- 1Ocean Technology and Engineering Group, National Oceanography Centre, Southampton, United Kingdom
- 2ClearWater Sensors Ltd., Westbourne, United Kingdom
We introduce for the first time a new product line able to make high accuracy measurements of a number of water chemistry parameters in situ: i.e., submerged in the environment including in the deep sea (to 6,000 m). This product is based on the developments of in situ lab on chip technology at the National Oceanography Centre (NOC), and the University of Southampton and is produced under license by Clearwater Sensors Ltd., a start-up and industrial partner in bringing this technology to global availability and further developing its potential. The technology has already been deployed by the NOC, and with their partners worldwide over 200 times including to depths of ∼4,800 m, in turbid estuaries and rivers, and for up to a year in seasonally ice-covered regions of the arctic. The technology is capable of making accurate determinations of chemical and biological parameters that require reagents and which produce an electrical, absorbance, fluorescence, or luminescence signal. As such it is suitable for a wide range of environmental measurements. Whilst further parameters are in development across this partnership, Nitrate, Nitrite, Phosphate, Silicate, Iron, and pH sensors are currently available commercially. Theses sensors use microfluidics and optics combined in an optofluidic chip with electromechanical valves and pumps mounted upon it to mix water samples with reagents and measure the optical response. An overview of the sensors and the underlying components and technologies is given together with examples of deployments and integrations with observing platforms such as gliders, autonomous underwater vehicles and moorings.
Introduction
The oceans cover over 70% of our planet and directly contribute $2.5Trillion/yr in economic benefit, which is equivalent to the world’s 7th largest economy (2016) (Hoegh-Guldberg, 2015). They provide $25Trillion in ecosystem services such as production of food. Whilst 90% of their value depends on healthy ecosystems, 30–35% of critical marine habitats are overused or have been destroyed, ocean acidity is up 26%, and oxygen is depleting in key areas (G7 Science and Technology Ministers, 2016).
Oceanic chemistry plays a central role in ecosystems through biogeochemical processes. State of the art operational Earth System/lower trophic level ecosystem models (such as Butenschoen et al., 2016) include the carbonate system and macro nutrient variables in both pelagic (water) and benthic (sediment) representations as well as iron (Fe) in the pelagic and CO2 flux between the ocean and atmosphere. Hence their measurement in both process studies and operationally are critical in the development and calibration of these models and therefore our understanding and operational awareness of ocean health. Further the outputs from such lower trophic levels can be used to force models predicting higher trophic levels from “primary producers to top predators” (Coll et al., 2020) under a range of climate and hence ocean chemistry change scenarios. Therefore, the measurement of ocean chemistry is critical to understanding the health and productivity (including fisheries and bioresources) of our oceans in a changing climate and in mitigating negative effects.
Ocean chemistry also has an impact on the marine environment’s capacity to absorb CO2 and act as a climate mitigating carbon sink (DeVries et al., 2019). Whilst the flux of CO2 at the ocean surface is a physicochemical process (Woolf et al., 2019) this is modulated by the difference in ocean and atmospheric CO2 concentration. This difference is affected by biogeochemical processes which are influenced by variations in nutrient, iron and other carbonate system chemical variables (Macovei et al., 2020) and is likewise modeled with ecosystem models (see above).
Elevated atmospheric, and therefore oceanic, CO2 concentrations also cause ocean acidification (Doney et al., 2009). This reduction of pH, currently at a global average of about 0.002 pH units per year (Bates et al., 2014; Iida et al., 2021), occurs as CO2 dissolves in the oceans to form carbonic acid (H2CO3), which readily dissociates to bicarbonate (HCO3–), carbonate (CO32–), and H+. “Impacts of unmitigated ocean acidification are estimated to represent a loss to the world economy of more than US $1 trillion annually by 2100” (Secretariat of the Convention on Biological Diversity, 2014). This includes for example reductions due to damage and critical endangerment of corals and calcifying invertebrates. Decreased pH also decreases the ability of the oceans to absorb more CO2 and hence will accelerate climate change.
Harmful Algal Blooms (HABs) can have devastating impacts on both fisheries and aquaculture with single events resulting in export losses of $800M (Trainer et al., 2020) as well as significant effects on tourism and public health (Grattan et al., 2016). Whilst their prevalence is increasing with climate change, the role of ocean chemistry is complex. In many settings ocean stratification suppresses nutrient and micronutrient supply creating conditions where HABs can outcompete other phytoplankton. But also, when dominant HAB species do encounter elevated nutrients, for example due to coastal pollution, a population explosion or bloom can then lead to widespread negative impacts (Trainer et al., 2020). Regardless of these differing mechanisms, monitoring of nutrients is a useful tool for predicting and observing HAB occurrence and mitigating their impact.
Given the importance of ocean nutrient and carbonate system variables in multiple processes with large environmental, economic and societal impact, it is perhaps not surprising that the requirement for their measurement has been highlighted by the international ocean observing community. For example, the Framework for Ocean Observing (Tanhua et al., 2019) adopted by the Global Ocean Observing System (GOOS) lists macronutrients and carbonate system variables in the top three biogeochemical measurements in terms of impact and importance to this global effort. Beyond ocean environments there are also numerous applications for example in water industries and agriculture.
Despite the value of measurement of nutrient and carbonate system variables, their routine measurement is still dominated by sample collection and laboratory analysis with concomitant high cost, low spatial and temporal resolution, and risk of systematic error from contamination or degradation of samples. There has been considerable effort in both the academic community and in industry to develop nutrient and carbonate system sensors.
Optical (direct spectrophotometry of seawater) sensors (Finch et al., 1998; Johnson and Coletti, 2002) offer reagent free, high frequency and low energy per measurement data, are commercially available (e.g., SUNA v2, Seabird Scientific, United States), robust and deployable on the small platforms such as profiling floats (Johnson et al., 2010). Whilst they currently only measure nitrate operationally, they could also access nitrite (Wang et al., 2021) using spectral chemometrics and with the addition of acid titration can also measure [CO32–] (Martz et al., 2009). However, they are prone to interference from overlapping absorption spectra of other natural water constituents, biofouling and calibration drift (Sakamoto et al., 2009; Wang et al., 2021) and are less accurate/precise (2 μM/0.3 μM; Suna V2, Seabird Scientific, United States) than traditional nutrient analysis (0.1/0.1 μM; Hydes et al., 2010; Becker et al., 2020).
Optode based in situ pH sensors have been developed by the Technical University Graz and PyroScience GmbH (Aachen, Germany) and these devices are now commercially available (PyroScience GmbH, Germany). They have accuracy in the order of 0.02 pH (Staudinger et al., 2018) and worst case drift 0.003 pH/day at 24 or 25°C, but undetectable at 10°C (Staudinger et al., 2018, 2019). The optodes consist of two essential components: a pH sensing material and the read-out module. The pH sensing material utilizes the pH-dependent fluorescence of an aza-BODIPY indicator dye (Klimant et al., 2001; Staudinger et al., 2018, 2019). The optodes have a low power consumption (∼1 mW at acquisition rate of one measurement point in 10 s) and have been demonstrated with a 2-month deployment (Fritzsche et al., 2018).
Electrochemical sensors for oceanic nutrients are not currently used for operational nutrient sensing because of metrology and robustness challenges (Wei et al., 2021). However, low limits of detection (6.1 nM) have been demonstrated in the lab for phosphate with plastic electrodes decorated with an alkyl Mo-polyoxometalate (Figueredo et al., 2021). Devices for silicate (Legrand et al., 2021) with limit of detection 0.32 μM have been deployed in shallow demonstrations and similar devices for phosphate characterized in laboratory conditions (Barus et al., 2016).
Electrochemical sensors for pH (Shitashima et al., 2002; Martz et al., 2010; Easley and Byrne, 2012; Johnson et al., 2016; Briggs et al., 2017; McLaughlin et al., 2017; Gonski et al., 2018; Miller et al., 2018; Takeshita et al., 2018; Saba et al., 2019) are the most mature and widely used in operational oceanography. This is motivated by small size and robustness (Johnson et al., 2016), low power (e.g., 340–400 mW, SeaFET v2, Seabird Scientific, United States), fast response (<5 s, MSFET 3330, Microsens Switzerland) (Flohr et al., 2021), and good metrology performance (precision 0.004 pH, accuracy ± 0.05 pH, SeaFET v2, Seabird Scientific, United States). This has resulted in use on gliders (Saba et al., 2019; Takeshita et al., 2021) and profiling floats (Takeshita et al., 2018). However, a conditioning period when immersed in a new ionic concentration (4–5 days to settle to within 0.005 of spectrophotometric reference measurements; Saba et al., 2019), biofouling (in shallow warm waters on a glider offsets of < 0.2 pH were observed Saba et al., 2019), and sensor drift (0.003 pH/month, SeaFET v2) all affect performance. Solutions to these challenges include operation downstream of antifouling chemistry used in conductivity sensors (Takeshita et al., 2021), the presentation of in situ standards to the sensor (requiring fluidics) (Bresnahan et al., 2021) or calibration in stable waters, such as in the deep-sea (Johnson et al., 2017; Takeshita et al., 2018) the latter can achieve uncertainties of 0.005 ± 0.007 but adds additional operational constraints.
Electrochemical sensors for oceanic carbonate ion (Choi et al., 2002) and Total Alkalinity (Briggs et al., 2017) have also been developed but are yet to be demonstrated for in situ deployment.
Despite these advances there remains a requirement to improve nutrient and carbonate system metrology in sensors. Because of the superior metrology performance of reagent based analytical methods (Waterbury et al., 1996; Patey et al., 2008, 2010; Ma et al., 2014a; Nagul et al., 2015; Birchill et al., 2019; Takeshita et al., 2020), numerous sensors and analytical systems have been developed employing these techniques. This includes in situ deployed systems for Nitrate (Jannasch et al., 1994; David et al., 1998; Steimle et al., 2002; Adornato et al., 2007; Vuillemin et al., 2009; Yaqoob et al., 2012; Bodini et al., 2015), Phosphate (Adornato et al., 2007; Barnard et al., 2009; Moscetta et al., 2009; Ma et al., 2014b; Bodini et al., 2015; Yang et al., 2020), Iron (Chapin et al., 2002; Johnson et al., 2007; Huang et al., 2012; Meyer et al., 2012), and pH (Waterbury et al., 1996; Bellerby et al., 2002; Martz et al., 2003; Liu et al., 2006; Nakano et al., 2006; Wang et al., 2007, 2015; Seidel et al., 2008; Assmann et al., 2011; Spaulding et al., 2011; Lai et al., 2018). Some of these systems are commercially available (Hanson, 2000; Barnard et al., 2009; Spaulding et al., 2011; Bodini et al., 2015; Lai et al., 2018). However, previously there has not been widespread uptake of these technologies for operational oceanography because of perceived and actual barriers presented by: reliability (many moving parts); fragility; complexity of operation (such as reagent preparation and pre/post deployment protocols); device size, weight and power; and significant volumes of reagent consumption and waste production.
Microfluidics technologies (Nightingale et al., 2015; Mowlem et al., 2019) have been proposed as a solution to some of these barriers and there are examples in the literature of in situ systems targeting nutrients and carbonate. These include systems for nitrate (Beaton et al., 2012; Cogan et al., 2015; Nightingale et al., 2018, 2019), phosphate (Cleary et al., 2007; McGraw et al., 2007; Slater et al., 2010; Legiret et al., 2013; Clinton-Bailey et al., 2017; Grand et al., 2017), silicate (Cao et al., 2017), iron (Milani et al., 2015; Geißler et al., 2017), and pH (Rerolle et al., 2012, 2013; Pinto et al., 2019).
The advantages of microfluidics include integrated fluidic manifolds that reduce fluidic and electrical connectors (improving reliability and robustness) as well as complexity of operation: mixers, fluidic junctions and optical cells can be included in a continuous channel within the manifold. Similarly, this integration can reduce device size, weight and power. Whilst dispersion (Aris and Taylor, 1956) increases flushing volumes beyond the internal volume of the device, reagent consumption and waste production are dramatically reduced vs. macrofluidics [e.g., from 50 mL/sample (Barnard et al., 2009) to 0.3 mL/sample (Beaton et al., 2012) total waste]. Because volumes of reagents and waste are minimized, reagent cartridges (e.g., see also Barnard et al., 2009) can be extended to include capture of all waste from extended deployments.
It is the low sample consumption enjoyed by microfluidics, that enables use of relatively small inlet filters [e.g., 0.45-μm pore size Millex HP inline filter (Millipore, United States) (Beaton et al., 2012)]. In our experience, whilst the sensors may experience biofouling externally, the filters maintain operation without blocking. This enables superior biofouling resistance/robustness compared to other technologies. Filter blocking is only seen in very high turbidity settings (e.g., in the Maumee River Johengen et al., 2016) and then only rarely, and can be dealt with using larger or multiple filters, or more frequent changes.
Small fluidic consumption also enables regular measurement of onboard fluid standards and blanks which enables improved accuracy. In addition, microfluidics also enable inclusion of multiple sensing cells (e.g., colorimetric absorption channels of differing lengths) in a single device (Beaton et al., 2012; Clinton-Bailey et al., 2017; Geißler et al., 2017; Grand et al., 2017) which can be used to extend dynamic range and optimize limits of detection. Microfluidics also enable stable laminar/low Reynolds number flows with advantages in repeatable dosing and accelerated diffusive mixing over shorter diffusion length scales. Furthermore, dispersion (Aris and Taylor, 1956) can also be used to create time or length dependent titrations (e.g., Rerolle et al., 2013) and can smooth out the effects of flow rate variations in matched pumps that would otherwise couple directly into analytical signals.
However, the use of microfluidics does not, without additional engineering measures, fully address the barriers of complexity of operation and fragility/lack of robustness common to reagent-based systems. Key challenges remain in the production of reliable and matched microfluidic flow (rates) into analytical devices to avoid variable dilution of sample with reagents or incorrect assay dosing. This requires robust and precision pump and fluidic manifold design together with well controlled valve backlash/elasticity (if valves are used). Similarly addressing complexity of operation also requires robust automation of setup, calibration, deployment, and post deployment calibration procedures.
An example of the use of microfluidics, coupled with the engineering measures to address use barriers (as above) is the in situ lab on chip (LOC) technology developed at the National Oceanography Centre (NOC), and the University of Southampton (Beaton et al., 2012; Rerolle et al., 2013; Clinton-Bailey et al., 2017; Geißler et al., 2017; Grand et al., 2017; Mowlem et al., 2018, 2019) and now produced under license by Clearwater Sensors Ltd.1 a startup and industrial partner in bringing this technology to worldwide availability and further developing its potential.
The technology is capable of making accurate determinations of chemical and biological parameters that require reagents and that produce an electrical, absorbance, fluorescence is a subset of luminescence signal. As such it is suitable for a wide range of environmental measurements. Whilst further parameters are in development across this partnership, Nitrate, Nitrite, Phosphate, Silicate, Iron and pH sensors are currently available commercially.
Theses sensors use microfluidics (Mowlem et al., 2019) and optics (Mowlem et al., 2018) combined in an optofluidic chip with electromechanical valves and pumps mounted upon it to mix water samples with reagents and measure the optical response. Each type uses a different analytical chemistry method/assay to access a different variable, but these are all based upon certified or trusted existing gold standard laboratory methods. This enables intercomparison with existing data sets and speeds transition from sample-based measurements. All of the nutrient sensors as well as the iron sensor carry with them small volumes of standards and blanks allowing in situ calibration. The pH sensor instead uses a stable and laboratory characterized indicating dye obviating the need for in situ calibration. In all of the sensors, drift is virtually eliminated and service intervals extended to just short of the lifetime of the reagents used which can be many months, or years in cold climates or in the case of pH.
The hardware differences between the sensors are minimized as all of the assays for nutrients, iron and pH use colorimetry. The only differences between the variants are the fluidic layout of the chip, the optics (e.g., LED wavelength and absorption cell pathlengths) and minor electronics differences. The latter are minimized by using multiple Printed Circuit Boards (PCBs) with the main PCB containing the microprocessor and most of the functionality common to all systems. Only smaller daughter PCBs are specific to each variant. These accommodate small differences in components, layouts and functions. This use of common components enables improved robustness and reliability (through more extensive testing and focused design effort) in addition to economies of scale. An example of the robustness enabled is the depth rating of this technology: 6,000 m for all variants offered commercially.
The technology has already been deployed by the NOC, and with their partners worldwide over 200 times including to depths of ∼4,800 m, in turbid estuaries and rivers, and for up to a year in seasonally ice-covered regions of the arctic. We briefly review some of those deployments as well as presenting new data and deployment information.
The public-private sector collaboration between the National Oceanography Centre, the University of Southampton, and the startup Clearwater Sensors Ltd. has been critical for making this technology available to the ocean observing, and wider water chemistry measurement customers/stakeholders. Without commercialization, this technology could only be produced in limited numbers and could only be deployed in collaboration. Because of the legal status of the public sector institutions, without commercialization the sensors could not be offered easily for commercial sale nor with indemnity or warranty. In addition, repetitive production of a consistent product is also at odds with research institutions remit for novelty and invention and there is a risk because of constant innovation, that the technologies developed would never be used at scale for the purpose they were developed.
In contrast commercial partnership enables: investment in productization and production, a focus on quality, engineering and testing to ensure compliance with regulations and standards (e.g., CE and ISO), customer support and documentation, warranties and services including calibration, servicing, reagent supply, installation support, communication, and data solutions. Investment can come from private funds, recycled sales income, or in limited circumstances grant funding. In the latter case some funds are only accessible if led by a company, and therefore would be inaccessible to public sector institutions without a commercial partnership.
Clearwater Sensors Ltd. has been founded by members of the Ocean Technology and Engineering Group at the National Oceanography Centre. The principal reasons for this arrangement were to ease knowledge transfer and upskilling of a commercial partner, and to reward/incentivize academic staff who could otherwise see this activity as a distraction from their core role. This has the effect of reducing the knowledge transfer burden on the public sector partners as well as shortening the time to market. It also enables the company to continue to improve the product and make innovations from a high knowledge base. To maximize the benefits for the overall partnership improvements made by the company can flow back to the public sector partners which benefits further research, and also minimizes divergence in the technology between the partners and duplication of effort. In addition, the public sector partners benefit from commercial success through license payments and other incentives for innovation (e.g., UKRI Research Excellence Framework). The public sector institutions now also have access to off the shelf products to include in research work that focuses on applications, whilst reserving their technology research and development effort for innovations and research rather tie it up with repetitive manufacture.
Materials and Methods
In this section we give details of the technology and example deployments.
Technology
A photograph of sensor (in this case the silicate variant) with cable attached is shown in Figure 1. And with reagent cartridge disassembled in Figure 2. Fully assembled the sensor is 56 cm in height. The advantage of a reagent cartridge is that the operator does not need have the analytical chemistry experience or skills required to make up reagents, standards, or blanks. The reagent cartridge can be supplied as a unit to the user and spent cartridges can be returned to Clearwater Sensors Ltd. for safe disposal of waste and refilled for subsequent deployments. The use of wet mating fluidic connectors enables cartridges to be easily swapped in the field: simplifying and shortening field servicing. For some deployments (see below) a cartridge may not be suitable because of limited space in a vehicle for example, in which case individual flexible tube linked storage bags can be used and packed into available space.
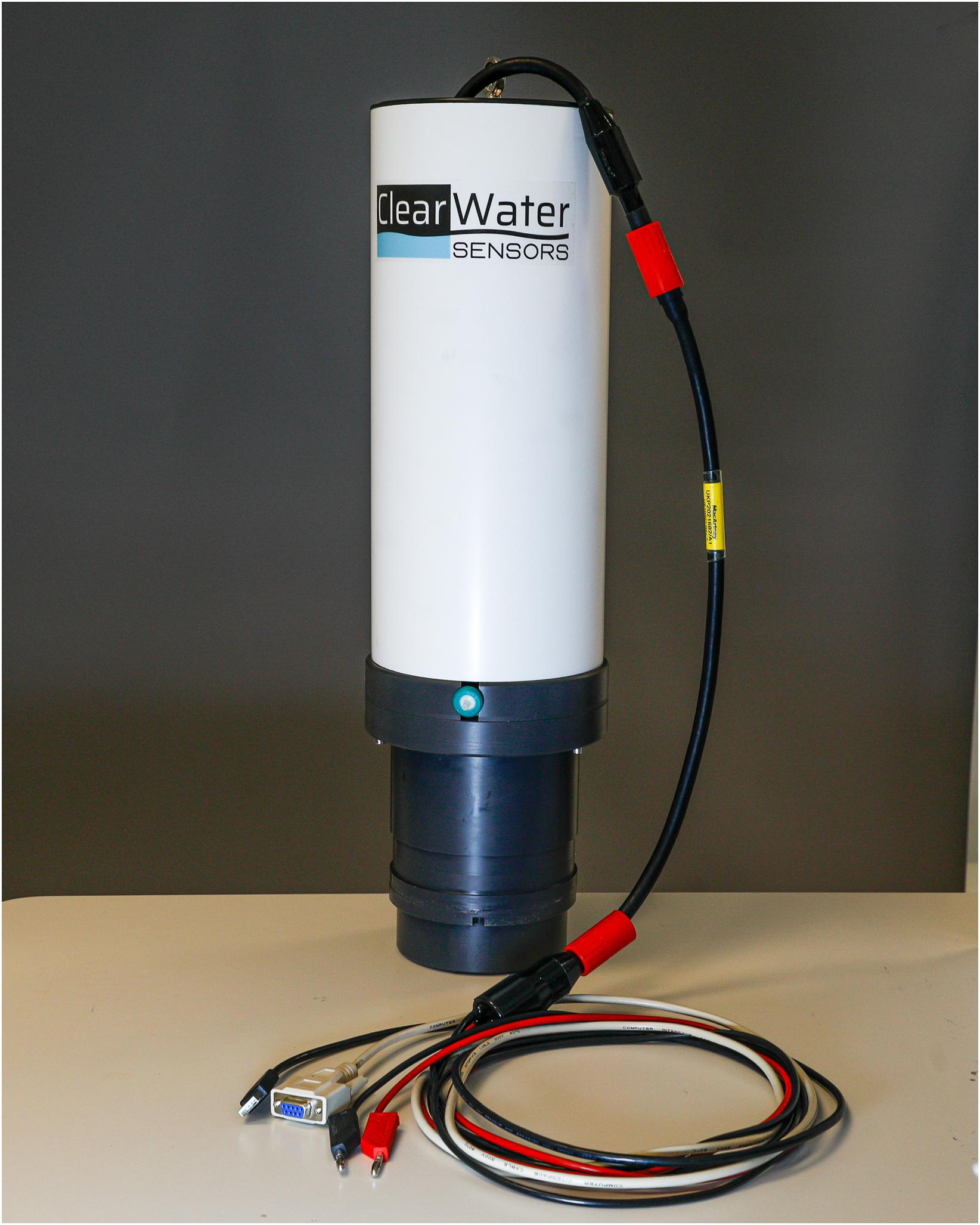
Figure 1. Photograph of a silicate sensor (Clearwater Sensors Ltd., total height 56 cm) connected to a deployment cable which is then connected to a lab cable for connection to a bench power supply and PC for testing/calibration. The USB connection is used for setup and download of raw data, while RS232 can provide real time processed data in the field. The upper part including a larger gray collar and white tube is a reagent cartridge containing standards, blanks, reagents and waste storage capacity (all waste is captured). The lower gray cylindrical part houses the microfluidics, optics, pumps, valves and electronics (the sensor itself).
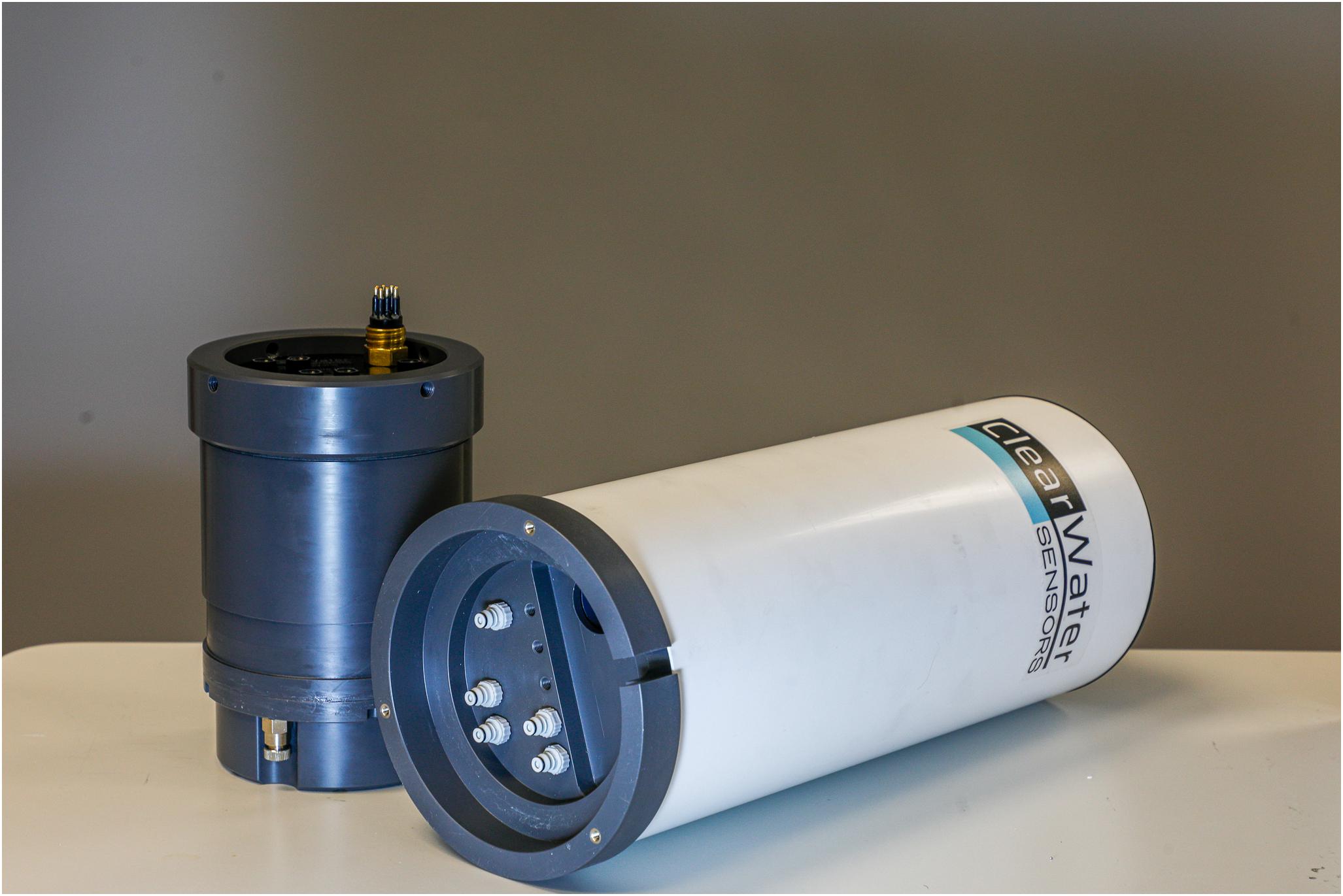
Figure 2. Photograph of a silicate sensor (Clearwater Sensors Ltd.) shown with reagent cartridge (white) separated from the sensor (gray).
The lower gray sensor’s housing has the microfluidic chip as one of its endcaps. This arrangement minimizes the fluid connections and shortens the connection to the environment. This is advantageous for minimizing fluid consumption (because of a reduction in the flushing requirement which increases with long pipes due to dispersion) and also greatly reduces risks of failure and leaks. The outer surface of the microfluidic chip has fluidic connections for the input filter (typically 0.4 μm pore size), the corresponding wet mating fluidic ports used with the reagent cartridge, and connections for off chip components (for example the reduction column for the nitrate variant). It also hosts the wet mating electrical connector (SubConn MCBH8M, MacArtney UK Ltd.).
The chip itself is formed from three layers of stray, scattered and ambient light suppressing “tinted” polymethyl methacrylate (PMMA) with tint optimized to each assay and location of each layer (Floquet et al., 2011). Channels (typically 150 μm width) and features, including pockets for Light Emitting Diodes (LEDs), photodiodes, thermistors, waveguides, encapsulated fixing nuts, and seats for pumps valves and PCBs, hydraulic pressure compensation channels and mounting holes for electrical and fluidic connectors are formed in the PMMA by micro-milling prior to solvent mediated polishing and bonding (Ogilvie et al., 2010). An advantage of this arrangement is that including multiple absorption cells of differing lengths has little impact on chip cost or production times, and this greatly improves both the limits of detection (long cells, typically 25–80 mm long) and range of linearity (short cells, typically < 1 mm long). Thus, a single chip can offer excellent metrology across wide concentration ranges with very simple and hence robust optical cells (LEDs and photodiode pairs). For example, the nitrate sensor variant has a limit of detection of 20 nM (0.28 mg/L as Nitrate) and a linear range to 1 μM (14 mg/L as Nitrate): a ratio of 1–50,000.
The sensor housing is formed from PVC, is watertight, oil filled and includes an elastomeric membrane/bellows to compensate for volume changes due to temperature and pressure variation. In this way the internals of the sensor are also at ambient (submerged) pressure and there is no differential pressure on the end cap. This cost-effective setup enables the polymer housing and chip/endcap to survive extreme ambient pressures of the deep sea (tested to 6,000 m). Polymers are also resistant to corrosion (including in high salt brines), are stable over environmental temperature and pH ranges. In addition, the inclusion of a compensating oil and bladder system enables mitigation of combined effects of pressure and temperature in both cold high-pressure deep-sea and warm low-pressure surface ocean environments. Prior to previous deployments (which are summarized in section “Applications and Deployments”) this concept was tested within the temperature controlled hyperbaric facility at NOC (−10°C to +35°C, 0–70 MPa) and optimized.
On the inner surface of the chip are mounted valves (LFNA1250125H, Lee Co., United States) and bespoke syringe pumps with multiple pump barrels driven by a single sliding plate via a stepper motor. This arrangement ensures that the ratios of reagent to fluid analyzed (sample, standard, blank) remain fixed and with very low variability: otherwise for the nutrient and iron sensors any relative variation in pump flow rates couples directly into noise in the measurement by analyte dilution with reagent. The pump is controlled with position feedback.
Also mounted on the inside of the chip are the microprocessor and daughter PCBs. The microprocessor PCB is based on the low-power Microchip (previously Atmel) SAM4L utilizing an ARM Cortex-M4 chipset (Microchip, United States) and performs all logging and control scheduling functions. It controls the pumps and valves and interfaces with the daughter boards which have variant specific combinations of LED drivers, analog electronics (for photodiodes and thermistors for on chip temperature measurement) and analog to digital conversion (16 bit). The electronics store all raw data (flash memory card) and can stream processed data when deployed over RS232 via the electrical connector (SubConn MCBH8M, MacArtney UK Ltd.). The latter enables connection to ocean vehicle/observing platforms or communication systems for near real time data relay. Clearwater Sensors Ltd also offer GSM and other communication solutions/hardware. A key feature of the electronics is their specification to work in oil at ambient pressure (tested to 6,000 m) and hence they do not need protecting in a pressure case. This has cost, weight and robustness advantages.
The sensors do not have an internal battery other than a small reserve to preserve the operation of the real time clock. The external power required is 12 v (10–16 v) and a typical power consumption of 1.8 W. Each measurement typically consumes ∼500 J and hence a deployment with 2,000 measurements of samples and/or calibration materials requires 1,000 kJ (∼23 Ah) battery capacity at operation temperature. Such batteries are widely available including in deep-sea rated versions.
The sensors are setup and programmed via a Microsoft Windows Graphical User Interface application that enables the user to pick and adjust a range or predefined schedules or to specify when the sensor is quiescent, sampling, flushing or making standard and blank measurements. These “states” can also be triggered via the RS232 interface and the GUI enables this to be setup, e.g., to respond to environmental conditions or external communications. In this way the sensors can form part of a smart sensing network.
Variants and Assays Used
Table 1 summarizes the assays used, power requirements and performance for each currently commercially available variant. In all cases the commercial versions of the instruments are developed from the research devices in the published literature and from the public sector partners know how. Changes include reduced diameter of the chip to match stock tube internal diameter of 4” (101.6 mm, from 119 mm) to aid manufacture and reduce waste (swarf) in housing production, a rework of the electronics to replace legacy/obsolete components, improved positioning and conformal coating of the electronics to enhance robustness, new valve component ported for better response to dynamic pressure changes and oil filling, new valve electrical connection.
The nitrate and nitrite sensor developed from Beaton et al. (2012, 2017b), Yuecel et al. (2015), and Vincent et al. (2018) uses the Greiss assay which detects nitrite by diazotization with sulphanilamide and subsequent coupling with N-(1-naphthyl)-ethylenediamine dihydrochloride (NED) to form an intensely colored azo dye. Nitrate is reduced to nitrite using a copperised cadmium column in the presence of an imidazole buffer. To aid safety and recyclability this is formed from a solid cadmium/copper tube that is processed to reduce internal volume and encapsulated in epoxy resin. It is attached to the chip via connecting tubes on the outside face and can therefore be removed for recycling (e.g., returned to Clearwater Sensors Ltd.). The encapsulation ensures users do not come into contact with the cadmium and concentrations of this metal in the waste are low, and all of the waste is retained and is returned together with the depleted cartridge.
The phosphate sensor, developed from Clinton-Bailey et al. (2017); Grand et al. (2017), and Birchill et al., 2019) utilizes a modified molybdenum blue assay which in its standard form (Nagul et al., 2015) is used widely to determine an operationally defined Soluble Reactive Phosphorus (SRP) frequently referred to in the literature as PO43– by convention. Key adaptations for in situ use include proprietary catalyst and surfactants able to work over the full operational temperature and salinity ranges. In contrast to the previous published literature, this improved assay enables accurate determinations below 5°C.
The silicate sensor uses a novel proprietary formulation based on the standard assay (see segmented flow analyser method in Birchill et al., 2019) which is based on the formation of silicomolybdic acid by reaction with ammonium molybdate tetrahydrate in an acidic solution including sulphuric and oxalic acids as well as surfactants and stabilizing agents. Optimisation of the assay has focused on performance over a wide range of environmental conditions including minimizing interference from phosphate.
The iron sensor developed from Geißler et al. (2017) uses an assay with a buffered ferrozine solution and a ferrozine/ascorbic acid mixture for Fe(II) and labile Fe(III) analyses, respectively. The commercial version of this sensor employs both an acidification step and a longer assay development (wait) time in the protocol as suggested in Milani et al. (2015); Geißler et al. (2017) which as anticipated resolves the low and variable recovery of DFe [Fe(II)+Fe(III)] they encountered without these modifications.
The pH sensor developed from Rerolle et al. (2013, 2018) has been fully implemented in the common lab on chip platform, including the use of on chip mounted LEDs and the 6,000 m rated infrastructure. The device uses a spectrophotometric assay using purified meta-Cresol Purple. Unlike all the other assays above, pH determination does not use in situ calibration using standards and blanks, nor does it use stopped flow analysis to allow time for reaction in an axially homogenous sample contained in an absorption cell. Instead pH is determined in continuous flow where a range of indicator dye concentrations are produced as a function of time through dispersion of an injected slug of dye upstream of the optical cell. The dye response at a range of concentrations is measured at two wavelengths corresponding approximately to the absorbance maxima of the protonated and unprotonated forms of the dye. The pH is a function of the ratio of these absorbances, but to achieve high accuracy the measured ratio of a purified and standardized dye is corrected for (a) the effects of perturbation in pH by the presence of the dye; and (b) the mismatch of the optics (ideal 578 nm vs. 590 nm in the device). The former is achieved by regressing in situ data at a range of dye concentrations back to the y intercept (zero dye) whilst the latter is corrected by laboratory calibration at a range of temperatures using high accuracy TRIS/HCl buffers. A correction is also required if the detection in the optical cell is at a different temperature to ambient. The effect of these corrections is to improve accuracy to better than 0.006 pH units at pH 8.5 with no temperature difference (≤0.009 pH units with typical temperature differences) for example. This compares to a stated accuracy of 0.050 pH units for best in class commercial ISFET based pH sensors. In addition, the drift of the spectrophotometric method is negligible.
Deployments
The sensors have been deployed over 200 times by the NOC. This has enabled the sensors to reach Technology Readiness Level 7 (TRL 7, see ISO 16290:2013) prior to commercialization through real world fault finding and robust engineering response over many years.
Because of strong user demand as well as excellent metrology performance with certified or widely accepted assays, robustness, depth capability, low power, small size, low reagent consumption, low (and fully captured) waste, long duration, ease of integration and ease of use; the sensors have also been deployed widely in applications delivering data for science and stakeholders. Examples are given in section “Results.”
Integration is enabled either by simple mechanical integration (e.g., with brackets) and connection to a battery/power source or by utilizing the power and communications (RS 232) interface provided. For the latter a command set enables external control and triggering of pre-programmed states as well as either platform driven (polled) or sensor-initiated transfer of data.
For the first of the deployments detailed in this paper, the nitrate analyser was deployed at an Environment Agency gauging station on the River Avon (Hampshire, United Kingdom), between 15th October 2014 and 15th October 2015. It collected hourly nitrate data (subject to some short gaps) for the entire year. Data was telemetered in real time via a YSI Storm logger. The sensor was visited every 3 months in order that the reagents and onboard standard could be changed, the waste bag emptied, and the inlet filter changed. The analyser and the cadmium reduction tube were not changed for the entire year.
The accuracy of the nitrate analyser is dependent on the stability of the onboard calibration standard. In this case, the onboard standard (300 μM) was preserved using 0.1% chloroform. Subsamples of the standard were analyzed before and after each deployment, and no evidence of degradation was found over the duration of the deployment. Co-samples were collected by the Environment Agency, and as part of the Macronutrient Cycles program (Panton et al., 2020).
For the second example data set a nitrate and phosphate sensor were deployed at approximately 1 m depth off a pontoon in Empress Dock, Southampton United Kingdom in December 2016. No manual sampling or reference measurement were made: the sensors were simply deployed following analysis of the onboard standards and blanks which provide in situ calibration.
For both deployments A YSI EXO2 sonde was deployed alongside nutrient sensors to collect temperature and salinity data.
Results
Applications and Deployments
We summarize here some of the over two hundred deployments undertaken with the in situ chemical sensor technology described above.
Thirty-three LOC sensors were deployed in total measuring Dissolved Inorganic Carbon (DIC), Total Alkalinity (TA), pH, nitrate and phosphate on a variety of platforms/vehicles throughout the STEMM-CCS experiment (Research expedition JC180 in particular) (Flohr et al., 2021). This ambitious but successful experiment demonstrated the ability to monitor and quantify the integrity of offshore Carbon Capture and Storage (CCS) reservoirs through detecting and measuring the chemical signature of a simulated (but in operation extremely unlikely) leak. A leak was simulated by injecting a known volume of CO2 into the sediments at a depth of ∼3 m in the region of the proposed Goldeneye CCS reservoir (Dean and Tucker, 2017), a depleted field offshore from Scotland in the Outer Moray Firth (North Sea). LOC sensors made measurements at various locations around the site of CO2 release to both determine the spatial extent of the releases impact and quantify the release. Sensors were fully integrated with the ROV Isis (German et al., 2003) and benthic landers. These were deployed to measure baseline chemical characteristics and also to quantify the volume of CO2 released. For example, the pH sensors were used to quantify vertical gradients in pH upstream and downstream of the benthic release and in combination with hydrodynamic measurements to calculate CO2 flux (Schaap et al., 2021). The ability to detect a leak at very low release rates was demonstrated (14 kg/day) well below the suggested 0.01%/year of stored gas proposed in the literature (Hepple and Benson, 2005) which would equate to 274–5,480 kg/day in year 1 and after 20 years of injection, respectively, for this site (Flohr et al., 2021).
The sensors have been deployed on some of the smallest and lowest power platforms used in oceanography including on profiling floats as part of the SenseOcean project (614141, FP7, EU) where profiles of nutrients were obtained in the Mediterranean. They have also been deployed on ocean gliders numerous times including nitrate sensing on a Kongsberg Seaglider (Vincent et al., 2018) where the sensor was fully integrated with the glider electronics allowing command and data transfer and enabling nitrate data to be transmitted to shore via the glider’s communication capability. There was “excellent agreement between the LOC and shipboard nitrate + nitrite measurements (r2 = 0.98, n = 11)” and the sensor data revealed nitrate uptake due to phytoplankton spring bloom. The combination of sensor and glider enabled accurate data at high resolution, on both spatial and temporal scales. A phosphate sensor has been integrated with a Seaglider as part of the AlterEco project (NE/P013899/1, NERC, United Kingdom) completing a 120 km transect in North Sea in 44 days observing reduction in surface concentrations and variations in the bottom mixed layer (Birchill et al., 2021). A pH sensor was also integrated into a Seaglider (iRobot/Kongsberg, part of the UEA fleet, United Kingdom) and used in the North Sea for 10 days together with O2 and salinity data to derive CO2 content air sea fluxes of oxygen and CO2 demonstrating high spatial and temporal resolution (Possenti et al., 2021). The sensor platform has also been integrated with a Slocum glider (Teledyne Webb, United States) and demonstrated (with a nitrate sensor) as part of the project AutoNuts (NE/P020798/1, NERC, United Kingdom).
The sensors have also been deployed by scientists external to the Ocean Technology and Engineering Group at NOC who developed them. For example, a silicate and nitrate sensor were deployed for a year on a mooring at the site of the US NSF funded Ocean Observatories Initiative (OOI) Southern Ocean Array for over a year and returned excellent data funded by the CUSTARD project (NE/P021247/2, NERC, United Kingdom). In another deployment, a nitrate + nitrite and a separate nitrite sensor were deployed in the Mauritanian oxygen minimum zone, offshore Western Africa (Yuecel et al., 2015). Here sensor data augmented with oxygen and physical data enable observation of cross-shelf transport contributing to the understanding of the benthic biogeochemical dynamics.
Sensors have also been deployed at physical and geographical extremes including: to depths of ∼4,800 m [nitrate (Stinchcombe, 2017), phosphate (Ruhl, 2018), Total Alkalinity and pH (Hartman, 2019)] at the Porcupine Abyssal Plain Sustained Observatory (PAP-SO, 49N 16.5W) in the north-west Atlantic Ocean, in ice littered and sediment laden pro-glacial melt waters (Beaton et al., 2017b) as well as in rivers and estuaries (see example data).
A promising recent development is the integration of large numbers of sensors in medium sized autonomous underwater vehicles and their use in autonomous surface vehicles. For example, the OCEANIDS project (Industrial Strategy Challenge Fund, BEIS, United Kingdom) and the allied sensors projects AutoNuts (NE/P020798/1, NERC, United Kingdom) and CarCASS (NE/P02081X/1, NERC, United Kingdom) have enabled the integration of 9 different lab on chip sensors [DIC, TA, pH, nitrate, nitrite, phosphate, silicate, iron (Ferrozine method), trace iron (luminol method)] into the Autosub Long Range (ALR) platform (Furlong et al., 2012; Roper et al., 2017; Salavasidis et al., 2019) as well as up to two sensors at a time (including a new ammonia LOC sensor) into a Waveglider SV3 (Liquid Robotics, United States). Such capability opens the possibility of measuring all the major inorganic chemical variables in biogeochemical models over wide spatial and temporal scales from the surface to the deep ocean at a fraction of the cost and carbon expenditure of traditional ship-based sampling and laboratory analysis-based monitoring.
Example Data
A subset of the nitrate data returned from the LOC nitrate sensor deployed at the Environment Agency gauging station on the Hampshire Avon in 2014/15 is shown in Figure 3. The nitrate sensor data (top panel, blue line) shows excellent correlation with traditional manual and laboratory analyzed samples (red discs). The differing frequency of these two data sets demonstrates the advantage of a sensor in returning high temporal resolution data with reduced logistical effort (∼3 monthly site visits). The consistency of the sensor data also shows there is no loss in accuracy before or after replenishment of reagents (approximately at the same time as manual samples in end December, end February and early June). Operation in water at 500 μS/cm conductivity underlines the ability of the sensor and assay to work across a wide salinity range. Whilst there are common features and timing of dilution events in both the nitrate and conductivity data, there is not a direct correlation across the data set. For example, nitrate levels are supressed more than conductivity in the months at the beginning and the end of the dataset. There are also periods of a pronounced diurnal signal (in April particularly) in the nitrate data which are not present in the conductivity data. This difference is not simply explained by discharge/river flow emphasizing the utility of chemical sensors in greater understanding of biogeochemical fluxes, processes and concentrations within a river catchment.
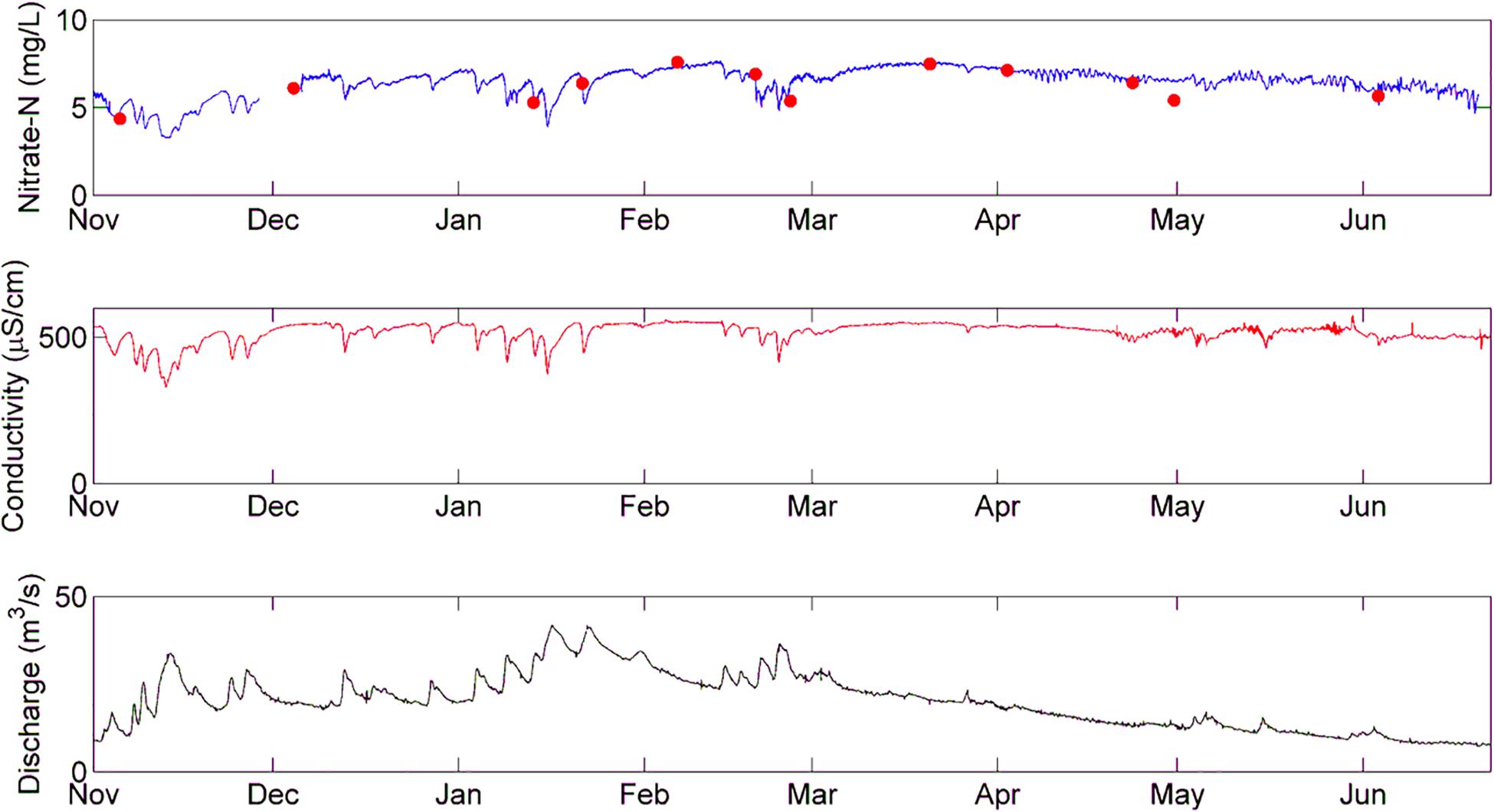
Figure 3. A ∼7.5 month subset of a year-long data series (Nov 2014–late June 215) from the deployment of a Lab On Chip nitrate sensor, and reference physical sensors at an Environment Agency gauging station on the Hampshire Avon. Data sourced from BODC archive (Beaton et al., 2017a). Top panel shows sensor nitrate timeseries (blue line) and discrete spot samples (red discs). The middle and bottom panels show conductivity and river discharge timeseries, respectively.
Example data from nitrate and phosphate sensors deployed in Empress Dock in 2016 is show in Figure 4. The top panel with blue line shows the nitrate concentration recorded by a LOC sensor whilst the middle and bottom panels show LOC phosphate and EXO2 derived salinity, respectively. There is no manual seawater sampling in this data set, the sensors were simply deployed following analysis of the onboard standards and blanks which provide in situ calibration. Best practice is to measure sensor performance against a nutrient reference material or measure sample(s) of the standard solutions analyzed against reference materials in accordance with recommendations by SCOR working group 147.2 Data from previous deployments (e.g., above) has underlined confidence that analysis of the standards and blanks is sufficient to assure the accuracy of the data produced. The data show an approximate inverse relationship between salinity and both nitrate and phosphate which illustrates dilution of high concentration freshwater inputs with lower concentration sea water in the estuarine setting. However, there are significant deviations from a direct correlation between nutrients and salinity, with nitrate and phosphate showing different features. Sources of these differences will include differing benthic fluxes, temporal variation in both river and seawater inputs and local inputs of nutrients, e.g., from direct rainwater/waste water inputs in the region as well as directly into Empress Dock.
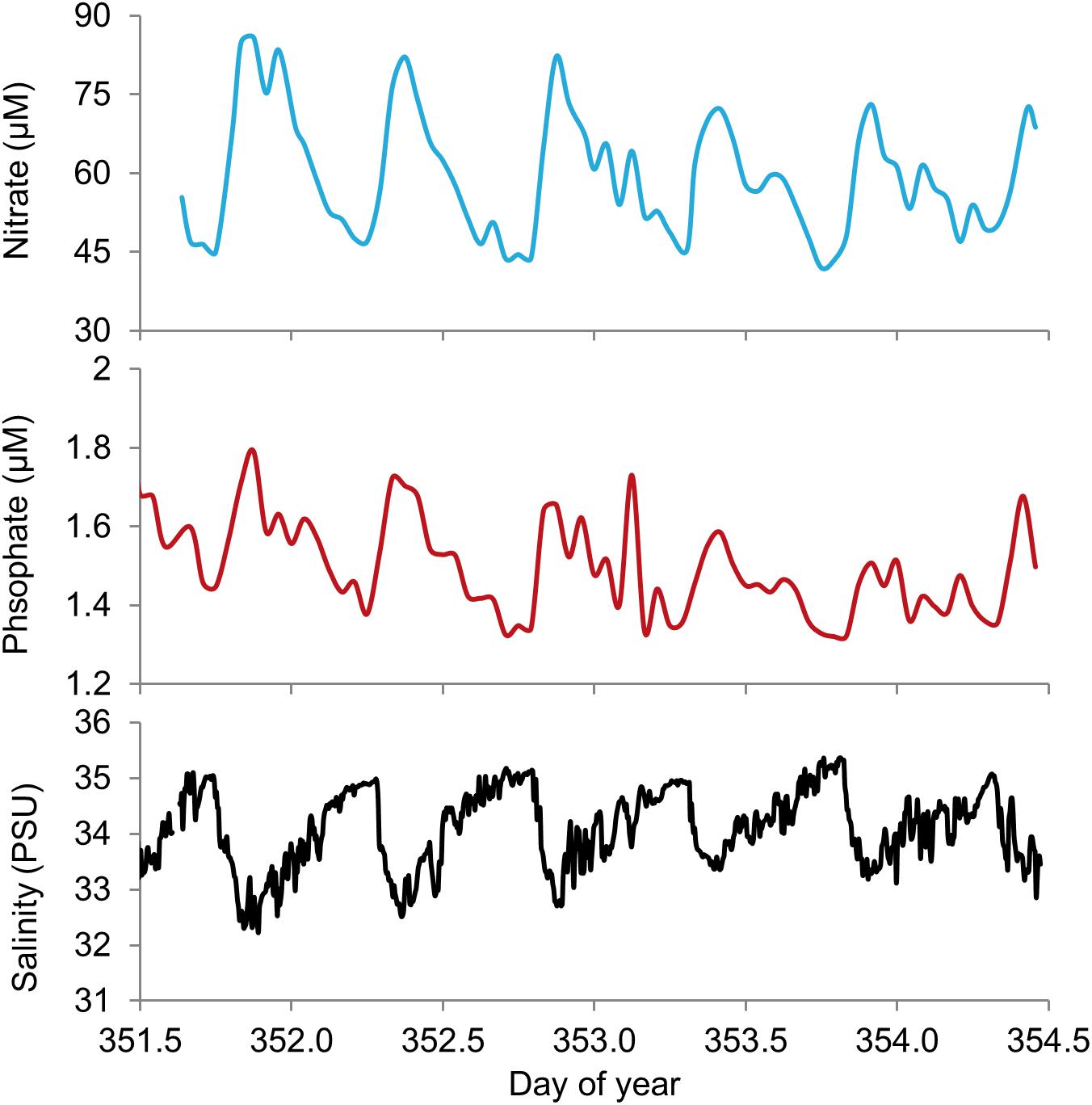
Figure 4. A 3 day timeseries of sensor nitrate and phosphate together with salinity (EXO2, YSI, United States) from deployment in Empress Dock, Southampton, United Kingdom in December 2016. The top panel with blue line shows the nitrate concentration recorded by a LOC sensor whilst the middle and bottom panels show LOC phosphate and EXO2 derived salinity, respectively.
Discussion
The principal findings from this work are that with sufficient attention to robustness, and extensive testing and feedback into design, reagent-based sensors can progress the Technology Readiness Level scale to proven commercial products (TRL 9) and can deliver unique high spatial and temporal resolution data sets from our oceans and seas. This is also possible in the deep-sea and in inhospitable environments such as high turbidity and low (icing) temperatures. Further the use of microfluidics allows processing of low (hundreds of microliters to 2 mL) of sample, and smaller volumes of reagent per sample enabling all waste to be captured even for deployments of months to years. Despite the narrow channels used in the sensors (typically 150 mm × 300 mm) biofouling has not been seen to impact the quality of the data produced. If this were encountered in the future, the inlet filter is the vulnerable element, with clogging and a change in the chemical environment (e.g., nutrient uptake, respiration/photosynthesis and change of [CO2]/pH) possible. However, because of the ability to automate variations to the fluid flow within the chip it is possible to backflush and even clean the filter in situ should this be an issue. In many settings the sensor is regularly (e.g., ∼3 months in the example data from the Hampshire Avon, Figure 3.) retrieved and reagents replaced and a conservative approach is to replace the inlet filter at this time. However, it should be noted that operations producing long datasets where this measure is not possible (e.g., the CUSTARD mooring deployment of nutrient sensors for > 12 months as reported in section “Results” above) have not shown vulnerability to fouling.
For many oceanographic applications the combination of a small, low power sensor and an autonomous vehicle presents a cost effective method for large spatial and temporal scale data collection. This approach can enable high spatial and temporal biogeochemical data acquisition, alongside existing physical sensing on the host vehicle. A promising approach, for minimizing both the cost of deployment and also carbon emissions from operations, is to remove the requirement of a research ship to make sensor deployments by hosting the sensors on a shore or small/coastal boat launched vehicle. As has been reviewed above, the LOC chemical sensors have been successfully integrated into various ocean gliders, surface vehicles and even a profiling float. A powerful capability is generated by the use of multiple sensors in a shore launched autonomous vehicle, such as Autosub Long Range (see above) where it is possible to include sensors for all of the chemical variables represented in biogeochemical models. As has been demonstrated in the STEMM-CCS project such chemical sensing can also be used for verification of offshore CCS reservoir integrity. The ability to do surveys for this purpose with shore launched autonomy is a powerful tool in reducing the cost and carbon footprint of reservoir monitoring which would otherwise reduce the carbon storage and cost efficiency of this approach.
An advantage of the deployment of chemical sensors, and the upscaling in spatial and temporal resolution of the data is that events, patches and transitions in biogeochemistry can be observed at greater detail. Without high frequency data, features such as diurnal and tidal signals in estuarine environments (e.g., Figure 4) would be missed entirely by daily or weekly sampling. Events such as pollution events, storm water runoff, or progression of planktonic blooms can also be captured and observed in near real time (e.g., using communication systems such as the in the Hampshire Avon and Seaglider deployments above) enabling redirection of monitoring effort or management response (e.g., harvesting in aquaculture).
Reagent based sensors, particularly those with in situ calibration, offer superior performance (particularly accuracy and limits of detection) when compared to existing optical (e.g., nitrate sensors based on reagent-less UV spectrophotometry; Finch et al., 1998; Johnson and Coletti, 2002) and electrochemical sensors (e.g., Johnson et al., 2016; Briggs et al., 2017; McLaughlin et al., 2017; Gonski et al., 2018; Takeshita et al., 2018). However, the in situ calibration of electrochemical sensors is a promising advancement for improved accuracy (Bresnahan et al., 2021) if somewhat at the expense of complexity. A key advantage of reagent-based sensors remains the ability to use certified, or widely accepted assays, and to access the majority of chemical variables required by users and stakeholders.
Research currently underway to continue to extend the capability of the LOC technology includes: further reductions in power and reagent/sample consumption, modifications to enable faster measurements. The current sensors report a value in 3–10 min depending on the assay. Simple multiplexing (Ogilvie et al., 2011) has been demonstrated as a possible solution and is being developed for in situ deployment. An alternative is to combine the accuracy of the reagent-based sensors with faster, but less accurate (e.g., electrochemical pH) sensors and using data fusion produce both a high resolution and accurate dataset. This approach is in active development as part of the CarCASS project for example.
The ability to manipulate fluids and observe electrical and optical responses can also be used for biological detection such as cytometry (Benazzi et al., 2007; Barat et al., 2012) of phytoplankton or molecular analysis of organisms or biomolecules (Loukas et al., 2017; Walker et al., 2017). Whilst each new assay presents its own challenges (such as the lifetime of molecular assay reagents Loukas et al., 2017) the use of microfluidics is attractive for enabling higher spatial and temporal resolution data for these variables also.
Partnership with a company enables the capability of this technology to reach a wider audience/user group and ensures its continued improvement and progression to high Technology Readiness Levels. In addition, partnership unloads research teams from the burden of routine manufacture, enables additional investment and innovation funding, and provides mechanisms to incentivise and reward further exploitation and innovation. By including the inventing team in commercial partnerships the benefits include improved knowledge transfer and reduced time to market. However, this requires careful navigation of conflicts of interest but this is possible if given due time and attention.
Data Availability Statement
Data are from Beaton et al. (2017a).
Author Contributions
MM and AB wrote the first draft of the manuscript. AS and AB produced Figures 4, 3, respectively. RP provided photographs used in Figures 1, 2. SL provided expertise and edited sections on carbonate variables. SM, AB, CC, and RP produced the commercial version of the sensors shown in Figures 1, 2. AM provided advise and edited sections on commercial partnership. All authors contributed to editing and writing of the manuscript.
Funding
The development, deployment and commercialization of this technology has been funded in part by: UK Natural Environmental Research through grants NE/P013902/1; NE/P020798/1; NE/P02081X/1; NE/P021247/2; NE/M021858/1; NE/J011975/1; NE/J007129/1; NE/I012974/1; NE/H001328/1; 4D Biogeochemical Sensors, NERC, Oceans2025, theme 8.1. EPSCR through grant EP/E016774/1. Industrial Strategy Challenge Fund, BEIS, UK through the OCEANIDS project. Funding from the European Union’s Horizon 2020 Research and Innovation Programme under grant agreement no. 101000858 (TechOceanS). Funding provided by the European Union’s Horizon 2020 Research and Innovation Programme under the grant agreement no. 654462 (STEMM-CCS). Funding provided by the European Union’s Horizon 2020 Research and Innovation Programme under the grant agreement no. 635359 (BRIDGES). Funding provided by the European Union’s Horizon 2020 Research and Innovation Programme under the grant agreement no. 633211 (AtlantOS). Funding from the European Union Seventh Framework Program (FP7/2007-2013) under grant agreement no. 614141 (SenseOCEAN). Funding from the European Union Seventh Framework Program (FP7/2007-2013) under grant agreement no. 237868 (SENSEnet). Funding from innovate UK (UKRI) project 53925 “Assuring water quality : a new generation of miniaturised microfluidic chemical analysers”.
Conflict of Interest
MM was a director, CTO, shareholder and employee of ClearWater Sensors Ltd. that manufacture and sell Lab on Chip chemical sensors utilizing intellectual property licensed from the University of Southampton and the National Oceanography Centre. This IP is used in the sensors described in this paper. Matt maintains employment in the NOC with interactions governed by a detailed conflict of interest policy. AB, RP, and CC were shareholders and employees of ClearWater Sensors Ltd. whilst maintaining employment in the NOC with interactions governed by a detailed conflict of interest policy. SM was a student and then postdoc at the University of Southampton and NOC during the development of sensors and the fieldwork described in the paper, but now is a visiting associate at the NOC and works as an employee exclusively for ClearWater Sensors Ltd.
The remaining authors declare that the research was conducted in the absence of any commercial or financial relationships that could be construed as a potential conflict of interest.
Publisher’s Note
All claims expressed in this article are solely those of the authors and do not necessarily represent those of their affiliated organizations, or those of the publisher, the editors and the reviewers. Any product that may be evaluated in this article, or claim that may be made by its manufacturer, is not guaranteed or endorsed by the publisher.
Acknowledgments
We would like to acknowledge the support of the Ocean Technology and Engineering Group and the Marine Autonomous and Robotic Systems group at the National Oceanography Centre as well as the wider teams at NOC, Clearwater Sensors Ltd. and associated project partners worldwide for their support in developing and deploying and applying the technology described in this paper.
Footnotes
References
Adornato, L. R., Kaltenbacher, E. A., Greenhow, D. R., and Byrne, R. H. (2007). High-resolution In situ analysis of nitrate and phosphate in the oligotrophic ocean. Environ. Sci. Technol. 41, 4045–4052. doi: 10.1021/es0700855
Aris, R., and Taylor, G. I. (1956). On the dispersion of a solute in a fluid flowing through a tube. Proc. R. Soc. Lond. A Math. Phys. Sci. 235, 67–77. doi: 10.1098/rspa.1956.0065
Assmann, S., Frank, C., and Kortzinger, A. (2011). Spectrophotometric high-precision seawater pH determination for use in underway measuring systems. Ocean Sci. 7, 597–607. doi: 10.5194/os-7-597-2011
Barat, D., Spencer, D., Benazzi, G., Mowlem, M. C., and Morgan, H. (2012). Simultaneous high speed optical and impedance analysis of single particles with a microfluidic cytometer. Lab Chip 12, 118–126. doi: 10.1039/c1lc20785g
Barnard, A. H., Rhoades, B., Wetzel, C., Derr, A., Zaneveld, J. R. V., Moore, C., et al. (2009). “Real-time and long-term monitoring of phosphate using the in-situ CYCLE sensor,” in Proceedings of the Oceans 2009, Vol. 1-3, (Biloxi, MS: IEEE), 1698–1703.
Barus, C., Romanytsia, I., Striebig, N., and Garcon, V. (2016). Toward an In situ phosphate sensor in seawater using Square Wave Voltammetry. Talanta 160, 417–424. doi: 10.1016/j.talanta.2016.07.057
Bates, N. R., Yrene, M. A., Kim, C., John, E. D., Melchor, G.-D., Laura, L., et al. (2014). A time-series view of changing ocean chemistry due to ocean uptake of anthropogenic CO2 and ocean acidification. Oceanography 27, 126–141.
Beaton, A. D., Cardwell, C. L., Thomas, R. S., Sieben, V. J., Legiret, F.-E., Waugh, E. M., et al. (2012). Lab-on-chip measurement of nitrate and nitrite for In situ analysis of natural waters. Environ. Sci. Technol. 46, 9548–9556. doi: 10.1021/es300419u
Beaton, A. D., Mowlem, M., Purdie, D. A., Panton, A., and Owsianka, D. R. (2017a). Nitrate and Nitrite Data from a National Oceanography Centre (NOC) Lab-on-Chip (LOC) Analyser at Knapp Mill. Hampshire Avon: British Oceanographic Data Centre - Natural Environment Research Council U.K.
Beaton, A. D., Wadham, J. L., Hawkings, J., Bagshaw, E. A., Lamarche-Gagnon, G., Mowlem, M. C., et al. (2017b). High-resolution In situ measurement of nitrate in runoff from the Greenland ice sheet. Environ. Sci. Technol. 51, 12518–12527. doi: 10.1021/acs.est.7b03121
Becker, S., Aoyama, M., Woodward, E. M. S., Bakker, K., Coverly, S., Mahaffey, C., et al. (2020). GO-SHIP repeat hydrography nutrient manual: the precise and accurate determination of dissolved inorganic nutrients in seawater, using continuous flow analysis methods. Front. Mar. Sci. 7:581790. doi: 10.3389/fmars.2020.581790
Bellerby, R. G. J., Olsen, A., Johannessen, T., and Croot, P. (2002). A high precision spectrophotometric method for on-line shipboard seawater pH measurements: the automated marine pH sensor (AMpS). Talanta 56, 61–69. doi: 10.1016/s0039-9140(01)00541-0
Benazzi, G., Holmes, D., Sun, T., Mowlem, M. C., and Morgan, H. (2007). Discrimination and analysis of phytoplankton using a microfluidic cytometer. IET Nanobiotechnol. 1, 94–101. doi: 10.1049/iet-nbt:20070020
Birchill, A. J., Beaton, A. D., Hull, T., Kaiser, J., Mowlem, M., Pascal, R., et al. (2021). Exploring ocean biogeochemistry using a lab-on-chip phosphate analyser on an underwater glider. Front. Mar. Sci. 8:698102. doi: 10.3389/fmars.2021.698102
Birchill, A. J., Clinton-Bailey, G., Hanz, R., Mawji, E., Cariou, T., White, C., et al. (2019). Realistic measurement uncertainties for marine macronutrient measurements conducted using gas segmented flow and Lab-on-Chip techniques. Talanta 200, 228–235. doi: 10.1016/j.talanta.2019.03.032
Bodini, S., Sanfilippo, L., Savino, E., and Moscetta, P., and IEEE. (2015). Automated Micro Loop Flow Reactor Technology to Measure Nutrients in Coastal Water: State of the Art and Field Application. New York, NY: IEEE.
Bresnahan, P. J., Takeshita, Y., Wirth, T., Martz, T. R., Cyronak, T., Albright, R., et al. (2021). Autonomous In situ calibration of ion-sensitive field effect transistor pH sensors. Limnol. Oceanogr. Methods 19, 132–144. doi: 10.1002/lom3.10410
Briggs, E. M., Sandoval, S., Erten, A., Takeshita, Y., Kummel, A. C., and Martz, T. R. (2017). Solid state sensor for simultaneous measurement of total alkalinity and pH of seawater. ACS Sens. 2, 1302–1309. doi: 10.1021/acssensors.7b00305
Butenschoen, M., Clark, J., Aldridge, J. N., Allen, J. I., Artioli, Y., Blackford, J., et al. (2016). ERSEM 15.06: a generic model for marine biogeochemistry and the ecosystem dynamics of the lower trophic levels. Geosci. Model Dev. 9, 1293–1339. doi: 10.5194/gmd-9-1293-2016
Cao, X., Zhang, S. W., Chu, D. Z., Wu, N., Ma, H. K., and Liu, Y. (2017). “A design of spectrophotometric microfluidic chip sensor for analyzing silicate in seawater,” in Proceedings of the 3rd International Conference on Water Resource and Environment, ed. P. Li (Quingdao: IOP Publishing Ltd).
Chapin, T. P., Jannasch, H. W., and Johnson, K. S. (2002). In situ osmotic analyzer for the year-long continuous determination of Fe in hydrothermal systems. Anal. Chim. Acta 463, 265–274. doi: 10.1016/s0003-2670(02)00423-3
Choi, Y. S., Lvova, L., Shin, J. H., Oh, S. H., Lee, C. S., Kim, B. H., et al. (2002). Determination of oceanic carbon dioxide using a carbonate-selective electrode. Anal. Chem. 74, 2435–2440. doi: 10.1021/ac0108459
Cleary, J., Slater, C., and Diamond, D., and IEEE. (2007). “Field-deployable microfluidic sensor for phosphate in natural waters,” in Proceedings of the 2007 IEEE Sensors, Vol. 1-3, (New York, NY: IEEE), 1001–1004.
Clinton-Bailey, G. S., Grand, M. M., Beaton, A. D., Nightingale, A. M., Owsianka, D. R., Slavikt, G. J., et al. (2017). A lab-on-chip analyzer for In situ measurement of soluble reactive phosphate: improved phosphate blue assay and application to fluvial monitoring. Environ. Sci. Technol. 51, 9989–9995. doi: 10.1021/acs.est7b01581
Cogan, D., Fay, C., Boyle, D., Osborne, C., Kent, N., Cleary, J., et al. (2015). Development of a low cost microfluidic sensor for the direct determination of nitrate using chromotropic acid in natural waters. Anal. Methods 7, 5396–5405. doi: 10.1039/c5ay01357g
Coll, M., Steenbeek, J., Pennino, M. G., Buszowski, J., Kaschner, K., Lotze, H. K., et al. (2020). Advancing global ecological modeling capabilities to simulate future trajectories of change in marine ecosystems. Front. Mar. Sci. 7:567877. doi: 10.3389/fmars.2020.567877
David, A. R. J., McCormack, T., Morris, A. W., and Worsfold, P. J. (1998). A submersible flow injection-based sensor for the determination of total oxidised nitrogen in coastal waters. Anal. Chim. Acta 361, 63–72. doi: 10.1016/s0003-2670(98)00023-3
Dean, M., and Tucker, O. (2017). A risk-based framework for Measurement, Monitoring and Verification (MMV) of the Goldeneye storage complex for the Peterhead CCS project, UK. Int. J. Greenhouse Gas Control 61, 1–15. doi: 10.1016/j.ijggc.2017.03.014
DeVries, T., Le Quere, C., Andrews, O., Berthet, S., Hauck, J., Ilyina, T., et al. (2019). Decadal trends in the ocean carbon sink. Proc. Natl. Acad. Sci. U.S.A. 116, 11646–11651. doi: 10.1073/pnas.1900371116
Doney, S. C., Fabry, V. J., Feely, R. A., and Kleypas, J. A. (2009). Ocean acidification: the other CO2 problem. Annu. Rev. Mar. Sci. 1, 169–192. doi: 10.1146/annurev.marine.010908.163834
Easley, R. A., and Byrne, R. H. (2012). Spectrophotometric calibration of pH electrodes in seawater using purified m-cresol purple. Environ. Sci. Technol. 46, 5018–5024. doi: 10.1021/es300491s
Figueredo, F., Girolametti, F., Aneggi, E., Lekka, M., Annibaldi, A., and Susmel, S. (2021). Plastic electrode decorated with polyhedral anion tetrabutylammonium octamolybdate N(C4H9)(4) (4) Mo8O26 for nM phosphate electrochemical detection. Anal. Chim. Acta 1161:10. doi: 10.1016/j.aca.2021.338469
Finch, M. S., Hydes, D. J., Clayson, C. H., Weigl, B., Dakin, J., and Gwilliam, P. (1998). A low power ultra violet spectrophotometer for measurement of nitrate in seawater: introduction, calibration and initial sea trials. Anal. Chim. Acta 377, 167–177. doi: 10.1016/s0003-2670(98)00616-3
Flohr, A., Schaap, A., Achterberg, E. P., Alendal, G., Arundell, M., Berndt, C., et al. (2021). Towards improved monitoring of offshore carbon storage: a real-world field experiment detecting a controlled sub-seafloor CO2 release. Int. J. Greenhouse Gas Control 106:103237. doi: 10.1016/j.ijggc.2020.103237
Floquet, C. F. A., Sieben, V. J., Milani, A., Joly, E. P., Ogilvie, I. R. G., Morgan, H., et al. (2011). Nanomolar detection with high sensitivity microfluidic absorption cells manufactured in tinted PMMA for chemical analysis. Talanta 84, 235–239. doi: 10.1016/j.talanta.2010.12.026
Fritzsche, E., Staudinger, C., Fischer, J. P., Thar, R., Jannasch, H. W., Plant, J. N., et al. (2018). A validation and comparison study of new, compact, versatile optodes for oxygen, pH and carbon dioxide in marine environments. Mar. Chem. 207, 63–76. doi: 10.1016/j.marchem.2018.10.009
Furlong, M. E., Paxton, D., Stevenson, P., Pebody, M., McPhail, S. D., Perrett, J., et al. (2012). “Autosub long range: a long range deep diving AUV for ocean monitoring,” in Proceedings of the 2012 IEEE/Oes Autonomous Underwater Vehicles, (Southampton: IEEE).
Geißler, F., Achterberg, E. P., Beaton, A. D., Hopwood, M. J., Clarke, J. S., Mutzberg, A., et al. (2017). Evaluation of a ferrozine based autonomous In situ lab-on-chip analyzer for dissolved iron species in coastal waters. Front. Mar. Sci. 4:322. doi: 10.3389/fmars.2017.00322
German, C., Tyler, P., and Griffiths, G. (2003). The maiden voyage of UK ROV Isis. Ocean Chall. 12, 16–18.
Gonski, S. F., Cai, W.-J., Ullman, W. J., Joesoef, A., Main, C. R., Pettay, D. T., et al. (2018). Assessment of the suitability of Durafet-based sensors for pH measurement in dynamic estuarine environments. Estuar. Coast. Shelf Sci. 200, 152–168. doi: 10.1016/j.ecss.2017.10.020
Grand, M. M., Clinton-Bailey, G. S., Beaton, A. D., Schaap, A. M., Johengen, T. H., Tamburri, M. N., et al. (2017). A lab-on-chip phosphate analyzer for long-term In situ monitoring at fixed observatories: optimization and performance evaluation in estuarine and oligotrophic coastal waters. Front. Mar. Sci. 4:255. doi: 10.3389/fmars.2017.00255
Grattan, L. M., Holobaugh, S., and Morris, J. G. (2016). Harmful algal blooms and public health. Harmful Algae 57, 2–8. doi: 10.1016/j.hal.2016.05.003
Hanson, A. K. (2000). “A new In situ chemical analyzer for mapping coastal nutrient distributions in real time,” in Proceedings of the OCEANS 2000 MTS/IEEE Conference and Exhibition, (Providence, RI: IEEE).
Hartman, S. (2019). RRS Discovery Cruise 103, 21 June-10 July 2019. Water Column and Seafloor Time-Series Studies at the Porcupine Abyssal Plain Sustained Observatory, in: 61. Southampton: National Oceanography Centre.
Hepple, R. P., and Benson, S. M. (2005). Geologic storage of carbon dioxide as a climate change mitigation strategy: performance requirements and the implications of surface seepage. Environ. Geol. 47, 576–585. doi: 10.1007/s00254-004-1181-2
Hoegh-Guldberg, O. (2015). Reviving the Ocean Economy: the Case for Action-2015. Geneva: WWF International.
Huang, Y. M., Yuan, D. X., Dai, M. H., and Liu, Y. X. (2012). Reverse flow injection analysis method for catalytic spectrophotometric determination of iron in estuarine and coastal waters: a comparison with normal flow injection analysis. Talanta 93, 86–93. doi: 10.1016/j.talanta.2012.01.050
Hydes, D., Aoyama, M., Aminot, A., Bakker, K., Becker, S., Coverly, S., et al. (2010). Determination of Dissolved Nutrients (N, P, Si) in Seawater with High Precision and Inter-Comparability Using Gas-Segmented Continuous Flow Analysers. Paris: UNESCO.
Iida, Y., Takatani, Y., Kojima, A., and Ishii, M. (2021). Global trends of ocean CO2 sink and ocean acidification: an observation-based reconstruction of surface ocean inorganic carbon variables. J. Oceanogr. 77, 323–358. doi: 10.1007/s10872-020-00571-5
Jannasch, H. W., Johnson, K. S., and Sakamoto, C. M. (1994). Submersible, osmotically pumped analyzers for continuous determination of nitrate in-situ. Anal. Chem. 66, 3352–3361. doi: 10.1021/ac00092a011
Johengen, T., Purcell, H., Tamburri, M., Loewensteiner, D., Smith, G. J., Schar, D., et al. (2016). Performance Verification Statement for Noc Nitrate Analyzer. Solomons, MD: Alliance For Coastal Technologies, 47.
Johnson, K. S., and Coletti, L. J. (2002). In situ ultraviolet spectrophotometry for high resolution and long-term monitoring of nitrate, bromide and bisulfide in the ocean. Deep Sea Res. Part I Oceanogr. Res. Papers 49, 1291–1305. doi: 10.1016/s0967-0637(02)00020-1
Johnson, K. S., Jannasch, H. W., Coletti, L. J., Elrod, V. A., Martz, T. R., Takeshita, Y., et al. (2016). Deep-sea DuraFET: a pressure tolerant pH sensor designed for global sensor networks. Anal. Chem. 88, 3249–3256. doi: 10.1021/acs.analchem.5b04653
Johnson, K. S., Needoba, J. A., Riser, S. C., and Showers, W. J. (2007). Chemical sensor networks for the aquatic environment. Chem. Rev. 107, 623–640. doi: 10.1021/cr050354e
Johnson, K. S., Plant, J. N., Coletti, L. J., Jannasch, H. W., Sakamoto, C. M., Riser, S. C., et al. (2017). Biogeochemical sensor performance in the SOCCOM profiling float array. J. Geophys. Res. Oceans 122, 6416–6436. doi: 10.1002/2017JC012838
Johnson, K. S., Riser, S. C., and Karl, D. M. (2010). Nitrate supply from deep to near-surface waters of the North Pacific subtropical gyre. Nature 465, 1062–1065. doi: 10.1038/nature09170
Klimant, I., Huber, C., Liebsch, G., Neurauter, G., Stangelmayer, A., and Wolfbeis, O. S. (2001). “Dual lifetime referencing (DLR) — a new scheme for converting fluorescence intensity into a frequency-domain or time-domain information,” in New Trends in Fluorescence Spectroscopy: Applications to Chemical and Life Sciences, eds B. Valeur and J.-C. Brochon (Berlin: Springer Berlin Heidelberg), 257–274.
Lai, C. Z., DeGrandpre, M. D., and Darlington, R. C. (2018). Autonomous optofluidic chemical analyzers for marine applications: insights from the submersible autonomous moored instruments (SAMI) for pH and pCO(2). Front. Mar. Sci. 4:438. doi: 10.3389/fmars.2017.00438
Legiret, F.-E., Sieben, V. J., Woodward, E. M. S., Bey, S. K. A. K., Mowlem, M. C., Connelly, D. P., et al. (2013). A high performance microfluidic analyser for phosphate measurements in marine waters using the vanadomolybdate method. Talanta 116, 382–387. doi: 10.1016/j.talanta.2013.05.004
Legrand, D. C., Mas, S., Jugeau, B., David, A., and Barus, C. (2021). Silicate marine electrochemical sensor. Sens. Actuators B Chem. 335:8. doi: 10.1016/j.snb.2021.129705
Liu, X. W., Wang, Z. H. A., Byrne, R. H., Kaltenbacher, E. A., and Bernstein, R. E. (2006). Spectrophotometric measurements of pH in-situ: laboratory and field evaluations of instrumental performance. Environ. Sci. Technol. 40, 5036–5044. doi: 10.1021/es0601843
Loukas, C. M., McQuillan, J. S., Laouenan, F., Tsaloglou, M. N., Ruano-Lopez, J. M., and Mowlem, M. C. (2017). Detection and quantification of the toxic microalgae Karenia brevis using lab on a chip mRNA sequence-based amplification. J. Microbiol. Methods 139, 189–195. doi: 10.1016/j.mimet.2017.06.008
Ma, J., Adornato, L., Byrne, R. H., and Yuan, D. X. (2014a). Determination of nanomolar levels of nutrients in seawater. Trac Trends Anal. Chem. 60, 1–15. doi: 10.1016/j.trac.2014.04.013
Ma, J., Li, Q. L., and Yuan, D. X. (2014b). Loop flow analysis of dissolved reactive phosphorus in aqueous samples. Talanta 123, 218–223. doi: 10.1016/j.talanta.2014.02.020
Macovei, V. A., Hartman, S. E., Schuster, U., Torres-Valdes, S., Moore, C. M., and Sanders, R. J. (2020). Impact of physical and biological processes on temporal variations of the ocean carbon sink in the mid-latitude North Atlantic (2002-2016). Progr. Oceanogr. 180:14. doi: 10.1016/j.pocean.2019.102223
Martz, T. R., Carr, J. J., French, C. R., and DeGrandpre, M. D. (2003). A submersible autonomous sensor for spectrophotometric pH measurements of natural waters. Anal. Chem. 75, 1844–1850. doi: 10.1021/ac020568l
Martz, T. R., Connery, J. G., and Johnson, K. S. (2010). Testing the Honeywell Durafet (R) for seawater pH applications. Limnol. Oceanogr. Methods 8, 172–184. doi: 10.4319/lom.2010.8.172
Martz, T. R., Jannasch, H. W., and Johnson, K. S. (2009). Determination of carbonate ion concentration and inner sphere carbonate ion pairs in seawater by ultraviolet spectrophotometric titration. Mar. Chem. 115, 145–154. doi: 10.1016/j.marchem.2009.07.002
McGraw, C. M., Stitzel, S. E., Cleary, J., Slater, C., and Diamond, D. (2007). Autonomous microfluidic system for phosphate detection. Talanta 71, 1180–1185. doi: 10.1016/j.talanta.2006.06.011
McLaughlin, K., Dickson, A., Weisberg, S. B., Coale, K., Elrod, V., Hunter, C., et al. (2017). An evaluation of ISFET sensors for coastal pH monitoring applications. Reg. Stud. Mar. Sci. 12, 11–18. doi: 10.1016/j.rsma.2017.02.008
Meyer, D., Prien, R. D., Dellwig, O., Connelly, D. P., and Schulz-Bull, D. E. (2012). In situ determination of iron(II) in the anoxic zone of the central Baltic Sea using ferene as spectrophotometric reagent. Mar. Chem. 130, 21–27. doi: 10.1016/j.marchem.2011.12.002
Milani, A., Statham, P. J., Mowlem, M. C., and Connelly, D. P. (2015). Development and application of a microfluidic in-situ analyzer for dissolved Fe and Mn in natural waters. Talanta 136, 15–22. doi: 10.1016/j.talanta.2014.12.045
Miller, C. A., Pocock, K., Evans, W., and Kelley, A. L. (2018). An evaluation of the performance of Sea-Bird Scientific’s SeaFET (TM) autonomous pH sensor: considerations for the broader oceanographic community. Ocean Sci. 14, 751–768. doi: 10.5194/os-14-751-2018
Moscetta, P., Sanfilippo, L., Savino, E., Moscetta, P., Allabashi, R., Gunatilaka, A., et al. (2009). “Instrumentation for continuous monitoring in marine environments,” in Proceedings of the Oceans 2009, Vol. 1-3, (New York, NY: IEEE), 737.
Mowlem, M., Beaton, A., and Slavik, G. (2018). Oceanographic and Aquatic: Applications of Optic Sensing Technologies. Boca Raton, FL: CRC Press-Taylor & Francis Group.
Mowlem, M., Schaap, A., and Beaton, A. (2019). Microfluidics-Based Sensors: a Lab on a Chip. Amsterdam: Elsevier Science.
Nagul, E. A., McKelvie, I. D., Worsfold, P., and Kolev, S. D. (2015). The molybdenum blue reaction for the determination of orthophosphate revisited: opening the black box. Anal. Chim. Acta 890, 60–82. doi: 10.1016/j.aca.2015.07.030
Nakano, Y., Kimoto, H., Watanabe, S., Harada, K., and Watanabe, Y. W. (2006). Simultaneous vertical measurements of In situ pH and CO2 in the sea using spectrophotometric profilers. J. Oceanogr. 62, 71–81. doi: 10.1007/s10872-006-0033-y
Nightingale, A. M., Beaton, A. D., and Mowlem, M. C. (2015). Trends in microfluidic systems for In situ chemical analysis of natural waters. Sens. Actuators B Chem. 221, 1398–1405. doi: 10.1016/j.snb.2015.07.091
Nightingale, A. M., Hassan, S. U., Warren, B. M., Makris, K., Evans, G. W. H., Papadopoulou, E., et al. (2019). A droplet microfluidic-based sensor for simultaneous In situ monitoring of nitrate and nitrite in natural waters. Environ. Sci. Technol. 53, 9677–9685. doi: 10.1021/acs.est.9b01032
Nightingale, A. M., Sammer-ul, H., Evans, G. W. H., Coleman, S. M., and Niu, X. Z. (2018). Nitrate measurement in droplet flow: gas-mediated crosstalk and correction. Lab Chip 18, 1903–1913. doi: 10.1039/c8lc00092a
Ogilvie, I. R. G., Sieben, V. J., Floquet, C. F. A., Zmijan, R., Mowlem, M. C., and Morgan, H. (2010). Reduction of surface roughness for optical quality microfluidic devices in PMMA and COC. J. Micromech. Microeng. 20:065016. doi: 10.1088/0960-1317/20/6/065016
Ogilvie, I. R. G., Sieben, V. J., Mowem, M. C., and Morgan, H. (2011). Temporal optimization of microfluidic colorimetric sensors by use of multiplexed stop-flow architecture. Anal. Chem. 83, 4814–4821. doi: 10.1021/ac200463y
Panton, A., Couceiro, F., Fones, G. R., and Purdie, D. A. (2020). The impact of rainfall events, catchment characteristics and estuarine processes on the export of dissolved organic matter from two lowland rivers and their shared estuary. Sci. Total Environ. 735:14. doi: 10.1016/j.scitotenv.2020.139481
Patey, M. D., Achterberg, E. P., Rijkenberg, M. J. A., Statham, P. J., and Mowlem, M. (2010). Interferences in the analysis of nanomolar concentrations of nitrate and phosphate in oceanic waters. Anal. Chim. Acta 673, 109–116. doi: 10.1016/j.aca.2010.05.029
Patey, M. D., Rijkenberg, M. J. A., Statham, P. J., Stinchcombe, M. C., Achterberg, E. P., and Mowlem, M. (2008). Determination of nitrate and phosphate in seawater at nanomolar concentrations. Trac Trends Anal. Chem. 27, 169–182. doi: 10.1016/j.trac.2007.12.006
Pinto, V. C., Araujo, C. F., Sousa, P. J., Goncalves, L. M., and Minas, G. (2019). A low-cost lab-on-a-chip device for marine pH quantification by colorimetry. Sens. Actuators B Chem. 290, 285–292. doi: 10.1016/j.snb.2019.03.098
Possenti, L., Humphreys, M. P., Bakker, D. C., Cobas-García, M., Fernand, L., Lee, G. A., et al. (2021). Air-sea gas fluxes and remineralisation from a novel combination of pH and O2 sensors on a glider. Front. Mar. Sci. 8. doi: 10.3389/fmars.2021.696772
Rerolle, V. M. C., Achterberg, E. P., Ribas-Ribas, M., Kitidis, V., Brown, I., Bakker, D. C. E., et al. (2018). High resolution pH measurements using a lab-on-chip sensor in surface waters of northwest European shelf seas. Sensors 18:2622. doi: 10.3390/s18082622
Rerolle, V. M. C., Floquet, C. F. A., Harris, A. J. K., Mowlem, M. C., Bellerby, R. R. G. J., and Achterberg, E. P. (2013). Development of a colorimetric microfluidic pH sensor for autonomous seawater measurements. Anal. Chim. Acta 786, 124–131. doi: 10.1016/j.aca.2013.05.008
Rerolle, V. M. C., Floquet, C. F. A., Mowlem, M. C., Bellerby, R., Connelly, D. P., and Achterberg, E. P. (2012). Seawater-pH measurements for ocean-acidification observations. Trac Trends Anal. Chem. 40, 146–157. doi: 10.1016/j.trac.2012.07.016
Roper, D. T., Phillips, A. B., Harris, C. A., Salavasidis, G., Pebody, M., Templeton, R., et al. (2017). “Autosub Long Range 1500: an ultra-endurance AUV with 6000 Km range,” in Proceedings of the Oceans 2017, (Aberdeen: IEEE).
Ruhl, H. (2018). RRS James Cook Cruise 165 19 May–12 Jun 2018. Water Column and Seafloor Time-Series Studies at the Porcupine Abyssal Plain Sustained Observatory, in (National Oceanography Centre Cruise Report, No. 57. Southampton: National Oceanography Centre.
Saba, G. K., Wright-Fairbanks, E., Chen, B. S., Cai, W. J., Barnard, A. H., Jones, C. P., et al. (2019). The development and validation of a profiling glider deep ISFET-based pH sensor for high resolution observations of coastal and ocean acidification. Front. Mar. Sci. 6:17. doi: 10.3389/fmars.2019.00664
Sakamoto, C. M., Johnson, K. S., and Coletti, L. J. (2009). Improved algorithm for the computation of nitrate concentrations in seawater using an In situ ultraviolet spectrophotometer. Limnol. Oceanogr. Methods 7, 132–143. doi: 10.4319/lom.2009.7.132
Salavasidis, G., Munafo, A., Harris, C. A., Prampart, T., Templeton, R., Smart, M., et al. (2019). Terrain-aided navigation for long-endurance and deep-rated autonomous underwater vehicles. J. Field Robot. 36, 447–474. doi: 10.1002/rob.21832
Schaap, A., Koopmans, D., Holtappels, M., Dewar, M., Arundell, M., Papadimitriou, S., et al. (2021). Quantification of a subsea CO2 release with lab-on-chip sensors measuring benthic gradients. Int. J. Greenhouse Gas Control 110:103427. doi: 10.1016/j.ijggc.2021.103427
Secretariat of the Convention on Biological Diversity. (2014). “An updated synthesis of the impacts of ocean acidification on marine biodiversity, technical series No. 75,” in Technical Series, eds S. Hennige, J. M. Roberts, and P. Williamson (Montreal: Secretariat of the Convention on Biological Diversity), 99.
Seidel, M. P., DeGrandpre, M. D., and Dickson, A. G. (2008). A sensor for In situ indicator-based measurements of seawater pH. Mar. Chem. 109, 18–28. doi: 10.1016/j.marchem.2007.11.013
Shitashima, K., Kyo, M., Koike, Y., and Henmi, H., and IEEE. (2002). Development of In situ pH Sensor Using ISFET. New York, NY: IEEE.
Slater, C., Cleary, J., Lau, K. T., Snakenborg, D., Corcoran, B., Kutter, J. P., et al. (2010). Validation of a fully autonomous phosphate analyser based on a microfluidic lab-on-a-chip. Water Sci. Technol. 61, 1811–1818. doi: 10.2166/wst.2010.069
Spaulding, R., DeGrandpre, M., and Harris, K. (2011). Autonomous pH and pCO(2) measurements in marine environments quantifying the inorganic carbon system with In-Situ SAMI technology. Sea Technol. 52:15.
Staudinger, C., Strobl, M., Breininger, J., Klimant, I., and Borisov, S. M. (2019). Fast and stable optical pH sensor materials for oceanographic applications. Sens. Actuators B Chem. 282, 204–217. doi: 10.1016/j.snb.2018.11.048
Staudinger, C., Strobl, M., Fischer, J. P., Thar, R., Mayr, T., Aigner, D., et al. (2018). A versatile optode system for oxygen, carbon dioxide, and pH measurements in seawater with integrated battery and logger. Limnol. Oceanogr. Methods 16, 459–473. doi: 10.1002/lom3.10260
Steimle, E. T., Kaltenbacher, E. A., and Byrne, R. H. (2002). In situ nitrite measurements using a compact spectrophotometric analysis system. Mar. Chem. 77, 255–262. doi: 10.1016/s0304-4203(02)00003-8
Stinchcombe, M. (2017). “RRS discovery cruise DY050, 18 Apr - 08 May 2016. Cruise to the porcupine abyssal plain sustained observatory,” in National Oceanography Centre Cruise Report, 45, (Southampton: National Oceanography Centre).
Takeshita, Y., Johnson, K. S., Coletti, L. J., Jannasch, H. W., Walz, P. M., and Warren, J. K. (2020). Assessment of pH dependent errors in spectrophotometric pH measurements of seawater. Mar. Chem. 223:7. doi: 10.1016/j.marchem.2020.103801
Takeshita, Y., Johnson, K. S., Martz, T. R., Plant, J. N., and Sarmiento, J. L. (2018). Assessment of autonomous pH measurements for determining surface seawater partial pressure of CO2. J. Geophys. Res. Oceans 123, 4003–4013. doi: 10.1029/2017jc013387
Takeshita, Y., Jones, B. D., Johnson, K. S., Chavez, F. P., Rudnick, D. L., Blum, M., et al. (2021). Accurate pH and O-2 measurements from spray underwater gliders. J. Atmos. Ocean. Technol. 38, 181–195. doi: 10.1175/jtech-d-20-0095.1
Tanhua, T., McCurdy, A., Fischer, A., Appeltans, W., Bax, N., Currie, K., et al. (2019). What we have learned from the framework for ocean observing: evolution of the global ocean observing system. Front. Mar. Sci. 6:471. doi: 10.3389/fmars.2019.00471
Trainer, V. L., Moore, S. K., Hallegraeff, G., Kudela, R. M., Clement, A., Mardones, J. I., et al. (2020). Pelagic harmful algal blooms and climate change: lessons from nature’s experiments with extremes. Harmful Algae 91:14. doi: 10.1016/j.hal.2019.03.009
Vincent, A. G., Pascal, R. W., Beaton, A. D., Walk, J., Hopkins, J. E., Woodward, E. M. S., et al. (2018). Nitrate drawdown during a shelf sea spring bloom revealed using a novel microfluidic In situ chemical sensor deployed within an autonomous underwater glider. Mar. Chem. 205, 29–36. doi: 10.1016/j.marchem.2018.07.005
Vuillemin, R., Le Roux, D., Dorval, P., Bucas, K., Sudreau, J. P., Hamon, M., et al. (2009). CHEMINI: a new In situ CHEmical MINIaturized analyzer. Deep Sea Res. Part I Oceanogr. Res. Papers 56, 1391–1399. doi: 10.1016/j.dsr.2009.02.002
Walker, D. I., McQuillan, J., Taiwo, M., Parks, R., Stenton, C. A., Morgan, H., et al. (2017). A highly specific Escherichia coil qPCR and its comparison with existing methods for environmental waters. Water Res. 126, 101–110. doi: 10.1016/j.watres.2017.08.032
Wang, H., Ju, A. B., and Wang, L. Q. (2021). Ultraviolet spectroscopic detection of nitrate and nitrite in seawater simultaneously based on partial least squares. Molecules 26:12. doi: 10.3390/molecules26123685
Wang, Z. A., Sonnichsen, F. N., Bradley, A. M., Hoering, K. A., Lanagan, T. M., Chu, S. N., et al. (2015). In situ sensor technology for simultaneous spectrophotometric measurements of seawater total dissolved inorganic carbon and pH. Environ. Sci. Technol. 49, 4441–4449. doi: 10.1021/es504893n
Wang, Z. H. A., Liu, X. W., Byrne, R. H., Wanninkhof, R., Bernstein, R. E., Kaltenbacher, E. A., et al. (2007). Simultaneous spectrophotometric flow-through measurements of pH, carbon dioxide fugacity, and total inorganic carbon in seawater. Anal. Chim. Acta 596, 23–36. doi: 10.1016/j.aca.2007.05.048
Waterbury, R. D., Byrne, R. H., Kelly, J., Leader, B., McElligott, S., and Russell, R. (1996). Development of an Underwater in-Situ Spectrophotometric Sensor for Seawater pH. Bellingham, WAS: SPIE.
Wei, H., Pan, D. W., and Han, H. T. (2021). Electrochemical monitoring of marine nutrients: from principle to application. Trac Trends Anal. Chem. 138:14. doi: 10.1016/j.trac.2021.116242
Woolf, D. K., Shutler, J. D., Goddijn-Murphy, L., Watson, A. J., Chapron, B., Nightingale, P. D., et al. (2019). Key uncertainties in the recent air-sea flux of CO2. Glob. Biogeochem. Cycles 33, 1548–1563. doi: 10.1029/2018gb006041
Yang, Z. M., Li, C., Zhang, Z. Z., Lu, G. X., Cai, Z. F., and Cao, W. X. (2020). Development of an In Situ analyzer based on sequential injection analysis and liquid waveguide capillary flow cell for the determination of dissolved reactive phosphorus in natural waters. Sensors 20:12. doi: 10.3390/s20102967
Yaqoob, M., Biot, B. F., Nabi, A., and Worsfold, P. J. (2012). Determination of nitrate and nitrite in freshwaters using flow-injection with luminol chemiluminescence detection. Luminescence 27, 419–425. doi: 10.1002/bio.1366
Keywords: submersible, autonomous, in situ, lab on chip, microfluidics, analytical chemistry, chemical sensors
Citation: Mowlem M, Beaton A, Pascal R, Schaap A, Loucaides S, Monk S, Morris A, Cardwell CL, Fowell SE, Patey MD and López-García P (2021) Industry Partnership: Lab on Chip Chemical Sensor Technology for Ocean Observing. Front. Mar. Sci. 8:697611. doi: 10.3389/fmars.2021.697611
Received: 19 April 2021; Accepted: 08 September 2021;
Published: 20 October 2021.
Edited by:
Ole Mikkelsen, Sequoia Scientific, United StatesReviewed by:
Feng Pan, Xiamen University, ChinaJoseph Needoba, Oregon Health and Science University, United States
Copyright © 2021 Mowlem, Beaton, Pascal, Schaap, Loucaides, Monk, Morris, Cardwell, Fowell, Patey and López-García. This is an open-access article distributed under the terms of the Creative Commons Attribution License (CC BY). The use, distribution or reproduction in other forums is permitted, provided the original author(s) and the copyright owner(s) are credited and that the original publication in this journal is cited, in accordance with accepted academic practice. No use, distribution or reproduction is permitted which does not comply with these terms.
*Correspondence: Matt Mowlem, bWF0bUBub2MuYWMudWs=; bWF0dC5tb3dsZW1AY2xlYXJ3YXRlcnNlbnNvcnMuY29t