Social Network Analysis Reveals the Subtle Impacts of Tourist Provisioning on the Social Behavior of a Generalist Marine Apex Predator
- 1Institute of Zoology, Zoological Society of London, London, United Kingdom
- 2Lancaster Environment Centre, Lancaster University, Lancaster, United Kingdom
- 3Centre for Biodiversity and Environmental Research, University College London, London, United Kingdom
- 4South Carolina Department of Natural Resources, Marine Resources Research Institute, Charleston, SC, United States
- 5Beneath the Waves, Herndon, VA, United States
- 6Institute of Environment, Department of Biological Science, Florida International University, North Miami, FL, United States
- 7Fish Ecology and Conservation Physiology Laboratory, Department of Biology and Institute of Environmental and Interdisciplinary Science, Carleton University, Ottawa, ON, Canada
- 8Rosenstiel School of Marine and Atmospheric Science, University of Miami, Miami, FL, United States
- 9Leonard and Jayne Abess Center for Ecosystem Science and Policy, University of Miami, Coral Gables, FL, United States
Shark dive ecotourism is a lucrative industry in many regions around the globe. In some cases, sharks are provisioned using bait, prompting increased research on how baited dives influence shark behavior and yielding mixed results. Effects on patterns of habitat use and movement seemly vary across species and locations. It is unknown, however, whether wide-ranging, marine apex predators respond to provisioning by changing their patterns of grouping or social behavior. We applied a tiered analytical approach (aggregation-gregariousness-social preferences) examining the impact of provisioning on the putative social behavior of tiger sharks (Galeocerdo cuvier) at a dive tourism location in The Bahamas. Using network inference on three years of acoustic tracking data from 48 sharks, we tested for non-random social structure between non-provisioned and provisioned monitoring sites resulting in 12 distinct networks. Generally considered a solitary nomadic predator, we found evidence of sociality in tiger sharks, which varied spatiotemporally. We documented periods of both random (n = 7 networks) and non-random aggregation (n = 5 networks). Three of five non-random aggregations were at locations unimpacted by provisioning regardless of season, one occurred at an active provisioning site during the dry season and one at the same receivers during the wet season when provision activity is less prevalent. Aggregations lasted longer and occurred more frequently at provisioning sites, where gregariousness was also more variable. While differences in gregariousness among individuals was generally predictive of non-random network structure, individual site preferences, size and sex were not. Within five social preference networks, constructed using generalized affiliation indices, network density was lower at provisioning sites, indicating lower connectivity at these locations. We found no evidence of size assortment on preferences. Our data suggest that sociality may occur naturally within the Tiger Beach area, perhaps due to the unusually high density of individuals there. This study demonstrates the existence of periodic social behavior, but also considerable variation in association between tiger sharks, which we argue may help to mitigate any long-term impacts of provisioning on this population. Finally, we illustrate the utility of combining telemetry and social network approaches for assessing the impact of human disturbance on wildlife behavior.
Introduction
Shark dive ecotourism has grown significantly as an enterprise over the last two decades, bolstering support for the argument that sharks are more valuable alive than they are dead where ecotourism is viable (Gallagher and Hammerschlag, 2011; Cisneros-Montemayor et al., 2013; Gallagher et al., 2015). This argument resonates particularly when we consider that many of these iconic, large-bodied species are often the focus of ecotourism dive ventures, but are continuing to decline amid widespread and persistent overexploitation (Worm et al., 2013; Queiroz et al., 2019; MacNeil et al., 2020; Pacoureau et al., 2021).
To ensure reliable experiences can be offered to paying clients, the provisioning of food to attract sharks to divers is commonplace (Meyer et al., 2021). This has led to debate within the public and scientific community as to whether the potential economic and conservation advantages outweigh the possible negative impacts, which might include changes in shark behavior, increased human-wildlife conflict, increased prevalence of disease, or a possible reliance of sharks on provisioned food sources (Semeniuk and Rothley, 2008; Brena et al., 2015; Gallagher et al., 2015; Macdonald et al., 2017). At face value, shark ecotourism appears to be a conservation “win-win” providing localized protection to species, while generating local income and employment (of particular importance in developing countries) and raising public awareness of imperiled species (Apps et al., 2018). Since the initial boom of these tourism operations, considerable research effort has focused on the potential ecological impacts of this industry, resulting in a number of species-specific studies exploring the influence of shark dive tourism on movement ecology (Hammerschlag et al., 2012), residency patterns (Mourier et al., 2020), trophic ecology (Abrantes et al., 2018), community composition (Clarke et al., 2013), field metabolic rates (Barnett et al., 2016), and harmful human-wildlife encounters such as shark bites [see Brena et al. (2015), Gallagher et al. (2015) for reviews]. On balance, each operation, as well as each species/ecosystem response to dive ecotourism are different. Past research examining the ecological implications of provisioning have ranged from negligible behavioral impacts (e.g., Hammerschlag et al., 2017) to community-level reorganization (Brunnschweiler et al., 2014) of large shark species, suggesting that further research is needed to begin to build a framework for predicting the consequences of different types of provisioning ecotourism.
Diverse behavioral tactics, in conjunction with the ability of many species of apex predatory sharks to undertake long-range movements, make quantifying the potential impacts of seasonal provisioning challenging. In some instances, these very characteristics might buffer these species from any persistent biological impacts. For example, individuals with large activity spaces (and no or diffuse core areas) are unlikely to be exposed to the same intensity of provisioning as site-attached sharks such as reef sharks (Mourier et al., 2020). At a well-studied dive tourism site in The Bahamas, tiger sharks (Galeocerdo cuvier) appear to neither change their long-range migratory behavior – compared to sharks from areas unimpacted by human activities – nor their short-term diel space use in response to provisioning (Hammerschlag et al., 2012, 2017). By contrast, equally wide-ranging white sharks (Carcharodon carcharias) have been found to shift their three dimensional, fine-scale, space use to spend more time in close proximity to the dive boats during berleying (chumming) activities that attract individuals to shark cage-diving operators, with sharks spending significantly more time in close proximity to the dive boats (Huveneers et al., 2013). Broad variation in provisioning practices, species ecology and habitats indicate that only through the investigation of more contexts and species will any widespread predictable impacts be revealed.
While the impacts of provisioning on individual shark behavior appears to vary both within and among species (Brena et al., 2015), impacts at the group level on species that form aggregations (groups of sharks forming on a regular to semi-regular basis) or engage in social association behavior (i.e., non-random co-occurrence in space and time) are less well studied (Becerril-García et al., 2019). With increasing numbers of shark populations found to feature social associations (Jacoby et al., 2012; Mourier et al., 2018) and compelling evidence that conditioning can occur leading to anticipatory behaviors at dive sites (Bruce and Bradford, 2013; Clarke et al., 2013; Heinrich et al., 2021), understanding both the direct and indirect impacts of provisioning on the potential social structuring of shark groups remains an important and unexplored area of research. Indeed, compelling evidence from long-term studies on highly social cetaceans (Indo-Pacific bottlenose dolphins, Tursiops aduncus) suggests that tourist provisioning can have significant implications beyond simple changes in space use and movement. Specifically, daily provisioning of dolphins (Tursiops aduncus) in Shark Bay, Western Australia, negatively affected the reproductive success of female dolphins through reduced parental care, changes in calf foraging behavior, and higher calf mortality (Mann et al., 2000). However, long-term monitoring and Before-After-Control-Impact approaches to the evolution of tourism practices, has proven crucial for this species in Shark Bay, not least because it has been tailored to the ecological nuances of the species in question (e.g., slow life histories; complex, structured societies that allow for the social transmission of behaviors), resulting in positive changes to management practices and feeding protocols in the area (Foroughirad and Mann, 2013). Management of Shark Bay dolphins suggests that significant progress could be made by working closely with dive operators and managers to refine tourism activities for other areas and species. In addition, such studies emphasize the importance of understanding the complexities of species responses to provisioning at both the individual and population level, which has implications for human safety, and is particularly important for social species (Gallagher and Huveneers, 2018). For shark dive tourism, these group-level responses remain largely unexplored. Social network analyses can offer important insights that will likely help to reveal the extent to which social behavior and social structure might provide a degree of resilience against potential anthropogenic impacts (Snijders et al., 2017) or conversely reveal the potential for indirect costs associated with shark dive tourism.
Here, we use long-term acoustic tracking (across years) and social network inference to explore the potential existence of social behavior in a wide-ranging, generalist, marine apex predator, as well investigating the group-level impacts of dive tourist food provisioning on the patterns of association in tiger sharks. Large numbers of female tiger sharks occur naturally, particularly during colder months (November to April), on the northwestern edge of Little Bahama Bank, The Bahamas (Hammerschlag et al., 2012). Within the region is a popular dive site, nicknamed “Tiger Beach”, where tiger sharks are chummed and provisioned regularly at specific locations to support shark dive tourism that occurs almost exclusively during colder months, coinciding with the seasonally high numbers of tiger sharks found there (Hammerschlag et al., 2017).
Thought to be predominantly solitary for large proportions of their life histories, tiger sharks are observed to aggregate predictably at this female-dominated site. Although the specific reasons for this aggregation are not fully resolved, it is hypothesized that subadult females may benefit from reduced male harassment and the warm shallow waters may aid gestation for pregnant females (Sulikowski et al., 2016). Regardless of the reasons for this aggregation, high densities of sharks facilitate the potential for non-random associations and social preferences to form. Here social preferences are defined as pairs of individuals occurring together in space and time more than would be expected from chance, after controlling for individual patterns of space use and an individual’s propensity to group with others (hereafter termed “gregariousness”). It is not yet known whether tiger sharks exhibit such preferential associations with conspecifics at Tiger Beach or elsewhere, nor is it known whether provisioning might influence potential structuring of associations within the population. What is known, however, is that tiger sharks at this location occur at densities that are higher than usual for large apex sharks, raising the prospect that sociality might exist within the population. Indeed, non-random co-occurrences have been observed in another large, apex shark (white sharks, Carcharodon carcharias) when observed aggregating at pinniped colonies off South Australia, where provisioning for shark dive tourism also occurs (Schilds et al., 2019). Given these recent findings and the widespread evidence of social structuring in more site-attached and smaller shark species (Mourier et al., 2018), it is not inconceivable that tiger sharks structure themselves through non-random associations.
In this study, we use tracking data from acoustic receivers on the northwestern edge of Little Bahama Bank to explore whether tiger sharks form non-random associations with one another, and if such associations differ at provisioning and non-provisioning sites at Tiger Beach. We took a tiered approach to analyze social behavior (from aggregation, to individual gregariousness, to distinct social preferences – all defined above) to address the following hypotheses: (1) provisioning increases the level of aggregation behavior at dive sites, (2) tiger sharks are capable of forming non-random social associations maintained by individual social preferences that break down when food is made available at the dive sites, and (3) social preferences are assorted by size.
Materials and Methods
Study Site
This study was carried out on the north-western side of Little Bahama Bank, which extends off Grand Bahama Island, The Bahamas (Figure 1A). Formed of relatively shallow carbonate platforms, this area is predominantly sand flats interspersed with patches of seagrass and coral. On the bank edge, lies an area known as Tiger Beach (26.86° N, 79.04° W) where dive operators have been reliably operating shark dives since 2003. Sharks are attracted using crates of minced fish and on occasion are fed fish carcasses during dives. This activity occurs at several key dive sites (used to define our assignment of provisioned or non-provisioned receivers) predominantly during the colder months (i.e., the subtropical dry season; November through April), to coincide with the seasonal occurrence of large female tiger sharks that dominate the site (Hammerschlag et al., 2017). Up to seven dive tourism boats operate at Tiger Beach, with four regular live-a-board vessels operating weekly during the dry season, and provisioning occurring during daylight hours. While obtaining information on precisely when and where all shark diving activity occurred was not possible, we were able to obtain summaries from the logbook from one regular operator, which was used to infer shark diving provisioning activity. The logbook, while not overlapping entirely with the study period, contained 163 entries (tourism events) between 1 Nov 2013–16 Oct 2015 (714 days). The average duration of presence at dive sites was 7.33 h per day (range 1:10–17:00 h) all during daylight hours and predominantly during the dry season. Given that this log represents just one of four regular vessels, we estimate that during the dry season there is likely at least one vessel chumming at the dive sites during all available daylight hours.
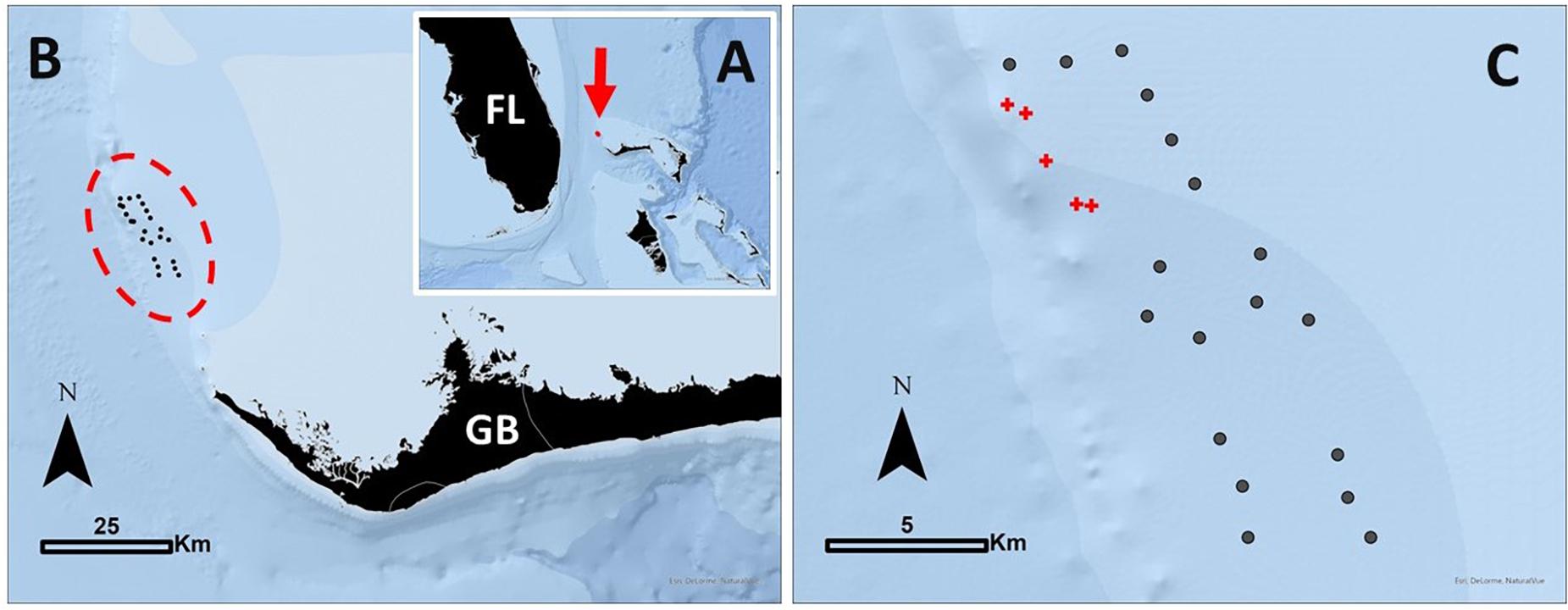
Figure 1. (A) The location of the study area in the northern Bahamas identified with a red arrow. FL, Florida, United States, as a spatial reference. (B) Positioning of the telemetry array on the north-western edge of the Little Bahama Bank, off Grand Bahama [GB] Island. The 23 receivers used in this study are outlined in a red dashed oval. (C) Receivers were arranged in a roughly 12 km × 3 km rectangle, with the western line just inshore of the bank edge. Receivers in locations exposed to provisioning from commercial shark dive operations are identified with red crosses, referred to in the text as provisioning [P] sites. All other receivers are identified with gray circles, referred to in the text as non-provisioning [NP] sites.
Shark Tagging and Acoustic Telemetry
Tiger sharks were predominantly tagged near Tiger Beach in Grand Bahama (n = 41), but several individuals that frequent Tiger Beach were tagged in Florida (n = 2) and South Carolina (n = 5) using the same methodology. Sharks were captured using standardized circle-hook drum-lines following Tinari and Hammerschlag (2021), and acoustic transmitters (Vemco V16, 69 kHz, 68 × 16 mm, 60–90 s nominal delay) were surgically implanted in the body cavity of tiger sharks as per Hammerschlag et al. (2017) while the sharks were in tonic immobility (Kessel and Hussey, 2015). Individual sex was recorded, total length (TL) was measured, and reproductive status of each individual at the time of tagging was determined from a combination of ultrasonography and hormone analysis of blood samples (see Sulikowski et al., 2016 for details).
An acoustic array of 32 VR2W receivers (VEMCO Division, AMIRIX systems) were installed by June 2014, with receivers anchored to the seafloor approximately 750 m apart in a 12 km × 3.2 km rectangular format (Figure 1). Due to receiver failure, the final array of functioning receivers to the completion of the study consisted of 23 receivers (Figures 1B,C). This included receivers placed within the proximity of four primary dive sites at Tiger Beach which were considered provisioned receivers (n = 5, Figure 1C). Diurnal range testing revealed that on average receivers had a detection efficiency of 50% at 200 m. See Hammerschlag et al. (2017) for more detail on the study site, receivers and tagging procedures.
Data Manipulation and Social Network Construction
Data from the receivers were downloaded every 6 months and raw detections were filtered to remove false detections which can arise from tag collisions (i.e., when two tags within a receiver’s range ping at the exact same time) and from acoustic pollution (Simpfendorfer et al., 2015). A time-series of the detection data was then visually inspected to determine the final data set, which was chosen as the time window that maximized the overlap of individuals at liberty within the array. This window spanned Nov 2014–Oct 2017 providing three complete years of data. We removed the first 24 h of data for any individuals that were tagged during this study period (∼15% of studies individuals). We deemed 24 h as sufficient as tiger sharks are known for being robust to capture and handling, exhibiting a muted capture stress response (Gallagher et al., 2014a, b). Previous studies have revealed strong seasonal trends in the detection of tiger sharks at Tiger Beach with increased detection probabilities during the cold, “dry” season (Nov–April) relative to the warmer “wet” season (May–Oct). There are no apparent diel differences in shark use of this area (Hammerschlag et al., 2017). Consequently, the data were divided into dry and wet seasons in addition to “non-provisioned” (hereafter, NP) and “provisioned” (hereafter, P) sites for each year, producing 12 subsets of data, grouped by year, season, and provisioning status, in which social structure was explored.
Importantly, provisioning occurred predominantly during the dry season when sharks were already there in high densities; provisioning during the wet season was negligible; however, the distinction between NP and P receivers was retained into the wet season to account for any carryover effects between seasons and to ensure that each network represented an aggregation of six months of data.
To infer social associations from the telemetry data, a Gaussian mixture model (GMM) was first applied to the acoustic time-series to identify clustering events at each location where multiple sharks were detected within the same receiver range (approximately 200 m) at the same time, signified by temporally and spatially overlapping clusters of detections (Jacoby et al., 2016). Crucially, the GMM retrieves clusters of detections that vary in duration, which likely better reflects the fact that some pairs of individuals may socialize for short periods while others might socialize for tens of minutes to hours. This approach also nullifies the subjective assignment of a specific and fixed temporal sampling window, favoring instead that this is determined by the distribution of the data (Psorakis et al., 2015). Using the “gmmevents” function in the R package asnipe (Farine, 2013), a group-by-individual bipartite graph was generated across the relevant receiver locations combined, which outlined individual co-occurrences through time. The GMM was applied separately to all 12 subsets of the data and a matrix of association extracted using the Simple Ratio Index (SRI).
Analysis of Social Data
Aggregation and Group-Level Social Structure
From the GMM metadata, the frequency of clustering events, that is the number of times that two or more sharks were deemed to be aggregating at a location based on their detection profile, and typical duration of these events were explored qualitatively between NP and P sites across the three years of the study. The median, interquartile ranges, 95% confidence intervals and density spread of the data were visualized using violin plots. We then used a chi-squared test to assess whether the frequency of aggregations was dependent on whether provisioning occurred at a receiver location or not. Finally, we tested for differences in the mean duration of these aggregations between NP and P receivers with independent Mann-Whitney U tests on the dependent variable of aggregation duration (min). To explore the potential relationship between the number of aggregation events as a function of distance from the nearest provisioning site we used a GLM with a quasipoisson (log) link function to account for overdispersion in the count data. The models were run for both dry and wet seasons separately to determine whether there was a spatial influence of provisioning in both seasons.
To explore the overall structuring of the sharks across each of the data subsets, we extracted the weighted degree (node strength, Si) of individuals present within the subset networks and compared the mean Si to that of 30,000 randomized networks – constrained to swaps within location – using the “network_permutation” function in the package asnipe (Farine, 2013). Importantly the null model included all individuals that were detected within the subset which resulted in networks (both observed and null) containing unconnected nodes (i.e., individuals within that area that did not participate in any aggregation behavior). Mean Si of our observed networks that fell within the upper or lower 2.5% threshold for our posterior null distribution were deemed to be highly structured, and significantly more or less connected than might be expected by chance (two tailed test). Those networks that showed significant non-random structure were then explored in greater detail to determine whether social preferences between conspecifics were driving this structure. Binomial logistic regressions were used to explore whether shark attribute data [number of individuals, mean and standard deviation of size (TL)], presence or absence of provisioning, while controlling for year as a random effect, were predictive of non-random social structure as a binary response variable.
Gregariousness, Social Preferences and Assortment
To explore non-random networks in more detail, we were interested in testing and consequently controlling for possible non-social drivers of social network structure. To do so we used generalized affiliation indices (GAIs) that use the deviance residuals from a generalized linear model with binomial error structure as an indication of significant dyadic affiliations or avoidances (Whitehead and James, 2015). To explore the role of individual variation in gregariousness on social network structure we first calculated pairwise gregariousness between individuals (Godde et al., 2013), using the equation, Gab = log(ΣSRIaΣSRIb), as implemented in Perryman et al. (2019) where ΣSRI are the sums of all simple ratio indices for individuals a and b (0 being individuals that had exactly the same level of gregariousness with all other conspecifics). Pairwise gregariousness took the form of a matrix that corresponded to the SRI matrix of association derived from the GMM. A size matched matrix was also constructed to reflect the pairwise distance in meters between individual site preferences. For each individual, the receiver location that recorded the highest number of detections was used as a proxy for individual site preferences. The distance matrix was produced from an edge list of coordinates using the “distm” function in the package geosphere (Hijmans et al., 2017).
Matrices of pairwise gregariousness and site preference dissimilarity were then regressed (with 5000 permutations) against the adjacency matrix of SRIs using Multiple Regression Quadratic Assignment Procedure (MRQAP) implementing the double semi-partialing, DSP approach advocated by Dekker et al. (2007). This enabled us to determine whether social network structure of any non-random networks was predicted by these non-social, potentially confounding variables. Estimates of social affiliations and avoidances (GAIs) were then derived as the residuals from our regression of significant structural predictor variables on our association indices for each network (Whitehead and James, 2015) using the “assoc.gfi” in the Animal Network Toolkit Software (ANTs) package in R (Sosa et al., 2020). Controlling for both gregariousness and site preference like this we then constructed networks of tiger shark social preferences. Networks were visualized and edge density extracted using the igraph package in R (Csardi and Nepusz, 2006).
To explore size assortment, tiger sharks were categorized into three size classes based on their total length (TL). Previous analysis of reproductive hormones and use of ultrasonography on tiger sharks at this site, suggest that size of sexual maturity is typically at 300 cm (Sulikowski et al., 2016). Therefore, sharks were assigned to a size class of “small” (TL < 300 cm), “medium” (TL = 300–350 cm), or “large” (TL > 350 cm) corresponding to immature, recently matured, and matured older individuals and the assortnet package in R was used to calculate weighted assortativity (Farine, 2014). This was then compared to a null distribution from an edge-swap permutation test. This way we could test whether social preferences (GAIs) were assorted non-randomly based on size class.
Results
Aggregation and Group-Level Social Structure
The final acoustic data set consisted of 154,897 detections from 48 different tiger sharks (Table 1). The mixture models revealed 9201 aggregation events in total across three years, 23 locations [including both non-provisioned (NP; n = 3389) and provisioned (P; n = 5812) sites] and both seasons each year. Aggregation events occurred more frequently at provisioned sites during the provisioning (dry) season (χ2 = 153.61, d.f. = 2, p < 0.001, Figure 2A). Regardless of season, these aggregations typically lasted twice as long at P sites over NP sites (Dry: W = 5816600, p < 0.01, Wet: W = 432652, p < 0.05, Figure 2B). There was no significant effect of distance from the nearest provisioning site on the number of aggregations in either the dry season (GLM: NS p = 0.599) or the wet season (GLM: NS p = 0.533, Figure 2C).
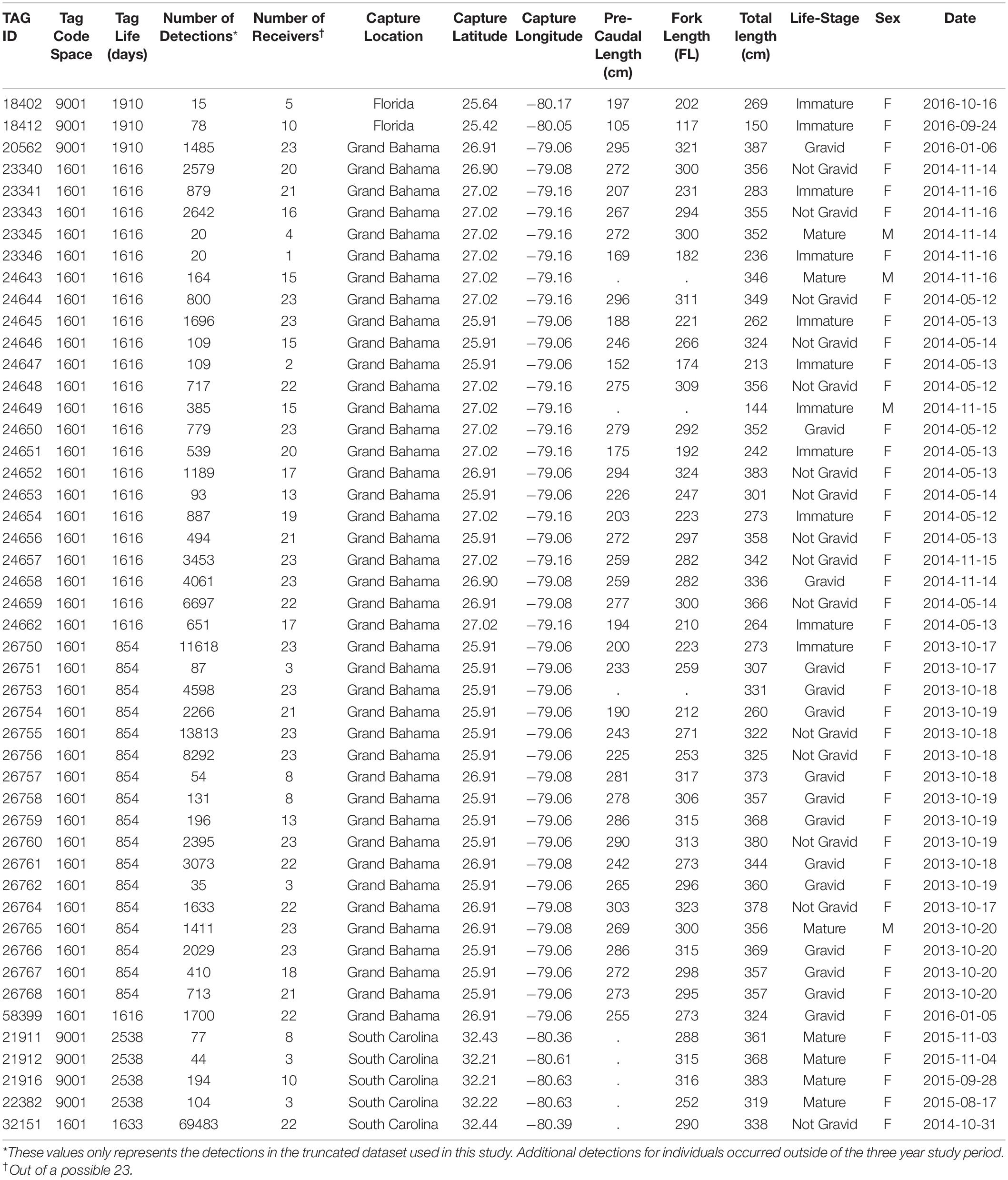
Table 1. Summary of the 48 tiger sharks tagged with acoustic transmitters that occurred during our three-year study at Tiger Beach, The Bahamas.
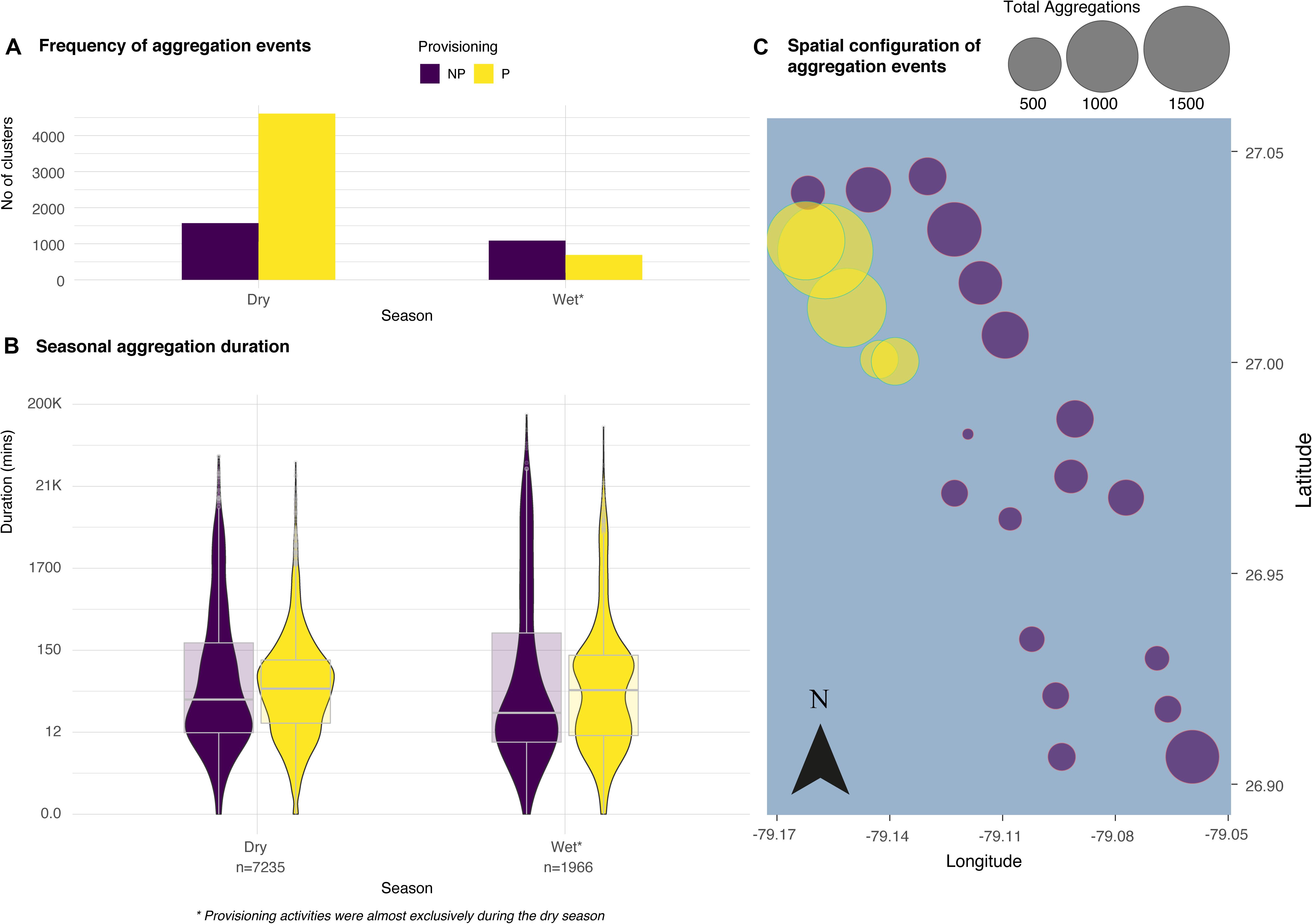
Figure 2. The frequency, duration and configuration of aggregations derived from spatio-temporal clustering events from the GMM between non-provisioned and provisioned sites from data spanning three years. The bar chart (A) reveals more frequent clustering events at provisioned locations and the violin plots (B) illustrate the median, interquartile range, 95% confidence interval and spread of the typical duration (in min, log scale) of aggregations between NP and P (n represents the number of aggregations per year). No relationship was found between the number of aggregations at each receiver and the distance to the nearest provisioning site (C).
Of the 12 networks split by season and year, five had a mean weighted degree higher than would be expected by chance (p < 0.025, two tailed), meaning that seven of the 12 networks were characterized by random assortment and mixing. Of the five non-random networks, three were at locations not impacted by provisioning (NP) in either season, one represented receivers influenced by active provisioning during the dry season (Dry P) and one at the same receivers but during the wet season (Wet Pneg) when provision activity is negligible (Table 2). Mean weighted degree was higher at NP sites for all seasons and all years, than P sites. The binomial logistic regression revealed that the number of sharks within the network, as a proxy for shark density (p = 0.225), nor the mean (p = 0.883) or standard deviation (p = 0.475) of shark size, nor the presence of provisioning (p = 0.492) were predictive of whether non-random social structure was found.
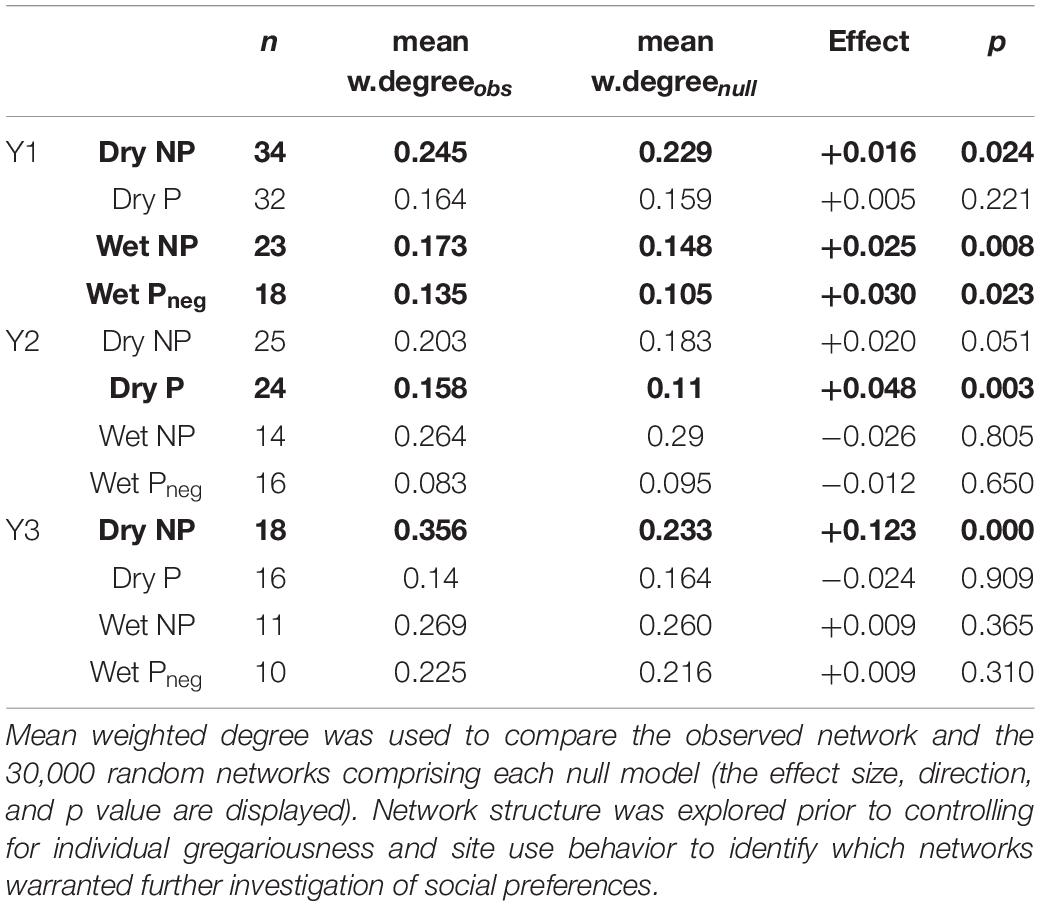
Table 2. Testing for non-random social structure across season and provisioned (P)/non-provisioned (NP) sites monitored by acoustic receivers (note Pneg indicates the wet season when provisioning was negligible).
Gregariousness, Social Preference and Assortment
Site preference was not predictive of network structure within any of the five non-random networks, and pairwise gregariousness was predictive of just three (Table 3). Gregariousness appeared to be more variable at the provisioned site [CV: NP = −34.71 (mean); Pneg = −20.41, P = −50.33]. Significant social preferences (visualized in Figure 3) represent the positive GAIs with edge weights indicative of the relative strength of those affiliations. For those receivers impacted by provisioning, either directly during the dry season or indirectly during the wet season through possible carryover effects and low level provisioning, network density was typically lower (NPmean = 0.277, Pmean = 0.199) representing a near 10% decrease in connectivity of social preferences at P sites. Finally, there was no evidence that social preferences were assorted by size class either at NP sites (r = 0.056, 0.139, and −0.102 all NS) or at P sites (r = −0.112 and 0.094, both NS). Interestingly, there was surprising little year-to-year or season-to-season consistency in pairwise GAIs, as well as high variation in within-individual GAI scores (i.e., an individual’s level of preference to all other individuals within any given time period, Figure 3). Because reproductive status was only determined at tagging, we excluded any statistical analyses that included this information.
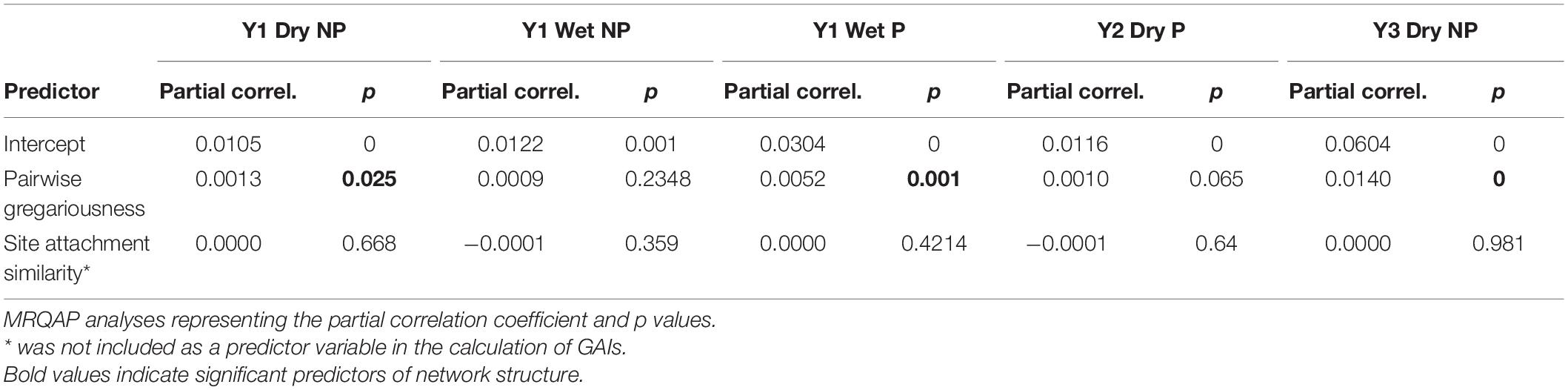
Table 3. Matrix regression of non-social predator variables on association matrices across the five non-random networks.
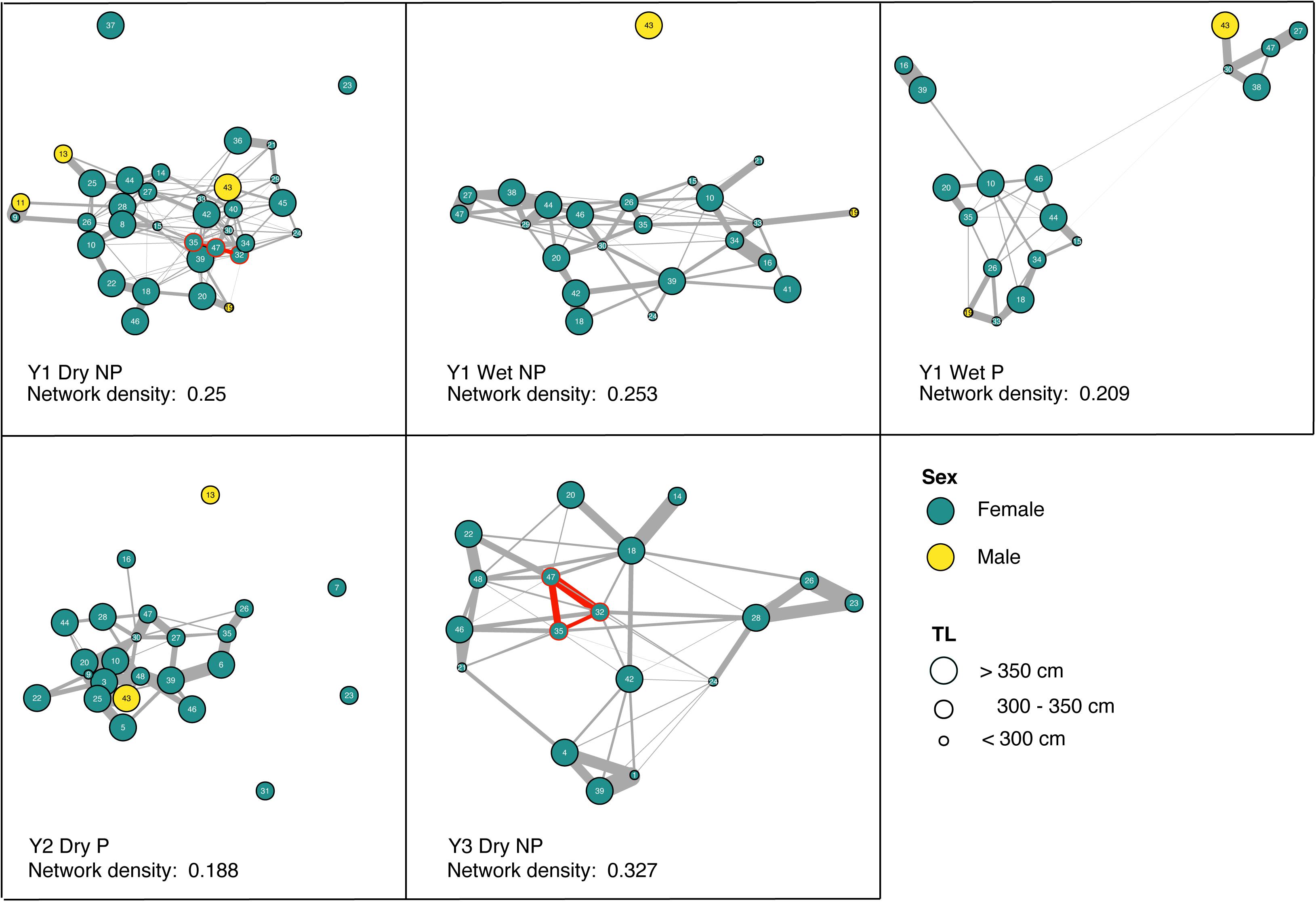
Figure 3. Generalized Affiliation Indices (GAIs) indicating social preferences between tiger sharks within the five non-random social networks. Edge weight (gray) is indicative of the strength of social preference, while node color indicates sex and node size, the size class of the individual. Network density (0–1 scale) is reported illustrating the relative connectedness of the network, where 1 would indicate a network with all conspecifics preferentially associated with all others and 0, no social preferences exist at all. Red nodes and edges illustrate a particular triad of tiger sharks that preferentially socialize in both years 1 and 3.
Discussion
Sharks are a valuable commodity within the dive tourism industry (Gallagher and Hammerschlag, 2011) and there are potential conservation benefits to be gained through these practices (Vianna et al., 2012; Macdonald et al., 2017; Apps et al., 2018). Attracting wide-ranging, apex marine predators in high densities to areas through food provisioning however, may have unintended consequences at both the individual and group level (Brena et al., 2015), and it is the latter that we still know very little about. In this study we demonstrate for the first time that tiger sharks, often considered a solitary nomadic species, are highly flexible in their capacity to associate with one another, and that provisioning of food for tourism can enhance gregarious behavior, as well as subtly influence the level of social behavior within the population.
Supporting our first hypothesis, provisioning activities increased the frequency of aggregations during the dry season when this practice was most prevalent. The provisioning site also featured longer durations of aggregations year-round. This might be indicative of continued effects during much lower levels of provisioning and/or possible anticipatory aggregation during the wet season. With no evidence of a linear reduction in the number of aggregations with distance from provisioning activity, this might simply be evidence that this particular area of Tiger Beach is highly suitable for tiger sharks, for example offering increased natural foraging opportunities, thus supporting higher numbers. In partial support of our second hypotheses, we demonstrated that tiger sharks are capable of sociality but that at Tiger Beach this sociality is highly variable: sometimes they mix randomly with one another and at other times aggregate in ways that are structured by distinct social preferences. Only 1 of 3 possible networks, demonstrated non-random social structure at provisioning locations during times of the year when provisioning occurred (Y2 Dry P), while 4 of 9 networks were non-random when provisioning was minimal or non-existent. Consequently, the probability of social preferences was not detectably different at provisioned and non-provisioned locations, but statistical power remains relatively low, as does our knowledge of the number of untagged sharks that might complicate this picture. It is also important to mention that our knowledge of the scale of provisioning activity is not perfect due to a lack of information. While difficult to conclusively determine whether non-random preferences at provisioned sites were the result of provisioning or natural preferences (regardless of provisioning), the social preference networks that did occur at provisioned sites (both dry and wet) were less well connected, indicated by lower network density suggestive of a qualitative reduction in strength and diversity of associations amongst individuals. Finally, our third hypothesis was rejected following no evidence of assortment based on individual size categories indicative of maturity and age.
Aside from regular provisioning and natural prey sources, Tiger Beach appears to provide other benefits to female tigers sharks which may include a potential refuge site from male harassment for sub-adult and gravid female sharks and warm shallow waters that could aid female gestation (Sulikowski et al., 2016). These could explain the heavy female bias of the population at this location. Aside from one individual male tiger shark (“43,” Figure 3), the small number of males that showed social preferences were more loosely connected to the network providing further evidence of socially mediated segregation and female refuging behavior as a potential male avoidance strategy in elasmobranchs (Sims et al., 2001; Wearmouth and Sims, 2008; Jacoby et al., 2010).
Differences in the reproductive status of females co-occurring at this site (Sulikowski et al., 2016) may explain the high variability in site preferences seen amongst these wide-ranging individuals which appeared not to influence the formation of social preferences. Most tagged individuals in this study did appear to be detected on a high proportion of the available receivers (Table 1) suggesting that these are genuine preferences for specific locations rather than limited use of the overall area. In a previous study, tiger sharks at this location tagged with Smart Position and Temperature Transmitting (SPOT) tags were found to travel as far as 3500 km from Tiger Beach and exhibited a collective activity space of 8549 km2 (Hammerschlag et al., 2012). It is thus unsurprising then that individuals have different site preferences within Tiger Beach, perhaps determined by timing of arrival, density of conspecifics or human presence. Wide-ranging movements may also explain why space use and diel movement patterns were found to be relatively unimpacted by provisioning in this species (Hammerschlag et al., 2017), compared to highly site-attached and resident species of elasmobranchs that exhibit marked shifts in behavior in response to provisioning (Fitzpatrick et al., 2011; Mourier et al., 2020).
The mixed and emerging results within this study, which are perhaps emphasized by the largely nomadic and solitary nature of this species, indicate that social preferences amongst conspecifics are, in fact, preferences. The occurrence of social preferences within this population might be entirely dependent on the composition of sharks that arrive at Tiger Beach attracted either by the promise of regular food during the dry season or the presence of warm sheltered waters that could be beneficial given their reproductive state. Interestingly, the preferences of individual sharks varied across seasons and years. In spite of a largely similar suite of individuals present in non-random networks that we detected, as well as some pairs of individuals that prefer one another across multiple spatial or temporal network representations (Figure 3), high pairwise-associations of individuals varied considerably across these networks. A lack of year-to-year or season-to-season consistency in pairwise preferences, as well as high within-individual variation in GAI scores, seems to imply that generally social preferences are not particularly long-lasting. The occurrence of a triad of social preferences between three individuals in year 1 (“47,” “35,” and “32,” Figure 3), which appeared again at the NP sites in year 3, however, is indication that perhaps under the certain conditions, social preferences among individuals are able to reform within future aggregations. The putative benefits of long-term, preferential associations remains an interesting area of investigation in tiger sharks, particularly in the context of a recent study that demonstrated possible foraging benefits to such long-term stable preferences in reef sharks (Papastamatiou et al., 2020).
Studies of sociality in another large, apex predatory sharks (white sharks, Carcharodon carcharias), have also produced mixed results. Within natural aggregations of this species around pinniped colonies in South Africa, biological traits (e.g., sex and size) were a more important determinant of aggregation than social preferences (Findlay et al., 2016). Conversely, at a pinniped colony in Southern Australia which also supports shark cage-diving operators, similar photo-identification methods revealed four distinct communities of white sharks underpinned by non-random co-occurrences of individuals (Schilds et al., 2019). With only a proportion of the population tagged with acoustic transmitters in our study, the results here reveal only a component of the social behavior within this population. More in depth examination of dyadic and triadic preferences, as well as exploration of the longevity of sociality and within the context of reproduction for this species in the future, may help to tease apart some of the ecological drivers of these affiliations (Perryman et al., 2019; Papastamatiou et al., 2020).
Our binomial regression indicated that neither provisioning, shark density nor size were predictive of whether non-random networks formed. Significant structure during the dry season in years 1 and 3, and a marginally non-significant result from the permutation test in year 2 (Table 2), however, suggest that social structuring may occur naturally under higher densities of individuals (e.g., dry season) which can shape the formation of social traits within a population (Webber and Vander Wal, 2018). Indeed, our proxy for density was limited to the number of tagged individuals within a network (our independent variable with the regression models) and while this was not predictive of non-random structure, it did appear to be the most likely candidate; a result that perhaps reflects the fact that social structure was also underpinned by numerous associations between tagged/untagged and untagged/untagged individuals. The interpretation that the provisioning activity at Tiger Beach is not pervasive enough to influence the long-term structuring of the population through social associations, is not unreasonable. However, further data from this species from areas completely free of tourism, which would serve as a full control location, would be useful for comparison of network metrics in the future.
In summary, we revealed that provisioning influences the opportunities for tiger sharks to socialize by promoting a higher turnover of aggregations and increased mixing resulting in lower likelihood of social preferences forming. How the sharks respond to this disruption, however, appears quite nuanced and variable. It is plausible that the social flexibility demonstrated here may buffer the population, to some extent, from any long-term changes to social behavior at the group level.
The impacts of dive tourist food provisioning on shark biology and behavior should continue to be assessed on a case by case basis (Brena et al., 2015; Gallagher et al., 2015). We advocate that such assessments should also evaluate the impacts of provisioning at the group level and in ways that incorporate the social ecology of the species in question (Foroughirad and Mann, 2013; Meyer et al., 2021). Social network analyses offer a useful toolkit for the quantitative appraisal of such impacts as they consider behavior at both the individual and group/population level. Applied here, we were able to partially reveal the social complexity (and flexibility) of a wide-ranging, “solitary” marine apex predator and demonstrate that the impacts of provisioning on gregariousness and social behavior were limited both spatially (to the specific dive locations) and temporally (to predominantly the dry season when most diving occurs). By continuing to limit provisioning activities to certain times of year, our study suggests that tourism is unlikely to be significantly disruptive to the structuring of the tiger shark population at Tiger Beach. The extent to which that may hold true elsewhere remains unclear.
Data Availability Statement
Raw acoustic tracking data are archived in the Ocean Tracking Network (Project V2LUMI): https://members.oceantrack.org/OTN/project?ccode=V2LUMI.
Ethics Statement
This work was conducted under permits from the Bahamas Department of Marine Resources, South Carolina Department of Natural Resources, Florida Fish and Wildlife Conservation Commission, National Oceanic and Atmospheric Administration and the University of Miami Institutional Animal Care and Use Committee (Protocol #18-154).
Author Contributions
NH and DJ conceived the study. NH obtained funding and alongside AG and BrF conducted fieldwork. MH and SC loaned equipment for the study. BeF contributed to data analysis. DJ conducted the data analysis and wrote the manuscript. All authors contributed to manuscript editing and writing.
Funding
DJ was funded by the Bertarelli Foundation through the Bertarelli Programme in Marine Science and through Research England. SC was supported by the Canada Research Chairs Program and NSERC. NH was supported by the Batchelor Foundation. Equipment and field work were supported by the Batchelor Foundation and the Disney Conservation Fund. Twenty receivers were obtained through a grant from the Ocean Tracking Network via support from the Canada Foundation for Innovation.
Conflict of Interest
The authors declare that the research was conducted in the absence of any commercial or financial relationships that could be construed as a potential conflict of interest.
Publisher’s Note
All claims expressed in this article are solely those of the authors and do not necessarily represent those of their affiliated organizations, or those of the publisher, the editors and the reviewers. Any product that may be evaluated in this article, or claim that may be made by its manufacturer, is not guaranteed or endorsed by the publisher.
Acknowledgments
We thank Captain Jim Abernethy and the past and present crew of Jim Abernethy Scuba Adventures who provided logistical support for this research, in particular George Hughes, Jamin Martineli, Jay Castellano, Matt Heath, and Mike Black. For donating their time and expertise as well as enabling receiver deployments and data downloads, thank you to Carl Hampp, Bill Parks, Cheryl Carroll, and Angela Rosenberg. This research benefited greatly from the dedicated contributions of all the University of Miami’s Shark Research and Conservation Program team leaders and interns who assisted infield expeditions as well as data organization for this project, especially Stephen Cain, Mitchel Rider, and Abby Tinari. Data organization was facilitated by the FACT Network and the Ocean Tracking Network. We thank the International SeaKeepers Society as well as the owners, captain, and crew of the yachts Fugitive, Penne Mae, and Xiang for providing boats for this research.
References
Abrantes, K. G., Brunnschweiler, J. M., and Barnett, A. (2018). You are what you eat: examining the effects of provisioning tourism on shark diets. Biol. Conserv. 224, 300–308. doi: 10.1016/j.biocon.2018.05.021
Apps, K., Dimmock, K., and Huveneers, C. (2018). Turning wildlife experiences into conservation action: can white shark cage-dive tourism influence conservation behaviour? Mar. Policy 88, 108–115. doi: 10.1016/j.marpol.2017.11.024
Barnett, A., Payne, N. L., Semmens, J. M., and Fitzpatrick, R. (2016). Ecotourism increases the field metabolic rate of whitetip reef sharks. Biol. Conserv. 199, 132–136. doi: 10.1016/j.biocon.2016.05.009
Becerril-García, E. E., Hoyos-Padilla, E. M., Micarelli, P., Galván-Magaña, F., and Sperone, E. (2019). The surface behaviour of white sharks during ecotourism: a baseline for monitoring this threatened species around Guadalupe Island, Mexico. Aquat. Conserv. Mar. Freshw. Ecosyst. 29, 773–782. doi: 10.1002/aqc.3057
Brena, P. F., Mourier, J., Planes, S., and Clua, E. (2015). Shark and ray provisioning functional insights into behavioral, ecological and physiological responses across multiple scales. Mar. Ecol. Prog. Ser. 538, 273–283. doi: 10.3354/meps11492
Bruce, B. D., and Bradford, R. W. (2013). The effects of shark cage-diving operations on the behaviour and movements of white sharks, Carcharodon carcharias, at the Neptune Islands, South Australia. Mar. Biol. 160, 889–907. doi: 10.1007/s00227-012-2142-z
Brunnschweiler, J. M., Abrantes, K. G., and Barnett, A. (2014). Long-term changes in species composition and relative abundances of sharks at a provisioning site. PLoS One 9:e86682. doi: 10.1371/journal.pone.0086682
Cisneros-Montemayor, A. M., Barnes-Mauthe, M., Al-Abdulrazzak, D., Navarro-Holm, E., and Sumaila, U. R. (2013). Global economic value of shark ecotourism: implications for conservation. Oryx 47, 381–388. doi: 10.1017/S0030605312001718
Clarke, C., Lea, J. S. E., and Ormond, R. F. G. (2013). Changing relative abundance and behaviour of silky and grey reef sharks baited over 12 years on a Red Sea reef. Mar. Freshw. Res. 64, 909–919.
Csardi, G., and Nepusz, T. (2006). The igraph software package for complex network research. InterJournal Complex Syst. 1695, 1–9. doi: 10.3724/sp.j.1087.2009.02191
Dekker, D., Krackhardt, D., and Snijders, T. A. B. (2007). Sensitivity of MRQAP tests to collinearity and autocorrelation conditions. Psychometrika 72, 563–581. doi: 10.1007/s11336-007-9016-1
Farine, D. R. (2013). Animal social network inference and permutations for ecologists in R using asnipe. Methods Ecol. Evol. 4, 1187–1194. doi: 10.1111/2041-210X.12121
Farine, D. R. (2014). Measuring phenotypic assortment in animal social networks: weighted associations are more robust than binary edges. Anim. Behav. 89, 141–153. doi: 10.1016/j.anbehav.2014.01.001
Findlay, R., Gennari, E., Cantor, M., and Tittensor, D. P. (2016). How solitary are white sharks: social interactions or just spatial proximity? Behav. Ecol. Sociobiol. 70, 1735–1744. doi: 10.1007/s00265-016-2179-y
Fitzpatrick, R., Abrantes, K. G., Seymour, J., and Barnett, A. (2011). Variation in depth of whitetip reef sharks: does provisioning ecotourism change their behaviour? Coral Reefs 30, 569–577. doi: 10.1007/s00338-011-0769-8
Foroughirad, V., and Mann, J. (2013). Long-term impacts of fish provisioning on the behavior and survival of wild bottlenose dolphins. Biol. Conserv. 160, 242–249. doi: 10.1016/j.biocon.2013.01.001
Gallagher, A. J., and Hammerschlag, N. (2011). Global shark currency: the distribution, frequency, and economic value of shark ecotourism. Curr. Issues Tour. 14, 797–812. doi: 10.1080/13683500.2011.585227
Gallagher, A. J., and Huveneers, C. P. M. (2018). Emerging challenges to shark-diving tourism. Mar. Policy 96, 9–12. doi: 10.1016/j.marpol.2018.07.009
Gallagher, A. J., Orbesen, E. S., Hammerschlag, N., and Serafy, J. E. (2014a). Vulnerability of oceanic sharks as pelagic longline bycatch. Glob. Ecol. Conserv. 1, 50–59. doi: 10.1016/j.gecco.2014.06.003
Gallagher, A. J., Serafy, J. E., Cooke, S. J., and Hammerschlag, N. (2014b). Physiological stress response, reflex impairment, and survival of five sympatric shark species following experimental capture and release. Mar. Ecol. Prog. Ser. 496, 207–218. doi: 10.3354/meps10490
Gallagher, A. J., Vianna, G. M. S., Papastamatiou, Y. P., Macdonald, C., Guttridge, T. L., and Hammerschlag, N. (2015). Biological effects, conservation potential, and research priorities of shark diving tourism. Biol. Conserv. 184, 365–379. doi: 10.1016/j.biocon.2015.02.007
Godde, S., Humbert, L., Côté, S. D., Réale, D., and Whitehead, H. (2013). Correcting for the impact of gregariousness in social network analyses. Anim. Behav. 85, 553–558. doi: 10.1016/j.anbehav.2012.12.010
Hammerschlag, N., Gallagher, A. J., Wester, J., Luo, J., and Ault, J. S. (2012). Don’t bite the hand that feeds: assessing ecological impacts of provisioning ecotourism on an apex marine predator. Funct. Ecol. 26, 567–576. doi: 10.1111/j.1365-2435.2012.01973.x
Hammerschlag, N., Gutowsky, L. F. G., Gallagher, A. J., Matich, P., and Cooke, S. J. (2017). Diel habitat use patterns of a marine apex predator (tiger shark, Galeocerdo cuvier) at a high use area exposed to dive tourism. J. Exp. Mar. Bio. Ecol. 495, 24–34. doi: 10.1016/j.jembe.2017.05.010
Heinrich, D., Dhellemmes, F., Guttridge, T. L., Smukall, M., Brown, C., Rummer, J., et al. (2021). Short-term impacts of daily feeding on the residency, distribution and energy expenditure of sharks. Anim. Behav. 172, 55–71. doi: 10.1016/j.anbehav.2020.12.002
Hijmans, R., Williams, E., Vennes, C., and Hijmans, M. (2017). Geosphere: Spherical Trigonometry. R Package. Available online at: https://CRAN.R-project.org/package=geosphere
Huveneers, C., Rogers, P. J., Beckmann, C., Semmens, J. M., Bruce, B. D., and Seuront, L. (2013). The effects of cage-diving activities on the fine-scale swimming behaviour and space use of white sharks. Mar. Biol. 160, 2863–2875. doi: 10.1007/s00227-013-2277-6
Jacoby, D. M. P., Busawon, D. S., and Sims, D. W. (2010). Sex and social networking: the influence of male presence on social structure of female shark groups. Behav. Ecol. 21, 808–818. doi: 10.1093/beheco/arq061
Jacoby, D. M. P., Croft, D. P., and Sims, D. W. (2012). Social behaviour in sharks and rays: analysis, patterns and implications for conservation. Fish Fish. 13, 399–417. doi: 10.1111/j.1467-2979.2011.00436.x
Jacoby, D. M. P., Papastamatiou, Y. P., and Freeman, R. (2016). Inferring animal social networks and leadership: applications for passive monitoring arrays. J. R. Soc. Interface 13:20160676. doi: 10.1098/rsif.2016.0676
Kessel, S. T., and Hussey, N. E. (2015). Tonic immobility as an anaesthetic for elasmobranchs during surgical implantation procedures. Can. J. Fish. Aquat. Sci. 72, 1287–1291. doi: 10.1139/cjfas-2015-0136
Macdonald, C., Gallagher, A. J., Barnett, A., Brunnschweiler, J., Shiffman, D. S., and Hammerschlag, N. (2017). Conservation potential of apex predator tourism. Biol. Conserv. 215, 132–141. doi: 10.1016/j.biocon.2017.07.013
MacNeil, M. A., Chapman, D. D., Heupel, M., Simpfendorfer, C. A., Heithaus, M., Meekan, M., et al. (2020). Global status and conservation potential of reef sharks. Nature 583, 801–806. doi: 10.1038/s41586-020-2519-y
Mann, J., Connor, R. C., Barre, L. M., and Heithaus, M. R. (2000). Female reproductive success in bottlenose dolphins (Tursiops sp.): life history, habitat, provisioning, and group-size effects. Behav. Ecol. 11, 210–219. doi: 10.1093/beheco/11.2.210
Meyer, L., Barry, C., Araujo, G., Barnett, A., Brunnschweiler, J. M., Chin, A., et al. (2021). Redefining provisioning in marine wildlife tourism. J. Ecotourism 1–20. doi: 10.1080/14724049.2021.1931253
Mourier, J., Claudet, J., and Planes, S. (2020). Human-induced shifts in habitat use and behaviour of a marine predator: the effects of bait provisioning in the blacktip reef shark. Anim. Conserv. 24, 230–238. doi: 10.1111/acv.12630
Mourier, J., Lédée, E., Guttridge, T., and Jacoby, D. M. P. (2018). “Network analysis and theory in shark ecology–methods and applications,” in Shark Research: Emerging Technologies and Applications for the Field and Laboratory, eds J. C. Carrier, M. R. Heithaus, and C. A. Simpfendorfer (Boca Raton, FL: CRC Press), 337–356.
Pacoureau, N., Rigby, C. L., Kyne, P. M., Sherley, R. B., Winker, H., Carlson, J. K., et al. (2021). Half a century of global decline in oceanic sharks and rays. Nature 589, 567–571. doi: 10.1038/s41586-020-03173-9
Papastamatiou, Y. P., Bodey, T. W., Caselle, J. E., Bradley, D., Freeman, R., Friedlander, A. M., et al. (2020). Multiyear social stability and social information use in reef sharks with diel fission-fusion dynamics. Proc. Biol. Sci. 287:20201063. doi: 10.1098/rspb.2020.1063
Perryman, R. J. Y., Venables, S. K., Tapilatu, R. F., Marshall, A. D., Brown, C., and Franks, D. W. (2019). Social preferences and network structure in a population of reef manta rays. Behav. Ecol. Sociobiol. 73:114. doi: 10.1007/s00265-019-2720-x
Psorakis, I., Voelkl, B., Garroway, C. J., Radersma, R., Aplin, L. M., Crates, R. A., et al. (2015). Inferring social structure from temporal data. Behav. Ecol. Sociobiol. 69, 857–866. doi: 10.1007/s00265-015-1906-0
Queiroz, N., Humphries, N. E., Couto, A., Vedor, M., da Costa, I., Sequeira, A. M. M., et al. (2019). Global spatial risk assessment of sharks under the footprint of fisheries. Nature 572, 461–466. doi: 10.1038/s41586-019-1444-4
Schilds, A., Mourier, J., Huveneers, C., Nazimi, L., Fox, A., and Leu, S. T. (2019). Evidence for non-random co-occurrences in a white shark aggregation. Behav. Ecol. Sociobiol. 73:138. doi: 10.1007/s00265-019-2745-1
Semeniuk, C. A. D., and Rothley, K. D. (2008). Costs of group-living for a normally solitary forager: effects of provisioning tourism on southern stingrays Dasyatis americana. Mar. Ecol. Prog. Ser. 357, 271–282. doi: 10.3354/meps07299
Simpfendorfer, C. A., Huveneers, C., Steckenreuter, A., Tattersall, K., Hoenner, X., Harcourt, R., et al. (2015). Ghosts in the data: False detections in VEMCO pulse position modulation acoustic telemetry monitoring equipment. Anim. Biotelemetry 3:55. doi: 10.1186/s40317-015-0094-z
Sims, D., Nash, J., and Morritt, D. (2001). Movements and activity of male and female dogfish in a tidal sea lough: alternative behavioural strategies and apparent sexual segregation. Mar. Biol. 139, 1165–1175. doi: 10.1007/s002270100666
Snijders, L., Blumstein, D. T., Stanley, C. R., and Franks, D. W. (2017). Animal social network theory can help wildlife conservation. Trends Ecol. Evol. 32, 567–577. doi: 10.1016/j.tree.2017.05.005
Sosa, S., Puga-Gonzalez, I., Hu, F., Pansanel, J., Xie, X., and Sueur, C. (2020). A multilevel statistical toolkit to study animal social networks: the animal network toolkit software (ANTs) R package. Sci. Rep. 10:12507. doi: 10.1038/s41598-020-69265-8
Sulikowski, J. A., Wheeler, C. R., Gallagher, A. J., Prohaska, B. K., Langan, J. A., and Hammerschlag, N. (2016). Seasonal and life-stage variation in the reproductive ecology of a marine apex predator, the tiger shark Galeocerdo cuvier, at a protected female-dominated site. Aquat. Biol. 24, 175–184. doi: 10.3354/ab00648
Tinari, A. M., and Hammerschlag, N. (2021). An ecological assessment of large coastal shark communities in South Florida. Ocean Coast. Manag. 211:105772. doi: 10.1016/j.ocecoaman.2021.105772
Vianna, G. M. S., Meekan, M. G., Pannell, D. J., Marsh, S. P., and Meeuwig, J. J. (2012). Socio-economic value and community benefits from shark-diving tourism in Palau: a sustainable use of reef shark populations. Biol. Conserv. 145, 267–277. doi: 10.1016/j.biocon.2011.11.022
Wearmouth, V. J., and Sims, D. W. (2008). Chapter 2 sexual segregation in marine fish, reptiles, birds and mammals behaviour patterns, mechanisms and conservation implications. Adv. Mar. Biol. 54, 107–170. doi: 10.1016/S0065-2881(08)00002-3
Webber, Q. M. R., and Vander Wal, E. (2018). An evolutionary framework outlining the integration of individual social and spatial ecology. J. Anim. Ecol. 87, 113–127. doi: 10.1111/1365-2656.12773
Whitehead, H., and James, R. (2015). Generalized affiliation indices extract affiliations from social network data. Methods Ecol. Evol. 6, 836–844. doi: 10.1111/2041-210X.12383
Keywords: behavioral ecology, ecotourism, gregariousness, predators, shark diving, social affiliations, sociality, tiger shark (Galeocerdo cuvier)
Citation: Jacoby DMP, Fairbairn BS, Frazier BS, Gallagher AJ, Heithaus MR, Cooke SJ and Hammerschlag N (2021) Social Network Analysis Reveals the Subtle Impacts of Tourist Provisioning on the Social Behavior of a Generalist Marine Apex Predator. Front. Mar. Sci. 8:665726. doi: 10.3389/fmars.2021.665726
Received: 09 February 2021; Accepted: 04 August 2021;
Published: 03 September 2021.
Edited by:
David W. Sims, Marine Biological Association of the United Kingdom, United KingdomReviewed by:
Charlie Huveneers, Flinders University, AustraliaNick Humphries, Marine Biological Association of the United Kingdom, United Kingdom
Copyright © 2021 Jacoby, Fairbairn, Frazier, Gallagher, Heithaus, Cooke and Hammerschlag. This is an open-access article distributed under the terms of the Creative Commons Attribution License (CC BY). The use, distribution or reproduction in other forums is permitted, provided the original author(s) and the copyright owner(s) are credited and that the original publication in this journal is cited, in accordance with accepted academic practice. No use, distribution or reproduction is permitted which does not comply with these terms.
*Correspondence: David M. P. Jacoby, d.jacoby@lancaster.ac.uk; davidjacoby1@gmail.com
†ORCID: David M. P. Jacoby, 0000-0003-2729-3811; Austin J. Gallagher, 0000-0003-1515-3440; Michael R. Heithaus, 0000-0002-3219-1003; Steven J. Cooke, 0000-0002-5407-0659; Neil Hammerschlag, 0000-0001-9002-9082