- 1National Research Council, Institute for Biological Resources and Marine Biotechnologies, Ancona, Italy
- 2Department of Information Engineering, Università Politecnica delle Marche, Ancona, Italy
In the past decades, the Automatic Identification System (AIS) has been employed in numerous research fields as a valuable tool for, among other things, Maritime Domain Awareness and Maritime Spatial Planning. In contrast, its use in fisheries management is hampered by coverage and transmission gaps. Transmission gaps may be due to technical limitations (e.g., weak signal or interference with other signals) or to deliberate switching off of the system, to conceal fishing activities. In either case such gaps may result in underestimating fishing effort and pressure. This study was undertaken to map and analyze bottom trawler transmission gaps in terms of duration and distance from the harbor with a view to quantifying unobserved fishing and its effects on overall trawling pressure. Here we present the first map of bottom trawler AIS transmission gaps in the Mediterranean Sea and a revised estimate of fishing effort if some gaps are considered as actual fishing.
Introduction
The Automatic Identification System (AIS) was originally introduced by the International Maritime Organization to enhance safety at sea by enabling navigators to view the position, identity, and direction of other ships in the area. Its characteristics include a considerable accuracy in providing vessel position (Gioia et al., 2013), high-frequency transmission (James et al., 2018), and accessibility through several online portals (Le Tixerant et al., 2018). Even though AIS was initially developed to avoid collisions between vessels (Goerlandt and Kujala, 2011; Wu et al., 2017), its features make it a useful tool for Maritime Spatial Planning (MSP) and for the management of a number of maritime activities (Shelmerdine, 2015). Indeed, AIS is now employed in a variety of research areas (Svanberg et al., 2019) that range from oil spill prevention (Eide et al., 2007; Schwehr and McGillivary, 2007) to identification of fishing vessel behavior (Natale et al., 2015; De Souza et al., 2016). Since May 2014, all European fishing vessels exceeding 15 m length overall are required to carry and operate an AIS device (European Commission [EC], 2011). The system has been providing valuable Monitoring, Control and Surveillance (MCS) data on a large amount of European fishing activities. Moreover, its voluntary adoption by several non-European fishing fleets has been supplying additional information to map the areas exploited by these fleets, such as the Mediterranean Sea (Ferrà et al., 2018).
Automatic Identification System is the only existing large-scale system supplying actively transmitted data (Ford et al., 2018) and provides valuable information to understand maritime routes and fishing activities. Nonetheless, a major disadvantage is that it suffers from transmission gaps. Such gaps may be due either to technical limitations or to deliberate manipulation of the AIS transponder, which may be switched off (Winward, 2015) to obscure the vessel’s destination or hide illegal fishing (Ahlberg and Danielsson, 2015). Manipulation is a cause for concern for financial and security stakeholders, since it undermines reliance on the system’s ability to track vessels and monitor areas (Winward, 2015). For this reason, identifying vessels engaging in this practice is especially important (Hsu et al., 2019).
The technical limitations of AIS, e.g., incomplete AIS reception, system saturation in areas with high vessel traffic, faulty equipment and/or line of sight limits, have been widely discussed (Harati-Mokhtari et al., 2007; Merchant et al., 2012; Papi et al., 2014). Some data providers have been developing solutions to address them, for example by combining land-based and satellite AIS signals which enable vessel detection hundreds of miles from any land-based receiver (Høye et al., 2008; Pallotta et al., 2013a). Some researchers have proposed a number of approaches that range from statistical models to anomaly detection algorithms (Guerriero et al., 2010; Mazzarella et al., 2017; Ford et al., 2018; Fernandes et al., 2019). Automatic Identification System reception quality maps of fishing vessels in EU (Vespe et al., 2016) and global waters (Kroodsma et al., 2019) have recently been published.
Where fishery management is concerned, mapping transmission gaps – be they accidental or deliberate – and integrating them with high temporal and spatial resolution data of fishing position is essential to gain an exhaustive and spatially explicit knowledge of the fishing grounds exploited by vessels. Moreover, it is a key requirement for the implementation of an effective ecosystem approach to fisheries management in areas under multiple (e.g., local, national and international) jurisdictions and in those involved by competing activities or encompassing sensitive habitats.
Based on such considerations, this study was undertaken to map the AIS transmission gaps of bottom trawlers operating in the Mediterranean Sea with a view to identifying those that may represent potential hidden fishing activities and to quantifying the unobserved fishing effort. The data subset suspected to conceal potential fishing activities was combined with the known fishing dataset (Tassetti et al., 2016; Ferrà et al., 2018), to compute the extent to which the overall trawling activity would increase if some of these gaps were considered as actual fishing activities. Accurate estimation of such increase would provide valuable information for Mediterranean fisheries, where trawling is the main fishing activity (Merino et al., 2019) and where in some areas its footprint exceeds 80% of the continental shelf (Amoroso et al., 2018).
Materials and Methods
Terrestrial AIS data at 5-min resolution were purchased from a private provider1 for all the fishing vessels (AIS type 30) operating in the Mediterranean Sea in 2014. Gaps in tracking data were identified by pre-processing the raw dataset with the statistical software R (R Development Core Team and R Core Team, 2018). Repeated points and outliers with a speed >20 knots (kn) or located inland were removed; the remaining pings were filtered so as to retain only the records of the bottom trawlers identified in a previous study by our group (Ferrà et al., 2018).
Subsequently, the Maritime Mobile Service Identity (MMSI) was used to create a tracking layer by ordering each MMSI record by datetime. Assuming that vessels travel in a straight line, track duration and vessel speed were computed for each segment based on the difference between consecutive pings. Since the acquired reporting rate was 5 min, tracks with a duration exceeding a predefined threshold of 30 min were tagged as “unknown,” retained and considered as transmission gaps (Figure 1). Computation of the monthly ratio of total unknown time/total AIS time in the Mediterranean (tests for differences in monthly patterns of gaps) demonstrated a nearly constant pattern and allowed subsequent analyses to be performed on a yearly scale.
The annual unknown activity was quantified by intersecting all unknown segments with a 1 km × 1 km grid of the Mediterranean Sea by spatial joining each cell with overlapping portions of unknown segments and then summing their relative length (in km) (Ferrà et al., 2018; Tassetti et al., 2019).
The unknown segments were grouped according to their duration (t) into four categories (t ≤ 1 h; 1 < t ≤ 2 h; 2 < t ≤ 24 h; > 24 h) and according to distance from the harbor into four categories (in the harbor, within 1 nautical mile [nm] of the harbor, between 1and 5 nm, and >5 nm from the harbor). The percent frequency of each category was then computed.
To establish whether some gaps, identified at the sites of the main fishing grounds, could conceal fishing activities, the unknown tracks overlaying the trawl footprint (Ferrà et al., 2018) hotspots were analyzed for speed and duration. Those inside harbors were excluded. The most frequent duration and speed values were extracted to create a subset of unknown segments. After their validation based on the common speed of bottom trawlers in the Mediterranean Sea (2–5 kn), the segments were considered as representing potential hidden fishing activity. They were then added to the trawling dataset (Ferrà et al., 2018), to estimate their contribution to fishing activity.
Finally, the percent potential fishing activities over total fishing (known fishing plus potential fishing), were mapped and aggregated in each 30 × 30 min cell of the General Fisheries Commission for the Mediterranean (GFCM) Statistical Grid.2 The procedure allowed identifying those areas where potential hidden fishing was more intense. One such area, the Strait of Sicily, was selected as a case study and analyzed in detailed maps combining known and potential fishing activity (sum and ratio: potential/[known + potential]).
Results
The initial dataset consisted of 57,029,372 position records of 4,343 vessels tracked in the Mediterranean Sea throughout 2014. Pre-processing allowed selecting 37,322,648 records broadcast by 2,123 bottom trawlers identified in a previous study (Ferrà et al., 2018), which accounted for 65% of the initial records. These records were retained and processed to estimate tracking gaps. The procedure allowed identifying 733,143 unknown tracks connecting 1,466,286 pings, corresponding to 4% of the AIS bottom trawl records.
The AIS data coverage in each month of 2014, expressed as the ratio of the duration of the unknown tracks to the duration of total activity, showed an almost constant value that ranged from a minimum of 65% (May) to a maximum of 72% (September). Altogether, 68% of the total time recorded by AIS in 2014 was categorized as unknown.
Unknown tracks were identified in all European, Turkish, and Israeli coastal areas, and rarely in areas off the coast, at depths up to 1,000 m (Figure 2). In the Mediterranean Sea they accounted for 3,954,925.2 km, of which 19% (741,114.47 km) were concentrated within 3 nm from the coast or up to a depth of 50 m. The unknown tracks were most numerous in the Thermaic Gulf, the western Mediterranean, the western Adriatic, and the Tyrrhenian coast. The southern area of the basin was largely free of unknown tracks except for the Strait of Sicily.
Slightly more than 60% of unknown tracks had a duration of ≤2 h (mean, 45.8 min); of these, about 50% lasted ≤1 h (mean, 38.6 min). The mean duration of gaps lasting 1–2 h was 79 min.
With regard to gaps >2 h, those ranging from 2 to 24 h had a mean duration of 594.7 min and accounted for 29% of all unknown tracks, whereas those >24 h had a mean duration of 3 days.
Tracks lying wholly or partially in harbors accounted for 32% and were excluded from subsequent analyses. Of the unknown tracks identified outside harbors, 35 and 58% of those lasting respectively 2–24 h and >24 h were wholly or partially located within 1 nm of a harbor (Table 1). Notably, the number of tracks >24 h diminished as the distance from the harbor increased, whereas those lasting 2–24 h were less numerous 1–5 nm from the harbor and more numerous at greater distances. In contrast, the number of gaps lasting up to 2 h increased gradually as vessels left the coastal waters (within 1 nm of the harbor) and sailed out to sea. The increment was very large for tracks of up to 1 h and slightly smaller for tracks whose duration was 1–2 h.
More than 78% of the unknown tracks overlapping trawling hotspots lasted up to 2 h and the interquartile range of speed was 2–5 kn. This type of tracks was filtered and mapped as representing potential hidden fishing (Figure 3). Summation of this track subset to the known fishing activities involved an 8% increase in the trawling effort in the Mediterranean basin as a whole.
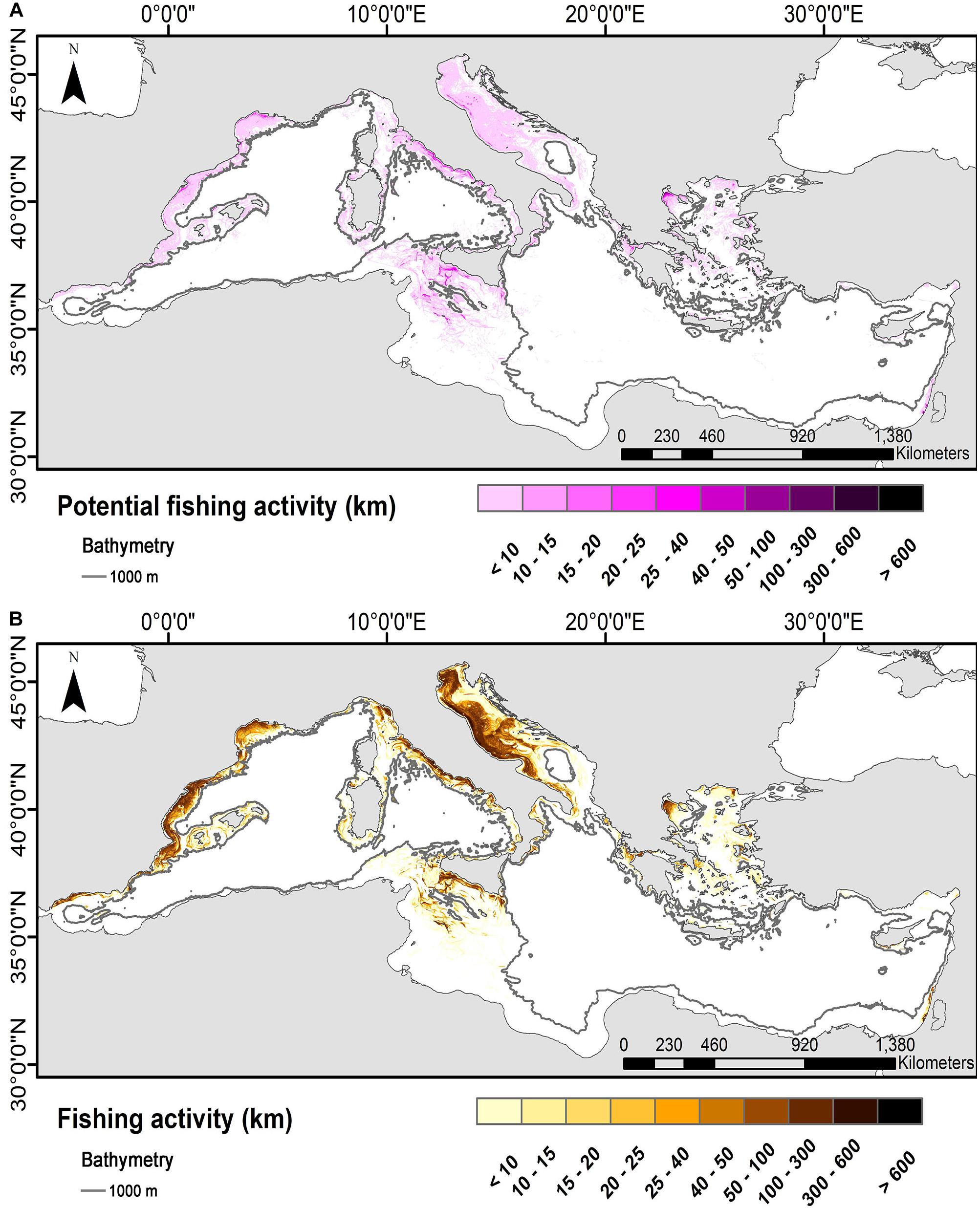
Figure 3. Potential (A) and known annual fishing activity (Ferrà et al., 2018, B) in the Mediterranean Sea in 2014.
A more detailed analysis using the GFCM grid highlighted a greater increase in the total trawling effort in some areas such as the Strait of Sicily, the Gulf of Alexandretta, and the waters south of Crete (Figure 4).
In the Strait of Sicily (black bounding box in Figure 4), the potential fishing subset added to the known fishing would increase the total fishing activity by around 20% (Figure 5). The potential hidden activity in this area showed no distinctive spatial pattern, even though it was very limited even in the most extensively exploited fishing grounds (Figure 6).
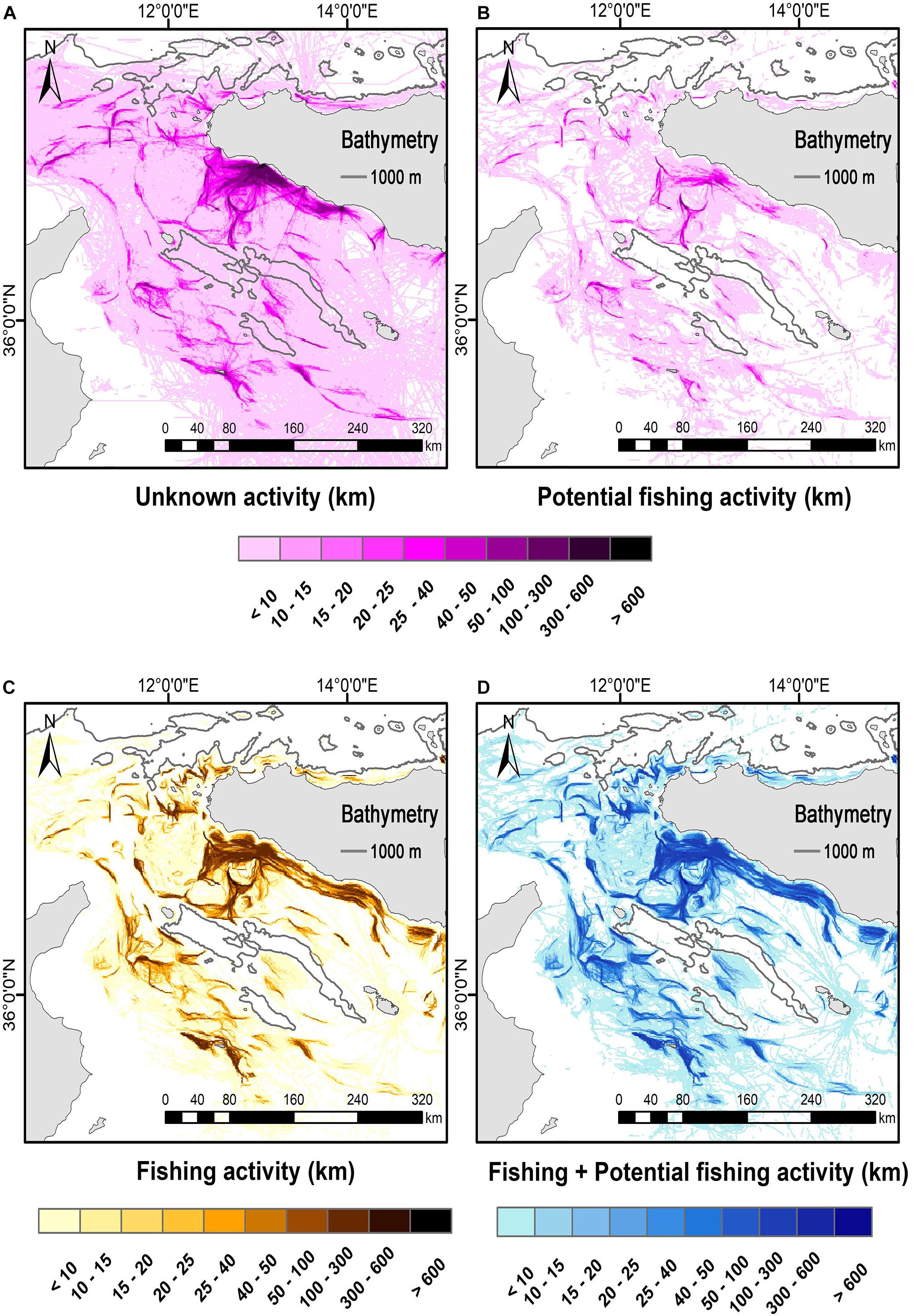
Figure 5. Unknown annual activity (A), potential (B), known (C), and total (known + potential) annual fishing activity (D) in the Strait of Sicily (2014).
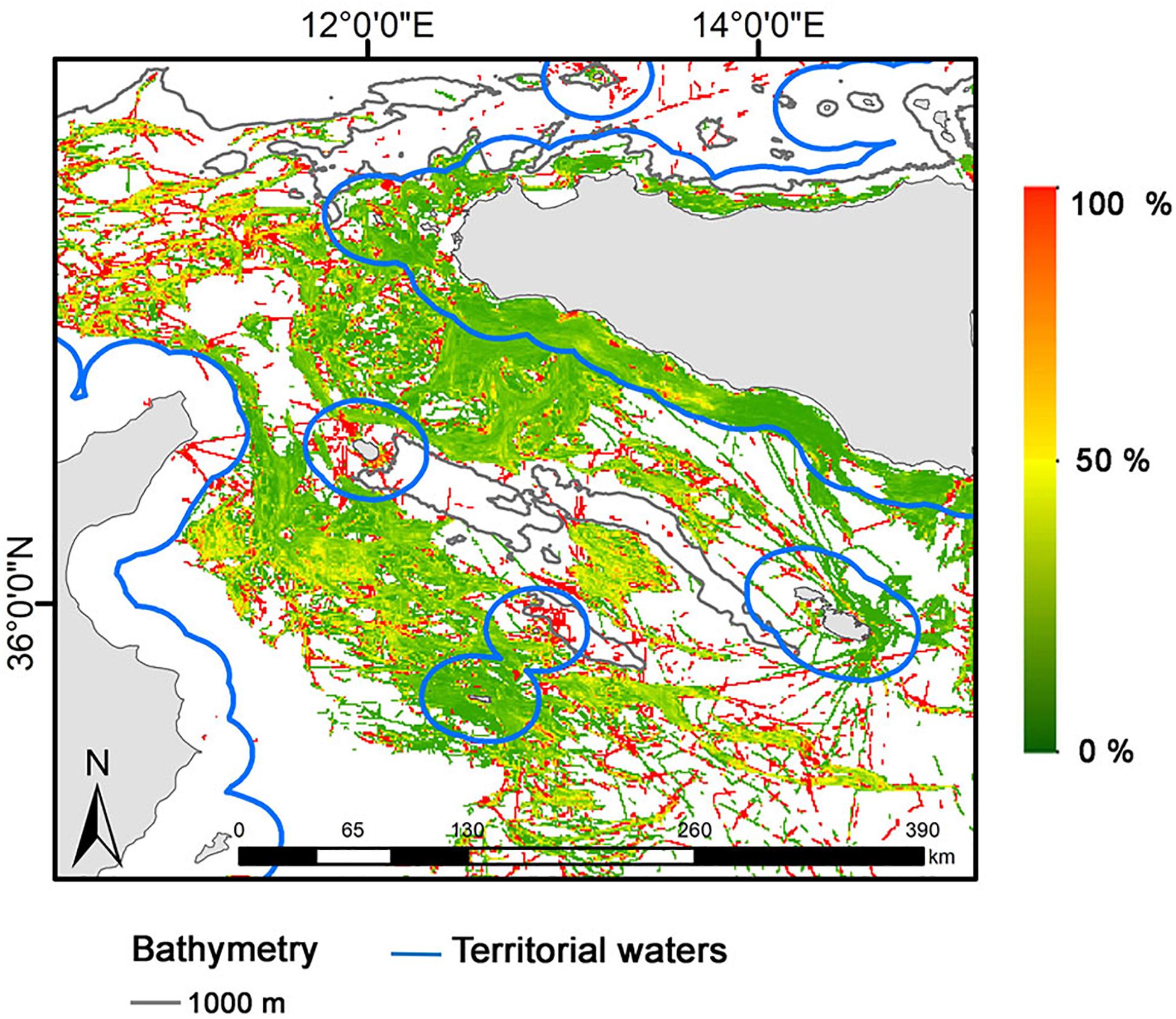
Figure 6. Map of unobserved out of total fishing activity in the Strait of Sicily (percent values, 2014).
Discussion
The use of AIS tracking data for Maritime Domain Awareness involves two major drawbacks: transmission gaps and the ability of the system to be switched off (McCauley et al., 2016; Shepperson et al., 2018). These disadvantages are particularly important in fisheries mapping, since they result in underestimating the extension of fishing grounds and total fishing effort and pressure.
Although AIS reception quality in the Mediterranean Sea has recently been mapped by FAO (Kroodsma et al., 2019), it involves all fishing activities, whereas maps for specific gears or studies aimed at interpreting them are not yet available. The aim of this study was to provide a map of the spatial distribution of bottom trawler transmission gaps in the Mediterranean in 2014, to identify any potential unobserved fishing activity and to assess the extent to which it contributes to the trawling pressure.
Clusters of high values of unknown activity were mostly found in the northern Mediterranean in correspondence to the hotspots identified by Ferrà et al. (2018). Even though these gaps were a small proportion of the total number of AIS records, they accounted for a large proportion of coverage in terms of duration, since some lasted more than 30 days. Considering that most clusters coincided with areas where signal strength is expected to be high (Vespe et al., 2016), it is reasonable to hypothesize that the gaps were due either to deliberate manipulation of the device or to technical problems related to transmission in high-density traffic areas (ICES, 2015; Plass et al., 2015) or to poor weather conditions (Pelich et al., 2014; Nguyen et al., 2020), rather than to inadequate coverage (Mazzarella et al., 2016). In contrast, the limited known and unknown activity seen in the southern Mediterranean is likely due to the fact that the area is largely exploited by trawlers from non-EU Mediterranean countries, few of which broadcast AIS.
The proportion of unknown tracks having a duration of up to 2 h was limited in harbors, it increased with increasing distance from the harbor, and it peaked in areas farther than 5 nm from the home harbor. The large proportion of relatively short unknown tracks within a 5 nm radius from harbors may be due to illegal fishing (European Commission [EC], 2006), delayed switching on of the device after departure, or early switching off at arrival. In contrast, the unknown tracks exceeding 24 h were more numerous in harbors and less numerous at a greater distance from them. These data can be explained by the switching off of all electric devices while at mooring. However, part of the transmission gaps can probably be explained by the fact that, since vessels can be tracked in real time through free-access AIS websites, fishermen switch the AIS off when leaving harbor to prevent competitors from learning which fishing grounds they will exploit. Finally, the irregular pattern of tracks lasting 2–24 h probably reflects a greater variability of the duration of this category of tracks rather than fishermen’s behavior.
A considerable proportion of the unknown tracks overlapping known fishing hotspots lasted no more than 2 h and their mean speed was 2–5 kn. Since these values are typical of the fishing operations of most Mediterranean bottom trawlers (Lucchetti, 2008; Lambert et al., 2012; Keskin et al., 2014; Arjona-Camas et al., 2019), this subset was felt to represent hidden fishing and was used to sketch a more realistic picture of the overall fishing activity.
Although towing duration can last more than 2 h, especially in the deeper fishing grounds, and although a vessel is unlikely to spend more than 24 h at sea with its AIS switched off without fishing, the segments longer than 24 h were not considered as potential fishing. This may have resulted in underestimating total fishing pressure; yet such segments were not felt to represent potential fishing, because vessels are unlikely to keep a straight course for long periods (Lambert et al., 2012) and because in such long periods they can fish and sail several times.
When potentially hidden and known fishing activities were summed, the percent increase of the fishing effort was not significant in the Mediterranean Sea as a whole. However, use of the 1 nm × 1 nm reference grid enabled a fine analysis of the spatial localization of potentially unobserved fishing and showed that in some areas it was quite large. In the Strait of Sicily the increase was 20%; yet, in the hotspots of this area, potential fishing contributed little to total trawling activity and was randomly distributed. This suggests that the unknown tracks were largely to be ascribed to system saturation in this heavily trafficked area rather than to deliberate switching off of the AIS device.
In conclusion, the present findings confirm that the reasoned interpretation of tracking gaps in relation to fishing data can provide more accurate information on fishing ground extension, fishermen’s behavior and fishing pressure. This information is useful to implement fisheries management strategies both on the regional and the local scale, as also required by EU regulations (European Commission [EC], 2002, 2013; The European Parliament and the Council of the European Union, 2008; European Parliament and the Council of the European Union, 2014).
Notably, quantification of the unobserved activity of each vessel would allow identifying those vessels that consistently switch the AIS device off, thus increasing transparency at sea (Malarky and Lowell, 2018) and contributing to curb Illegal Unreported and Unregulated fishing (Bastani and de Zegher, 2019). This is even more important in regulated areas, where AIS data gaps could unmask attempts to conceal illegal activities (Tassetti et al., 2019), and would allow decision-makers to implement mitigation strategies and MCS plans.
AIS optimization would be of great interest to policymakers and also to environmental and fisheries scientists (Svanberg et al., 2019), who are often called upon to help develop management measures aimed at marine environment preservation and fisheries regulation. Whereas the tracking gaps are expected to be overcome by satellite AIS (Pallotta et al., 2013b; Wu et al., 2017), strengthening policy provisions seems essential to enforce the proper use of AIS and reduce deliberate switching off of the device (McCauley et al., 2016).
Data Availability Statement
The data analyzed in this study is subject to the following licenses/restrictions: AIS raw data is not anonymous and owned by CNR. Requests to access the aggregated datasets should be directed to CF, Y2FybWVuLmZlcnJhdmVnYUBjbnIuaXQ=.
Author Contributions
CF and ANT conceived the ideas and designed the methodology. CF collected and analyzed the data. CF, ANT, and GF wrote the manucript. GS, ENA, and AG contributed critically to the manuscript drafting. All authors gave final approval for publication.
Conflict of Interest
The authors declare that the research was conducted in the absence of any commercial or financial relationships that could be construed as a potential conflict of interest.
Footnotes
References
Ahlberg, E., and Danielsson, J. (2015). Handling and Analyzing Marine Traffic Data. Available online at: http://publications.lib.chalmers.se/records/fulltext/237234/237234.pdf (accessed April, 2019).
Amoroso, R. O., Pitcher, C. R., Rijnsdorp, A. D., McConnaughey, R. A., Parma, A. M., Suuronen, P., et al. (2018). Bottom trawl fishing footprints on the world’s continental shelves. Proc. Natl. Acad. Sci. U.S.A. 115, E10275–E10282. doi: 10.1073/pnas.1802379115
Arjona-Camas, M., Puig, P., Palanques, A., Emelianov, M., and Durán, R. (2019). Evidence of trawling-induced resuspension events in the generation of nepheloid layers in the Foix submarine canyon (NW Mediterranean). J. Mar. Syst. 196, 86–96. doi: 10.1016/j.jmarsys.2019.05.003
Bastani, H., and de Zegher, J. F. (2019) Are Bans Effective under Limited Monitoring? Evidence from High Seas Management. Available online at: https://ssrn.com/abstract=3312963 (accessed January 15, 2019).
De Souza, E. N., Boerder, K., Matwin, S., and Worm, B. (2016). Improving fishing pattern detection from satellite AIS using data mining and machine learning. PLoS One 11:e158248. doi: 10.1371/journal.pone.0158248
Eide, M. S., Endresen, Ø, Brett, P. O., Ervik, J. L., and Røang, K. (2007). Intelligent ship traffic monitoring for oil spill prevention: risk based decision support building on AIS. Mar. Pollut. Bull. 54, 145–148. doi: 10.1016/j.marpolbul.2006.11.004
European Commission [EC] (2002). Recommendation of the European parliament and of the council concerning the implementation of integrated coastal zone management in Europe (2002/413/EC). Off. J. Eur. Commun. 148, 24–27.
European Commission [EC] (2006). Council Regulation (EC) No 1967/2006 of 21 December 2006 Concerning Management Measures for the Sustainable Exploitation of Fishery Resources in the Mediterranean Sea, Amending Regulation (EEC) No 2847/93 and Repealing Regulation (EC) No 1626/94. 75pp. Brussels: European Commission.
European Commission [EC] (2011). Commission directive 2011/15/EU of 23 February 2011 amending directive 2002/59/EC of the European parliament and of the council establishing a community vessel traffic monitoring and information system. Off. J. Eur. Union 49, 33–36.
European Commission [EC] (2013). Common fisheries policy (CFP) regulation No. 1380/2013 of the European parliament and of the council. Off. J. Eur. Commun. 354, 2–61.
European Parliament and the Council of the European Union (2014). Directive 2014/89/EU of the European Parliment and of the council of 23 July 2014 establishing a framework for maritime spatial planning. Off. J. Eur. Union L 257, 135–145.
Fernandes, J. A., Granado, I., Murua, H., Arrizabalaga, H., Zarauz, L., Mugerza, E., et al. (2019). “Bay of Biscay VMS/logbook comparison (FAO Subarea 27.8),” in Global Atlas of AIS-Based Fishing Activity - Challenges and Opportunities, eds M. Taconet, Kroodsma and J. A. Fernande (Rome: FAO).
Ferrà, C., Tassetti, A. N., Grati, F., Pellini, G., Polidori, P., Scarcella, G., et al. (2018). Mapping change in bottom trawling activity in the Mediterranean Sea through AIS data. Mar. Policy 94, 275–281. doi: 10.1016/j.marpol.2017.12.013
Ford, J. H., Peel, D., Kroodsma, D., Hardesty, B. D., Rosebrock, U., and Wilcox, C. (2018). Detecting suspicious activities at sea based on anomalies in automatic identification systems transmissions. PLoS One 13:e201640. doi: 10.1371/journal.pone.0201640
Gioia, C., Gaglione, S., and Angrisano, A. (2013). Performance assessment of aided global navigation satellite system for land navigation. IET Radar Sonar Navig. 7, 671–680. doi: 10.1049/iet-rsn.2012.0224
Goerlandt, F., and Kujala, P. (2011). Traffic simulation based ship collision probability modeling. Reliabil. Eng. Syst. Saf. 69, 91–107. doi: 10.1016/j.ress.2010.09.003
Guerriero, M., Coraluppi, S., and Carthel, C. (2010). “Analysis of AIS intermittency and vessel characterization using a hidden markov model,” in Proceedings of the Informatik 2010: Service Science - Neue Perspektiven für die Informatik, Beiträge der 40. Jahrestagung der Gesellschaft für Informatik e.V. (GI), Vol. 2, Leipzig.
Harati-Mokhtari, A., Wall, A., Brooks, P., and Wang, J. (2007). Automatic identification system (AIS): data reliability and human error implications. J. Navig. 60, 373–389. doi: 10.1017/s0373463307004298
Høye, G. K., Eriksen, T., Meland, B. J., and Narheim, B. T. (2008). Space-based AIS for global maritime traffic monitoring. Acta Astronaut. 62, 240–245. doi: 10.1016/j.actaastro.2007.07.001
Hsu, F.-C., Elvidge, C. D., Baugh, K., Zhizhin, M., Ghosh, T., Kroodsma, D., et al. (2019). Cross-Matching VIIRS boat detections with vessel monitoring system tracks in indonesia. Remote Sens. 11, 995. doi: 10.3390/rs11090995
ICES (2015). Report of the Working Group on Spatial Fisheries Data (WGSFD). ICES Scientific Reports. 1:52. Copenhagen: ICES.
James, M., Mendo, T., Jones, E. L., Orr, K., McKnight, A., and Thompson, J. (2018). AIS data to inform small scale fisheries management and marine spatial planning. Mar. Policy 91, 113–121. doi: 10.1016/j.marpol.2018.02.012
Keskin, Ç, Ordines, F., Ates, C., Moranta, J., and Massutí, E. (2014). Preliminary evaluation of landings and discards of the Turkish bottom trawl fishery in the northeastern Aegean Sea (eastern Mediterranean). Sci. Mar. 78, 213–225. doi: 10.3989/scimar.03942.30b
Kroodsma, D., Miller, N. A., Hochberg, T., Park, J., and Clavelle, T. (2019). “AIS-based methods for estimating fishing vessel activity and operations,” in Global Atlas of AIS-Based Fishing Activity - Challenges and Opportunities, eds M. Taconet, Kroodsma and J. A. Fernande (Rome: FAO).
Lambert, G. I., Jennings, S., Hiddink, J. G., Hintzen, N. T., Hinz, H., Kaiser, M. J., et al. (2012). Implications of using alternative methods of vessel monitoring system (VMS) data analysis to describe fishing activities and impacts. ICES J. Mar. Sci. 69, 682–693. doi: 10.1093/icesjms/fss018
Le Tixerant, M., Le Guyader, D., Gourmelon, F., and Queffelec, B. (2018). How can automatic identification system (AIS) data be used for maritime spatial planning? Ocean Coast. Manag. 166, 18–30. doi: 10.1016/j.ocecoaman.2018.05.005
Lucchetti, A. (2008). Comparison of diamond- and square-mesh codends in the hake (Merluccius merluccius L. 1758) trawl fishery of the Adriatic Sea (central Mediterranean). Sci. Mar. 72, 451–460. doi: 10.3989/scimar.2008.72n3451
Malarky, L., and Lowell, B. (2018). Avoiding Detection: Global Case Studies of Possible AIS Avoidance. Available online at: https://usa.oceana.org/sites/default/files/ais_onoff_report_final_5.pdf (accessed April, 2019).
Mazzarella, F., Vespe, M., Alessandrini, A., Tarchi, D., Aulicino, G., and Vollero, A. (2017). A novel anomaly detection approach to identify intentional AIS on-off switching. Expert Syst. Appl. 78, 110–123. doi: 10.1016/j.eswa.2017.02.011
Mazzarella, F., Vespe, M., Tarchi, D., Aulicino, G., and Vollero, A. (2016). “AIS reception characterisation for AIS on / off anomaly detection,” in Proceedings of the 19th International Conference on Information Fusion, Heidelberg.
McCauley, D. J., Woods, P., Sullivan, B., Bergman, B., Jablonicky, C., Roan, A., et al. (2016). Ending hide and seek at sea. Science 351, 1148–1150. doi: 10.1126/science.aad5686
Merchant, N. D., Witt, M. J., Blondel, P., Godley, B. J., and Smith, G. H. (2012). Assessing sound exposure from shipping in coastal waters using a single hydrophone and Automatic Identification System (AIS) data. Mar. Pollut. Bull. 64, 1320–1329. doi: 10.1016/j.marpolbul.2012.05.004
Merino, G., Coll, M., Granado, I., Gee, J., Kroodsma, D., Miller, N. A., et al. (2019). “FAO Area 37 - AIS-based fishing activity in the mediterranean and Black Sea,” in Global Atlas of AIS-Based Fishing Activity - Challenges and Opportunities, eds M. Taconet, Kroodsma and J. A. Fernande (Rome: FAO).
Natale, F., Gibin, M., Alessandrini, A., Vespe, M., and Paulrud, A. (2015). Mapping fishing effort through AIS data. PLoS One 10:e130746. doi: 10.1371/journal.pone.0130746
Nguyen, D., Simonin, M., Hajduch, G., Vadaine, R., Tedeschi, C., and Fablet, R. (2020). “Detection of abnormal vessel behaviors from AIS data using GeoTrackNet: from the laboratory to the ocean,” in Proceedings of the MBDW 2020 - 2nd Maritime Big Data Workshop part of MDM 2020 - 21st IEEE International Conference on Mobile Data Management, Jun 2020, Versailles. doi: 10.1109/MDM48529.2020.00061
Pallotta, G., Vespe, M., and Bryan, K. (2013a). “Traffic knowledge discovery from AIS data,” in Proceedings of the 16th International Conference on Information Fusion, Istanbul.
Pallotta, G., Vespe, M., and Bryan, K. (2013b). Vessel pattern knowledge discovery from AIS data: a framework for anomaly detection and route prediction. Entropy 15, 2218–2245. doi: 10.3390/e15062218
Papi, F., Aulicino, G., Vollero, A., Oliveri, F., Tarchi, D., Borghese, F., et al. (2014). Radiolocation and tracking of automatic identification system signals for maritime situational awareness. IET Radar Sonar Navig. 9, 568–580. doi: 10.1049/iet-rsn.2014.0292
Pelich, R., Longépé, N., Mercier, G., Hajduch, G., and Garello, R. (2014). AIS-based evaluation of target detectors and SAR sensors characteristics for maritime surveillance. IEEE J. Select. Top. Appl. Earth Observ. Remote Sens. 8, 3892–3901. doi: 10.1109/jstars.2014.2319195
Plass, S., Poehlmann, R., Hermenier, R., and Dammann, A. (2015). Global maritime surveillance by airliner-based AIS detection: preliminary analysis. J. Navig. 68, 1195–1209. doi: 10.1017/S0373463315000314
R Development Core Team and R Core Team (2018). R: A Language and Environment for Statistical Computing. Vienna: R Core Team.
Schwehr, K. D., and McGillivary, P. A. (2007). “Marine ship automatic identification system (AIS) for enhanced coastal security capabilities: an oil spill tracking application,” in Proceedings of the Oceans Conference Record (IEEE), Vancouver, BC. doi: 10.1109/OCEANS.2007.4449285
Shelmerdine, R. L. (2015). Teasing out the detail: how our understanding of marine AIS data can better inform industries, developments, and planning. Mar. Policy 54, 17–25. doi: 10.1016/j.marpol.2014.12.010
Shepperson, J. L., Hintzen, N. T., Szostek, C. L., Bell, E., Murray, L. G., and Kaiser, M. J. (2018). A comparison of VMS and AIS data: the effect of data coverage and vessel position recording frequency on estimates of fishing footprints. ICES J. Mar. Sci. 75, 988–998. doi: 10.1093/icesjms/fsx230
Svanberg, M., Santén, V., Hörteborn, A., Holm, H., and Finnsgård, C. (2019). AIS in maritime research. Mar. Policy 106:103520. doi: 10.1016/j.marpol.2019.103520
Tassetti, A. N., Ferrà, C., and Fabi, G. (2019). Rating the effectiveness of fishery-regulated areas with AIS data. Ocean Coast. Manag. 175, 90–97. doi: 10.1016/j.ocecoaman.2019.04.005
Tassetti, A. N., Ferrà, C., Scarcella, G., and Fabi, G. (2016). MEDSEA_CH5_Product_6 / Impact of Fisheries on the Bottom from AIS Data Combined with Habitat Vulnerability. New York, NY: EMODnet Medsea Checkpoint.
The European Parliament and the Council of the European Union (2008). Directive 2008/56/EC marine strategy framework directive. Off. J. Eur. Union 164, 19–40.
Vespe, M., Gibin, M., Alessandrini, A., Natale, F., Mazzarella, F., and Osio, G. C. (2016). Mapping EU fishing activities using ship tracking data. J. Maps 12(Suppl.1), 520–525. doi: 10.1080/17445647.2016.1195299
Winward (2015). AIS Data on the High Seas: An Analysis of the Magnitude and Implications of Growing Data Manipulation at Sea. San Diego, CA: Winward.
Keywords: Automatic Identification System (AIS), data gaps, Maritime Domain Awareness, Monitoring Control and Surveillance (MCS), fishing activity
Citation: Ferrà C, Tassetti AN, Armelloni EN, Galdelli A, Scarcella G and Fabi G (2020) Using AIS to Attempt a Quantitative Evaluation of Unobserved Trawling Activity in the Mediterranean Sea. Front. Mar. Sci. 7:580612. doi: 10.3389/fmars.2020.580612
Received: 06 July 2020; Accepted: 09 November 2020;
Published: 27 November 2020.
Edited by:
Maria Lourdes D. Palomares, Institute for the Oceans and Fisheries, University of British Columbia, CanadaReviewed by:
Fabio Pranovi, Ca’ Foscari University of Venice, ItalyAbbas Harati Mokhtari, Chabahar Maritime University, Iran
Copyright © 2020 Ferrà, Tassetti, Armelloni, Galdelli, Scarcella and Fabi. This is an open-access article distributed under the terms of the Creative Commons Attribution License (CC BY). The use, distribution or reproduction in other forums is permitted, provided the original author(s) and the copyright owner(s) are credited and that the original publication in this journal is cited, in accordance with accepted academic practice. No use, distribution or reproduction is permitted which does not comply with these terms.
*Correspondence: Anna Nora Tassetti, YW5uYW5vcmEudGFzc2V0dGlAY25yLml0