Numerical Simulation of Deep-Sea Sediment Transport Induced by a Dredge Experiment in the Northeastern Pacific Ocean
- 1MARUM Center for Marine Environmental Sciences and Faculty of Geosciences, University of Bremen, Bremen, Germany
- 2Department of Physics and Earth Science, Jacobs University Bremen, Bremen, Germany
- 3GEOMAR Helmholtz Center for Ocean Research Kiel, Kiel, Germany
- 4NIOZ Royal Netherlands Institute for Sea Research, Department of Ocean Systems, Texel, Netherlands
- 5Federal Institute for Geosciences and Natural Resources (BGR), Hannover, Germany
- 6Royal Belgian Institute of Natural Sciences, Brussels, Belgium
Predictability of the dispersion of sediment plumes induced by potential deep-sea mining activities is still very limited due to operational limitations on in-situ observations required for a thorough validation and calibration of numerical models. Here we report on a plume dispersion experiment carried out in the German license area for the exploration of polymetallic nodules in the northeastern tropical Pacific Ocean in 4,200 m water depth. The dispersion of a sediment plume induced by a small-scale dredge experiment in April 2019 was investigated numerically by employing a sediment transport module coupled to a high-resolution hydrodynamic regional ocean model. Various aspects including sediment characteristics and ocean hydrodynamics were examined to obtain the best statistical agreement between sensor-based observations and model results. Results show that the model is capable of reproducing suspended sediment concentration and redeposition patterns observed during the dredge experiment. Due to a strong southward current during the dredging, the model predicts no sediment deposition and plume dispersion north of the dredging tracks. The sediment redeposition thickness reaches up to 9 mm directly next to the dredging tracks and 0.07 mm in about 320 m away from the dredging center. The model results suggest that seabed topography and variable sediment release heights above the seafloor cause significant changes especially for the low sedimentation pattern in the far-field area. Near-bottom mixing is expected to strongly influence vertical transport of suspended sediment.
1. Introduction
For economic reasons, deep-sea mining has attracted renewed attention during the last two decades. Of great interest are manganese nodules that are rich in metals such as copper, nickel, and cobalt and are found on the ocean floor at water depths between 4,000 and 6,000 m (Burns et al., 1990; Halbach et al., 1998; Petersen et al., 2016). Possible future industrial mining of manganese nodules would exert significant pressure on the benthic ecosystem as benthic communities are strongly adapted to stable environmental conditions in the deep sea and are expected to be susceptible to increased sediment loads associated with mining-induced sediment plumes (Glover and Smith, 2003; Gollner et al., 2017). To reliably predict the sediment transport and quantify the distribution of a sediment plume and sediment deposition in the deep sea, thoroughly validated numerical models with well-calibrated input parameters are required.
This study is part of the European MiningImpact 2 project (2018–2022). Its aim is to investigate and better understand the inevitable impacts on the deep-sea ecosystem through mining by performing basic investigations to understand the physical behaviour of the suspended sediment plume and to suggest appropriate monitoring strategies. Therefore, during the expedition SO268 with the German research vessel SONNE in February–May 2019, a small dredge experiment was devised and executed in the German license area (GLA). Visual- and sensor data were collected to study the in-situ sediment plume and its redeposition (Haalboom et al., in preparation). This includes data on suspended particulate matter mass concentration and current speed and direction, as well as photographs on relative redeposition thicknesses. To monitor the dispersion of the generated sediment plumes, 15 sensor platforms were distributed at distances of 100–475 m away from the planned dredge tracks. The majority of the sensor platforms were distributed on two parallel lines which were 200 m apart, perpendicular to the WSW-ENE trending dredge tracks. Along these lines the sensor platforms were placed at 100, 200, and 300 m away from the dredge tracks, with two platforms located in the middle of the sensor array at both the northern and southern side (Figures 1, 2). In order to better understand the dynamics of deep-sea sediment transport, a numerical simulation using a combination of sensor data and seafloor images taken by a Remotely Operated Vehicle (ROV) was carried out in this study to investigate plume dispersal and sediment deposition and underlying key parameters in this region.
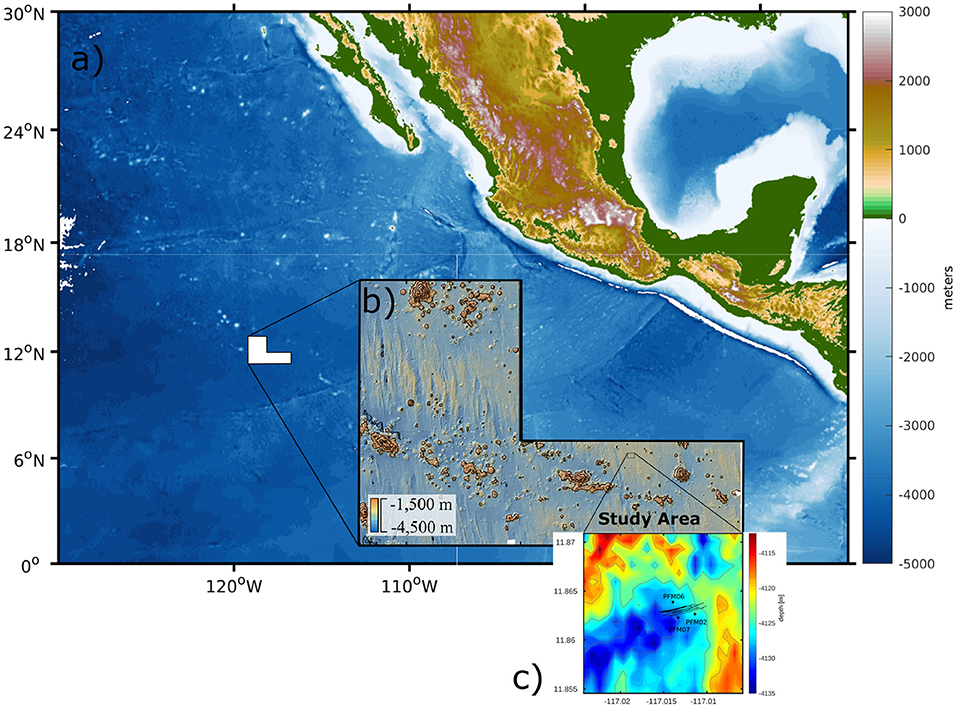
Figure 1. (a) Location of the eastern German license area (GLA) in the northeastern Pacific Ocean. (b) Sea-floor topography of the eastern GLA. The black square in (b) depicts the dredging area (DA). (c) A zooming toward the DA used for the numerical simulation, with 5 m depth contours. The dredging tracks are shown as black lines. PFM02, PFM07, and PFM06 represent the locations of bottom-moored sensors for SSC and near-bed current measurements.
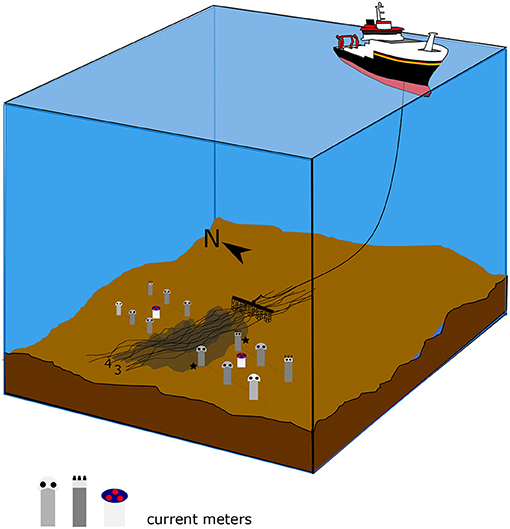
Figure 2. Schematic illustration of the deep-sea dredge experiment and the layout of the sensor array. The observation platforms with different sensors are depicted as four different elements. The monitoring stations marked by stars show the sensors that are used for model validation in our study. Dredge tracks with higher sediment discharge rate are highlighted with the track numbers. Areas of sediment deposition are shown as grey shaded areas. The figure is not scaled.
Previous resuspension experiments have used similar towed disturbance devices to generate a sediment plume to simulate the effects of potential deep-sea mining, such as in the Disturbance and Recolonisation Experiment (DISCOL) (e.g., Thiel and Schriever, 1990; Schriever, 1995), NOAA Benthic Impact Experiment (BIE) (e.g., Trueblood, 1993), or the Japan Deep Sea Impact Experiment (JET) (e.g., Fukushima, 1995). Due to longer duration, the greater spatial extent of the seafloor disturbances and the larger disturber, greater amounts of sediment have been released to the water in the BIE (1,450 t) and JET (352 t) experiments (Fukushima, 1995; Jankowski et al., 1996). The sediment redeposition in these experiments ranged from 1 to 12 mm in an area of about 2 km2. Our dredge experiment has released a considerably lower amount of sediment into the water column which makes plume monitoring and plume dispersion modelling in this study more challenging. Despite the small scale of seafloor disturbance, the more extensive sensor array deployed on the seafloor is a strength of our study as it enables us to understand plume dispersal more comprehensively (Haalboom et al., in preparation).
To date, knowledge of deep-sea sediment transport from numerical modelling is limited to a few studies that were carried out based on accompanied observations e.g., in Pacific Ocean (Jankowski et al., 1996; Nakata et al., 1997; Jankowski and Zielke, 2001; Roliniski et al., 2001; Schriever and Thiel, 2013; Aleynik et al., 2017) or in the vicinity of Canary Islands (Spearman et al., 2020). Many modelling efforts have been associated with a particular resuspension experiment and were developed commercially with a restricted access to the underlying data (personal communication with A.J. Jankowski and also see Spearman et al., 2020). In addition, the role of flocculation during plume dispersion and its direct impact on the settling velocity of particles has not been considered in the past. Instead, the settling velocity was obtained from Stokes' law considering laboratory analysis of sediment grain size from local sediments (Jankowski et al., 1996; Nakata et al., 1997). The impact of the flocculation process on settling velocities was considered in our study by using the ex-situ experiment results from Gillard et al. (2019). Determining the fate of sediments, the spreading of the plume and redeposition patterns on the seabed, the modelling of disturbance experiments requires an extended variety of oceanographic data as input, such as deep-sea current and sediment characteristics. The validation of a numerical ocean-sediment transport model with a large sensor array dataset helps to better predict future environmental impacts of deep-sea mining. The main focus of this study is to assess how much we can rely on a numerical model for the prediction of dispersion and redeposition of a sediment plume generated by a small-scale, but well-monitored, deep-seabed disturbance experiment. We first validate the numerical model using the available observations, assuming a constant release rate. The model performance, in particular the reproduction of suspended sediment concentration (SSC) signals, is tested considering a variable sediment discharge rate in different dredge tracks. In addition, the influence of key ocean and sediment parameters is examined by applying a series of sensitivity analyses to find the best match between model and observations. Finally, two applications are presented to investigate uncertainties caused by bottom topography and sediment release height during potential future industrial mining activities.
2. Methods
A combination of numerical simulation and direct seafloor observations from an array of sensors placed on the seabed was used to describe the dispersal of suspended sediment following its mobilisation by a dredge in the eastern GLA for the exploration of polymetallic nodules in the northeastern tropical Pacific Ocean (Figure 1a). The observations of current properties and suspended sediment concentration from the sensor array were first used to identify the dominant bottom currents and later to validate the model results at two sensor locations (see Figure 1). The measurements relevant for the model validation are described in this study. A comprehensive description of the hydrodynamic data, dredge experiment, mooring and sensor arrangements, and respective sediment concentrations are provided by Haalboom et al. (in preparation). After the model performance was validated by the observations, the model results were used to investigate sediment transport dynamics.
The bathymetry of the GLA is shown in Figure 1b and the location of the dredge experiment is indicated (shown as black square in Figure 1c). The area shown in Figure 1c is called the dredging area (DA) and represents the area of the numerical simulation. The seabed bathymetry was derived from ship-based multibeam data collected by the Federal Institute for Geosciences and Natural Resources (BGR) and was re-sampled to a higher resolution of 25 m (E-W) by 21 m (N-S). Water depths range from 4,114 m in the northwest to 4,135 m in the southwest. The E-W running dredging tracks are shown as black lines in Figure 1c.
3. Configuration of Hydrodynamic Model
3.1. Model Configuration
Ocean hydrodynamics are simulated using the Massachusetts Institute of Technology general circulation model (MITgcm). The model algorithm is described in detail in Marshall et al. (1997) and Adcroft et al. (2004). It solves the Boussinesq and hydrostatic form of the Navier-Stokes equations for an incompressible fluid. The prognostic equations for horizontal velocity, heat, salt, and sediment tracers are integrated forward in time on a staggered C grid (Arakawa and Lamb, 1977). A third-order advection scheme using the direct space-time method with flux limiting, is used to advect momentum, temperature and salinity. The same holds for the advection of suspended particles which is used in the sediment module. The horizontal resolution of our model is 25 × 21 m in the entire region. The vertical resolution of the model varies from 1 m close to the seafloor (up to 5 m above the seafloor) to 100 m in mid ocean depth. The model is forced at the open boundaries to the north, east, south, and west with temperature, salinity, zonal and meridional current velocities using the method devised by Stevens (1990). This method balances the net flow transport across the open boundaries and stabilises the sea surface height elevation, which is important in a small domain with four open boundaries. The lateral boundaries are supplied to the model from measurements obtained in this region (Haalboom et al., in preparation). To satisfy the model numerical stability criterion, a time step of 5 s was chosen in our simulation.
3.2. Model Forcing
The accurate reconstruction of the flow and its variability in a small domain with all boundaries open is a challenging but very crucial aspect for simulating the sediment plume distribution in the deep sea. Due to the small domain size used in this study, the model can be laterally forced by current velocity components obtained from an upward looking ADCP close to the dredging tracks (see Figure 2). Temperature and salinity profiles are taken from a CTD profile taken in the vicinity of the dredging tracks. A spin-up time of 2 days from 9 April to 11 April 2019 preceding the simulations was enough for the flow field to reach steady state before the sediment was released into the domain. The model was forced with ERA-Interim reanalysis atmospheric forcing (Berrisford et al., 2011). This includes horizontal wind speed, air temperature, short and long-wave radiation, surface specific humidity, and precipitation with 6 h temporal resolution.
3.3. The Sediment Module Equations
A sediment module was developed and coupled to the hydrodynamic MITgcm model to simulate the sediment distribution's fate caused by benthic disturbances in the deep sea. The following 3-D advection-diffusion equation for different size classes of particles is solved in the model:
where Ci is the SSC of each sediment class (i). The model includes 3 size classes which the user can define. The current velocity components are indicated as u, v, and w and the settling velocity of each sediment class are shown as wsi. The horizontal and vertical diffusivity coefficient are presented as Kh and Kv, respectively. The same advection and diffusion schemes as for salinity and temperature are also used for the SSC. The sediment input source due to the towing of the dredge on the seafloor is given as ASin. Here, A is a parameter used as a calibration coefficient to fit the observed SSC to the results of the sensor measurements at the monitoring stations. The suspended sediment that reaches the seafloor is added to the deposition layer which is depicted as Di in Equation (1) and is removed from further suspended sediment calculations in the model. The erosion of sediment for each size class (Ei) is added to the equation which may work as a source term in the model if the bed shear stress exceeds the critical shear stress (τcb).
In Equation (1), the temporal variation of the SSC (term 1) is balanced by the horizontal and vertical advection of the sediment concentration (term 2), the vertical movement of sediment due to the settling velocity (term 3), the horizontal and vertical diffusion of suspended sediment (term 4), and the sediment source and sink term (term 5). The background horizontal and vertical diffusivity coefficients used in the study are extensively discussed in section 4.4 for every tracer. The vertical flux of sediment in our calculations is solved using a third-order direct space and time advection scheme with flux limiting. To prevent a poor estimate of the energy dissipation and mixing in the ocean, the parameterisation method of Klymak and Legg (2010) is applied in our model. Similarly, the nonlocal parameterisation scheme of Large et al. (1994) is used to solve vertical mixing. The particle size classes in our study are characterised by their different settling velocities and are also introduced to the model (see section 3.4).
The following equation is used for calculating the sediment deposition Di. The near-bed suspended sediment concentration is shown as Ci and the settling velocity is ws. The subscript i shown in any parameter is related to the sediment class fraction:
The model-derived amount of deposited sediment mass is converted to the redeposition thickness, which is frequently used to determine the resilience of benthic communities (Gollner et al., 2017) and simplifies the interpretation of results obtained by integrating Equation (2) over the simulation time. The integration considers the model grid cell size, a dry bulk sediment density of 200–250 kgm−3, and a porosity of freshly deposited sediment of 0.9 (Jankowski and Zielke, 2001).
3.4. Sediment Classes and Settling Velocity
Particle settling is widely acknowledged as a complex process which is controlled by the particle composition, interaction of gravity, buoyancy, and drag forces. The settling velocity of cohesive sediments in marine environments with a high concentration of suspended particles is substantially influenced by flocculation and thus deviates from canonical Stoke's law (Dyer, 1989; Kranenburg, 1994; Van Leussen, 1994; Gillard et al., 2019). As a result of particle collisions due to turbulence or different settling velocities, primary particles stick together and form aggregates. Under elevated shear, flocs break up again into smaller particles (McCave, 1984). Many attempts have been made to improve our theoretical understanding of the flocculation process and its effects on the variation of the settling velocity in numerical models (Winterwerp, 1998; Winterwerp et al., 2006).
A recent laboratory study of Gillard et al. (2019) focused on floc behaviour and related variations of the settling velocities using local Clarion-Clipperton Zone (CCZ) sediments and mimicking deep-sea conditions present in the study area. The low sediment release rate of our dredge experiment resembles the characteristics of the experiment undertaken in Gillard et al. (2019) with initial plume concentration of 35 mgl−1 under a shear rate of G = 2.5s−1 as derived from the relatively low gradient between current velocities observed in different bins of the ADCP measurements. Adopting the results of Gillard et al. (2019), settling velocities for sediment particles in our modelling study are set at 25, 140, and 300 md−1 for aggregates in what we refer to as class 1 (D25: 70 μm), class 2 (D50: 340 μm), and class 3 (D75: 590 μm), respectively. The sediment mass distribution in our experiment was non-equally split so that class 1, class 2, and class 3 have 25, 50, and 25% of total mass weight. An extra sediment class 4 with settling velocity of 2,500 md−1 was introduced in our study to enable the model to simulate redeposition of sediment “lumps” that did not come into resuspension and were only dislocated by the dredging activity.
3.5. Dredging Tracks and Initial Estimation of Sediment Release
Dredging was conducted on 11 April 2019 between 6:30 and 19:00 UTC. The 1-m wide dredging device, a geological chain dredge, was towed across the seafloor at 0.2–0.5 ms−1 by first laying out about 500 m of cable and then hauling the dredge with the winch toward the ship. This procedure was repeated eleven times, thereby dragging the dredge across the seafloor in E-W direction. Each track took between 40 and 60 min to be completed while their length varied between 450 and 610 m (mean 500 m) (Linke and Haeckel, 2019). After completion of each track the dredge gear was lifted up from the seafloor and the ship changed its direction in line with the previous track and the same procedure was repeated until the entire experiment was completed. After the experiment, visual inspection by ROV and towed camera revealed that the gear had only grazed the upper 0–5 cm of the sediment in an irregular manner, at times missing the seabed surface completely. From underwater navigation and later visual inspection it was estimated that all eleven tracks were located in a 50 m wide corridor on the seafloor. A schematic illustration of the dredge experiment is shown in Figure 2, in which the dredge tracks, sediment plumes, and monitoring setup are indicated. A reasonable estimation of the sediment release rate in the DA requires knowledge of the length of dredging tracks, the penetration depth, the sediment particle density, and the sediment transition rate from deposition into resuspension mode. Except for the total track length, many of these parameters are basically unknown. Therefore, the discharge rate was tuned to match the suspended sediment concentration measured at two of the in-situ observatory stations. For this purpose, data from two stations, NIOZ-PFM07 and RBINS-PFM02, with similar distance from the dredge tracks at the eastern and western end were used (see the position of stations in Figure 1c). The sediment release of the best fit between observation and numerical outcome was used as final release rate for further analysis. In order to do that, a number of numerical simulations were carried out based on an initial hypothetical sediment release rate according to the length of the dredging tracks and the towing velocity until a good fit could be achieved with the observed sediment concentration measurements.
It must be noted that the dredging mostly just pushed the sediment to the sides of tracks and only a small fraction of the sediment went into suspension. The transition rate of particles from deposition into resuspension is given in Equation (1) as tuning factor. Nevertheless, the maximum sediment release rate considering the size of the dredging gear, its estimated penetration depth of 5 cm into the seafloor, the towing speed of 0.2–0.5 ms−1, the dry bulk density of the sediment of 200–250 kgm−3 and a surface sediment porosity of 0.9, can be estimated as 2.5–6.25 kgs−1.
4. Results
4.1. Current Properties
A feather plot of the current measurements at PFM06 station (Figure 1c) between 11 April and 15 April 2019 is shown in Figure 3A. A relatively strong northeastward current velocity is observed at the beginning of 11 April. The current velocity weakens and changes direction to the southeast at the beginning of the dredge experiment (Figure 3B). The current remains stable with a dominant southward direction and a mean speed of about 4 cms−1 during most of the dredging time.
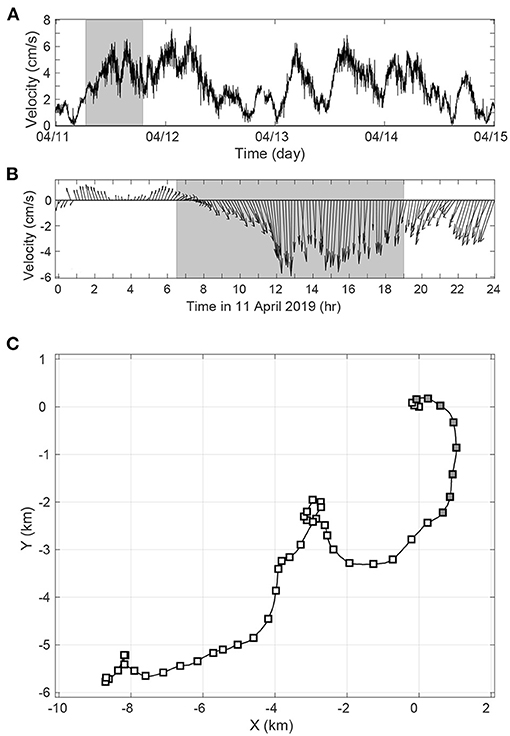
Figure 3. (A) Current velocity measurements at station PFM06 at 1 m asf from 11 to 15 April 2019. The dredging period is marked in grey. (B) Feather plot of the current velocity measurements at the same station on 11 April with a time interval of 10 min. (C) Progressive vector diagram (PVD) of the deep-sea currents observed at the same station at 1 m asf starting on 11 April 2019. Squares mark 2-hourly time intervals, those corresponding with the dredging period are coloured in grey. The displacements in x and y directions are shown in kilometres.
The progressive vector diagram (PVD) of the deep-sea current measured at 1 m above the seafloor (asf) is shown in Figure 3C with position (0,0) located at the PFM06 station on 11 April 2019. This indicates that the flow is directed mainly south to southwestward during the dredge experiment. At 1 m asf, the maximum north-south water movement is about 5.5 km, and the maximum east-west movement is 9 km. On 11 April 2019, a weak north-northeastward current was observed at the beginning of the dredge experiment (00–08 h) which turned to a southeastward current for a period of 3 h (08–11 h). A dominant southward current was observed till the dredge experiment was completed (11–19 h; Figure 3B). The mean current speeds at 1 m asf reach 4.4 cms−1, with a dominant southward velocity component on the first day and a westward one during the following days (Figure 3C). The dominant southwestward flow trajectory depicted in our analysis reflects the geomorphologic barrier of the small topographic high to the south and east of the DA (see Figure 1c).
4.2. Sediment Release Rate
The sediment release rate is one of the main parameters for a reasonable model validation based on the performed dredge experiment. Initializing the sediment model with a maximum release rate of 2.5 kgs−1 causes predicted SSC far higher than the values measured at the sensor platforms. We thus reduced the release rate to a total of only 0.1 kgs−1 including only sediment classes 1–3 to achieve a good SSC fit to the measurements. This means that presumably only about 4% of the “maximum” available sediment was resuspended. However, the amount of re-mobilised sediment that does not go into resuspension was found to be notably larger. A recent plume study by Spearman et al. (2020) carried out on a seamount near the Canary Islands, showed that a release rate of 0.2 kgs−1 of sediment into the water led to a maximum SSC similar to our measurements. Using a different release rate results in a weaker correlation between modelled and sensor data.
Qualitative assessments indicated a maximum deposition thickness of a few millimeters in the vicinity of the dredging tracks (Linke and Haeckel, 2019). This cannot be obtained if only 3 sediment classes are considered in the model. In addition to the underestimated sediment thickness predicted by the model, the area of thick sediment coverage obtained from ROV and towed camera imagery cannot be reproduced (Figures 7m,n and Table 2). Thus, sediment class 4 is included in the sediment fractions without violating the SSCs at the observatory stations to compensate for the thick sediment deposition in the dredge tracks. The amount of re-mobilised sediment (class 4), without affecting the amount of sediment release into the water column, reaches 0.63 kgs−1, which is about 25% of maximum available sediment on the seafloor. The release rate of class 4 was validated with photo imagery of seafloor redeposition coverage provided by the ROV (see Table 2). Further analysis indicates that the presence of sediment class 4 does not change the total predicted SSC at the observatory stations, meaning that this sediment class only contributes to sediment redeposition at near-field areas due to its high settling velocity. Therefore, the required release rate of suspended sediment in the water column to obtain the measured SSC at the stations is satisfied when considering 3 classes only.
4.3. Model Validation and Tuning of Sediment Release
We used sensor data of the two monitoring stations PFM07 and PFM02 to validate the model. For this, the simulated SSC was interpolated between the location of the stations and the results are shown in comparison to the sensor observation data in Figures 4A,B. In general, the maximum total SSC at the location of the dredging tracks reaches up to 28 mgl−1 and exponentially decreases in southward direction as the distance increases from the tracks. Despite marginal discrepancies, the main features of SSC and its variation are well predicted by the model and closely follow the observation. Moreover, the magnitude and occurrence time of SSC signals are predicted very well by the model (Figures 4A,B). A quantitative evaluation of the model performance at station PFM07 shows a significant statistical correlation coefficient of 0.66. The root mean square error (RMSE) is 0.5 mgl−1 which is mainly caused by the time lag between simulated and observed signals and is further increased due to the smoother model signal. The model-data comparison at station PFM02 indicates a weaker correlation with a coefficient of 0.45 and a larger RMSE of 1.5 mgl−1. The model could not predict the largest SSC signal at this station, which may cause misinterpretation of the total amount of sediment release and redeposition thickness during further analysis. However, in our simulations, the significant statistical relationship generally indicates a reasonable model performance for a constant release rate (Figures 4A,B). The comparison of model-observation at other stations also showed reasonable agreements with significant statistical relationships.
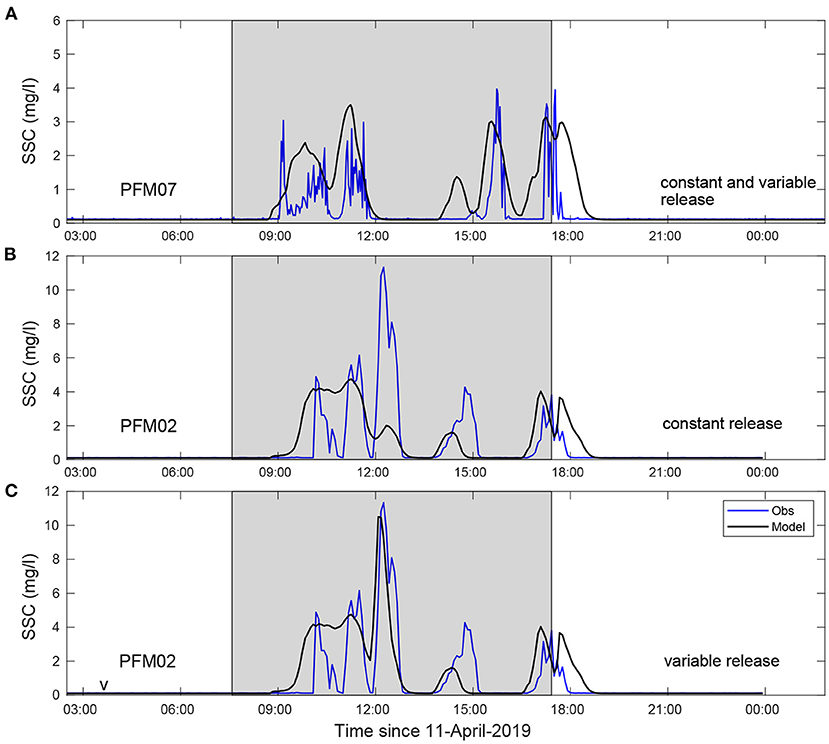
Figure 4. Comparison of observations and modeled SSC at (A) station PFM07 and (B) station PFM02 with a constant release, and at (C) station PFM02 with a variable release. The time interval when dredging was carried out is marked in grey. Observation and model results are shown by the blue and black lines in each graph, respectively.
To fine-tune the correlation between model and sensor data we evaluated the release rate in more detail by linking it specifically to the behaviour of the dredge during the deployment. It became clear that the dredging process did not create a constant sediment mobilisation, as the relatively light-weight dredge once in a while “bounced” off the bottom as seen by visual inspection. This is supported by the variable magnitude of the SSC signal in our measurements, especially at the PFM02 station. In our simulation at PFM02 a distinct SSC signal seems to be missing around +12:30, during which a plume generated in dredge tracks 3 and 4 would be expected. This has been determined by switching off individual releases from dredging tracks in our simulations. We have therefore increased the release rate of the finest sediment (5 times the normal release rate) on the last 100 m of track 3 and the first 100 m of track 4 in order to evaluate the model ability to simulate the missing signal at the station PFM02. The results of this fine-tuning of the sediment release input parameters are shown in Figure 4C. The variable release rate has enabled the model to predict the measured signal and increased the statistical relationship between model and observation with a correlation coefficient of 0.70 for station PFM02. This reduced the RMSE to 1.18 mgl−1 at this station. Nevertheless, the higher release rate at track 3 and 4 did not generate the excess SSC signal at the other station. Although our successful fine-tuning method is a hypothetical way to increase the model hindcast efficiency in our experiment, it may not be applicable to other studies with a larger number of dredging tracks. The penetration depth, and therefore the sediment release rate, which is the main source of uncertainties in our study, can be more easily assessed in advance with an industrial mining crawler that provides this information to facilitate modelling efforts.
Based on the good agreement between model and observation for the variable release rate scenario, these model parameters have been used for a further sediment transport analysis. The observed signals also show high-frequency oscillations at both stations which cannot be captured by the model simulations. They are probably a result of a relatively coarse model grid resolution and unresolved topographic features. Chaotic fine-scale mixing also complicates model-data comparison of sediment concentration.
4.4. Sensitivity Analysis
A series of sensitivity analyses was carried out to investigate the effect of the background ocean viscosity and horizontal diffusivity of sediment as important parameters for the model performance. The settling velocity and ocean hydrodynamics were adopted from observations and ex-situ lab analysis and were considered identical in our sensitivity analysis. An overview of parameter combinations is given in Table 1. The constant release rate is applied to the sensitivity analysis and the vertical diffusivity parameter is set to 10−5m2s−1 in all experiments. This allowed to select parameter values to obtain the most realistic model performance for the prediction of SSC during the dredging experiment. For a more quantitative illustration of the model performance, the sensitivity analysis results are presented in a Taylor diagram (Taylor, 2001). Generally, the impact of viscosity and diffusivity on the model performance at PFM07 is greater than at PFM02 as the results are less scattered due to the lower standard deviation (Figure 5). For individual viscosity values, increasing the sediment diffusivity parameter in the model will significantly decrease the model performance followed by lower model-data correlation and larger RMSE. The model performance slightly improves when lower viscosity values are taken in the model assuming a constant sediment diffusivity value. We also found that the sediment diffusivity has a crucial impact on the model performance, which creates a closer fit between model-observation comparison. The parameters taken in Exp 6 (see Table 1) resulted in the best fit for the total amount of SSC measured at the monitoring stations for model and observation comparison (see black arrows in Figures 5A,B). To compare our results with a previous modelling study in the region, the parameters considered in Aleynik et al. (2017), hereafter referred as Aley17, are shown in the Taylor diagram as well (green dots at Figures 5A,B) and were well-chosen. Their model performance could potentially have been improved by using parameter values from Exp 6, which indicates higher statistical relationship with the observations of all other experiments.
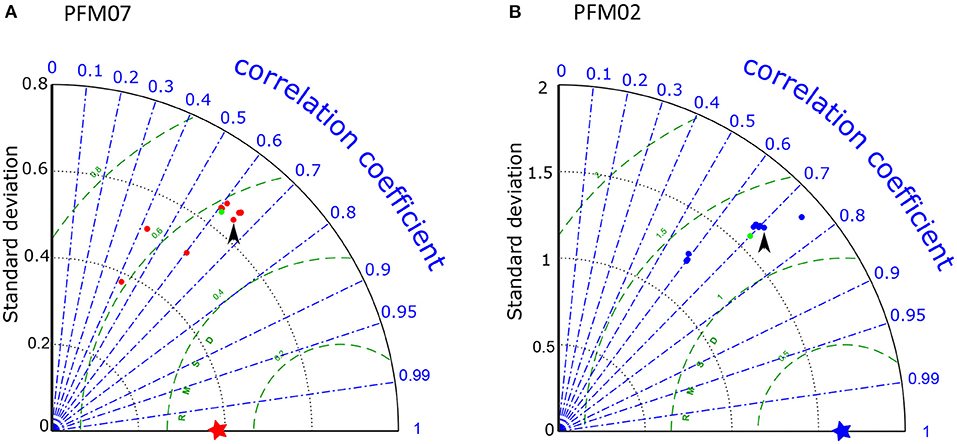
Figure 5. Taylor diagrams for the model skill assessment of the sensitivity analysis at (A) station PFM07 in red dots and (B) station PFM02 in blue dots. Corresponding observations are depicted as star in respective colours at each plot. The best fit model-observation in the sensitivity analysis is indicated by the black arrows. For a comparison, results adopting parameters from Aley17 are shown in green dots.
4.5. Sediment Deposition
Adopting variable sediment release rate and model parameters obtained from Exp6, the spatial and temporal variation of sediment deposition was investigated. Sediment redeposition maps for particle classes 1–3 for 12 h following the dredging in 2 h time intervals are depicted in Figure 6. Our results show that time and space regulate the deposition pattern (Figures 6a–s). The sediment deposition for particle class 2 and class 3 started immediately (see Figures 6b,c). However, the first redeposition for particle class 1 took a longer time and occurred only after 4 h (Figures 6a–d). The greatest redeposition appears in the vicinity of the dredging tracks, especially for particle classes 2 and 3 with high settling velocities. Following the dominant southwest current direction during dredging, no sediment deposition was found to the north of the dredging tracks, and the deposition pattern was expanded in a southwest direction for all classes. In addition, the final deposition pattern seems to follow the seabed topography features. Despite the rather smooth topography in the study area, the deeper parts located in the southwest received most of the distal sedimentation (compare Figure 1c and Figures 7m,n). This agrees with the analysis of dominant current direction from the moored current meters. Despite the different settling velocity and release rate, the general pattern of sediment deposition for class 2 and class 3 looks similar. However, the deposition pattern of sediment class 1 shows a lower deposition thickness and a more extended southeastward dispersion of deposition, reaching the outer domain boundaries.
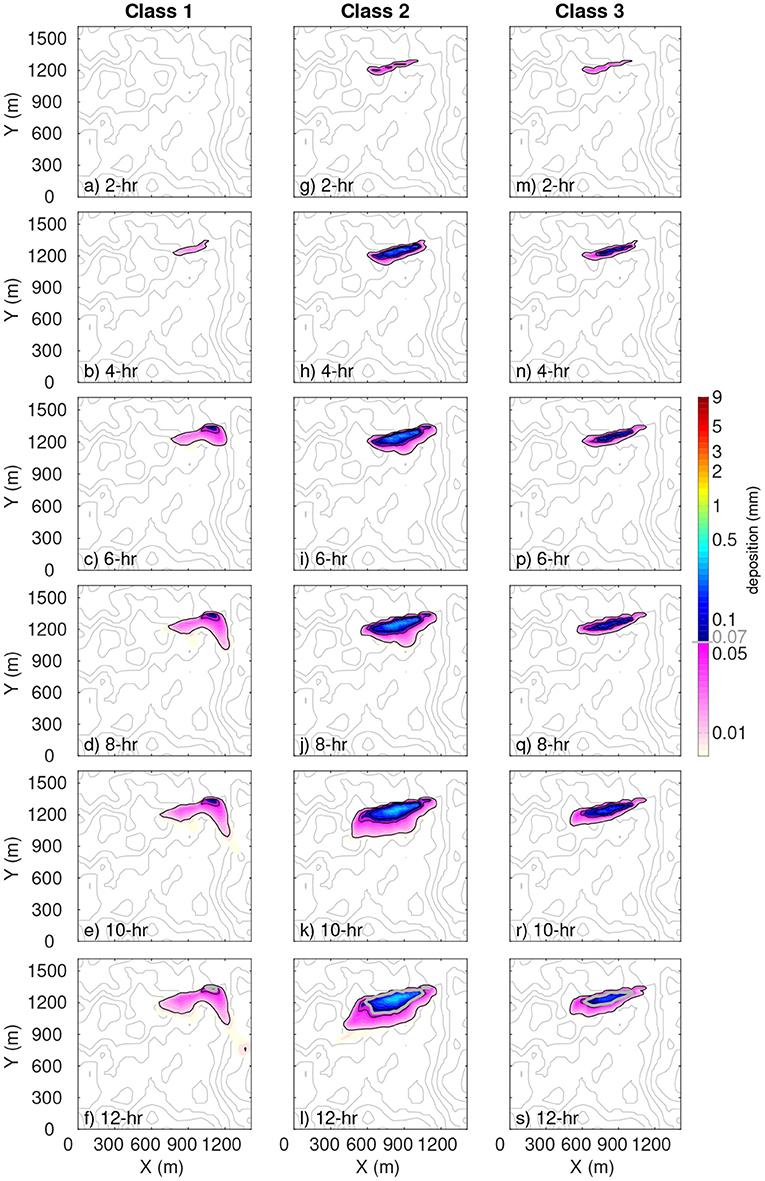
Figure 6. Sediment redeposition patterns at (a) 2-h, (b) 4-h, (c) 6-h, (d) 8-h, (e) 10 h, and (f) 12 h after dredging for particle size class1. (g–s) Redeposition of particle size class 2 and class 3, respectively, for same time steps as presented for particle size class1. The colourbar shows sediment thickness ranging from 0.01 to 9 mm in logarithmic scale. Thin grey lines in the background show the seafloor topography. The 0.07 mm deposition thickness shown as thick grey contour marks the boundary between near- and far-field areas. The near- and far-field areas are customised in the colourbar with different colour scale.
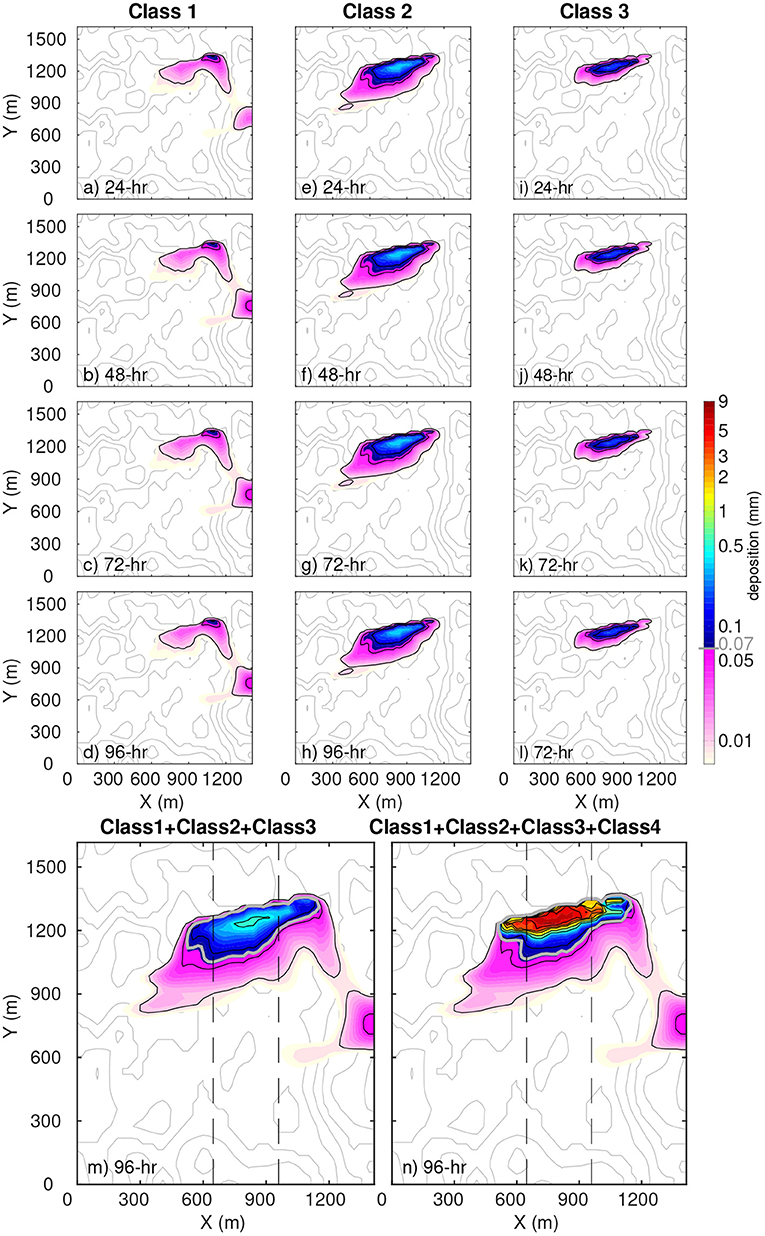
Figure 7. Sediment redeposition patterns at (a) 24 h, (b) 48 h, (c) 72 h, and (d) 96 h after dredging for particle size class1. Subplots (e–l) show similar for particle size class 2 and class 3, respectively. The colourbar indicates the thickness of sediment redeposition from 0.01 to 9 mm in logarithmic scale. Black solid contours refer to labels at the colourbar axis. Total accumulation of sediment deposition is shown in (m) for class 1–3 and in (n) for class 1–4. The 0.07 mm deposition thickness shown as thick grey contours marks the boundary between near- and far-field areas. The dashed lines show the position of transects X1 and X2 in Figure 11.
The daily sediment redeposition patterns for each of the sediment classes are shown in Figure 7. No significant changes for class 2 and 3 after 12 h are observed, as most of the sediments have already deposited earlier. On the contrary, the far-field sediment deposition of class 1 still evolves in area and thickness. The general deposition pattern for class 1 is slightly different from other classes and bifurcates southeastward with a sparse deposition (Figures 7a–d). The dominant southeastward deposition pattern of class 1 comes from the increased release rate adopted at track 3 and 4 to compensate for the missing SSC signal at the PFM02 station, and the dominant southeast current direction in the early dredging time on 11 April. Using a bulk density of freshly deposited sediments of 200 kgm−3 and considering a porosity of 90% for the newly deposited material, the greatest deposition thickness reaches 0.12, 0.39, and 0.2 mm for the different sediment classes (1, 2, and 3, respectively) at the end of the simulation period (Figures 7d–l). The final accumulated sediment deposition of class 1–3 reaches up to 0.7 mm after 4 days (Figure 7m). We have also added sediment class 4 in our calculation to mimic re-mobilisation of particles that do not resuspend. Following the increase of maximum total sediment deposition in the dredging tracks to 9 mm, a significant rise in average deposition height is evident in the near-field area (Figure 7n). Including sediment class 4 in our calculation has improved prediction of near-field deposition thickness without influencing the far field deposition pattern (compare isoline's locations of 0.07 mm at Figures 7m,n). In addition, the distribution of simulated sediment deposition with a thickness >0.07 mm resembles the deposition patterns obtained from seafloor photo image measurements (Figure 8).
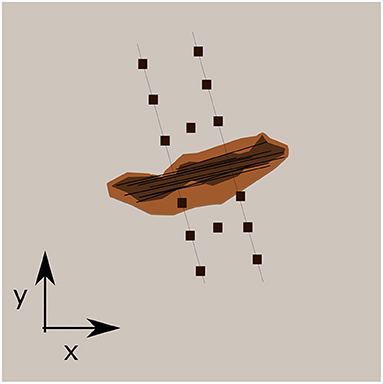
Figure 8. Schematic picture illustrating sediment redeposition obtained from ROV and towed camera imagery. The sediments deposition thicknesses are qualitatively referred to as thick and thin coverage and are shown in dark brown and light brown, respectively. The layout of sensors is depicted in the background as black squares. The figure is not scaled.
The thickness of sediment redeposition in our model results agree with the previous measurements from deep-sea benthic disturbance experiments such as JET and BIE in the CCZ, which show that the maximum deposition thickness reached 12 and 1.9 mm for much larger sediment release of 1,450 and 352 t albeit in larger regions than the DA (Trueblood, 1993; Barnett and Yamauchi, 1995; Nakata et al., 1997). The quantitative comparison of our results agrees with those experiments.
4.6. Mass Balance and Residence Time
The time evolution of the total suspended and deposited sediment mass within 4 days of the simulation are shown in Figure 9. Sediment class 4 does not influence SSC and thus is excluded from mass balance analysis. The total sediment release in the entire study region is in equilibrium with the suspended and deposited mass (Figure 9A). The time evolution of the different sediment class masses indicates a distinct behaviour for each sediment class. At the end of the simulation period 1,240, 2,907, and 995 kg of sediment from class 1, 2, and 3 are deposited on the seafloor. Therefore, total suspended mass released into the water from class 1 to 3 during the dredge experiment is about 5,140 kg (Figure 9A). However, including sediment class 4 in our calculation increases the total mobilised sediment up to about 21,740 kg. Only about 0.1% of sediment class 1 remains in suspension at the end of the simulation period, which is negligible (Figure 9A). The mass deposition for class 2 and 3 reaches an equilibrium shortly after the sediment release is stopped; due to lower settling velocities it takes longer for class 1 to meet mass deposition stability (Figure 9A). It is interesting to mention that higher vertical eddy viscosity in the model did not result in any notable change for the residence time of any sediment class.
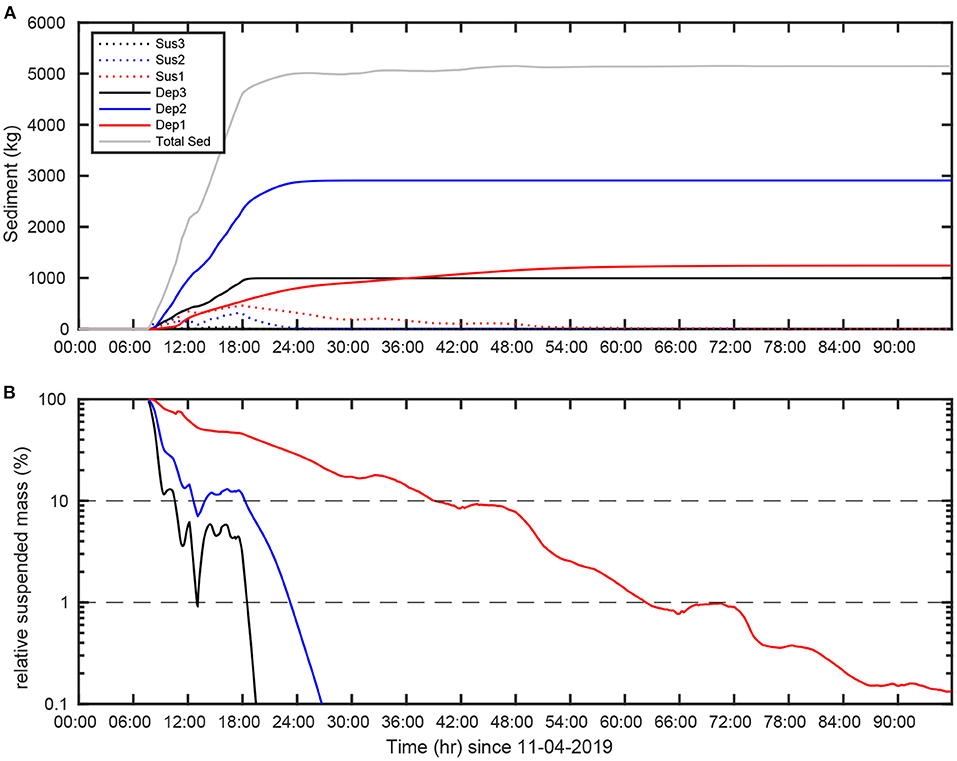
Figure 9. (A) Mass balance for total sediment release (solid grey line), deposited mass (solid colour lines) and suspended mass (dotted lines) in the entire area of simulation for each class for 4 days of simulation. Black, blue, and red colour lines refer to sediment class 1, class 2, and class 3, respectively. (B) Time evolution of relative suspended mass in the water for the given sediment class in percent. The y-axis is in logarithmic scale.
A similar observation was made for the sediment plume analysed by residence time, defined here as the time in which the suspended mass is reduced by 95%. While the residence time for sediment class 1 is about 1.5 days (at time snapshot of 50:00 h), this is much shorter for sediment classes 2 and 3 which took place immediately after completion of the dredging when no sediment is available in the water column. The time series of relative proportion of the suspension mass in the water indicates a non-linear decline tendency (Figure 9B). The suspended mass of size class 1 particles drops by 90% within the first 34 h (see time snapshot at 40:00 h in Figure 9B), in the next 32 h it drops by only 9% (see time snapshot at 72:00 h). In the last 24 h, it only drops another 0.9%. The mass balance analysis indicates that the residence time of sediment classes, independent of the amount of sediment released into the water column, is related to the settling velocity of different particle size classes. Our results are in agreement with the modelling study of Jankowski and Zielke (2001) with a larger sediment release rate during the DISCOL experiment (1,450 t), which indicates that irrespective of the higher amount of sediment released a similar residence time of 1–6 days is obtained.
4.7. Area of Deposition, Model-Data Comparison
The total surface area of deposition of different thickness calculated for particle size classes 1–3 and classes 1–4 is shown in Table 2. Note that sediment class 4 in our simulation neither impacts SSC observed at the monitoring stations nor the area of sediment deposition in the far-field (deposition with a thickness lower than 0.07 mm). The 0.07 mm redeposition isoline is taken as threshold for distinguishing between near- and far-field area. For a better visualisation, the colourbar is customised as different colour scale for near and far-field area. The variability of the area with a deposition >1 mm is only affected by the release of sediment class 4 in our experiment (see Figure 7n). However, the deposition thickness of 0.07 mm can be seen at a distance of up to 320 m from the release center at the southwest edge following the dominant current direction during the dredge experiment (see Figures 3, 7m,n). The farther westward displacement of the deposition pattern for all deposition thickness classes confirms the higher meridional (E-W) component of the prevailing current.
Based on the analysis of photos from the seabed deposition in the dredging area, one area with faint sediment deposition was visually distinguished as well as one with comparably thick sediment deposition, with surface areas of 58,597 and 32,353 m2, respectively. It must be noted that a full overview image of the areas and relevant blanketing thickness of sediment redeposition could have been obtained only with a more detailed measurements using an autonomous underwater vehicle. Therefore, our definition of different thicknesses is rather arbitrary. Qualitative model-data comparison shows that the model satisfactorily predicts the observed deposition pattern (see Figure 8). The region which according to the modelling results has 1 mm or more deposition has a size of 33,000 m2, which almost equals the shape and the absolute value of area with thick cover obtained from the photo observations (compare Figure 7n and Figure 8). The simulated area of faint coverage (⩾ 0.5 mm) is 46,000 m2 in size, showing that the model underestimated the area of the faint coverage by about 20% when compared with the observation, (see the third row of Table 2). Nevertheless, due to lack of quantitative validation of the observations the model-data comparison for the blanketing area remains uncertain. The modelling results show that the deep-sea area in the vicinity of dredging tracks is significantly impacted by the dredging experiment.
4.8. Natural Resuspension of Plume Deposit
Using long-term sea surface height anomaly data, Purkiani et al. (2020) show that between 4 and 5 long-lived anticyclonic eddies with a life-time longer than 90 d are formed annually in the northeastern Pacific Ocean. The study shows that most of the long-lived mesoscale eddies pass the GLA and depending on their strength may increase the mean current velocity at the abyssal seabed by a factor of 3–4 (Aleynik et al., 2017) or at least change the prevailing southeastward current directions to a northward current direction for a period of a few weeks (Purkiani et al., 2020). Strong deep-sea current velocities e.g., at the northwestern Bermuda Rise related to sea surface eddies caused by the meandering Gulf stream are able to episodically erode seafloor sediment and develop a benthic nepheloid layer (Gardner et al., 1985, 2017). Deep-sea current variability due to the passage of a surface mesoscale eddy can have significant impacts on sediment plume dispersion in the GLA (Aleynik et al., 2017). Therefore, the influence of current variability, if any, on potential resuspension of the fresh plume deposit was investigated in our study.
During the dredge experiment, the northern edge of an anticyclonic eddy generated off the coast of Central America reached the GLA during early May (Linke and Haeckel, 2019). This means that, considering a 2-week delay between sea surface anomaly and its bottom impacts, the deep-sea current alteration could have reached the seafloor during the dredge experiment. In agreement with the observations in the field (Haalboom et al., in preparation), our modelling results do not show any resuspension of sediment stirred up and redeposited during the dredge experiment. This is likely due to the weak current intensification, if any, that did not exceed the critical shear stress required for sediment resuspension. Sediment plume resuspension could reduce the sediment deposition in the near-field area and disperse it over a larger area with a lower thickness.
5. Discussion
Here, motivated by available data obtained from a dredge experiment, we conducted high-resolution numerical simulations with special focus on the validation of a sediment transport model. In the following section the validated model and its relevant parameters are applied to different experimental conditions such as different topography and higher sediment release height above the seafloor to investigate their effects on sediment plume deposition.
5.1. Effect of Topography
A numerical simulation with flat and horizontal topography and identical model forcing and parameters adopted from Exp6 was examined to determine the importance of seabed topography for the deposition pattern. Results show that even though slopes in the dredging area are very gentle, the deposition patterns and the extent of the low deposition contours (0.07 mm and smaller ones) are significantly affected by these variations (Figure 10A). We observe a dominant deposition tendency to the south, and a substantial bifurcation of deposition in the southeast area. The southeast bifurcation is generated by a southeastward current, which started right after the dredging began (see the red circle in Figure 3), and the absence of any terrain barrier which could have prevented the sediment plume from moving to the southeast. No significant change relevant to the seabed topography was observed for the near-field deposition pattern thicker than 0.5 mm, but the maximum deposition thickness reduced to only 3 mm. Moreover, the general pattern of sediment deposition with a thickness >0.07 mm is different from previous model results (compare Figure 10A and Figure 7n). Thus, our modelling results suggest that the seabed morphology plays an important role in the variation of redeposition patterns in the far-field, confirming field observations from a nearby area in the GLA (Peukert et al., 2018).
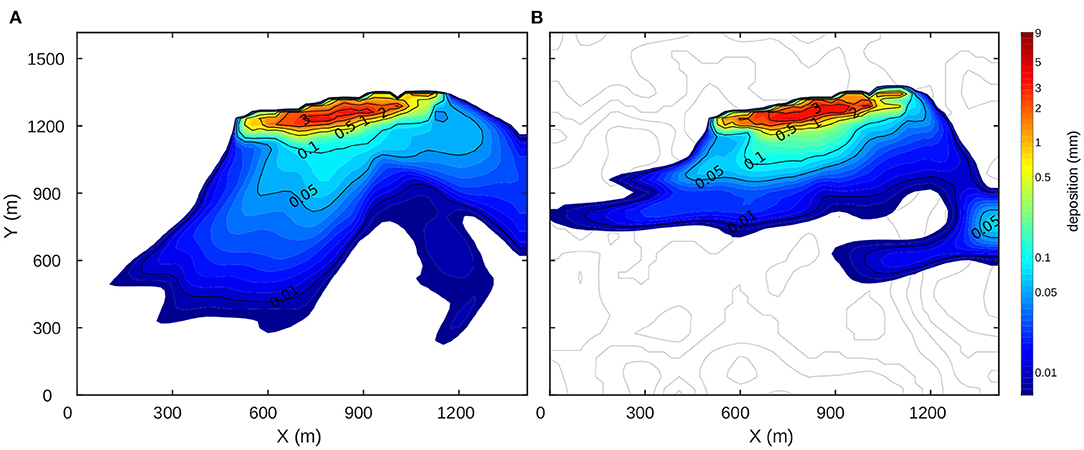
Figure 10. Numerical simulation of the deposition pattern for (A) a flat seabed and (B) higher release height above the sea floor.
5.2. Sensitivity to the Height of Sediment Release
During industrial manganese nodule mining, the collector vehicle will most likely release sediments at a height of 5–6 m above the seafloor (Oebius et al., 2001; BGR, 2019). Apart from the initial momentum given to the sediment particles by the injection from the miner exhaust, sediment is most likely pushed out of the bottom boundary layer (BBL). Owing to the smaller effect of seabed friction at that height, current velocities are relatively uniform and similar to the mean flow velocity, which is faster than closer to the seafloor where velocity is reduced by bottom friction (Perlin et al., 2005). The effect of a higher sediment release is examined by applying a release height of 5 m above the seafloor in contrast to the assumed seafloor level for the dredge experiment. The results are shown in Figure 10B. The most significant difference is seen for the low sedimentation area with a dominant pattern to the west. This is due to the stronger westward current velocity component at the greater release height. In addition, our results show a reduced southward extent of the sediment deposition pattern. The greater release height also increases deposition toward the southeast, where a thin blanketing is observed (Figure 7n). However, in the near-field area the general deposition pattern remains almost the same but with a lower maximum deposition of about 3.9 mm. This highlights the importance of considering adequate release height and the relevant current velocities in industrial-scale modelling scenarios, especially for environmental impact assessment in the far-field area.
5.3. Effect of Seawater Stratification on Vertical Extent of the Sediment Plume
In coastal seas and estuaries where tidal and wind mixing effects commonly reach the seafloor and lead to sediment resuspension, water stratification tends to limit the vertical distribution of sediment plumes and the resulting sediment dispersion (see e.g., Baeye et al., 2011; Hu et al., 2019). In the deep sea at more than 4,000 m water depth, stratification is generally very weak, and dynamic mixing processes that may result in sediment resuspension and dispersion are also rare. An exception is formed by intermittent strong near-bed flow resulting from tides or mesoscale eddy activity at the sea surface. It has been shown that these two effects have comparable contributions in controlling abysal current variability in the GLA (Aleynik et al., 2017). For this scenario, the vertical distribution of the sediment plume was analysed at two lateral transects (Figure 11), showing that it is strongly dependent on the seawater stratification. During the persistently stratified period, the sediment plume remains close to the seafloor (Figures 11A,G–I), but as the water undergoes strong mixing, the sediment cloud rises in the water column. Increased SSCs can be seen at higher altitude above the seafloor such that e.g., the 0.5 mgl−1 SSC isolines reach 8 m above the seafloor (Figures 11D–F,J,K). Where sediment release by mining coincides with a period of stronger mixing, the suspended sediment plume may rise to tens of meters above the seafloor. This may enhance horizontal dispersion of the sediment plume and eventually far-field deposition.
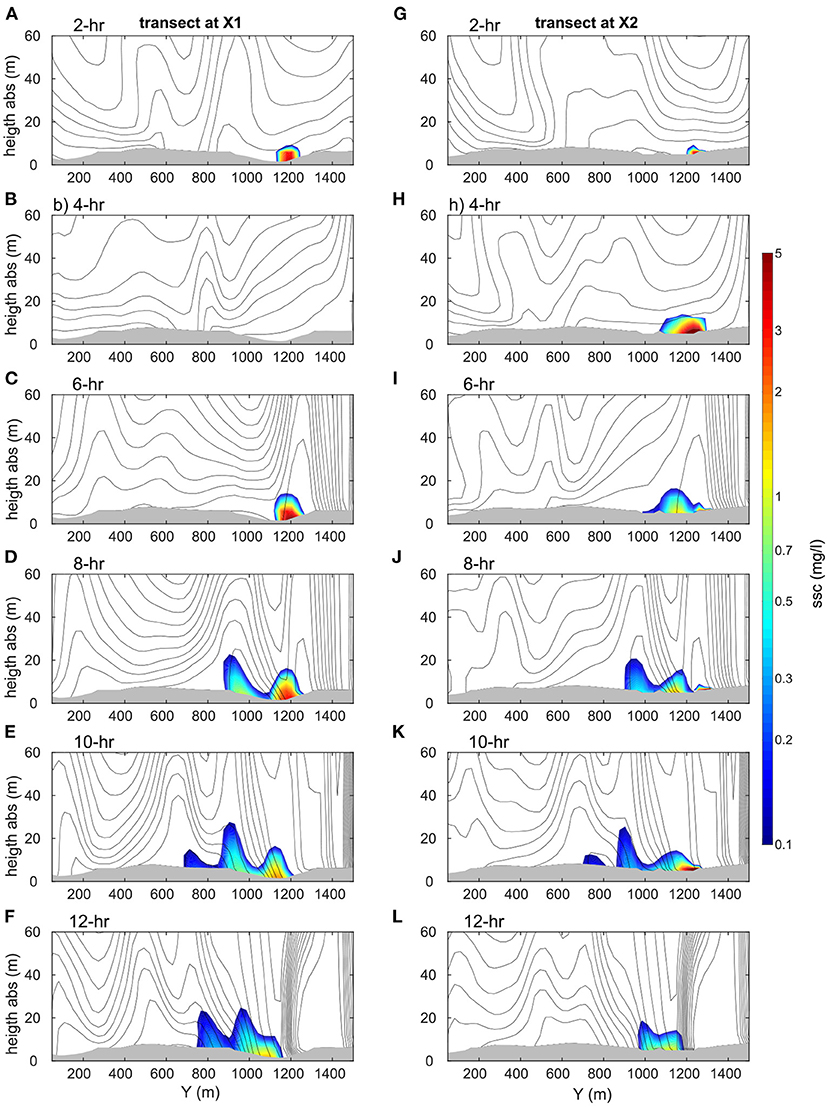
Figure 11. Lateral transect of sediment plume distribution at transect X1 at (A) 2 h, (B) 4 h, (C) 6 h, (D) 8 h, (E) 10 h, and (F) 12 h after dredging. Overlying contours show isolines of potential temperature. Temperature contour interval is 0.002°C with lowest temperature depicted as 1.2°C. (G–L) shows lateral transect of plume distribution similar to above but at transect X2. X1 and X2 are the lateral transects at the locations of stations PFM06 and PFM02 (see Figure 1c).
6. Conclusions and Recommendations
We performed a small-scale dredge experiment and combined a numerical model with observations from a monitoring array to investigate the spread of the sediment plume and the amount of redeposition induced by dredging. The meaningful statistical correlation between model and observational data shows that the developed model is capable to reproduce the spread and deposition of a sediment plume with some smaller uncertainties. While the spatial pattern of sediment deposition was satisfactorily simulated in accordance with photos taken during the experiment, the deposition height in the dredging tracks remains uncertain. Despite the low sediment release rate, the model results show a relatively high suspended sediment concentration even at a distance up to 200 m from the source. A blanketing layer of 0.5 mm extends to an area of about 46,000 m2 in the near-field area, which could already have detrimental consequences for certain deep-sea communities (Schaaning et al., 2008). However, the area with a deposition height of 0.07 mm following the dominant current direction in this area reaches up to 320 m away from the source. Thus, it can be inferred that a significantly greater sediment release during industrial mining would lead to a higher sediment deposition of up to a few centimeters in the near-field area and an expanded far-field low sedimentation area up to several kilometers away from the source. The correct properties of deep sea currents, the sediment settling velocity, and the sediment release rate are the main factors for a reliable simulation of the sediment transport in the deep sea. Other numerical parameters such as sediment diffusivity and the 3-D ocean viscosity background do not cause significant effects and are only of secondary importance to achieve a good agreement between model results and observations. The model was more sensitive to the ocean viscosity background coefficient. The impacts of the seafloor morphology and sediment release altitude on the deposition pattern are significant. A higher sediment release height can lead to wider horizontal dispersion of the sediment plume and extension of the far-field impacted area. Our study further shows that the onset and loss of seawater stratification both play a key role in the extension height of SSC from the bottom. For industrial-size release rates near seamounts or other morphological features, which are hotspots for increased turbulence in the deep ocean, the increased vertical dispersion of SSC should be considered as these might reach a couple of tens of meters above the seafloor. This might reduce the severity of impact of a thick and thus spatially more constrained deposition in the near-field. Eventually, this model and its parameters can be used for numerical simulation and prediction of sediment plume distribution induced by potential future industrial mining activities in the eastern CCZ. Furthermore, the model results can be used for a better informed positioning of seafloor monitoring arrays to collect the most informative field data for a meaningful recording of the fate of the sediment plume.
Data Availability Statement
The datasets for this study including current and turbidity data can be found in the https://doi.pangaea.de/10.1594/PANGAEA.934035. ERA-Interim reanalysis atmospheric data are online available at https://www.ecmwf.int/.
Author Contributions
KP conceived the study, designed the numerical simulations, analysed and interpreted the numerical results, and wrote the first draft of the manuscript. MHa and HS designed and conducted the field experiment. BG, MHo, SH, JG, HS, MB, AV, and LT have contributed with sampling data. AP and MS have assisted with the numerical simulations. All authors contributed to the final draft of the manuscript.
Funding
This study was supported financially by the German Federal Ministry of Education and Research through the MiningImpact II project (grant no. 03F0812A-H) as a part of the Joint Programming Initiative of Healthy Seas and Oceans (JPI Oceans).
Conflict of Interest
The authors declare that the research was conducted in the absence of any commercial or financial relationships that could be construed as a potential conflict of interest.
Publisher's Note
All claims expressed in this article are solely those of the authors and do not necessarily represent those of their affiliated organizations, or those of the publisher, the editors and the reviewers. Any product that may be evaluated in this article, or claim that may be made by its manufacturer, is not guaranteed or endorsed by the publisher.
Acknowledgments
We appreciate Martin Losch from Alfred Wegener Institute, Bremerhaven, Germany for providing us the preliminary version of the numerical sediment module. The authors are grateful to Miguel Angel Ahumada-Sempoal and Ian David Tuck for reviewing our manuscript.
References
Adcroft, A., Hill, C., Campin, J. M., Marshall, J., and Heimbach, P. (2004). “Overview of the formulation and numerics of the MIT GCM,” in Proceedings of the ECMWF Seminar Series on Numerical Methods, Recent Developments in Numerical Methods for Atmosphere and Ocean Modelling (Cambridge, MA), 139–149.
Aleynik, D., Inall, M., Dale, A., and Vink, A. (2017). Impact of remotely generated eddies on plume dispersion at abyssal mining sites in the Pacific. Sci. Rep. 7, 1–14. doi: 10.1038/s41598-017-16912-2
Arakawa, A., and Lamb, V. (1977). “Computational design of the basic dynamical processes of the UCLA general circulation model,” in Methods in Computational Physics (San Diego, CA), 174–265. doi: 10.1016/B978-0-12-460817-7.50009-4
Baeye, M., Fettwis, M., and Voulgaris, G. (2011). Sediment mobility in response to tidal and wind-driven flows along the Belgian inner shelf, southern North Sea. Ocean Dyn. 60, 611–622. doi: 10.1007/s10236-010-0370-7
Barnett, B., and Yamauchi, H. (1995). “Deep sea sediment resuspension system used for the Japan deep sea impact experiment,” in Proceedings of the First (1995) ISOPE Ocean Mining Symposium (Tsukuba), 175–179.
Berrisford, P., Dee, D., Poli, P., Brugge, R., Fielding, K., Fuentes, M., et al. (2011). The Era-Interim Archive Version 2.0, Era Report Series 1. Technical report, ECMWF, Shinfield Park, Reading, United Kingdom.
BGR (2019). Environmental Impact Assessment for the Testing of a Pre-Protoype Manganese Nodule Collector Vehicle in the Eastern German License Area (Clarion-Clipperton Zone) in the Framework of the European JPI-O Miningimpact 2 Research Project. BGR, 1–203.
Burns, R., Halbach, P., Friedrich, G., and Stackelberg, U. V. (1990). The manganese nodule belt of the pacific ocean. Geological environment, nodule formation, and mining aspects. Geol. Mag. 127:93. doi: 10.1017/S0016756800014394
Dyer, K. R. (1989). Sediment processes in estuaries: future research requirements. J. Geophys. Res. 94, 14327–14339. doi: 10.1029/JC094iC10p14327
Fukushima, T. (1995). “Japan overview deep-sea impact experiment (JET),” in Proceedings of ISOPE Ocean Mining Symposium (Tsukuba), 47–53.
Gardner, W., Southhard, J., and Hollister, C. (1985). Benthic storms, nepheloid layers, and linkage with upper ocean dynamics in the western North Atlantic. Mar. Geol. 65, 199–242.
Gardner, W., Tucholke, B., Richardson, M., and Biscaye, P. (2017). Benthic storms, nepheloid layers, and linkage with upper ocean dynamics in the western North Atlantic. Mar. Geol. 385, 304–327. doi: 10.1016/j.margeo.2016.12.012
Gillard, B., Purkiani, K., Chatzievangelou, D., Vink, A., Iversen, M. H., and Thomsen, L. (2019). Physical and hydrodynamic properties of deep sea mining-generated, abyssal sediment plumes in the clarion clipperton fracture zone (eastern-central pacific). Elem Sci. Anth. 7, 1–14. doi: 10.1525/elementa.343
Glover, A., and Smith, C. (2003). The deep-sea floor ecosystem: current status and prospects of anthropogenic change by the year 2025. Environ. Conserv. 30, 219–241. doi: 10.1017/S0376892903000225
Gollner, S., Kaiser, S., Menzel, L., Jones, D. O., Brown, A., Mestre, N. C., et al. (2017). Resilience of benthic deep-sea fauna to mining activities. Mar. Environ. Res. 129, 76–101. doi: 10.1016/j.marenvres.2017.04.010
Halbach, P., Friedrich, G., and von Stackelberg, U. (1998). The Manganese Nodule Belt of the Pacific Oceans. Stuttgart: Ferdinand Enke Verlag, 175–187.
Hu, Y., Yu, Z., Zhou, B., Li, Y., Yin, S., He, X., et al. (2019). Tidal-driven variation of suspended sediment in hangzhou bay based on goci data. Int. J. Appl. Earth Observ. Geoinform. 82:101920. doi: 10.1016/j.jag.2019.101920
Jankowski, J., Malcherek, A., and Zielke, W. (1996). Numerical modeling of due to deep-sea mining. J. Geophys. Res. 101, 3545–3560. doi: 10.1029/95JC03564
Jankowski, J., and Zielke, W. (2001). The mesoscale sediment transport due to technical activities in the deep sea. Deep Sea Res. Part II Top. Stud. Oceanogr. 48, 3487–3521. doi: 10.1016/S0967-0645(01)00054-6
Klymak, J., and Legg, S. (2010). A simple mixing scheme for models that resolve breaking internal waves. Ocean Model. 33, 224–234. doi: 10.1016/j.ocemod.2010.02.005
Kranenburg, C. (1994). The fractal structure of cohesive sediment aggregates. Estuar. Coast. Shelf Sci. 39, 451–460. doi: 10.1016/S0272-7714(06)80002-8
Large, W., McWilliams, J., and Doney, S. (1994). A review and a model with nonlocal boundary layer parameterization. Rev. Geophys. 363–403. doi: 10.1029/94RG01872
Linke, P., and Haeckel, M. (2019). Short Cruise Report RV Sonne so268/1+2. GEOMAR, Technical report.
Marshall, J., Adcroft, A., Hill, C., Perelman, L., and Heisey, C. (1997). A finite-volume, incompressible navier-stokes model for studies of the ocean on parallel computers. J. Geophys. Res. 102, 5753–5766. doi: 10.1029/96JC02775
McCave, I. N. (1984). Size spectra and aggregation of suspended particles in the deep ocean. Deep Sea Res. Part A Oceanogr. Res. Pap. 31, 329–352. doi: 10.1016/0198-0149(84)90088-8
Nakata, K., Kubota, M., Aoki, S., and Taguchi, K. (1997). “Dispersion of resuspended sediment by ocean mining activity modelling study,” in Proceedings of the Symposium on Environmental Studies for Deep-Sea Mining (Tokyo).
Oebius, H. U., Becker, H. J., Rolinski, S., and Jankowski, J. A. (2001). Parametrization and evaluation of marine environmental impacts produced by deep-sea manganese nodule mining. Deep Sea Res. Part II Top. Stud. Oceanogr. 48, 3453–3467. doi: 10.1016/S0967-0645(01)00052-2
Perlin, A., Moum, J. N., Klymak, J., Levine, M. D., Boyd, T., and Kosro, P. (2005). A modified law-of-the-wall applied to oceanic bottom boundary layers. J. Geophys. Res. 110, 1–9. doi: 10.1029/2004JC002310
Petersen, S., Krtschell, A., Augustin, N., Jamieson, J., Hein, J., and Hannington, M. (2016). News from the seabed - Geological characteristics and resource potential of deep-sea mineral resources. Mar. Policy 70, 175–187. doi: 10.1016/j.marpol.2016.03.012
Peukert, A., Schoening, T., Alevizos, E., Köser, K., Kwasnitschka, T., and Greinert, J. (2018). Understanding MN-nodule distribution and evaluation of related deep-sea mining impacts using AUV-based hydroacoustic and optical data. Biogeosciences 15, 2525–2549. doi: 10.5194/bg-15-2525-2018
Purkiani, K., Paul, A., Vink, A., Walter, M., Schulz, M., and Haeckel, M. (2020). Evidences of eddy-related deep-ocean current variability in the northeast tropical Pacific Ocean induced by remote gap winds. Biogeosciences 17, 1–18. doi: 10.5194/bg-2020-77
Roliniski, S., Segschneider, J., and Suendermann, J. (2001). Long-term propagation of tailings from deep-sea mining under variable conditions by means of numerical simulations. Deep Sea Res. Part II 48, 3469–3485. doi: 10.1016/S0967-0645(01)00053-4
Schaaning, M., Trannum, H., Øxnevad, S., Carroll, J., and Blake, T. (2008). Effects of drill cuttings on biogeochemical fluxes and macrobenthos of marine sediments. J. Exp. Mar. Biol. Ecol. 361, 49–57. doi: 10.1016/j.jembe.2008.04.014
Schriever, G. (1995). “Discol- disturbance and recolonization experiment of a manganese nodule area of the southeastern Pacific,” in Proceedings of the First (1995) ISOPE Ocean Mining Symposium (Tsukuba), 163–166.
Schriever, G., and Thiel, H. (2013). “Tailing and their disposal in deep-sea mining,” in Proceeding of the Tenth(2013) ISOPE Ocean Mining and Gas Hydrates Symposium (Szczecin), 1–14.
Spearman, J., Taylor, J., Crossouard, N., Cooper, A., Turnbull, M., Manning, A., et al. (2020). Measurement and modelling of deep sea sediment plumes and implications for deep sea mining. Sci. Rep. 10, 1–14. doi: 10.1038/s41598-020-61837-y
Stevens, D. P. (1990). On open boundary conditions for three dimensional primitive equation ocean circulation models. Geophys. Astrophys. Fl. Dyn. 51, 103–133. doi: 10.1080/03091929008219853
Taylor, K. E. (2001). Summarizing multiple aspects of model performance in a single diagram. J. Geophyd. Res. 106, 7183–7192. doi: 10.1029/2000JD900719
Thiel, H., and Schriever, G. (1990). Deep-sea mining, environmental impact and the DISCOL project. AMBIO 19, 245–250.
Trueblood, D. (1993). US Cruise Report for BIE II. NOAA Technical Memorandum NOS OCRM4, NOAA, National Ocean Service, 1–50.
Van Leussen, W. (1994). Estuarine macroflocs and their role in fine-grained sediment transport (Ph.D. thesis). University of Utrecht, Utrecht, Netherlands.
Winterwerp, J. C. (1998). A simple model for turbulence induced flocculation of cohesive sediment. J. Hydraul. Res. 36, 309–326. doi: 10.1080/00221689809498621
Keywords: dredge experiment, MITgcm, deep-sea mining, redeposition, sensor array, deep-sea sediment transport
Citation: Purkiani K, Gillard B, Paul A, Haeckel M, Haalboom S, Greinert J, de Stigter H, Hollstein M, Baeye M, Vink A, Thomsen L and Schulz M (2021) Numerical Simulation of Deep-Sea Sediment Transport Induced by a Dredge Experiment in the Northeastern Pacific Ocean. Front. Mar. Sci. 8:719463. doi: 10.3389/fmars.2021.719463
Received: 02 June 2021; Accepted: 29 July 2021;
Published: 31 August 2021.
Edited by:
Ashley Alun Rowden, National Institute of Water and Atmospheric Research (NIWA), New ZealandReviewed by:
Miguel Angel Ahumada-Sempoal, University of the Sea, MexicoIan David Tuck, Fisheries New Zealand, Ministry for Primary Industries, New Zealand
Copyright © 2021 Purkiani, Gillard, Paul, Haeckel, Haalboom, Greinert, de Stigter, Hollstein, Baeye, Vink, Thomsen and Schulz. This is an open-access article distributed under the terms of the Creative Commons Attribution License (CC BY). The use, distribution or reproduction in other forums is permitted, provided the original author(s) and the copyright owner(s) are credited and that the original publication in this journal is cited, in accordance with accepted academic practice. No use, distribution or reproduction is permitted which does not comply with these terms.
*Correspondence: Kaveh Purkiani, kpurkiani@marum.de