Mucus-associated microbiotas among different body sites of wild tuna from the South China Sea
- 1School of Life Sciences, State Key Laboratory of Marine Resource Utilization in South China Sea, Hainan University, Haikou, China
- 2Key Laboratory of Utilization and Conservation for Tropical Marine Bioresources, Hainan Key Laboratory for Conservation and Utilization of Tropical Marine Fishery Resources, College of Fisheries and Life Science, Hainan Tropical Ocean University, Sanya, China
The mucus-associated symbionts have profound impacts on the pathogen defense, metabolism, and development of aquatic animals. To understand the microbial structure of regional endothermic fish, a total of 52 samples from the skin, oral, gill, and hindgut of wild tuna Thunnus albacares and T. obesus were determined by 16S amplicon sequencing. The results showed the diversity and composition of microbial communities varied in the four different body sites of tunas, with a greater heterogeneity between the external surface and the gut. Phyla Proteobacteria, Firmicutes, Actinobacteria and genus Acinetobacter were found in high relative abundance in all body sites. The other abundant taxa were enriched in different body sites, such as Lactobacillus and Kocuria in the skin and Geobacillus in the gut. The core taxa interacted with each other to different degrees in the four body sites, which may be related to species’ co-evolution and microbial community stability. Finally, the correlation between biomarkers and COG functions highlighted the importance of microbial biomarkers to the host. This work firstly characterized the microbial feature in different body sites of wild tunas, providing a foundational dataset to understand the microbial role in endothermic fish and to find key microbial components beneficial to farmed tunas.
Introduction
The host and its diverse microbial communities are so closely linked that they are often described as a single entity: the holobiont, which is considered a unit of selection in host-microbiota co-evolution (Rosenberg and Zilber-Rosenberg, 2018). In that pattern, host genetics is considered a dominant driver in shaping host microbiotas, and microbiotas colonized in different body mucus play a paramount role in host phenotypes and traits (Lynch and Hsiao, 2019). Gut microbiotas are well-known contributors of host symbionts to a broad set of functions related to host immunity, metabolism, and development (Banerjee and Ray, 2017; Wang et al., 2018). The outer mucosal microbiotas serve as a preliminary dynamic interface between the fish and the environment and have essential roles in resisting pathogen invasion (Ross et al., 2019), and take surface mucus as an intermediate niche between the water and digestive tract (Carda-Dieguez et al., 2017).
The microbial stability in the mucus layer is pivotal for host health promotion and mutualistic microbiotas configuration (Wang et al., 2018; Fassarella et al., 2021). It depends on the high microbial diversity and abundance, which makes sure that microorganisms with similar functions can act as substitutes when probiotics are reduced (Fassarella et al., 2021). Further, the complex interactions within microbial communities from mutualism to competition, and the symbiotic relationship between microbes and their host, are essential for host homeostasis (Foster et al., 2017; Fassarella et al., 2021). For instance, gut microbiotas members, especially Firmicutes, were essential to lipid droplet formation and fatty acid uptake in the intestinal epithelium of zebrafish (Semova et al., 2012). The commensal skin-microbiotas are key factors in skin wounds healing, mediated by triggering IFN-dependent innate repair responses (Di Domizio et al., 2020). Additionally, core microbial community, including bacteria, Archaea, microeukaryotes and viruses, are more relatively conserved in composition and function, which is expected to stabilize the ecosystem (Shetty et al., 2017).
Fish can acquire microbiotas through surrounding water from the early developmental phases (Spor et al., 2011). Its larval microbiotas depend greatly on water quality, salinity, nutrients, and oxygen content (Dehler et al., 2017; Wang et al., 2018; Sylvain et al., 2020). Once the microbiome dynamic balance is affected by environmental changes, such as water contamination, living space limitation, or antibiotics exposure, the marked compositional change and diversity decrease will be found in microbial communities (Ross et al., 2019), resulting in host chronic diseases and recurrent infection over time. For example, common pathogens Vibrio, Flavobacterium, Arcobacter and Allorhizobium are persistently dominant in the ulcer mucus of unhealthy fish (Karlsen et al., 2017; Sultana et al., 2022). Anthropogenic antibiotics intervention is generally used to resist pathogen expansion but may lead to the accumulation of antibiotic resistance genes in the microbiome (Willmann et al., 2019). In a microbiota-mediated way, pathogen overgrowth can be prevented by the microbial colonization resistance, which is performed by means of niche and nutrient competitions, conjugation-dependent killing, and antagonistic molecules (Buffie and Pamer, 2013). A previous study has confirmed that Bacteroides spp. can produce short-chain fatty acid (SCFA) propionate and adjust intracellular pH homeostasis to directly inhibit Salmonella Typhimurium growth (Jacobson et al., 2018). Moreover, Stressmann et al. (2020) demonstrated that conventional zebrafish with 10 culturable bacterial species showed sufficiently reduced infection susceptibility than germ-free individuals. Therefore, the specific microbial components are critical for the resilience and stability of the microbial community when encountering perturbance.
Tuna is one of the most commercially valuable marine fish with high nutrients (FAO, 2020). Open-net pens aquaculture industry for tuna is in high demand because of limited wild resources. However, cultured tunas with high density are more susceptible to opportunistic pathogenic bacteria and infectious parasites (Nowak et al., 2021), since their living environment was changed and the structure of host-associated microbial communities is altered (Minich et al., 2020a). However, our understanding of mucosal microbial symbionts in wild tuna is limited, especially from multiple body sites.
Here, yellowfin tuna (Thunnus albacares) and bigeye tuna (Thunnus obesus) are chosen as wild hosts to study mucus-associated symbionts. They have similar habitats, shapes, and diets, and are common in the South China Sea (Varela et al., 2017; Ohshimo et al., 2018). The primary objectives of this study were (1) to compare the diversity and structure of the mucus-associated microbial community in three dimensionalities (intraspecies, interspecies, and interindividual), and (2) to determine the composition and feature of microbiotas of healthy tunas among different body sites, and (3) to detect potential microbial biomarkers of tuna’s health status. This study will let us better understand the relationship between the symbiotic microbes and host health, and further contribute to the aquaculture industry of tuna.
Materials and methods
Sample collection
Wild tunas (11 yellowfin tunas and 4 bigeye tunas) were captured by line lures from the South China Sea (17°24′N, 110°36′E) in August 2021. The two species were classified preliminarily on the spot and the final identification was determined by comparing cytochrome C oxidase subunit I (COI) gene sequences of muscle tissues to NCBI (Supplementary Table 1). The detailed process of mucus bacteria sampling is shown below. When tuna was hooked, external (skin, oral, and gill) mucus bacteria were wiped from alive fish immediately by sterile cotton swabs, and each site was wiped with at least two swabs to make sure enough mucus to extract DNA. After dissection, the hindgut mucus-associated bacteria were collected from hindgut contents squeezed out by sterilized scissors and tweezers. Sampling sites among individuals were the same. According to fork length, body weight, and species, the tuna individuals were divided into three groups and a total of 60 biological samples were collected (Table 1). We also collected environmental microorganisms from 40 m depth by filtering 250 mL of seawater through a 0.22-µm-pore-size polycarbonate membrane, and 6 seawater samples were preserved. All the biological and environmental samples were quickly frozen in liquid nitrogen and transferred to a -80°C refrigerator until the next procedure.
16S amplicon sequencing
The DNA extraction was processed using the FastDNA® Spin Kit for Soil (MP Biomedicals, Norcross, GA, U.S.) according to the manufacturer’s protocols. To obtain amplification of V3-V4 hypervariable regions of the 16S rRNA gene, triplicate PCR reactions of each sample were started at 95°C for denaturation and followed by 27 cycles at 95°C for 30 s, annealed at 55°C for 30 s, elongated at 72°C for 45 s, and finalized with an extension at 72°C for 10 min. Each 20 μL PCR mixture contained 4 μL of 5×FastPfu Buffer, 2 μL of 2.5 mM dNTPs, 0.8 μL of 5 μM primers (338F: 5′-ACTCCTACGGGAGGCAGCAG-3′ and 806R: 5′-GGACTACHVGGGTWTCTAAT-3′; Liu et al., 2016), 0.4 μL of FastPfu Polymerase (TransGen, Beijing, China), and 10 ng of template DNA. The amplified fragments were sent to Majorbio Bio-Pharm Technology Co. Ltd. (Shanghai, China) for paired-end sequencing (2×300 bp) using the Illumina MiSeq platform (Illumina, San Diego, USA). All raw reads were deposited in the NCBI Sequence Read Archive (SRA) database (BioProject ID: PRJNA884520 and PRJNA902642).
Bioinformatics processing
Quality control was done by fastp version 0.20.0 (Chen et al., 2018). The reads were truncated when their average quality score was <20 and filtrated when the read length was <50 bp after quality-controlling. PE reads were merged according to their overlap (>10 bp, allowing 2 bp mismatching) by FLASH version 1.2.7 (Magoč and Salzberg, 2011). Sequences of each sample were screened according to barcodes (exactly matching) and primers (allowing 2 bp mismatching).
Operational taxonomic units (OTUs) were clustered with a 97% similarity cut-off by Uparse version 7.0.1090 (Edgar, 2013). The taxonomy of each 16S rRNA gene sequence was analyzed by the RDP Classifier Bayesian algorithm against the Silva v1.3.8 16S rRNA database (default confidence threshold of 0.7) (Wang et al., 2007). After that, the OTUs were normalized to the smallest library to eliminate sample heterogeneity, by “subsample” function in Mothur version 1.30.2 (Schloss et al., 2009) following the method in Minniti et al. (2017).
Data analysis
The alpha diversity of the microbiome was estimated using Mothur. Significant differences of alpha diversity indices were tested by Welch’s t-test at the OTU level. Alpha diversity indices were visualized by Graphpad Prism (version 9.0.0). Beta diversity analysis was based on Bray-Curtis distance and visualized by principal coordinates analysis (PCoA) to conduct clustering at the OTU level of the sample community. Pairwise comparisons of beta diversity distances between groups were calculated by both Bray-Curtis metrics and weighted Unifrac metrics (Rosado et al., 2019). Briefly, Bray-Curtis distance was calculated by the vegan package in R version 4.0.3 based on the OTUs table. Weighted UniFrac distance was calculated by GUniFrac package, based on the phylogenetic tree constructed by the OTUs table, using phyloseq package. Significant differential species among four body parts were analyzed by Kruskal-Wallis H Test at the genus level by SPSS version 26.0 (Zhang et al., 2019). To analyze the difference between host-associated microbiotas and seawater microbiotas, Spearman correlation heatmap was analyzed in R, using the “corr.test” function and pheatmap package. To find the main species contributing microbial difference between body sites and seawater, similarity percentage (SIMPER) was utilized by PRIMER version 5.2.8 (Gardner et al., 2019).
Linear discriminant analysis Effect Size (LEfSe) was conducted to estimate the biomarkers from phylum to genus with the threshold of linear discriminant analysis (LDA) score of 3.5 (default setting), under the premise of the Kruskal-Wallis H Test (Segata et al., 2011).
The network analysis was based on core OTUs that occurred in at least 70% of all samples in each group. The network was constructed at the genus level by the NetworkX version 1.11 (Hagberg et al., 2008). The top 50 abundant genera in each body site were connected by Spearman’s correlation coefficient (r>0.75, p<0.05).
The function of the OTUs was predicted by the Phylogenetic Investigation of Communities by Reconstruction of Unobserved States (PICRUSt version 2.0; Douglas et al., 2020). The COG (Cluster of Orthologous Group) annotation was obtained by mapping to the EggNOG library version 5.0. The relative abundance difference of COG function classifications was determined by the Kruskal-Wallis H Test.
Results
In this study, 86.67% (52/60) of biological samples from four body sites of tunas were sequenced successfully, including 14 from skin, 13 from oral, 13 from gill, and 12 from hindgut. A total of 1,520,324 sequences were subsampled and 10,208 OTUs were obtained, representing 61 phyla, 182 classes, 445 orders, 786 families, and 1,924 genera. The number of sequences per sample ranged from 30,000 to 60,000, with an average length of 417 bp.
Variation of the microbial community does not correlate with host body size or species
The taxonomy composition of OTUs was used to test whether the body size or species of the host is related to the variation of the microbial community in different body sites. The results showed that no significant difference was found in mucus-associated microbial diversity with body size or species (Supplementary Table 2. Shannon index, p>0.05; PERMANOVA, p>0.05). Therefore, the following analysis will focus on the correlation between the microbial community and four body sites, i.e. group S (skin), group O (oral), group G (gill), and group H (hindgut).
Microbial diversity in different body sites
The results of six alpha diversity indices showed significantly distinct richness and diversity across body sites (Figure 1). In detail, the Sobs index detected four groups that had significant differences in community richness (Figure 1A. Welch’s t-test, S vs O: p=0.0018; S vs G: p=0.0008; O vs H: p=0.0018; G vs H: p=0.0009). And similar significances were observed in Chao index (Figure 1B). The Shannon index revealed a significantly high diversity in gill compared to skin or hindgut (Figure 1C, Welch’s t-test, G vs S: p=0.0017; G vs H: p=0.0284). The Shannoneven index indicated skin had a significant difference with gill (p=0.0143) and hindgut (p=0.0378) (Figure 1D). Significant differences of community coverage were detected by the Good’s coverage index (Figure 1E. Welch’s t-test, S vs O: p=0.0031; O vs H: p=0.0002; G vs H: p=0.0198). The Pd index showed a significant difference in phylogenetic diversity for all pairwise comparisons except oral vs gill (Figure 1F). No significant difference in microbial diversity index was found between the oral and gill (Figures 1A–F). In addition, the difference in diversity of microbiotas between the host surface mucus and the environment was compared. The results showed environmental microbial indices were significantly less than that in the skin (Welch’s t-test, Sobs: p=0.0002; Shannon: p=0.0001; Shannoneven: p=0.0007; Pd: p=0.0006) (Supplementary Table 3).
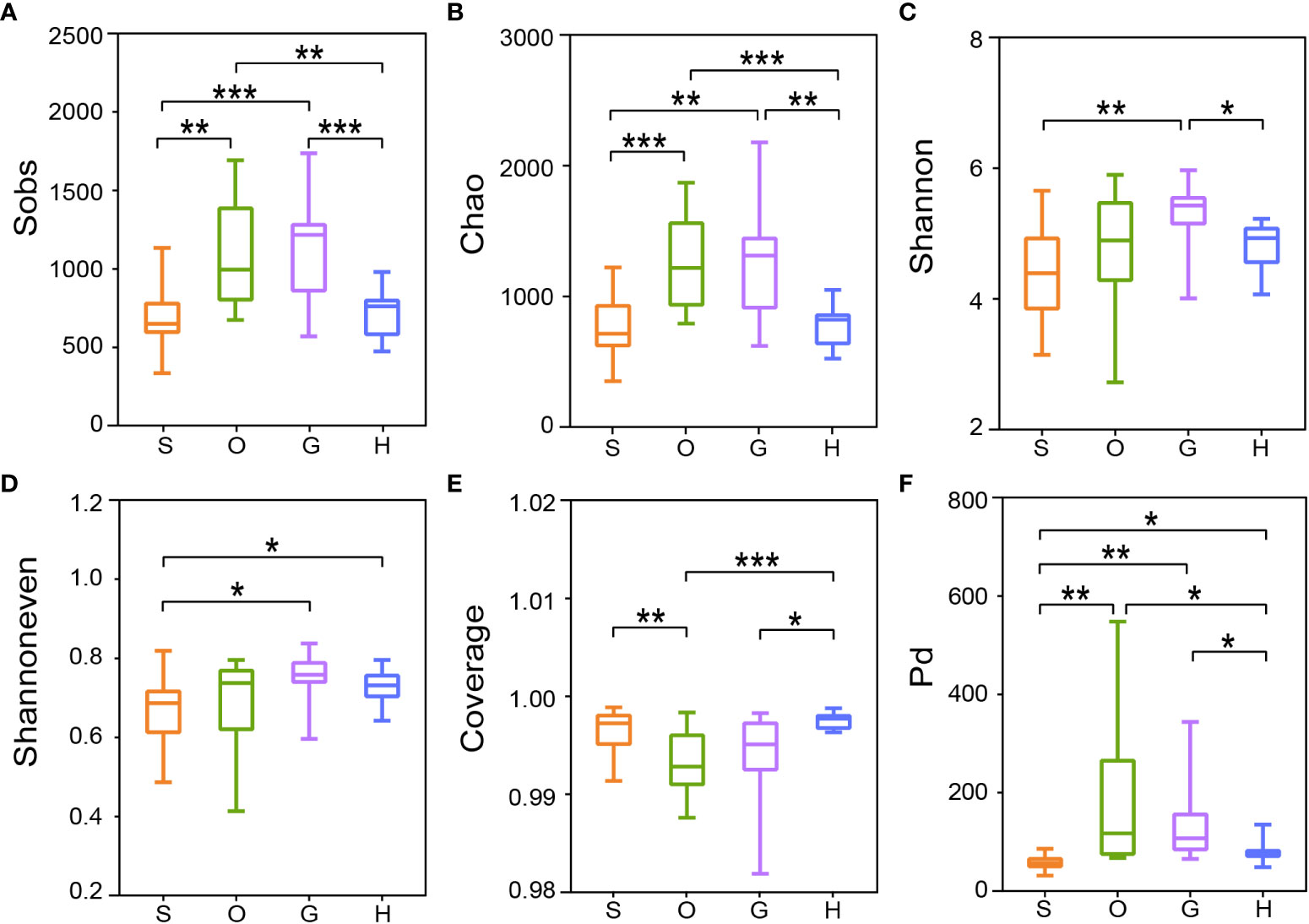
Figure 1 Alpha diversity of bacterial communities in four body sites of tunas. The statistical significance of six alpha diversity indices of (A) Sobs index, (B) Chao index, (C) Shannon index, (D) Shannoneven index, (E) Coverage index, and (F) Pd index were calculated by the Welch’s t-test (*p ≤ 0.05; **p ≤ 0.01; ***p ≤ 0.001). S, skin; O, oral; G, gill; H: hindgut.
The results of PCoA analysis showed that the factor of body sites explained approximately 20% of difference of microbial structure: 20.35% for four body sites and 19.2% without hindgut (Figures 2A, B). According to Figure 2A, the cluster of hindgut samples was completely separated from the external samples. From Figure 2B, the overlap of confidence ellipse within skin and gill or oral was much less than that of gill and oral, and correlation heatmap showed highest Spearman correlation coefficient between gill and oral (Supplementary Figure 1), indicating the microbiome communities of the oral and gill were similar. The statistically significant differences were shown among all pairwise comparisons based on Bray-Curtis metrics and weighted Unifrac metrics, except oral vs gill for weighted Unifrac metrics (Figure 2D).
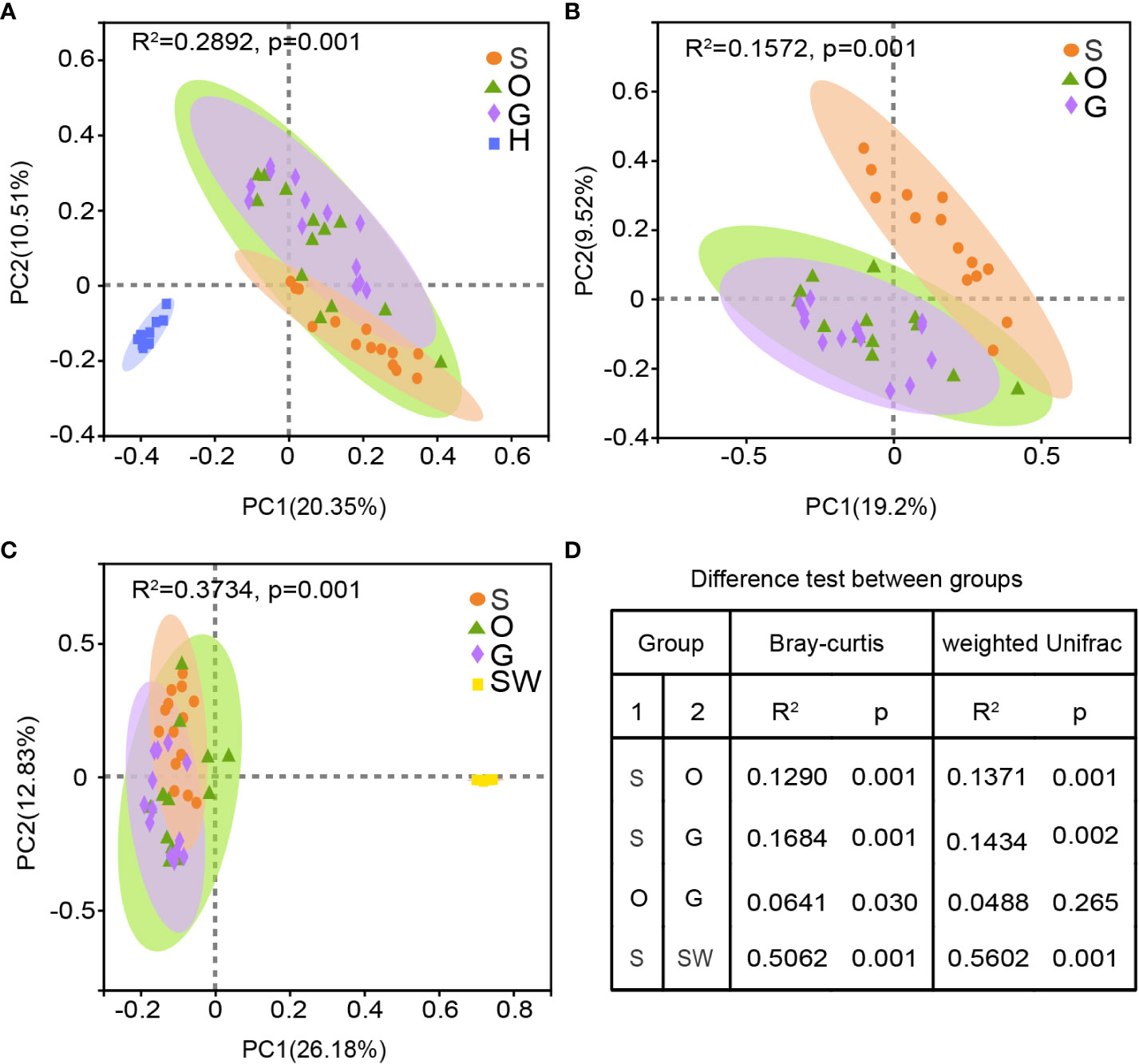
Figure 2 Beta diversity of bacterial communities in four body sites of tunas and seawater. (A) PCoA analysis of all microbiome across the external and hindgut. (B) PCoA analysis of external mucus microbiome. (C) PCoA analysis of external mucus microbiome and seawater. (D) Pairwise comparisons of beta diversity distances between groups are calculated by the Bray-Curtis metrics and weighted Unifrac metrics. S, skin; O, oral; G, gill; H, hindgut; SW, seawater.
Microbial diversity in external body sites showed highly taxonomic differentiation with seawater (Figure 2C), and host-associated microbiome showed weak correlation with environment microbiome (Supplementary Figure 1). According to similarity percentage analysis (SIMPER), hindgut had a highest average dissimilarity with seawater at the genus level (Supplementary Table 4). There were 46 species cumulatively contributed 60% dissimilarity in all groups, with Prochlorococcus MIT9313 contributed 21.19%~22.63% dissimilarity in each group.
Microbial relative abundance in different body sites
Bacteria belonging to 61 phyla were detected from all mucus-associated samples, including 44 from skin, 53 from oral, 50 from gill, and 48 from hindgut, respectively (Supplementary Figure 2A). There were 62% (38/61) of phyla shared in all body sites. Bacteria belonging to 5 phyla (i.e. Proteobacteria, Firmicutes, Actinobacteria, Bacteroidota, and Cyanobacteria) had high relative abundance and they account for about 90% of microbial composition in four groups (Figure 3A). The abundance of Proteobacteria in the oral was observed significantly higher than skin (Figure 3C. Kruskal-Wallis H Test, O vs S: p=0.0499), whereas Firmicutes in the oral was significantly lower (Kruskal-Wallis H Test, O vs S: p=0.0397; O vs H: p=0.0028). For Actinobacteria, no significant difference was found across all groups. Much fewer phyla (23) were identified from seawater compared with that from the host. Bacteria of Cyanobacteria and Proteobacteria had the highest relative abundance in seawater, making up more than 80% of the microbiome community (Figure 3A).
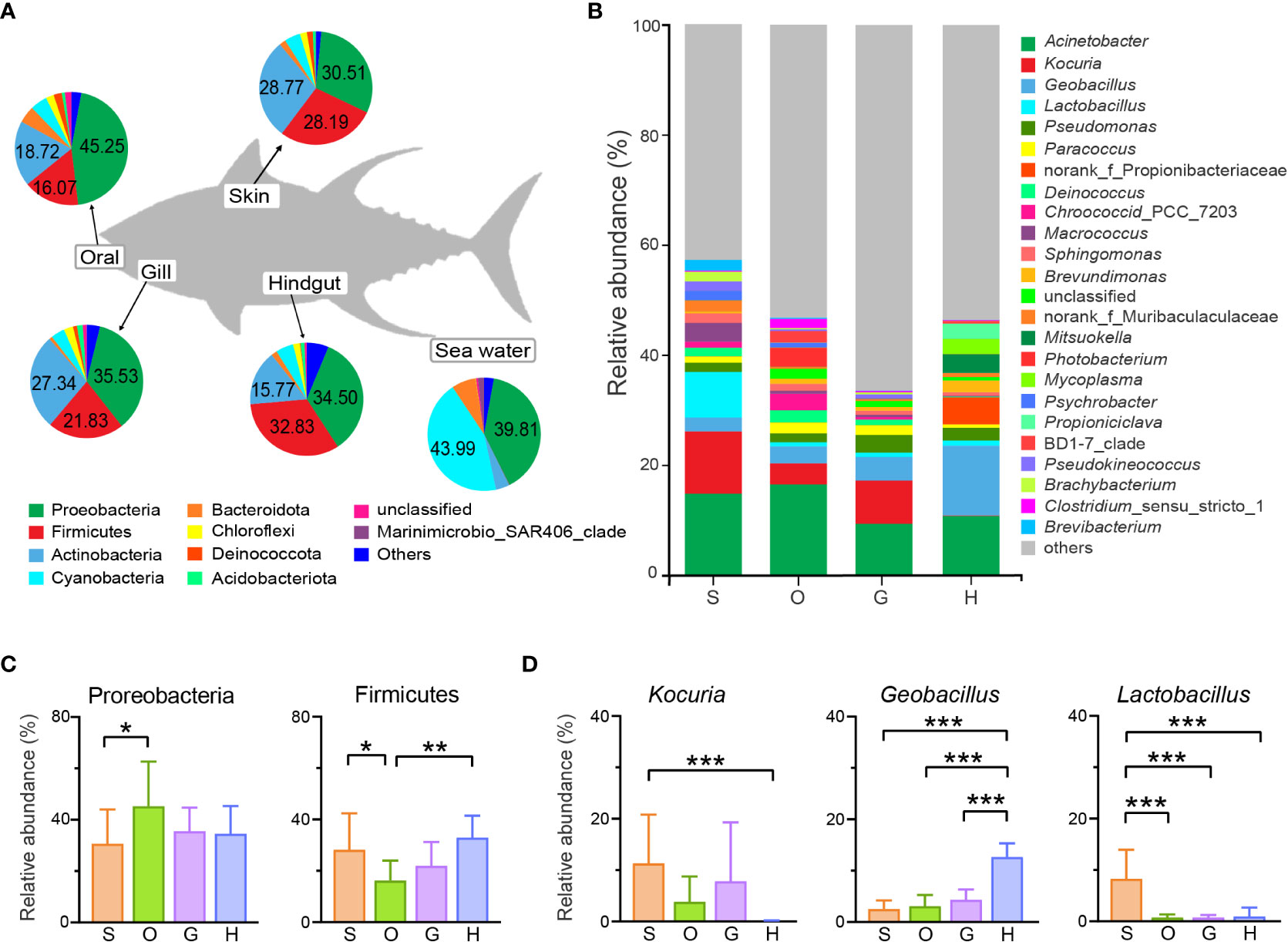
Figure 3 Relative abundance of the bacterial community. The microbial abundance of tunas and seawater at the (A) phylum level and (B) genus level. OTUs with low relative abundance (<1.5%) were clustered in “others”. The significant differences analysis of (C) abundant phyla and (D) abundant genera. Statistical test was carried out by the Kruskal-Wallis H Test followed by Bonferroni correction (*p ≤ 0.05; **p ≤ 0.01; ***p ≤ 0.001). S, skin; O, oral; G, gill; H, hindgut.
The number of genera ranged from 1053 to 1455 in each group, and the four groups shared 644 genera (Supplementary Figure 2B). Among the external group, three genera (i.e. Acinetobacter, Kocuria and Geobacillus) constituted 20.04%~28.56% of the surface mucus microbial community (Figure 3B). In the hindgut, the top 3 abundant genera were Geobacillus, Acinetobacter and g_norank_Propionibacteriaceae, accounting for 28.44% of the microbial community (Figure 3B). In addition, the most dominant genus Acinetobacter accounted for 9.44%~16.54% for each external group and was highest in oral. It showed no marked difference between the four groups. Kruskal-Wallis H test screened out 15 abundant genera showing significant difference in four groups (i.e. Kocuria, Geobacillus, Lactobacillus, Pseudomonas, Paracoccus, g_norank_f_Propionibacteriaceae, Deinococcus, Chroococcidiopsis_PCC_7203, Macrococcus, Sphingomonas, Brevundimonas, Knoellia, Supplementary Figure 3). Among them, Kocuria had the highest relative abundance in the skin (Kocuria: 11.32%) compared to that in the hindgut (Kocuria: 0.09%) (Figure 3D). Lactobacillus was one of the most abundant genera in the skin and accounted for 8.29% of the community, showing a significant difference from other groups (Figure 3D). The relative abundance of Geobacillus was significantly highest in the hindgut (H: 12.65%; S: 2.51%; O: 3.08%; G: 4.32%).
Core microbes and co-occurrence networks in each group
A total of 324 core OTUs (S: 115; O: 132; G: 176; H: 140) were presented in at least 70% replicates of mucus-associated samples. The relative abundance in each group was shown in Supplementary Figure 4. In each group, they composed only 2.46%~4.13% of OTUs but covered 55.20%~71.58% of the sequence reads. Proteobacteria (117 OTUs), Firmicutes (91 OTUs), Actinobacteria (66 OTUs), and Bacteroidota (21 OTUs) were the four most abundant phyla within the core taxa. Among the 324 core OTUs, 13 OTUs were present in all replicates. They represented only 0.13% of all OTUs but covered 18.66% of all the sequence reads. They were Acinetobacter (OTU6807, OTU6806, OTU7089, OTU6848), Pseudomonas (OTU7873, OTU1419), Geobacillus (OTU6817), Knoellia (OTU7006), Microbacterium (OTU7122), Escherichia-Shigella (OTU6820), Brevundimonas (OTU7071), Staphylococcus (OTU6907), and unclassified OTU6955 (Supplementary Table 5).
The co-occurrence network of core OTUs in each group was analyzed at the genus level (Figure 4). Different nodes and edges numbers presented significant differences in four groups: 37 and 56 in skin, 40 and 71 in oral, 38 and 61 in gill, 33 and 31 in hindgut. Generally, the positive edges of the network were much more than the negative edges in each group. The ratio of negative correlations was the lowest in oral (5.63%) and highest in hindgut (25.81%), indicating more competition relationships within the hindgut microbial community. For the most abundant core OTUs at the genus level, in the skin, Lactobacillus had a positive relationship with Streptococcus and Pediococcus, and Kocuria was in positive correlation with Microbacterium (Figure 4A). In the oral, Kocuria was in a negative correlation with Acinetobacter, whereas Geobacillus was in a positive relationship with others, including Lactobacillus (Figure 4B). Kocuria in the gill was positively related to many Actinobacteria species (such as Knoellia, Pseudokineococcus, Kytococcus, etc.), and they were negatively related to other phylum species (Figure 4C). Moreover, Acinetobacter had a positive relationship with Geobacillus. In the hindgut, Acinetobacter showed a negative correlation with Mitsuokella, which was also in high abundance (3.42%), and Geobacillus had a positive correlation with Massilia (Figure 4D).
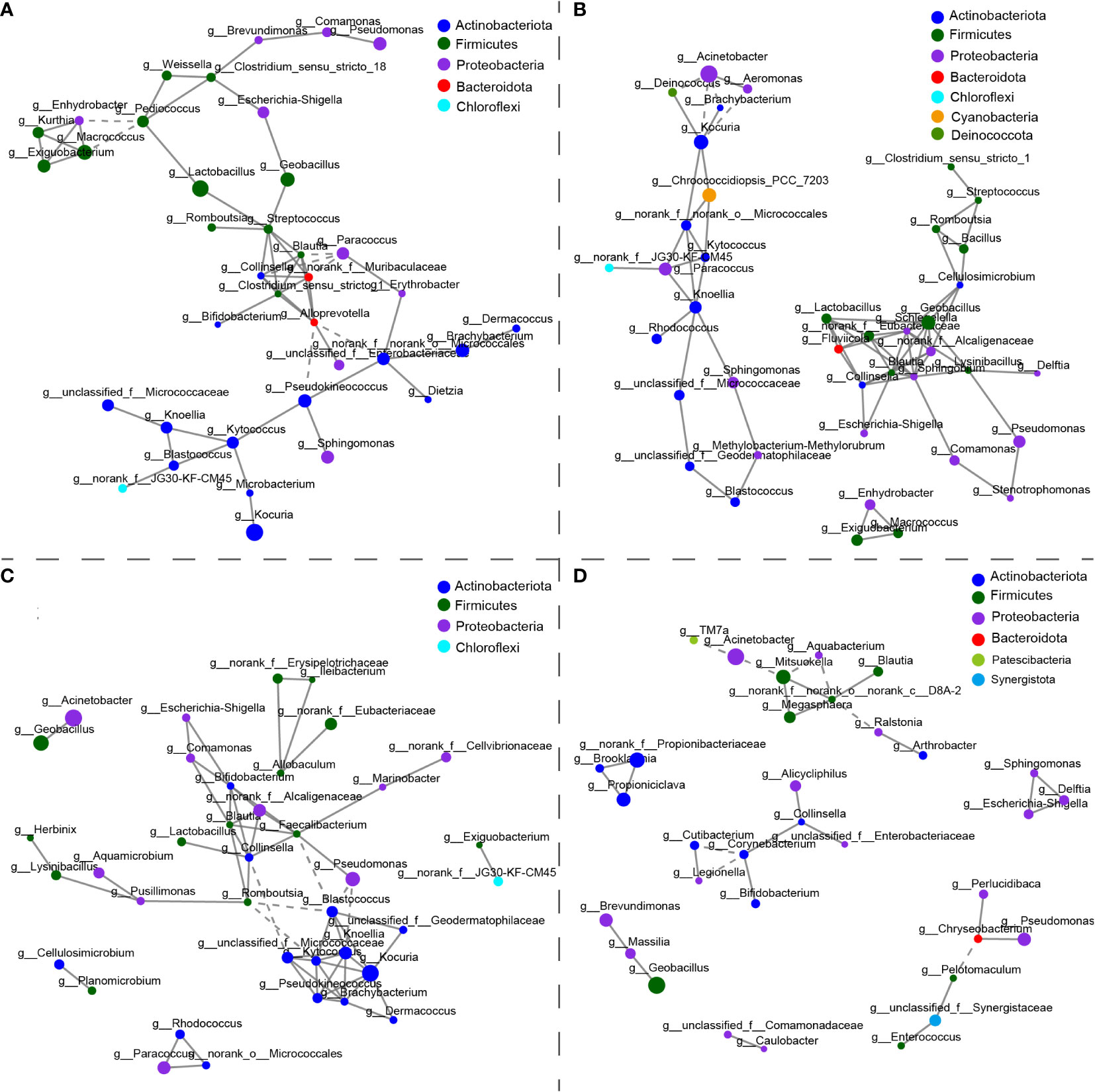
Figure 4 The co-occurrence networks of core microbiotas in the (A) skin, (B) oral, (C) gill, and (D) hindgut. The size of the node shows the relative abundance at the genus level. Node color represents taxonomic classification at the phylum level. Positive interactions are depicted in the solid line and negative interactions in the dashed line. The patterns of networks were measured by Spearman’s correlation coefficient (r>0.75, p<0.05).
Biomarkers in each group
Biomarkers were discovered in four different groups (Figure 5). The phylogenetic distribution of microbial communities in different groups was shown in Figure 5A, and 7 phyla clades contained at least one biomarker, i.e. Proteobacteria, Firmicutes, Bacteroidota, Cyanobacteria, Actinobacteria, and Synergistota.
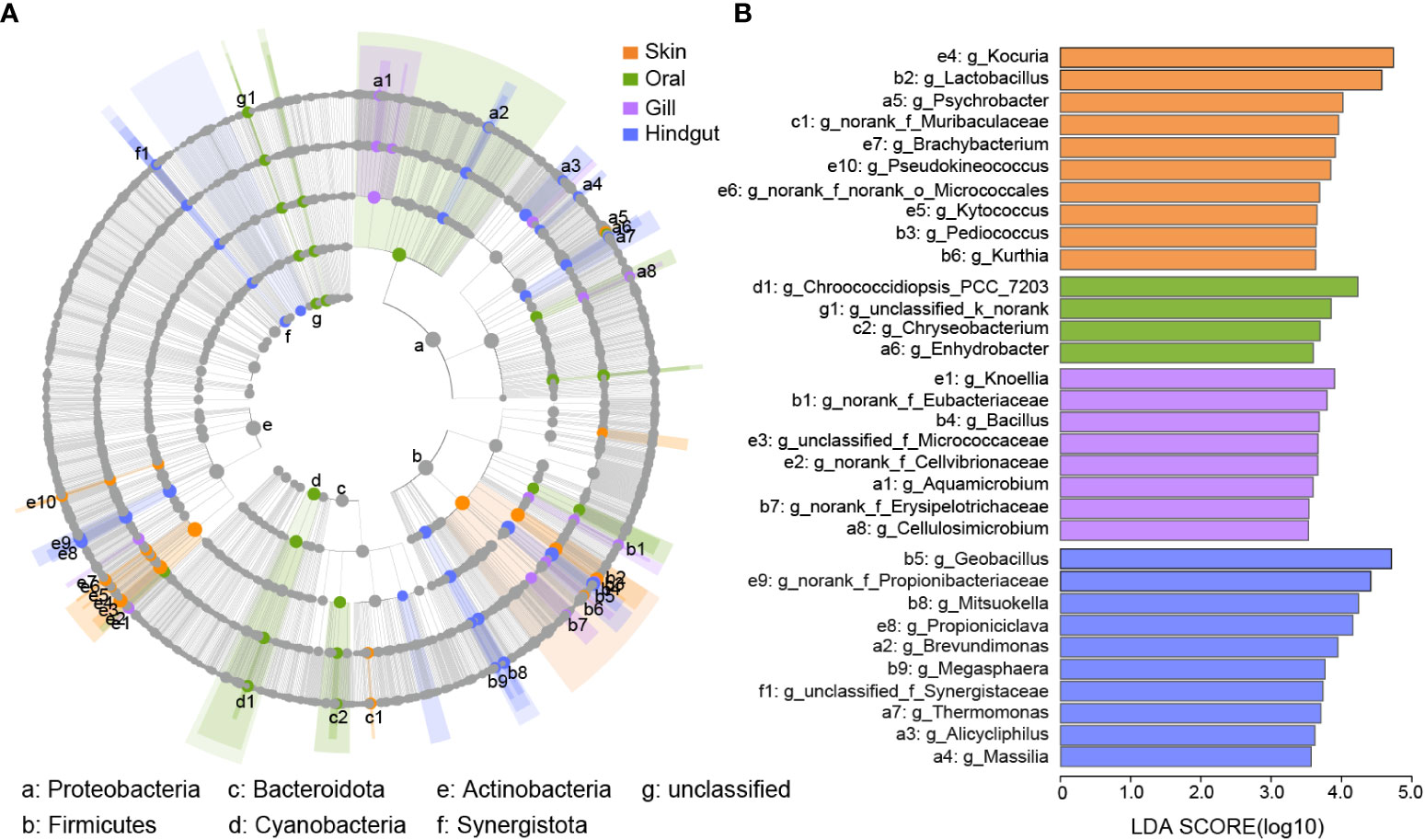
Figure 5 Biomarkers in four body sites. (A) Cladogram of the phylogenetic distribution of the bacterial lineages from phylum to the genus in the skin, oral, gill, and hindgut. The diameter of each dot repents the relative abundance. The species with a significant difference were represented by different colors among the four groups. The grey nodes indicate that there was no significant difference. (B) Bar plots showed the biomarkers from (A) with an LDA ≥ 3.5.
From Figure 5B and Supplementary Table 6, there were 10 biomarkers were identified in the skin, mainly belonging to the phyla of Firmicutes and Actinobacteria. Among them, Kocuria and Lactobacillus were abundant biomarkers. The abundance of other biomarkers was low, for example, Psychrobacter (1.78%), Brachybacterium (1.75%), Pseudokineococcus (1.65%) (Supplementary Table 6). In the oral, there were only 4 biomarkers from different phyla. Biomarkers in the gill belong to Proteobacteria, Firmicutes, and Actinobacteria, and they had low relative abundance, such as Knoellia (1.49%), Bacillus (1.12%), and Aquamicrobium (0.84%). Geobacillus was specifically enriched in the hindgut, as well as the Mitsuokella, Propioniciclava, and Brevundimonas. They had a relative abundance of more than 2%.
Microbial function prediction
According to function analysis, the top 5 most abundant classifications among 24 COGs were “Amino acid transport and metabolism”, “Translation, ribosomal structure and biogenesis”, “Energy production and conversion”, “Inorganic ion transport and metabolism”, and “Transcription” (Supplementary Figure 5). Thirteen COGs showed significant differences in the four groups (Figure 6A). COGs of L (Replication, recombination and repair) and F (Nucleotide transport and metabolism) had a significantly highest relative abundance in the skin, and T (Signal transduction and metabolism) and N (Cell motility) were significantly lowest. In the hindgut, COGs of M (Cell wall/membrane/envelope biogenesis) and U (Intracellular trafficking, secretion, and vesicular transport) had higher relative abundance than that in gill and skin, and lower in K (Transcription) compared to gill and skin. COG of O (Posttranslational modification, protein turnover, chaperones) had a higher relative abundance in the oral than skin or gill. COG of E (Amino acid transport and metabolism) had a higher relative abundance in gill than skin.
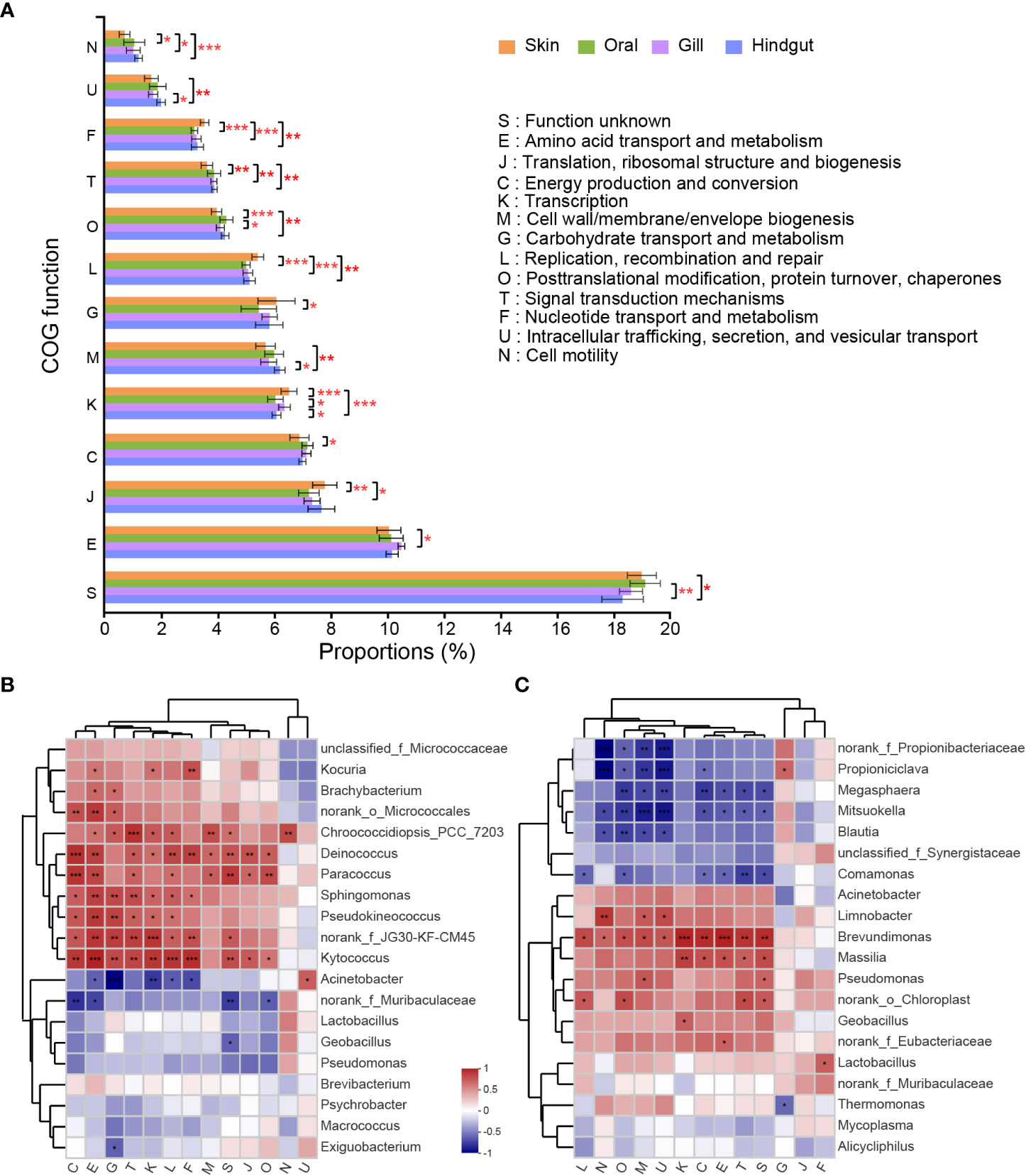
Figure 6 COG function classifications with a significant difference in the four groups and its correlation with high abundant species. (A) Statistical test of the COGs relative abundance was carried out by the Kruskal-Wallis H Test followed by Bonferroni correction. Heatmap for correlation between COG function classifications and top 20 high abundant species in the (B) skin and (C) hindgut. Correlation coefficients were calculated by Spearman. (*p ≤ 0.05; **p ≤ 0.01; ***p ≤ 0.001).
The correlation heatmap showed COG functional enrichment was related to most microbial biomarkers in each group (Figures 6B, C). In the skin, Kocuria was significantly related to the COGs of E, K, and F. Biomarkers identified in Figure 5B, such as Brachybacterium, Pseudokineococcus, g_norank_f_norank_o_Micrococcales, and Kytococcus in the skin were proved to have a positive correlation in COGs of E, G and T (Figure 6B). In the hindgut, the biomarker Brevundimonas was positively related to COGs of M and U (Figure 6C). COG of Amino acid transport and metabolism was significantly positively correlated with Brevundimonas and Massilia. Carbohydrate transport and metabolism was significantly positively correlated with Propioniciclava, but negatively correlated with Thermomonas. Mitsuokella was negatively correlated with most COGs except for Carbohydrate transport and metabolism. In the oral, Chroococcidiopsis_PCC_7203 was positively related to COGs G, and an unclassified genus was negatively related to most COGs (Supplementary Figure 6A). Bacillus in the gill was positively related to COGs of N, M and U (Supplementary Figure 6B).
Discussion
Microbial community structure varied in different body sites
Mucus-associated microbial communities were subjected to host-associated selection factors (Pratte et al., 2018). Body sites was proved as the dominant factor of microbial community diversity in our study, but no significant difference was found in the microbial structure from different body size or species. The high convergence of microbiome in yellowfin tuna and bigeye tuna may be caused by the process of “phylosymbiosis”, since the two tuna species are in a close phylogenetical relationship and host species with close phylogenetic relationships have more similar microbiotas (Brooks et al., 2016). It is worth noting that sample size of bigeye tuna was limited in this study. It could be a larger variation between microbiomes from yellowfin and bigeye tunas when the sample size is larger. In addition, an overlap in the ecological niche of the two tunas might be the other primary cause of their similarity in bacterial composition.
The marked microbial heterogeneity was obviously reflected in external and internal sites. It suggested different bacterial assemblages and microbial niches differentiation at the organ scale, further providing insight into how microbiome adapt to the host (Chiarello et al., 2015; Zhang et al., 2019; Sylvain et al., 2020). The different microenvironments among body sites were proposed as the leading cause of microbial heterogeneity (Chiarello et al., 2015). For surficial microorganisms, they are more susceptible to environmental influence or disturbance (Chiarello et al., 2015). While the gut microecosystem has relatively stable pH and temperature to keep homeostasis, promoting the specialized and modular populations to colonize (Ross et al., 2019; Sylvain et al., 2020). Furthermore, unlike most poikilothermic fish, tuna represents a regional warm-blood fish with a stable celiac temperature at 25~28°C and approximately 10°C difference from the external surface (Block et al., 2001). The relatively stable temperature in the celiac area may allow tuna to selectively recruit and assemble organ-specific symbionts for long-term co-evolution. It is necessary to point out that the microbiome of hindgut in this paper was from the content of the hindgut instead of its surface mucus and there might be difference of biodiversity and abundance of microbiota between gut mucus layer and the content (Kashinskaya et al., 2017). The aim in this study is to compare the mucus-associated microbiota from different body sites in tuna. We believe that the content of the hindgut can reflect the mucus-associated microbiota from hindgut, since sampling of feces and intestinal contents has been widely used in the study on gut mucosal microbiome, including the tuna (Minich et al., 2020a; Minich et al., 2020b). On the other hand, to make the description more clearly and let the comparison more reasonable, it would be better to pay attention to the difference of microbiome from gut mucus and content, and process the related results carefully in the future work.
Among external surface groups, the alpha diversity in the gill was significantly higher than that of skin, which was consistent with the cultured southern bluefin tunas (Minich et al., 2020a). However, it was the skin that possessed a higher Shannon index than gill in other studies on Pacific chub mackerel (Minich et al., 2020b), seabass and seabream (Rosado et al., 2019), grass carp and southern catfish (Zhang et al., 2019). The inconsistent results between tunas and other fish may be related to host habits and physiological characteristics. As we all know, tuna is remarkable in swimming performance and high speed. As the skin of tuna lacks scales, it would be heavily scoured by the water current to the disadvantage of bacterial colonization. Whereas, the gill is in a semi-enclosed space and the gill filaments are interlamellar fusional to relieve the impact of water flow (Evans et al., 2005).
The oral, primarily responsible for energy intake, was similar to the gill in community composition and diversity, probably because they are spatially connected. The environment of the host oral is complex, and the microbes that live here are closely related to diet and environmental parameters (Abdelhafiz et al., 2021). However, the related studies on fish oral microbiotas are limited. More work needs to be done to investigate the relationship between the diet and the microbiome community in the oral.
Microbial discrepancy between the external surface and the environment
The external surface of fish touched with seawater directly, but the bacterial diversity and components of surrounding seawater were significantly lower than that of the external surface. The same cases had been reported previously (Minniti et al., 2017; Sylvain et al., 2020; Steiner et al., 2021; McMurtrie et al., 2022). The high discrepancy between host-associated and environmental microbiotas suggests that fish surface microbial communities are not simple reflections of the microbial assemblages in their habitat. Heterotrophic bacteria were scarce in the water than on the external surface of fish, due to the oligotrophic nutrients in the ocean (Larsen et al., 2013). By contrast, fish body is taken as a eutrophic “island”, as there are multitudinous components secreted by mucosal layer cells, such as mucins, gel-forming glycoproteins and glycosaminoglycans (Chiarello et al., 2015; Ross et al., 2019). The higher bacterial diversity on the external surface reflects the host selection effect from the surrounding “bacterial pool” (Chiarello et al., 2015). Therefore, the quality of the aquaculture water system is crucial to the individual health of farmed fish.
Core species have a vital status and interaction in microbial community
Core microbiotas play a critical role in the formation of symbiotic communities, and many studies aimed to reduce the complexity of host-associated and determine the correlation between the core microbiome and host health (Dong et al., 2021). The four groups shared a part of core taxa including highly abundant phyla of Proteobacteria, Firmicutes, and Actinobacteria, which were also detected in farmed tuna (Minich et al., 2020a) and most teleost species (Wilson et al., 2008; Chiarello et al., 2015). At the genus level, Acinetobacter spp. were universally dominant in all body sites of wild tuna in this paper, as well as farmed southern bluefin tuna (Minich et al., 2020a), indicating that mucus of tuna is one of their natural niches. A previous study confirmed that the probiotic Acinetobacter strain (Acinetobacter KU011TH) isolated from the skin mucus of bighead catfish can significantly improve growth performance (Bunnoy et al., 2019).
The core taxa can affect the community structure by their high abundance or strong biological interaction with other species (Agler et al., 2016; Dong et al., 2021). Moreover, the dominant species may influence selection pressure on other resident microbial strains (Ferreiro et al., 2018). In the gut, 25.81% of relationships were defined as negative, such as Acinetobacter and Mitsuokella, which demonstrated potential competition. As described in the “Red Queen hypothesis”, competitions could accelerate microbial evolution in the microbial ecosystem, resulting in apparent stability of microecosystem (Ferreiro et al., 2018). Additionally, the Firmicutes species Lactobacillus and Streptococcus are both lactic acid bacteria (LAB), showing commensal interaction in the skin co-occurrence network. They are helpful in resisting aquatic pathogen colonization by producing inhibitory compounds and competing for nutrients (Pérez-Sánchez et al., 2011). Whereas, Pseudoalteromonas, Psychrobacter, and Vibrio were prominent in the skin and gill of the diseased tuna but lack of LAB (Minich et al., 2020a). Geobacillus spp. are important probiotics beneficial to the host’s digestion and absorption of nutrients (Miao et al., 2018). They were found significantly enriched in gut of wild tuna in this paper, and in a positive relationship with the colonization of Massilia, which was proposed to contribute to fish development (Califano et al., 2017; Fujimoto et al., 2020). This commensal relationship implied the co-evolution of their ecological niche and performed the parallel function for tunas’ health.
From the core microbial networks, some taxa were not dominant in abundance, but have a close relationship with others, such as Alloprevotella and Streptococcus in the skin and g_norank_c_DBA-2, Corynebacterium in the gut. They might be instrumental in stabilizing and regulating the community, as similar conditions were reported in a previous paper (Jousset et al., 2017; Dong et al., 2021). For the dominant core taxa, take Acinetobacter, Kocuria and Geobacillus for example, they had more simple interactions with others. These taxa were stable in population dynamics and played a vital role in the structure of host-associated microbial networks (Dong et al., 2021). Therefore, all the core microbiomes, no matter with high abundance or low, have an essential role in maintaining the relative stability of the community and more studies need to be done to further determine their functions.
Microbial biomarkers provide insights into the microecological function
The microbes on the external surface of fish are high environment-dependent. Some of them commonly act as indicator species when the host homeostasis or the surrounding environment is perturbed (Sylvain et al., 2020). Based on our result, the abundant Lactobacillus in the skin could be considered the best biomarker candidate for tuna to monitor the host health status and environmental quality. It has been also proposed as a biomarker for toxicogenic exposure (Spilsbury et al., 2022).
Host microbiotas are in highly complex interactions, and function prediction can help us to better understand their roles (Hicks et al., 2021). In the gut, several microbial biomarkers, such as Propioniciclava, Mitsuokella, Massilia, and Brevundimonas, were positively linked with functions related to the metabolism of nutrients and nucleotides. They were also reported to have similar functions or the other metabolism: Propioniciclava is propionate-producing bacteria, and was remarkably increased in presence in the seabream gut after intaking a high level of protein (Solé-Jiménez et al., 2021); Mitsuokella was related to carbohydrate metabolism (Tsukahara et al., 2002) and amino acid metabolism (Dai et al., 2010), and Mitsuokella multiacida can use lactate and acetate to form butyrate, which is an important SCFA in the intestine (Tsukahara et al., 2002); Massilia could be promoted by a commercial probiotic Pediococcus acidilactici, resulting in synergistic roles (Rasmussen et al., 2022). Although Lactobacillus is not abundant in the gut, it was positively related to energy metabolism. It was helpful for probiotics to increase the host metabolic level and obtain enough nutrients to meet the energy requirement of high-performance fish (Rasmussen et al., 2022). Brevundimonas was detected as a biomarker in the gut and is a potential pathogen in most situations (Minich et al., 2020a), indicating a complex co-evolution between commensal symbionts, pathogens, and host. It is essential to further work on the co-evolution of symbiotic and pathogenic bacteria.
Conclusion
The diversity and composition of microbial communities in the four different body sites (skin, oral, gill, and gut) of wild tunas were reported in this paper. They varied among body sites instead of species or body size. Proteobacteria, Actinobacteria, and Firmicutes were the dominant phyla in all body sites. Some abundant species Acinetobacter, Lactobacillus, and Geobacillus were in tight interaction with other probiotic species identified from core taxa, and may contribute to host nutrition and immunity. Tightly connected core microbiotas may promote the stability of the microbial community. Biomarkers are predicted in the four body sites. The skin biomarker Lactobacillus could be used in environmental disturbance monitoring. Gut biomarkers were shown to be closely related to the metabolism functions. The results of this study highlight the importance of symbiotic microorganisms for host health, especially for cultured tuna in the process of aquaculture.
Data availability statement
The datasets presented in this study can be found in online repositories. The names of the repository/repositories and accession number(s) can be found below: https://www.ncbi.nlm.nih.gov/, BioProject ID: PRJNA884520; https://www.ncbi.nlm.nih.gov/, BioProject ID: PRJNA902642.
Ethics statement
Ethical review and approval was not required for the animal study because all tunas were harvested by private company and as part of commerce.
Author contributions
YZ: sampling, data processing and analysis, drafted the manuscript. DW: sampling and data analysis. LW and JX: writing-review and editing, pre-processing of the samples. PZ and HH: sampling and pre-processing of the samples. YJZ and ZG: research design, manuscript editing. All authors discussed and approved the final version of the manuscript.
Funding
This work was supported by the Opening Foundation of Hainan Tropical Ocean University & Yazhouwan Innovation Research Institute (2022RHDKFKT03) and the Modern Agricultural Industry Technology System of Grouper in Hainan Province (HNARS2022-3-G4 & HNARS2022-3).
Acknowledgments
The authors would like to thank Shuai Pan and Anfu Sun at Hainan University for their generous help in the sample collection.
Conflict of interest
The authors declare that the research was conducted in the absence of any commercial or financial relationships that could be construed as a potential conflict of interest.
Publisher’s note
All claims expressed in this article are solely those of the authors and do not necessarily represent those of their affiliated organizations, or those of the publisher, the editors and the reviewers. Any product that may be evaluated in this article, or claim that may be made by its manufacturer, is not guaranteed or endorsed by the publisher.
Supplementary material
The Supplementary Material for this article can be found online at: https://www.frontiersin.org/articles/10.3389/fmars.2022.1073264/full#supplementary-material
References
FAO (2020). “The state of world fisheries and aquaculture 2020,” in Sustainability in action Rome: Food and Agriculture Organization of the United Nations. doi: 10.4060/ca9229en
Abdelhafiz Y., Fernandes J. M. O., Stefani E., Albanese D., Donati C., Kiron V. (2021). Power play of commensal bacteria in the buccal cavity of female Nile tilapia. Front. Microbiol. 12. doi: 10.3389/fmicb.2021.773351
Agler M. T., Ruhe J., Kroll S., Morhenn C., Kim S. T., Weigel D., et al. (2016). Microbial hub taxa link host and abiotic factors to plant microbiome variation. PloS Biol. 14, e1002352. doi: 10.1371/journal.pbio.1002352
Banerjee G., Ray A. K. (2017). Bacterial symbiosis in the fish gut and its role in health and metabolism. Symbiosis 72 (1), 1–11. doi: 10.1007/s13199-016-0441-8
Block B. A., Dewar H., Blackwell S. B., Williams T. D., Prince E. D., Farwell C. J., et al. (2001). Migratory movements, depth preferences, and thermal biology of Atlantic bluefin tuna. Science 293 (5533), 1310–1314. doi: 10.1126/science.1061197
Brooks A. W., Kohl K. D., Brucker R. M., van Opstal E. J., Bordenstein S. R. (2016). Phylosymbiosis: relationships and functional effects of microbial communities across host evolutionary history. Plos. Biol. 14 (11), e2000225. doi: 10.1371/journal.pbio.2000225
Buffie C. G., Pamer E. G. (2013). Microbiota-mediated colonization resistance against intestinal pathogens. Nat. Rev. Immunol. 13, 790–801. doi: 10.1038/nri3535
Bunnoy A., Na-Nakorn U., Srisapoome P. (2019). Probiotic effects of a novel strain, Acinetobacter KU011TH, on the growth performance, immune responses, and resistance against Aeromonas hydrophila of bighead catfish (Clarias macrocephalus Gunther 1864). Microorganisms 7 (12), 613. doi: 10.3390/microorganisms7120613
Califano G., Castanho S., Soares F., Ribeiro L., Cox C. J., Mata L., et al. (2017). Molecular taxonomic profiling of bacterial communities in a gilthead seabream (Sparus aurata) hatchery. Front. Microbiol. 8. doi: 10.3389/fmicb.2017.00204
Carda-Dieguez M., Ghai R., Rodriguez-Valera F., Amaro C. (2017). Wild eel microbiome reveals that skin mucus of fish could be a natural niche for aquatic mucosal pathogen evolution. Microbiome 5, 162. doi: 10.1186/s40168-017-0376-1
Chen S. F., Zhou Y. Q., Chen Y. R., Gu J. (2018). Fastp: an ultra-fast all-in-one FASTQ preprocessor. Bioinformatic 34 (17), 884–890. doi: 10.1093/bioinformatics/bty560
Chiarello M., Villéger S., Bouvier C., Bettarel Y., Bouvier T. (2015). High diversity of skin-associated bacterial communities of marine fishes is promoted by their high variability among body parts, individuals and species. FEMS Microbiol. Ecol. 91 (7), fiv061. doi: 10.1093/femsec/fiv061
Dai Z. L., Zhang J., Wu G. Y., Zhu W. Y. (2010). Utilization of amino acids by bacteria from the pig small intestine. Amino Acids 39 (5), 1201–1215. doi: 10.1007/s00726-010-0556-9
Dehler C. E., Secombes C. J., Martin S. A. M. (2017). Environmental and physiological factors shape the gut microbiota of Atlantic salmon parr (Salmo salar l.). Aquaculture 467, 149–157. doi: 10.1016/j.aquaculture.2016.07.017
Di Domizio J., Belkhodja C., Chenuet P., Fries A., Murray T., Mondejar P. M., et al. (2020). The commensal skin microbiota triggers type I IFN-dependent innate repair responses in injured skin. Nat. Immunol. 21 (9), 1034–1045. doi: 10.1038/s41590-020-0721-6
Dong C. B., Shao Q. Y., Zhang Q. Q., Yao T., Huang J. Z., Liang Z. Q., et al. (2021). Preferences for core microbiome composition and function by different definition methods: Evidence for the core microbiome of Eucommia ulmoides bark. Sci. Total. Environ. 790, 148091. doi: 10.1016/j.scitotenv.2021.148091
Douglas G. M., Maffei V. J., Zaneveld J. R., Yurgel S. N., Brown J. R., Taylor C. M., et al. (2020). PICRUSt2 for prediction of metagenome functions. Nat. Biotechnol. 38 (6), 685–688. doi: 10.1038/s41587-020-0548-6
Edgar R. C. (2013). UPARSE: highly accurate OTU sequences from microbial amplicon reads. Nat. Methods 10 (10), 996–998. doi: 10.1038/nmeth.2604
Evans D. H., Piermarini P. M., Choe K. P. (2005). The multifunctional fish gill: dominant site of gas exchange, osmoregulation, acid-base regulation, and excretion of nitrogenous waste. Physiol. Rev. 85 (1), 97–177. doi: 10.1152/physrev.00050.2003
Fassarella M., Blaak E. E., Penders J., Nauta A., Smidt H., Zoetendal E. G. (2021). Gut microbiome stability and resilience: elucidating the response to perturbations in order to modulate gut health. Gut 70 (3), 595–605. doi: 10.1136/gutjnl-2020-321747
Ferreiro A., Crook N., Gasparrini A. J., Dantas G. (2018). Multiscale evolutionary dynamics of host-associated microbiomes. Cell 172 (6), 1216–1227. doi: 10.1016/j.cell.2018.02.015
Foster K. R., Schluter J., Coyte K. Z., Rakoff-Nahoum S. (2017). The evolution of the host microbiome as an ecosystem on a leash. Nature 548 (7665), 43–51. doi: 10.1038/nature23292
Fujimoto M., Marsh T. L., Scribner K. T. (2020). Effects of water filtration and temperature on microbial colonization and survival of lake sturgeon eggs. N. Am. J. Aquacult. 83 (1), 26–37. doi: 10.1002/naaq.10169
Gardner S. G., Camp E. F., Smith D. J., Kahlke T., Osman E. O., Gendron G., et al. (2019). Coral microbiome diversity reflects mass coral bleaching susceptibility during the 2016 El niño heat wave. Ecol. Evol. 9, 938–956. doi: 10.1002/ece3.4662
Hagberg A. A., Schult D. A., Swart P. J. (2008). “Exploring network structure, dynamics, and function using networkx,” in Proceedings of the 7th Python in science conference. SciPy 2008: 7th Python in Science Conference. (Pasadena, California, USA), 11–15. Available at: https://www.osti.gov/biblio/960616.
Hicks L. C., Frey B., Kjoller R., Lukac M., Moora M., Weedon J. T., et al. (2021). Toward a function-first framework to make soil microbial ecology predictive. Ecology 103 (2), e03594. doi: 10.1002/ecy.3594
Jacobson A., Lam L., Rajendram M., Tamburini F., Honeycutt J., Pham T., et al. (2018). A gut commensal-produced metabolite mediates colonization resistance to Salmonella infection. Cell Host Microbe 24 (2), 296–307. doi: 10.1016/j.chom.2018.07.002
Jousset A., Bienhold C., Chatzinotas A., Gallien L., Gobet A., Kurm V., et al. (2017). Where less may be more: how the rare biosphere pulls ecosystems strings. ISME J. 11 (4), 853–862. doi: 10.1038/ismej.2016.174
Karlsen C., Ottem K. F., Brevik O. J., Davey M., Sorum H., Winther-Larsen H. C. (2017). The environmental and host-associated bacterial microbiota of Arctic seawater-farmed Atlantic salmon with ulcerative disorders. J. Fish Dis. 40 (11), 1645–1663. doi: 10.1111/jfd.12632
Kashinskaya E. N., Andree K. B., Simonov E. P., Solovyev M. M. (2017). DNA extraction protocols may influence biodiversity detected in the intestinal microbiome: a case study from wild Prussian carp, Carassius gibelio. 2016. FEMS Microbiol. Ecol 93 (2). doi: 10.1093/femsec/fiw240
Larsen A., Tao Z., Bullard S. A., Arias C. R. (2013). Diversity of the skin microbiota of fishes: evidence for host species specificity. FEMS Microbiol. Ecol. 85 (3), 483–494. doi: 10.1111/1574-6941.12136
Liu J. H., Zhang M. L., Zhang R. Y., Zhu W. Y., Mao S. Y. (2016). Comparative studies of the composition of bacterial microbiota associated with the ruminal content, ruminal epithelium and in the faeces of lactating dairy cows. Microb. Biotechnol. 9 (2), 257–268. doi: 10.1111/1751-7915.12345
Lynch J. B., Hsiao E. Y. (2019). Microbiomes as sources of emergent host phenotypes. Science 365 (6460), 1405–1408. doi: 10.1126/science.aay0240
Magoč T., Salzberg S. L. (2011). FLASH: fast length adjustment of short reads to improve genome assemblies. Bioinformatics 27 (21), 2957–2963. doi: 10.1093/bioinformatics/btr507
McMurtrie J., Alathari S., Chaput D. L., Bass D., Ghambi C., Nagoli J., et al. (2022). Relationships between pond water and tilapia skin microbiomes in aquaculture ponds in Malawi. Aquaculture 558, 738367. doi: 10.1016/j.aquaculture.2022.738367
Miao S. Y., Zhu J. Y., Zhao C. Z., Sun L. S., Dong X. J., Chen G. H. (2018). Effect of dietary soybean meal associated with feeding time on the growth performance and intestinal microbiota composition of northern snakehead. Aquac. Res. 50 (10), 2751–2759. doi: 10.1111/are.14035
Minich J. J., Petrus S., Michael J. D., Michael T. P., Knight R., Allen E. E. (2020b). Temporal, environmental, and biological drivers of the mucosal microbiome in a wild marine fish, Scomber japonicus. mSphere 5 (3), e00401–e00420. doi: 10.1128/mSphere.00401-20
Minich J. J., Power C., Melanson M., Knight R., Webber C., Rough K., et al. (2020a). The southern bluefin tuna mucosal microbiome is influenced by husbandry method, net pen location, and anti-parasite treatment. Front. Microbiol. 11. doi: 10.3389/fmicb.2020.02015
Minniti G., Hagen L. H., Porcellato D., Jorgensen S. M., Pope P. B., Vaaje-Kolstad G. (2017). The skin-mucus microbial community of farmed Atlantic salmon (Salmo salar). Front. Microbiol. 8. doi: 10.3389/fmicb.2017.02043
Nowak B. F., Dang M., Webber C., Neumann L., Bridle A., Bermudez R., et al. (2021). Changes in the splenic melanomacrophage centre surface area in southern bluefin tuna (Thunnus maccoyii) are associated with blood fluke infections. Pathogens 10 (1), 9. doi: 10.3390/pathogens10010079
Ohshimo S., Hiraoka Y., Sato T., Nakatsuka S. (2018). Feeding habits of bigeye tuna (Thunnus obesus) in the north pacific from 2011 to 2013. Mar. Freshw. Res. 69 (4), 585–606. doi: 10.1071/MF17058
Pérez-Sánchez T., Balcázar J. L., Garcia Y., Halaihel N., Vendrell D., de Blas I., et al. (2011). Identification and characterization of lactic acid bacteria isolated from rainbow trout, Oncorhynchus mykiss (Walbaum), with inhibitory activity against Lactococcus garvieae. J. Fish. Dis. 34 (7), 499–507. doi: 10.1111/j.1365-2761.2011.01260.x
Pratte Z. A., Besson M., Hollman R. D., Stewart F. J. (2018). The gills of reef fish support a distinct microbiome influenced by host-specific factors. Appl. Environ. Microbiol. 84 (9), e00063–e00018. doi: 10.1128/AEM.00063-18
Rasmussen J. A., Villumsen K. R., Ernst M., Hansen M., Forberg T., Gopalakrishnan S., et al. (2022). A multi-omics approach unravels metagenomic and metabolic alterations of a probiotic and synbiotic additive in rainbow trout (Oncorhynchus mykiss). Microbiome 10 (1), 21. doi: 10.1186/s40168-021-01221-8
Rosado D., Pérez-Losada M., Severino R., Cable J., Xavier R. (2019). Characterization of the skin and gill microbiomes of the farmed seabass (Dicentrarchus labrax) and seabream (Sparus aurata). Aquaculture 500, 57–64. doi: 10.1016/j.aquaculture.2018.09.063
Rosenberg E., Zilber-Rosenberg I. (2018). The hologenome concept of evolution after 10 years. Microbiome 6, 78. doi: 10.1186/s40168-018-0457-9
Ross A. A., Hoffmann A. R., Neufeld J. D. (2019). The skin microbiome of vertebrates. Microbiome 7 (1), 79. doi: 10.1186/s40168-019-0694-6
Schloss P. D., Westcott S. L., Ryabin T., Hartmann M., Hollister E. B., Lesniewski R. A., et al. (2009). Introducing mothur: open-source, platform-independent, community-supported software for describing and comparing microbial communities. Appl. Environ. Microbiol. 75, 7537–7541. doi: 10.1128/AEM.01541-09
Segata N., Izard J., Waldron L., Gevers D., Miropolsky L., Garrett W. S., et al. (2011). Metagenomic biomarker discovery and explanation. Genome Biol. 12 (6), R60. doi: 10.1186/gb-2011-12-6-r60
Semova I., Carten J., Stombaugh J., Mackey L. C., Knight R., Farber S. A., et al. (2012). Microbiota regulate intestinal absorption and metabolism of fatty acids in the zebrafish. Cell Host Microbe 12 (3), 277–288. doi: 10.1016/j.chom.2012.08.003
Shetty S. A., Hugenholtz F., Lahti L., Smidt H., de Vos W. M. (2017). Intestinal microbiome landscaping: insight in community assemblage and implications for microbial modulation strategies. FEMS Microbiol. Rev. 41 (2), 182–199. doi: 10.1093/femsre/fuw045
Solé-Jiménez P., Naya-Català F., Piazzon M. C., Estensoro I., Calduch-Giner J.À., Sitjà-Bobadilla A., et al. (2021). Reshaping of gut microbiota in gilthead sea bream fed microbial and processed animal proteins as the main dietary protein source. Front. Mar. Sci. 8. doi: 10.3389/fmars.2021.705041
Spilsbury F., Foysal M. J., Tay A., Gagnon M. M. (2022). Gut microbiome as a potential biomarker in fish: dietary exposure to petroleum hydrocarbons and metals, metabolic functions and cytokine expression in juvenile Lates calcarifer. Front. Microbiol. 13. doi: 10.3389/fmicb.2022.827371
Spor A., Koren O., Ley R. (2011). Unravelling the effects of the environment and host genotype on the gut microbiome. Nat. Rev. Microbiol. 9 (4), 279. doi: 10.1038/nrmicro2540
Steiner K., Heasman K., Laroche O., Pochon X., Preece M., Bowman J. P., et al. (2021). The microbiome of Chinook salmon (Oncorhynchus tshawytscha) in a recirculation aquaculture system. Aquaculture 534, 736227. doi: 10.1016/j.aquaculture.2020.736227
Stressmann F. A., Bernal-Bayard J., Perez-Pascual D., Audrain B., Rendueles O., Briolat V., et al. (2020). Mining zebrafish microbiota reveals key community-level resistance against fish pathogen infection. ISME J. 15 (3), 702–719. doi: 10.1038/s41396-020-00807-8
Sultana S., Khan M. N., Hossain M. S., Dai J., Rahman M. S., Salimullah M. (2022). Community structure and functional annotations of the skin microbiome in healthy and diseased catfish, Heteropneustes fossilis. Front. Microbiol. 13. doi: 10.3389/fmicb.2022.856014
Sylvain F.-E., Holland A., Bouslama S., Audet-Gilbert E., Lavoie C., Val A. L., et al. (2020). Fish skin and gut microbiomes show contrasting signatures of host species and habitat. Appl. Environ. Microbiol. 86 (16), e00789–e00720. doi: 10.1128/AEM.00789-20
Tsukahara T., Koyama H., Okada M., Ushida K. (2002). Stimulation of butyrate production by gluconic acid in batch culture of pig cecal digesta and identification of butyrate-producing bacteria. J. Nutr. 132 (8), 2229–2234. doi: 10.1093/jn/132.8.2229
Varela J. L., Intriago K. M., Flores J. C., Lucas-Pilozo C. R. (2017). Feeding habits of juvenile yellowfin tuna (Thunnus albacares) in Ecuadorian waters assessed from stomach content and stable isotope analysis. Fish Res. 194, 89–98. doi: 10.1016/j.fishres.2017.05.017
Wang Q., Garrity G. M., Tiedje J. M., Cole J. R. (2007). Naive Bayesian classifier for rapid assignment of rRNA sequences into the new bacterial taxonomy. Appl. Environ. Microbiol. 73 (16), 5261–5267. doi: 10.1128/AEM.00062-07
Wang A. R., Ran C., Ringo E., Zhou Z. G. (2018). Progress in fish gastrointestinal microbiota research. Rev. Aquacult. 10 (3), 626–640. doi: 10.1111/raq.12191
Willmann M., Vehreschild M. J. G. T., Biehl L. M., Vogel W., Dorfel D., Hamprecht A., et al. (2019). Distinct impact of antibiotics on the gut microbiome and resistome: a longitudinal multicenter cohort study. BMC Biol. 17 (1), 76. doi: 10.1186/s12915-019-0692-y
Wilson B., Danilowicz B. S., Meijer W. G. (2008). The diversity of bacterial communities associated with Atlantic cod Gadus morhua. Microb. Ecol. 55 (3), 425–434. doi: 10.1007/s00248-007-9288-0
Keywords: Thunnus, mucosal microbiome, core taxa, biomarkers, 16S rRNA
Citation: Zou Y, Wu D, Wei L, Xiao J, Zhang P, Huang H, Zhang Y and Guo Z (2023) Mucus-associated microbiotas among different body sites of wild tuna from the South China Sea. Front. Mar. Sci. 9:1073264. doi: 10.3389/fmars.2022.1073264
Received: 18 October 2022; Accepted: 19 December 2022;
Published: 09 January 2023.
Edited by:
Senjie Lin, University of Connecticut, United StatesReviewed by:
Mikhail Solovyev, Institute of Systematics and Ecology of Animals (RAS), RussiaGuoxing Nie, Henan Normal University, China
Copyright © 2023 Zou, Wu, Wei, Xiao, Zhang, Huang, Zhang and Guo. This is an open-access article distributed under the terms of the Creative Commons Attribution License (CC BY). The use, distribution or reproduction in other forums is permitted, provided the original author(s) and the copyright owner(s) are credited and that the original publication in this journal is cited, in accordance with accepted academic practice. No use, distribution or reproduction is permitted which does not comply with these terms.
*Correspondence: Yanjie Zhang, yanjiezhang@hainanu.edu.cn; Zhiqiang Guo, guozq@hainanu.edu.cn