container stack height (D). Storage incurs by far the greater costs for the floating platform, as the nature of a floating terminal demands rail-mounted gantry cranes present on all storage modules, which leads to proportionally significant capital and maintenance costs. At the same time, the cranes on each storage module are used far less individually than the STS cranes in the (un)loading procedures, which leads to low utilization rates and low operational costs.
Using a 3 days dwell time has a significant effect on all the related terminal costs, which increase by 100% or more compared to the 1 day dwell time, and the size of the terminal, which behaves accordingly. As is evident in Figure 5, the increase in the costs comes from the increase in storage costs, mainly capital costs for the additional equipment needed on each additional storage module. It is therefore imperative that the dwell time (thus storage requirements) of containers on the platform is kept to a minimum, as it is one of the parameters that has the largest and most direct effect on the terminal’s logistics. Inversely, the stacking height has a comparative effect in reducing the platform’s logistics, since the storage capacity of a 45 m module increases by 67% when the stack height is 5 containers instead of 3. Total costs can decrease by 25.5% (case A to B) to 39% (case C to D) by increasing the stack height of containers on each module.
Time series throughput
A multi-period optimization approach was also developed to handle a time series throughput, such as the dynamic scenario, where we assume that the floating terminal will handle on expected increase in container traffic until the year 2030. Figure 6 presents the necessary equipment purchase strategy, alongside the maintenance and operational costs of selected equipment throughout the whole time period into consideration. On the top left is the case for 1 day dwell time and 3 container stack height, and the bottom right is the case for 3 days dwell time and 5 container stack height. The constantly increasing throughput requires constant purchase of equipment, the overwhelming majority of which is tied to the constant need for new storage modules, in order to satisfy the storage constraints. As such, while the operational costs increase as well, since more containers are handled every year, the rate of increase is much lower, since the new equipment is not used to a high degree of its capacity.
Hinterland Logistics Optimization
In addition to the container terminal optimization, the hinterland waterway connections need to be examined as well, since they are also an integral part of the strategic design of a terminal, as are all hinterland connections - rail, truck etc. An optimization approach is used in this field as well, where two potential solutions are compared, a solution with a stopover in the Port of Antwerp where transshipment of cargo takes place, and a non-stop solution, where sea-going inland vessels transport cargo straight to the relevant hinterland region via the existing network of waterways.
A static scenario with 18193 TEU per 1 day dwell time was analyzed. For a complete comparison, both solutions with transshipment and the non-stop connection are calculated. The demand for the hinterland connection between the offshore platform and a conglomerations of hinterland terminals in the federal German state North Rhine Westphalia (NRW) breaks down to 1,002 containers (573 TEU and 429 FEU) per day. In Table 8, the durations for a round trip servicing the connection to NRW are compared for both track segments. The connection is realized by the combination of feeder vessels and the largest inland vessels (“Jowi” class”) available with a capacity of 510 TEU. The round trip of one feeder takes 1.22 days (about 102 km one way) and the inland vessel needs 3.83 days for about 296 km each way including loading and unloading at the port of destination and stopover. These durations depend on the vessels velocity on the respective segment and its capacity that defines the time needed for processing in the port.
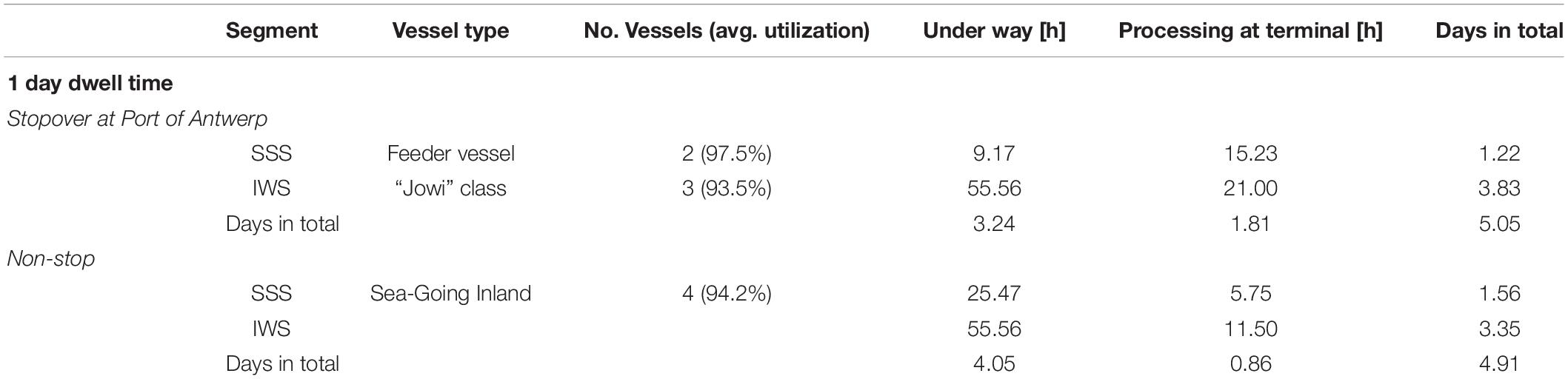
Table 8. Round trip durations per vessel for the hinterland connection transport and logistics hub – NRW insteady-state scenario with stopover at the Port of Antwerp and with non-stop solution, adapted from (Assbrock et al., 2020).
In Table 8, the solution with a Sea-Going Inland vessel is also summarized. This non-stop solution requires more time, since the Sea-Going Inland vessel is assumed to sail only with approximately one third of the feeder’s velocity, but saves compensates time at the terminals. Although the sea-going inland vessel’s capacity is about half of the feeder’s, only two more vessels per type and even in total one vessel less than for the solution with stopover at Port of Antwerp is needed to realize 1,002 containers each day. A detailed split of durations is shown in Figure 7. Here port 1 abbreviates the transport and logistics hub, port 2 the port of stopover in Antwerp and port 3 the conglomeration of terminals in NRW. All durations related to port 2, where the handling from sea-going to inland vessel takes place, are obsolete in case of the non-stop solution. This time saving amounts to about 3% of the round trip solution with stopover. The favorable solution is found to be the fastest and most beneficial one. Raising the fastest solution as the promising one for an economical operation of the offshore platform takes into account that fewer vessels are needed, since round trip durations reach a minimum even if the capacity of the deployed vessel is smaller.
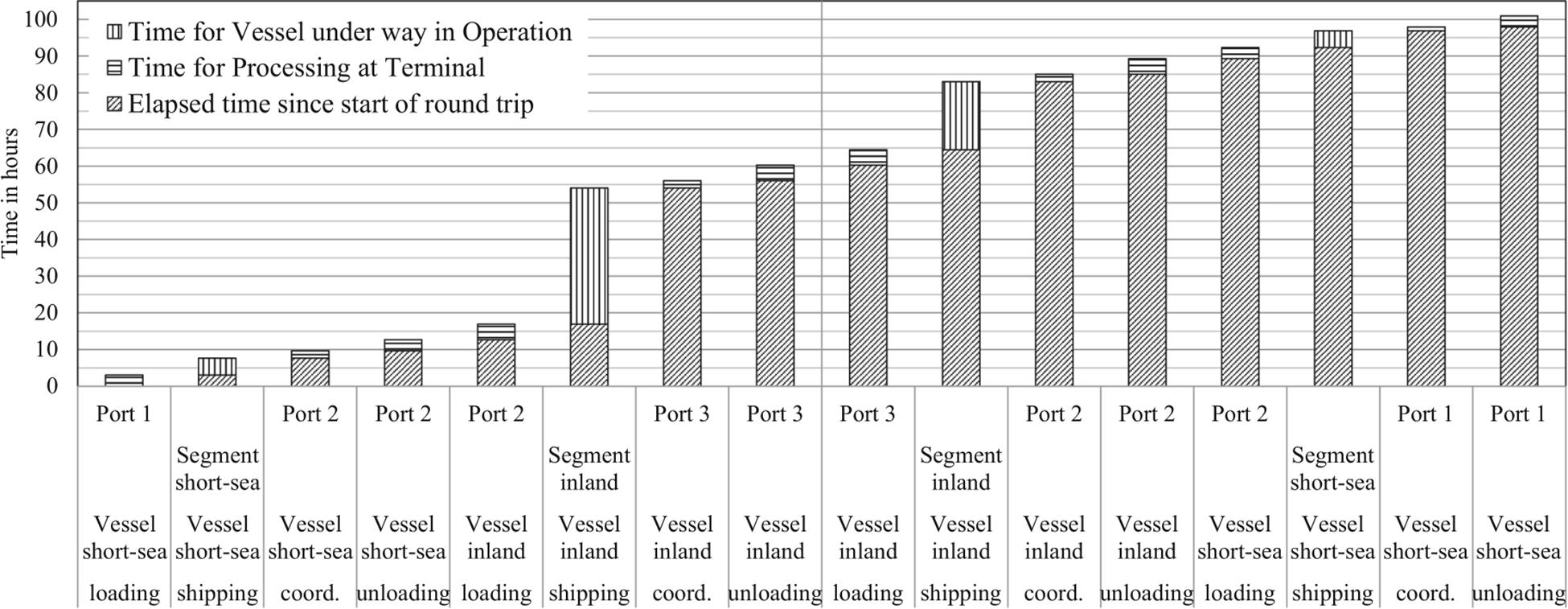
Figure 7. Split of durations for individual operations (hatched horizontally in port and vertically under way) along a round trip between the transport and logistics hub and NRW with a feeder and large inland vessel (Jowi). The cumulative sum shows the elapsed time (hatched diagonal) until the current operation (from left to right). Vessels are heading upstream/inbound first, left of the vertical line that indicates half way, and downstream on the way back (Assbrock et al., 2020).
As the non-stop solution reveals time savings, it is even worth to look at costs and their evolution. For the hinterland connection between the offshore hub and NRW a breakdown of costs per deployed vessel type and by the two operational modes is given by Table 9. These two tables deal with the costs per vessel whereas Table 10 contains the costs per TEU. Comparing both solutions by the total costs per TEU on a round trip the non-stop connection with the sea-going inland vessel is about 35% less expensive than the solution including additional handlings at the stopover between the large inland and the feeder vessel.
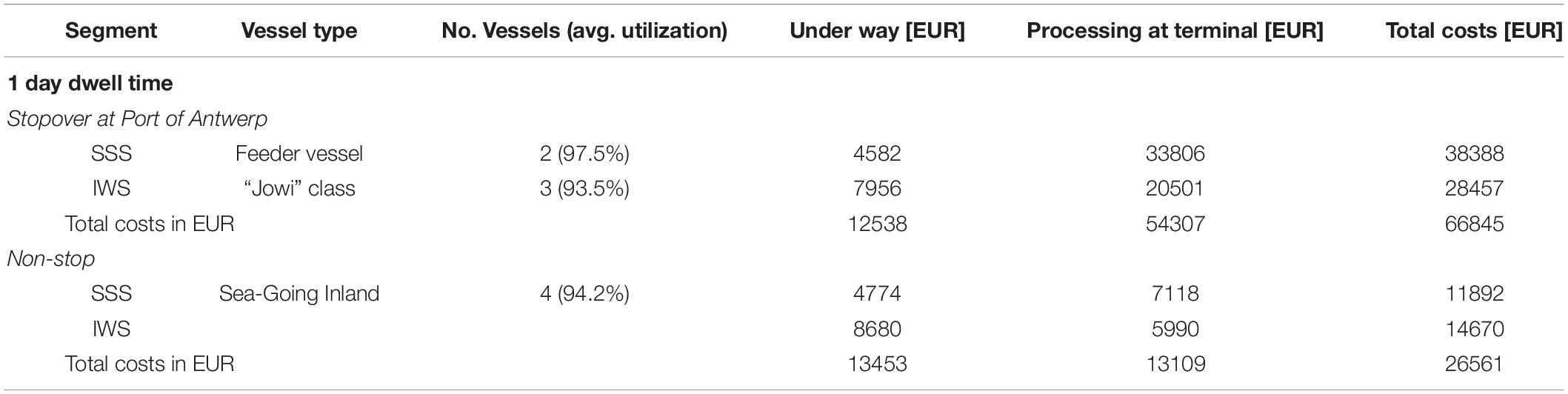
Table 9. Breakdown of costs per deployed vessel type and mode of operation in EUR on a round trip between transport and logistics hub and NRW with stopover at the Port of Antwerp and with a non-stop solution, adapted from (Assbrock et al., 2020).
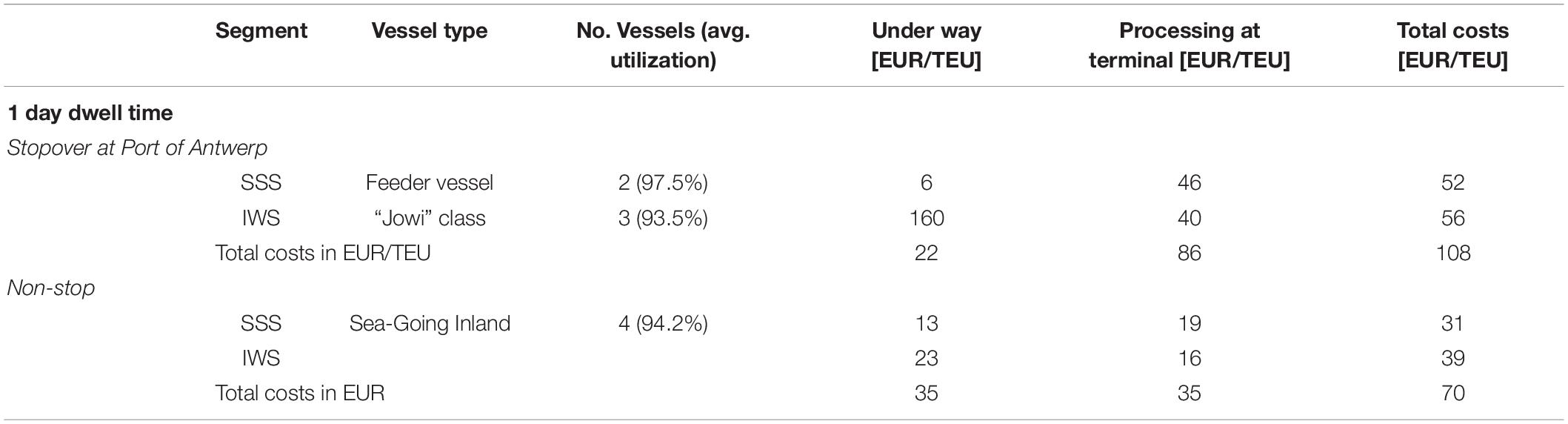
Table 10. Breakdown of costs on the hinterland connection between transport and logistics hub and NRW per TEU for the solution with stop over at the Port of Antwerp and for the non-stop solution, adapted from (Assbrock et al., 2020).
Another aspect can be observed at the partial costs on the two segments of SSS and IWS separately. Analyzing costs per vessel type and segment of the solution with stopover the feeder is much more expensive than the inland vessel, even though it completes only about a third of the distance on inland water ways. This is due to the high handling costs at the stopover. If the costs are normalized by the vessel capacity, the feeder vessel is slightly cheaper than the inland vessel on the IWS segment. Evaluating this split for the solution with one vessel type the SSS segment is always cheaper since the costs are almost equally distributed over both modes of operation (50.6% under way to 49.4% processing at terminal).
Operational Process Coordination Strategy
After the strategic logistics optimization has been applied, the proposed offshore platform is investigated at an operational level as well. To this end, we focus on tackling the stochastic floating QC scheduling problem considering stochastic crane productivity rates due to the presence of the offshore wind. The main objective of the considered problem is to minimize the expected time for (un)loading containers from a vessel to the offshore terminal. To do so, we apply a simulation/optimization framework (also referred as simheuristic) that combines the Iterated Local Search algorithm (Lourenço et al., 2019) with a typical Monte Carlo Sampling approach (Shapiro, 2003). Details on the employed Iterated Local Search algorithm are provided in Section “Optimization Approach.”
After a preliminary experimental study that compared different parameter configurations and algorithmic design options, the best Iterated Local search variant was identified. The best variant utilizes the shift heuristic and adopts the parameter values pst = 1, NLS = 101. As for the Monte Carlo Sampling approach, a maximum sample size of mmax = 2 × 104 per simulation along with a significance level of α = 0.95 was considered. The used simulation/optimization framework was executed iteratively until the total number of the available local search applications was attained. In the end of its execution, the best solution along with its objective function value was recorded.
To tackle the considered scheduling problem in a realistic setting, we have collected real-world data from the location of the offshore platform and specified several wind speed/crane productivity scenarios. Specifically, to generate these scenarios, we have gathered wind speed data and determined their impact on crane productivity. Typically, a 3-s gust speed below 20 m/s permits full crane productivity (100%) (Souravlias et al., 2019), while speed values higher than 25 m/s force the crane equipment to suspend its operation (PIANC, 2012). In this study, we only consider a gust speed range from 20 to 24 m/s, which correspond to crane productivity rates between 20 and 100%, following a simple linear conversion formula. We do not assume productivity rates equal to 100%, as this case corresponds to a deterministic scheduling problem, while this study is devoted to stochastic scheduling. Also, productivity rates lower than 20% render crane operations infeasible, therefore, in this case, the scheduling problem is not necessary to be addressed. Information per scenario with respect to the wind speed at 10 m, the corresponding 3-s gust speed at 40 m along with the corresponding crane productivity rates is shown in Table 11.
Figure 8 shows the (un)loading time in hours per instance (i.e., ocean-going vessel) under the four considered wind speed/crane productivity scenarios along with the deterministic case, in which the effect of wind is not taken into consideration. We have made the assumption that only vessels with capacities up to 6,000 TEUs visit the platform, while larger vessels call directly at the port of Antwerp An obvious conclusion is that higher wind speed results in lower crane productivity rates and thus more time is required for the entire (un)loading process to complete. However, we have managed to quantify the impact of wind on the (un)loading process of vessels than visit the offshore platform. Specifically, we notice that for case 1 the (un)loading time requires 33.93–101.68 h, case 2 demands 43.86–132.15 h, case 3 needs 61.39–189.31 h and case 4 involves 106.67–338.44 h. Therefore, we can conclude that vessels up to 6,000 TEUs require up to 101.68 h under case 1 (lowest wind speed case) and 338.44 h under case 4 (highest wind speed case) for both the loading and unloading process to complete. This result can be used by port operators and managers to determine under which weather conditions is possible to load/unload incoming vessels and decide when it is preferable to suspend temporarily the container handling operations. Therefore, the proposed simulation/optimization approach can be a beneficial decision-making tool that can be used in practice.
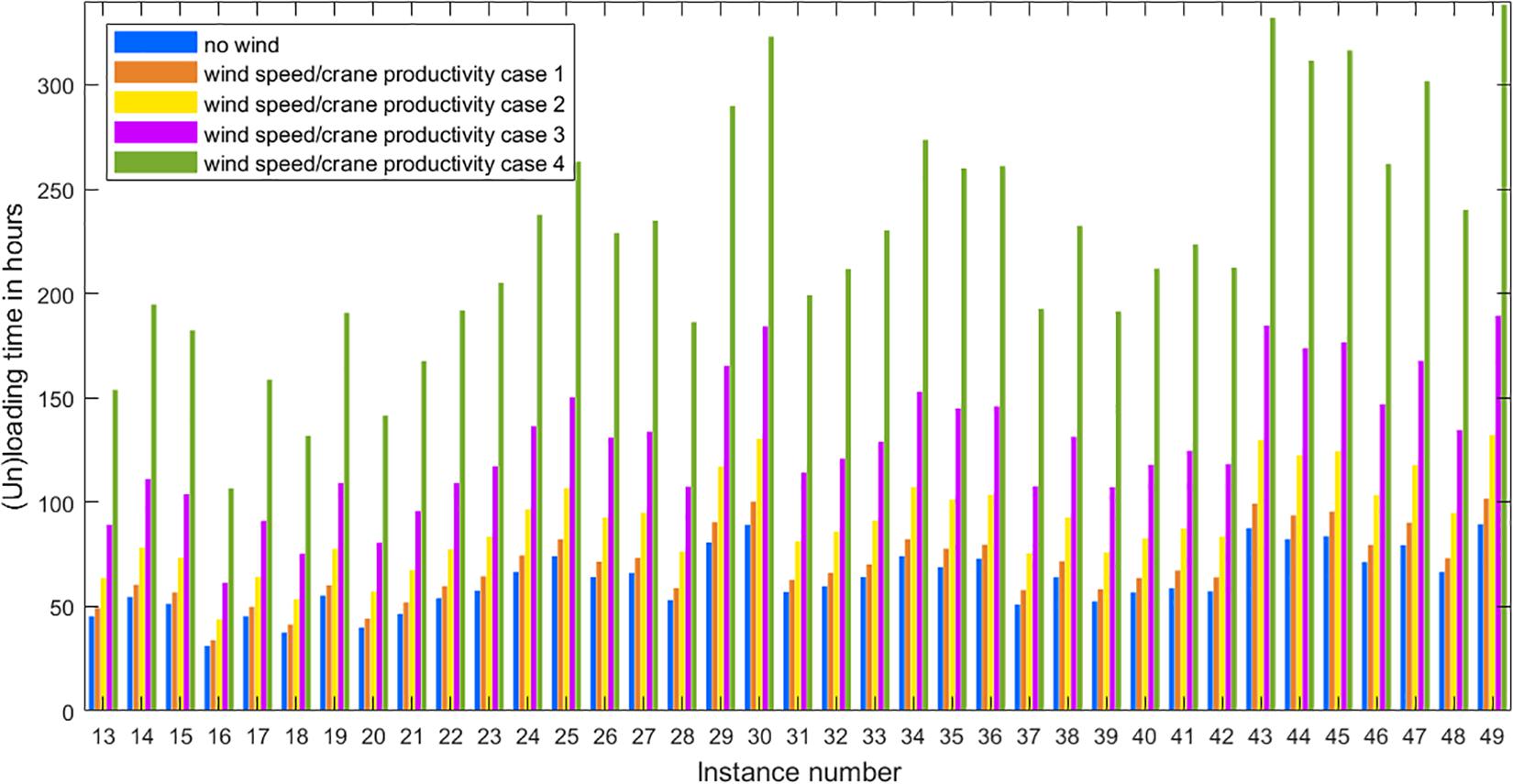
Figure 8. Time for the entire (un)loading process per ocean-going vessel in hours under the considered wind speed/crane productivity scenarios.
Conclusion
Designing a floating, modular, offshore port terminal is an intricate task, involving numerous and diverse challenges. In this paper, we presented a generic framework with the aim of addressing three key design and decision aspects of such a terminal: terminal layout design, strategic logistics optimization (involving terminal operations and hinterland connection integration), and operational process coordination.
Using a case study for a port in the Hamburg-Le Havre range, the main steps of the framework are shown. In particular, for this case study, in the design stage five terminal layout concept designs were generated and dimensioned. In addition, solutions were generated to enable moving containers to neighboring modules by spanning the gap between the modules. Based on a multi criteria analysis including complexity, storage efficiency, performance and scalability the final concept was chosen. This concept uses gantry cranes to (un)load vessels and extended RMGs for storage and handling of containers between modules.
According to the selected layout concept, an optimization approach that determines the optimal equipment, module selection, and utilization to minimize the total annual floating terminal logistics was developed. Low dwell times and higher stacking of containers provided the economically favorable terminal design, both in terms of total costs and island size, while ensuring that the throughput is efficiently handled. The main contributor to the terminal costs is the storage function, as the inability of rigid connections among modules forces the use of RMGs on each storage module, leading to disproportionally high capital and maintenance costs, while incurring low operational costs. A favorable solution would be to use only 90 m modules for storage, as they provide much more stability against environmental conditions. The developed optimization approaches can be implemented for both static floating terminals, and terminals that expect to expand their capacity and throughput in the future, if accurate throughput assumptions are made.
Additionally, the hinterland logistics of the considered offshore transport and logistics hub were analyzed. Two different solutions to realize a proposed connection between the federal German state NRW and the offshore hub were compared: a non-stop solution and a solution with an additional transshipment at the Port of Antwerp. The applied strategic model combines the round trip durations with a cost model for terminal and vessel related expenses. Servicing this hinterland connection with a stopover at the port results in longer round trip durations and higher transport costs of about 35% per TEU than with the non-stop solution. For the non-stop solution, the transport costs were 70 € per TEU, while the solution with one additional cargo handling yielded about 108 € per TEU in that given scenario. The duration of a round trip for the non-stop solution lasts up to 4.91 days, 3 h faster than the connection with stopover. Although the ocean-going inland vessels used in the non-stop solution are slower at sea waterways, the non-stop solution is economically favorable.
As for the operational process coordination, a simulation/optimization method for the scheduling of the QCs situated on the platform was introduced. The focus of our experimental evaluation was on computing estimates on the time required for an ocean-going vessel to (un)load its containers from/to the offshore platform, in the presence of offshore wind. To this end, we generated several realistic wind speed/crane productivity cases based on assumptions about the weather conditions that occur at the location of the platform. Assuming that only vessels up to 6,000 TEUs visit the platform, container loading and unloading requires up to 101.68 and 338.44 h, under the lowest and highest wind speed/crane productivity scenario, respectively. Given that the proposed method is easily applied in practice, it can be a beneficial decision-making tool, especially for port operators that need to solve related crane scheduling problems in real-time.
Future work should focus on investigating approaches for improving module construction and relaxing some hard constraints related to module connectivity. The objective of these approaches would be to reduce platform equipment and maintenance costs, directly affecting the design and decision aspects studied in this paper. Moreover, other benefits and opportunities of a floating terminal solution can be investigated. For example, the offshore platform can play the role of a disaster relief hub, especially for coastal areas, in which the access from land is difficult or time-consuming. An additional recommendation is to explore how a floating, modular platform is used not only as a port terminal, but also as an offshore energy production hub, aquafarming and potentially as a living location in the future.
Data Availability Statement
The datasets generated for this study are not readily available due to confidentiality reasons. Requests to access the datasets should be directed to DS, RC5MLlNjaG90dEB0dWRlbGZ0Lm5s.
Author Contributions
DS, ID, JL, GA, MBD, RRN, and DLS designed and carried out the research. All co-authors conducted the analyses and wrote the manuscript.
Funding
This project has received funding from the European Union’s Horizon 2020 Research and Innovation Program under grant agreement no. 774253. The opinions in this document reflect only the authors’ view and in no way reflect the European Commission’s opinions. The European Commission is not responsible for any use that may be made of the information it contains.
Conflict of Interest
The authors declare that the research was conducted in the absence of any commercial or financial relationships that could be construed as a potential conflict of interest.
Abbreviations
IWS, inland waterway shipping; NRW, North Rhine-Westphalia; QC, quay crane; QCSP, quay crane scheduling problem; RMG, rail mounted gantry crane; RSS, river-sea shipping; STS, ship-to-shore crane; SSS, short-sea shipping; TEU, twenty-foot equivalent units.
References
Abou Kasm, O., and Diabat, A. (2020). Next-generation quay crane scheduling. Transport. Res. Part C Emerg. Technol. 114, 694–715. doi: 10.1016/j.trc.2020.02.015
Ashar, A. (2013). Long-term trends in container shipping-the revised fourth revolution. Port Technol. Intern. 55, 77–93.
Assbrock, G., Ley, J., Dafnomilis, I., Duinkerken, M. B., and Schott, D. L. (2020). “Waterborne hinterland transports for floating port terminals,” in Computational Logistics. ICCL 2020. Lecture Notes in Computer Science, Vol. 12433, ed. M. M. E. Lalla-Ruiz (Cham: Springer).
Baird, A., and Rother, D. (2013). Technical and economic evaluation of the floating container storage and transshipment terminal. Transport. Res. Part C Emerg. Technol. 30, 178–192. doi: 10.1016/j.trc.2012.12.013
Bierwirth, C., and Meisel, F. (2009). A fast heuristic for quay crane scheduling with interference constraints. J. Sched. 12, 345–360. doi: 10.1007/s10951-009-0105-0
Bilican, M. S., Evren, R., and Karatas, M. (2020). A Mathematical model and two-stage heuristic for the container stowage planning problem with stability parameters. IEEE Access 8, 113392–113413. doi: 10.1109/access.2020.3003557
Bundesministerium für Verkehr und digitale Infrastruktur (2017). Bundesministerium für Verkehr und Digitale Infrastruktur, Verkehr in Zahlen. Berlin: Bundesministerium für Verkehr und digitale Infrastruktur.
Cheng, Y. L., Sen, H. C., Natarajan, K., Teo, C. P., and Tan, K. C. (2005). “Dispatching automated guided vehicles in a container terminal,” in Supply Chain Optimization. Applied Optimization, Vol. 98, eds J. Geunes and P. M. Pardalos (Boston, MA: Springer), 355–389. doi: 10.1007/0-387-26281-4_11
Dulebenets, M. A. (2016). A new simulation model for a comprehensive evaluation of yard truck deployment strategies at marine container terminals. Open Sci. J. 1, 1–28. doi: 10.1155/2016/6421943
Dulebenets, M. A., Golias, M. M., Mishra, S., and Heaslet, W. C. (2015). Evaluation of the floaterm concept at marine container terminals via simulation. Simul. Model. Pract. Theory 54, 19–35. doi: 10.1016/j.simpat.2015.02.008
Flikkema, M., and Waals, O. (2019). Space@Sea the floating solution. Front. Mar. Sci. 6:553. doi: 10.3389/fmars.2019.00553
Galle, V., Barnhart, C., and Jaillet, P. (2018). Yard crane scheduling for container storage, retrieval, and relocation. Eur. J. Operat. Res. 271, 288–316. doi: 10.1016/j.ejor.2018.05.007
Gharehgozli, A., Zaerpour, N., and de Koster, R. (2019). Container terminal layout design: transition and future. Mar. Econ. Logist. 1–30.
Gideonse, P. (2019). Conceptual Harbour Design for the Transport and Logistics Hub of Space@Sea. Delft: Delft University of Technology.
Günther, H. O., and Kim, K. H. (2006). Container terminals and terminal operations. OR Spect. 28, 437–445. doi: 10.1007/s00291-006-0059-y
Havenbedrijf Antwerpen NV (2018). Havenbedrijf Antwerpen NV Van Publiek Recht, Yearbook of Statistics 2018. Belgium: Havenbedrijf Antwerpen NV.
He, J., Huang, Y., Yan, W., and Wang, S. (2015). Integrated internal truck, yard crane and quay crane scheduling in a container terminal considering energy consumption. Expert Syst. Appl. 42, 2464–2487. doi: 10.1016/j.eswa.2014.11.016
HWWI (2006). Maritime Trade and Transport Logistics HWWI (Part A) Perspectives for Maritime Trade - Cargo Shipping and Port Economics Berenberg Bank (Part B) Perspectives of Maritime Trade and Transport Logistics - Strategies for Companies and Investors Strategy 2030. Available online at: http://www.hwwi.org/fileadmin/hwwi/Publikationen/Partnerpublikationen/Berenberg/Strategy_2030_Maritime_Trade_and_Transport_Logistics.pdf (accessed March 1, 2019).
Iannone, R., Miranda, S., Prisco, L., Riemma, S., and Sarno, D. (2016). Proposal for a flexible discrete event simulation model for assessing the daily operation decisions in a Ro-Ro terminal. Simul. Model. Pract. Theory 61, 28–46. doi: 10.1016/j.simpat.2015.11.005
Jordan, M., Morris, C., and Dix, A. (2007). “The Floaterm Concept: Reducing Terminal Congestion with Waterside Cranes,” in Proceedings of the 11th Triennial International Conference on Ports 2007: 30 Years of Sharing Ideas: 1977-2007, San Diego, CA.
Kim, K., and Park, Y.-M. (2004). A crane scheduling method for port container terminals. Eur. J. Operat. Res. 156, 752–768. doi: 10.1016/s0377-2217(03)00133-4
Lamas-Pardo, M., Iglesias, G., and Carral, L. (2015). A review of very large floating structures (VLFS) for coastal and offshore uses. Ocean Eng. 109, 677–690. doi: 10.1016/j.oceaneng.2015.09.012
Lourenço, H. R., Martin, O. C., and Stützle, T. (2019). “Iterated local search: framework and applications,” in Handbook of Metaheuristics, eds F. Glover and G. Kochenberger (Dordrecht: Kluwer Academic Publishers), 129–168. doi: 10.1007/978-3-319-91086-4_5
Maletić, J., Prigoda, L., and Čekerevac, Z. (2018). Technical solutions and assessment of economic effects of construction of an offshore terminal. Mech. Transport Commun. Acad. J. 15:97.
Monaco, M. F., and Sammarra, M. (2011). Quay crane scheduling with time windows, one-way and spatial constraints. Intern. J. Ship. Transp. Logist. 3, 454–474. doi: 10.1504/ijstl.2011.041137
Petering, M. E. (2010). Development and simulation analysis of real-time, dual-load yard truck control systems for seaport container transshipment terminals. OR Spect. 32, 633–661. doi: 10.1007/s00291-009-0183-6
PIANC (2012). Criteria for the (un)Loading of the Container Vessels, Technical Report. PIANC Working Group 115. Available online at: https://www.pianc.org/publications/marcom/criteria-for-the-unloading-of-container-vessels (accessed February 15, 2019).
Qiu, L., Hsu, W. J., Huang, S. Y., and Wang, H. (2002). Scheduling and routing algorithms for AGVs: a survey. Intern. J. Product. Res. 40, 745–760. doi: 10.1080/00207540110091712
Rahimikelarijani, B., Abedi, A., Hamidi, M., and Cho, J. (2018). Simulation modeling of Houston Ship Channel vessel traffic for optimal closure scheduling. Simul. Modell. Pract. Theory 80, 89–103. doi: 10.1016/j.simpat.2017.10.004
Sammarra, M., Cordeau, J. F., Laporte, G., and Monaco, M. F. (2007). A tabu search heuristic for the quay crane scheduling problem. J. Sched. 10, 327–336. doi: 10.1007/s10951-007-0029-5
Souravlias, D., Duinkerken, M. B., Morshuis, S., Schott, D. L., and Negenborn, R. R. (2019). “Stochastic floating quay crane scheduling on offshore platforms: a simheuristic approach,” in Proceedings of the 21th International Conference on Harbor, Maritime and Multimodal Logistic Modelling and Simulation (HMS 2019), Lisbon. 62–71.
van den Bos, W. (2011). Wind influence on container handling, equipment and stacking. Port Technol. Intern. J. Edn. 29, 89–95.
Wang, K., Zhen, L., Wang, S., and Laporte, G. (2018). Column generation for the integrated berth allocation, quay crane assignment, and yard assignment problem. Transport. Sci. 52, 812–834. doi: 10.1287/trsc.2018.0822
Wawrzyniak, J., Drozdowski, M., and Sanlaville, É (2020). Selecting algorithms for large berth allocation problems. Eur. J. Operat. Res. 283, 844–862. doi: 10.1016/j.ejor.2019.11.055
Xin, J., Negenborn, R. R., and Lodewijks, G. (2014). Energy-aware control for automated container terminals using integrated flow shop scheduling and optimal control. Transport. Res. Part C Emerg. Technol. 44, 214–230. doi: 10.1016/j.trc.2014.03.014
Keywords: terminal design, offshore terminals, floating islands, logistics hub, logistics optimization, operational coordination
Citation: Souravlias D, Dafnomilis I, Ley J, Assbrock G, Duinkerken MB, Negenborn RR and Schott DL (2020) Design Framework for a Modular Floating Container Terminal. Front. Mar. Sci. 7:545637. doi: 10.3389/fmars.2020.545637
Received: 25 March 2020; Accepted: 20 October 2020;
Published: 12 November 2020.
Edited by:
Ingo Drummen, Maritime Research Institute Netherlands, NetherlandsReviewed by:
Lida Teneva, Independent Researcher, Sacramento, United StatesMaxim A. Dulebenets, FAMU-FSU College of Engineering, United States
Copyright © 2020 Souravlias, Dafnomilis, Ley, Assbrock, Duinkerken, Negenborn and Schott. This is an open-access article distributed under the terms of the Creative Commons Attribution License (CC BY). The use, distribution or reproduction in other forums is permitted, provided the original author(s) and the copyright owner(s) are credited and that the original publication in this journal is cited, in accordance with accepted academic practice. No use, distribution or reproduction is permitted which does not comply with these terms.
*Correspondence: Dingena L. Schott, RC5MLlNjaG90dEB0dWRlbGZ0Lm5s