Editorial: Managing for the Future: Challenges and Approaches for Disentangling the Relative Roles of Environmental Change and Fishing in Marine Ecosystems
- 1Fisheries and Oceans Canada, Bedford Institute of Oceanography, Dartmouth, NS, Canada
- 2Akvaplan-niva, Fram Centre for Climate and the Environment, Tromsø, Norway
- 3Department of Arctic Biology, University Centre in Svalbard, Longyearbyen, Norway
- 4Department of Renewable Marine Resources, Institute of Marine Sciences (ICM-CSIC), Barcelona, Spain
- 5Institute of Marine Sciences, University of California Santa Cruz, Santa Cruz, CA, United States
- 6Stockholm Resilience Centre, Stockholm University, Stockholm, Sweden
- 7Centro Oceanografico de Vigo, Instituto Español de Oceanografía (IEO, CSIC), Vigo, Spain
- 8Ifremer, EMH, rue de l'île d'Yeu, BP 2011, Nantes, France
Editorial on the Research Topic
Managing for the Future: Challenges and Approaches for Disentangling the Relative Roles of Environmental Change and Fishing in Marine Ecosystems
Introduction: What Limits Our Understanding of Ecosystem Variability?
In the Anthropocene era, characterized by significant human impact on Earth's systems, the old saying “plus ça change, plus c'est la même chose” does not apply to the natural world. The oceans, long held to be relatively stable physically, biogeochemically and ecologically, are warming, acidifying, and de-oxygenating (Gruber, 2011; IPCC, 2019; Kwiatkowski et al., 2020). In short, marine ecosystems are changing (Doney et al., 2012; Hollowed et al., 2013; Gao et al., 2019), and in some areas of the globe, the change is very rapid (Henson et al., 2017; Johnson and Lyman, 2020). At the same time, human-induced stressors such as fisheries and pollution continue, with sometimes dramatic observed and predicted declines in commercial stocks (e.g., Vasilakopoulos et al., 2014; Lotze et al., 2019). Therefore, in the 21st century, fisheries and ocean managers require science advice that accounts for the cumulative effects of multiple stressors, fisheries removals, human-induced climate change, anthropogenic stressors, environmental variability, and trophic dynamics. Ongoing climate change and increasing pressure to intensify Blue Growth and the Blue Economy highlight the immediacy of the situation. There is strong need to better understand the impacts of these stressors and ecosystem variability so that we may proactively contribute to the management process.
In parts of the world, such as the data rich northern hemisphere, over a century of monitoring marine fisheries and ecosystems has produced a substantial database from which to study ecosystem response to climate change. This has contributed to the development of policies for sustainable management of fisheries and ecosystems, and in some jurisdictions, development of ecosystem-based action plans, such as the Baltic Sea Plan (HELCOM, 2007) and the Norwegian Sea Integrated Management Plan (Norwegian Ministry of Climate and Environment, 2017). In other parts of the world where there may be lower availability of observational data, shorter time series and fewer resources, the challenge of understanding ecosystem variability and thus managing for the future may be greater.
To date, we have had some success describing variability on multiple scales of space and time, and from the genetic to the ecosystem levels (e.g., Rijnsdorp et al., 2009; Petitgas et al., 2018). Managing for the future to mitigate the impacts of human-induced ecosystem change requires that we incorporate this variability, and its consequences, into management policies. This requires integration of empirical studies, modelling and the development of system indicators that describe relevant variability and change.
This Frontiers research topic (RT) “Managing for the future: understanding variability and the relative roles of environment, climate, fishing, and trophic dynamics in marine ecosystems” explores these questions using theoretical, empirical, and modelling approaches across a variety of spatial scales and levels of description. Here we review the contributions from these studies and identify remaining challenges.
Novel Approaches Yield New Knowledge
This RT presents a range of questions, approaches, and challenges to both understand system response to various climate and human-related drivers, and to develop scenarios of response to better inform management of marine ecosystems for the future. The 21 papers address ecosystem, species, and population-level effects over broad spatial and temporal scales using modeling and statistical approaches that represent the state of the art. Effective ecosystem and fisheries management is put into the important context of multiple drivers, especially climate change and fisheries activity. The span of these studies reveals some key commonalities, as well as important sources of variability that must be considered. Figure 1 summarizes the contributions across 5 dimensions: level of biological organization, spatial scale, research approach, stressor examined, and possibility for application of results to management. The bulk of studies address species or ecosystem levels on broader scales, with a split between empirical and modeling studies. In half the cases the combination of fisheries and climate change was studied, and most authors identified implications for management. In the following section, we highlight some of the main findings across these dimensions and how they address the theme of the RT.
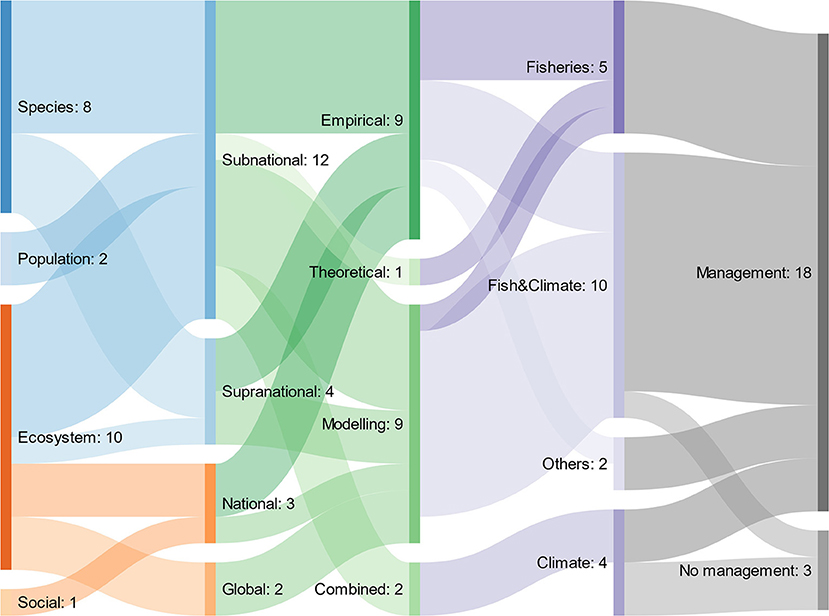
Figure 1. Sankey diagram summarizing the articles contributed to this research topic (source: http://sankeymatic.com/build/). Distribution of articles across (from left to right) level of organization, spatial scale, research approach, type of stressor studied, and whether there was an application to management discussed. Numbers represent the number of papers in each category.
Level of Organization
The level of organization considered in the suite of papers included population, species, ecosystem, and the social system, with most focusing on the species or ecosystem level (Figure 1). In general, studies at the ecosystem level used food-web or multi-species modelling approaches (see below) whereas those at the species and population levels used statistical approaches to explore questions such as environmental impacts on life history characteristics.
Processes at the base of ecosystems vary considerably across the year, often tracking seasonal cycles in primary production and phenology of taxa throughout the food web. Lloret-Lloret et al. linked seasonal variation in the spatial distribution of European hake (Merluccius merluccius) to ontogenetic changes and trophic ecology using species distribution models (SDMs) and further revealed substantial differences in trophic strategies between seasons and ontogenetic stages. Albo-Puigserver et al. used Generalized Linear and Additive Mixed Models (GLMs/GAMMs) to explore spatial/temporal variation in key life history traits of two small pelagic species (European sardine Sardina pilchardus and European anchovy Engraulis encrasicolus) from northern and southern regions of the Mediterranean Sea and concluded that the two species respond differently to environmental pressures in the north compared to the south. Véron et al., exploring the life history of European sardine in the Bay of Biscay, concluded that short term variability in length at maturation was “strongly dependent upon individual growth which is likely driven by environmental factors.” In the eastern US, black sea bass Centropristis striata distribution has shifted north into the Gulf of Maine. McMahan et al. used multivariate analysis and GLMs to explore life-history traits of black sea bass and found that sea bass from the newly expanded range had a less diverse diet, lower condition, and reached maturity at a younger age than fish from the historic region off southern Massachusetts. This result has implications for the stock assessment of this economically valuable species. Using GLMs, GAMs and Random Forest (RF) approaches, Liu et al. investigated Japanese anchovy Engraulis japonicus population dynamics and found strong relationships between environmental characteristics (salinity and sea surface temperature—SST) and catch statistics. These relationships were further linked to climatic oscillation indices.
One study directly addressed the critical level of the social system in determining the effects of fishing and climate change on marine ecosystems. Using social network analysis of results from semi-structured interviews, Obregón et al. identified the importance of communication flow among stakeholders, and the imbalance in this communication in managing a small-scale fishery. In this example, the public and academic stakeholders were the least connected. This study highlights structural barriers in the information-sharing network necessary for fully informed management decisions. Heymans et al. in an opinion piece, highlight such communication barriers between stakeholders as a major challenge for the Ocean Decade.
Scale of Study
Most papers in this RT were focused within national boundaries, including bays, shelves and seas, reflecting management mandates that rarely extend past national boundaries. However, large-scale studies are required when managing highly migratory populations/species, or when addressing general questions of broader trajectories of ecosystem response to changing pressures. Cleeland et al. used spatially resolved demographic modelling to isolate the effects of climate change, oceanography, fisheries effort, and habitat degradation on the demographic rates of multiple threatened albatross species. Results illustrated the need to integrate fisheries, oceanographic, and also terrestrial processes when assessing demographic variability and formulating the appropriate management response. Considering such a wide range of processes over broad scales and multiple ecosystems clearly shows the complexity of managing species with wide ecological ranges and habitat use. Coll et al. developed a global ecological model coupling climate, ocean conditions, and food-web dynamics (EcoOcean) which was used to assess the individual and combined effects of climate and fisheries in the global ocean. They concluded that fisheries overall exacerbates the negative impact of climate change, and weakens the positive consequences. Such “whole-ecosystem” models and their development are key steps toward an integrated approach to projecting ecosystem variability and developing plausible ocean-based solutions to mitigate and adapt to climate change.
Selection of spatial scales within which to study migratory species is complicated by residency patterns, and the exposure to different environmental and human-induced pressures, including climate change effects, encountered during migration. Papers by Lloret-Lloret et al. and Albo-Puigserver et al. (discussed above) demonstrate that temporal and spatial patterns of variability are often confounded, representing a challenge to management.
Research Approach
Approximately half the studies used one or more models to produce their results and half used empirical data, with two papers combining approaches (Figure 1). In this section we focus on the insights derived from novel modeling approaches.
The importance of spatial scale and variability in space was underscored by the use of spatial modelling approaches to address key ecological questions. Shannon et al., in an update of their temporal EwE model of the Benguela upwelling system, highlighted the need to consider various environmentally-driven changes, including upwelling effects on phytoplankton availability to zooplankton and small pelagic fish and geographic shifts in sardine distribution, in addition to trophic interactions and fishing effects. It concluded that spatially explicit ecosystem models would provide further mechanistic understanding of variability in the ecosystem. The spatially-explicit version of the Ecopath with Ecosim (EwE) marine ecosystem model, Ecospace, was used by Hernvann et al. to identify the relative importance of drivers of change in determining the structure and functioning of the Celtic Sea ecosystem. They found that integrating a spatial perspective helped explain the changing spatial distributions of commercial fish species, and the strong role of bottom-up processes (primary productivity) in the system. Fu et al. used the OSMOSE (Object-oriented Simulator of Marine ecOSystEms) model to understand how stressors interactively and cumulatively affect commercially important fish species. They simulated favorable and unfavorable conditions based on varying food supply at the base of the food web and different levels of fishing pressure, and found synergistic effects of the two drivers, with more pronounced negative effects. Travers-Trolet et al. used OSMOSE to assess the performance of fisheries management tools under climate change. They explored the management-relevant response of two main biological reference points, Maximum Sustainable Yield (MSY) and fishing mortality at MSY (FMSY), to two contrasting climate change scenarios, and showed that both MSY and FMSY of overexploited cold-water species were likely to decline with climate warming.
In a creative use of bio-energetic models, Koen-Alonso et al. “transplanted” cod from the Barents Sea to the Newfoundland-Labrador model and vice-versa by switching estimated key parameters between models and then following spatial dynamics in cod stocks. They concluded that the stocks were biologically similar and that differences in their trajectories were due to lack of prey (capelin) in Newfoundland. In a more general sense, this highlights a message in the RT: the need to integrate food-web perspectives into dynamic models that integrate climate and other drivers.
Ensemble modeling was advocated by Pethybridge et al., who demonstrated that this is a robust means of exploring ecosystem responses to climate change in the face of uncertainty about the structure, function, and change in marine ecosystems. The ensemble approach enabled them to include confidence intervals in their results and account for uncertainty in model structure. Their results indicate that demersal systems appear to be more susceptible to climate change than pelagic systems. This perspective is central in the work of Horn et al., who reviewed the modeling literature on climate change effects on coastal ecosystems. They conclude that models that couple deterministic ocean models and food-web models will be essential tools for providing scenarios to guide ecosystem-based management.
Stressors and Multiple-Stressor Effects
Analysis of cumulative effects, using both modelling and statistical approaches, was a common theme in this RT. Stressors examined mainly included fishing and climate change, and results clearly demonstrate the negative cumulative effects of both (e.g., Coll et al., Fu et al., Pennino et al., Travers-Trolet et al.). Hernvann et al., (described above) for example, demonstrated the predominance of fisheries impacts over climate change. Subramaniam et al. using EwE, found the opposite: climate change effects much greater than those from fishing in the Southern Ocean region of the Kerguelan Plateau. Here the authors suggest current management strategies are sustainable, but fishing effort here is relatively low to other areas studied, including the Celtic and Yellow Seas. Stenson et al. investigated three harp seal populations in the North Atlantic and identified an interactive effect between climate conditions and hunting pressure. Importantly, trends and responses among the three populations varied, with important consequences for management now and in the future. These results complement the studies of Fu et al. and Coll et al. who also found synergistic effects between fishing and climate change.
The main pathway explored for climate impacts was through bottom-up action. This was exemplified by Friedland et al. for the northeast coast of the US, one of the most rapidly warming marine ecosystems. Using time series analyses and GLMs, these authors explored the changes in SST, salinity, and chlorophyll concentration (chl) in 5 areas and their impacts on higher trophic levels. They showed that a decrease in chl and a shift to smaller species of phytoplankton has been observed with warming temperatures. Declines in fish and benthic invertebrate populations were linked to lower chl or increased temperature. Whereas, it is logical that climate effects through potential resource availability (chl) and energetics (SST) should be a dominant driver of ecosystem variability, it is interesting to note that Shannon et al.'s EwE study from Benguela indicated specific climate-sensitive trophic links as being decisive in how the ecosystem responds to climate change.
In a study of the robustness and sensitivity of fish community structure indicators, Li et al. investigated the response of 22 indicators of fish community structure to 3 types of pressures, fishing, large-scale climate change, and regional environmental variables in the Yellow Sea. Using INDperform (Otto et al., 2018), they showed that most indicators were more sensitive to fishing than climate change, with some notable exceptions (e.g., temperature of catch, functional evenness of thermally defined fish groups).
Management Applications
Over 80% of the studies in this RT indicate that their research results are relevant to management. In the long view, this is certainly true, but as Obregón et al. pointed out, the academic community is one of the least interactive stakeholders in the decision-making process. Indeed, this is a well-known disconnect in the process of policy development (Stephenson et al., 2021). We identify five results from studies in this RT that can likely be transferred directly to managers for integration into policy. Fu et al., Cleeland et al. and Travers-Trolet et al. provide specific population and demographic parameters and fisheries indicators that can be integrated into management strategies. As just mentioned, Shannon et al. identified sensitive trophic links that could be monitoring or mitigation targets. Finally, Pennino et al. used the results from their RF analysis of environmental drivers of European sardines and anchovies in the Northwestern Mediterranean to test the past and future impact of different climate change scenarios using species distribution models. They identified specific locations that could serve as future refuges for these species in the face of climate change. Clearly, these areas can become immediate targets for conservation/protection to secure the species for the future within a regional context of overexploitation. Coll et al.'s global modelling results are part of a larger ensemble of model projections initiative (Tittensor et al., 2018) that informs global scientific initiatives within the Intergovernmental Panel on Climate Change (IPCC) context to motivate management action to halt climate change (Lotze et al., 2019).
Other studies have produced relevant tools and findings for management, but work remains if these results are to be immediately useful in development of management, mitigation, and adaptation policies.
Main Challenges
The studies outlined in this RT demonstrate excellent, innovative science with the potential of being used by managers. However, challenges remain and we must continue to work to address knowledge gaps and needs for methodological improvements and developments. Below we include a short, non-prioritized and non-exhaustive discussion of the challenges in incorporating marine ecosystem variability into assessment and management.
Tractability of Multiple Stressors
At any time, an ecosystem is affected by multiple climate-related, human-induced and natural drivers, each with direct and indirect pathways of action on target response variables. This makes interpreting impacts of specific stressors, including such multi-faceted stressors as climate change, challenging. Some recent approaches to explore cumulative effects that were not covered in this RT include statistical and dynamical modeling, such as path analysis (Tenenhaus et al., 2005; Fu et al., 2015; Selim et al., 2016), empirical dynamic modelling (Perretti et al., 2013; Munch et al., 2020), ecosystem dynamic programming (Brias and Munch, 2021) and Gaussian graphical models (Liang et al., 2021). Existing knowledge of system dynamics gained from decades of scientific study provides a solid baseline from which to explore the effects of multiple stressors, but the challenge increases as we enter a period without historical (or prehistorical) precedent. Experimental studies and mathematical simulations can shed light onto the process behind the observed pattern, and determining interactions of multiple stressors can be simplified through reduced factorial designs (Boyd et al., 2018).
Studying Processes Over Relevant Spatial and Temporal Scales
Climate change occurs over decadal and multi-decadal scales, time scales beyond most empirical studies. It also changes baselines for comparison and control. Long time-series exist and are often used in empirical studies to test for effects of different drivers on ecosystem variability; and these offer interesting insights. However, observational data on ecosystem drivers (e.g., fishing effort, pollution, pH) are not always collected in comparable ways over long temporal scales, making analysis of its effects challenging. Migrations and species range extensions can complicate evaluating processes taking place in a single region as these organisms are affected by changes elsewhere. Understanding these limitations/challenges when designing studies and interpreting results is critical.
Model Development Needs and Quantification Uncertainty
Statistical and mechanistic modeling techniques are probably the best options for integrating climate, human impacts, environmental variability, and ecosystem interaction in a meaningful way. As the studies above point out, increased use of spatially-explicit models, multiple spatio-temporal scales and coupling of ecosystem and trophic models are key elements for explaining ecosystem variability that need further development. However, current challenges include validation and fitting models to data, which is especially problematic for more complex models that require both more high-quality data for validation and supercomputer access. Quantifying uncertainty in models is also key and includes measurement uncertainty, parameter uncertainty and structural uncertainty (Payne et al., 2016). Methods to account for measurement and parameter uncertainty include Monte Carlo analysis and Bayesian approaches (Dilks et al., 1992; Spiegelhalter and Best, 2003), and ensemble modelling is one approach to account for structural uncertainty (Pethyridge et al.; Lotze et al., 2019).
On smaller spatial scales, relevant downscaling methods and incorporation of local factors that can have significant structuring impacts (e.g., variation in flow from small rivers) are important to consider. At some levels of organization, seasonal data are needed to fully understand the (varying) links within the ecosystem, and thus their sensitivity to change (Lloret-Lloret et al.).
In their opinion piece, Heymans et al. point out that we also need to enhance research and modeling impact through capacity building and co-design of models with different forms of knowledge and working “together to achieve the ensemble of well-parameterized, calibrated and validated ecosystem models needed to address the questions asked of us in the Ocean Decade.”
Integrating Social Dimensions
Humans are part of what has been termed a socio-ecological system (SES, Berkes et al., 2000; Colding and Barthel, 2019). SES are complex, integrated systems consisting broadly of humans (social) and ecological (biophysical) subsystems, between which there exist two-way feedback relationships. This RT did not explicitly discuss SES, but human activity affects and is affected by ecosystem structure and function, and variability in these parameters. Climate change produces yet another nested network of impacts and effects that are embedded in decisions about resource extraction, protection of natural systems, and adaptation strategies for sustainable activities. Environmental management is a socio-political decision, where academic science may be detached from decision making processes. Thus, co-generation of goals and strategies of scientific study with multiple stakeholders is urgently needed.
Communication of Results to Management
It is a well-acknowledged point of frustration for empiricists and ecosystem modelers that scientific results are often not directly integrated into management plans. In this RT, Heymans et al. challenged the marine ecosystem modelling community to address how we: (i) enable ocean managers and decision-makers to use our science, (ii) communicate our science, and most importantly (iii) ensure co-design of our science to achieve sustainable development. Five papers were identified to contain very specific advice, ready to integrate into policy (see above). These examples can offer a roadmap for successfully bridging the gap between science and management. Concrete targets for protection or monitoring and use of accepted, robust, and easily defined indicators make implementation of advice more straightforward. Dialogue between scientists and managers defining specific study questions and contexts for presenting findings can also help.
Conclusions
The studies presented in this RT provide new knowledge and perspectives on impacts of fisheries and climate change on marine ecosystems and species. In addition, they demonstrate use of new methods and combinations of methods, and offer complementary results from different systems to investigate generalities in findings. The importance of spatial and temporal scale is woven through many of the papers and must be integrated to address important management questions. However, translating science into advice for management remains a frontier that was not fully explored here. The nature and type of science advice required for management will differ across issues and spatial/jurisdictional scales. For the former, it is perhaps more straightforward to provide advice when dealing with single species issues rather than ecosystem concerns. Similarly, science advice is required at multiple scales (local, regional, national international), and while it may be most straightforward at the local scale, stated goals of ecosystem-based management, which are context specific, require consideration of multiple species and scales. Regardless, the current climate crisis creates a pressing need for better communication of research findings to end-users.
Author Contributions
AB and PR conceived the idea for the RT and led the writing of the editorial. MC created Figure 1. All authors reviewed manuscripts in the RT and contributed ideas and text.
Conflict of Interest
The authors declare that the research was conducted in the absence of any commercial or financial relationships that could be construed as a potential conflict of interest.
Publisher's Note
All claims expressed in this article are solely those of the authors and do not necessarily represent those of their affiliated organizations, or those of the publisher, the editors and the reviewers. Any product that may be evaluated in this article, or claim that may be made by its manufacturer, is not guaranteed or endorsed by the publisher.
Acknowledgments
This RT originated from a session led by the CoArc project at the IMBeR 2019 Open Science Conference, Future Oceans2, held in Brest, France on 17-21 June 2019. This is a contribution of the CoArc project, which received funding from the Norwegian Foreign Ministry and its Arctic 2030 program. PR acknowledges support from the Research Council of Norway through ‘The Nansen Legacy project’ (RCN no. 276730). MC acknowledges funding from the European Union's Horizon 2020 research and innovation programme under grant agreement No. 817578 (TRIATLAS project) and the Institutional Support of the ‘Severo Ochoa Centre of Excellence’ accreditation (CEX2019-000928-S) to the Institute of Marine Science (ICM-CSIC; Barcelona, Spain).
References
Berkes, F., Folke, C., and Colding, J. (Eds.). (2000). Linking Social and Ecological Systems: Management Practices and Social Mechanisms for Building Resilience. Cambridge: Cambridge University Press.
Boyd, P. W., Collins, S., Dupont, S., Fabricius, K., Gattuso, J. P., Havenhand, J., et al. (2018). Experimental strategies to assess the biological ramifications of multiple drivers of global ocean change—a review. Glob. Chang. Biol. 24, 2239–2261. doi: 10.1111/gcb.14102
Brias, A., and Munch, S. B. (2021). Ecosystem based multi-species management using Empirical Dynamic Programming. Ecol. Model. 441:109423. doi: 10.1016/j.ecolmodel.2020.109423
Colding, J., and S. Barthel. (2019). Exploring the social-ecological systems discourse 20 years later. Ecol. Soc. 24:2. doi: 10.5751/ES-10598-240102
Dilks, D. W., Canale, R. P., and Meier, P. G. (1992). Development of Bayesian Monte Carlo techniques for water quality model uncertainty. Ecol. Model. 62, 149–162. doi: 10.1016/0304-3800(92)90087-U
Doney, S. C., Ruckelshaus, M., Emmett Duffy, J., Barry, J. P., Chan, F., English, C. A., et al. (2012). Climate change impacts on marine ecosystems. Annu. Rev. Mar. Sci. 4, 11–37. doi: 10.1146/annurev-marine-041911-111611
Fu, C., Large, S., Knight, B., Richardson, A. J., Bundy, A., Reygondeau, G., et al. (2015). Relationships among fisheries exploitation, environmental conditions, and ecological indicators across a series of marine ecosystems. J. Marine Syst. 148, 101–111. doi: 10.1016/j.jmarsys.2015.01.004
Gao, K., Beardall, J., Häder, D. P., Hall-Spencer, J. M., Gao, G., and Hutchins, D. A. (2019). Effects of ocean acidification on marine photosynthetic organisms under the concurrent influences of warming, UV radiation, and deoxygenation. Front. Marine Sci. 6:322. doi: 10.3389/fmars.2019.00322
Gruber, N. (2011). Warming up, turning sour, losing breath: ocean biogeochemistry under global change. Philos. Transac. R. Soc. A 369, 1980–1996. doi: 10.1098/rsta.2011.0003
HELCOM (2007). “HELCOM baltic sea action plan,” in Proceedings of the Adopted by the HELCOM Ministerial Meeting (Krakow).
Henson, S. A., Beaulieu, C., Ilyina, T., John, J. G., Long, M., Séférian, R., et al. (2017). Rapid emergence of climate change in environmental drivers of marine ecosystems. Nat. Commun. 8:14682. doi: 10.1038/ncomms14682
Hollowed, A. B., Barange, M., Beamish, R. J., Brander, K., Cochrane, K., Drinkwater, K., et al. (2013). Projected impacts of climate change on marine fish and fisheries. ICES J. Mar. Sci. 70, 1023–1037. doi: 10.1093/icesjms/fst081
IPCC (2019). “IPCC special report on the ocean and cryosphere in a changing climate,” in Working Group II Technical Support Unit Intergovernmental Panel on Climate Change, eds H.- O. Pörtner, D. C. Roberts, V. Masson-Delmotte, P. Zhai, M. Tignor, et al. Available online at: https://www.ipcc.ch/site/~assets/uploads/sites/3/2019/12/SROCC_FullReport_FINAL.pdf
Johnson, G. C., and Lyman, J. M. (2020). Warming trends increasingly dominate global ocean. Nat. Clim. Change 10, 757–761. doi: 10.1038/s41558-020-0822-0
Kwiatkowski, L., Torres, O., Bopp, L., Aumont, O., Chamberlain, M., Christian, J. R., et al. (2020). Twenty-first century ocean warming, acidification, deoxygenation, and upper-ocean nutrient and primary production decline from CMIP6 model projections. Biogeosciences 17, 3439–3470. doi: 10.5194/bg-17-3439-2020
Liang, D., Nesslage, G. M., Wilberg, M. J., and Miller, T. J. (2021). Ranking ecosystem impacts on Chesapeake Bay blue crab (Callinectes sapidus) using empirical Gaussian Graphical Models. Can. J. Fish. Aquat. Sci. 78, 245–254. doi: 10.1139/cjfas-2019-0439
Lotze, H. K., Tittensor, D. P., Bryndum-Buchholz, A., Eddy, T. D., Cheung, W. L., Galbraith, E. D., et al. (2019). Global ensemble projections reveal trophic amplification of ocean biomass declines with climate change. Proc. Natl. Acad. Sci. U.S.A. 116, 12907–12912.
Munch, S. B., Brias, A., Sugihara, G., and Rogers, T. L. (2020). Frequently asked questions about nonlinear dynamics and empirical dynamic modelling. ICES J. Marine Sci. 77, 1463–1479. doi: 10.1093/icesjms/fsz209
Norwegian Ministry of Climate and Environment. (2017). Update of the integrated management plan for the Norwegian Sea. Oslo: St. Meld. 35. Norwegian Parliament.
Otto, S. A., Kadin, M., Casini, M., Torres, M. A., and Blenckner, T. (2018). A quantitative framework for selecting and validating food web indicators. Ecol. Indic. 84, 619–631. doi: 10.1016/j.ecolind.2017.05.045
Payne, M. R., Barange, M., Cheung, W. W., MacKenzie, B. R., Batchelder, H. P., Cormon, X., et al. (2016). Uncertainties in projecting climate-change impacts in marine ecosystems. ICES J. Marine Sci. 73, 1272–1282. doi: 10.1093/icesjms/fsv231
Perretti, C. T., Munch, S. B., and Sugihara, G. (2013). Model-free forecasting outperforms the correct mechanistic model for simulated and experimental data. Proc. Natl. Acad. Sci. U.S.A. 110, 5253–5257. doi: 10.1073/pnas.1216076110
Petitgas, P., Huret, M., Dupuy, C., Spitz, J., Authier, M., Romagnan, J. B., et al. (2018). Ecosystem spatial structure revealed by integrated survey data. Prog. Oceanogr. 166, 189–198. doi: 10.1016/j.pocean.2017.09.012
Rijnsdorp, A. D., Peck, M. A., Engelhard, G. H., Möllmann, C., and Pinnegar, J. K. (2009). Resolving the effect of climate change on fish populations. ICES J. Marine Sci. 66, 1570–1583. doi: 10.1093/icesjms/fsp056
Selim, S. A., Blanchard, J. L., Bedford, J., and Webb, T. J. (2016). Direct and indirect effects of climate and fishing on changes in coastal ecosystem services: a historical perspective from the North Sea. Reg. Environ. Change 16, 341–351. doi: 10.1007/s10113-014-0635-7
Spiegelhalter, D. J., and Best, N. G. (2003). Bayesian approaches to multiple sources of evidence and uncertainty in complex cost-effectiveness modelling. Stat. Med. 22, 3687–3709. doi: 10.1002/sim.1586
Stephenson, R. L., Hobday, A. J., Allison, E. H., Armitage, D., Brooks, K., Bundy, A., et al. (2021). The quilt of sustainable ocean governance: Patterns for practitioners. Front. Marine Sci. 8:120. doi: 10.3389/fmars.2021.630547
Tenenhaus, M., Vinzi, V. E., Chatelin, Y. M., and Lauro, C. (2005). PLS path modeling. Comput. Stat. Data Anal. 48, 159–205. doi: 10.1016/j.csda.2004.03.005
Tittensor, D. P., Eddy, T. D., Lotze, H. K., Galbraith, E. D., Cheung, W., Barange, M., et al. (2018). A protocol for the intercomparison of marine fishery and ecosystem models: Fish-MIP v1.0. Geosci. Model Dev. 11, 1421–1442. doi: 10.5194/gmd-2017-209-supplement
Keywords: ecosystem variability, management, multiple stressors, cumulative effects, spatial scale, climate change, fisheries
Citation: Bundy A, Renaud PE, Coll M, Koenigstein S, Niiranen S, Pennino MG, Tam JC and Travers-Trolet M (2021) Editorial: Managing for the Future: Challenges and Approaches for Disentangling the Relative Roles of Environmental Change and Fishing in Marine Ecosystems. Front. Mar. Sci. 8:753459. doi: 10.3389/fmars.2021.753459
Received: 04 August 2021; Accepted: 08 September 2021;
Published: 08 October 2021.
Edited and reviewed by: Yngvar Olsen, Norwegian University of Science and Technology, Norway
Copyright © 2021 Bundy, Renaud, Coll, Koenigstein, Niiranen, Pennino, Tam and Travers-Trolet. This is an open-access article distributed under the terms of the Creative Commons Attribution License (CC BY). The use, distribution or reproduction in other forums is permitted, provided the original author(s) and the copyright owner(s) are credited and that the original publication in this journal is cited, in accordance with accepted academic practice. No use, distribution or reproduction is permitted which does not comply with these terms.
*Correspondence: Alida Bundy, alida.bundy@dfo-mpo.gc.ca