Total factor productivity of China’s marine economy: A meta-analysis
- Management School, Hainan University, Haikou, China
With the rapid development of the global economy and the depletion of land resources, the ocean has gradually become a new area for human society to seek resources and space utilization. In China, the marine economy also has become an essential part of the national economy. Therefore, it is crucial to evaluate marine productivity to understand China’s marine industry’s input-output status and development level. We conducted a meta-analysis using 622 observations from 33 primary empirical studies to quantify the discrepancies in measurement findings and identify the influencing factors of total factor productivity (TFP) growth in the marine industry. The results indicate that: (1) In the existing literature, the mean TFP growth of China’s marine economy at the provincial level is 1.002, which is lower than that at the national level (1.022); (2) The time span of the data, the estimation model, the evaluation indicator, and the journal’s rank all have a significant effect on the estimation results of the marine economic TFP growth, whereas the year of publication does not affect the estimation results; (3) The TFP growth of China’s marine economy peaked during the Ninth Five-Year Plan period, after which it began to decline year after year; (4) The TFP growth of the marine economy in the Yangtze River Delta region and Circum Bohai Sea region is significantly higher than that in the Pan-Pearl River Delta region; (5) Economic factors such as gross ocean product, level of opening-up, level of marine science and technology, and industrial structure all have an impact on the marine economic TFP growth. Accordingly, the following insights were obtained: In terms of marine economic development policies, we should continue to enhance the investment in marine environmental governance, strengthen the construction of marine ecological civilization, and pay attention to synergistic regional development, opening up to the outside world, scientific and technological innovation, and industrial structure optimization. In addition, the follow-up study should use long-period sample data as much as possible, pay attention to the parametric SFA model, and strip the negative environmental impact by constructing a green evaluation index system.
1 Introduction
As a large country with a long coastline, China has a wealth of maritime resources and a vast ocean territory, and the Chinese government places a premium on the development of the marine economy (Gai et al., 2016; Sun et al., 2018). The 13th Five-Year Development Plan for the National Marine Economy, released in May 2017, highlighted the need to improve the quality and efficiency of marine economic development. In March 2021, the Outline of the 14th Five-Year Plan for National Economic and Social Development and Vision 2035 of the People’s Republic of China proposed to establish a number of high-quality marine economic development demonstration areas and characterized marine industry clusters, illustrating China’s firm resolve and staunch will to the high-quality development of the marine economy. In 2021, the National Gross Ocean Product, with a growth rate being 8.3 percent, totaled 1.41 trillion dollars which accounted for 8.0 percent of the Gross Domestic Product (The State Council of the PRC, 2022), showing that the marine economy has emerged as an essential driving force of the national economy.
However, the rapid growth of China’s marine economy partly depends on the overdraft of marine resources, which belongs to the resource-intensive development model driven by an increase in factor inputs (Li et al., 2021). As China’s economic development has entered a new normal, the marine economy needs to transition to an innovation-led and high-quality development mode. According to the Theory of Endogenous Growth, TFP is a proxy for an economy’s long-term productivity growth or the quality of growth, and is regarded as the improvement of productivity with the exclusion of input contribution (Mahadevan, 2003). Thus, as the core of national wealth growth, especially in developing countries, TFP has emerged as a critical metric for measuring the high-quality development of the marine economy (Krugman, 1994; Johnson, 1997; Feng et al., 2019; Xia and Xu, 2020). In the above context, studying the trend and influencing factors of China’s marine economic TFP growth is significant in transforming the marine economic growth pattern and achieving high-quality development of China’s marine economy.
Solow (1957) first proposed the concept of TFP in his growth theory. Following this, subsequent scholars conducted substantial studies on TFP. In the estimation method of TFP, the data envelopment analysis (DEA) and the stochastic frontier analysis (SFA) are utilized by most studies (Aigner et al., 1977; Charnes et al., 1978). Previous studies mainly utilized the DEA-Malmquist model developed by Färe and Grosskopf (1992) to measure the marine economic TFP related to a specific industry or sector, such as marine aquaculture, marine fisheries, and maritime transportation (Shang et al., 1998; Jin et al., 2002; Turner et al., 2004). Later, Estache et al. (2004) measured the TFP of Mexican ports and found that the TFP estimates of ports increased significantly after the reform and opening up.
Unlike foreign scholars who focus more on the TFP of the marine industry (Mohammed and Williamson, 2004; Hassanpour et al., 2010), Chinese scholars, even if they start studying the TFP of the marine economy late, tend to measure the overall TFP of the marine economy and its regional variations (Ren et al., 2018a; Hua et al., 2021). Among them, Wang (2017) measured the TFP of the marine economy in China from 2001 to 2011, discovering that the TFP of the marine economy in coastal regions showed a declining trend to varying degrees. However, Zhu and Gai (2019) found that the growth trend of TFP in China’s marine economy was stable from 2005 to 2015, except for the Guangxi Autonomous Region, which had a decline. Moreover, Zhang (2019) discovered that the average annual TFP growth rate of China’s marine economy from 1998 to 2013 was 6.5 percent, with the growth rate of eastern coastal areas being relatively high.
In recent years, due to limited marine resources and unbalanced coastal development, there has been an increasing focus on the marine economic green TFP growth that considers environmental constraints (Munisamy and Arabi, 2015; Song and Ning, 2020). However, the traditional measures of TFP growth, such as the DEA-CCR and DEA-BCC models, are radial models that strictly require the input and output to change in the same proportion. Therefore, they do not embody the idea of maximizing the desirable output while minimizing the undesirable output, resulting in a certain insufficiency (Li et al., 2017). Accordingly, Chung et al. (1997) and Tone et al. (2001) developed the Malmquist-Luenberger index combined with directional distance function (DDF) and the slacks-based measure (SBM) model based on the DEA method to incorporate both desirable and undesirable output into the study of TFP. Following that, from the perspective of input, a few scholars employed the entropy method to construct a resource consumption index and used it as an input indicator to measure the green TFP growth (Sun and Song, 2019; Xiang et al., 2019). In contrast, other researchers chose to examine the marine economic green TFP growth under environmental constraints such as the amount of carbon emission and wastewater emissions from an output perspective (Kumar, 2006; Hu, 2018). Among them, Hu (2018) discovered that the estimation results of TFP growth were significantly lower after including marine wastewater emissions in the evaluation system. It is demonstrated that ignoring undesirable outputs in the analysis of TFP growth of the marine economy could lead to false conclusions and mislead policymakers.
In addition, the SFA model has also been applied to estimate the TFP of the marine economy due to the capability of distinguishing the effects of statistical noise from those of insufficient (Managi et al., 2006; Fall et al., 2018). However, there is a significant difference in the TFP growth of China’s marine economy, as obtained by Ji et al. (2017) using the SFA model and Ding et al. (2017b) using the DEA model.
A longitudinal literature review found that existing studies have made significant contributions to the study of TFP growth of the marine economy to provide an essential foundation for an in-depth understanding of the development of China’s marine economy. However, few systematic analysis is carried out on the TFP growth of the marine economy. There is still a divergence in the estimation results regarding China’s marine economic TFP growth reported in the current studies. Thus, what are the key determinants of the observed variations in TFP growth in the marine economy? Are they the result of varying estimation models or evaluation indicators, or are they the result of varying characteristics of published literature? Along with the estimation results in the current literature, what spatial and temporal variation characteristics does China’s marine economic TFP exhibit, and what factors influence it? These questions are yet unaddressed. Therefore, this paper employs a meta-analysis to quantitatively analyze existing research findings on the TFP growth of China’s marine economy to serve as a reference point for future research and relevant policy formulation.
2 Methodology
Meta-analysis is a statistical analysis that combines and integrates the results of several studies into a unified analysis (den Besten and Zwietering, 2012). It helps to find out the limitations of previous studies and explore new research directions. The main goal of a meta-analysis is to make the empirical results of specific studies comparable and suitable by controlling for the effect size (TFP growth in this case) (Angelini et al., 2022). The meta-analysis process mainly involves the selection and processing of effect quantities, the determination of heterogeneity test models, cumulative meta-analysis, and meta-regression analysis. The specific analysis process adopted is as follows:
2.1 Heterogeneity test and combination of effect size
The degree of heterogeneity of the collection of effects is one of the critical parts of a meta-analysis. Effect model selection should depend on the degree of variability among the included studies when combining effect size (Schneider et al., 2017). Two models are commonly used for the combination of effect size: the fixed-effect (FE) model and the random-effect (RE) model. The main difference between FE and RE model assumptions involves the characteristics of the studies: in the first case, studies should represent the entire population of interest, while in the second case, they should represent a random sample from a population of interest and the inference target is to extend the results from the sample to the entire population of interest (Hershey, 2021). For small heterogeneity, a fixed effect model is used, for considerable heterogeneity, a random effect model is more reliable (Egger et al., 1997). In this study, heterogeneity analysis was tested using the Q test and I2 statistics to analyze whether there were statistically significant differences between the results of different studies. If the Q test is statistically significant or I2 >50%, a heterogeneous distribution of effect size is assumed (Trong Ho et al., 2022).
2.2 Publication bias test
Even when conducted thoroughly, meta-analyses can be subject to publication bias—studies being less likely to be published, hence less likely to be included in a meta-analysis because the researchers and reviewers often have a preference for statistically significant results or for results that conform to prior theoretical expectations, or both (Benos and Zotou, 2014). The subsequent meta-analysis of published literature may be misleading if publication bias occurs.
Therefore, prior to the meta-analysis, this paper first used the Funnel Asymmetry Test and Precision Effect Test (FAT-PET) to determine whether there was a publication bias in the current literature. Regarding the model specification, this paper used a semi-log linear model to reduce heteroskedasticity and improve the estimation accuracy (Brander et al., 2006; Chaikumbung, 2021).
Where Yij denotes the i th reported TFP growth estimate of the marine economy from the j th primary study; SEij denotes the standard error of the i th reported TFP growth estimate from the j th study; βse denotes the coefficient of the standard error to be estimated, which, if significant, indicates publication bias; β0 and ϵij denote the intercept term and random error term, respectively.
However, since the standard error of TFP growth, is rarely provided in most primary studies, we employed the inverse of the square root of the number of observations in the primary studies as a measure of the standard error with reference to the practices of relevant studies (Stanley and Rosenberger, 2009). At this point, the FAT-PET estimation model can be rewritten as follows:
where Nj denotes the number of observations for the j th study, and the other parameters have the same meaning as in equation (1).
2.3 Meta-regression analysis
Meta-regression analysis is defined as a quantitative method used to evaluate the effect of methodological and other study-specific characteristics on published empirical estimates of some indicators (Stanley et al., 2008). This technique is no longer limited to qualitative descriptions and literature summaries compared to traditional literature surveys. Instead, it combines empirical results of existing studies based on specific criteria and uses statistical and econometric methods to conduct systematic quantitative analyses to explore the findings that are not evident in case studies but are valuable for solving new problems (Thompson and Higgins, 2002; Djokoto et al., 2020). To a certain extent, meta-regression analysis allows for the reanalysis of many studies on the same topic with specific conditions, overcoming the limitations of case studies, and allowing for the avoidance of selectivity bias and model setting bias in the original literature (Aiello and Bonanno, 2016).
The meta-regression model used in this paper is a multiple linear regression model based on the weighted least squares (WLS) method. This model can avoid the correlation between the results from primary empirical studies, which are TFP growth estimates of the marine economy in this paper (Yan et al., 2019). For the setting of model weights, this paper referred to the practice of some scholars, using the reciprocal number of observations as weights to reduce the influence of sample correlation (Salem and Mercer, 2012; Tan et al., 2020). Also, this paper transforms the observed estimates of TFP growth into a natural logarithmic form1. The estimation model is expressed as:
In equation (3), Yij denotes the i th reported TFP growth estimate of the marine economy reported from the j th study; Xk denote the explanatory variables that summarize various characteristics of the primary studies; βk denote the meta-regression coefficients which reflect the effect of particular study characteristics; α is the intercept term; and ϵij is the random error term.
3 Data collection and variable selection
3.1 Data collection
The representativeness and completeness of the primary studies are the basis of meta-regression analysis (Card et al., 2010). In this study, the 11 coastal provinces, municipalities, or autonomous regions of the Chinese Mainland (excluding Hong Kong, Macao, and Taiwan) were used as the study area (Figure 1).
In order to obtain as much primary literature as possible for analysis, a comprehensive search was conducted on literature databases such as China National Knowledge Infrastructure (CNKI), Wanfang Data, Web of Science, ScienceDirect, and Google Scholar. Search keywords include “Marine Economy”, “Total factor productivity”, “China” and “TFP”. At the same time, the literature related to the topic “Total factor productivity of China’s marine economy” was also reviewed. Furthermore, secondary screening was performed for the relevant literature that was initially retrieved using the following inclusion criteria: (1) The selected literature evaluated one or two types of TFP growth in the marine economy, rather than focusing solely on a specific industry2; (2) The selected literature measured the TFP growth of the marine economy in coastal provinces and municipalities (or autonomous regions) or nationwide; (3) The selected literature reported quantitative research results, and the TFP growth of the marine economy estimates can be obtained directly or after simple processing; (4) The selected literature reported on the evaluation indicators, estimation models, and other information used in the estimation of TFP growth in the marine economy. Following the second screening based on the above-mentioned criteria, 33 primary studies were obtained, with 26 Chinese and 7 English literature. Table 1 presents some key characteristics of all the studies reviewed. A portion of the primary studies estimated two types of TFP growth, and all of these estimates were retained in this paper to round out the dataset. Since scholars have measured the TFP growth of China’s marine economy at provincial and national levels, this paper builds two literature databases at provincial and national levels, respectively, in the subsequent analysis. Among them, 29 studies estimated TFP growth in the marine economy at the provincial level, with 408 observations; 17 studies estimated TFP growth in the marine economy at the national level, with 214 observations.
3.2 Variable selection
The TFP growth estimates of the marine economy are the effect size as well as the dependent variable of the meta-regression model in this paper, and the independent variables are the characteristic variables extracted from the corresponding primary studies. These characteristics are classified into five categories in this paper: the spatial and temporal characteristics, the estimation model characteristics, the evaluation indicator characteristics, the economic factors characteristics, and the publication characteristics.
3.2.1 Spatial and temporal characteristics
According to previous research, TFP growth tends to exhibit dynamic changes in different periods and regions (Liu et al., 2021). Therefore, this paper will explore the impact of spatial and temporal characteristics on TFP growth in the marine economy from both temporal and spatial dimensions.
At the provincial level, primary studies often examined the development status of provinces based on the average estimates of TFP growth of the marine economy over a period of time. However, the length of the time period and the year of the data used by different scholars frequently differed significantly, making it difficult to unify their time intervals of data (Li et al., 2015; Zhao et al., 2018). The length of the time period affects the amount of information contained in the data, and the year of the data reflects the TFP growth of the marine economy in a specific period. Therefore, this paper, following the practice of existing literature (Efendic et al., 2011; Ogundari, 2014), has incorporated the time span and the year of data into the model as independent variables to investigate the influence of data used in primary studies on the measurement results of TFP growth in the marine economy. In addition, this paper has divided China’s coastal areas into the Circum Bohai Sea region, the Yangtze River Delta region, and the Pan-Pearl River Delta region referring to existing research and incorporating them into the model in the form of dummy variables to explore the differences in TFP growth of the marine economy in different regions (Zhang and Wang, 2021).
At the national level, the primary studies estimated the TFP growth of China’s marine economy from 1998 to 2017. The years of observed TFP growth of China’s marine economy estimated by primary research were incorporated into the regression model to investigate changes in TFP growth over time. Meanwhile, considering the impact of China’s Five-Year Plan development policies, this paper created dummy variables corresponding to the Five-Year Plan period in which the year of estimation falls to accurately measure the change in TFP growth of China’s marine economy over time.
3.2.2 Estimation model characteristics
Since different estimation models will affect the estimation results of the marine economic TFP growth (Tian and Yu, 2012). Therefore, this paper incorporated the estimation model characteristics as independent variables into the regression model. Currently, most primary studies use the traditional DEA model to estimate the TFP growth of China’s marine economy, but some scholars have also used the SBM model, the DDF, and the SFA model. Therefore, This article created corresponding dummy variables to distinguish DEA, SBM, DDF, and SFA in order to investigate the effect of model differences on estimation results.
3.2.3 Evaluation indicator characteristics
It has been demonstrated that the marine ecological environment imposes a significant constraint on the marine economy, and ignoring the existence of undesirable outputs may result in discrepancies in estimation results (Ye et al., 2021). Some scholars in primary studies attempt to incorporate undesirable outputs such as wastewater, carbon, and solid waste emissions into the estimation model when conducting TFP growth estimations of the marine economy. Therefore, this paper divides the evaluation indicators of TFP growth in the marine economy into traditional and green indicators based on whether the output indicators of the primary studies contain undesirable outputs and includes them as explanatory variables in the meta-regression model.
3.2.4 Publication characteristics
The characteristics of an academic journal might also account for the variation in estimated TFP growth. This study incorporated the journal’s rank as an explanatory variable in the meta-regression model to investigate the influence of the journal’s rank to which the primary studies belong on the TFP growth estimate findings of the marine economy. Accordingly, we divided the journal’s rank into core and non-core journals based on the indexing status of the journals in which the primary studies are published. At the same time, the year of publication of the primary studies is also included in the meta-regression model because literature from different periods might reflect the views and ideas of that period.
3.2.5 Economic factor characteristics
It has been demonstrated that indicators like GDP and FDI in national economic development, as well as factors such as the level of land-based economic development and marine industry structure, can all have a significant impact on the marine economic TFP growth (Wang et al., 2021; Wei et al., 2021). As a result, variables like the gross ocean product, total imports and exports as a percentage of GDP, the number of marine research topics, and the share of marine tertiary industry in the gross ocean product are included in the meta-regression model in this paper to examine their effects on TFP growth in the marine economy.
3.3 Variable coding and descriptive statistics
3.3.1 Variable coding and descriptive statistics at the provincial level
As shown in Table 2, the average TFP growth of the marine economy in all provinces is 1.006. The average growth rate of the TFP growth of the marine economy in the coastal provinces is 0.6%. From the perspective of spatial and temporal characteristics, the average data years of the primary studies spanned 10.490 years, and the median data years were concentrated from 2006 to 2010. The estimated provinces belonging to the Circum Bohai Sea region, the Yangtze River Delta region, or the Pan-Pearl River Delta region are roughly equal. Judging from the characteristics of the estimation model, the number of primary studies using the DEA model still accounts for the majority. Still, different from the meta-analysis database at the national level, 8.8% of the literature applied the SFA model to estimate the TFP growth of the provincial marine economy. Regarding the characteristics of evaluation indicators, the green TFP growth observations are slightly higher than the traditional TFP growth observations. Regarding publication characteristics, the core journals reported more TFP growth observations of the marine economy, accounting for 66.7% of the total observations. In terms of economic factor characteristics, the total imports and exports of the provinces account for an average of 63.9% of GDP. The average proportion of the marine tertiary industry was 46.3%. In addition, considering that the volatility and magnitude differences of the raw data may affect the analysis results, this paper followed the previous research and used natural logarithms of the continuous variables when estimating the meta-regression model in order to improve the estimation accuracy of the model (Chaikumbung et al., 2016).
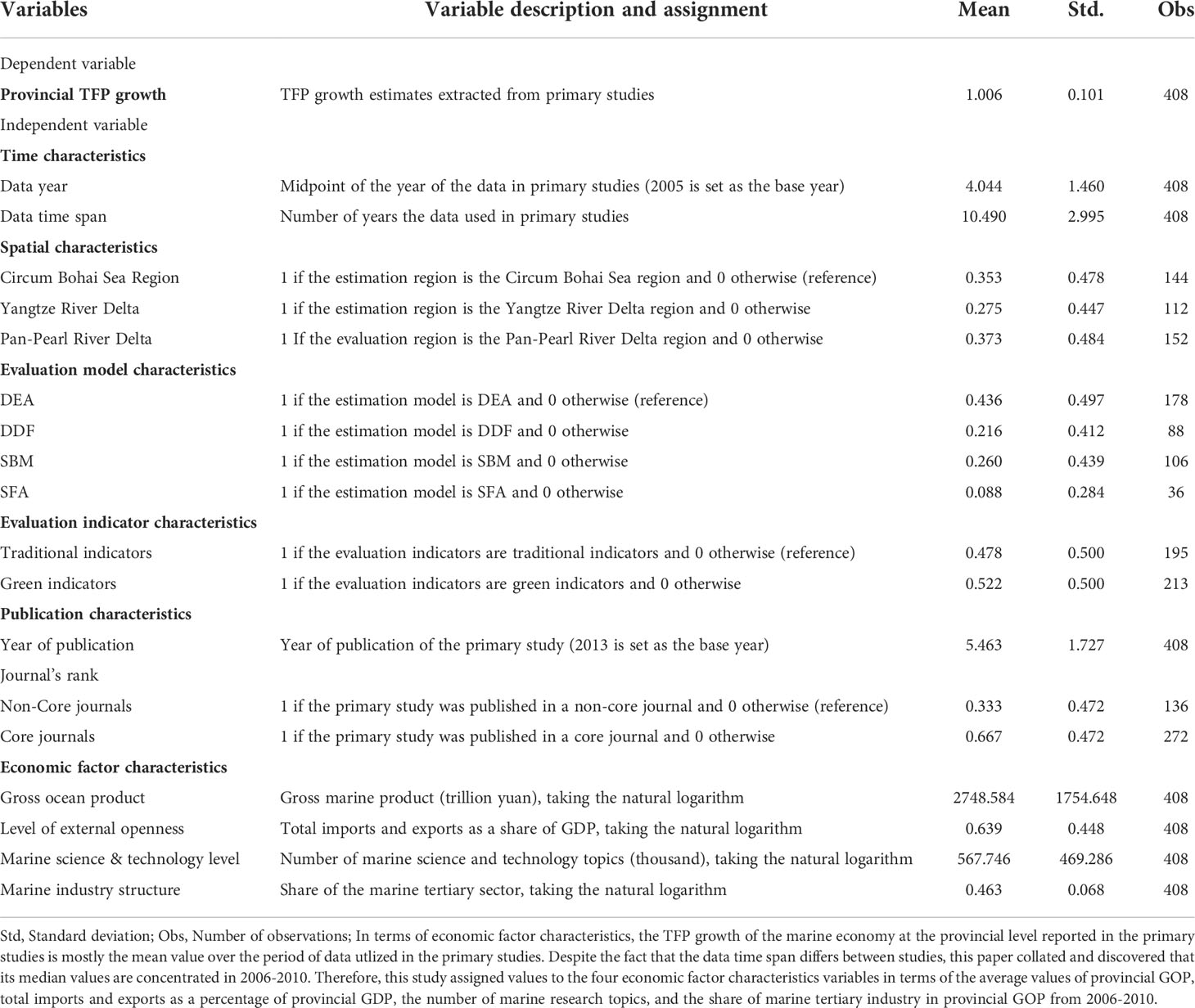
Table 2 Results of descriptive statistics of characteristic variables that incorporate with subsequent meta-regression analysis at the provincial level.
3.3.2 Variable coding and descriptive statistics at the national level
As shown in Table 3, the average value of TFP growth for China’s marine economy is 1.036, which means that the average growth rate of TFP growth of China’s overall marine economy is 3.6%, with overall positive growth3. In terms of time characteristics, the primary studies have estimated the TFP growth of China’s marine economy from the Ninth Five-Year Plan to the Thirteenth Five-Year Plan, with the majority of studies on the Eleventh and Twelfth Five-Year Plans. In terms of estimation model characteristics, the traditional DEA model is used in most primary studies and is also used as the benchmark in this paper. The TFP growth of the green marine economy and the TFP growth of the traditional marine economy account for 61.2 percent and 38.8 percent of the total observations, respectively. Regarding publication characteristics, the observed estimates of TFP growth of the marine economy reported by core journals accounted for 80.4 percent of the total observed estimates. Among economic factor characteristics, China’s average share of total imports and exports in GDP is 51.2 percent, and the average share of the marine tertiary industry is 48.0 percent. Furthermore, the logarithmic processing of the raw data is the same as described in the previous section at the provincial level.
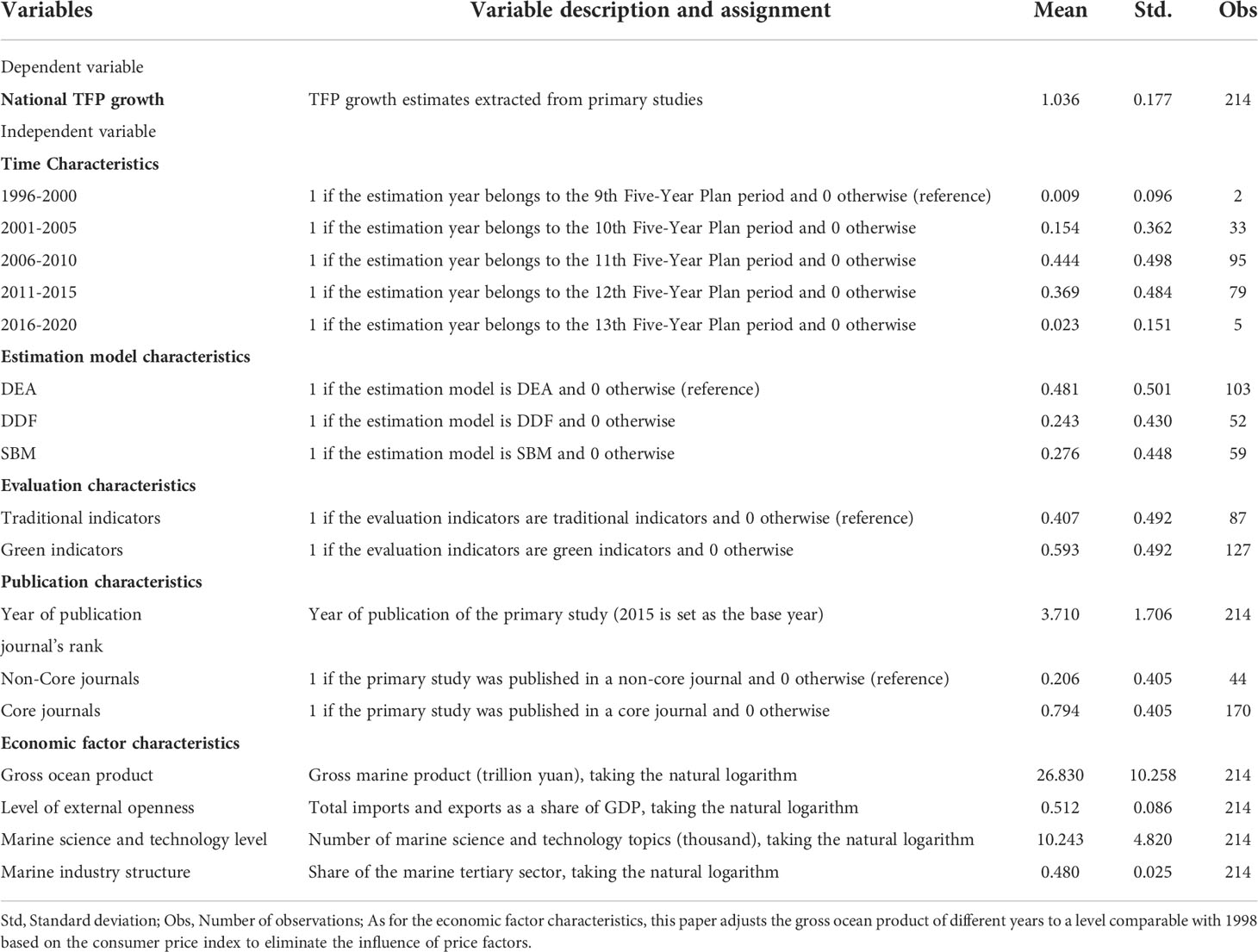
Table 3 Results of descriptive statistics of characteristic variables that incorporate with subsequent meta-regression analysis at the national level.
4 Results
4.1 Combination of overall effects
As shown in Table 4, Heterogeneity analyses of both provincial level TFP growth and national level TFP growth reached very significant levels (PQ<0.001, I2>75%). Based on the results from heterogeneity assessment and in order to generalize the results obtained from the set of collected studies, a more restrictive random-effect model was used to combine effect sizes. In this model, each study was assigned a relative weight, which is the inverse of the sum of the sampling error and the between-study variance. At the same time, the relative weight for each study provides the basis for calculating the weighted mean corresponding to the overall effect size. The overall random-effect weighted effect size was 1.002, with the 95% confidence interval ranging from 0.972 to 1.032 at the provincial level and 1.022 at the national level, with the 95% confidence interval ranging from 0.994 to 1.051 (Figure 2). It demonstrated that TFP growth estimates of the marine economy in the selected studies are centered at 1.002 and 1.022 at the provincial and national levels, respectively.
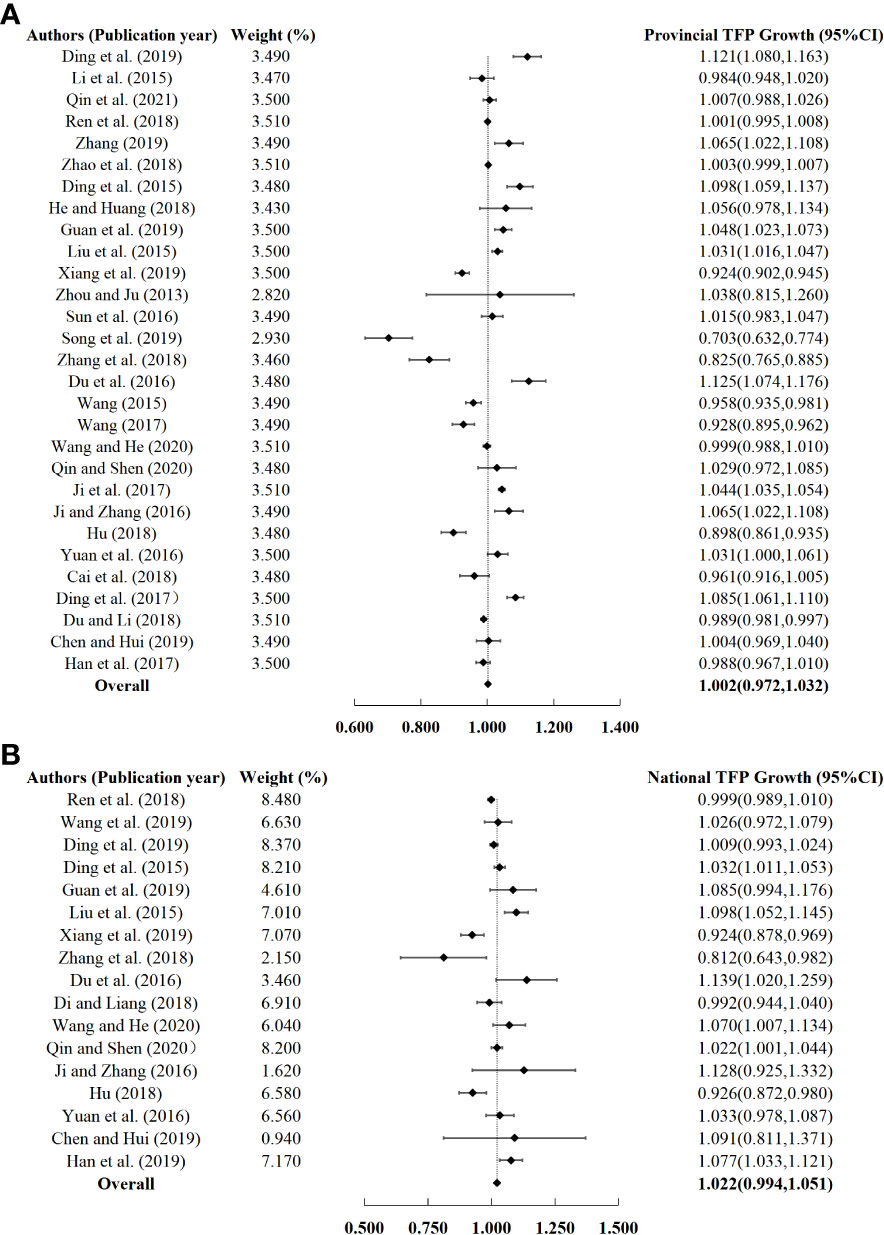
Figure 2 Forest plot of the weighted effect sizes and their 95% confidence intervals (CI). (A) Forest plot at the provincial level; (B) Forest plot at the national level. The solid vertical line represents a mean difference of zero or no effect. Points to the left of the line represent a reduction in final TFP growth, while points to the right of the line indicate an increase. Each diamond around the point effect represents the mean effect size for that study and reflects the relative weighting of the comparison to the overall effect size estimate. The weights that each comparison contributed are in the left-hand column. The upper and lower limit of the line connected to the diamond represents the upper and lower 95% CI for the effect size. The overall polled effect size pooled using the random effects models are indicated by the respective diamonds at the bottom.
However, the Q test and I2 statistic are evidence for heterogeneity but cannot prove that the studies affect the overall heterogeneity. Therefore, we will continue to perform a meta-regression analysis to explore the source(s) of response heterogeneity and investigate the study characteristics’ effect on the TFP growth estimates.
4.2 Results of the publication bias test
The FAT-PET was performed using ordinary least squares (OLS) and weighted least squares (WLS) in this article, and the results are shown in Table 5. When weighted least squares are used, precision may be increased due to the larger sample size used in the literature analysis with more observations. As a result, the square root of the number of TFP growth observations in the marine economy in the primary studies is used as a weight here with reference to existing studies to ensure that observations with a higher degree of research precision receive a higher weight. The test results showed that the standard error coefficients are not significant in either the provincial or national meta-analysis databases for both models, indicating that the primary studies included in this paper are free of publication bias and meet the meta-regression analysis requirements.
4.3 Results of the meta-regression analysis
In this paper, the meta-regression analysis was performed using Stata version 15.1 software, and the regression results at the provincial and national levels are shown in Tables 6, 7, respectively.
4.3.1 Meta-regression results at the provincial level
Spatial and temporal characteristics. Firstly, the data time span has a significant positive effect on the estimates of TFP growth at the provincial level, indicating that the longer the time period of data used in the primary studies, the higher the TFP growth in the marine economy. In addition, a longer time period of data also improves the reliability and explanatory power of the estimation results to a certain extent. Secondly, the data year in the primary studies has a significant negative effect on the marine economic TFP growth (Table 6), suggesting that the estimates decrease as the year of data extends backward. This is likely because China’s marine economy is transitioning from resource intensive to environmentally friendly development, and the emphasis on development quality has resulted in a decline in TFP growth. Thirdly, the TFP growth of the marine economy in the Yangtze River Delta and Circum Bohai Sea regions is significantly higher than in the Pan-Pearl River Delta region, which may be explained by the fact that the Pan-Pearl River Delta region includes areas with a relatively weak marine economy, such as the Guangxi Zhuang Autonomous Region and Hainan Province (Figure 2).
Estimation model characteristics. First, there is no significant difference in TFP growth between the DDF and the DEA model. This could be because some studies use the DDF by selecting the evaluated unit’s input and output vectors as the directional vectors, effectively equating the DDF and the radial DEA model. Second, the TFP growth of the marine economy, measured by the SFA model, is 6.8 percent higher than that measured by the DEA method. The reason could be that the non-parametric DEA model assumes no random error and thus interprets all deviations from the production frontier in the actual input-output mix as technological inefficiency, thereby underestimating TFP growth. Third, the TFP growth of the marine economy measured by the SFA model is 6.8 percent higher on average than that measured by the DEA method. Fourth, estimation results obtained by the SBM model were significantly lower than those of the DEA model. Characteristics of the SBM model could explain this. In contrast to the traditional DEA model, the SBM model can solve the problem of non-zero slack in inputs or outputs. Therefore, the SBM model has been primarily used by academics to estimate TFP growth with undesirable outputs included.
Evaluation indicator characteristics. The results of the TFP growth estimates of the marine economy using green indicators are significantly lower than those using traditional indicators. This could be because traditional evaluation indicators overlook the negative environmental consequences of marine economic development, allowing the TFP growth of the marine economy to be overestimated (Huang et al., 2022).
Publication characteristics. The year of publication does not pass the significance test, indicating that the year of publication has no significant effect on the estimation results of TFP growth in the provincial marine economy. The result also demonstrates that the TFP growth estimates in the marine economy are mature in terms of concepts and methods and that the estimation results of primary studies from various periods are highly consistent and unaffected by the publication date. In terms of the journal’s rank, there are some differences between the TFP growth estimates of the marine economy in core journals and non-core journals. The coefficient of core journals is significant at the 5% level, indicating that core journals tend to get higher estimation results.
Economic factors characteristics. The gross ocean product has a negative effect on the TFP growth of the marine economy, which is significant at the 1% level. This finding indicates that, while the marine economy has developed rapidly over the last three decades and achieved a breakthrough in total volume, the marine economic TFP growth has not improved concurrently. The result may be attributed to the current situation of excess physical factor input, insufficient scientific and technological innovation, and the deterioration of the marine environment in previous development processes. In addition, the regression coefficient for the level of openness to the outside world is insignificant. This might be due to the significant differences in the length of each province’s coastline, port conditions, and marine resources, which cause the level of marine economic development in some provinces to be inconsistent with their overall stage of economic growth. The level of marine science and technology and the proportion of marine tertiary industry also significantly positively affect the improvement of the TFP growth of the provincial marine economy. For every 1% increase in the level of marine science and technology and the proportion of the marine tertiary industry, the TFP growth of the provincial marine economy will increase by 3.9% and 10.7% on average, respectively.
4.3.2 Meta-regression results at the national level
Time characteristics. The time dummy variables are significantly negative, indicating that the marine economy’s TFP growth is lower in other periods than in the Ninth Five-Year Plan. This result could be explained as follows: First, the Ninth Five-Year Plan is the earliest estimation cycle and the first five-year plan following the implementation of China’s socialist market economy reform, which boosted the development of China’s marine economy. Second, during the Ninth Five-Year Plan, China introduced a series of policy initiatives to support the development of the marine economy formulated by the State Oceanic Administration in 1996, ushering in a new era of development for China’s marine economy. Third, during the Ninth Five-Year Plan, China implemented a science and technology strategic plan to promote the sea, and made more breakthroughs in high marine technology and scientific research, which contributed to the rapid growth of TFP in China’s marine economy through technological progress. Additionally, according to mean descriptive statistics, China’s marine economic TFP grew at a positive rate from the Ninth to the Thirteenth Five-Year Plans, but at a slower pace4.
Estimation model characteristics, evaluation indicator characteristics, and publication characteristics. In contrast to the results of the meta-regression analysis at the provincial level, the regression coefficients for the estimation model characteristics variables, evaluation indicator characteristics variables, and publication characteristics variables were not significant at the national level. This is most likely because the observations at the national level were insufficient to compare the variability among the various characteristics.
Economic factor characteristics. The results of the meta-regression on gross ocean product, marine science and technology level, and marine industry structure are generally consistent with the TFP growth of the marine economy at the provincial level. The difference is that the regression coefficient for level of external openness is significantly positive at the 1% level, indicating that increasing external openness has a beneficial effect on the TFP growth of the marine economy at the national level.
5 Conclusions and discussions
This paper collected 622 observations of TFP growth for China’s marine economy from 33 primary studies and used a meta-analysis to synthesize the TFP growth estimates and analyze the impacts of several related factors on the heterogeneities of TFP growth in the primary studies. Our results show that all characteristics variables of five categories can cause heterogeneities in the TFP growth of China’s marine economy.
First, as suggested by the heterogeneity test results, there is significant variation among the TFP growth estimates presented in primary studies. On this basis, the overall mean TFP growth of China’s marine economy over the data period is close to zero at the provincial level and about 2.2 percent at the national level. Therefore, there is still room for improvement in the TFP growth of China’s marine economy.
Second, at the national level, the TFP growth of China’s marine economy during the Tenth Five-Year Plan and succeeding periods was significantly lower than that of the Ninth Five-Year Plan period. Generally, the overall growth rate showed a downward trend.
Third, at the provincial level, a longer time span of data will not only significantly improve the estimation results of TFP growth in the marine economy, but also help to improve the explanatory power of the estimation results. Moreover, the TFP growth of the marine economy in the Yangtze River Delta and Circum Bohai Sea region is significantly higher than that in the Pan-Pearl River Delta region.
In addition, other characteristics variables affect the TFP growth and show differences. Regarding the estimation model characteristics, the SFA model produces higher estimation results than the traditional DEA model, whereas the SBM model tends to get lower estimation results. Regarding the evaluation indicator characteristics, the TFP growth of the marine economy estimated using green indicators will be lower than traditional indicators. About the publication characteristics, the year of publication in publication characteristics does not affect the estimation results. However, there is some variability between the estimation results of core and non-core journals. Concerning the economic factor characteristics, the improvement of the level of opening up to the outside world, the level of marine science and technology, and the optimization of the marine industry structure all positively affect the TFP growth of the marine economy. In contrast, the rise in the gross ocean product has a negative impact on TFP growth.
According to the above research conclusions, this study proposes the following recommendations for further research. First, subsequent studies should collect data and information extensively and extend the time span of sample data as much as possible to improve the explanatory power of the estimation results. Second, as the statistical data cycle continues to be extended, the provincial sample size will grow annually. Therefore, a greater focus on the parametric SFA model could produce exciting findings. Third, with the continuous extension of the statistical data cycle, the sample size at the provincial level will increase year by year, which provides more possibilities for the application of the parametric SFA model. Therefore, given the reliability of the SFA model, it should receive greater attention in future studies. Fourth, further research regarding the impact of environmental variables on the TFP of the marine economy would be worthwhile. Moreover, green evaluation index systems that can consider non-desired inputs and outputs should be applied more to fulfill the high-quality development goals.
Furthermore, the empirical results in this paper have some practical implications for policy making. First, China should actively seek new growth points for the marine economy as it transitions to a new stage of high-quality development to avoid a further decline in TFP growth. For example, the government should prioritize marine science and technology innovation and focus on marine technological progress. Second, more extraordinary efforts are required to ensure the regional growth of the marine economy. In particular, the Pan-Pearl River Delta region should fully exploit the established advantages of the Guangdong-Hong Kong-Macao Greater Bay Area to drive late-developing provinces such as Guangxi and Hainan to achieve synergistic development and shared prosperity. Third, China should further expand the degree of openness to the outside world and improve the ability to develop marine resources based on sound marine ecological civilization construction. Furthermore, it is also crucial to vigorously develop marine transportation, diversify coastal tourism and optimize the structure of the marine industry to accelerate the high-quality development of China’s marine economy.
Data availability statement
The raw data supporting the conclusions of this article will be made available by the authors, without undue reservation.
Author contributions
JD: Investigation, Formal analysis, Data curation, Writing – original draft, Writing – review and editing. DQ: Conceptualization, Data curation, Writing – original draft, Writing – review and editing. BY: Methodology, Formal analysis, Writing – original draft, Funding acquisition. TX: Software, Writing – original draft, Writing – review and editing, Funding acquisition, Supervision. All authors contributed to the article and approved the submitted version.
Funding
This study was supported by the National Natural Science Foundation of China (No.72003054; No.72103052), the Hainan Provincial Natural Science Foundation of China (No.2019RC139; No.722RC630; No.720RC576). The Education Department of Hainan Province (No.Qhys2021–80).
Conflict of interest
The authors declare that the research was conducted in the absence of any commercial or financial relationships that could be construed as a potential conflict of interest.
Publisher’s note
All claims expressed in this article are solely those of the authors and do not necessarily represent those of their affiliated organizations, or those of the publisher, the editors and the reviewers. Any product that may be evaluated in this article, or claim that may be made by its manufacturer, is not guaranteed or endorsed by the publisher.
Footnotes
- ^ Since the TFP growth may be negative in the maritime economy, this study first added 1 to the TFP growth and then calculated its natural logarithm.
- ^ In this paper, the TFP of the marine economy is divided into traditional TFP and green TFP according to whether the non-desired output is considered in the evaluation indicators of the literature selected. And some studies estimate both types of TFP growth in the marine economy.
- ^ According to the descriptive statistics of TFP growth, the average value at the provincial level is lower than the average at the national level. This could be due to considerable differences in the volume and TFP growth of the marine economy between coastal provinces. When the TFP growth of the marine economy is estimated at the national level, the average value is assigned based on the volume of the marine economy in each province, resulting in a more objective average value. When the TFP growth of the marine economy in each province is estimated separately and the average value is calculated, each province is given the same weight, resulting in smaller volumes of the marine economy being given larger weights. In that case, the average estimates at the provincial level would be underestimated. The difference between the average provincial and national estimates has no bearing on the following empirical analysis.
- ^ During the period from the Ninth Five-Year Plan to the Thirteenth Five-Year Plan, the average estimates of total factor productivity of China's marine economy were 1.577, 1.072, 1.025, 1.023, and 1.019, respectively.
References
Aiello F., Bonanno G. (2016). Efficiency in banking: a meta-regression analysis. Int. Rev. Appl. Econ. 30, 112–149. doi: 10.1080/02692171.2015.1070131
Aigner D., Lovell C. A. K., Schmidt P. (1977). Formulation and estimation of stochastic frontier production function models. J. Econ. 6, 21–37. doi: 10.1016/0304-4076(77)90052-5
Angelini F., Castellani M., Vici L. (2022). Restaurant sector efficiency frontiers: a meta-analysis. J. Foodservice Bus. Res. 2022, 1–19. doi: 10.1080/15378020.2022.2077090
Benos N., Zotou S. (2014). Education and economic growth: A meta-regression analysis. World Dev. 64, 669–689. doi: 10.1016/j.worlddev.2014.06.034
Brander L. M., Florax R. J. G. M., Vermaat J. E. (2006). The empirics of wetland valuation: A comprehensive summary and a meta-analysis of the literature. Environ. Resour. Econ. 33, 223–250. doi: 10.1007/s10640-005-3104-4
Cai Y. Y., Tian Z. G., Wang Q. M., Gong W. (2018). Research on the competitiveness of marine economy based on DEA method. Prod. Res. 2018, 14–17. doi: 10.19374/j.cnki.14-1145/f.2018.10.003
Card D., Kluve J., Weber A. (2010). Active labour market policy evaluations: A meta-analysis. Econ. J. 120, F452–F477. doi: 10.1111/j.1468-0297.2010.02387.x
Chaikumbung M. (2021). Institutions and consumer preferences for renewable energy: A meta-regression analysis. Renewable Sustain. Energy Rev. 146, 111143. doi: 10.1016/j.rser.2021.111143
Chaikumbung M., Doucouliagos H., Scarborough H. (2016). The economic value of wetlands in developing countries: A meta-regression analysis. Ecol. Econ. 124, 164–174. doi: 10.1016/j.ecolecon.2016.01.022
Charnes A., Cooper W. W., Rhodes E. (1978). Measuring the efficiency of decision making units. Eur. J. Oper. Res. 2, 429–444. doi: 10.1016/0377-2217(78)90138-8
Chen S., Hui Q. (2019). Dynamic DEA efficiency evaluation of marine economy in hainan province. Mod. Bus. 2019, 91–93. doi: 10.14097/j.cnki.5392/2019.14.045
Chung Y. H., Färe R., Grosskopf S. (1997). Productivity and undesirable outputs: A directional distance function approach. J. Environ. Manage. 51, 229–240. doi: 10.1006/jema.1997.0146
den Besten H. M. W., Zwietering M. H. (2012). Meta-analysis for quantitative microbiological risk assessments and benchmarking data. Trends Food Sci. Technol. 25, 34–39. doi: 10.1016/j.tifs.2011.12.004
Di Q. B., Liang Q. Y. (2018). The spatio-temporal differences of marine economic efficiency in China and analysis of influencing factors under carbon emission constraints. Mar. Sci. Bull. 37, 272–279. doi: 10.11840/j.issn.1001-6392.2018.03.004
Ding L. L., Liu S. B., Wang C., Yang Y. (2019). Research on biased technology progress and measurement of total factor productivity in green marine economy. Mar. Econ. 9, 12–19. doi: 10.19426/j.cnki.cn12-1424/p.2019.04.002
Ding L. L., Zheng H. H., Kang W. L. (2017b). Measuring the green efficiency of ocean economy in China: An improved three-stage DEA model. Rom. J. Econ. Forecast. 20, 5–22. Available at: https://ipe.ro/rjef/rjef1_17/rjef1_2017p5-22.pdf.
Ding L. L., Zheng H. H., Wang W. (2017a). Measurement and analysis of marine economic productivity in China based on improved RAM-undesirable model. J. Cent. Univ. Financ. Econ. 2017, 119–128. Available at: https://kns.cnki.net/kcms/detail/detail.aspx?FileName=ZYCY201709013&DbName=CJFQ2017
Ding L. L., Zhu L., He G. S. (2015). Measurement and influencing factors of green total factor productivity of marine economy in China. Forum Sci. Technol. China 2, 72–78. doi: 10.13580/j.cnki.fstc.2015.02.014
Djokoto J. G., Gidiglo F. K., Srofenyoh F. Y., Agyei-Henaku K. A. A.-O., Afrane Arthur A. A., Badu-Prah C. (2020). Sectoral and spatio-temporal differentiation in technical efficiency: A meta-regression. Cogent. Econ. Financ. 8:1773659. doi: 10.1080/23322039.2020.1773659
Du X. X., Li J. M. (2018). Analysis of the impact of discharge of wastewater on marine economic efficiency. Mar. Econ. 8, 38–47. doi: 10.19426/j.cnki.cn12-1424/p.2018.01.005
Du J., Yan B., Feng R. M. (2016). Evaluation of the efficiency of the marine economies of china’s coastal regions. J. Agrotechnical Econ. 2016, 47–55. doi: 10.13246/j.cnki.jae.2016.06.005
Efendic A., Pugh G., Adnett N. (2011). Institutions and economic performance: A meta-regression analysis. Eur. J. Polit. Econ. 27, 586–599. doi: 10.1016/j.ejpoleco.2010.12.003
Egger M., Smith G. D., Phillips A. N. (1997). Meta-analysis: Principles and procedures. BMJ 315, 1533–1537. doi: 10.1136/bmj.315.7121.1533
Estache A., de la Fé B. T., Trujillo L. (2004). Sources of efficiency gains in port reform: a DEA decomposition of a malmquist TFP index for Mexico. Util. Policy 12, 221–230. doi: 10.1016/j.jup.2004.04.013
Fall F., Akim A., Wassongma H. (2018). DEA and SFA research on the efficiency of microfinance institutions: A meta-analysis. World Dev. 107, 176–188. doi: 10.1016/j.worlddev.2018.02.032
Färe R., Grosskopf S. (1992). Malmquist productivity indexes and fisher ideal indexes. Econ. J. 102, 158–160. doi: 10.2307/2234861
Feng Y., Zhong S., Li Q., Zhao X., Dong X. (2019). Ecological well-being performance growth in china, (1994–2014): From perspectives of industrial structure green adjustment and green total factor productivity. J. Clean. Prod. 236:117556. doi: 10.1016/j.jclepro.2019.07.031
Gai M., Liu D. D., Qu B. L. (2016). The research for spatial-temporal differentiation and influencing factors of green marine economic effificiency in China. Ecol. Econ. 32, 97–103. doi: 10.3969/j.issn.1671-4407.2016.12.021
Guan H. J., Sun Z. Z., Gao H. N., Zhao A. W. (2019). An analysis of spatio-temporal evolution of marine economy green total factor productivity and its influencing factors in China. J. Ocean Univ. China (Soc. Sci.) 2019, 40–53. doi: 10.16497/j.cnki.1672-335X.201906004
Han Z. L., Wang X. C., Peng F. (2019). Dynamic measurement and prediction of total factor productivity of marine economy in China. Geogr. Geo-Information Sci. 35, 95–101. doi: 10.3969/j.issn.1672-0504.2019.01.015
Han Z. L., Xia K., Guo J. K., Sun C., Deng Z. (2017). Research of the level and spatial differences of land-sea coordinate development in coastal areas based on global-Malmquist-Luenberger index. J. Natural Resour. 32, 1271–1285. doi: 10.11849/zrzyxb.20160775
Hassanpour B., Ismail M. M., Mohamed Z., Kamarulzaman N. H. (2010). Sources of productivity growth in rainbow trout aquaculture in Iran: Technical efficiency change or technological progress? Aquacult. Econ. Manage. 14, 218–234. doi: 10.1080/13657305.2010.503474
He F., Huang M. (2018). Study on the evaluation of marine economic efficiency in eastern China. Market Modernization 2018, 168–169. doi: 10.14013/j.cnki.scxdh.2018.10.104
Hershey H. (2021). Updating the consensus on fishway efficiency: A meta-analysis. Fish and Fisheries 22, 735–748. doi: 10.1111/faf.12547
Hu X. Z. (2018). Regional growth differences and convergence analysis of green total factor productivity in china’s marine economy. Stat Decision 34, 137–140. doi: 10.13546/j.cnki.tjyjc.2018.17.034
Hua X. Y., Lv H. P., Jin X. R. (2021). Research on high-quality development efficiency and total factor productivity of regional economies in China. Sustainability 13:8287. doi: 10.3390/su13158287
Huang X., Feng C., Qin J., Wang X., Zhang T. (2022). Measuring china’s agricultural green total factor productivity and its drivers during 1998–2019. Sci. Total Environ. 829:154477. doi: 10.1016/j.scitotenv.2022.154477
Jin D., Thunberg E., Kite-Powell H., Blake K. (2002). Total factor productivity change in the new England groundfish fishery: 1964–1993. J. Environ. Econ. Manage. 44, 540–556. doi: 10.1006/jeem.2001.1213
Ji J. Y., Wang Q., Ren W. H. (2017). An empirical study on the growth pattern of marine economy in China–based on stochastic frontier analysis of translog production function. Proc. 19th Chin. Annu Conf. Manage. Sci., 615–621 Available at: https://kns.cnki.net/kcms/detail/detail.aspx?FileName=ZHYJ201710001091&DbName=CPFD2018.
Ji Y. J., Zhang Y. Y. (2016). Research on performance measurement of regional marine economic development in China: Positive analysis based on model of SBM and index of malmquist-luenberger. J. Guangdong Ocean Univ. 36, 1–8. doi: 10.3969/j.issn.1673-9159.2016.02.001
Johnson D. G. (1997). Agriculture and the wealth of nations. Am. Econ. Rev. 87, 1–12. Available at: https://www.jstor.org/stable/2950874
Kumar S. (2006). Environmentally sensitive productivity growth: A global analysis using malmquist–luenberger index. Ecol. Econ. 56, 280–293. doi: 10.1016/j.ecolecon.2005.02.004
Li Z., Meng N., Yao X. (2017). Sustainability performance for china’s transportation industry under the environmental regulation. J. Clean. Prod. 142, 688–696. doi: 10.1016/j.jclepro.2016.09.041
Liu X. M., Liu G. D., Ding L. L. (2015). Analysis on the difference and convergence of marine low carbon economic efficiency of area of China. J. Ind. Technol. Econ. 34, 37–46. doi: 10.3969/j.issn.1004-910X.2015.09.005
Liu D. D., Zhu X. Y., Wang Y. F. (2021). China’s agricultural green total factor productivity based on carbon emission: An analysis of evolution trend and influencing factors. J. Clean. Prod. 278:123692. doi: 10.1016/j.jclepro.2020.123692
Li J. K., Zhang J., Gong L. T., Miao P. (2015). Research on the total factor productivity and decomposition of Chinese coastal marine economy: Based on DEA-malmquist index. J. Coast. Res. 73, 283–289. doi: 10.2112/SI73-050.1
Li X., Zhou S., Yin K., Liu H. (2021). Measurement of the high-quality development level of china’s marine economy. Mar. Econ. Manage. 4, 23–41. doi: 10.1108/MAEM-10-2020-0004
Mahadevan R. (2003). To measure or not to measure total factor productivity growth? Oxford Dev. Stud. 31, 365–378. doi: 10.1080/1360081032000111742
Managi S., Opaluch J. J., Jin D., Grigalunas T. A. (2006). Stochastic frontier analysis of total factor productivity in the offshore oil and gas industry. Ecol. Econ. 60, 204–215. doi: 10.1016/j.ecolecon.2005.11.028
Mohammed S. I. S., Williamson J. G. (2004). Freight rates and productivity gains in British tramp shipping 1869–1950. Explor. Econ. Hist. 41, 172–203. doi: 10.1016/S0014-4983(03)00043-3
Munisamy S., Arabi B. (2015). Eco-efficiency change in power plants: using a slacks-based measure for the meta-frontier malmquist–luenberger productivity index. J. Clean. Prod. 105, 218–232. doi: 10.1016/j.jclepro.2014.12.081
Ogundari K. (2014). The paradigm of agricultural efficiency and its implication on food security in Africa: What does meta-analysis reveal? World Dev. 64, 690–702. doi: 10.1016/j.worlddev.2014.07.005
Qin L., Miao X., Tan J., Li S. (2021). Environmental regulation and green total factor productivity: Evidence from china’s marine economy. Pol. J. Environ. Stud. 30, 5117–5131. doi: 10.15244/pjoes/136010
Qin L. G., Shen T. Y. (2020). Does technological innovation promote the high quality development of china’s marine economy–empirical test based on effect of technological innovation on GTFP. Sci. Technol. Prog. Policy 37, 105–112. doi: 10.6049/kjjbydc.2019090755
Ren W. H., Ji J. Y., Chen L., Zhang Y. (2018b). Evaluation of china’s marine economic efficiency under environmental constraints–an empirical analysis of china’s eleven coastal regions. J. Clean. Prod. 184, 806–814. doi: 10.1016/j.jclepro.2018.02.300
Ren W. H., Wang Q., Ji J. Y. (2018a). Research on china’s marine economic growth pattern: An empirical analysis of china’s eleven coastal regions. Mar. Policy 87, 158–166. doi: 10.1016/j.marpol.2017.10.021
Salem M. E., Mercer D. E. (2012). The economic value of mangroves: A meta-analysis. Sustainability 4, 359–383. doi: 10.3390/su4030359
Schneider M., Beeres K., Coban L., Merz S., Susan Schmidt S., Stricker J., et al. (2017). Associations of non-symbolic and symbolic numerical magnitude processing with mathematical competence: A meta-analysis. Dev. Sci. 20:e12372. doi: 10.1111/desc.12372
Shang Y. C., Leung P. S., Ling B. H. (1998). Comparative economics of shrimp farming in Asia. Aquaculture 164, 183–200. doi: 10.1016/S0044-8486(98)00186-0
Solow R. M. (1957). Technical change and the aggregate production function. Rev. Econ. Stat 1957, 312–320. doi: 10.2307/1926047
Song Z. M., Ning L. (2020). Research hotsopts, frontiers and prospects of high-quality development of marine economy in China: Quantitative analysis based on citespace knowledge map. Ocean Dev. Manage. 37, 3–9. doi: 10.3969/j.issn.1005-9857.2020.12.001
Song Q. M., Sun C. Z., Gai M. (2019). Calculation of marine ecological efficiency and analysis of influencing factors in coastal areas of liaoning based on unexpected super efficiency model. Mar. Sci. Bull. 38, 508–518. doi: 10.11840/j.issn.1001-6392.2019.05.004
Stanley T. D., Doucouliagos C., Jarrell S. B. (2008). Meta-regression analysis as the socio-economics of economics research. J. Socio-Econ. 37, 276–292. doi: 10.1016/j.socec.2006.12.030
Stanley T., Rosenberger R. S. (2009). Are recreation values systematically underestimated? reducing publication selection bias for benefit transfer. Paper presented at the 2009 MAER-Net Colloquium, Corvallis, OR.
Sun C., Li X., Zou W., Wang S., Wang Z. (2018). Chinese Marine economy development: Dynamic evolution and spatial difference. Chin. Geogr. Sci. 28, 111–126. doi: 10.1007/s11769-017-0912-8
Sun P., Song L. F. (2019). Calculation of china’s marine environmental efficiency based on undesired super efficiency-malmquist model. China Popul., Resour. Environ. 29, 43–51. doi: 10.12062/cpre.20180722
Sun K., Zhou X. J., Bai L., Chai R. R. (2016). Study on time-space evolution of TFP in Shandong peninsula blue economic zone. Resour. Dev. Market 32, 522–526+613. doi: 10.3969/j.issn
Tan L., Wu X. H., Li L. S. (2020). Impact of climatedisasters on economic development: A meta-analysis. Stud. Sci. Sci. 38, 208–217. doi: 10.16192/j.cnki.1003-2053.2020.02.003
The State Council of the PRC (2022) China’s marine economy logs steady growth in 2021. Available at: http://english.www.gov.cn/archive/statistics/202204/06/content_WS624d5248c6d02e5335328d3c.html (Accessed April 06, 2022).
Thompson S. G., Higgins J. P. T. (2002). How should meta-regression analyses be undertaken and interpreted? Statist. Med. 21, 1559–1573. doi: 10.1002/sim.1187
Tian X., Yu X. H. (2012). The enigmas of TFP in China: A meta-analysis. China Econ. Rev. 23, 396–414. doi: 10.1016/j.chieco.2012.02.007
Tone K. (2001). A slacks-based measure of efficiency in data envelopment analysis. Eur. J. Oper. Res. 130, 498–509. doi: 10.1016/S0377-2217(99)00407-5
Trong Ho P., Burton M., Ma C., Hailu A. (2022). Quantifying heterogeneity, heteroscedasticity and publication bias effects on technical efficiency estimates of rice farming: A meta-regression analysis. J. Agric. Econ. 73, 580–597. doi: 10.1111/1477-9552.12468
Turner H., Windle R., Dresner M. (2004). North American containerport productivity: 1984–1997. Transp. Res. Part E: Logist. Transp. Rev. 40, 339–356. doi: 10.1016/j.tre.2003.06.001
Wang L. L. (2015). The dynamic decomposition on total factor productivity of marine economy: an empirical analysis with the three-stage malmquist index. Trans. Oceanol. Limnol. 2015, 199–206. doi: 10.13984/j.cnki.cn37-1141.2015.03.025
Wang L. L. (2017). Study on total factor productivity of marine economic under environmental restrains. Mar. Econ. China 2017, 228–249. Available at: https://kns.cnki.net/kcms/detail/detail.aspx?FileName=HYJN201701016&DbName=CCJD2017
Wang Q., He C. Y. (2020). Research on regional differences of marine economic efficiency in China. J. Liaoning Univ. (Philos. Soc. Sci. Edition) 48, 54–65. doi: 10.16197/j.cnki.lnupse.2020.01.007
Wang S., Lu B., Yin K. (2021). Financial development, productivity, and high-quality development of the marine economy. Mar. Policy 130:104553. doi: 10.1016/j.marpol.2021.104553
Wang L., Qiu X., Liu Z., Chen S. (2019). Ecological efficiency of china’s marine economy: A convergence analysis. J. Coast. Res. 94:983–987. doi: 10.2112/SI94-193.1
Wei X. Y., Hu Q. G., Shen W., Ma J. T. (2021). Influence of the evolution of marine industry structure on the green total factor productivity of marine economy. Water 13:1108. doi: 10.3390/w13081108
Xiang X. M., Zhang S. H., Hu X. Z. (2019). The dynamic mechanism and realization path of supply-side structural reform of marine economy–a study based on total factor productivity index of marine economy. Soc. Sci. Guangdong 2019, 27–35. doi: 10.3969/j.issn.1000-114X.2019.05.004
Xia F., Xu J. T. (2020). Green total factor productivity: A re-examination of quality of growth for provinces in China. China Econ. Rev. 62:101454. doi: 10.1016/j.chieco.2020.101454
Yan Y., Yao L. Y., Lang L. M., Zhao M. J. (2019). Revaluation of ecosystem services in inland river basins of China: based on meta-regression analysis. Acta Geogr. Sin. 74, 1040–1057. doi: 10.11821/dlxb201905015
Ye F., Quan Y. B., He Y. X., Lin X. F. (2021). The impact of government preferences and environmental regulations on green development of china’s marine economy. Environ. Impact Assess. Rev. 87, 106522. doi: 10.1016/j.eiar.2020.106522
Yuan Q. M., Zhang W. L., Feng D. (2016). An analysis of Chinese marine economic efficiency change and productivity change under constraints of resources and environment. Econ. Survey 33:13–18. doi: 10.15931/j.cnki.1006-1096.2016.03.004
Zhang Y. (2019). Dynamic research on total factor productivity of china’s ocean economy. J. Coast. Res. 98, 227–230. doi: 10.2112/SI98-056.1
Zhang N., Guo X., Yan Y. L. (2018). Marine economy, green total factor productivity and informatization level: empirical analysis based on 11 coastal provinces and cities in China. Prod. Res. 2018:87–92+100+161. doi: 10.19374/j.cnki.14-1145/f.2018.09.016
Zhang Y., Wang S. H. (2021). Influence of marine industrial agglomeration and environmental regulation on marine innovation efficiency–from an innovation value chain perspective. Mar. Policy 134:104807. doi: 10.1016/j.marpol.2021.104807
Zhao X., Xue Y. M., Kang W. L., Ding L. L., Zhu L. (2018). Measuring efficiency of ocean economy in China based on a novel luenberger approach. Rom. J. Econ. Forecast. 21, 5–21. Available at: https://ipe.ro/rjef/rjef2_18/rjef2_2018p5-21.pdf
Zhou M. H., Ju Z. (2013). Empirical study on total factor productivity of new marine strategic industries in guangdong. J. Hubei Univ. Econ. 11, 43–47. doi: 10.3969/j.issn.1672-626x.2013.02.008
Keywords: marine economy, total factor productivity, meta-analysis, high-quality development, China
Citation: Dong J, Qiao D, Yuan B and Xu T (2022) Total factor productivity of China’s marine economy: A meta-analysis. Front. Mar. Sci. 9:1014112. doi: 10.3389/fmars.2022.1014112
Received: 08 August 2022; Accepted: 31 August 2022;
Published: 16 September 2022.
Edited by:
Ibukun Jacob Adewumi, University of Wollongong, AustraliaReviewed by:
Lyndon Edwin Llewellyn, Australian Institute of Marine Science, AustraliaJuan Portillo, University of Lisbon, Portugal
Copyright © 2022 Dong, Qiao, Yuan and Xu. This is an open-access article distributed under the terms of the Creative Commons Attribution License (CC BY). The use, distribution or reproduction in other forums is permitted, provided the original author(s) and the copyright owner(s) are credited and that the original publication in this journal is cited, in accordance with accepted academic practice. No use, distribution or reproduction is permitted which does not comply with these terms.
*Correspondence: Tao Xu, xutao_2013@outlook.com