- 1College of Marine Sciences, Shanghai Ocean University, Shanghai, China
- 2National Engineering Research Center for Oceanic Fisheries, Shanghai Ocean University, Shanghai, China
- 3Key Laboratory of Sustainable Exploitation of Oceanic Fisheries Resources, Ministry of Education, Shanghai Ocean University, Shanghai, China
- 4Fisheries College, Ocean University of China, Qingdao, China
The estuarine areas provide necessary spawning habitat, nursing habitat, and migratory for a variety of fishes and the environmental conditions are of significant heterogeneity. Coilia mystus is the key commercial fish that spawns in the Yangtze Estuary and the yield has declined sharply in the past 30 years. In order to understand the spawning habitat selection mechanism of C. mystus, the geographically weighted regression (GWR) model was applied to explore the non-stationary effect of environmental variables [e.g., the sea surface temperature (SST) and the sea surface salinity (SSS)] and geographical variables [e.g., the distance to the coast (DTC) and the depth (DEP) of water] on the egg density distribution of C. mystus. The data were derived from the spring and summer ichthyoplankton surveys carried out from 2019 to 2020 in the Yangtze Estuary, China. The GWR model results showed that the key variables in different seasons had spatial non-stationary effects on the distribution of spawning habitat of C. mystus, which were mainly caused by regional rise in temperature and saltwater intrusion of the South Branch. In the spring, the SSS, the DTC, and the DEP were the main impact factors and saltwater intrusion in the South Branch might lead to the trend that the spawning habitat moved further upstream to the Changxing Island. The SST was most important in the summer and the relatively lower SST was more crucial in the spawning habitat selection than the DTC and the DEP. The GWR model performed well in the study of C. mystus potential spawning habitat in the Yangtze Estuary. We recommend that more attention should be paid in regional variation of environmental factors to explore fish potential spawning habitat in the estuarine areas.
Introduction
The distribution of fish spawning habitat is usually determined by the adaptation of reproductive physiology and species response to the surrounding environmental conditions (Gallego et al., 2007; Zorica et al., 2013; Gonzalez-Irusta and Wright, 2016; Reglero et al., 2017). The spawning distribution could be influenced by various environmental factors, e.g., temperature, salinity, topography (Opdal, 2010; Dumont et al., 2011; Shi et al., 2014). There is evidence that compared with adults, the early life history stages of fish species seem to be more sensible and vulnerable to the variations in the environmental conditions (Portner and Farrell, 2008). To ensure more survival of eggs and larvae, spawning activity usually happens in the recurrent hydrographic features (Munk et al., 2009) such as estuaries, upwellings, or tidal mixing front (Tian et al., 2009; Zorica et al., 2013; Gibson et al., 2018). The estuaries, as important fish spawning and nursery habitats, are usually characterized by complex hydrological systems and spatially varying environmental conditions (Andrew et al., 2009). A study on fish spawning habitat is the fundamental step for the fish recruitment process (Lelievre et al., 2014), especially for the estuarine species. Examining fish spawning activity and its response to the spatial non-stationary environment is important for gaining a comprehensive understanding of spawning habitat selection mechanism, which helps to understand fish stock fluctuations and, hence, establish appropriate protection strategies for estuarine fish.
To evaluate statistical relationships between the spawning habitat and environmental variables, “global” regression models (Brunsdon et al., 1996; Windle et al., 2010) such as generalized additive model (GAM) and generalized linear model (GLM) are fully developed and used (Bacheler et al., 2010; Asch and Checkley, 2013; Gonzalez-Irusta and Wright, 2016). These models treat the entire data set as “whole map” and function under the assumption of spatial stationarity (Brunsdon et al., 2002; Windle et al., 2010). In the recent years, more and more studies have focused on spatial heterogeneity and the changes in species distribution caused by variations in the environmental conditions (Li et al., 2018). The geographically weighted regression (GWR) model provides a promising approach for investigating spatial non-stationarity effect and could make good predictions of fish species distribution (Lauri et al., 2014). The GWR model has been applied successfully to both the marine and freshwater habitats, e.g., North Atlantic Ocean (Windle et al., 2010), Gulf of Maine (Li et al., 2018), and Lake Malawi (Likongwe et al., 2015). However, rarely study has been reported that applies the GWR model method to demonstrate the non-stationary effect of the environment on the fish spawning habitat.
The Yangtze Estuary in China is the largest estuary in the western Pacific Ocean with three brunches and four outlets to the East China Sea (Figure 1). It is characterized by high biological productivity associated with relatively extreme and varying environmental conditions (Shan et al., 2010). It serves as an important spawning habitat, nursing ground, and migration channel for varieties of fish species because of the advantaged conditions (Zhuang et al., 2006; Yu and Zhang, 2017). More than 300 fish species have been found inhabiting the Yangtze Estuary (Zhuang et al., 2006). Among them, Coilia mystus is considered one of the most important commercial fish species in the Yangtze Estuary (Shi and Wang, 2002) with an annual yield of more than 1,500 tons in the 1990s (Ni, 1999). However, in 2019, China had to announce that it would stop issuing special fishing licenses for the wild populations of C. mystus because of the severe depletion of the resources (Zhai and Pauly, 2019, 2020) caused by overfishing and the habitat degradation (Ni et al., 1999; Liu et al., 2013).
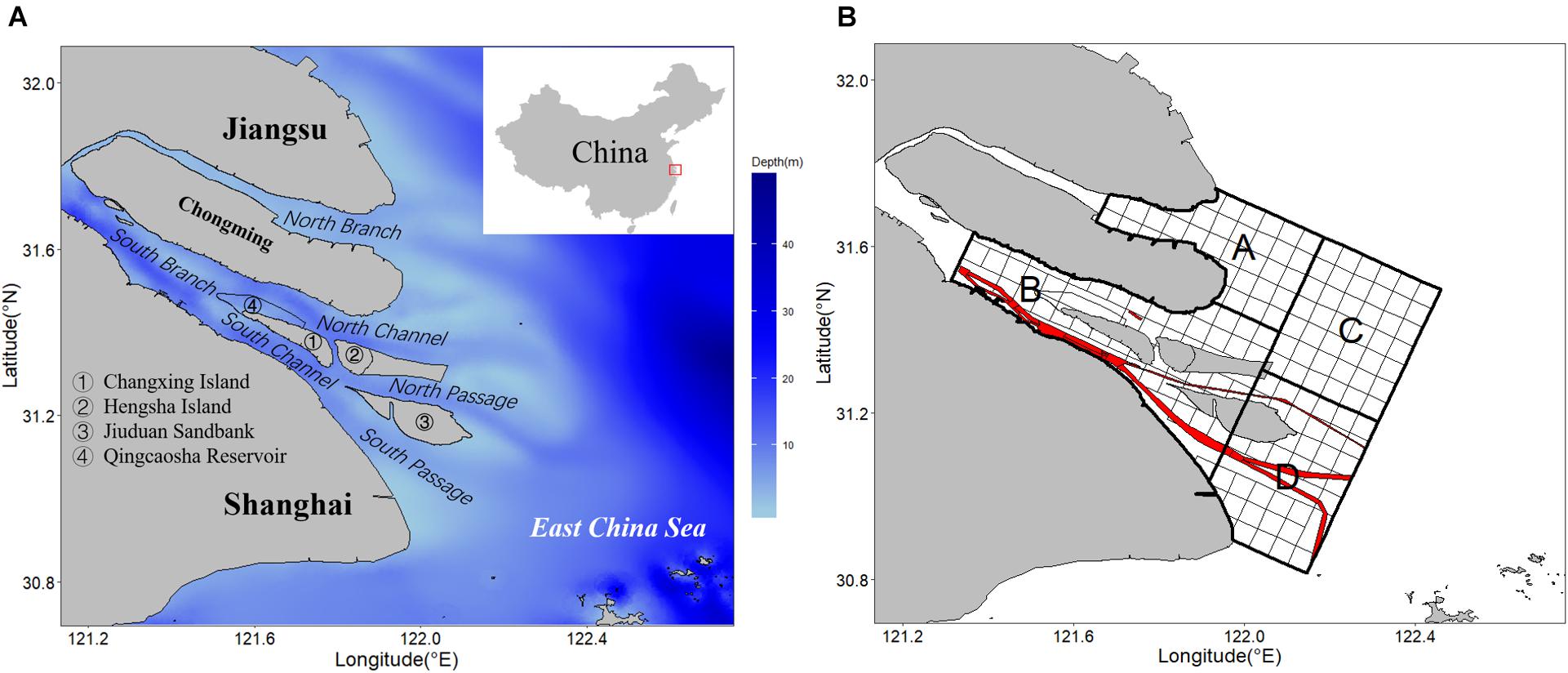
Figure 1. (A) Study area with depth of water in the Yangtze Estuary. The red rectangle on the China map is the location of the Yangtze Estuary. (B) Subareas A, B, C, and D. Red line is the Deepwater Navigation Channel (DNC).
Coilia mystus belongs to Clupeiformes, with a short lifespan, seasonal migrations, and long spawning periods (Zhuang et al., 2006; Zhao et al., 2020). Also, it is a semi-anadromous fish (Elliott et al., 2007) that generally lives in the shallow marine area and spawns in the estuarine area (Zhuang et al., 2006; Zhao et al., 2020). The South Branch of the Yangtze Estuary is considered the main spawning location for C. mystus (Ni et al., 1999). The spawning period of C. mystus starts from late April to September and peaking in late May and June (Zhuang et al., 2006). In the spawning season, adults of C. mystus migrate to the brackish water in the Yangtze Estuary to spawn, but will never enter the freshwater areas above the Jiangyin section of the river (Yang et al., 2006b). There are few studies on the selection mechanism of spawning habitat of C. mystus and its response to environmental conditions, except for some previous work examining the migration pattern and biology trait (Yang et al., 2006a; Zhao et al., 2020). It is generally believed that the sea surface temperature (SST) and the sea surface salinity (SSS) would influence the spawning habitat of C. mystus (Jiang et al., 2006; Jiang and Shen, 2006). However, some geographical variables such as the distance to the coast (DTC) and the depth (DEP) of water, which were involved in other estuarine ichthyoplankton study (Locke and Courtenay, 1995; Halvor et al., 2007), were barely explored. An updated study on spawning activity and specific locations of spawning habitat in the area is necessary. Especially, understanding how the spawning habitat of C. mystus shifts over subtle spatial changes in the environment is the key problem.
In this study, the spawning habitat selection mechanism under the locally varying environmental conditions was explored based on a case study of C. mystus in the Yangtze Estuary. The spatial GWR model was applied to investigate the non-stationary effect of the environmental variables, i.e., the SST, the SSS, and geographical variables, i.e., the DTC and the DEP on the distribution of spawning habitat of C. mystus. The conclusions are helpful in gaining a comprehensive understanding of fish spawning habitat selection mechanism in the estuarine areas and conductive to the protection of the population.
Materials and Methods
Sample Collection and Analysis
The biological and environmental data were collected from four ichthyoplankton surveys carried out in 2019 and 2020 (Table 1) covering the peak and end of the spawning period of C. mystus. The sampling area covered both the South and North Branches of the Yangtze Estuary and its coastal zones (Figure 1). Considering the navigation risk and difficulties, the Deepwater Navigation Channel (DNC) was not involved in the survey. The study area was divided into four subareas (A, B, C, and D) based on the topographic features and salinity condition in the Yangtze Estuary (Luo and Shen, 1994; Yan et al., 2001). Besides, it was divided into 159 oblique grids of equal size 3′ × 3′ (Figure 1B) based on the central axis of the Chongming Island. In each cruise, around 55 stations were chosen from the grids by using a stratified random sampling method. A higher weight (25 stations) was allocated in subarea B according to the historical study of C. mystus spawning habitat (Ni et al., 1999).
The surveys started around 4 a.m. and were conducted in the day. Eggs were collected by a plankton net with an opening diameter of 80 cm and a mesh size of 505 μm. At each survey station, the net was towed horizontally against the current at a speed of 2–3 knots for a duration of 10 min and a calibrated flow meter was mounted in the net opening to capture the filtered water volume. Samples were gathered from the net and fixed in a 5% buffered formalin seawater solution immediately for further identification in the laboratory. Eggs of C. mystus have distinguishing features such as spherical with a diameter of 0.83–1.03 mm, transparent, and contain multiple oil globules with different sizes (Zhang et al., 1985). The eggs were identified by morphology observation under a microscope according to the study by Thompson and Riley (1981) and Zhang et al. (1985). The identified eggs were standardized in terms of number of specimens per cubic meter (ind m–3). The stations where no specimens were found were recorded as zero. The environmental profile was recorded by the SBE 19plus V2 SeaCAT conductivity-temperature-depth system (CTD). SST (°C) and SSS were defined by mean data of 3 m below the sea surface. Spatial data such as the DEP (m) was derived by the electronic charts and the DTC (km), the shortest distance from the station to the coast or islands, was calculated in use of the R package “raster.”
Data Analysis
Model Development
The GWR model was applied to evaluate the relationship between the distribution of spawning habitat of C. mystus and the environmental constraints for each month. The square root of egg density with corresponding independent variables was formulated as below (Gollini et al., 2015):
Where, yi is the dependent variable at location i, xik is the value of kth independent variable at location i, m is the number of independent variables, βik is the local regression coefficient for the kth independent variable at location i, and є i is random error at location i. All the model development is based on the R package “GWmodel”.
The spatial weighting matrix of the GWR model was built of three key elements: the type of distance, the kernel function, and the bandwidth. In this study, a great circle distance matrix, bisquare kernels function, and “adaptive” bandwidth (number of local data) were applied. Besides, bandwidth was obtained via cross-validation. For more detail, please see Gollini et al. (2015).
Before model development, collinearity between explanatory variables in four months was tested based on variance inflation factors (VIFs). By using a GLM model including all the independent variables (e.g., SST, SSS, DTC, DEP), a given predictor with VIFs greater than 10 should be removed from the model development (O’Brien, 2007). The result of the diagnostics of collinearity (Figure 2) showed that only SSS in August 2019 had a collinearity relationship with other indicators. However, the VIFs (10.16) were close to 10, so it was still involved in the following model development.
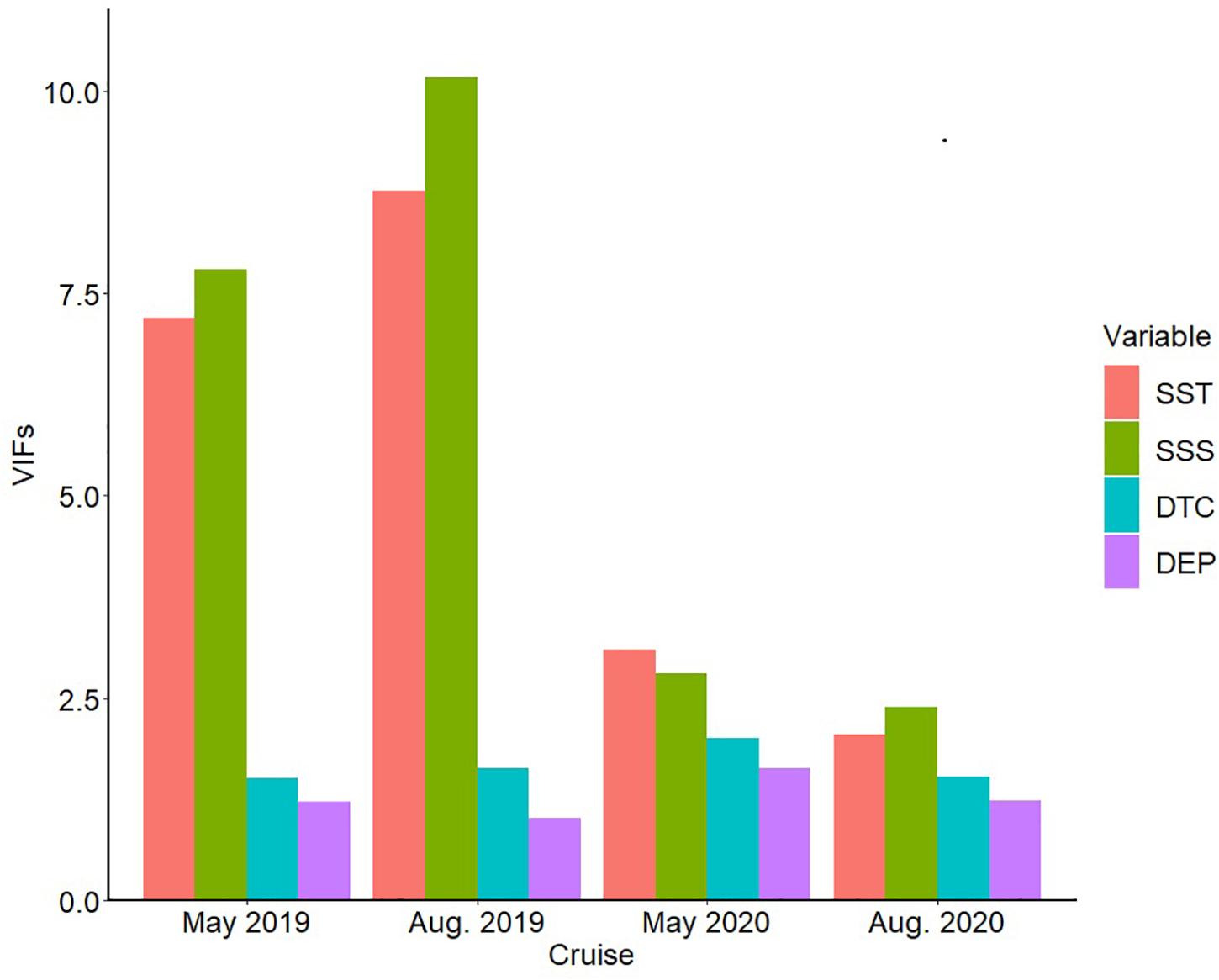
Figure 2. Monthly variance inflation factor (VIFs) of all the selected abiotic variables in the Yangtze Estuary in 2019 and 2020.
A global selection procedure (Brooks et al., 2014) was conducted by the GWR model to find the optimal model for each month and the best performing model comes to a variable combination that produces the minimum akaike information criterion (AIC) value among all the regressing models. Among four independent variables analyzed (SSS, SST, DTC, and DEP), the optimal independent variable subset for models showed both the consistency and difference. The DTC was subsequently involved in all the four models. The DEP was included in all the models, except for May 2020. The SSS was only removed from the model in August 2020 and the SST was included in August 2019 and 2020.
Model Fit and Prediction
The GWR model fit was tested by the Pearson correlation coefficient (r2). Significant levels (p) of spatial autocorrelation in regression residuals were evaluated by the Moran’s I statistics. As the quantity of data in each month is small, the leave-one-out cross-validation (LOOCV) procedure was used to obtain test errors (Molinaro et al., 2005). In this procedure, each station was sequentially picked to be the validation data and the remaining data were used as the training data. Meanwhile, the root mean square error (RMSE) was used to validate the model (Christoph and Jose, 2012). The RMSE can be calculated as follows:
Where, N is the number of samples and is the predicted value from the model for the ith observation. In the GWR model prediction, the entire survey area was divided into 6,000 oblique grids of equal size 0.52′ × 0.52′ and the selected environmental variables were evaluated by inverse distance weighting based on the survey data from each cruise.
Results
Model Performance and Validation
Based on the minimum AIC scores, the GWR models that best approximated the observed distribution of C. mystus eggs were listed in Table 2. Compared to the total number of stations (Table 1), the year 2019 required a larger bandwidth size than the year 2020 to develop the GWR model, in which model of August 2019 yielded results increasingly close to the universal model. Smaller bandwidth for models in other months might lead to more rapid spatial variations in the results. Moran’s I of residuals for the GWR models were randomly structured and the relevant p-value (0.13–0.78) indicated that spatial autocorrelation was not significant (Windle et al., 2010). In addition, the GWR models had good performance according to r2 (0.53–0.69).

Table 2. Summary of optimal bandwidth and model performance for the geographically weighted regression (GWR) models.
The GWR models had reasonable prediction skills with RMSE ranging from 0.05 to 0.24 (Table 2). In general, the observed C. mystus eggs were confined in the South Branch, near the Chongming, Changxing, and Hengsha Islands (Figure 3). The predicted distribution of egg concentration was broadly consistent with the pattern where most C. mystus eggs appeared. The monthly and interannual variations in the predicted egg density basically corresponded to that of the survey data. There were far more eggs collected in May than in August. In May 2019, the highest egg density was up to 0.34 ind.m–3 (predicted 0.26 ind.m–3), while it was only 0.07 ind.m–3 (predicted 0.08 ind.m–3) in August. Besides, the egg density sampled in 2020 was higher compared with that in the same month in 2019. Of the four months compared, the highest egg density was 2.72 ind.m–3 (predicted 1.61 ind.m–3) in May 2020 and eggs were highly aggregated in the upper end of the Changxing Island. In August 2020, the highest egg density was as much as 0.32 ind.m–3 (predicted 0.14 ind.m–3). Although there were a few eggs sampled in the North Branch (3 eggs in May 2019 and 2 eggs in May 2020) and subarea C (16 eggs in May 2019 and 1 egg in August 2020), no obvious egg concentration was predicted in these areas.
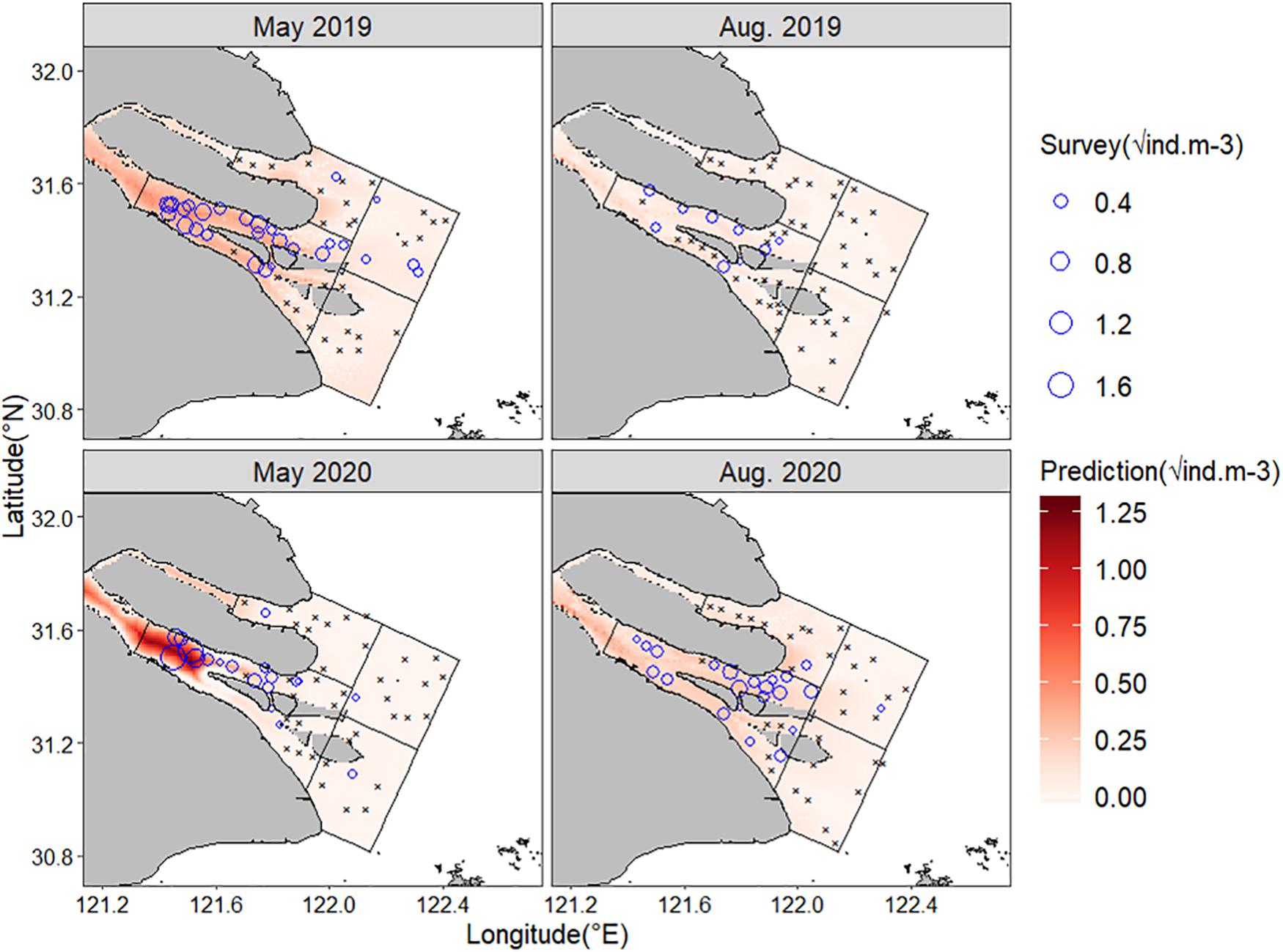
Figure 3. The distribution of eggs sampled and predicted in each survey. The blue circle is egg density sampled in each station and the black × denotes stations with zero egg sampled. The color gradation represents the prediction result.
Environmental Variables and Non-stationary Environmental Effects
In this study, the coefficients estimated of each independent variable determined in the GWR models were mapped to explore the non-stationary environmental effects. The value of coefficients is not limited between −1 and 1 (Li et al., 2018). All the independent variables showed non-stationary effects on the dependent variable.
The overall SST in the Yangtze Estuary showed a decreasing trend from the South to the North Branch and from the branch to the offshore area (Figure 4A). The SST in May 2019 and 2020 ranged from 20 to 24°C. SST in August was included in best performing models in both 2019 and 2020 models. In August 2019, the SST exceeded 25°C in all the sites and ranged from 28 to 30°C in the South Branch and the surrounding waters. The positive relationship between SST and egg density widely occupied the study area (coefficients up to 0.037), except for a slight negative relationship near the Hengsha Island (Figure 5). August 2020 had a general higher SST across the South Branch and the highest SST (34.17°C) showed up in the North Channel. Meanwhile, the relationship between SST and egg density was negative in the North Channel and waters around the Changxing Island and positive in the rest of the study area. The whole estimated coefficients ranged from −0.029 to 0.04 in August 2020.
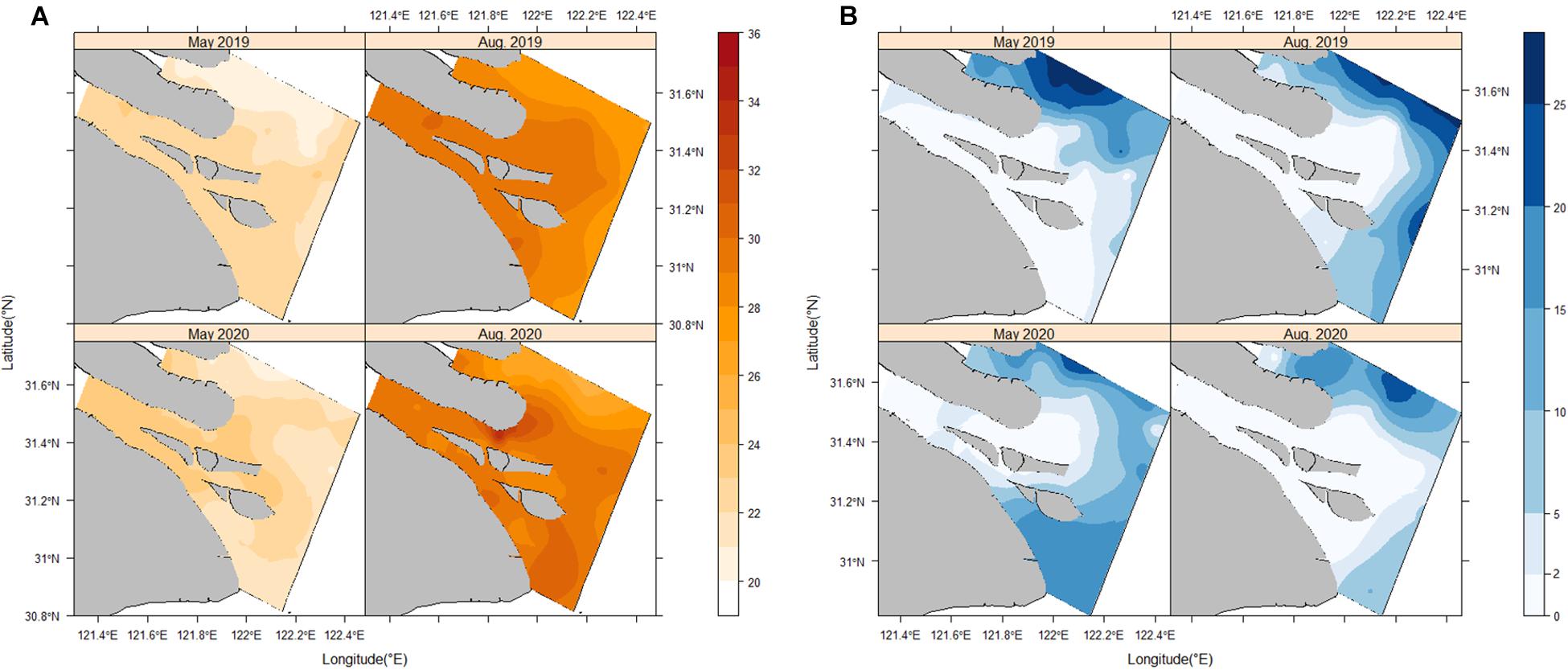
Figure 4. (A) Distribution patterns of the sea surface temperature for each cruise. (B) Distribution patterns of the sea surface salinity for each cruise in the study area.
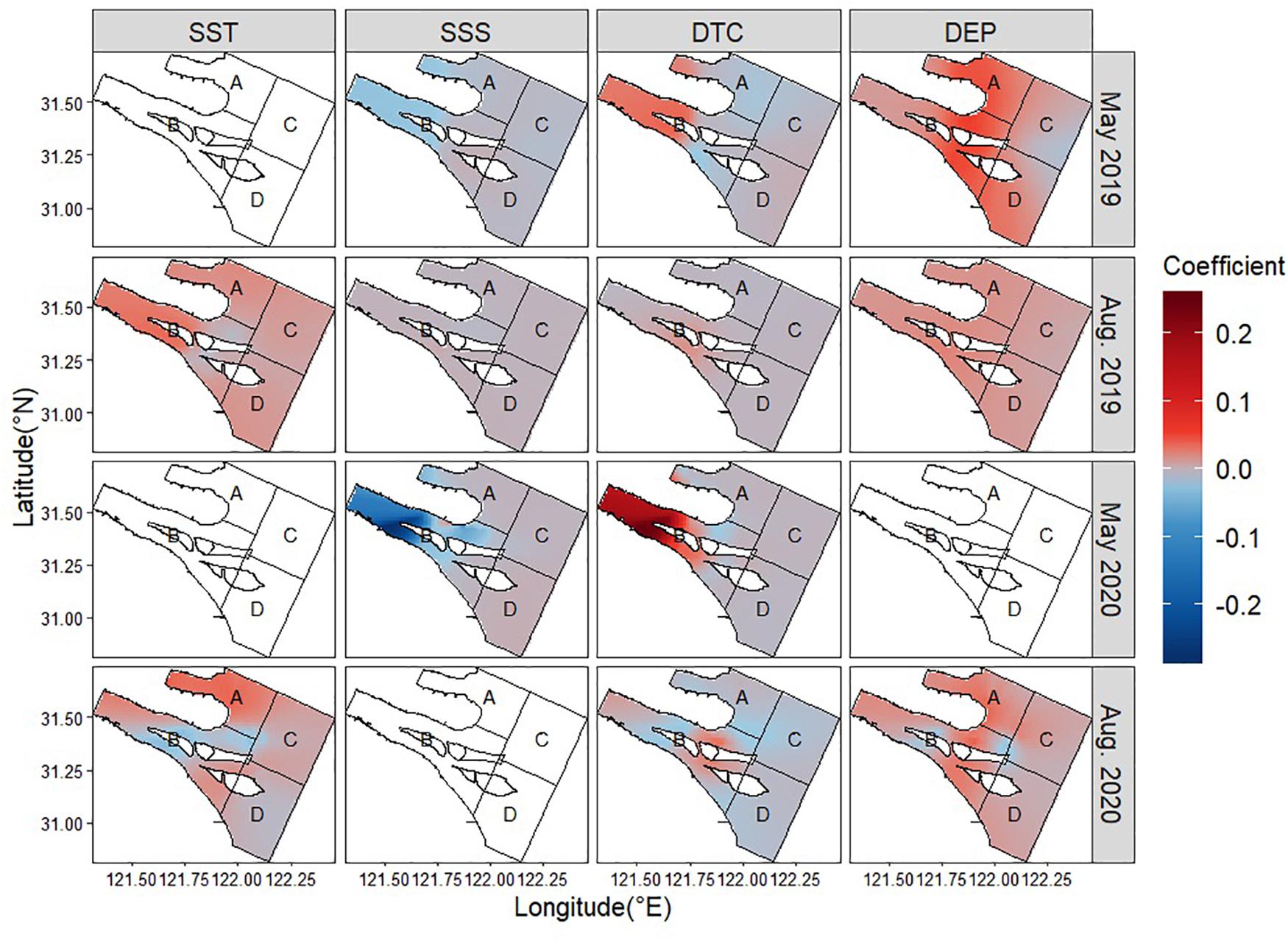
Figure 5. Distribution of coefficients estimated for each cruise. The red color represents a positive relationship with the square root of egg density and the blue color represents a negative relationship with the square root of egg density. The blank figure represents independent variables that were not involved in the model.
The SSS ranged from 0.01 to 28.5 in the study area showing an opposite distribution pattern to SST (Figure 4B). The salinity of water between the South Branch and the North Branch was of significant heterogeneity as the brackish water (salinity < 2) occupied most of the South Branch and the high salinity water (salinity > 10) distributed mainly in the North Branch and the offshore area. In May 2019 and 2020, the estimated coefficients of SSS were generally negative upstream of the Hengsha Island and upstream of the North Branch and close to zero in the rest of the study area (Figure 5). The strength of the negative effect of SSS was stable and the coefficients were approximately −0.022 in May 2019. However, it had an unstable negative effect on egg density in May 2020, as the strength of the negative effect in the upstream of the Changxing Island (coefficients up to −0.275) was stronger than that around the Hengsha Island (coefficients up to −0.1). The salinity in August 2019 had a weaker effect and the estimated coefficients were close to 0.
The relationship between DTC and egg density was obvious in subareas A and B (Figure 5). In May 2019 and 2020, the distribution pattern of a positive relationship between DTC and egg density was similar to that of a negative effect of SSS. The magnitude of positive coefficients in May 2020 (up to 0.249) was much higher than that (up to 0.04) in May 2019. The negative coefficients were in the rest of subareas A and B and the negative effect of SSS in May 2019 took up a wider area than that in May 2020 (Figure 5). In August 2020, the relationship between DTC and egg density showed high spatial variability in subarea B and the coefficients were from −0.019 to 0.039.
The DEP showed strong heterogeneity across the whole study area, especially in subarea B. The upstream of the Hengsha Island was occupied by both the water depths up to 25 m deep and shallow waters less than 5 m. Most of the waters in the rest area were less than 5 m deep, except for the right side of subarea C, which was appropriately 20 m deep. The mapping of estimated coefficients of DEP showed a wide range of positive relationships with egg density in the three models. In May 2019, it had positive coefficients in subareas A, B, and D, except for a weak negative effect in the bottom of subarea C. The positive strength in the upstream of the Hengsha Island (coefficients up to 0.015) was weaker than that in the rest of subareas A and B and the South Passage (coefficients up to 0.025). The DEP in August 2019 was completely positively related to egg density and displayed a weaker effect with coefficients around 0.005. The coefficients ranged from −0.20 to 0.040 in August 2020 and negative effects were located in the middle of the Changxing Island and the right end of the Hengsha Island.
Discussion
The spatial regression GWR model was used to explore the spawning habitat of C. mystus and its response to the rapidly changing abiotic variables in the Yangtze Estuary. The estimated coefficients of each variable changed locally across the study area, indicating non-stationary effects caused by environment heterogeneity in the Yangtze Estuary. Seasonal variations can be found in environmental factors that affect the distribution of the spawning habitat of C. mystus. In the spring, SSS, DTC, and DEP were the main impact factors and saltwater intrusion in the South Branch might lead to the trend that the spawning habitat moved further upstream to the Changxing Island. SST was most important in the summer and the relatively lower SST was more crucial in the spawning habitat selection than DTC and DEP. The predicted spawning habitat of C. mystus was mainly distributed in the middle of the South Branch, near the Chongming, Changxing, and Hengsha Islands, which basically corresponded with the known area of spawning habitat defined in previous studies (Ni et al., 1999; Zhuang et al., 2006). However, there was possibility that the spawning habitat of C. mystus moved further upstream (Figure 3: May 2020) and adults of C. mystus might have the habit of spawning in the freshwater (Yang et al., 2006a,b).
The non-stationary effects of abiotic variables were reflected by the spatially varying relationship between the independent variables and dependent variables (Li et al., 2018). Both SST and SSS represented the primary habitat environment in defining C. mystus spawning habitat (Jiang et al., 2006; Jiang and Shen, 2006; Shi et al., 2014). In the reproductive season, adults of C. mystus would spawn to the brackish water with salinity from 0.14 to 7.51 and temperature of 18–28°C in the Yangtze Estuary (Ni et al., 1999; Zhuang et al., 2006; Ding, 2011).
The temperature across the study area in May 2019 and May 2020 (19–24°C) was within the range of suitable temperature for spawning, resulting in SSS the decisive environmental factor in defining the spawning habitat in the spring (Jiang and Shen, 2006). Although SSS was also involved in August 2019, the non-stationary effect of SSS was only significant in spring. Several studies based on the stepwise model and GAM have shown a universal negative correlation between SSS and the number of C. mystus eggs (Jiang et al., 2006; Ding, 2011; Hu et al., 2021). However, results from the GWR models indicated that the strength of the negative relationship between SSS and egg density varied spatially upstream of the Hengsha Island in May 2020 (Figure 5). There was saltwater intrusion of the South Branch in this month, as high salinity water was found along the Jiuduan sandbank (Figure 4B; Chen and Zhu, 2014). To avoid that eggs being transported to the unfavorable salinity waters (Fortier and Gagné, 1990), more adult fish might choose to spawn further upstream of the Changxing Island (Figure 3) and resulted in the strength difference. There was also a strong difference in the negative effect of SSS between May 2020 and May 2019. Overfishing and environmental degradation were the main reasons for the resource decline of C. mystus (Ni et al., 1999; Liu et al., 2013). The policy that stopping issuing special fishing licenses of C. mystus in 2019 might contribute to more egg production in 2020 and lead to the strength difference.
Sea surface temperature was the dominant environmental factor in summer, as the strength of the effect of SST was much stronger than that of SSS in August 2019. The conclusion was contradicted with that of Jiang and Shen (2006), who thought that SSS influenced greater than SST in summer. It might because the average SST in the summer of 2002 was lower than 28°C (Jiang and Shen, 2006). Previous studies have proved that the increase of temperature would accelerate fish gonadal maturation and, thus, promoting spawning activity (Jiang et al., 2006; Jiao et al., 2019). The widely distributed positive coefficients estimated of SST in August 2019 agreed with the conclusion. However, under higher SST in the North Channel, the area around the Changxing Island, and the South Passage in August 2020 (Figure 4A), the relationship between SST and egg density was negative (Figure 5), which indicated that the “extreme” temperature might restrain the spawning activity or harm the survival of eggs. Ni et al. (2020) reported that under the gradual warming of the Yangtze Estuary, the yield of adults of C. mystus have declined dramatically since a surge by 2°C occurred in 1997 and did not recover in the recent years. The regional temperature rise in the South Branch might be one of the reasons that influence the recruitment success of C. mystus. More attention should be paid to such areas in the future surveys.
There has been no obvious alteration in the distribution of C. mystus spawning habitat over the years. Homing of mature adults to their natal area is observed in a huge variety of migratory fishes such as diadromous fish and Gadiformes (Stabell, 1984; Robichaud and Rose, 2001; Knutsen et al., 2007). However, cues for the homing behavior were still uncertain and complex to explain. In this study, DTC and DEP were applied to explore the geographic effect on the distribution of spawning. It was shown that the relationship between DTC and egg density is positive in the upstream of the Hengsha Island and negative in the rest of subareas A and B (Figure 5). The spatially varying relationship suggests that adult C. mystus might prefer to spawn in the middle of the river upstream of the Hengsha Island, while close to the coast near waters around the Hengsha Island (Yang et al., 1990). The less egg production around the Hengsha Island caused by weaker runoff in May 2020 might also account for the smaller area of the negative effect of DTC in the North Channel (Figure 5). In August 2020, the non-stationary effect of DTC around the Changxing and Hengsha Islands was different from that in the other months. The choice of relatively lower temperature areas in August 2020 rather than the suitable DTC might be the main reason for the “abnormal” phenomenon. Since it is possible to ensure the survival of eggs under “extreme” temperature, the environmental condition of SST would determine the spawning habitat of C. mystus. The reproductive migration process of C. mystus was from offshore to inshore waters and finally stopped upstream of the Hengsha Island (Zhuang et al., 2006); based on the relationship analyzed above, the gradually reducing DTC might be one cue for the homing process of adults of C. mystus.
It was shown that the estimated coefficients of DEP were positively correlated to egg density in most of the study areas (Figure 5) in all the 3 months, which validated the result that C. mystus would spawn in deep waters (Zhou et al., 2011). The non-stationary effect caused by DEP was obvious in May 2019 and August 2020. As the deepest DEP yielded approximately the same level of egg density as other areas where water depth was relatively shallow (Figures 1A, 3), the strength of the positive effect of DEP was weaker in the upstream of the Hengsha Island in May 2019 (Figure 5). The negative relationship between DEP and egg density was due to the eggs found in the shallow waters at the bottom of subarea C in May 2019. However, these eggs might be a result of the transport of local current (Ye et al., 2004; Wan and Wang, 2018) rather than a choice of spawning, as the environmental conditions are unsuitable for spawning. In August 2020, there were small areas of negative relationship in waters near the Changxing and Hengsha Islands. Such a phenomenon might also be the result of lower temperature preference in summer, as eggs were found in the shallow water of relatively lower SST rather than the deep water with “extreme” waters.
Conclusion
In conclusion, the specific location of the spawning habitat of C. mystus was predicted and defined by the GWR model in the Yangtze Estuary. The GWR model showed capability in explaining the response of species to the environmental variation in an estuarine habitat and the non-stationary effect of local variables had been analyzed for better understanding the spawning habitat selection mechanism of C. mystus. However, it was difficult to predict the actual spawning habitat specifically based on our limited data. In the future, more surveys covering the upstream of the South and North Branch throughout the spawning season should be carried out. Meanwhile, there was inference about the potential spawning habitat of C. mystus in the North Branch (Zhao et al., 2020). The potential spawning habitat can be defined as “a habitat where the environmental conditions are suitable for spawning” (Planque et al., 2010). We have collected a few eggs in the North Branch and subarea C; however, such phenomenon did not appear repeatedly and the environmental condition in the North Branch seemed unsuitable for spawning. The member/vagrant hypothesis proposed that the successful stock recruitment depended heavily on the local current system for preventing the eggs from being dispersed to unfavorable conditions (Fortier and Gagné, 1990). The eggs found might be under the transport of local currents to the nursing ground (Hu et al., 2021), as residual currents in the North Channel to the offshore area have the direction first to East then to the North (Ye et al., 2004; Wan and Wang, 2018). Thus, a study on the transport mechanism of the early life stage of C. mystus is necessary to verify the inference and hypothesis.
Data Availability Statement
The raw data supporting the conclusions of this article will be made available by the authors, without undue reservation.
Author Contributions
DW and ZL conceived and designed the study. RW, JZ, LZ, and SZ were involved in revising the manuscript for important intellectual content. XL and PS collected the data. DW compiled and analyzed the data and drafted the manuscript. All authors approved the final version of the manuscript and agreed to be accountable for all the aspects of the work.
Funding
This work was funded by projects: the Tracking Evaluation on Recovery Effect of Fishing Ban in Key Water Areas of the Yangtze River Basin (Grant No. D-8021-20-0073), the Estimation of Spawning Population Biomass of Coilia mystus in the Yangtze Estuary Based on Daily Egg Production Method (Grant No. A2-2006-21-200309), the Scientific Research Foundation for the Imported Talent by RW (Grant No. A1-2006-21-7009), and the Population Dynamics Mechanism and Maintenance Management Strategy of Important Fish Species in the Yangtze Estuary and Adjacent Waters (Grant No. 21010502200).
Conflict of Interest
The authors declare that the research was conducted in the absence of any commercial or financial relationships that could be construed as a potential conflict of interest.
Publisher’s Note
All claims expressed in this article are solely those of the authors and do not necessarily represent those of their affiliated organizations, or those of the publisher, the editors and the reviewers. Any product that may be evaluated in this article, or claim that may be made by its manufacturer, is not guaranteed or endorsed by the publisher.
Acknowledgments
We are indebted to the vessels and colleagues who contributed to collecting the data used for this study. Additionally, we thank Bai Li for her valuable and constructive comments on the manuscript.
References
Andrew, M. W., Charles, E. T., William, B. D., and James, A. S. (2009). Observations on the distribution of ichthyoplankton within the saco river estuary system. Northeastern Naturalist 16, 647–654. doi: 10.1656/045.016.n414
Asch, R. G., and Checkley, D. M. (2013). Dynamic height: a key variable for identifying the spawning habitat of small pelagic fishes. Deep Sea Res. 1 Oceanogr. Res. Pap. 71, 79–91. doi: 10.1016/j.dsr.2012.08.006
Bacheler, N. M., Ciannelli, L., Bailey, K. M., and Duffy-Anderson, J. (2010). Spatial and temporal patterns of walleye pollock (Theragra chalcogramma) spawning in the eastern Bering Sea inferred from egg and larval distributions. Fish. Oceanogr. 19, 107–120. doi: 10.1111/j.1365-2419.2009.00531.x
Brooks, W., Zhu, J., and Lu, Z. (2014). Local Adaptive Grouped Regularization and its Oracle Properties for Varying Coefficient Regression. arXiv [Preprint]. Available online at: https://arxiv.org/pdf/1411.5725.pdf (accessed October 27, 2021).
Brunsdon, C., Fotheringham, A. S., and Charlton, M. (2002). Geographically weighted summary statistics-a framework for localised exploratory data analysis. Comput. Environ. Urban Syst. 26, 501–524.
Brunsdon, C., Fotheringham, A. S., and Charlton, M. E. (1996). Geographically weighted regression: a method for exploring spatial nonstationarity. Geogr. Anal. 28, 281–298. doi: 10.1111/j.1538-4632.1996.tb00936.x
Chen, J., and Zhu, J. R. (2014). Sources for saltwater intrusion at water intake of qingcaosha reservoir in the yangtze estuary. Acta Oceanol. Sin. 36, 131–141.
Christoph, B., and Jose, M. B. (2012). On the use of cross-validation for time series predictor evaluation. Inf. Sci. 191, 192–213. doi: 10.1016/j.ins.2011.12.028
Ding, Y. W. (2011). The Temporal And Spatial Variation Of The Fish Recruitment Resource In Yangtze River Estuary. [Master’s thesis]. Qingdao: University of Chinese Academy of Sciences.
Dumont, P., D’Amours, J., Thibodeau, S., Dubuc, N., Verdon, R., Garceau, S., et al. (2011). Effects of the development of a newly created spawning ground in the Des Prairies River (Quebec, Canada) on the reproductive success of lake sturgeon (Acipenser fulvescens). J. Appl. Ichthyol. 27, 394–404. doi: 10.1111/j.1439-0426.2011.01718.x
Elliott, M., Whitfield, A. K., Potter, I. C., Blaber, S. J. M., Cyrus, D. P., Nordlie, F. G., et al. (2007). The guild approach to categorizing estuarine fish assemblages: a global review. Fish Fish. 8, 241–268. doi: 10.1111/j.1467-2679.2007.00253.x
Fortier, L., and Gagné, J. A. (1990). Larval Herring (Clupea harengus) Dispersion. Growth, and Survival in the St, Lawrence Estuary: match/Mismatch or Membership/Vagrancy? Can. J. Fish. Aquat. Sci. 47, 1898–1912. doi: 10.1139/f90-214
Gallego, A., North, E. W., and Petitgas, P. (2007). Introduction: status and future of modelling physical-biological interactions during the early life of fishes. Mar. Ecol. Prog. Ser. 347, 122–126.
Gibson, G. A., Stockhausen, W. T., Coyle, K. O., Hinckley, S., Parada, C., Hermann, A. J., et al. (2018). An individual-based model for sablefish: exploring the connectivity between potential spawning and nursery grounds in the Gulf of Alaska. Deep Sea Res. 2 Top. Stud. Oceanogr. 165, 89–112. doi: 10.1016/j.dsr2.2018.05.015
Gollini, I., Lu, B. B., Charlton, M., Brunsdon, C., and Harris, P. (2015). GWmodel: an R package for exploring spatial heterogeneity using geographically weighted models. J. Stat. Softw. 63, 1–52. doi: 10.18637/jss.v063.i17
Gonzalez-Irusta, J. M., and Wright, P. J. (2016). Spawning grounds of Atlantic cod (Gadus morhua) in the North Sea. ICES J. Mar. Sci. 73, 1–12. doi: 10.1093/icesjms/fsv180
Halvor, K., Esben, M. O., Lorenzo, C., Sigurd, H. E., Jan, A. K., Jan, H. S., et al. (2007). Egg distribution, bottom topography and small-scale cod population structure in a coastal marine system. Mar. Ecol. Prog. Ser. 2007, 249–255.
Hu, L. J., Song, C., Geng, Z., Zhao, F., Jiang, J., Liu, R. H., et al. (2021). Temporal and spatial distribution of Coilia mystus larvae and juveniles in the Yangtze Estuary during the main breeding season. J. Fish. Sci. China 28, 1152–1161.
Jiang, M., and Shen, X. Q. (2006). Abundance distributions of pelagic fish eggs and larva in the Changjiang River estuary and vicinity waters in summer. Mar. Sci. 30, 92–97.
Jiang, M., Shen, X. Q., and Chen, L. F. (2006). Relationship between with abundance distribution of fish eggs, larvae and environmental factors in the Changjiang Estuary and vicinity waters in spring. Mar. Environ. Sci. 25, 37–44.
Jiao, W. J., Zhang, P., Chang, J. B., Tao, J. P., Liao, X. L., and Zhu, B. (2019). Variation in the suitability of Chinese sturgeon spawning habitat after construction of dams on the Yangtze River. J. Appl. Ichthyol. 35, 637–643. doi: 10.1111/jai.13914
Knutsen, H., Olsen, E. M., Ciannelli, L., Espeland, S. H., Knutsen, J. A., Simonsen, J. H., et al. (2007). Egg distribution, bottom topography and small-scale cod population structure in a coastal marine system. Mar. Ecol. Prog. Ser. 333, 249–255. doi: 10.3354/meps333249
Lauri, L. S., Nathan, J. M., Timothy, E., Barbara, H., and Steven, H. (2014). Distribution patterns of Pacific halibut (Hippoglossus stenolepis) in relation to environmental variables along the continental shelf waters of the US West Coast and southern British Columbia. Fish. Oceanogr. 23, 225–241. doi: 10.1111/fog.12057
Lelievre, S., Vaz, S., Martin, C. S., and Loots, C. (2014). Delineating recurrent fish spawning habitats in the North Sea. J. Sea Res. 91, 1–14. doi: 10.1016/j.seares.2014.03.008
Li, B., Cao, J., Guan, L. S., Mazur, M., Chen, Y., and Wahle, R. A. (2018). Estimating spatial non-stationary environmental effects on the distribution of species: a case study from American lobster in the Gulf of Maine. ICES J. Mar. Sci. 1473–1482. doi: 10.1093/icesjms/fsy024
Likongwe, P., Kihoro, J., Ngigi, M. M., and Jamu, D. (2015). Modeling spatial non-stationarity of Chambo in South-East Arm of Lake Malawi. Asian J. Appl. Sci. Eng. 4, 81–90.
Liu, K., Xu, D. P., Duan, J. R., Zhang, M. Y., Fang, D. A., Zhou, Y. F., et al. (2013). Fluctuation of biological characteristics and yield of Coilia mystus in fishing season after impoundment of the Three Gorges Dam in Yangtze River Estuary. Resour. Environ. Yangtze Basin 22, 1282–1288.
Locke, A., and Courtenay, S. C. (1995). Effects of environmental factors on ichthyoplankton communities in the Miramichi estuary, Gulf of St Lawrence. J. Plankton Res. 17, 333–349. doi: 10.1093/plankt/17.2.333
Luo, B., and Shen, H. (eds) (1994). The Three Gorges Project and Ecology in Estuaries. Beijing: China Science Publishing & Media LTD.
Molinaro, A. M., Simon, R., and Pfeiffer, R. (2005). Prediction error estimation: a comparison of resampling methods. Bioinformatics 21, 3301–3307. doi: 10.1093/bioinformatics/bti499
Munk, P., Fox, C. J., Bolle, L. J., Damme, C. J. G., Fossum, P., and Kraus, G. (2009). Spawning of North Sea fishes linked to hydrographic features. Fish. Oceanogr. 18, 458–469. doi: 10.1111/j.1365-2419.2009.00525.x
Ni, J. F., Guo, H. Y., Tang, W. Q., Zhang, Y., and Zhang, X. G. (2020). On interannual variations of Coilia mystus catches in the Yangtze River Estuary. Mar. Fish. 42, 192–204.
Ni, Y. (1999). Fishery resources conservation for Coilia mystus in the Changjiang estuary. J. Fish. Sci. China 6, 75–77.
Ni, Y., Wang, Y. L., Jiang, M., and Chen, Y. Q. (1999). Biological characteristics of Coilia mystus in the Changjiang estuary. J. Fish. Sci. China 6, 69–71.
O’Brien, R. M. (2007). A Caution Regarding Rules of Thumb for Variance Inflation Factors. Qual. Quant. 41, 673–690.
Opdal, A. F. (2010). Fisheries change spawning ground distribution of northeast Arctic cod. Biol. Lett. 6, 261–264. doi: 10.1098/rsbl.2009.0789
Planque, B., Bellier, E., and Lazure, P. (2010). Modelling potential spawning habitat of sardine (Sardina pilchardus) and anchovy (Engraulis encrasicolus) in the Bay of Biscay. Fish. Oceanogr. 16, 16–30. doi: 10.1111/j.1365-2419.2006.00411.x
Portner, H. O., and Farrell, A. P. (2008). Ecology: physiology and climate change. Science 322, 690–692. doi: 10.1126/science.1163156
Reglero, P., Santos, M., Balbin, R., Laiz-Carrion, R., Alvarez-Berastegui, D., Ciannelli, L., et al. (2017). Environmental and biological characteristics of Atlantic bluefin tuna and albacore spawning habitats based on their egg distributions. Deep Sea Res. 2 Top Stud. Oceanogr. 140, 105–116. doi: 10.1016/j.dsr2.2017.03.013
Robichaud, D., and Rose, G. A. (2001). Multiyear homing of Atlantic cod to a spawning ground. Can. J. Fish. Aquat. Sci. 58, 2325–2329. doi: 10.1139/cjfas-58-12-2325
Shan, X. J., Jin, X. S., and Yuan, W. (2010). Fish assemblage structure in the hypoxic zone in the Changjiang (Yangtze River) estuary and its adjacent waters. Chin. J. Oceanol. Limnol. 28, 459–469. doi: 10.1007/s00343-010-9102-6
Shi, W. G., and Wang, B. (2002). Status quo of tapertail anchovy resource in the estuaries of the Yangtze River. Acta Hydrobiol. Sin. 26, 648–653.
Shi, Y. R., Chao, M., Quan, W. M., and Shen, X. Q. (2014). Spatial and seasonal variations in fish assemblages of the Yangtze River estuary. J. Appl. Ichthyol. 30, 844–852. doi: 10.1111/jai.12448
Stabell, O. B. (1984). Homing and olfaction in salmonds: a critical review with special reference to the atlantic salmon. Biol. Rev. 59, 333–388. doi: 10.1111/j.1469-185X.1984.tb00709.x
Thompson, B. M., and Riley, J. D. (1981). Egg and larval development studies in the North Sea cod (Gadus morhua L.). Rapports et Proce‘s-Verbaux des Re’unions du Conseil International pour l’Exploration de la Mer 178, 553–559.
Tian, R. C., Chen, C., Stokesbury, K. D. E., Rothschild, B. J., Cowles, G. W., Xu, Q., et al. (2009). Dispersal and settlement of sea scallop larvae spawned in the fishery closed areas on Georges Bank. ICES J. Mar. Sci. 66, 2155–2164. doi: 10.1093/icesjms/fsp175
Wan, Y., and Wang, L. (2018). Study on the seasonal estuarine turbidity maximum variations of the Yangtze Estuary, China. J. Waterw. Port Coast. Ocean Eng. 144:05018002. doi: 10.1061/(ASCE)WW.1943-5460.0000442
Windle, M. J. S., Rose, G. A., Devillers, R., and Fortin, M. J. (2010). Exploring spatial non-stationarity of fisheries survey data using geographically weighted regression (GWR): an example from the Northwest Atlantic. ICES J. Mar. Sci. 67, 145–154. doi: 10.1093/icesjms/fsp224
Yan, Y., Gao, J., Zhu, Y., and Zheng, J. (2001). Preliminary study on relationship between Deepwater Channel regulation and riverbed evolution. J. Hohai Univ. 29, 7–12.
Yang, D., Wu, G., and Sun, J. (1990). The investigation of pelagic eggs, larvae and juveniles of fishes at the mouth of the Changjiang River and adjacent areas. Oceanol. Limnol. Sin. 21, 346–355.
Yang, J., Arai, T., Liu, H., and Miyazaki, N. (2006a). Environmental signature in the otolith elemental fingerprint of the tapertail anchovy, Coilia mystus, from the Changjiang estuary, China. J. Appl. Ichthyol. 22, 459–462. doi: 10.1111/j.1439-0426.2006.00776.x
Yang, J., Arai, T., Liu, H., Miyazaki, N., and Tsukamoto, K. (2006b). Reconstructing habitat use of Coilia mystus and Coilia ectenes of the Yangtze River estuary, and of Coilia ectenes of Taihu Lake, based on otolithstrontium and calcium. J. Fish Biol. 69, 1120–1135. doi: 10.1111/j.1095-8649.2006.01186.x
Ye, S., Lv, J., Ding, D., and Huang, X. (2004). A preliminary study on the impacts of large-scale estuarine engineering on habitat fragmentation in the Yangtze River estuary. Ocean Eng. 22, 41–47.
Yu, W., and Zhang, Z. (2017). Recognition of tide and tidal current movement in the Yangtze Estuary. J. Water Resour. Res. 6, 475–485.
Zhai, L., and Pauly, D. (2019). Yield-per-recruit, utility-per-recruit, and relative biomass of 21 exploited fish species in China’s coastal seas. Front. Mar. Sci. 6:724. doi: 10.3389/fmars.2019.00724
Zhai, L., and Pauly, D. (2020). Construction and interpretation of particle size distribution spectra from 19 Ecopath Models of Chinese coastal ecosystems. Front. Mar. Sci. 7:298. doi: 10.3389/fmars.2020.00298
Zhang, R., Lu, S., Zhao, C., Chen, L., Zang, Z., and Jiang, Y. (1985). Fish Eggs and Larvae in the Offshore Waters of China. Shanghai: Shanghai Scientific & Technical Publishers.
Zhao, F., Yang, Q., Song, C., Zhang, T., and Zhaung, P. (2020). Biological characteristics and resource utilization of Coilia mystus in the Yangtze Estuary. Mar. Fish. 42, 110–119.
Zhou, Y., Jin, H., Zhang, H., Zhang, Y., Jiang, R., and Xu, K. (2011). Distribution of Ichthyoplankton of Coilia mystus in the coast of north Zhejiang Province in spring and summer. J. Zhejiang Ocean Univ. (Nat. Sci.) 30, 307–312.
Zhuang, P., Wang, Y., Li, S., Deng, S., Li, C., Ni, Y., et al. (2006). Fishes Of The Yangtze Estuary. Shanghai: Shanghai Scientific & Technical Publishers.
Keywords: geographically weighted regression (GWR) model, estuarine areas, Coilia mystus, spawning habitat, the Yangtze Estuary
Citation: Wang D, Wan R, Li Z, Zhang J, Long X, Song P, Zhai L and Zhang S (2021) The Non-stationary Environmental Effects on Spawning Habitat of Fish in Estuaries: A Case Study of Coilia mystus in the Yangtze Estuary. Front. Mar. Sci. 8:766616. doi: 10.3389/fmars.2021.766616
Received: 29 August 2021; Accepted: 11 October 2021;
Published: 08 November 2021.
Edited by:
Yuan Li, Third Institute of Oceanography, ChinaReviewed by:
Cui Liang, Institute of Oceanology, Chinese Academy of Sciences (CAS), ChinaDelian Huang, South China Sea Fisheries Research Institute (CAFS), China
Copyright © 2021 Wang, Wan, Li, Zhang, Long, Song, Zhai and Zhang. This is an open-access article distributed under the terms of the Creative Commons Attribution License (CC BY). The use, distribution or reproduction in other forums is permitted, provided the original author(s) and the copyright owner(s) are credited and that the original publication in this journal is cited, in accordance with accepted academic practice. No use, distribution or reproduction is permitted which does not comply with these terms.
*Correspondence: Zengguang Li, emdsaUBzaG91LmVkdS5jbg==