- 1GEOMAR - Helmholtz Centre for Ocean Research, Kiel, Germany
- 2Kiel University, Kiel, Germany
- 3University of Bremen, Bremen, Germany
- 4AWI - Alfred Wegner Institute for Polar and Ocean Research, Bremerhaven, Germany
Earth System Sciences have been generating increasingly larger amounts of heterogeneous data in recent years. We identify the need to combine Earth System Sciences with Data Sciences, and give our perspective on how this could be accomplished within the sub-field of Marine Sciences. Marine data hold abundant information and insights that Data Science techniques can reveal. There is high demand and potential to combine skills and knowledge from Marine and Data Sciences to best take advantage of the vast amount of marine data. This can be accomplished by establishing Marine Data Science as a new research discipline. Marine Data Science is an interface science that applies Data Science tools to extract information, knowledge, and insights from the exponentially increasing body of marine data. Marine Data Scientists need to be trained Data Scientists with a broad basic understanding of Marine Sciences and expertise in knowledge transfer. Marine Data Science doctoral researchers need targeted training for these specific skills, a crucial component of which is co-supervision from both parental sciences. They also might face challenges of scientific recognition and lack of an established academic career path. In this paper, we, Marine and Data Scientists at different stages of their academic career, present perspectives to define Marine Data Science as a distinct discipline. We draw on experiences of a Doctoral Research School, MarDATA, dedicated to training a cohort of early career Marine Data Scientists. We characterize the methods of Marine Data Science as a toolbox including skills from their two parental sciences. All of these aim to analyze and interpret marine data, which build the foundation of Marine Data Science.
Motivation
Earth System Sciences have seen enormous technological progress within the past decades, generating huge data sets from various sources. Increasingly, applications of big data are being used to generate policy advice, monitor regulations, and test potential mitigation measures. Using science to guide decision making requires transparent analyses and impartial communication. Uncertainties and limitations of scientific output must be clear to public, policy makers, and the media.
At the same time, Data Scientists apply, redesign, and develop new methods in statistics, data mining, and machine learning. These methods can be established to tackle specific challenges of research questions in Earth System Sciences. They have to prove their usefulness in applications to data stemming from this area of research, as it is often unknown whether the assumptions that regulate Data Science methods are met by real data from other disciplines. Therefore, combining unique non-conforming data sets not typically used together, with relevant research questions, provides a scientific benefit. Such research also serves to improve the development of data and computer science methods themselves.
We argue that it is time to integrate the fields of Earth System Sciences and Data Science. Data Science should be established as a fourth paradigm (Hey et al., 2009) in Earth System Sciences beyond observations, theory and modeling, requiring its own experts and specialists. We will present Marine Sciences as an example for Earth System Sciences since these are the areas of expertise of our consortium. We call this emerging interface field Marine Data Science (MDS) and the scientists within this field Marine Data Scientists (MDSc). To define this field and identify its needs, we conducted a workshop with eight principal investigators from both Marine and Data Sciences, and 14 doctoral candidates conducting research within MDS projects. This expert team was formed by members of a graduate school (MarDATA), which aims to educate early career MDSc and shall serve as an example of how such a pathway can be implemented.
Marine Data Science as an Emerging Field
Marine Sciences have been generating huge amounts of heterogeneous data stemming from, for example, experiments, observations, and model results including high frequency data streams (Williams et al., 2016; Mayer et al., 2018; Tanhua et al., 2019). Major advances in automated and remote observation capacity and the simultaneous collection of increasingly diverse data challenge conventional data handling methods. As more of the ocean is measured and mapped, and modeling increases in complexity, the need for innovative tools and methods will increase. Marine Scientists must extract scientific information from sparse data through smart analyses. However, Marine Scientists have little or no formal training in Data Science methods such as machine learning approaches. Data Scientists, similarly, lack formal knowledge of Marine Sciences. By merging disciplinary expertise in Marine Data Science, both groups of scientists can harness mutual advantages and provide maximal insights from complex data.
The integration of Data and Marine Sciences already takes place for numerous scientific applications but mostly in an ad-hoc manner (with the exception the established research field of bioinformatics). Successful approaches have been implemented originating in Marine Sciences (Malde et al., 2020; Sonnewald et al., 2020), as well as Data Science (Faghmous et al., 2015; Adibi et al., 2020). These approaches highlight the potential in combining the knowledge and power of these two scientific fields. They need to be differentiated from efforts like pangeo.io1 or Pangaea2. Pangeo focuses on making computer science technology (not necessarily Data Science methods) available to natural scientists. Pangaea focuses on offering a platform for publishing research data.
These examples show that establishing Marine Data Science can be approached from two perspectives: from a specialized field in Marine Sciences with an expansion toward Data Science methodologies, or from Data Science with a specialization toward the Marine Sciences.
In both cases new Data Science methods have the potential to generate added value to marine research, for example in ocean models by aiding the scientific interpretation of 4D model data. They help in improving the workflow and analysis of large volumes of model data. Also, they may help formulate and construct parameterizations of unresolved and underrepresented marine processes.
Even though we find the first way of establishing Marine Data Science important to expand the education of Marine Scientists into this new methodological field, the latter approach was the motivation for the establishment of the Helmholtz School for Marine Data Science (MarDATA)3. We view MarDATA as an example for strategic fusion of both methodologies and an application of Data Science methods in Marine Sciences.
In this paper, we present a template to establish a profile for Marine Data Scientists that offers structured training and career perspectives for researchers entering the field. In the following sections, we expand on the MDS components in Figure 1, which is followed by a concept on how to train MDSc. We provide an overview and discussion that can help both in designing and conducting MDS research, and guiding the research profile of early career researchers. This shall serve as an example of integrating Earth System Sciences and Data Science while focusing on the specific needs of Marine Sciences.
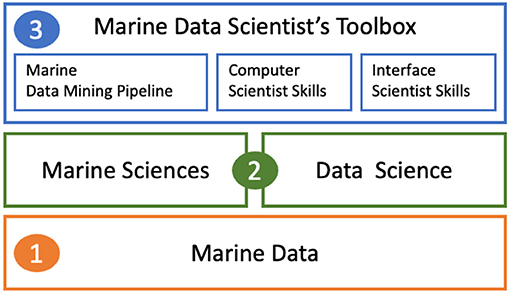
Figure 1. Elements of Marine Data Science. The object of MDS research is marine data (1). Knowledge involved in MDS bases on the parental sciences, Marine and Data Sciences (2). Key methods of MDS are collected in the Marine Data Scientist's Toolbox (3). This includes the Marine Data Mining Pipeline as well as Computer and Interface Scientist Skills.
1. Marine Data
Marine data form the base of MDS research. Since Marine Sciences include the full range of natural sciences, MDSc deal with data that originate from small-scale experiments to globally operating autonomous instruments, satellites, and ocean model outputs. In consequence, their origin, format, scope, and characteristics are diverse. It is crucial for MDSc to understand the background of these data. This includes gathering knowledge about the method of data collection and learning about the suitability of the data for answering specific research questions. Interestingly, Marine and Data Scientists may apply different criteria to evaluate the usefulness of data. Marine Scientists are concerned with the ability of their data to resolve particular processes, while Data Scientists put more emphasis on completeness, consistency, and uncertainties in the data. Both the structural organization and content of data sets are vital for analyses to reach their full potential.
Accessing data from various sources, MDSc face the challenge of combining highly heterogeneous data. This heterogeneity can affect the following areas: Sources, Data Formats and Data Structures, Origin, Processing Levels, Spatial and Temporal Resolution.
Heterogeneity of Sources, Data Formats and Data Structures arises in the absence of a single, consistent, standardized, global, and generic infrastructure for marine data. Data acquisition, processing, and accessibility depends on national efforts, and repositories are often uncoordinated. Few ongoing efforts exist that coordinate and streamline data repositories, formats, and accessibility (e.g., Ocean Observatories4, GOOS Moltmann et al., 2019, OOI Schofield et al., 2010, 2013 based on cyberstructure from Farcas et al. (2011)).
Heterogeneity of Origin distinguishes marine data by the disciplinary expertise who generated them. We identified three categories, that are not mutually exclusive:
1. Observational Data are collected and preprocessed by researchers. The researchers hold expertise in measurement devices and protocols, instrument calibration, data cleaning, and quality control. The data sources might be ship based measurements, moorings, gliders, autonomous underwater vehicles, drifters and floats, sea-floor optic cables, or laboratory measurements.
2. Highly Processed Data Products are extrapolated and interpolated in space and time, such as the objectively analyzed data of the World Ocean Atlas [WOA, (e.g,. Garcia et al., 2013; Locarnini et al., 2019)]. Remote sensing measurements can be combined with field observations. Algorithms, models and neural networks derive estimates of ocean properties, which can utilize and expand field observations.
3. Synthetic data from Simulations and Models are generated from models that are imperfect representations of the real world. They cover temporal and spatial scales beyond the observational data (e.g., Matthes et al., 2020) and include, for example, future climate projections (e.g., Eyring et al., 2016). Unlike data (1) and (2), simulation output data are usually available on a unique grid depending on the specific model simulation. Climate models, for example, typically provide a four-dimensional space-time grid. Thus, a comparison of model output and measurements always involves interpolation or data aggregation.
Heterogeneity of processing levels is concerned with the implicit uncertainty of the data in the specific level, depending on individual processing steps, as well as their underlying assumptions. The levels span raw measurements (level 0), quality-controlled data sets (level 1), derived data and data-model synthesis products (level 2 and higher) to synthetic data from simulations. Although the explicit assignment of processing levels has become common practice in Marine Science, the levels may be defined differently by scientists from different research perspectives.
Heterogeneity of spatial and temporal resolution is a common feature in ocean observations. Most field data describe properties of the upper ocean's pelagic layers (upper 500 m), where substantial variability can occur on much shorter time scales than changes in the deep ocean. Global oceanographic data from greater depth remain more scarce. Some ocean regions are still hardly covered at all, such as the southeastern Indian Ocean or the ice-covered polar oceans. Thus, observational data from great depths and from remote ocean regions are highly valuable and these data ought to be well-prepared and made accessible.
Marine data typically reflect multiple dynamical processes that are interconnected or simply overlap, while spanning a wide range of scales (Dickey, 2001). These scales can often not be regarded in isolation. Figure 2 shows the continuum of features and processes changing in time and space in the marine environment. Failing to account for heterogeneity in the spatio-temporal resolution of data may lead to misinterpretation of results. It might even mask processes of interest, potentially mistaking a relevant signal for noise.
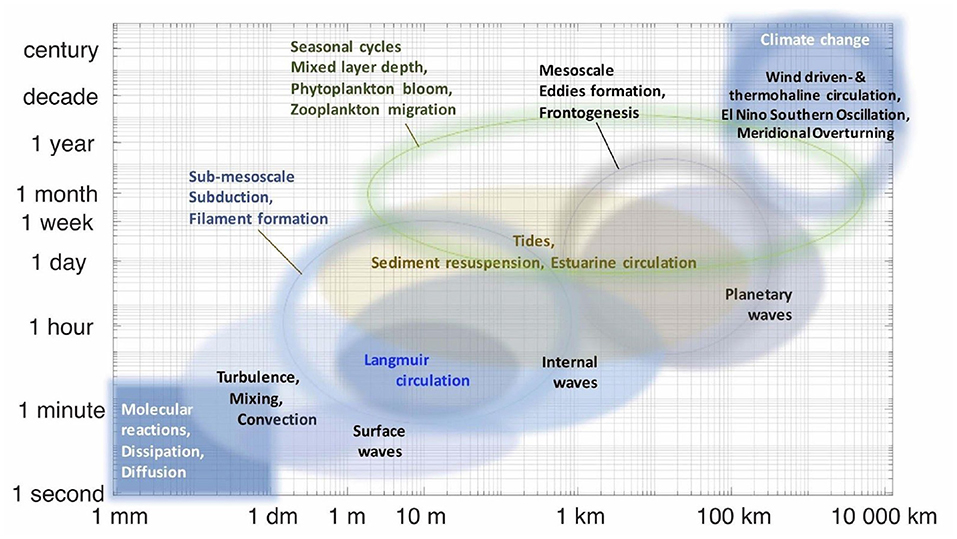
Figure 2. Processes and features that generate variability in the ocean on various temporal and spatial scales. The sketch has been refined and redrawn, inspired by an illustration by Dickey (2001).
2. Knowledge from Marine and Data Sciences
Having noted the challenges that arise from the heterogeneity of marine data, we now describe the core aspects of Marine and Data Sciences that join to form MDS.
2.1. Marine Sciences
An understanding of the ocean requires comprehension of its physical, chemical, biological, and geological processes as well as their interconnection with society. The methods and conceptual approaches of these disciplines differ substantially. They are based on mathematical, physical, biological, geological, or societal system understanding. They range from theoretical approaches, lab-based experiments and in situ measurements to global models. Consequently, specific toolboxes (methods and software) and the conceptual framework used depend strongly on the research question and the data type.
It is neither possible nor necessary for any individual MDSc to have a deep understanding of all Marine Sciences. As in any field, it is possible to understand the big questions and the areas of possible breakthrough that big data can mediate. MDSc strive to attain a meta-level understanding of marine processes, and a focused deeper knowledge of the specific research theme they work on. They know the state-of-the-art analytical tools. The tool's strengths, weaknesses, and limitations influence the framing of MDS research questions.
At all scales, ocean models help to test hypotheses and simulate projections. These simulations solve systems of differential equations, using techniques from advanced numerics, parallelization, and high performance computing. Model calibration is usually a high-dimensional nonlinear optimization problem, which requires observational data as constraints. Model parameterizations, for example of ocean mixing or biogeochemical processes, are imperfect and data assimilation methods are one option to provide improved model solutions. Typically, model optimizations require a high number of simulations, increasing the computational power requirement. Model calibration and validation is difficult because of the sparseness and uncertainty of observational data.
Increasingly, Marine Sciences address global challenges such as climate change, biodiversity loss, and the sustainable use of natural resources. Scientific advances, often enabled by data-driven insights, provide the knowledge base for policy and societal action. Examples are the predictions of climate change given by earth system models (Masson-Delmotte et al., 2018), and the development of a digital twin for the ocean that can assess solution options to marine problems (Voosen, 2020). MDSc find themselves at the forefront of this research. They are challenged not just by the research complexity, but by the need to communicate its socioeconomic and ethical implications.
2.2. Data Science
As is the case for Marine Sciences, Data Science is also not a research discipline on its own. It encompasses fields such as statistics, probability theory, or machine learning from traditional disciplines such as mathematics and computer science.
Handling and processing data involves established computer science methods. These include standard algorithms (searching and sorting, usage and manipulation of graphs, etc.) and data structures. MDSc have to understand their functionalities in order to use these tools effectively and efficiently. This also applies to data management concepts. While every domain-specific database system has its own characteristics, basic concepts such as primary and secondary keys, queries, etc. must be known and understood.
Core definitions and theorems of pure mathematics are required in essentially all fields of Data Science. A strong background in these topics forms the foundation necessary to utilize and further develop Data Science tools. Calculations on data points are basically fundamental operations on elements of fields or vector spaces. Their foundations lie in pure mathematics, algebra, and analysis. Utilizing data for finding, for example, a best procedure, requires methods from optimization or optimal control theory. Implementing these concepts into computer programs is part of numerics. Knowledge about convergence, consistency and stability of such algorithms is important to judge their suitability to address a problem as well as to judge the reliability of the result. Application of statistical methods incorporates results from probability theory, hence understanding of basic stochastic calculus is essential to choose the correct statistical method and understand its outcome. Ordinary and partial differential equations are essential to assess the existence and uniqueness of solutions of numerical models.
Finally, it is crucial to transform data into information, and ultimately into knowledge. Specifically in MDS, advanced machine learning and data mining methods that are designed for complex-structured data are required. Such data include high-dimensional data, sequence data, time series data, data streams, graph data as well as spatial and spatio-temporal data and unstructured data such as text. Awareness of the capabilities and limitations of these techniques is crucial.
3. The Marine Data Scientist's Toolbox
We next discuss the skills that guide MDSc to successfully take on their research challenges (see Figure 1). We emphasize that substantial discussion with Marine Scientists about the expected scientific achievements is essential at the start of any MDS project. Only after this exchange can the choice of the specific tool be made.
3.1. Marine Data Mining Pipeline
To facilitate knowledge discovery, MDSc work along a data mining pipeline. This includes selection, preprocessing, and transformation of the data for feature selection for the machine learning and pattern mining algorithms. It is important to emphasize that data put into this pipeline's preprocessing step have already been processed, cleaned and maybe even imputed by Marine Scientists in their own data preprocessing routines. At the end of the marine data mining pipeline stands a meaningful evaluation of model performance utilizing expressive visualization. Along this pipeline, MDSc have to cope with data sparsity, problems of overfitting, treatment of outliers and noise, and sorting and weighting data according to quality and uncertainty. Due to this complexity, knowledge discovery is tackled by an iterative process with multiple loops over the pipeline steps. In the following we will focus on aspects of this data mining pipeline applicable to MDS.
During data selection, MDSc must keep in mind the scientific question, differences in processing levels and uncertainties between data types. Close collaboration with Marine Scientists is crucial in this step. This includes consideration of boundary conditions and an assessment of the plausibility of a solution, which distinguishes this approach from blind data mining.
In the preprocessing step the data is integrated, completed, and made consistent. MDSc consider the origin, temporal and spatial coverage, available metadata, and preprocessing performed on the data. When handed to MDSc, marine data is usually already preprocessed to a higher data level (see section 1).
The next step is transformation of the data into a format for machine learning and data mining. This includes feature selection, feature transformation, and dimensionality reduction. Gaussianity can facilitate some analyses and may even be a prerequisite. It can be met for e.g., by applying Gaussian anamorphosis for improved state estimations (Amezcua and Leeuwen, 2014) or logarithmic transformations. Data that exhibit non-Gaussian characteristics might be transformed by other parametric or non-parametric statistical measures (e.g., Tsybakov, 2009).
At the heart of Data Science are knowledge discovery methods such as machine learning and pattern mining. Their application presumes familiarity with the range of the spatio-temporal scales of the data and the processes involved (see sections 1 and 2). When working with complex, multi-source data, MDSc adapt methods of data mining to account for uncertainties in the data (Liu et al., 2016).
The evaluation of the results involves experts from Marine and Data Sciences. Techniques such as explainable artificial intelligence might be more useful than black-box solutions. They facilitate communication of the results and their origins to non-Data Scientists. Although blind data mining might expose unknown and unexpected interdependencies, it requires close collaboration (see section 3.3) to assess whether identified patterns are useful. Once the quality of the results is assessed, the pipeline can be backtracked to repeat steps, applying alternative approaches.
Visualization communicates the message extracted from the data. Descriptive statistics support visualization by removing noise, and summarizing core features. Graphic and dynamic visualization are utilized to communicate results to (Marine Science) colleagues. Innovative ways of presenting or animating data contribute significantly to dissemination of results.
3.2. Computer Science and Programming Skills
To facilitate the steps of the marine data mining pipeline, MDSc apply classical programming skills such as handling databases and UNIX platforms as well as different programming languages.
Database systems build the foundation to access and store marine data in a standardized and well-defined format. MDSc know how to work with relational as well as other database designs, such as NoSQL solutions. MDSc run and parallelize analyses on diverse systems, such as High-performance architectures with numerous CPUs or GPUs and associated storage systems.
Programming languages are essential for performing computer-supported calculations and analyses. Currently, huge marine models are often written in classical programming languages like C, C++, and Fortran5. Statistical analyses and data visualization in Marine Sciences are often performed in R6, MATLAB7, and programs such as Excel8 out of convenience. For the data mining pipeline e.g., Python9 is a helpful choice.
To ensure transferability, reproducibility and sustainability of the developed scripts and software they need to be created using standard routines such as version control (e.g., git10) and modularity as well as good documentation. Hence MDSc need to apply best practices of software engineering.
3.3. Interface Scientists Skills
MDSc are scientists working across disciplines with different conceptual approaches, academic cultures, and disciplinary languages. Hence, they must develop personal and communication skills to allow them to contribute to a joint understanding of scientific questions and research design. In-depth bilateral scientific exchange between Marine and Data Scientists for co-definition of research questions and expected outcomes is the first step of any MDS project. A succinct guide to how such a collaboration could be established and nurtured is given by Ebert-Uphoff and Deng (2017). They suggest that rather than Data Scientists and Marine Scientists trying to gain proficiency in the field of the other, active and persistent collaboration is the way to join expertise. Defining the problem, approach and expectation of the scientific outcome will lead to the selection and application of appropriate data methods. Together with the interpretation of results these are a joint responsibility and must be iterated throughout the entire research process.
Interface skills for MDSc include grasping the conceptual approach and specific terminology of the marine problem while avoiding excessive detail. An innate, curiosity driven motivation, that enables research to be fun, stimulates lateral and innovative thinking and an openness for serendipity help greatly in this process. At a personal level, MDSc are continually operating beyond their comfort zone—they interact as non-experts in new fields. They must navigate among their collaborators and communicate their expertise at conferences and meetings with domain scientists. This requires confidence, questioning the input they receive and having an entrepreneurial mindset that shows resilience, determination, and an enthusiasm for multitasking.
In communication of results to stakeholders and decision makers, MDSc need to address and clarify uncertainties and limitations of their scientific output. This is fundamental to contributing MDS input to policy making and public understanding.
4. How to train a Marine Data Scientist
By defining MDS as a new research field with characteristic methods, workflows and required skills, we see the need for targeted education of early career scientists. This is motivated by Marine Data Science as an example of how Data Science could be fused with all fields of Earth System Sciences into a new interface discipline. We present here our experiences in developing and implementing targeted training for doctoral researchers in MDS within a dedicated graduate school of the Helmholtz Association. This can serve as an example of how data and earth sciences can be bridged to form a new interface discipline.
The Helmholtz School for Marine Data Science (MarDATA)11, established in 2019, aims at training doctoral candidates (the German equivalent of a Ph.D) in MDS. It is a cooperation of GEOMAR - Helmholtz Centre for Ocean Research Kiel and the Alfred Wegener Institute (AWI) Helmholtz Centre for Polar and Marine Research with partner Universities in Kiel and Bremen, and was initiated by the Helmholtz Association to prepare the next generation of scientists for a data-heavy future. Conducting MDS requires highly specialized Data Science methods, thus we chose doctoral candidates with a Masters degree in Data Sciences. Their doctoral training is conceived to provide a Marine Sciences background as well as targeted in-depth training in information and Data Sciences. They also receive training to sharpen transferable skills that enhance their research output. Their research projects range from the improvement of autonomous underwater navigation over pattern recognition in large data sets to the development of new tools for data analysis. Most of the doctoral researchers aim at a degree in Data Science that could lead to a post-doctoral career inside Earth System Sciences (not necessarily restricted to Marine Sciences), and also prepares them for a career outside academia.
The core of MarDATA is the joint definition of research questions by professors and senior scientists from both Marine and Data Sciences. Regular meetings between the doctoral candidates and both their supervisors (one each from the Marine and Data Science disciplines) have proven to be the most effective. They are essential for a joint understanding of the research question and monitoring research progress. All participants share responsibility for exchange of disciplinary understanding and maintaining useful dialogue. It quickly became apparent, however, that doctoral candidates cannot be the only “glue” between their supervisors.
MarDATA supports scientific exchange by offering joint events, such as datathons12, hacky hours13, and other networking opportunities. Doctoral researchers in the MarDATA school gain lateral, interface skills by contributing lectures or workshops to early career Marine Scientists. This contributes visibility to the profile of MDSc in the marine field.
Training measures draw on project-specific expertise from the supervisors, as well as block courses and summer schools. The training is always open for a number of external participants providing an opportunity for knowledge transfer and exchange. Recurring lecture formats allow training in particular methodologies and provide an overview of state-of-the-art research in both domains. Workshop formats allow all involved researchers to strengthen their interface skills, for example in lateral thinking and design thinking.
5. Summary and Challenges
In this paper we provide our perspective of the current state and future of Marine Data Science (MDS) as a marine example for the fusion of Earth System Sciences with Data Science. To suggest a pathway for its development, we propose a model for the training for early career Marine Data Scientists (MDSc). The discussed ideas are inspired by the Helmholtz Graduate School for Marine Data Science (MarDATA), which is an example of training for a future generation of MDSc. MDSc should be trained both in classical Data Science skills as well as developing strong communication skills across disciplines. The Data Science skills include handling databases and programming languages, while maintaining software development standards, as well as dealing with diverse marine data types. The Marine Science skills include an overview of the marine environment and the characteristics of its data. MDS potentially extricates information from marine data, leading to new knowledge, and can identify new research questions.
Despite the obvious benefits of joining forces of Marine and Data Sciences, MDS comes with its own challenges as regards perspectives for a career after the doctorate. MDSc might struggle with appropriate scientific recognition, since publication strategies in Marine and Data Sciences differ greatly. Marine Scientists usually publish in journals whereas Data Scientists mostly publish in conference proceedings. The latter do not have the same assessment-metrics as journal publications, such as the impact factor. MDSc need to position themselves between these cultures. To attain publication recognition in Marine and Data Science, there is a risk that they will need to publish double the amount expected of pure Marine or Data Scientists.
The definition and education of MDSc is only the first step. Structural change is the necessary second step. Structural support could come through involving MDSc in new projects, assigning permanent positions to MDSs, and offering a pathway to an academic career including professorships in MDS. Besides their scientific expertise, MDSc draw from a range of interface and transferable skills. These qualify MDSc also for a career path outside of academia, in innovation sectors such as business, product development, public and private research and entrepreneurship. MDSc can thus easily transition between or merge the academic with the private and public sectors.
Although this is a Marine Sciences example, we believe that similar potentials and challenges exist for any variant of Earth System Data Science.
6. Conclusion
MDS is an example of a novel and highly demanded interdisciplinary research field between the Earth System Sciences and Data Science that needs to be properly defined and established. MDS comes with a high demand on knowledge and skills from both Marine and Data Sciences to be able to effectively work with marine data. It still has to develop a strategy for publishing with best impact, and recognition to facilitate entering a academic career. Also, it has to find a balance between incorporating experiences from the parental sciences while exploring new ways of combining and advancing Marine and Data Sciences simultaneously. We envision MDSc will be able to provide major benefits in advancing Marine as well as Data Sciences, understanding marine data and bridging two distinct scientific fields.
The approach and pitfalls of establishing Marine Data Science mapped out in this paper could be used as a blueprint for establishing other fields of research that fuse Earth System Sciences and Data Science.
Data Availability Statement
The original contributions presented in the study are included in the article/supplementary material, further inquiries can be directed to the corresponding author/s.
Author Contributions
CT and M-TV initiated and guided the work, hosted the workshop, developed the structure and framework of the manuscript, its introduction and discussion, the Data Science toolbox, the Marine Science toolbox, and edited the entire manuscript. AA developed the parts about the necessary depths of Marine Science knowledge, interface skills, soft skills, and edited the entire manuscript. AB is the head of the MarDATA research school; he developed the parts about the methods usually applied in Marine Sciences for data analyses. TD developed the parts about traditional education in mathematics, software engineering, and programming. MP developed the Data Science toolbox and the Marine Science toolbox. EP developed the parts about example Ph.D projects, interface skills, and soft skills. MR developed the parts about the general knowledge on machine learning and Data Science methods and solution patterns for common data problems. MS developed the parts about marine data. CS developed the parts about marine data repositories. TS developed the parts about simulation and simulation data. All authors contributed to the article and approved the submitted version.
Funding
This work was supported by the Helmholtz School for Marine Data Science (MarDATA) funded by the Helmholtz Association (Grant HIDSS-0005).
Conflict of Interest
The authors declare that the research was conducted in the absence of any commercial or financial relationships that could be construed as a potential conflict of interest.
The reviewer RS declared a past collaboration with one of the authors AB to the handling editor.
Publisher's Note
All claims expressed in this article are solely those of the authors and do not necessarily represent those of their affiliated organizations, or those of the publisher, the editors and the reviewers. Any product that may be evaluated in this article, or claim that may be made by its manufacturer, is not guaranteed or endorsed by the publisher.
Acknowledgments
We want to thank all participants of the of the MarDATA workshop Marine Data Science: Opportunities and Challenges in November 2020 for their valuable input and enrichment of our discussion. We thank the referees and editors for their constructive feedback regarding the initial version of the manuscript.
Footnotes
3. ^MarDATA: https://www.mardata.de/
4. ^https://oceanobservatories.org/
5. ^Fortran https://fortran-lang.org/ (accessed January 10, 2021).
6. ^R Core Team (2020). R: A Language and Environment for Statistical Computing. R Foundation for Statistical Computing Vienna. Available online at: https://www.R-project.org/ (accessed January 10, 2021).
7. ^MATLAB (2010). version 7.10.0 (R2010a). Natick, MA: The MathWorks Inc. Available online at: https://de.mathworks.com/ (accessed April 25, 2021).
8. ^Microsoft Excel https://www.microsoft.com/de-de/microsoft-365/excel (accessed January 10, 2021).
9. ^Python Software Foundation. Python Language Reference, version 3.9. https://www.python.org/ (accessed January 10, 2021).
10. ^Software Freedom Conservancy. Git. https://git-scm.com/ (accessed January 10, 2021).
11. ^MarDATA: https://www.mardata.de/.
12. ^A datathon is an event where Data Scientists meet to solve Data Science challenges. These challenges can originate from applied fields or other data-heavy research disciplines.
13. ^A hacky hour is a fixed informal weekly meeting, where researchers and programmers come together to discuss and solve code and programming related problems.
References
Adibi, P., Pranovi, F., Raffaetà, A., Russo, E., Silvestri, C., Simeoni, M., et al. (2020). “Predicting fishing effort and catch using semantic trajectories and machine learning,” in Lecture Notes in Computer Science (Würzburg: Springer International Publishing), 83–99.
Amezcua, J., and Leeuwen, P. J. V. (2014). Gaussian anamorphosis in the analysis step of the enkf: a joint state-variable/observation approach. Tellus A 66:23493. doi: 10.3402/tellusa.v66.23493
Dickey, T. D. (2001). The role of new technology in advancing ocean biogeochemical research. Oceanography 14, 1078–120. doi: 10.5670/oceanog.2001.11
Ebert-Uphoff, I., and Deng, Y. (2017). Causal discovery in the geosciences using synthetic data to learn how to interpret results. Comput. Geosci. 99, 50–60. doi: 10.1016/j.cageo.2016.10.008
Eyring, V., Bony, S., Meehl, G. A., Senior, C. A., Stevens, B., Stouffer, R. J., et al. (2016). Overview of the coupled model intercomparison project phase 6 (CMIP6) experimental design and organization. Geosci. Model Dev. 9, 1937–1958. doi: 10.5194/gmd-9-1937-2016
Faghmous, J. H., Frenger, I., Yao, Y., Warmka, R., Lindell, A., and Kumar, V. (2015). A daily global mesoscale ocean eddy dataset from satellite altimetry. Sci. Data 2:150028. doi: 10.1038/sdata.2015.28
Farcas, C., Meisinger, M., Stuebe, D., Mueller, C., Ampe, T., Arrott, M., et al. (2011). “Ocean observatories initiative scientific data model,” in OCEANS'11 MTS/IEEE KONA, 1–10.
Garcia, H. E., Locarnini, R. A., Boyer, T. P., Antonov, J. I., Baranova, O. K., Zweng, M. M., et al. (2013). “World ocean atlas 2013,” in Dissolved Inorganic Nutrients (Phosphate, Nitrate, Silicate). Vol. 4, eds S. Levitus and Mishonov (NOAA Atlas NESDIS 76), 25. doi: 10.7289/V5J67DWD
Hey, A. J., Tansley, S., Tolle, K. M. (eds.). (2009). The Fourth Paradigm: Data-Intensive Scientific Discovery. (Redmond, WA; Microsoft Research). Available online at: http://fourthparadigm.org
Liu, Y., Qiu, M., Liu, C., and Guo, Z. (2016). Big data challenges in ocean observation: a survey. Pers. Ubiquitous Comput. 21, 55–65. doi: 10.1007/s00779-016-0980-2
Locarnini, R. A., Mishonov, A. V., Baranova, O. K., Boyer, T. P., Zweng, M. M., Garcia, H. E., et al. (2019). World Ocean Atlas 2018, Volume 1: Temperature. A. Mishonov (NOAA Atlas NESDIS 81), 52.
Malde, K., Handegard, N. O., Eikvil, L., and Salberg, A.-B. (2020). Machine intelligence and the data-driven future of marine science. ICES J. Mar. Sci. 77, 1274–1285. doi: 10.1093/icesjms/fsz057
Masson-Delmotte, V., Zhai, P., Prtner, H.-O., Roberts, D., Skea, J., Shukla, P., et al. (2018). Ipcc, 2018: Global Warming of 1.5c. an Ipcc Special Report on the Impacts of Global Warming of 1.5c Above Pre-industrial Levels and Related Global Greenhouse Gas Emission Pathways, in the Context of Strengthening the Global Response to the Threat of Climate Change, Sustainable Development, and Efforts to Eradicate Poverty.
Matthes, K., Biastoch, A., Wahl, S., Harlaß, J., Martin, T., Brücher, T., et al. (2020). The flexible ocean and climate infrastructure version 1 (FOCI1): mean state and variability. Geosci. Model Dev. 13, 2533–2568. doi: 10.5194/gmd-13-2533-2020
Mayer, L., Jakobsson, M., Allen, G., Dorschel, B., Falconer, R., Ferrini, V., et al. (2018). The nippon foundation—GEBCO seabed 2030 project: the quest to see the world's oceans completely mapped by 2030. Geosciences 8:63. doi: 10.3390/geosciences8020063
Moltmann, T., Turton, J., Zhang, H.-M., Nolan, G., Gouldman, C., Griesbauer, L., et al. (2019). A global ocean observing system (GOOS), delivered through enhanced collaboration across regions, communities, and new technologies. Front. Mar. Sci. 6:291. doi: 10.3389/fmars.2019.00291
Schofield, O., Glenn, S., Orcutt, J., Arrott, M., Meisinger, M., Gangopadhyay, A., et al. (2010). Automated sensor network to advance ocean science. Eos Trans. Am. Geophys.l Union 91, 345–346. doi: 10.1029/2010EO390001
Schofield, O., Glenn, S. M., Moline, M. A., Oliver, M., Irwin, A., Chao, Y., et al. (2013). Ocean Observatories and Information: Building a Global Ocean Observing Network. New York, NY: Springer, 319–336.
Sonnewald, M., Dutkiewicz, S., Hill, C., and Forget, G. (2020). Elucidating ecological complexity: Unsupervised learning determines global marine eco-provinces. Sci. Adv. 6:eaay4740. doi: 10.1126/sciadv.aay4740
Tanhua, T., Pouliquen, S., Hausman, J., O'Brien, K., Bricher, P., de Bruin, T., et al. (2019). Ocean FAIR data services. Front. Mar. Sci. 6:440. doi: 10.3389/fmars.2019.00440
Tsybakov, A. B. (2009). Introduction to Nonparametric Estimation. New York, NY: Springer Science & Business Media.
Voosen, P. (2020). Europe builds ‘digital twin’ of earth to hone climate forecasts. Science 370, 16–17. doi: 10.1126/science.370.6512.16
Keywords: Marine Data Science, interface science, emerging science, Ph.D training, Data Science, Marine Sciences, Earth System Sciences
Citation: Verwega M-T, Trahms C, Antia AN, Dickhaus T, Prigge E, Prinzler MHU, Renz M, Schartau M, Slawig T, Somes CJ and Biastoch A (2021) Perspectives on Marine Data Science as a Blueprint for Emerging Data Science Disciplines. Front. Mar. Sci. 8:678404. doi: 10.3389/fmars.2021.678404
Received: 09 March 2021; Accepted: 11 November 2021;
Published: 02 December 2021.
Edited by:
Viola Liebich, Bremen Society for Natural Sciences, GermanyReviewed by:
Robert William Schlegel, Institut de la Mer de Villefranche (IMEV), FranceMaike Sonnewald, Princeton University, United States
Copyright © 2021 Verwega, Trahms, Antia, Dickhaus, Prigge, Prinzler, Renz, Schartau, Slawig, Somes and Biastoch. This is an open-access article distributed under the terms of the Creative Commons Attribution License (CC BY). The use, distribution or reproduction in other forums is permitted, provided the original author(s) and the copyright owner(s) are credited and that the original publication in this journal is cited, in accordance with accepted academic practice. No use, distribution or reproduction is permitted which does not comply with these terms.
*Correspondence: Carola Trahms, ctrahms@geomar.de
†These authors have contributed equally to this work and share first authorship