- 1Department of Integrative Biology, University of Windsor, Windsor, ON, Canada
- 2Department of Biology, University of New Mexico, Albuquerque, NM, United States
- 3School of Marine Science and Policy, University of Delaware, Lewes, DE, United States
- 4Fisheries Resources Division, Southwest Fisheries Science Center, National Marine Fisheries Service (NMFS), National Oceanic and Atmospheric Administration (NOAA), La Jolla, CA, United States
Blue sharks (Prionace glauca) are globally distributed, large-bodied pelagic sharks that make extensive migrations throughout their range. In the North Pacific, mark-recapture studies have shown trans-Pacific migrations, but knowledge gaps in migration frequency hinder understanding of regional connectivity and assessments of regional demography for stock assessments. Here, we use oceanographic gradients of stable isotope ratios (i.e., regional isoscapes) to determine exchange rates of blue sharks between the East and West North Pacific Ocean (EPO and WPO). We generated regional δ13C and δ15N distributions for blue sharks from published values in the North Pacific (n = 180; both sexes, juveniles and adults combined). Discriminant analysis suggested low trans-Pacific exchange, categorizing all western (100%) and most eastern (95.3%) blue sharks as resident to their sampling region, with isotopic niche overlap of WPO and EPO highly distinct (0.01–5.6% overlap). Limited trans-Pacific movements suggest that other mechanisms maintain genetic mixing of the North Pacific blue shark population. Potential finer scale movement structure was indicated by isotopic differences in sub-regions of the eastern and western Pacific, though application of mixing models are currently limited by aberrantly low blue shark δ13C values across studies. Our results suggest that blue shark population dynamics may be effectively assessed on a regional basis (i.e., WPO and EPO). We recommend further studies to provide size- and sex-specific movement patterns based on empirical isotopic values with large sample sizes from targeted regions. Strategically applied stable isotope approaches can continue to elucidate migration dynamics of mobile marine predators, complementing traditional approaches to fisheries biology and ecology.
Introduction
Blue sharks (Prionace glauca) are large-bodied, highly migratory sharks with a global distribution extending throughout temperate and subtropical waters (Nakano and Stevens, 2008; Coelho et al., 2018). Blue shark populations have declined broadly, with high longline bycatch and mortality rates due to extensive overlap with commercial fisheries across much of their global range (Queiroz et al., 2016, 2019). This is true for the North Atlantic (50–79% decline over 30 years; ICCAT, 2015) and Mediterranean (96.5–99.8% decline since the early 19th century; Ferretti et al., 2008) populations as a result of both targeted fisheries (i.e., for fins, meat, squalene) and bycatch (Clarke et al., 2006a, b; Cardeñosa et al., 2020), though the North Pacific population has recently been assessed as not overfished (ISC, 2017). While studies have challenged model-based inferences of shark population declines (Burgess et al., 2005), blue sharks are the major bycatch species in high-seas fisheries regionally (McKinnell and Seki, 1998; Francis et al., 2001) and perhaps globally (Clarke et al., 2006b; Campana et al., 2009). Limited genetic structure has been observed across populations sampled from disparate oceanic regions (King et al., 2015; Taguchi et al., 2015; Veríssimo et al., 2017; Bailleul et al., 2018), though regional populations are managed separately. Mechanisms for high genetic homogeneity across global blue shark populations remain mostly speculative, mainly because studies are lacking in adequate sample sizes and sufficient demographic coverage to allow for robust conclusions to be made (Veríssimo et al., 2017). This limitation spans not only genetic information, but also a robust understanding of other aspects of the species’ life history, particularly movements and migrations in relation to proposed mating and parturition grounds.
In the North Pacific Ocean, data suggest that mature blue sharks migrate to a latitudinal band spanning ∼20–30°N for mating during the early summer months, with pupping typically occurring the following summer after a ∼12-month gestation. Gravid females are believed to move further north to parturition grounds located in sub-arctic waters between 35 and 45°N (Nakano, 1994). Males and females typically segregate spatially prior to mating events, but some overlap between immature and mature individuals of opposite sexes can occur (Maxwell et al., 2019). Broadscale movements in the Pacific have been described with conventional and electronic tagging studies (Musyl et al., 2011; Maxwell et al., 2019), and although some blue sharks exhibit long-distance migrations over thousands of kilometers (Maxwell et al., 2019), most tracking data shows predominately latitudinal movements. However, recent conventional mark-recapture information has shown that some individuals migrate across the northern Pacific Ocean from the western Pacific Ocean (WPO) into the eastern Pacific Ocean (EPO) and vice versa (Sippel et al., 2011), a behavior that also has been observed in the North Atlantic (Howey et al., 2017). The extent of connectivity between North Pacific sub-populations (e.g., WPO and EPO), however, remains unquantified. For example, it remains unclear whether genetic mixing and homogeneity (King et al., 2015) is maintained by trans-Pacific migrations of juveniles or adults, or by some other mechanism such as pupping and recruitment dynamics. Thus, the proportion of blue sharks performing trans-Pacific migrations warrants investigation, as quantifying movement connectivity can clarify regional source-sink dynamics, inform spatial scales of management, and help explain the mechanisms that facilitate genetic homogeneity.
While electronic and conventional tagging approaches have provided useful information on blue shark movement and migration dynamics, studies are limited by high cost (for electronic tagging) and often require high sample sizes and protracted study duration to yield necessary ecological information (Sequeira et al., 2019). Consequently, complementary approaches are required for rapid assessment of blue shark migration and movement connectivity. Intrinsic chemical tracers measured in animal tissues, such as stable isotope (SI) ratios, are useful for reconstructing prior animal migrations (Graham et al., 2010; Trueman et al., 2019; Madigan et al., 2021). Stable isotope analysis (SIA)-based movement studies utilize the distinct isotopic composition of prey baselines (i.e., regional isoscapes) across oceanic sub-regions, driven by local oceanographic and biogeochemical regimes (McMahon et al., 2013; Brault et al., 2018; Espinasse et al., 2020). In the North Pacific Ocean, pelagic prey fields in the EPO and WPO are isotopically distinct, particularly for nitrogen isotope ratios (δ15N) (Matsubayashi et al., 2020). In the EPO, upwelling of nutrient-rich water in the California Current promotes larger nitrate metabolizing primary producers such as diatoms, which creates a 15N-enriched isotopic composition of regional prey (Altabet et al., 1999; Montoya, 2007; Madigan et al., 2012a, 2017). Comparatively, WPO waters are nutrient-poor, which promotes dominance of nitrogen-fixing picophytoplankton at the base of the food web and lower (i.e., 15N depleted) δ15N composition of regional prey pools (Takai et al., 2007; Fujinami et al., 2018; Ohshimo et al., 2019).
Knowledge of regional isotopic baseline variation can be combined with measured predator stable isotope ratios and tissue-specific incorporation rates to identify individuals that have recently migrated from one system into another (Madigan et al., 2021; Shipley et al., 2021). Tissues of recent migrants will reflect an isotopic mix of prey baselines from the prior and current regions, provided that sampling has occurred prior to the consumer reaching isotopic steady-state with prey from their current region (Heady and Moore, 2013; Moore et al., 2016; Madigan et al., 2021). Residents are then defined as those individuals that are at isotopic steady-state with prey baselines in their current region (Madigan et al., 2014). This technique has been applied to characterize trans-Pacific migrations of Pacific bluefin tuna (Thunnus orientalis), using machine learning algorithms to define migrants vs. residents, and thus predicting the extent of mixing between WPO and EPO populations (Madigan et al., 2014). More recently, SIA approaches have been used to determine movement transitions across systems spanning marine, brackish, and freshwater habitats (Moore et al., 2016; Shipley et al., 2021), and have proven to be a robust and insightful approach for clarifying aspects of animal migration.
In the current study, we use regional δ13C and δ15N values from sampled sharks and prey to quantify potential trans-Pacific exchange rates of blue sharks between the WPO and EPO. We use multiple analytical methods with isotopic data to examine the extent of regional residency and foraging connectivity between oceanic sub-regions. This approach provides a tracer-based assessment of habitat use that is complementary to traditional tagging approaches and provides a framework than can be adopted across other study taxa and ecosystems. Inferred movements from isotopic signatures aid in constraining the extent of blue shark movements in the North Pacific basin, clarify the migratory mechanisms that may drive a mixed genetic stock, and can inform appropriate multi-national or regional management strategies for North Pacific blue sharks.
Materials and Methods
Data Compilation
Blue Shark SI Data
A literature search was performed to obtain all SI data to-date for blue sharks in the North Pacific. Based on conventional tagging data and available SI data, we categorized North Pacific data into the WPO and EPO. From all studies, mean, reported error (SD or SE), and minimum and maximum values (when reported) of blue shark δ13C and δ15N values were tabulated. The literature search showed studies in four discrete regions of the EPO, so EPO blue sharks were further categorized into these four EPO sub-regions: Northern California Current (NCC), Southern California Bight (SCB), southern Baja (SBaja), and the eastern Tropical Pacific (ETP) (see section “Results”). We only used studies that accounted for lipid and urea effects on δ13C and δ15N through either chemical extraction or arithmetic correction and DI rinsing. While we recognize that different treatments can affect isotopic values, lipid content in most shark species is low (Hussey et al., 2012) and correction for urea in available data was not feasible. As a result, values were used as reported in published studies. Blue shark δ13C and δ15N values were estimated to represent their past foraging behavior for ∼0.5–1.5 years before sampling, based on published turnover rate estimates (Madigan et al., 2012b) and blue shark body size ranges across published studies (Thomas and Crowther, 2015; Vander Zanden et al., 2015).
Estimating Population-Wide Blue Shark SI Values
We used an iterative bootstrapping approach to resample each published δ13C and15N data distribution (n = 5 studies) to generate estimates of population-wide blue shark SI values for each ocean region. Blue shark δ13C and15N estimates were bootstrapped (1000×) by randomly sampling from mean (±SD) values published for each study region. This resulted in 1 × 103 estimates for the WPO (n = 1 study) and 4 × 103 estimates for the EPO (n = 4 studies), with separate, region-specific resampled populations for sub-regions of the EPO.
Prey SI Data
We searched the literature for published studies reporting SI values of epi- and mesopelagic prey in the WPO and EPO, based on known regional blue shark diets of epi- and mesopelagic forage fish, cephalopods, and crustaceans (Preti et al., 2012; Fujinami et al., 2018). Across relevant studies, prey-specific δ13C and δ15N means (±SD) were tabulated for subsequent analyses. As with blue sharks above, prey values were also obtained and regional means (±SD) calculated for the four defined sub-regions of the EPO (NCC, SCB, SBaja, and ETP). As with blue shark data, we only used studies that accounted for lipid effects on δ13C through chemical extraction or arithmetic correction, and values were used as they were reported in published studies.
Prey δ13C and δ15N values were used to generate regional mean diet δ13C and δ15N values in the EPO and WPO for subsequent analyses (see sections “Discriminant Analysis” and Isotopic Mixing Models”). For prey data in each region, we accounted for associated error of each prey mean δ13C and δ15N values by bootstrapping 1000 values from reported means (±SD) for each prey item. We then randomly selected from these prey distributions (1000×) to generate a mean blue shark diet value for the WPO (n = 145 prey species) and EPO (n = 75 prey species). Each prey item was weighed equally based on demonstrably broad and opportunistic blue shark diets. This resulted in 1 × 103 estimated mean (±SD) diet δ15N values for both the WPO and EPO. We also used the above approach to generate mean δ13C and δ15N diet estimates in the four EPO sub-regions. Combining data from studies that span different time periods accepts potential temporal variation in isotopic baselines and consumers, but given the observed distinction between isotope values between the WPO and EPO (see section “Results”), we deemed it unlikely that this would significantly impact overall results.
Data Analyses
Three analytical approaches were applied to blue shark and prey SI data to characterize blue shark movements (explained in detail below). Isotopic niche overlap of blue shark values was used to obtain general, quantitative metrics of likely blue shark exchange between sub-regions. We then used discriminant analysis to explicitly categorize individual shark isotope values as indicative of prior use of WPO or EPO waters. Finally, mixing models were used to estimate blue shark use of region-specific prey based on the isotopic composition of sharks and prey in each region.
Regional Variability and Overlap of Blue Shark SI Values
For the WPO and four EPO sub-regions (NCC, SCB, SBaja, ETP), integrated δ13C and δ15N niche areas (Bayesian ellipses: SEAB) were determined, generating ellipses for each sub-region that incorporate 40% of the available data (Jackson et al., 2011). Isotopic overlap between each sub-region was then inferred using a Bayesian approach implemented in the R package “nicheROVER.” Overlap estimates were generated from 1000 posterior draws based on 95% probabilistic niche regions (Swanson et al., 2015).
Diet-Dependent Diet-Tissue Discrimination Factors (DTDFs)
Discriminant analysis and isotopic mixing models required application of blue shark δ15N and δ13C diet-tissue discrimination factors (DTDFs). Because trophic discrimination factors can vary due to a suite of environmental and physiological processes (Hussey et al., 2012; Shipley and Matich, 2020) and have been shown to co-vary with diet δ15N and δ13C values (Caut et al., 2009; Hussey et al., 2014), we calculated diet-specific DTDFs for blue sharks within each region. For each calculated prey mean, we calculated mean DTDFs from algorithms reported in two studies: (1) Caut et al. (2009):
and (2) Hussey et al. (2014):
where Δ15N and Δ13C represent diet-derived DTDFs for δ15N and δ13C, and δ15Ndiet and δ13Cdiet represent mean diet δ15N and δ13C values, respectively. For each regional diet δ15N mean, we calculated two DTDF values following Eqs 1, 2 and used the mean of both DTDF values (i.e., estimated DTDF values from equations) for applied diet-based blue shark Δ15N and Δ13C values for each sub-region.
Discriminant Analysis
Only δ15N values were used in discriminant analysis due to non-differentiation of WPO and EPO δ13C values (see sections “Results” and “Discussion”), following methods in Madigan et al. (2014). We generated training data for discriminant analysis using regional prey means and regional prey-based DTDFs. Specifically, regional DTDFs were added to regional prey means to generate 1 × 103 estimated blue shark δ15N values for the WPO, EPO, and EPO sub-regions. We then applied these training data to discriminant analysis of WPO and EPO blue shark δ15N data to classify individual sharks as recent migrants or long-term (≥1 year, based on ectotherm isotopic turnover rates; Thomas and Crowther, 2015; Vander Zanden et al., 2015) residents to the region in which they were sampled. Discriminant analysis reported an error value for the classification of unknown data, which estimates the percentage of individuals that were classified incorrectly (Klecka, 1980).
Isotopic Mixing Models
We applied isotopic mixing models to assess their efficacy for describing foraging across sub-regions in both the EPO and WPO. Bayesian isotope mixing models (R package “simmr”; Parnell, 2020) were used to estimate regional prey inputs to EPO and WPO blue sharks, providing estimates of regional connectivity. For the WPO, mixing models were run for a single blue shark population (due to n = 1 study in the WPO) and regional prey were based on reported prey values for four WPO sub-regions (Eastern Japan, Kuroshio-Oyashio, Sea of Japan, and offshore Taiwan) in Madigan et al. (2015), with additional prey data from Ohshimo et al. (2019). For the EPO, mixing models were run for regional blue shark populations and the pooled EPO population; regional prey endmembers were the NCC, SCB, SBaja, ETP, and the WPO. Endmember values and DTDFs were based on compilations of regional prey fields (Table 1).
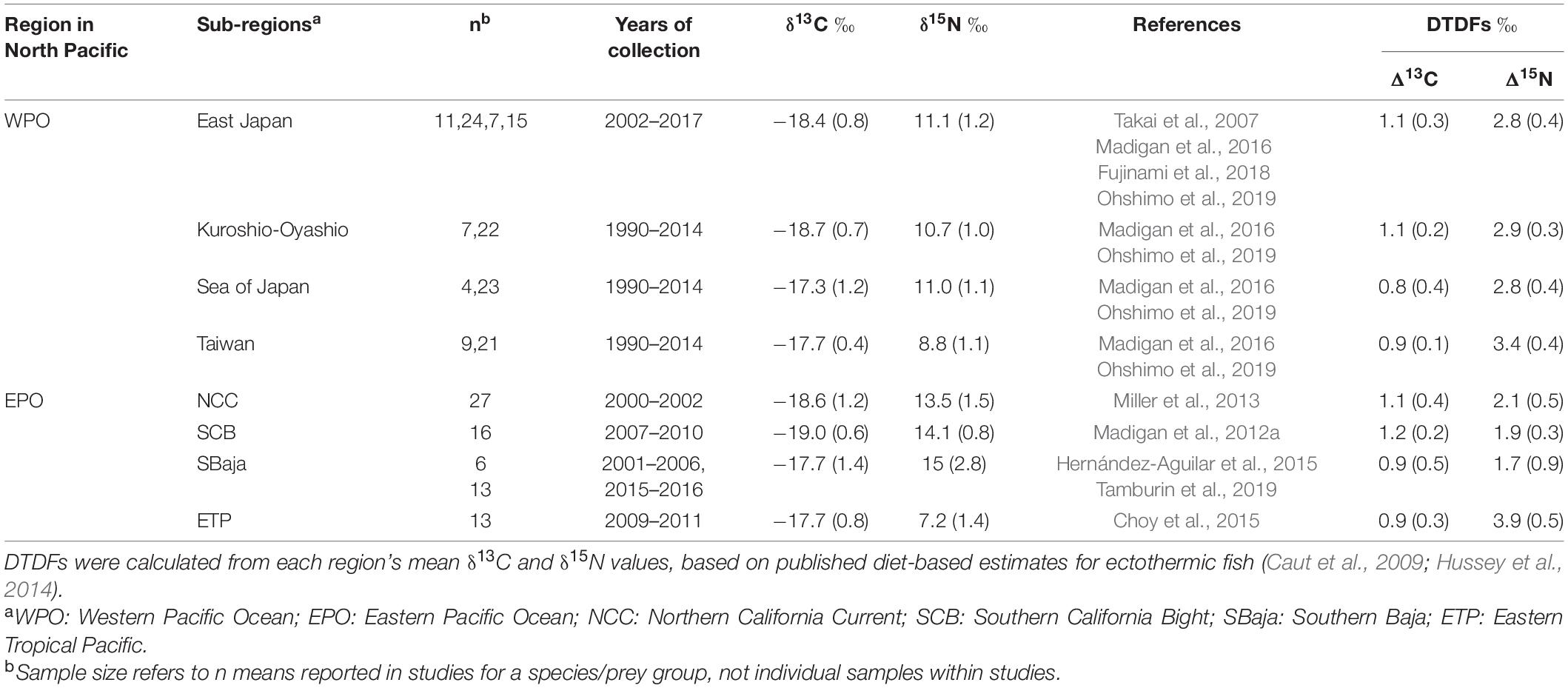
Table 1. δ13C and δ15N values (mean ± 1 SD) of published prey items by North Pacific Ocean sub-region, and calculated diet-dependent diet-tissue discrimination factors (DTDFs; Δ13C and Δ15N) estimated for blue sharks (Prionace glauca).
We estimated the accuracy of regional endmembers (n = 4 for WPO, n = 5 for EPO) by simulating 10,000 prey mixing polygons (Smith et al., 2013) and quantifying the probability of each individual consumer falling outside of the 95% prey mixing space. A relatively high proportion of blue sharks had >95% probability of falling outside the mixing space due to low δ13C values (see section “Results”), as has been observed in other studies (Rabehagasoa et al., 2012; Li et al., 2014; Kiszka et al., 2015). We consequently evaluated the two-isotope mixing model results to assess the effects of potential bias toward low δ13C diet inputs. We also performed a single isotope (δ15N only) mixing model for both the WPO and EPO to compare to the two-isotope model results. The probable contributions of regional prey endmembers to blue shark diet were inferred from 10,000 model iterations, with a burn-in period of 1000 and a thinning interval of 100. Model convergence was evaluated based on inspection of Gelman-Rubin diagnostics, where values for each parameter should equal ∼1.0 (Phillips et al., 2014; Parnell, 2020).
Results
Data Compilation
Blue Shark SI Data
We obtained δ13C and δ15N values for blue shark muscle from one WPO study (Fujinami et al., 2018) (total n = 120 individuals) and four EPO studies (Miller et al., 2010; Madigan et al., 2012a; Li et al., 2014; Hernández-Aguilar et al., 2015) (total n = 60) from different regions of the EPO (NCC, SCB, SBaja, and ETP) (Figure 1). All studies included male and female sharks across juvenile and adult size ranges, though reporting of size and sex metadata did not allow for this information to be associated with individual δ13C and δ15N values.
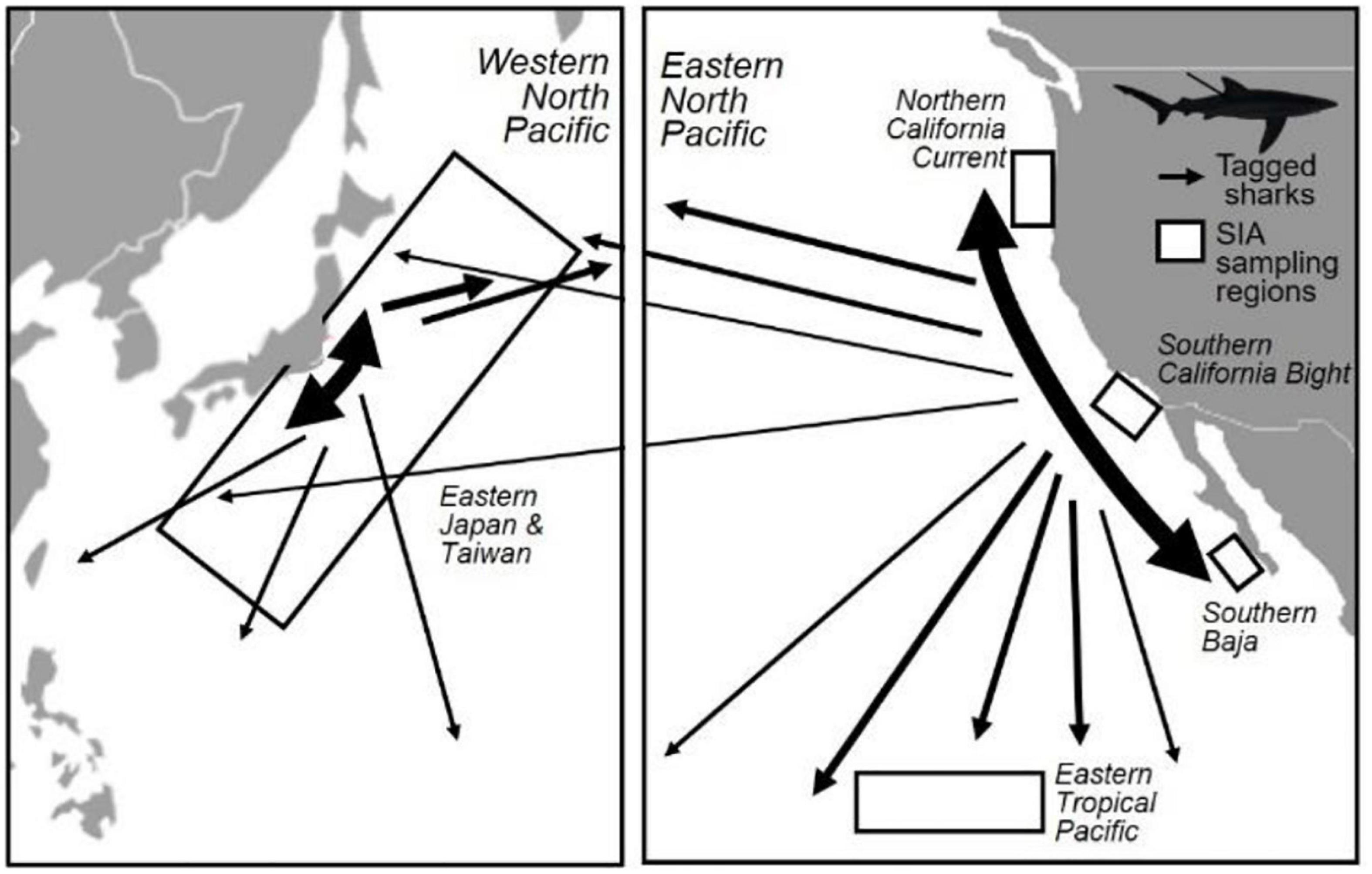
Figure 1. Map of summarized blue shark (Prionace glauca) movements and sampling locations for isotopic studies in the North Pacific Ocean. Movements (black arrows) are based on conventional tagging data in the western and eastern Pacific Ocean (WPO and EPO), with arrow size scaled to relative proportion of observed movements. Boxes show regions of blue shark tissue sampling for stable isotope analysis (SIA). Tagging data are summarized from Sippel et al. (2011); SIA sampling locations from Miller et al. (2010), Madigan et al. (2012a), Li et al. (2014), Hernández-Aguilar et al. (2015), Fujinami et al. (2018).
We assumed that published blue shark δ13C and δ15N values were generally normally distributed, based on reporting of mean ± SD or SE. Blue shark δ13C values showed high overlap between the WPO and EPO, with the majority of data in both regions falling between −20.0 and −17.0‰ (Figure 2); this was also observed in WPO and EPO prey that were used to calculate isoscape “baselines” for both regions (see Figure 2 and section below). In contrast, blue shark δ15N values were distinct between the WPO and EPO (Figure 2).
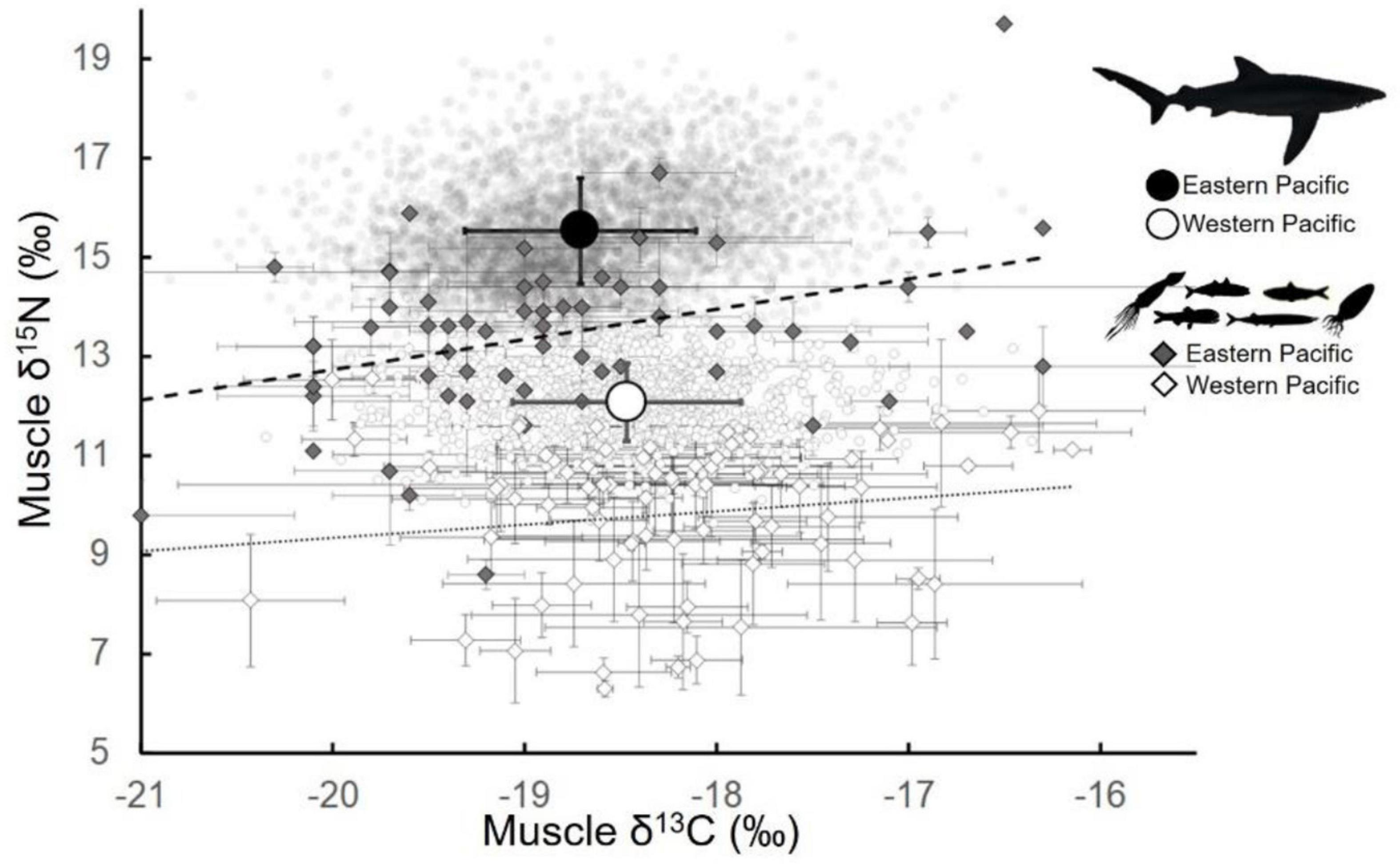
Figure 2. Stable isotope values of blue sharks (Prionace glauca) and prey in the western and eastern North Pacific Ocean. Blue shark values in the eastern (dark circles) and western (open circles) were estimated from published distributions of blue shark values in each ocean basin, with means shown (large filled and open circles; ± SD). Prey data (diamonds; species mean ± SD) were selected based on general prey groups (e.g., mesopelagic squids, forage fish) reported in published diet studies, and include epi- and mesopelagic forage fish, squids, and crustaceans. Lines represent linear fits to eastern (dashed line) and western (solid line) prey data.
Consistent with previous studies, mean blue shark δ15N values were consistently lower in the WPO (12.1‰; Fujinami et al., 2018) than in the EPO (14.7, 15.2, 15.8, and 16.5‰; Miller et al., 2010; Madigan et al., 2012a; Li et al., 2014; Hernández-Aguilar et al., 2015; Figures 2, 3). The range of δ15N values was relatively small in all regions, with minimal outliers (i.e., high values in WPO, low values in EPO) reported in any studies. Based on studies that reported δ15N minima and maxima, these values were (WPO) 10.3–14.0‰ and (EPO) 13.8–18.8‰.
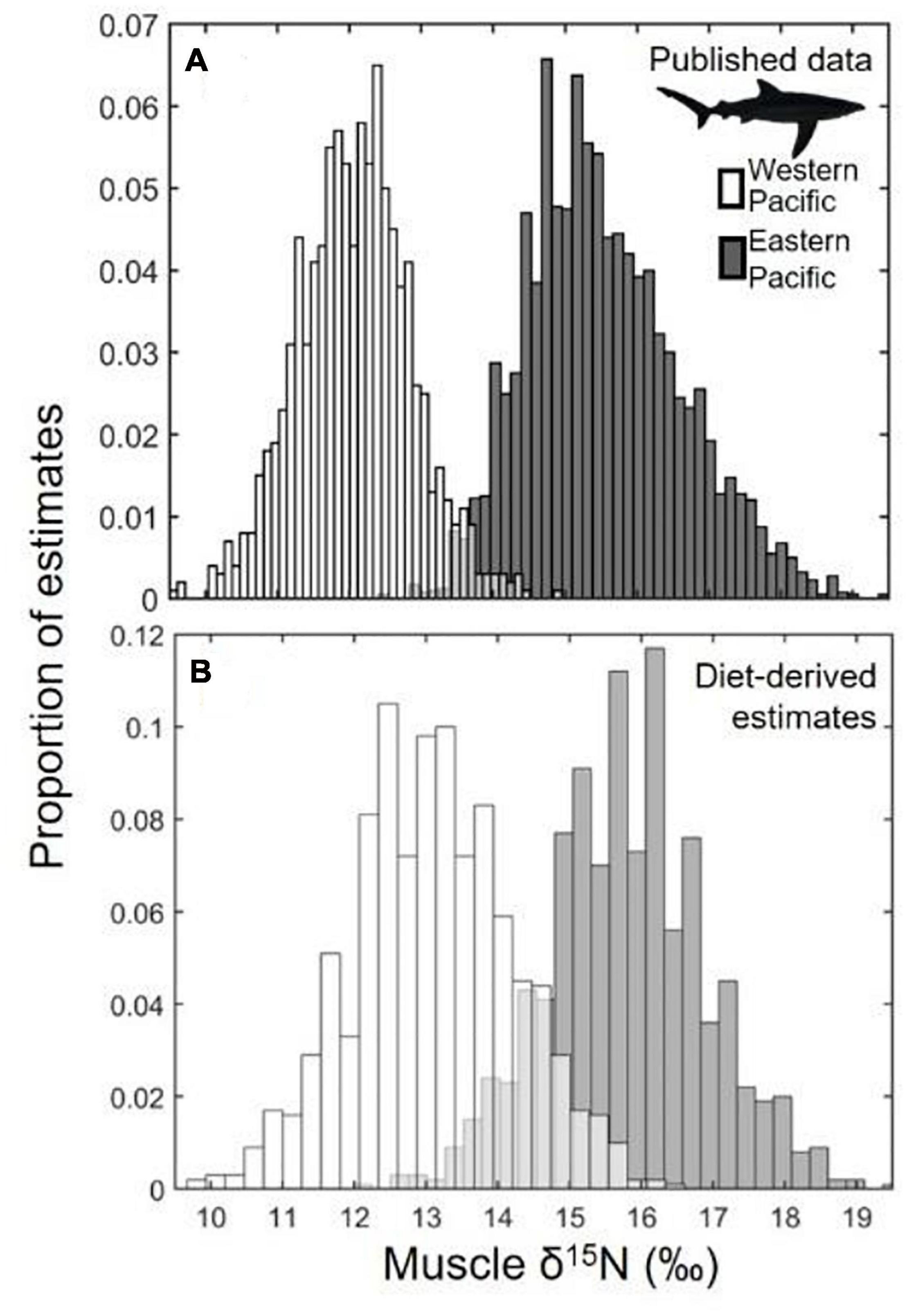
Figure 3. Distributions of bootstrapped blue shark (Prionace glauca) δ15N values from the North Pacific Ocean. (A) Blue shark muscle δ15N values were taken from the published literature from sampling regions off Japan and Taiwan (western Pacific Ocean; open bars) and the California Current and the Eastern Tropical Pacific (eastern Pacific Ocean; gray bars). (B) Blue shark diet-derived δ15N values were estimated by calculating regional prey baselines, based on published data, and adding resampled blue shark diet-dependent DTDF estimates for each region.
Prey SI Data
We obtained δ13C and δ15N data for WPO prey (muscle tissue) from five studies (Takai et al., 2007; Madigan et al., 2016; Ohshimo et al., 2016, 2019; Fujinami et al., 2018) for a total of 145 prey species, and obtained data for EPO prey from five studies (Madigan et al., 2012a; Miller et al., 2013; Choy et al., 2015; Hernández-Aguilar et al., 2015; Tamburin et al., 2019) for a total of 75 prey species. Prey species included the most commonly observed species in WPO and EPO blue shark diet (Preti et al., 2012; Hernández-Aguilar et al., 2015; Fujinami et al., 2018), including forage fish (anchovy Engraulis spp., sardine Sardinops spp., scombrids), squids (Ommastrephidae, Gonatidae, Oegopsidae), and crustaceans (red crab Pleuroncodes planipes), as well as other epi- and mesopelagic fish, squids, and crustaceans that have also been observed in blue shark diet (Preti et al., 2012; Hernández-Aguilar et al., 2015; Fujinami et al., 2018).
As with WPO and EPO blue shark data, prey δ13C values showed high overlap while prey δ15N values were discrete between the WPO and EPO (Table 1 and Figure 2). For this reason, we used only δ15N values for discriminant analysis, consistent with previous studies that used δ15N to quantify exchange rates of WPO and EPO Pacific bluefin tuna (Madigan et al., 2017; Tawa et al., 2017). Overall WPO prey δ13C values were −18.2 ± 0.9‰; range −21.5 to −16.1‰, and δ15N values were 9.9 ± 1.6‰; range 4.3–12.6‰. EPO prey SI values were (δ13C) −18.6 ± 1.0‰; range −20.1 to −16.3‰, and (δ15N) 14.1 ± 1.0‰; range 12.3–15.9‰. Prey δ13C and δ15N differences were variable across sub-regions (Table 1). Total diet estimates based on bootstrapped prey SI values were highly variable across regions (Table 1). Diet estimates were used in discriminant analysis and mixing models (below).
Data Analyses
Regional Variability and Overlap of Blue Shark SI Values
Across the five sub-regions, isotopic variability measured in terms of niche space (which was uniform across SEA and SEAB estimates) was greatest for individuals captured in the ETP (1.8‰2), and lowest in the NCC (0.6‰2). SCB, SBaja, and WPO individuals exhibited intermediate variability (1.1‰2–1.2‰2, Table 2 and Figure 4).
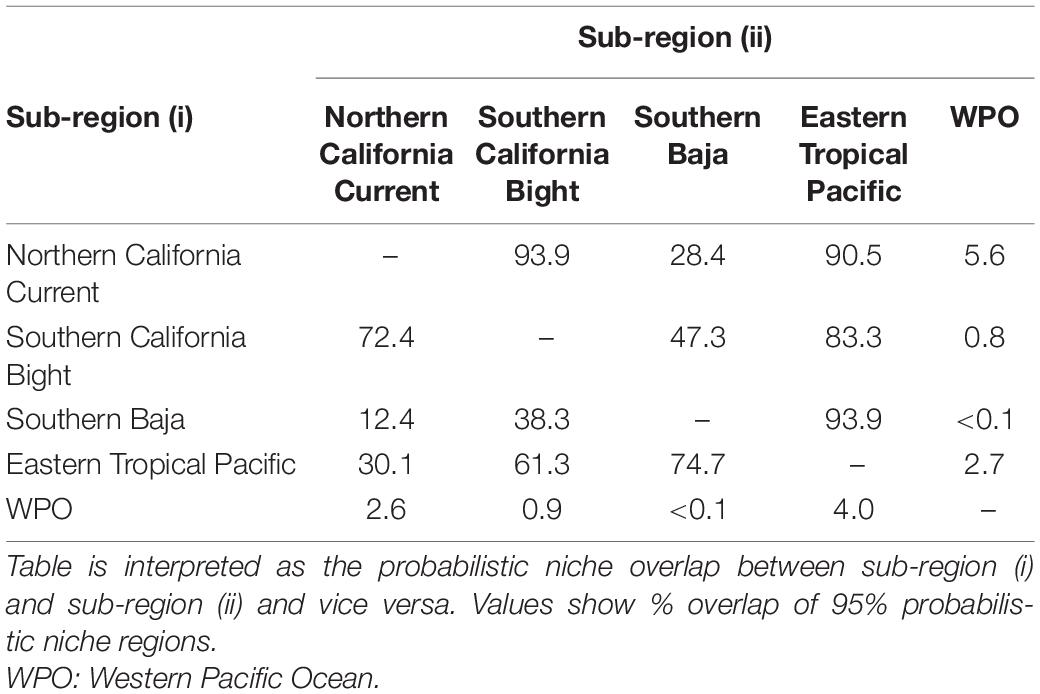
Table 2. Bayesian inferred probabilistic niche overlap of blue sharks (Prionace glauca) captured from five sub-regions.
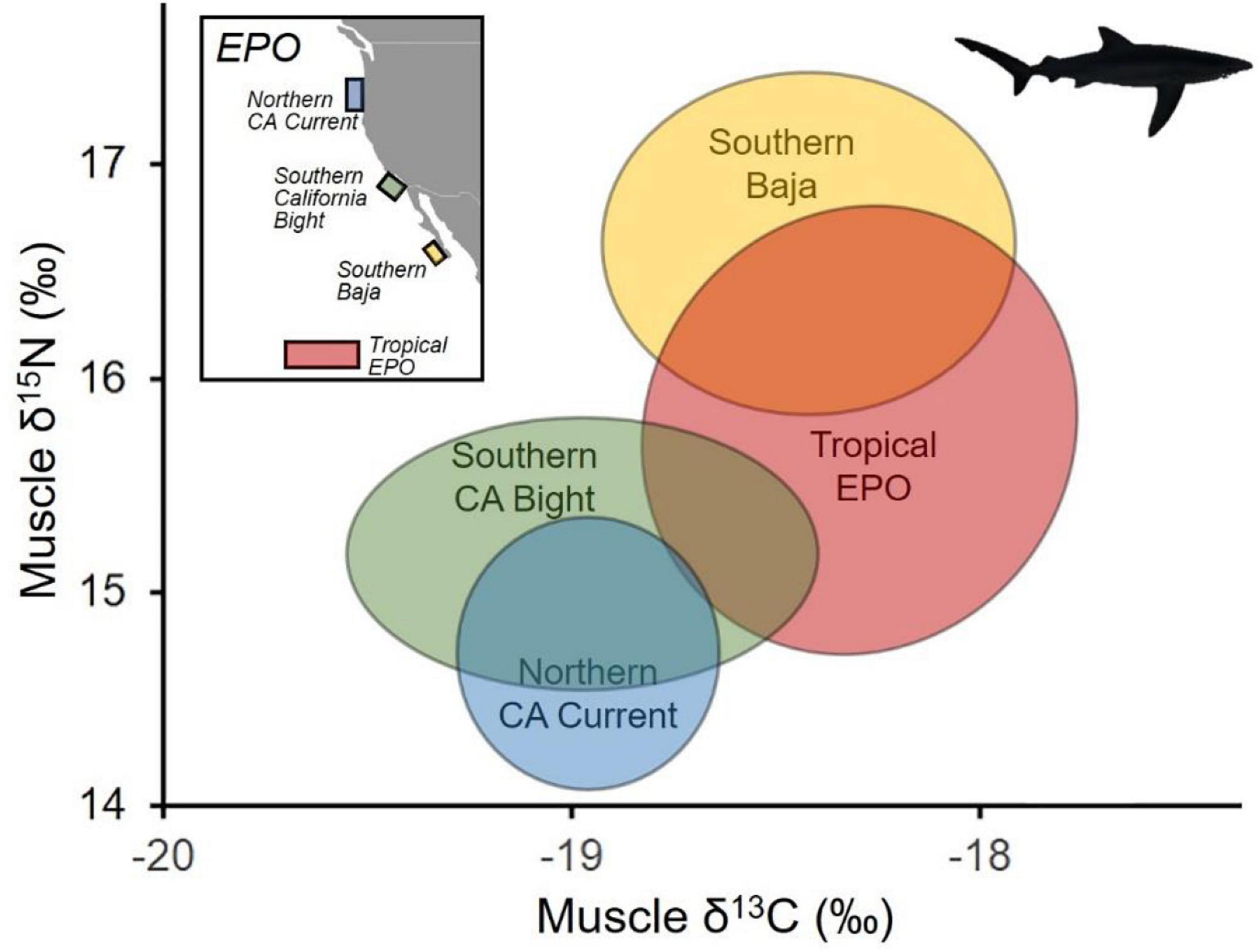
Figure 4. Differences in isotopic niches of blue shark (Prionace glauca) across discrete regions within the eastern North Pacific Ocean. Map (upper left) shows regions where blue sharks were sampled and subsequently analyzed for δ13C and δ15N values. Ellipses represent 95% of blue shark δ13C and δ15N values following Swanson et al. (2015).
Isotopic niche overlap was extremely low between all possible combinations of EPO sub-regions and WPO blue sharks (<6%, Table 2 and Figure 4). Overlap between sub-regions of the EPO was substantial, but highly variable, with overlap estimates ranging from 12 to 94% (Table 2 and Figure 4). Blue sharks sampled in the NCC and SCB generally overlapped significantly with the isotopic niches of those from the ETP (>70%), but there was less overlap with SBaja (<48%). SBaja blue sharks overlapped minimally with NCC and SCB sharks (<39%), but overlapped highly with ETP sharks (94%, Table 2 and Figures 4, 5). Overlap of ETP sharks was relatively high with SCB and SBaja sharks (61 and 75%, respectively), but lower with the NCC (30%, Table 2 and Figures 4, 5). Overall, bilateral isotopic niche overlap (see Table 2) suggested that the highest level of WPO↔EPO connectivity was in the NCC (12%, 28%; Table 2), and the lowest level of WPO↔EPO connectivity in SBaja (<1%; Table 2 and Figure 5). Within the EPO, the highest δ15N-inferred connectivity of blue sharks was between the NCC and SCB (72%, 94%; Table 2) and SBaja and the ETP (94%, 75%; Table 2), and the lowest connectivity between SBaja and the NCC (12%, 28%; Table 2 and Figure 5).
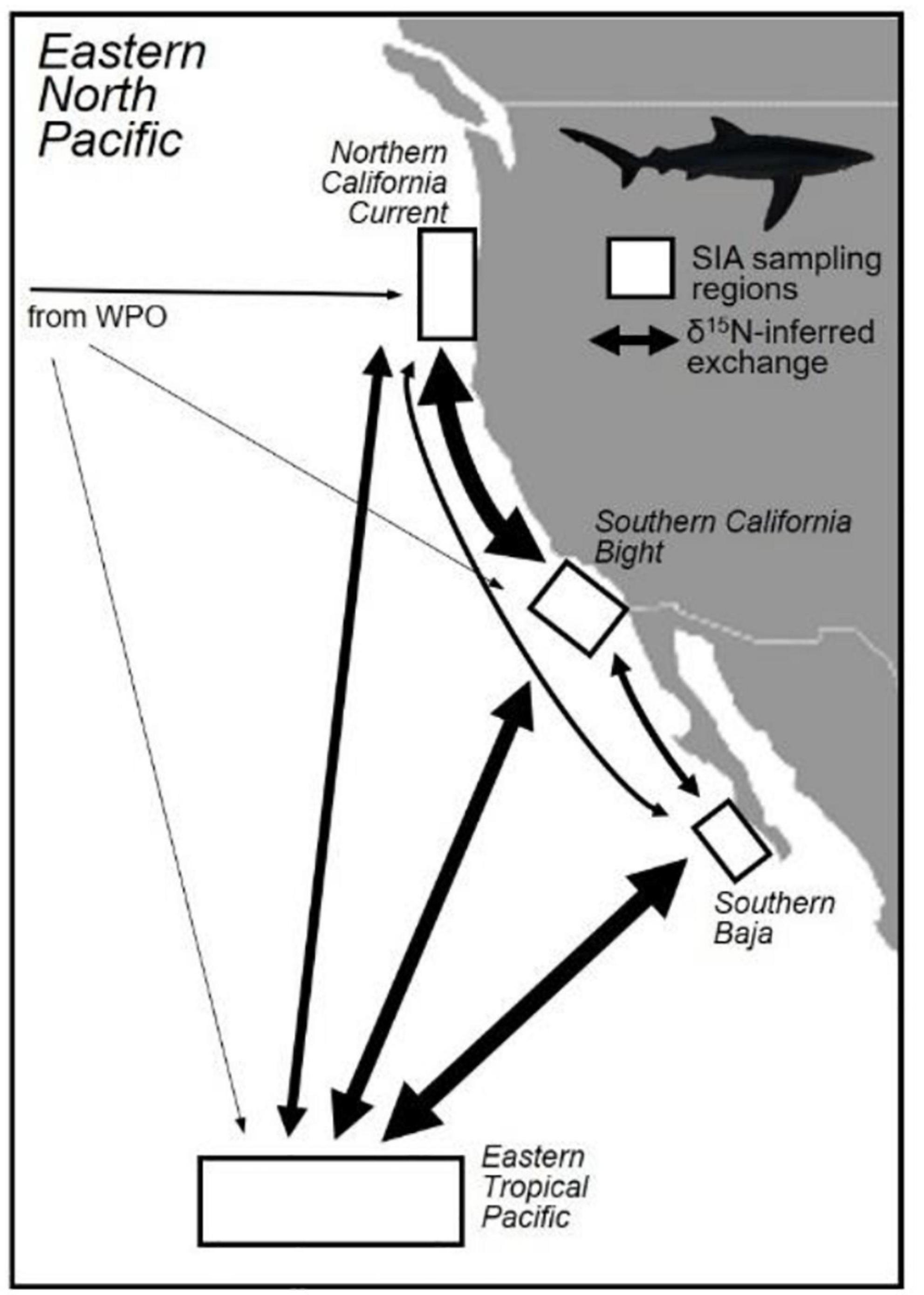
Figure 5. Summarized estimates of blue shark (Prionace glauca) migratory exchange between eastern North Pacific Ocean regions, inferred from niche overlap of regional δ13C and δ15N values. Migratory exchange was inferred quantitatively by calculating overlap between 95% probabilistic isotopic niches of each sub-region, using a Bayesian approach (“nicheROVER”; Swanson et al., 2015). Arrows are scaled to the degree of isotopic niche overlap between regions; note that exchange rates are relative and approximate.
Diet-Dependent DTDFs
Across all regions (i.e., EPO and WPO values, including all sub-regions), calculated blue shark Δ13C values ranged from 0.8 to 1.2‰ and for Δ15N from 1.7 to 3.9‰. Due to substantial variation in WPO diet δ15N values, overall WPO DTDFs generated in bootstrapped estimates ranged from 1.8 to 4.8‰ (3.1 ± 0.5‰), and EPO DTDFs from 1.4 to 1.9‰ (1.5 ± 0.1‰) (see Table 1 for all regional DTDFs). Sub-region diet-dependent DTDFs varied substantially within both the EPO and WPO, based on differences in sub-region prey baselines (Table 1).
Discriminant Analysis
Discriminant analysis classified all WPO blue sharks as residents to the WPO (0% EPO migrants). In the EPO, 95.3% of blue sharks were categorized as residents to the EPO (∼5% WPO migrants), which varied by EPO sub-region: NCC (11% migrants), ETP (5% migrants), SCB (2% migrants), and SBaja (0% migrants), with <1% classification error across all regional discriminant analyses. The value representing the cutoff point in discriminant analyses (threshold δ15N value between WPO- and EPO-classified sharks) was similar across all analyses conducted, at ∼14.0‰. Most δ15N values of WPO migrants in the EPO (δ15N < 14.0‰) were in the tails of bootstrapped population δ15N values, though one empirical EPO value (δ15N = 13.8‰; minimum reported in ETP) was classified as a WPO migrant.
Training data for discriminant analysis (estimated blue shark δ15N values from WPO and EPO prey, calculated from region-specific diet estimates and DTDFs), were (WPO) 13.1 ± 1.1‰ and (EPO) 15.8 ± 1.1‰. WPO and EPO training data were highly discrete between the two regions (13% overlap; Figure 3) and highly coherent with bootstrapped blue shark δ15N distributions (Figure 3).
Isotopic Mixing Models
Substantial blue shark SI data (9–32%) fell outside of the prey mixing space due to low shark δ13C values (Supplementary Figure 1 and Supplementary Table 2), leading to unreliable mixing model results. There were large discrepancies between dual (δ13C and δ15N) and single (δ15N) isotope mixing models in both the EPO and WPO (Supplementary Figures 2, 3 and Supplementary Tables 2, 3). In the EPO, the dual isotope model suggested high SCB inputs to all regions (66–100%), likely biased by the SCB having the lowest prey δ13C values (Supplementary Figure 2). Results suggested low contributions from the WPO (i.e., trans-Pacific migrants) in the NCC (15%) and SCB (12%), and minimal inputs from the NCC and SBaja (0–5%) (Supplementary Figure 2 and Supplementary Table 2). The single isotope model indicated higher contributions from all EPO sub-regions, though SCB inputs were still important to all sub-regions (Supplementary Figure 2 and Supplementary Table 2).
In the WPO, a similarly high proportion of blue shark SI data (55%) fell outside of prey mixing space due to low shark δ13C values (Supplementary Figure 1 and Supplementary Table 2). The dual isotope model suggested blue sharks foraged most on prey from Kuroshio-Oyashio (65%), though model results were likely biased by that region’s relatively low prey δ13C values (Supplementary Figure 3). When δ13C values were excluded from mixing models, the single-isotope δ15N model suggested greater contributions to blue shark diet from all sub-regions, with the southern region (offshore Taiwan) contributing the most (56%; Supplementary Figure 3 and Supplementary Table 3). The 95% credible intervals for all mixing models results are shown in Supplementary Tables 2, 3.
Discussion
Region-specific stable isotope values of blue sharks and prey allowed for inferences of prior movement patterns and regional connectivity in the North Pacific Ocean, quantifying dynamics that have been observed (e.g., trans-Pacific migrations), but for which frequency and exchange rates are unknown. Our results suggest minimal trans-Pacific movements and indicate potential finer-scale movement and residency dynamics within sub-regions of the EPO and WPO, though key caveats were evident in estimates of finer scale movements. High coherence of prey-estimated blue shark δ15N values with empirical SI values demonstrated the effectiveness and predictive value of North Pacific isoscapes. Overall, our results demonstrate the efficacy of our multi-analytical stable isotope approach to identify movements of a highly migratory pelagic species in the North Pacific, and the potential for future analyses with consideration of sample treatment and quantification of crucial species-specific isotopic parameters.
Trans-Pacific migrations in North Pacific blue sharks were first demonstrated by conventional tagging efforts in the eastern Pacific (Sippel et al., 2011), and we used SI data to assess the frequency of EPO↔WPO movements. In the WPO, SI datasets revealed no evidence of migrations from the EPO, compared to conventional tagging studies that reported 4 of 205 (∼2%) EPO-tagged (NCC, SCB, and SBaja) blue sharks migrating to the WPO (Sippel et al., 2011). In the EPO, discriminant analysis indicated trans-Pacific migration from the WPO in low proportions (∼5%) of our EPO bootstrapped population estimates, including at least one empirical migrant value (δ15N = 13.8‰; ETP). However, conventional tagging found no trans-Pacific migration to the EPO from the WPO (n = 207) (Sippel et al., 2011), and more recent satellite tagging in the EPO (n = 47) and WPO (n = 21) showed movements only within those respective ocean regions (Maxwell et al., 2019; Fujinami et al., 2021). While tagging studies have thus provided quantitative metrics of trans-Pacific migrations, tag-inferred movements have limitations, including simplistic movement information and potential non-reporting of tag recovery (conventional tags), limited sample size (electronic tags), and short-term tracks that do not capture long-distance movements (both tag types) (Siskey et al., 2019). Tagging data is also prospective, capturing future rather than prior movements that are potentially biased by tagging location. In contrast, isotopic measurements coupled with isotopic turnover rates and DTDFs can provide retrospective, quantifiable timeframes of prior movements. While SI-inferred movements are limited by isotopic turnover rates of analyzed tissue, prior movements can be characterized due to mobile predators integrating prey isotopic signatures during movements through isotopically distinct regions (Graham et al., 2010; MacKenzie et al., 2012; Carlisle et al., 2015; Madigan, 2015; Trueman and Glew, 2019). As such, isotope- and tag-inferred migration patterns are effective complementary techniques (Carlisle et al., 2012, 2015; Madigan et al., 2015, 2018; Shipley et al., 2021). Here, comparing isotopic estimates to past conventional tagging suggests that blue sharks make both eastward and westward trans-Pacific migrations, though the number of sharks that make these migrations appears to be low (<5%).
The low trans-Pacific exchange inferred here improve understanding of blue shark population dynamics in the North Pacific. Genetic analyses have shown global panmixia across regional blue shark populations, with minimal evidence of regional population structure (Taguchi et al., 2015; Veríssimo et al., 2017; Bailleul et al., 2018). This lack of observed population structure requires some mechanism of regional population mixing, and while maintenance of population genetic homogeneity does not necessarily require high mixing (Bremer et al., 2005; Waples and Gaggiotti, 2006), the minimal trans-Pacific exchange we observed here is unlikely to be the primary driver. Current understanding of blue shark life history provides several alternative scenarios for mixing of EPO and WPO populations. If WPO and EPO sharks mate (central-southern waters; ∼20–30°N) and pup (northern waters; ∼35–45°N) (Nakano, 1994; Nakano and Stevens, 2008) in their respective ocean basins, EPO- and WPO-origin young-of-the-year (YOYs) could subsequently recruit to either the WPO or EPO. In addition, the Central Pacific Ocean (CPO) (i.e., waters around Hawaii) could serve as a mixing region for WPO and EPO sharks, as some exchange of blue sharks in this region has been observed previously (Sippel et al., 2011). Consequently, dispersive YOY recruitment and/or partial, temporary mixing of adults in the CPO (if for mating or parturition) could drive genetic mixing while maintaining regional WPO and EPO isotopic signatures that indicate regional residency of juveniles and adults. Alternatively, genetic analysis has demonstrated the possibility of “genetic time-lag” effects in blue sharks (Bailleul et al., 2018), with the possibility of discrete sub-populations despite genetic homogeneity; thus, WPO and EPO separation observed here could indicate discrete, minimally mixed sub-populations. As such, the degree to which YOY recruitment and adult mixing contribute to North Pacific blue shark population dynamics warrants further study.
Comparison of blue shark and prey values between the WPO and EPO demonstrated that δ15N, and not δ13C, serves as a regional diagnostic tracer in the North Pacific Ocean as has been previously observed in Pacific bluefin tuna (Madigan et al., 2017). We observed high overlap of WPO and EPO prey δ13C values, but almost no overlap of prey δ15N values (Figure 2). Mechanisms for this have been demonstrated, with photosynthetic pathways in pelagic primary producers (C3 photosynthesis) varying more with latitude (i.e., due to variable productivity, temperature, and seawater pCO2 regimes), rather than longitude (Bowen, 2010; Magozzi et al., 2017; Brault et al., 2018; Ohshimo et al., 2019). Coherence of prey-based estimated δ15N blue shark values with shark-derived values (Figure 3) further supports both the robustness of the δ15N isoscape approach and the marked separation between δ15N values of WPO and EPO sharks. Similar patterns of distinct EPO and WPO isotopic signatures have been found in Pacific bluefin tuna, a large-bodied pelagic teleost in the WPO and EPO that makes seasonal migrations through the same WPO and EPO regions (Boustany et al., 2010; Madigan et al., 2017; Tawa et al., 2017). In Pacific bluefin tuna, δ15N-based estimates of trans-Pacific migration were demonstrably effective based on coherence with other chemical tracers, while non-differentiation of tuna δ13C made it an ineffective migration tracer (Madigan et al., 2017). This differs from more typical isotopic applications to ecology, which generally use δ15N to estimate trophic dynamics and δ13C to trace energy source and foraging location(s), due to lower trophic fractionation of δ13C than of δ15N (Post, 2002). However, more recent studies have demonstrated that in some systems and for certain predators, baseline δ15N values across ecoregions can result in predators acquiring regional δ15N signatures that outweigh trophic effects (Graham et al., 2010; Hobson et al., 2010; Madigan et al., 2017; Shipley et al., 2021). Isoscapes will be basin- and ecosystem-dependent. In the North Pacific, the δ15N gradient is caused by upwelling-driven enrichment of 15N in the EPO and low δ15N values due to oligotrophic N-fixation in the WPO (Takai et al., 2007; Madigan et al., 2012a, 2017; Fujinami et al., 2018; Ohshimo et al., 2019). The finer scale structure of δ13C and δ15N values in the WPO and EPO shown here demonstrate the utility of SIA in these ecosystems to track inter- and intra-basin predator movements.
We used niche overlap of blue shark δ13C and δ15N values to quantify finer scale connectivity dynamics within EPO and WPO sub-regions. In the EPO, isotopic niche overlap suggested variable mixing between the NCC, SCB, SBaja, and ETP (Figure 5). High exchange was suggested between the NCC and SCB; this is supported by conventional (Sippel et al., 2011) and electronic tag studies, which also found sexual segregation between these two regions (Maxwell et al., 2019). Lower overlap between SBaja and the NCC/SCB suggests that sharks may be more resident to this region. Regional proximity likely plays a role in exchange dynamics, as in general, more proximate regions showed greater migratory exchange (Figure 5). Collectively, estimated connectivity in the ETP and SBaja supports the premise that coastal and pelagic waters off SBaja may serve as both an overwintering ground for juveniles and a potential reproductive function for adults (Vögler et al., 2012), with isotopic overlap suggesting migration from SBaja to the ETP (Figure 5). Importantly, movement dynamics related to size and sex structure have been observed across these regions (Nakano and Stevens, 2008; Vögler et al., 2012; Maxwell et al., 2019), which we could not evaluate here due to a lack of size- and sex-specific information to match with blue shark isotopic values. Improved insight into size- and sex-specific movement dynamics could be accomplished with robust sampling of blue sharks for empirical isotopic measurements, coupled with size and sex metadata across the defined study regions. This could easily be achieved through sampling of fisheries [by]catch, potentially incorporating tissues of different turnover rates (i.e., fast [plasma, liver]; slow [muscle]; Thomas and Crowther, 2015; Vander Zanden et al., 2015).
While mixing models provided exploratory and potentially informative estimates of regional connectivity, results of finer scale sub-regional movements appeared biased and thus unreliable. Although the δ15N values of sharks and DTDF-corrected prey highly overlapped, shark δ13C values were low relative to prey in both the EPO and WPO (Supplementary Figures 2, 3). Furthermore, prey mixing polygons (Smith et al., 2013) showed up to 32% and 55% of blue shark values falling outside of the simulated prey mixing space, due to these low shark δ13C values (Supplementary Figure 1 and Supplementary Tables 2, 3). This likely biased mixing model estimates toward the lowest δ13C prey input(s); this confounding factor was observed in the two-isotope mixing models in both the WPO (high input of Kuroshio-Oyashio) and EPO (high input of SCB) (Supplementary Figures 2, 3). A similar effect of low blue shark δ13C values was observed in a previously published estimate of diet based on isotope mixing models in the SBaja sub-region, in which low blue shark δ13C values relative to prey resulted in the two lowest δ13C prey items (pelagic octopus Argonauta spp. and pelagic red crab P. planipes) dominating diet estimates (Hernández-Aguilar et al., 2015). Consequently, mixing model results here demonstrate that biased results will likely occur when predator SI values are substantially offset from prey inputs. While this is unsurprising and has previously been identified in the literature, studies continue to adopt this approach and report findings without identifying potential bias. It is imperative that investigators use appropriate diagnostic tools (i.e., quantitative assessment of prey vs. predator data following Smith et al., 2013) to ensure results are accurate or that potential bias is reported. For blue sharks, low δ13C values that are irreconcilable with local prey is consistent across studies and requires clarification.
There are three possible explanations for the consistently low δ13C values observed in blue sharks: (i) feeding in a region, within timeframes, or on prey not represented in regional studies; (ii) estimated Δ13C values for blue sharks are too high, and fractionation between prey and blue shark muscle is lower than our estimated DTDF values; or (iii) shark sample preparation for SIA resulted in artificially low δ13C values across studies. Missing or isotopically misrepresented prey sources (scenario [i]) is a common issue across studies (Smith et al., 2013); here, sampling too close to coastlines could result in unrealistically high prey δ13C and/or δ15N values for a pelagic shark. However, most studies used in these analyses explicitly sampled in offshore epi- and mesopelagic zones. In addition, prey data are available for two offshore regions that were not included here, the TZCF and CPO, and prey δ13C values from those regions (−18.2 ± 0.8‰ and −17.7 ± 0.8‰, respectively) (Gould et al., 1997; Choy et al., 2015) are also not low enough to explain the observed shark δ13C values. Temporal variability in isotopic values, while possibly influential, is also an unlikely explanation here, as studies spanned multiple seasons and years and different EPO and WPO values are driven by coarsely consistent oceanographic conditions (Madigan et al., 2017). It is possible that our DTDF estimates are imprecise (scenario [ii]), as they were calculated as diet-based DTDFs following Caut et al. (2009) and Hussey et al. (2014), rather than empirically derived from laboratory experiments. Differential amino acid composition and subsequent 13C fractionation in blue sharks could drive atypical DTDFs (McMahon et al., 2010). Laboratory-derived Δ13C DTDFs available for other elasmobranch species (Hussey et al., 2010; Logan and Lutcavage, 2010; Kim et al., 2012) are rarely <1.0‰ (the mean DTDF applied here), and a DTDF of ≤0‰ would be necessary for blue shark values to be highly coherent with prey. Negative Δ13C values for sharks are rare in available studies, though one negative value (−0.5‰) has been estimated for one prey type (of 8 total diet items in natural diet) in the catshark Scyliorhinus canicula, so this DTDF in wild blue sharks cannot be ruled out (Caut et al., 2013). Similar observations in other ocean basins of low blue shark δ13C values relative to prey and/or other sharks (Rabehagasoa et al., 2012; Li et al., 2014; Kiszka et al., 2015) support the possibility that a unique aspect of blue shark muscle composition or physiology could drive atypically low Δ13C, but this likely can only be validated with captive studies. Finally, it is possible that sample preparation partially contributed to low δ13C values in at least some studies (scenario [iii]). Studies included here performed lipid and/or urea extraction, but not always both (Madigan et al., 2012a; Li et al., 2014; Hernández-Aguilar et al., 2015; Fujinami et al., 2018), or mathematically corrected for lipids (Miller, 2006). Many of these studies preceded thorough published analyses demonstrating the importance of both lipid and urea extraction in elasmobranch tissues, which prevents artificially low δ13C and/or δ15N values (Carlisle et al., 2016; Li et al., 2016; Arostegui et al., 2019); recent work has also shown that water rinses for urea extraction may actually increase δ13C values (Carlisle et al., 2016). Our analyses, which draw upon published studies, underscore the need for standardized, consistent sample treatment in ongoing and future isotopic studies (Wolf et al., 2009; Shipley and Matich, 2020), while also noting the value of large archival datasets to address questions at an ocean basin scale. While laboratory-derived DTDFs will be difficult to obtain directly for blue sharks in captivity, improved understanding of 13C dynamics in this species will be necessary to refine the accuracy of mixing model results, an issue that may also be applicable across other elasmobranch species.
While the data used here came from known high-use regions for blue sharks in the North Pacific, there are other relevant regions that could not be included in our analyses. In particular, the North Pacific Transition Zone (NPTZ) (also referred to as the Transition Zone Chlorophyll Front) has been shown as a region of high blue shark abundance (Pearcy, 1991; Polovina et al., 2001; Kubodera et al., 2007; Vögler et al., 2012). Recently, the NPTZ has been demonstrated as a migratory corridor for pregnant females that were satellite tagged in the WPO (Fujinami et al., 2021), has been suggested as a nursery ground for young sharks (Nakano and Stevens, 2008), and may be a migratory corridor for other blue shark life stages/sexes as well as tunas, swordfish, and turtles (Block et al., 2011). While blue shark isotope data were not available from the NPTZ, prey δ13C and δ15N appear to be similar to the WPO (Gould et al., 1997). As such, blue sharks with long-term residency in the NPTZ may be isotopically indistinguishable from WPO residents. It is currently unknown whether blue sharks in the NPTZ are residential to the frontal region for adequate timeframes (i.e., months to >1 yr) to acquire the NPTZ isotopic signal or whether they use the region temporarily during inter-region migrations; the WPO migrants we observed here in the NCC could reasonably be entering the California Current via the NPTZ. Isotopic sampling of NPTZ sharks, likely accessible from bycatch in offshore fisheries, could ascertain migration dynamics in NPTZ sharks. Similarly, blue sharks are common in the Central Pacific (i.e., waters around Hawaii; CPO), where conventional tagging suggests mixing with both the EPO and CPO (Sippel et al., 2011). With no isotopic characterizations of blue sharks in the CPO, we could not include this region here; however, isotopic analysis of CPO sharks could reveal the extent to which these sharks are residential to the region, as local prey seem to be distinctive from other regions, particularly prey δ15N values (Choy et al., 2015). Collecting samples across the spatial range of North Pacific fisheries is tractable, especially since a small biopsy sample from a subsequently released, live shark is sufficient for SIA.
This study was limited to the use of bootstrap-estimated blue shark δ13C and δ15N values rather than using direct empirical measurements. While our approach allowed for population-wide estimates based on empirically derived data distributions, some regional blue shark studies had relatively low sample sizes (e.g., n = 9 in SCB, n = 10 in NCC), and in this context, the “tails” of bootstrapped distributions likely lead to unrealistically high and low SI values (Madigan et al., 2017). Bootstrapped estimates can also lead to de-coupled shark δ13C and δ15N values (see shark data ellipses in Figures 2, 4 and Supplementary Figure 2), when empirical δ13C and δ15N values are often positively correlated. This could affect mixing model estimates of prey/regional contributions to blue shark diet. The lack of size and sex data also limited our ability to undertake more detailed reconstruction of blue shark residency and movement dynamics across life history. Finally, isotopic identification of migrants is dictated by tissue turnover to steady-state conditions (here, likely ∼0.5 to 1.5 year before sampling), precluding identification of long-distance migrations that occurred prior to these timeframes. As such, results here should be taken in the context of these limitations and be viewed as a preliminary framework for regionally focused, empirical investigation.
Conclusion
Our results, drawing upon published δ13C and δ15N data for blue sharks and prey sampled at multiple locations in the EPO and WPO, provide a new and replicable means to assess blue shark residency and migration dynamics in the North Pacific. The analyzed data provide strong evidence for limited direct migrations between the WPO and EPO and reiterate the utility of δ15N isoscapes for the reconstruction of migratory predator movements in the North Pacific Ocean. Limited trans-Pacific migrations suggest that other mechanisms maintain genetic homogeneity of the North Pacific blue shark population, including YOY movements and/or partial mixing of adults in the Central Pacific. Regional structure in δ13C and δ15N data have promise for further quantification of finer-scale blue shark movements, increasing the resolutions of movement patterns suggested here, but consideration of isotopic parameters (e.g., accurate species-specific DTDFs), appropriate sample preparation of shark tissues, and length/sex metadata of sampled sharks are necessary. With emerging research showing varying residency and trans-regional movements in migratory predators, isoscapes can employ high sample sizes across a breadth of animal life stages, regions, and timeframes to reconstruct habitat use of highly mobile marine animals. Through these isotopic approaches, population-level estimates of movement dynamics are feasible on scales that may not be readily available from conventional tagging or telemetry studies.
Data Availability Statement
The data analyzed in this study is subject to the following licenses/restrictions: Data came from published manuscripts, which are only publicly available through purchase of publications. Requests to access these datasets should be directed to ZGFuaWVsLm1hZGlnYW5Ac3Rvbnlicm9vay5lZHU=.
Author Contributions
DJM, ONS, NEH, and ABC conceived the study. DJM, ONS, and ABC performed the analyses. DJM and ONS wrote the manuscript, with input from all authors. All authors interpreted results and refined analyses.
Funding
This project was supported by WWF Canada and an NSERC Discovery Grant (#04922-2017) to NEH.
Conflict of Interest
The authors declare that the research was conducted in the absence of any commercial or financial relationships that could be construed as a potential conflict of interest.
Acknowledgments
Information and comments regarding conventional tagging data were generously provided by T. Sippel, and K. James provided valuable comments on the manuscript.
Supplementary Material
The Supplementary Material for this article can be found online at: https://www.frontiersin.org/articles/10.3389/fmars.2021.653606/full#supplementary-material
Supplementary Figure 1 | Comparisons of blue shark (Prionace glauca) δ13C and δ15N values to regional prey. Prey fields (filled gray forms) were generated by simulating 10,000 polygons using prey δ13C and δ15N values, following Smith et al. (2013). All prey values are adjusted by the addition of calculated diet-based DTDFs to allow quantification of overlap with blue shark δ13C and δ15N values (black circles). Proportion of blue shark values falling outside prey polygons, mostly due to low shark δ13C values, were 55% in the WPO (upper left panel) and 9–32% in regions of the EPO (other panels). These data highlight the importance of quantitatively assessing prey/predator isotope dynamics to ensure accurate interpretation of mixing models results and/or to determine (and report) the level of potential bias.
Supplementary Figure 2 | Isotopic overlap of regional blue shark (Prionace glauca) data with regional prey, and exploratory mixing model estimates of regional prey contributions, in sub-regions of the eastern Pacific Ocean. (A) Bootstrapped blue shark δ13C and δ15N values (small circles, colored by EPO sampling sub-region) and regional prey means (large circles; error bars ± SD), from the western (WPO) and eastern (EPO) Pacific Ocean. Mean prey δ13C and δ15N values are adjusted by the addition of calculated diet-dependent diet-tissue discrimination factors (DTDFs) (Caut et al., 2009; Hussey et al., 2014). After prey mean adjustment for DTDF, most blue shark δ13C values were left-shifted (lower δ13C) relative to prey δ13C values. (B) Estimated regional prey inputs to EPO blue shark diet from Bayesian mixing models. Left panel shows results from the dual isotope model (δ13C and δ15N), which were biased toward the regional prey with lowest δ13C values, and right panel the single isotope (δ15N) model.
Supplementary Figure 3 | Isotopic overlap of blue shark (Prionace glauca) data with regional prey, and exploratory mixing model estimates of regional prey contributions, in the western Pacific Ocean. (A) Bootstrapped blue shark δ15N values (small gray circles) and regional prey means (large circles, colored by WPO sub-region) from the western Pacific Ocean (WPO). Mean prey δ13C and δ15N values are adjusted by the addition of calculated diet-dependent diet-tissue discrimination factors (DTDFs) (Caut et al., 2009; Hussey et al., 2014). After prey mean adjustment for DTDF, most blue shark δ13C values were left-shifted (lower δ13C) from expected prey-based values. (B) Estimated regional prey inputs to WPO blue shark diet from Bayesian mixing models. Left panel shows results from two isotope model (13C and δ15N), which were biased toward the regional prey with lowest δ13C values, and right panel shows a single isotope (δ15N) model.
Supplementary Table 1 | Isotopic niche metrics generated from carbon and nitrogen stable isotope values of blue sharks (Prionace glauca). Standard ellipse (SEA) areas (‰2) are derived from Northern California Current, Southern California Bight, Southern Baja, Eastern Tropical Pacific, and West Pacific Ocean (WPO). SEA estimates represent maximum likelihood (SEA) and Bayesian (SEAB; [75% Cis]) derived estimates based on 40% of the data.
Supplementary Table 2 | Reliance of EPO blue shark populations on regional prey groups as inferred from Bayesian isotope mixing models. Results are median estimates (95% credible intervals [CIs]) derived from the posterior distributions of dual (δ13C and δ15N) and single (δ15N) isotope models. For dual isotope models, the percentage of individuals with >95% probability of falling outside of the simulated prey mixing space is shown.
Supplementary Table 3 | Reliance of WPO blue shark populations on regional prey groups as inferred from Bayesian isotope mixing models. Results are median estimates (95% credible intervals [CIs]) derived from the posterior distributions of dual (δ13C and δ15N) and single (δ15N) isotope models. For dual isotope models the percentage of individuals that had a >95% probability of falling outside of the simulated prey mixing space is indicated.
References
Altabet, M. A., Pilskaln, C., Thunell, R., Pride, C., Sigman, D., Chavez, F., et al. (1999). The nitrogen isotope biogeochemistry of sinking particles from the margin of the Eastern North Pacific. Deep Sea Res. Part I Oceanogr. Res. Pap. 46, 655–679. doi: 10.1016/s0967-0637(98)00084-3
Arostegui, M. C., Schindler, D. E., and Holtgrieve, G. W. (2019). Does lipid-correction introduce biases into isotopic mixing models? Implications for diet reconstruction studies. Oecologia 191, 745–755. doi: 10.1007/s00442-019-04525-7
Bailleul, D., Mackenzie, A., Sacchi, O., Poisson, F., Bierne, N., and Arnaud-Haond, S. (2018). Large-scale genetic panmixia in the blue shark (Prionace glauca): a single worldwide population, or a genetic lag-time effect of the “grey zone” of differentiation? Evol. Appl. 11, 614–630. doi: 10.1111/eva.12591
Block, B. A. I, Jonsen, D., Jorgensen, S. J., Winship, A. J., Shaffer, S. A., Bograd, S. J., et al. (2011). Tracking apex marine predator movements in a dynamic ocean. Nature 475, 86–90. doi: 10.1038/nature10082
Boustany, A. M., Matteson, R., Castleton, M., Farwell, C., and Block, B. A. (2010). Movements of Pacific bluefin tuna (Thunnus orientalis) in the Eastern North Pacific revealed with archival tags. Prog. Oceanogr. 86, 94–104. doi: 10.1016/j.pocean.2010.04.015
Bowen, G. J. (2010). Isoscapes: spatial pattern in isotopic biogeochemistry. Annu. Rev. Earth Planet. Sci. 38, 161–187. doi: 10.1146/annurev-earth-040809-152429
Brault, E. K., Koch, P. L., McMahon, K. W., Broach, K. H., Rosenfield, A. P., Sauthoff, W., et al. (2018). Carbon and nitrogen zooplankton isoscapes in West Antarctica reflect oceanographic transitions. Mar. Ecol. Prog. Ser. 593, 29–45. doi: 10.3354/meps12524
Bremer, J. R. A., Viñas, J., Mejuto, J., Ely, B., and Pla, C. (2005). Comparative phylogeography of Atlantic bluefin tuna and swordfish: the combined effects of vicariance, secondary contact, introgression, and population expansion on the regional phylogenies of two highly migratory pelagic fishes. Mol. Phylogenet. Evol. 36, 169–187. doi: 10.1016/j.ympev.2004.12.011
Burgess, G. H., Beerkircher, L. R., Cailliet, G. M., Carlson, J. K., Cortés, E., Goldman, K. J., et al. (2005). Is the collapse of shark populations in the Northwest Atlantic Ocean and Gulf of Mexico real? Fisheries 30, 19–26. doi: 10.1577/1548-8446(2005)30[19:itcosp]2.0.co;2
Campana, S. E., Joyce, W., and Manning, M. J. (2009). Bycatch and discard mortality in commercially caught blue sharks Prionace glauca assessed using archival satellite pop-up tags. Mar. Ecol. Prog. Ser. 387, 241–253. doi: 10.3354/meps08109
Cardeñosa, D., Fields, A. T., Babcock, E. A., Shea, S. K., Feldheim, K. A., and Chapman, D. D. (2020). Species composition of the largest shark fin retail-market in mainland China. Sci. Rep. 10, 1–10.
Carlisle, A. B., Goldman, K. J., Litvin, S. Y., Madigan, D. J., Bigman, J. S., Swithenbank, A. M., et al. (2015). Stable isotope analysis of vertebrae reveals ontogenetic changes in habitat in an endothermic pelagic shark. Proc. R. Soc. Lond. B Biol. Sci. 282:20141446. doi: 10.1098/rspb.2014.1446
Carlisle, A. B., Kim, S. L., Semmens, B. X., Madigan, D. J., Jorgensen, S. J., Perle, C. R., et al. (2012). Using stable isotope analysis to understand migration and trophic ecology of northeastern Pacific white sharks (Carcharodon carcharias). PLoS One 7:e30492. doi: 10.1371/journal.pone.0030492
Carlisle, A. B., Litvin, S. Y., Madigan, D. J., Lyons, K., Bigman, J. S., Ibarra, M., et al. (2016). Interactive effects of urea and lipid content confound stable isotope analysis in elasmobranch fishes. Can. J. Fish. Aquat. Sci. 99, 1–10.
Caut, S., Angulo, E., and Courchamp, F. (2009). Variation in discrimination factors (Δ15N and Δ13C): the effect of diet isotopic values and applications for diet reconstruction. J. Appl. Ecol. 46, 443–453. doi: 10.1111/j.1365-2664.2009.01620.x
Caut, S., Jowers, M. J., Michel, L., Lepoint, G., and Fisk, A. T. (2013). Diet-and tissue-specific incorporation of isotopes in the shark Scyliorhinus stellaris, a North Sea mesopredator. Mar. Ecol. Prog. Ser. 492, 185–198. doi: 10.3354/meps10478
Choy, C. A., Popp, B. N., Hannides, C. C. S., and Drazen, J. C. (2015). Trophic structure and food resources of epipelagic and mesopelagic fishes in the North Pacific Subtropical Gyre ecosystem inferred from nitrogen isotopic compositions. Limnol. Oceanogr. 60, 1156–1171. doi: 10.1002/lno.10085
Clarke, S. C., Magnussen, J. E., Abercrombie, D. L., McAllister, M. K., and Shivji, M. S. (2006a). Identification of shark species composition and proportion in the Hong Kong shark fin market based on molecular genetics and trade records. Conserv. Biol. 20, 201–211. doi: 10.1111/j.1523-1739.2005.00247.x
Clarke, S. C., McAllister, M. K., Milner-Gulland, E. J., Kirkwood, G., Michielsens, C. G., Agnew, D. J., et al. (2006b). Global estimates of shark catches using trade records from commercial markets. Ecol. Lett. 9, 1115–1126. doi: 10.1111/j.1461-0248.2006.00968.x
Coelho, R., Mejuto, J., Domingo, A., Yokawa, K., Liu, K. M., Cortés, E., et al. (2018). Distribution patterns and population structure of the blue shark (Prionace glauca) in the Atlantic and Indian Oceans. Fish Fish. 19, 90–106.
Espinasse, B., Hunt, B. P., Batten, S. D., and Pakhomov, E. A. (2020). Defining isoscapes in the Northeast Pacific as an index of ocean productivity. Glob. Ecol. Biogeogr. 29, 246–261. doi: 10.1111/geb.13022
Ferretti, F., Myers, R. A., Serena, F., and Lotze, H. K. (2008). Loss of large predatory sharks from the Mediterranean Sea. Conserv. Biol. 22, 952–964. doi: 10.1111/j.1523-1739.2008.00938.x
Francis, M. P., Griggs, L. H., and Baird, S. J. (2001). Pelagic shark bycatch in the New Zealand tuna longline fishery. Mar. Freshw. Res. 52, 165–178. doi: 10.1071/mf00086
Fujinami, Y., Nakatsuka, S., and Ohshimo, S. (2018). Feeding habits of the blue shark (Prionace glauca) in the Northwestern Pacific based on stomach contents and stable isotope ratios. Pacif. Sci. 72, 21–39. doi: 10.2984/72.1.2
Fujinami, Y., Shiozaki, K., Hiraoka, Y., Semba, Y., Ohshimo, S., and Kai, M. (2021). Seasonal migrations of pregnant blue sharks Prionace glauca in the northwestern Pacific. Mar. Ecol. Prog. Ser. 658, 163–179. doi: 10.3354/meps13557
Gould, P., Ostrom, P., and Walker, W. (1997). Trophic relationships of albatrosses associated with squid and large-mesh drift-net fisheries in the North Pacific Ocean. Can. J. Zool. 75, 549–562. doi: 10.1139/z97-068
Graham, B. S., Koch, P. L., Newsome, S. D., McMahon, K. W., and Aurioles, D. (2010). “Using isoscapes to trace the movements and foraging behavior of top predators in oceanic ecosystems,” in Isoscapes, eds J. B. West, G. J. Bowen, T. E. Dawson, and K. P. Tu (Netherlands: Springer), 299–318. doi: 10.1007/978-90-481-3354-3_14
Heady, W. N., and Moore, J. W. (2013). Tissue turnover and stable isotope clocks to quantify resource shifts in anadromous rainbow trout. Oecologia 172, 21–34. doi: 10.1007/s00442-012-2483-9
Hernández-Aguilar, S. B., Escobar-Sánchez, O., Galván-Magaña, F., and Abitia-Cárdenas, L. A. (2015). Trophic ecology of the blue shark (Prionace glauca) based on stable isotopes (δ13C and δ15N) and stomach content. J. Mar. Biol. Assoc.U. K. 96:1403. doi: 10.1017/S002531541500139
Hobson, K. A., Barnett-Johnson, R., and Cerling, T. (2010). “Using isoscapes to track animal migration,” in Isoscapes: Understanding Movement, Pattern, and Process on Earth Through Isotope Mapping, eds B. J. West, J. G. Bowen, E. T. Dawson, and P. K. Tu (Dordrecht: Springer), 273–298. doi: 10.1007/978-90-481-3354-3_13
Howey, L. A., Wetherbee, B. M., Tolentino, E. R., and Shivji, M. S. (2017). Biogeophysical and physiological processes drive movement patterns in a marine predator. Mov. Ecol. 5:16.
Hussey, N. E., Brush, J. I, McCarthy, D., and Fisk, A. T. (2010). δ15N and δ13C diet-tissue discrimination factors for large sharks under semi-controlled conditions. Comparat. Biochem. Physiol. Part A Mol. Integrat. Physiol. 155, 445–453. doi: 10.1016/j.cbpa.2009.09.023
Hussey, N. E., MacNeil, M. A., McMeans, B. C., Olin, J. A., Dudley, S. F. J., Cliff, G., et al. (2014). Rescaling the trophic structure of marine food webs. Ecol. Lett. 17, 239–250.
Hussey, N. E., MacNeil, M. A., Olin, J. A., McMeans, B. C., Kinney, M. J., Chapman, D. D., et al. (2012). Stable isotopes and elasmobranchs: tissue types, methods, applications and assumptions. J. Fish Biol. 80, 1449–1484. doi: 10.1111/j.1095-8649.2012.03251.x
ISC (2017). “Stock assessment and future projections of blue shark in the North Pacific Ocean through 2015,” in Proceedings of the 17th Meeting of the International Scientific committee on Tuna and Tuna-like Species in the North Pacific Ocean (ISC), Vancouver.
Jackson, A. L., Inger, R., Parnell, A. C., and Bearhop, S. (2011). Comparing isotopic niche widths among and within communities: SIBER-stable isotope Bayesian Ellipses in R. J. Anim. Ecol. 80, 595–602. doi: 10.1111/j.1365-2656.2011.01806.x
Kim, S. L., Casper, D. R., Galván-Magaña, F., Ochoa-Díaz, R., Hernández-Aguilar, S. B., and Koch, P. L. (2012). Carbon and nitrogen discrimination factors for elasmobranch soft tissues based on a long-term controlled feeding study. Environ. Biol. Fish. 95, 37–52. doi: 10.1007/s10641-011-9919-7
King, J., Wetklo, M., Supernault, J., Taguchi, M., Yokawa, K., Sosa-Nishizaki, O., et al. (2015). Genetic analysis of stock structure of blue shark (Prionace glauca) in the north Pacific ocean. Fish. Res. 172, 181–189. doi: 10.1016/j.fishres.2015.06.029
Kiszka, J., Aubail, A., Hussey, N., Heithaus, M., Caurant, F., and Bustamante, P. (2015). Plasticity of trophic interactions among sharks from the oceanic south-western Indian Ocean revealed by stable isotope and mercury analyses. Deep Sea Res. Part I Oceanogr. Res. Pap. 96, 49–58. doi: 10.1016/j.dsr.2014.11.006
Kubodera, T., Watanabe, H., and Ichii, T. (2007). Feeding habits of the blue shark, Prionace glauca, and salmon shark, Lamna ditropis, in the transition region of the Western North Pacific. Rev. Fish Biol. Fish. 17:111. doi: 10.1007/s11160-006-9020-z
Li, Y., Gong, Y., Chen, X., Dai, X., and Zhu, J. (2014). Trophic ecology of sharks in the mid-east Pacific ocean inferred from stable isotopes. J. Ocean Univ. China 13, 278–282. doi: 10.1007/s11802-014-2071-1
Li, Y., Zhang, Y., Hussey, N. E., and Dai, X. (2016). Urea and lipid extraction treatment effects on δ15N and δ13C values in pelagic sharks. Rapid Commun. Mass Spectrom. 30, 1–8. doi: 10.1002/rcm.7396
Logan, J., and Lutcavage, M. (2010). Stable isotope dynamics in elasmobranch fishes. Hydrobiologia 644, 231–244. doi: 10.1007/s10750-010-0120-3
MacKenzie, K. M., Trueman, C. N., Palmer, M. R., Moore, A., Ibbotson, A. T., Beaumont, W. R., et al. (2012). Stable isotopes reveal age-dependent trophic level and spatial segregation during adult marine feeding in populations of salmon. ICES J. Mar. Sci. 69, 1637–1645. doi: 10.1093/icesjms/fss074
Madigan, D. J. (2015). “Understanding bluefin migration using intrinsic tracers in tissues,” in Biology and Ecology of Bluefin Tuna, eds T. Kitagawa and S. Kimura (Boca Raton, FL: CRC Press), 211–224.
Madigan, D. J., Baumann, Z., Carlisle, A. B., Hoen, D. K., Popp, B. N., Dewar, H., et al. (2014). Reconstructing trans-oceanic migration patterns of Pacific bluefin tuna using a chemical tracer toolbox. Ecology 95, 1674–1683. doi: 10.1890/13-1467.1
Madigan, D. J., Baumann, Z., Carlisle, A. B., Snodgrass, O., Dewar, H., and Fisher, N. S. (2017). Isotopic insights into migration patterns of Pacific bluefin tuna in the eastern Pacific Ocean. Can. J. Fish. Aquat. Sci. 75, 260–270. doi: 10.1139/cjfas-2016-0504
Madigan, D. J., Brooks, E. J., Bond, M. E., Gelsleichter, J., Howey, L. A., Abercrombie, D. L., et al. (2015). Diet shift and site-fidelity of oceanic whitetip sharks Carcharhinus longimanus along the Great Bahama Bank. Mar. Ecol. Prog. Ser. 529, 185–197. doi: 10.3354/meps11302
Madigan, D. J., Carlisle, A. B., Dewar, H., Snodgrass, O. E., Litvin, S. Y., Micheli, F., et al. (2012a). Stable isotope analysis challenges wasp-waist food web assumptions in an upwelling pelagic food web. Sci. Rep. 2:e654.
Madigan, D. J., Litvin, S. Y., Popp, B. N., Carlisle, A. B., Farwell, C. J., and Block, B. A. (2012b). Tissue turnover rates and isotopic trophic discrimination factors in the endothermic teleost, Pacific bluefin tuna (Thunnus orientalis). PLoS One 7:e49220. doi: 10.1371/journal.pone.0049220
Madigan, D. J., Chiang, W.-C., Wallsgrove, N. J., Popp, B. N., Kitagawa, T., Choy, C. A., et al. (2016). Intrinsic tracers reveal recent foraging ecology of giant Pacific bluefin tuna at their primary spawning grounds. Mar. Ecol. Prog. Ser. 553, 253–266. doi: 10.3354/meps11782
Madigan, D. J., Shipley, O. N., and Hussey, N. E. (2021). “Applying isotopic clocks to identify prior migration patterns and critical habitats in mobile marine predators,” in Conservation Physiology: Applications for Wildlife Conservation and Management, eds C. L. Madliger, C. E. Franklin, O. P. Love, and S. J. Cooke (Oxford: Oxford University Press), 69–85. doi: 10.1093/oso/9780198843610.003.0005
Madigan, D. J., Snodgrass, O. E., and Fisher, N. S. (2018). From migrants to mossbacks: tracer- and tag-inferred habitat shifts in the California yellowtail Seriola dorsalis. Mar. Ecol. Prog. Ser. 597, 221–230. doi: 10.3354/meps12593
Magozzi, S., Yool, A., Vander Zanden, H., Wunder, M., and Trueman, C. (2017). Using ocean models to predict spatial and temporal variation in marine carbon isotopes. Ecosphere 8:e01763. doi: 10.1002/ecs2.1763
Matsubayashi, J., Osada, Y., Tadokoro, K., Abe, Y., Yamaguchi, A., Shirai, K., et al. (2020). Tracking long-distance migration of marine fishes using compound-specific stable isotope analysis of amino acids. Ecol. Lett. 23, 881–890. doi: 10.1111/ele.13496
Maxwell, S. M., Scales, K. L., Bograd, S. J., Briscoe, D. K., Dewar, H., Hazen, E. L., et al. (2019). Seasonal spatial segregation in blue sharks (Prionace glauca) by sex and size class in the Northeast Pacific Ocean. Diver. Distribut. 25, 1304–1317.
McKinnell, S., and Seki, M. P. (1998). Shark bycatch in the Japanese high seas squid driftnet fishery in the North Pacific Ocean. Fish. Res. 39, 127–138. doi: 10.1016/s0165-7836(98)00179-9
McMahon, K. W., Fogel, M. L., Elsdon, T. S., and Thorrold, S. R. (2010). Carbon isotope fractionation of amino acids in fish muscle reflects biosynthesis and isotopic routing from dietary protein. J. Anim. Ecol. 79, 1132–1141. doi: 10.1111/j.1365-2656.2010.01722.x
McMahon, K. W., Hamady, L. L., and Thorrold, S. R. (2013). A review of ecogeochemistry approaches to estimating movements of marine animals. Limnol. Oceanogr. 58, 697–714. doi: 10.4319/lo.2013.58.2.0697
Miller, T. W. (2006). Trophic Dynamics of Marine Nekton and Zooplankton in the Northern California Current Pelagic Ecosystem. Corvallis: Oregon State University.
Miller, T. W., Bosley, K. L., Shibata, J., Brodeur, R. D., Omori, K., and Emmett, R. (2013). Contribution of prey to Humboldt squid Dosidicus gigas in the northern California Current, revealed by stable isotope analyses. Mar. Ecol. Prog. Ser. 477, 123–134. doi: 10.3354/meps10133
Miller, T. W., Brodeur, R. D., Rau, G., and Omori, K. (2010). Prey dominance shapes trophic structure of the northern California Current pelagic food web: evidence from stable isotopes and diet analysis. Mar. Ecol. Prog. Ser. 420, 15–26. doi: 10.3354/meps08876
Montoya, J. P. (2007). “Natural abundance of 15N in marine planktonic ecosystems,” in Stable Isotopes in Ecology and Environmental Science, eds R. Michener and K. Lajtha (Boston, FL: Blackwell), 176–201. doi: 10.1002/9780470691854.ch7
Moore, J. W., Gordon, J., Carr-Harris, C., Gottesfeld, A. S., Wilson, S. M., and Russell, J. H. (2016). Assessing estuaries as stopover habitats for juvenile Pacific salmon. Mar. Ecol. Prog. Ser. 559, 201–215. doi: 10.3354/meps11933
Musyl, M. K., Brill, R. W., Curran, D. S., Fragoso, N. M., McNaughton, L. M., Nielsen, A., et al. (2011). Postrelease survival, vertical and horizontal movements, and thermal habitats of five species of pelagic sharks in the central Pacific Ocean. Fish. Bull. 109, 341–368.
Nakano, H. (1994). Age, reproduction and migration of blue shark in the North Pacific Ocean. Bull. Natl. Res. Insti. Far Seas Fish. 31, 141–256.
Nakano, H., and Stevens, J. D. (2008). “The biology and ecology of the blue shark, Prionace glauca,” in Sharks of the Open Ocean: Biology, Fisheries and Conservation, eds M. D. Canhi, E. K. Pikitch, and E. A. Babcock (Hoboken, NJ: Wiley Online Library), 140–151. doi: 10.1002/9781444302516.ch12
Ohshimo, S., Madigan, D. J., Kodama, T., Tanaka, H., Komoto, K., Suyama, S., et al. (2019). Isoscapes reveal patterns of δ13C and δ15N of pelagic forage fish and squid in the Northwest Pacific Ocean. Prog. Oceanogr. 175, 124–138. doi: 10.1016/j.pocean.2019.04.003
Ohshimo, S., Tanaka, H., Nishiuchi, K., and Yasuda, T. (2016). Trophic positions and predator-prey mass ratio of the pelagic food web in the East China Sea and Sea of Japan. Mar. Freshw. Res. 67, 1692–1699. doi: 10.1071/mf15115
Phillips, D. L., Inger, R., Bearhop, S., Jackson, A. L., Moore, J. W., Parnell, A. C., et al. (2014). Best practices for use of stable isotope mixing models in food-web studies. Can. J. Zool. 92, 823–835. doi: 10.1139/cjz-2014-0127
Polovina, J. J., Howell, E., Kobayashi, D. R., and Seki, M. P. (2001). The transition zone chlorophyll front, a dynamic global feature defining migration and forage habitat for marine resources. Prog. Oceanogr. 49, 469–483. doi: 10.1016/s0079-6611(01)00036-2
Post, D. M. (2002). Using stable isotopes to estimate trophic position: models, methods, and assumptions. Ecology 83, 703–718. doi: 10.1890/0012-9658(2002)083[0703:usitet]2.0.co;2
Preti, A., Soykan, C., Dewar, H., Wells, R., Spear, N., and Kohin, S. (2012). Comparative feeding ecology of shortfin mako, blue and thresher sharks in the California Current. Environ. Biol. Fish. 95, 127–146. doi: 10.1007/s10641-012-9980-x
Queiroz, N., Humphries, N. E., Couto, A., Vedor, M., Costa, I. Da, Sequeira, A. M., et al. (2019). Global spatial risk assessment of sharks under the footprint of fisheries. Nature 572, 461–466.
Queiroz, N., Humphries, N. E., Mucientes, G., Hammerschlag, N., Lima, F. P., Scales, K. L., et al. (2016). Ocean-wide tracking of pelagic sharks reveals extent of overlap with longline fishing hotspots. Proc. Natl. Acad. Sci. U.S.A. 113, 1582–1587. doi: 10.1073/pnas.1510090113
Rabehagasoa, N., Lorrain, A., Bach, P., Potier, M., Jaquemet, S., Richard, P., et al. (2012). Isotopic niches of the blue shark Prionace glauca and the silky shark Carcharhinus falciformis in the southwestern Indian Ocean. Endang. Spec. Res. 17, 83–92. doi: 10.3354/esr00418
Sequeira, A., Heupel, M., Lea, M. A., Eguíluz, V. M., Duarte, C. M., Meekan, M., et al. (2019). The importance of sample size in marine megafauna tagging studies. Ecol. Appl. 29:e01947.
Shipley, O. N., and Matich, P. (2020). Studying animal niches using bulk stable isotope ratios: an updated synthesis. Oecologia 193, 27–51. doi: 10.1007/s00442-020-04654-4
Shipley, O. N., Newton, A. H., Frisk, M. G., Henkes, G. A., Walters, H., LaBelle, J., et al. (2021). Telemetry validated nitrogen stable isotope clocks identify ocean-to-estuarine habitat shifts in mobile organisms. Methods Ecol. Evol. 12, 897–908. doi: 10.1111/2041-210X.13567
Sippel, T., Wraith, J., Kohin, S., Taylor, V., Holdsworth, J., Taguchi, M., et al. (2011). “A summary of blue shark (Prionace glauca) and shortfin mako shark (Isurus oxyrinchus) tagging data available from the North and Southwest Pacific Ocean,” in Proceedings of the California: Working Document Submitted to the ISCShark Working Group Workshop, La Jolla, CA.
Siskey, M. R., Shipley, O. N., and Frisk, M. G. (2019). Skating on thin ice: Identifying the need for species-specific data and defined migration ecology of Rajidae spp. Fish Fish. 20, 286–302. doi: 10.1111/faf.12340
Smith, J. A., Mazumder, D., Suthers, I. M., and Taylor, M. D. (2013). To fit or not to fit: evaluating stable isotope mixing models using simulated mixing polygons. Methods Ecol. Evol. 4, 612–618. doi: 10.1111/2041-210x.12048
Swanson, H. K., Lysy, M., Power, M., Stasko, A. D., Johnson, J. D., and Reist, J. D. (2015). A new probabilistic method for quantifying n-dimensional ecological niches and niche overlap. Ecology 96, 318–324. doi: 10.1890/14-0235.1
Taguchi, M., King, J. R., Wetklo, M., Withler, R. E., and Yokawa, K. (2015). Population genetic structure and demographic history of Pacific blue sharks (Prionace glauca) inferred from mitochondrial DNA analysis. Mar. Freshw. Res. 66, 267–275. doi: 10.1071/mf14075
Takai, N., Hirose, N., Osawa, T., Hagiwara, K., Kojima, T., Okazaki, Y., et al. (2007). Carbon source and trophic position of pelagic fish in coastal waters of south-eastern Izu Peninsula, Japan, identified by stable isotope analysis. Fish. Sci. 73, 593–608. doi: 10.1111/j.1444-2906.2007.01372.x
Tamburin, E., Kim, S. L., Elorriaga-Verplancken, F. R., Madigan, D. J., Hoyos-Padilla, M., ánchez-González, A. S., et al. (2019). Isotopic niche and resource sharing among young sharks (Carcharodon carcharias and Isurus oxyrinchus) in Baja California, Mexico. Mar. Ecol. Prog. Ser. 613, 107–124. doi: 10.3354/meps12884
Tawa, A., Ishihara, T., Uematsu, Y., Ono, T., and Ohshimo, S. (2017). Evidence of westward transoceanic migration of Pacific bluefin tuna in the Sea of Japan based on stable isotope analysis. Mar. Biol. 4:94.
Thomas, S. M., and Crowther, T. W. (2015). Predicting rates of isotopic turnover across the animal kingdom: a synthesis of existing data. J. Anim. Ecol. 84, 861–870. doi: 10.1111/1365-2656.12326
Trueman, C. N., and Glew, K. S. J. (2019). “Isotopic tracking of marine animal movement,” in Tracking animal Migration with Stable Isotopes, eds C. N. Trueman and K. S. J. Glew (Amsterdam: Elsevier), 137–172. doi: 10.1016/b978-0-12-814723-8.00006-4
Trueman, C. N., Jackson, A. L., Chadwick, K. S., Coombs, E. J., Feyrer, L. J., Magozzi, S., et al. (2019). Combining simulation modeling and stable isotope analyses to reconstruct the last known movements of one of Nature’s giants. PeerJ 7:e7912. doi: 10.7717/peerj.7912
Vander Zanden, M. J., Clayton, M. K., Moody, E. K., Solomon, C. T., and Weidel, B. C. (2015). Stable isotope turnover and half-life in animal tissues: a literature synthesis. PLoS One 10:e0116182. doi: 10.1371/journal.pone.0116182
Veríssimo, A. Í, Sampaio, J. R., Alexandrino, M. P., Mucientes, G., Queiroz, N., da Silva, C., et al. (2017). World without borders—genetic population structure of a highly migratory marine predator, the blue shark (Prionace glauca). Ecol. Evol. 7, 4768–4781. doi: 10.1002/ece3.2987
Vögler, R., Beier, E., Ortega-García, S., Santana-Hernández, H., and Valdez-Flores, J. J. (2012). Ecological patterns, distribution and population structure of Prionace glauca (Chondrichthyes: Carcharhinidae) in the tropical-subtropical transition zone of the north-eastern Pacific. Mar. Environ. Res. 73, 37–52.
Waples, R. S., and Gaggiotti, O. (2006). INVITED REVIEW: what is a population? An empirical evaluation of some genetic methods for identifying the number of gene pools and their degree of connectivity. Mol. Ecol. 15, 1419–1439. doi: 10.1111/j.1365-294x.2006.02890.x
Keywords: stable isotope, isoscape, pelagic ecology, isoclock, trophic ecology, migration
Citation: Madigan DJ, Shipley ON, Carlisle AB, Dewar H, Snodgrass OE and Hussey NE (2021) Isotopic Tracers Suggest Limited Trans-Oceanic Movements and Regional Residency in North Pacific Blue Sharks (Prionace glauca). Front. Mar. Sci. 8:653606. doi: 10.3389/fmars.2021.653606
Received: 14 January 2021; Accepted: 12 April 2021;
Published: 26 May 2021.
Edited by:
Mourier Johann, Institut de Recherche Pour le Développement (IRD), FranceReviewed by:
Vincent Raoult, The University of Newcastle, AustraliaMario Espinoza, Universidad Creativa de Costa Rica, Costa Rica
Boris Espinasse, UiT The Arctic University of Norway, Norway
Copyright © 2021 Madigan, Shipley, Carlisle, Dewar, Snodgrass and Hussey. This is an open-access article distributed under the terms of the Creative Commons Attribution License (CC BY). The use, distribution or reproduction in other forums is permitted, provided the original author(s) and the copyright owner(s) are credited and that the original publication in this journal is cited, in accordance with accepted academic practice. No use, distribution or reproduction is permitted which does not comply with these terms.
*Correspondence: Daniel J. Madigan, ZGFuaWVsLm1hZGlnYW5Ac3Rvbnlicm9vay5lZHU=