- 1Department of Engineering “Enzo Ferrari”, University of Modena and Reggio Emilia, Modena, Italy
- 2Consorzio Nazionale Interuniversitario per le Scienze del Mare (CoNISMa), Rome, Italy
- 3Biological, Geological and Environmental Sciences Department, University of Bologna, Bologna, Italy
- 4Centro Interdipartimentale di Ricerca Industriale Fonti Rinnovabili, Ambiente, Mare ed Energia, University of Bologna, Ravenna, Italy
- 5Life Sciences Department, University of Modena and Reggio Emilia, Modena, Italy
- 6Department of Topography, School of Rural and Surveying Engineering, National Technical University of Athens, Athens, Greece
- 7Department of History, Cultures and Civilizations, University of Bologna, Bologna, Italy
- 8Department of Mechanical, Energy and Management Engineering, University of Calabria, Rende, Italy
- 9Department of Life and Environmental Sciences, Polytechnic University of Marche, Ancona, Italy
- 10Stazione Zoologica Anton Dohrn, Naples, Italy
- 11Istituto di Scienza e Tecnologie dell’Informazione – Consiglio Nazionale delle Ricerche (ISTI-CNR), Pisa, Italy
- 12LIS UMR 7020, Aix-Marseille Université, Centre national de la recherche scientifique – École nationale Supérieure d’Architecture de Montpellier (CNRS-ENSAM), Université De Toulon, Domaine Universitaire de Saint-Jérôme, Marseille, France
- 13Department of Evolutionary Biology, Ecology and Environmental Sciences, Institut de Recerca de la Biodiversitat (IRBio), University of Barcelona, Barcelona, Spain
- 14Computer Vision and Robotics Institute, University of Girona, Girona, Spain
- 15Institute of Marine Sciences Consejo Superior de Investigaciones Científicas (CSIC), Barcelona, Spain
- 16Centro Interdisciplinar de Investigação Marinha e Ambiental (CIIMAR), Porto, Portugal
- 173DOM – 3D Optical Metrology Unit, Fondazione Bruno Kessler (FBK) – Bruno Kessler Foundation, Trento, Italy
- 18Underwater Bio-Cartography (UBICA) srl, Genova, Italy
- 19Habitats Edge Ltd., Norwich, United Kingdom
- 20Department of Industrial Engineering, University of Florence, Florence, Italy
- 21Interuniversity Center of Integrated Systems for the Marine Environment (ISME), Genova, Italy
- 22Department of Biological and Environmental Sciences and Technologies, University of Salento, Lecce, Italy
- 23Labomar, Universidade Federal do Ceará, Fortaleza, Brazil
- 24Civil Engineering and Geomatics Department, Cyprus University of Technology, Limassol, Cyprus
- 25School of Marine Sciences, University of Haifa, Haifa, Israel
- 26Department of Cultural Heritage, University of Bologna, Ravenna, Italy
Marine animal forests are benthic communities dominated by sessile suspension feeders (such as sponges, corals, and bivalves) able to generate three-dimensional (3D) frameworks with high structural complexity. The biodiversity and functioning of marine animal forests are strictly related to their 3D complexity. The present paper aims at providing new perspectives in underwater optical surveys. Starting from the current gaps in data collection and analysis that critically limit the study and conservation of marine animal forests, we discuss the main technological and methodological needs for the investigation of their 3D structural complexity at different spatial and temporal scales. Despite recent technological advances, it seems that several issues in data acquisition and processing need to be solved, to properly map the different benthic habitats in which marine animal forests are present, their health status and to measure structural complexity. Proper precision and accuracy should be chosen and assured in relation to the biological and ecological processes investigated. Besides, standardized methods and protocols are strictly necessary to meet the FAIR (findability, accessibility, interoperability, and reusability) data principles for the stewardship of habitat mapping and biodiversity, biomass, and growth data.
Introduction
Precise maps are nowadays easily available on the land surface, providing location, extent, and topography of terrestrial ecosystems. Interestingly, it is roughly calculated that only 5% of the oceans floor is accurately mapped at the ecological community level (Rossi and Orejas, 2019). Thus, a complete 3D and semantically enriched description of benthic habitats is required through a technologically improved and methodologically robust approach.
Marine animal forests are benthic communities dominated by sessile suspension feeders (such as sponges, gorgonians, scleractinian corals, and bivalves) able to generate 3D frameworks with high structural complexity (Rossi et al., 2017). These organisms act as habitat-forming species, since they “modulate the availability of resources (other than themselves) to other species by causing physical state changes in biotic or abiotic materials” (Jones et al., 1994). The complex structures resulting from their growth significantly enhance the heterogeneity in most environmental factors (such as light, current, food availability, or sediment suspension), providing habitats for other species, enhancing species diversity, and directly or indirectly participating in or promoting building of biogenic reefs (Rossi et al., 2017).
The overall bioconstruction rate of the biogenic reefs made or promoted by animal forests varies according to different prevailing species, but has often low values: for example, growth in height is estimated to range from less than a millimeter to few centimeters per year, depending on species and environmental conditions (Coma et al., 1998; Kružić and Benković, 2012; Ordoñez et al., 2019). Higher rates are typical of healthy tropical coral reefs dominated by branching corals, while lower rates occur in temperate and cold seas dominated by sponges, gorgonians or bivalves. Negative growth rates of biogenic structures may arise when biological and chemical-physical erosion processes dominate over the bioconstruction ones (Davidson et al., 2018). For example, in the Mediterranean biogenic reefs, the integrity of gorgonian populations is threatened by: (i) mechanical damage due to fishing lines and nets, anchorages, and recreational divers; (ii) suffocation by mucilaginous benthic aggregates; (iii) increased water turbidity and sedimentation rates as a result from poor land management (Ponti et al., 2018 and references therein); (iv) the invasion from non-indigenous and/or predator species (Maldonado et al., 2013; Righi et al., 2020). Moreover, climate change is one of the most concern threat since seawater warming, and higher frequency and severity of marine heatwaves have increased the mass mortalities of animal forests in both tropical and temperate seas (Garrabou et al., 2019). The vast decline of erect sponges and gorgonians in the Mediterranean Sea (Cerrano et al., 2000; Turicchia et al., 2018) is reducing considerably the structural complexity of these ecosystems, with important consequences for ecosystem biodiversity, functioning, and the services they provide (Verdura et al., 2019).
The study of marine animal forests down to the mesophotic zone is usually performed by scuba-diving surveys (Cerrano et al., 2019), using visual estimations, photo-sampling, and direct assessments of variables (e.g., colony size, percent cover, presence of necrosis/bleaching). Yet, accuracy of measurements and spatial replication are often not taken into consideration and some direct measures (e.g., annual growth rates) may still be based on destructive sampling (Peirano et al., 2001; Marschal et al., 2004). Since many invertebrates and their symbiotic algae contain fluorescent pigments, fluorometry and photographic image analysis are increasingly employed as a non-invasive proxy for organism health state and pathogenesis patterns (see Caldwell et al., 2017 for references), and to analyze their population dynamic; however, these studies are labor-intensive and require manual interpretation (Zweifler et al., 2017; Montero-Serra et al., 2019).
Technological developments have made optical and acoustic techniques practical to provide non-destructive measurement estimates of large sections of tropical coral reefs in 3D (House et al., 2018; Rossi et al., 2020), but their application in cold and temperate animal marine forests is still limited (Palma et al., 2018). Moreover additional efforts are still needed to obtain high definition reconstructions that range from aggregations to single organisms as well as subsequent reconstructions to allow tracing of a possible structural shift over time. Indeed, ranging from small to broad spatial scale of marine animal forests (Figure 1), the use of photogrammetry for georeferencing the samples and for spatial data acquisition is a promising tool to study many aspects of these communities and evaluate ecological key parameters, from organism health to the species interactions at the basis of bioconstruction and bioerosion processes. Within this viewpoint paper we mainly focus on optical techniques, in particular photogrammetry. Acoustic techniques are effective and widely used, but at present they do not guarantee to reach the same accuracy and resolution as photogrammetry (Czechowska et al., 2020). At the scale of single organisms, accurate reconstructions of 3D individual morphology may considerably enhance the capability for species recognition, as well as the accurate quantification of survival and growth rates under variable environmental conditions (Olinger et al., 2019). At the population level, morphological information about organisms may allow for a precise description of the population size structure, its dynamic variation through time (Montero-Serra et al., 2019) and its functioning (Ledoux et al., 2010). At the community level, the careful reconstruction of the framework built up by the structural and the associated species may allow to precisely describe the community structure in terms of species composition, abundance, and evenness. Finally, at the large scale of habitat or ecosystems, 3D georeferenced reconstructions may allow for the application of landscape indexes and descriptors to the analysis of benthic communities.
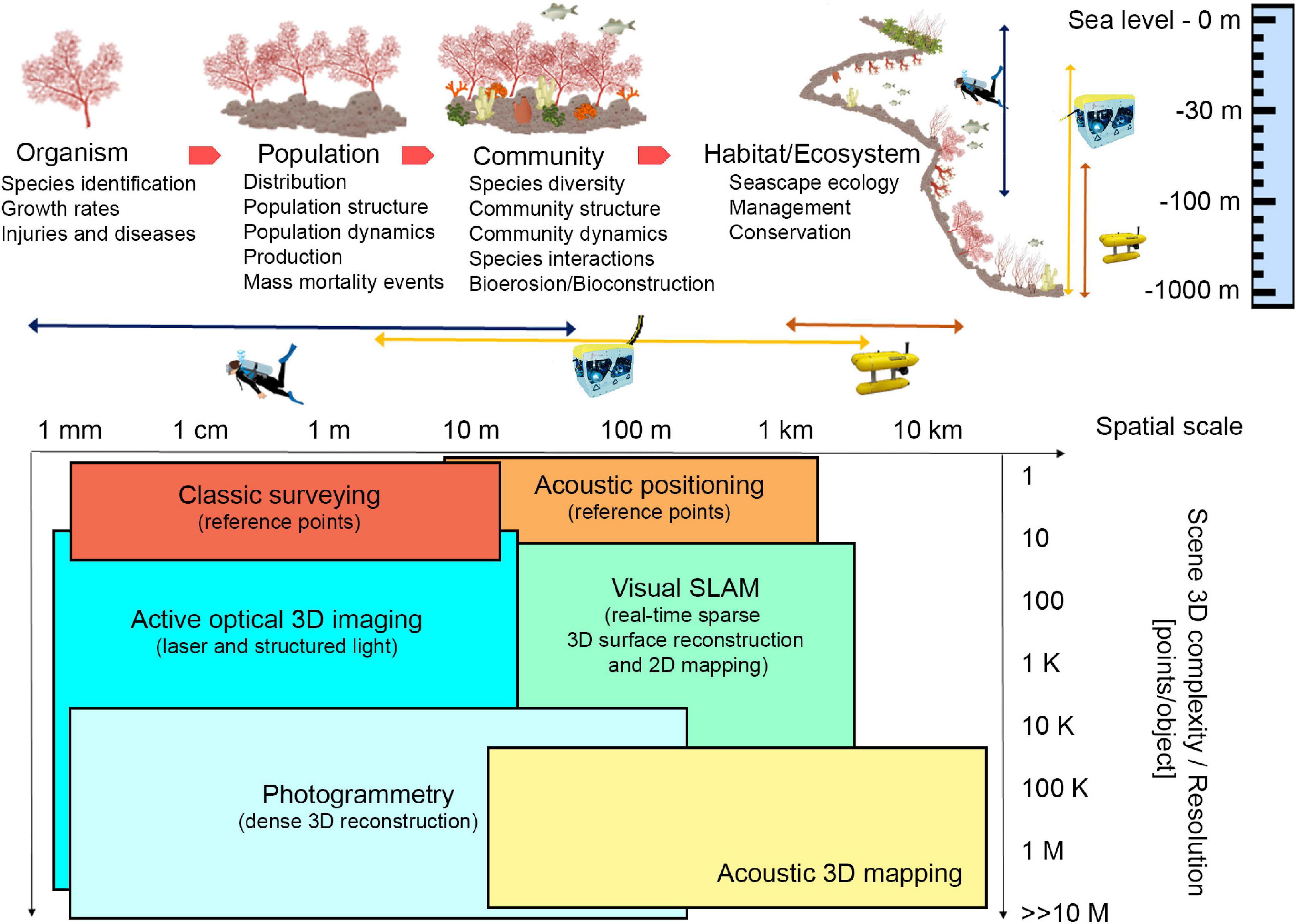
Figure 1. Ecological key aspects of marine animal forests and different technological approaches that can be applied at different spatial scales, depth, and 3D complexity of investigated area. In the table at the bottom, SLAM stands for Simultaneous Localization And Mapping. Illustrations modified from Montero-Serra (2018), and NOAA. Source: IAN Symbols Library, University of Maryland.
Animal forests represent a perfect natural lab to develop complex new methodologies, aiming at understanding long-term dynamics and evolutionary processes (Ledoux et al., 2020) shaping these high-biodiversity and structurally complex systems, and eventually the existence of recovery mechanisms that can enhance their resilience to increasing threats (Kersting and Linares, 2019).
Technologies and Methodologies
Data Acquisition
The underwater environment poses multiple challenges to 3D reconstruction-oriented explorations based on optical methodologies, such as low visibility, turbidity, light attenuation, and low data transfer rates. It is still challenging to acquire spatial data through photogrammetric methods at a wide area (wider than few square meters) at a few centimeters level of accuracy, which could be limiting for the study of large benthic species. New technologies and automation are required both in data acquisition and analysis to quantify the structural complexity of animal forests. Besides, survey planning and adaptation strategies that are tailored for optical and acoustic 2D/3D maps reconstruction, dynamically adapted to local conditions, and oriented toward the optimal coverage of a site, need to be explored.
Scuba divers are still widely employed for surveying tasks at accessible diving depths, in clear warm and turbid/cold waters complying with safety limitations (Piazza et al., 2018; Nocerino et al., 2019). However, remotely operated vehicles (ROVs) and autonomous underwater vehicles (AUVs) have become increasingly reliable tools and have led to inspections at depths and across spatio-temporal scales hostile both to divers and crewed vehicles (Figure 1; Gori et al., 2011; Shihavuddin et al., 2013; Montseny et al., 2019). In both cases, reference information is required to support spatial continuous methodologies and produce a georeferenced 3D model. The position of stable reference points, installed on the sea floor, or the position of sensors can be performed through classic survey technique or acoustic positioning, depending on the dimension and depth of the investigated site (Bruno et al., 2019; Rossi et al., 2020; Figure 1). Moreover, acoustic positioning systems, combined with underwater tablet, may allow divers to plan specific paths to be followed during the survey (Scaradozzi et al., 2018), thus optimizing the acquisition phase and guaranteeing repeatable and complete data. For deeper surveys, acoustic positioning and ROV/AUV navigation data may only provide approximate georeferencing information that may be too coarse for adequately scaling the 3D models when these are created from a single camera. In such cases, the use of laser calipers can solve this problem (Istenič et al., 2020).
Undoubtedly, automatic optical and acoustic imagery classification, as well as 2D mapping (Franchi et al., 2018), would speed up and enhance animal forests investigation allowing non-destructive visual surveys, measuring morphological variables, and paving the way to more specific 3D reconstruction-oriented explorations. Acoustic based techniques are well-suited in large areas of interest (from 10 m to 10 km), and acoustic 3D mapping is successfully employed to map a wide portion of the sea floor at various depths, but in case of organism biometry, higher accuracy and resolution techniques are required. These systems operate in the long-range acquisition and do not suffer from turbidity, but the resulting 3D reconstructions have low resolution and accuracy compared to the optical ones (Lagudi et al., 2016; Figure 1).
On the other hand, optical systems are well-suited for close-range acquisition (a few centimeters to tenths of meters). Active optical 3D imaging techniques project different patterns on the investigated area. The triangulation is computed by intersecting the laser beam that passes through a pixel lit up by the pattern, and the projected pattern itself (Palomer et al., 2019). These tools allow to obtain accurate and high-resolution 3D reconstructions, but results are influenced by visibility conditions and acquisition distance (Figure 1). Passive techniques (photogrammetry) are based on multiple views of a scene and take advantage of structure from motion (SfM) and Multi View Stereo (MVS) algorithms (Burns et al., 2015). Natural or artificial light can be used to illuminate the scene. The triangulation is done by intersecting the optic rays that pass through a pixel in each image where the same feature is automatically identified and allows the generation of an accurate, dense 3D reconstruction (Westoby et al., 2012). Underwater photogrammetry has long been used because of its flexibility and low cost, potentially providing high-resolution imagery in the underwater context under well-planned protocols of data collection (Nocerino et al., 2019). RGB sensors are the most used in underwater applications because they allow achieving measurable and photorealistic products. Other sensors may offer new opportunities to visualize the internal and external morphology of bioconstructions, such as multispectral acquisitions (Nocerino et al., 2017) and high-resolution x-ray computed tomography, which can be applied to collected samples (Farber et al., 2016). For shallow water applications, surface autonomous vehicles equipped with acoustic and optical sensors (Stanghellini et al., 2020), and low altitude Unmanned Aerial Vehicle (UAV)-based photogrammetry (Casella et al., 2017; Agrafiotis et al., 2020) can provide important information outlining bathymetric profile, filling the gap between satellite and underwater imagery. In the latter case, compensation for water refraction is required (Agrafiotis et al., 2020). In addition, underwater multispectral and hyperspectral imagery is an emerging technique for applications in shallow and deep-sea environments. It is a passive technique in shallow waters; instead, at high depth, when solar illumination is not available, an active light source is necessary. Anyway, this technique works properly at an acquisition distance of 1–5 m, because of the strong absorption caused by the water column (Liu et al., 2020).
Simultaneous localization and mapping (SLAM) methodologies have proved to be effective for underwater explorations and subsea metrology applications. SLAM relies on data integration from navigation sensors (e.g., global positioning system—GPS, inertial measurements unit—IMU) and remote sensing or perception (e.g., camera, lidar, sonar) systems; it comprises a wide range of algorithms designed to provide an autonomous vehicle with the ability to move in an uncharted environment while producing a map (or model) of the scene (Menna et al., 2019).
3D animal forest formations can occur in flat and gently sloping seabeds, or even in vertical slopes, often with crevices, terraces and overhangs. This poses major difficulties in surveying from the surface and requires complex acquisition paths from ROVs/AUVs/divers to collect images for proper 3D reconstruction. When utilizing underwater photogrammetry (or other optical methodologies) to survey marine animal forests, there is an important trade-off, as well as specific drawbacks and limitations that need to be considered. The spatial resolution of final maps depends on the quality of input images: underwater images suffer from wavelength-specific attenuation and scattering, which cause image degradation to increase significantly with distance. Thus, the distance to the object has a strong effect on the quality of the obtained images and consequently the 3D reconstruction. Additionally, in very shallow waters, rippling caustic are adversely affecting SfM and MVS methods, leading to less accurate matches (Agrafiotis et al., 2018) and causing issues in the SLAM based navigation of the ROVs and AUVs (Trabes and Jordan, 2017). A full reconstruction of the 3D complexity of underwater animal forests is challenging, occlusions can occur, and different view angles must be designed. Additionally, high data redundancy is required. Accurately designed acquisition paths (Vidal et al., 2020) and/or omnidirectional cameras (Bosch et al., 2019) could be used as well as forward-looking cameras and modular survey designs, adaptable in real-time. This requires serious navigation abilities from the AUV and is substantially unenforceable with human divers. New navigation technologies are needed, including visibility enhancement (Berman et al., 2020) for visual SLAM and obstacle avoidance to enable close-range photogrammetry of specific features. Neural networks might offer a solution to improve the AUV capabilities in terms of obstacle avoidance and real time decision-making. Computer simulations may help in training the neural networks and achieve such improvement. The photogrammetric approach has been successfully applied to hard corals, while gorgonians, soft corals and erect sponges pose important challenges due to their passive movement with water flow. However, a SfM technique has recently been tested on images from a gorgonian forest (Palma et al., 2018).
Image and Data Analysis
The collection of underwater imagery for the monitoring of marine animal forests became a widespread resource, leading to the accumulation of millions of images each year. However, the rate of image acquisition and the amount of the produced 2D orthophotos dramatically outpaces the human ability to extract information from them (Beijbom et al., 2015). The most commonly required data are the organisms’ size and abundance, the spatial distribution of populations on a broader landscape, and their evolution in relation to the changes of the underwater environment. Detailed information about 2D statistical methods for assessing these parameters, but also to evaluate growth and mortalities using ortho-mosaics are summarized in Edwards et al. (2017), Pedersen et al. (2019), and Sandin et al. (2020). These large data collections consisting of images can also be coupled with previous experimental or field work observations and quantifications in single species or communities, enabling a validation of ex situ analysis and a large scale investigation. For example: crossing the numbers of carbon input and output of a single organism together with its distribution, density, population structure and biomass in large spatial approaches, may be the key to understand the role of the organism as a carbon immobilizer. Terrestrial tools (used to study land communities acting as carbon immobilizers for example) in marine systems are in implementation thanks to the advances in 3D-image analysis and quantification. Acquired digital data (images and 3D models) are also perfectly suited for the sharing within the scientific community and the public. This could enhance a multidisciplinary and shared research, in accordance with FAIR principles.
Regardless of the biological purpose, spatial analysis, such as detecting changes in organisms, implies the need to segment target species with a high degree of accuracy. Manual 2D segmentation, e.g., a per-pixel classification, is an extremely time-consuming process that can be accelerated using deep learning-based methodologies (Pavoni et al., 2020).
The information on the depth axis is fundamental to evaluate the volumetric change caused by the structural growth or erosion. Additionally, many of the sessile organisms that constitute marine animal forests are hardly recognizable from a simple orthographic top-down view. The main prospect is to extend the semantic segmentation from images to height fields (2.5D) or 3D models. Open source segmentation software specifically targeted to marine species that handling 2.5D data have been already developed and distributed.
For example, TagLab1 enables assisted and automatic annotation, leveraging a human-in-the-loop approach to improve the accuracy of automatic predictions. Besides, it supports the comparison of multi-temporal surveys, automatically extracting useful historical information from segmented regions.
3D automatic semantic segmentation is the open challenge yet to face. As highlighted by a comprehensive survey paper (Han et al., 2019), available deep learning architectures might fail in producing segmentation that meets the standards of ecological analysis. Moreover, the limits of architectures working on point clouds, such as the PointNet++ (Qi et al., 2017) and the one by Çiçek et al. (2016), that is a variant of the U-Net architecture originally developed for biomedical data analysis, are not clearly assessed in this application field due to lack of benchmark dataset. Multi-modal learning approaches, combining oriented images, RGB and depth information, might help to fill this gap and solve the task (Dai and Nießner, 2018).
Discussion
In the context of animal forests mapping and 3D structural complexity analysis, the integration of different technologies could support multi-resolution and multi-scale surveying and monitoring. AUVs have the potential to efficiently investigate vast unexplored underwater regions, while ROVs allow for a higher resolution and more detailed mapping of smaller areas of interest that exceed the scuba-diving depth range.
Even if the scientific community is developing new approaches for improving “low” cost surveying and monitoring of the marine environment, their adoption is still not widespread since further testing and optimization of methodologies are needed. In addition, the limited funding for research, and the low interest of the general public in the seabed, slow these developments. Broad scale 3D reconstructions could improve the communication about the importance and diversity of marine environment and increase the public interest.
The main needs and gaps regarding the study of the 3D complexity of underwater animal forests are related to data collection and analysis (Figure 2), with concern to the improvement of diver-based or mobile robots (ROV/AUVs) methods in terms of positioning, guidance, and communication toward the surface. Adequate surveying performances of data collection platforms should be improved, depending on the level of robotic and further developments on real time decision capabilities (e.g., real time evaluation of the quality of the data gathered). Likewise, rigorous modeling of sensors, evaluation of metric accuracies and other methodological errors along with the standardization of data formats and analysis tools will be crucial focuses.
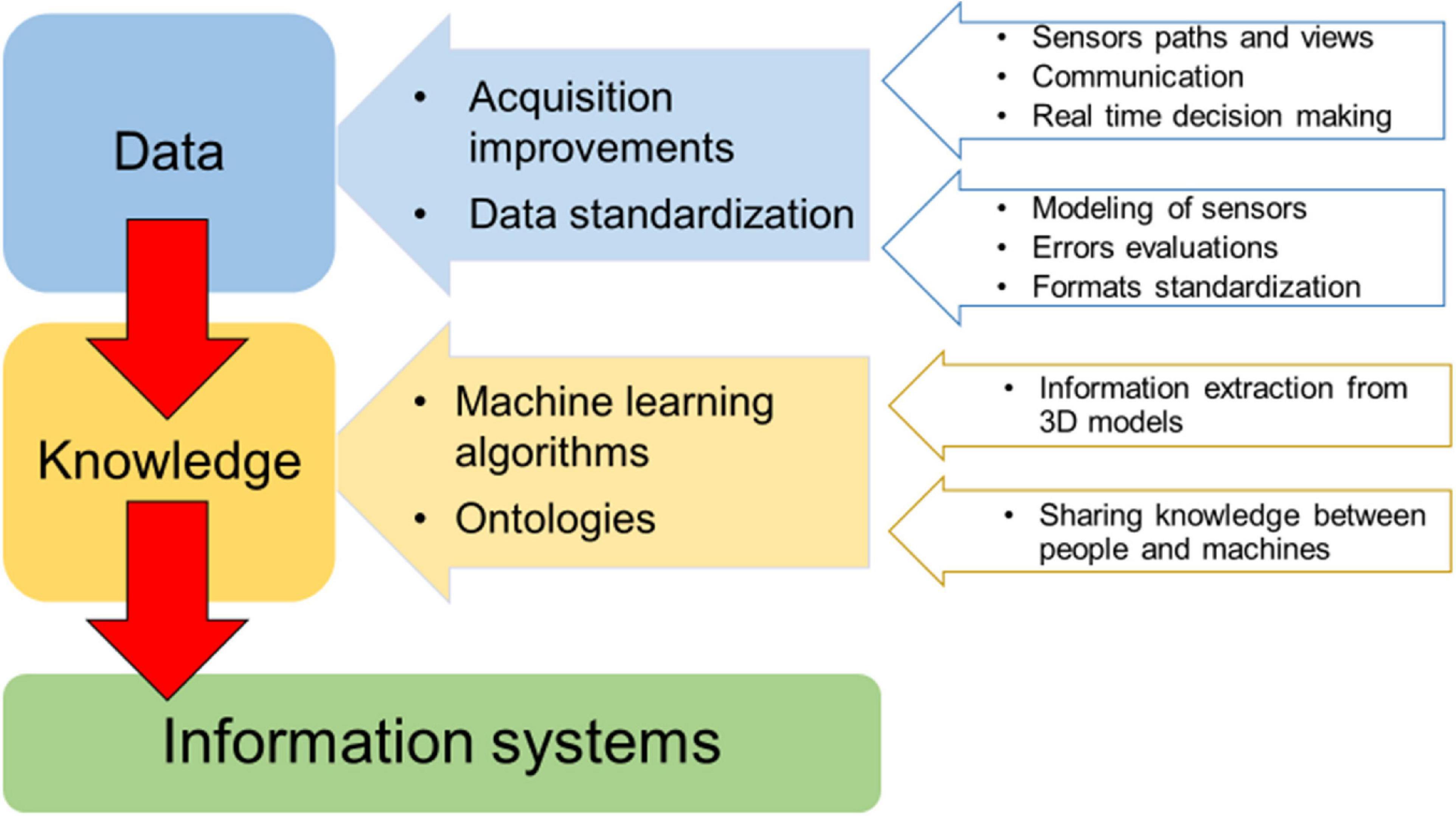
Figure 2. Main needs and gaps for 3D complexity investigations of underwater marine animal forests. The generation of exchangeable knowledge (information system) starts from standardized data.
The efficiency and quality of collected data, including multiple sensors, data acquisition at multiple scales, and data fusion from multiple platforms and times, need to be supported by the development of machine learning algorithms and methods for biodiversity classification/segmentation and 3D models definition of underwater changing objects. Indeed, benthic populations display 3D behaviors in time whose accurate analysis on the high-resolution 3D representation of these habitats is still beyond modern solutions based either on geometry processing or on deep learning. Fully automated solutions dramatically reduce the human effort in 2D/3D analysis; however, the accuracy of deep learning-based methodologies in the recognition/segmentation of benthic organisms is still lower than human experts, and the dense marine animal forests with a large 3D development pose even more difficulties in the discrimination of organisms. An efficient analysis tool must consider a human in the loop approach, leaving the experts the chance to edit the automatic results thus improving the overall accuracy. In addition, the analysis of large image sets can directly or indirectly benefit from public engagement, through citizen science programs, as was the case with plankton (Robinson et al., 2017). Citizen science data need to be carefully pre-analyzed in terms of accuracy and reliability to evaluate their effective use for mapping and 3D reconstruction applications.
Finally, the development of an information system to better retrieve and manage information on 3D complexity from collected data through time should be addressed. The integration of data belonging to various expeditions, methodologies and purposes is an important need too. The integration of all the existing habitat mapping data in a common and open information system could provide a more accurate, spatially representative assessment of habitat degradation and a proper design and management of restoration actions (Gerovasileiou et al., 2019). Ecological data are highly heterogeneous and complex to manage, therefore providing a shared and common understanding of data and processes will improve data accessibility and analysis. Ontologies (a knowledge graph-based Web tool, see Guarino et al., 2009) have a meaningful role in supporting knowledge sharing expectations. A common understanding facilitates communication between people and information systems (machines) and enhances the ability to search for information across different knowledge repositories, in light of meeting the FAIR (i.e., findability, accessibility, interoperability, and reusability) data principles (Wilkinson et al., 2016).
Data Availability Statement
All datasets generated for this study are included in the article/supplementary material, further inquiries can be directed to the corresponding author/s.
Author Contributions
AC, CCa, MPo, and PR contributed to the conception of the study. PR wrote the first draft of the manuscript and collected contributions and experiences of all the authors. AC, CCa, FMa, MPo, PR, RS, and SRi contributed to the revision of the first draft. All authors contributed to manuscript revision, read, and approved the submitted version.
Funding
This perspective manuscript is the result of the 3DSeaFor online workshop funded by EUROMARINE Call 2019 Foresight Workshops. This work was partially supported by an Italian Research Projects of National Interest (PRIN), funded by the Italian Ministry of University and Research: “Reef ReseArcH – Resistance and resilience of Adriatic mesophotic biogenic habitats to human and climate change threats” (Call 205; Prot. 2015J922E; 2017-2020) and by the TAO (Tecnologie per il monitorAggio cOstiero) project in the frame of the program POR-FESR (European Regional Development Fund, ERDF) 2014-2020 of the Emilia-Romagna Region. This work was partially supported by the U.S. National Science Foundation under Grant No. OCE 16-37396 (and earlier awards) as well as a generous gift from the Gordon and Betty Moore Foundation.
Conflict of Interest
MPa owns and was employed by Habitats Edge Ltd and UBICA srl.
The remaining authors declare that the research was conducted in the absence of any commercial or financial relationships that could be construed as a potential conflict of interest.
Acknowledgments
We are grateful to the reviewers for their valuable comments and suggestions.
Footnotes
References
Agrafiotis, P., Karantzalos, K., Georgopoulos, A., and Skarlatos, D. (2020). Correcting image refraction: towards accurate aerial image-based bathymetry mapping in shallow waters. Remote Sens. 12:322. doi: 10.3390/rs12020322
Agrafiotis, P., Skarlatos, D., Forbes, T., Poullis, C., Skamantzari, M., and Georgopoulos, A. (2018). Underwater photogrammetry in very shallow waters: main challenges and caustics effect removal. Int. Arch. Photogramm. Remote Sens. Spatial Inf. Sci. XLII-2, 15–22. doi: 10.5194/isprs-archives-XLII-2-15-2018
Beijbom, O., Edmunds, P. J., Roelfsema, C., Smith, J., Kline, D. I., Neal, B. P., et al. (2015). Towards automated annotation of benthic survey images: variability of human experts and operational modes of automation. PLoS One 10:e0130312. doi: 10.1371/journal.pone.0130312
Berman, D., Levy, D., Avidan, S., and Treibitz, T. (2020). Underwater single image color restoration using haze-lines and a new quantitative dataset. IEEE Trans. Pattern Anal. Mach. Intell. doi: 10.1109/tpami.2020.2977624
Bosch, J., Isteniè, K., Gracias, N., Garcia, R., and Ridao, P. (2019). Omnidirectional multi-camera video stitching using depth maps. IEEE J. Oceanic Eng. 99, 1–16. doi: 10.1109/JOE.2019.2924276
Bruno, F., Barbieri, L., Muzzupappa, M., Tusa, S., Fresina, A., Oliveri, F., et al. (2019). Enhancing learning and access to underwater cultural heritage through digital technologies: the case study of the “Cala Minnola” shipwreck site. Digit. Appl. Archaeol. Cult. Herit 13:e00103. doi: 10.1016/j.daach.2019.e00103
Burns, J. H. R., Delparte, D., Gates, R. D., and Takabayashi, M. (2015). Integrating structure-from-motion photogrammetry with geospatial software as a novel technique for quantifying 3D ecological characteristics of coral reefs. PeerJ 3:e1077. doi: 10.7717/peerj.1077
Caldwell, J. M., Ushijima, B., Couch, C. S., and Gates, R. D. (2017). Intra-colony disease progression induces fragmentation of coral fluorescent pigments. Sci. Rep. 7:14596. doi: 10.1038/s41598-017-15084-3
Casella, E., Collin, A., Harris, D., Ferse, S., Bejarano, S., Parravicini, V., et al. (2017). Mapping coral reefs using consumer-grade drones and structure from motion photogrammetry techniques. Coral Reefs 36, 269–275. doi: 10.1007/s00338-016-1522-0
Cerrano, C., Bastari, A., Calcinai, B., Di Camillo, C., Pica, D., Puce, S., et al. (2019). Temperate mesophotic ecosystems: gaps and perspectives of an emerging conservation challenge for the Mediterranean Sea. Eur. Zool. J. 86, 370–388. doi: 10.1080/24750263.2019.1677790
Cerrano, C., Bavestrello, G., Bianchi, C. N., Cattaneo-Vietti, R., Bava, S., Morganti, C., et al. (2000). A catastrophic mass-mortality episode of gorgonians and other organisms in the Ligurian Sea (North-western Mediterranean), summer 1999. Ecol. Lett. 3, 284–293. doi: 10.1046/j.1461-0248.2000.00152.x
Çiçek, Ö, Abdulkadir, A., Lienkamp, S. S., Brox, T., and Ronneberger, O. (2016). “3D U-Net: learning dense volumetric segmentation from sparse annotation,” in MICCAI Medical. Image Computing and Computer-Assisted Intervention, Vol. 424–432, eds S. Ourselin, W. S. Wells, M. R. Sabuncu, G. Unal, and L. Joskowicz (New York, NY: Springer). doi: 10.1007/978-3-319-46723-8_49
Coma, R., Ribes, M., Zabala, M., and Gili, J. M. (1998). Growth in a modular colonial marine invertebrate. Estuar. Coast. Shelf Sci. 47, 459–470. doi: 10.1006/ecss.1998.0375
Czechowska, K., Feldens, P., Tuya, F., de Esteban, M. C., Espino, F., Haroun, R., et al. (2020). Testing side-scan sonar and multibeam echosounder to study black coral gardens: a case study from Macaronesia. Remote Sens. 12:3244. doi: 10.3390/rs12193244
Dai, A., and Nießner, M. (2018). “3dmv: joint 3d-multi-view prediction for 3d semantic scene segmentation,” in Proceedings of the 15th European Conference, Munich, Germany, September 8–14, 2018, Munich, 452–468.
Davidson, T. M., Altieri, A. H., Ruiz, G. M., and Torchin, M. E. (2018). Bioerosion in a changing world: a conceptual framework. Ecol. Lett. 21, 422–438. doi: 10.1111/ele.12899
Edwards, C., Eynaud, Y., Williams, G. J., Pedersen, N. E., Zgliczyn-ski, B. J., Gleason, A. C. R., et al. (2017). Large-area imaging reveals biologically driven non-random spatial patterns of corals at a remote reef. Coral Reefs 36, 1291–1305. doi: 10.1007/s00338-017-1624-3
Farber, C., Titschack, J., Schonberg, C., Ehrig, K., Boos, K., Illerhaus, B., et al. (2016). Long-term macrobioerosion in the Mediterranean Sea assessed by micro-computed tomography. Biogeoscience 13, 3461–3474. doi: 10.5194/bg-13-3461-2016
Franchi, M., Ridolfi, A., and Zacchini, L. (2018). “A forward-looking sonar-based system for underwater mosaicing and acoustic odometry,” in Proceedings of the IEEE/OES Autonomous Underwater Vehicle Workshop (AUV), Porto. doi: 10.1109/auv.2018.8729795
Garrabou, J., Gómez-Gras, D., Ledoux, J. B., Linares, C., Bensoussan, N., López-Sendino, P., et al. (2019). Collaborative database to track mass mortality events in the Mediterranean Sea. Front. Mar. Sci. 6:707. doi: 10.3389/fmars.2019.00707
Gerovasileiou, V., Smith, C. J., Sevastou, K., Papadopoulou, N., Dailianis, T., Bekkby, T., et al. (2019). Habitat mapping in the European Seas-is it fit for purpose in the marine restoration agenda? Mar. Policy 106:103521. doi: 10.1016/j.marpol.2019.103521
Gori, A., Rossi, S., Berganzo, E., Pretus, J. L., Dale, M. R. T., and Gili, J. M. (2011). Spatial distribution patterns of the gorgonians Eunicella singularis, Paramuricea clavata, and Leptogorgia sarmentosa (Cap de Creus, northwestern Mediterranean Sea). Mar. Biol. 158, 143–158. doi: 10.1007/s00227-010-1548-8
Guarino, N., Oberle, D., and Staab, S. (2009). What is an Ontology? Handbook on Ontologies. Heidelberg: Springer, 1–17.
Han, X. F., Laga, H., and Bennamoun, M. (2019). Image-based 3d object reconstruction: state-of-the-art and trends in the deep learning era. IEEE Trans. Pattern. Anal. Mach. Intell, 1. doi: 10.1109/tpami.2019.2954885
House, J. E., Brambilla, V., Bidaut, L. M., Christie, A. P., Pizarro, O., Madin, J. S., et al. (2018). Moving to 3D: relationships between coral planar area, surface area and volume. PeerJ 6:e4280. doi: 10.7717/peerj.4280
Istenič, K., Gracias, N., Arnaubec, A., Escartín, J., and Garcia, R. (2020). Automatic scale estimation of structure from motion based 3D models using laser scalers in underwater scenarios. ISPRS J. Photogramm. Remote Sens. 159, 13–25. doi: 10.1016/j.isprsjprs.2019.10.007
Jones, C. G., Lawton, J. H., and Shachak, M. (1994). Organisms as ecosystem engineers. Oikos 69, 373–386. doi: 10.2307/3545850
Kersting, D. K., and Linares, C. (2019). Living evidence of a fossil survival strategy raises hope for warming-affected corals. Sci. Adv. 5:eaax2950. doi: 10.1126/sciadv.aax2950
Kružić, P. S., and Benković, L. (2012). The impact of seawater temperature on coral growth parameters of the colonial coral Cladocora caespitosa (Anthozoa, Scleractinia) in the eastern Adriatic Sea. Facies 58, 477–491. doi: 10.1007/s10347-012-0306-4
Lagudi, A., Bianco, G., Muzzupappa, M., and Bruno, F. (2016). An alignment method for the integration of underwater 3D data captured by a stereovision system and an acoustic camera. Sensors 16:536. doi: 10.3390/s16040536
Ledoux, J. B., Frias-Vidal, S., Montero-Serra, I., Antunes, A., Casado, B. C., Civit, S., et al. (2020). Assessing the impact of population decline on mating system in the overexploited mediterranean red coral. Aquatic Conserv. Mar. Freshw. Ecosyst. 30, 1149–1159. doi: 10.1002/aqc.3327
Ledoux, J. B., Garrabou, J., Bianchimani, O., Drap, P., Féral, J. P., and Aurelle, D. (2010). Fine-scale genetic structure and inferences on population biology in the threatened mediterranean red coral, Corallium rubrum. Mol. Ecol. 19, 4204–4216. doi: 10.1111/j.1365-294X.2010.04814.x
Liu, B., Liu, Z., Men, S., Li, Y., Ding, Z., He, J., et al. (2020). Underwater hyperspectral imaging technology and its applications for detecting and mapping the seafloor: a review. Sensors 20:4962. doi: 10.3390/s20174962
Maldonado, M., López-Acosta, M., Sánchez-Tocino, L., and Sitjà, C. (2013). The rare, giant gorgonian Ellisella paraplexauroides: demographics and conservation concerns. Mar. Ecol. Prog. Ser. 479, 127–141. doi: 10.3354/meps10172
Marschal, C., Garrabou, J., Harmelin, J. G., and Pichon, M. (2004). A new method for measuring growth and age in the precious mediterranean red coral Corallium rubrum (L.). Coral Reefs 23, 423–432. doi: 10.1007/s00338-004-0398-6
Menna, F., Nocerino, E., Nawaf, M. M., Seinturier, J., Torresani, A., Drap, P., et al. (2019). “Towards real-time underwater photogrammetry for subsea metrology applications,” in Proceedings of the OCEANS 2019, Marseille, 1–10. doi: 10.1109/OCEANSE.2019.8867285
Montero-Serra, I. (2018). Resilience of Long-Lived Mediterranean Gorgonians in a Changing World: Insights From Life History Theory and Quantitative Ecology. Ph.D. thesis, University of Barcelona, Barcelona.
Montero-Serra, I., Garrabou, J., Doak, D. F., Ledoux, J. B., and Linares, C. (2019). Marine protected areas enhance structural complexity but do not buffer the consequences of ocean warming for an overexploited precious coral. J. Appl. Ecol 56, 1063–1074. doi: 10.1111/1365-2664.13321
Montseny, M., Linares, C., Viladrich, N., Olariaga, A., Carreras, M., Palomeras, N., et al. (2019). First attempts towards the restoration of gorgonian populations on the Mediterranean continental shelf. Aquat. Conserv. Mar. Freshwater Ecosyst. 29, 1278–1284. doi: 10.1002/aqc.3118
Nocerino, E., Dubbini, M., Menna, F., Remondino, F., Gattelli, M., and Covi, D. (2017). Geometric calibration and radiometric correction of the MAIA multispectral camera. Int. Arch. Photogramm. Remote Sens. Spat. Inf. Sci. 42, 149–156. doi: 10.5194/isprs-archives-xlii-3-w3-149-2017
Nocerino, E., Neyer, F., Grün, A., Troyer, M., Menna, F., Brooks, A. J., et al. (2019). Comparison of diver-operated underwater photogrammetric systems for coral reef monitoring. Int. Arch. Photogramm. Remote Sens. Spat. Inf. Sci. 42, 143–150. doi: 10.5194/isprs-archives-xlii-2-w10-143-2019
Olinger, L. K., Scott, A. R., McMurray, S. E., and Pawlik, J. R. (2019). Growth estimates of Caribbean reef sponges on a shipwreck using 3D photogrammetry. Sci. Rep. 9:18398. doi: 10.1038/s41598-019-54681-2
Ordoñez, A., Wangpraseurt, D., Lyndby, N. H., Kühl, M., and Diaz-Pulido, G. (2019). Elevated CO2 leads to enhanced photosynthesis but decreased growth in early life stages of reef building coralline algae. Front. Mar.Sci. 5:495. doi: 10.3389/fmars.2018.00495
Palma, M., Casado, M., Pantaleo, U., Pavoni, G., Pica, D., and Cerrano, C. (2018). SfM-based method to assess gorgonian forests (Paramuricea clavata (Cnidaria. Octocorallia). Remote Sens. 10:1154. doi: 10.3390/rs10071154
Palomer, A., Ridao, P., Forest, J., and Ribas, D. (2019). Underwater laser scanner: Ray-based model and calibration. IEEE/ASME Trans. Mechatron. 24, 1986–1997. doi: 10.1109/tmech.2019.2929652
Pavoni, G., Corsini, M., Fiameni, G., Callieri, M., Edwards, C., and Cignoni, P. (2020). On improving the training of models for the semantic segmentation of benthic communities from orthographic imagery. Remote Sens. 12:3106. doi: 10.3390/rs12183106
Pedersen, N. E., Edwards, C. B., Eynaud, Y., Gleason, A. C. R., Smith, J. E., and Sandin, S. A. (2019). The influence of habitat and adults on the spatial distribution of juvenile corals. Ecography 42, 1703–1713. doi: 10.1111/ecog.04520
Peirano, A., Morri, C., Bianchi, C. N., and Rodolfo-Metalpa, R. (2001). Biomass, carbonate standing stock and production of the mediterranean coral Cladocora caespitosa (L.). Facies 44, 75–80. doi: 10.1007/bf02668168
Piazza, P., Cummings, V. J., Lohrer, D. M., Marini, S., Marriott, P., Menna, F., et al. (2018). Divers-operated underwater photogrammetry: applications in the study of antarctic benthos. Int. Arch. Photogramm. Remote Sens. Spat. Inf. Sci. 42, 885–892. doi: 10.5194/isprs-archives-XLII-2-885-2018
Ponti, M., Turicchia, E., Ferro, F., Cerrano, C., and Abbiati, M. (2018). The understorey of gorgonian forests in mesophotic temperate reefs. Aquat. Conserv. 28, 1153–1166. doi: 10.1002/aqc.2928
Qi, C. R., Yi, L., Su, H., and Guibas, L. J. (2017). “Pointnet++: deep hierarchical feature learning on point sets in a metric space,” in Proceedings of the 31st International Conference on Neural Information Processing Systems, 5099–5108.
Righi, S., Prevedelli, D., and Simonini, R. (2020). Ecology, distribution and expansion of a mediterranean native invader, the fireworm Hermodice carunculata (Annelida). Mediterr. Mar. Sci. 21, 575–591. doi: 10.12681/mms.23117
Robinson, K. L., Luo, J. Y., Sponaugle, S., Guigand, C., and Cowen, R. K. (2017). A tale of two crowds: public engagement in plankton classification. Front. Mar. Sci. 4:7. doi: 10.3389/fmars.2017.00082
Rossi, P., Castagnetti, C., Capra, A., Brooks, A. J., and Mancini, F. (2020). Detecting change in coral reef 3D structure using underwater photogrammetry: critical issues and performance metrics. Appl. Geomatics 12, 1–15. doi: 10.1007/s12518-019-00263-w
Rossi, S., Bramanti, L., Gori, A., and Orejas, C. (2017). Marine Animal Forests. The Ecology of Benthic Biodiversity Hotspots. Cham: Springer International Publishing.
Rossi, S., and Orejas, C. (2019). “Approaching cold-water corals to the society: novel ways to transfer knowledge,” in Proceding of the Mediterranean Cold-Water Corals: Past, Present and Future, (Cham: Springer), 473–480. doi: 10.1007/978-3-319-91608-8_39
Sandin, S. A., Edwards, C. B., Pedersen, N. E., Vid, P., Gaia, P., Esmeralda, A., et al. (2020). Considering the rates of growth in two taxa of coral across Pacific islands. Adv. Mar. Biol. 87, 167–191. doi: 10.1016/bs.amb.2020.08.006
Scaradozzi, D., Zingaretti, S., Ciuccoli, N., Costa, D., Palmieri, G., Bruno, F., et al. (2018). Lab4Dive mobile smart lab for augmented archaeological dives. IOP Conf. Ser. Mater. Sci. Eng. 364:012054. doi: 10.1088/1757-899x/364/1/012054
Shihavuddin, A. S. M., Gracias, N., Garcia, R., Gleason, A., and Ginter, B. (2013). Image-based coral reef classification and thematic mapping. Remote Sens. 5, 1809–1841. doi: 10.3390/rs5041809
Stanghellini, G., Del Bianco, F., and Gasperini, L. (2020). OpenSWAP, an open architecture, low cost class of autonomous surface vehicles for geophysical surveys in the shallow water environment. Remote Sens. 12:2575. doi: 10.3390/rs12162575
Trabes, E., and Jordan, M. A. (2017). A node-based method for SLAM navigation in self-similar underwater environments: a case study. Robotics 6:29. doi: 10.3390/robotics6040029
Turicchia, E., Abbiati, M., Sweet, M., and Ponti, M. (2018). Mass mortality hits gorgonian forests at montecristo island. Dis. Aquat. Org. 131, 79–85. doi: 10.3354/dao03284
Verdura, J., Linares, C., Ballesteros, E., Coma, R., Uriz, M. J., Bensoussan, N., et al. (2019). Biodiversity loss in a mediterranean ecosystem due to an extreme warming event unveil the role of an engineering gorgonian species. Sci. Rep. 9:5911. doi: 10.1038/s41598-019-41929-0
Vidal, E., Palomeras, N., Istenič, K., Gracias, N., and Carreras, M. (2020). Multisensor online 3D view planning for autonomous underwater exploration. J. Field Rob. 37, 1–25. doi: 10.1002/rob.21951
Westoby, M. J., Brasington, J., Glasser, N. F., Hambrey, M. J., and Reynolds, J. M. (2012). Structure-from-motion’ photogrammetry: a low-cost, effective tool for geoscience applications. Geomorphology 179, 300–314. doi: 10.1016/j.geomorph.2012.08.021
Wilkinson, M. D., Dumontier, M., Aalbersberg, I. J., Appleton, G., Axton, M., Baak, A., et al. (2016). The FAIR guiding principles for scientific data management and stewardship. Sci. Data 3:160018. doi: 10.1038/sdata.2016.18
Keywords: biodiversity, 3D monitoring, semantic segmentation, underwater photogrammetry, biogenic reefs conservation
Citation: Rossi P, Ponti M, Righi S, Castagnetti C, Simonini R, Mancini F, Agrafiotis P, Bassani L, Bruno F, Cerrano C, Cignoni P, Corsini M, Drap P, Dubbini M, Garrabou J, Gori A, Gracias N, Ledoux J-B, Linares C, Mantas TP, Menna F, Nocerino E, Palma M, Pavoni G, Ridolfi A, Rossi S, Skarlatos D, Treibitz T, Turicchia E, Yuval M and Capra A (2021) Needs and Gaps in Optical Underwater Technologies and Methods for the Investigation of Marine Animal Forest 3D-Structural Complexity. Front. Mar. Sci. 8:591292. doi: 10.3389/fmars.2021.591292
Received: 04 August 2020; Accepted: 10 February 2021;
Published: 05 March 2021.
Edited by:
Christos Dimitrios Arvanitidis, Hellenic Centre for Marine Research, GreeceReviewed by:
Thanos Dailianis, Hellenic Centre for Marine Research, GreeceJulie Bremner, Centre for Environment, Fisheries and Aquaculture Science (CEFAS), United Kingdom
Copyright © 2021 Rossi, Ponti, Righi, Castagnetti, Simonini, Mancini, Agrafiotis, Bassani, Bruno, Cerrano, Cignoni, Corsini, Drap, Dubbini, Garrabou, Gori, Gracias, Ledoux, Linares, Mantas, Menna, Nocerino, Palma, Pavoni, Ridolfi, Rossi, Skarlatos, Treibitz, Turicchia, Yuval and Capra. This is an open-access article distributed under the terms of the Creative Commons Attribution License (CC BY). The use, distribution or reproduction in other forums is permitted, provided the original author(s) and the copyright owner(s) are credited and that the original publication in this journal is cited, in accordance with accepted academic practice. No use, distribution or reproduction is permitted which does not comply with these terms.
*Correspondence: Paolo Rossi, cGFvbG8ucm9zc2lAdW5pbW9yZS5pdA==