- 1Max Planck Institute for the Science of Human History, Jena, Germany
- 2Aquaculture and Nutrition Research Unit (ANRU) and Irish Seaweed Research Group, Ryan Institute, National University of Ireland Galway, Carna Research Station, Carna, Ireland
To meet future seafood demands, ingredients derived from algae and other novel and sustainable sources are increasingly being tested and used as replacers to traditional aquafeed ingredients. Algal ingredients in particular are being promoted for their sustainability and their additional functional attributes in farmed aquatic animals. Test on algal supplemented aquafeeds typically focus on a suite of immunological and physiological indicators along with fish growth performance or muscle quality. However, to optimize the replacement of fish meal with algal derived ingredients, it is crucial to understand the metabolic fate in the algal macronutrients (carbohydrates, fats, and proteins), and their nutritional interactions with other ingredients after ingestion. Here, we assess the potential of using the emerging technology- stable carbon isotope (δ13C) analysis of single amino acids (AAs) as a nutritional biomarker in aquaculture. Applications of δ13CAA-based approaches in feeding trials show promise in closing the knowledge gap in terms of understanding how fish and other aquaculture taxa assimilate and metabolize algal derived macronutrients. Source diagnostic δ13C fingerprints among the essential AAs can trace the protein origins to broad phylogenetic groups such as red macroalgae, brown macroalgae, bacteria, and terrestrial plants. Among the non-essential AAs, δ13C patterns have the potential to inform about metabolic routing and utilization of dietary lipids and carbohydrates. Despite the potential of δ13CAA as a nutritional biomarker, the few applications to date in fish feeding trials warrant further development and implementation of δ13CAA-based approaches to improve understanding of protein origins and macronutrient metabolic routing.
Introduction
Novel macro aquafeed ingredients are continually being developed and validated to lower the demand of marine-derived proteins, i.e., fish meal and oil, but also to allow the sustainable increase in fish production. For instance, marine macroalgae, or seaweeds, possess a number of positive nutritional and functional attributes that could be exploited in aquafeeds (Xu et al., 2017; Gomez-Zavaglia et al., 2019; Thépot et al., 2021). Seaweeds can be classified into three major phylogenetic groups that each have unique pigmentation and biochemical characteristics: (1) brown seaweed (Phaeophyceae); (2) red seaweed (Rhodophyceae); and (3) green seaweed (Chlorophyceae). These multicellular macroscopic algae are considered to have a smaller environmental footprint compared to fish meal and terrestrial plants crops (e.g., soybean, cereals, and legumes) that are typically used in aquafeeds. This is because algae can be grown at scale without the need for arable land, freshwater, and substantial amounts of non-renewable resources (e.g., fertilizers and pesticides). Furthermore, the use of seaweeds in aquafeed can play a role in mitigating the effects of eutrophication and climate impact through the bioremediation of nitrogen and phosphorous, and carbon capture (Duarte et al., 2017; Xiao et al., 2017).
One of the key attractiveness of using macroalgae is that they possess bioactive compounds and functional properties that can be conferred to the aquafeeds and the farmed aquatic animal, e.g., as immunomodulation, maintaining fish growth, and feed physical-chemical integrity (Figure 1; Gomez-Zavaglia et al., 2019). Inclusions of macroalgal ingredients have also been tested for crustaceans and echinoderms (Schleder et al., 2017; Omont et al., 2021; Xu et al., 2021). Besides supplying a source of dietary protein in aquafeeds, the proteins and peptides found in seaweeds have shown an extensive range of properties that include antihypertensive, antioxidative, and immune-supportive activities in fish (Harnedy and Fitzgerald, 2011). These bioactives could potentially be further augmented and enhanced by biorefinery techniques, such as hydrolyzing the seaweed proteins, optimizing the amino acid (AA) composition, and releasing previously bound bioactive sites and/or compounds (Choi et al., 2015; Yan and Wang, 2019; Thépot et al., 2021). Furthermore, some polysaccharides found in macroalgae such as those classified as phycocolloid and their derivatives can modify the gut microbiome (prebiotic) and possess immunostimulatory effects (Roberfroid, 2007; Xu et al., 2017). Similarly, many secondary metabolites produced by the algae such as mycosporine-like amino acids (Carreto and Carignan, 2011), carotenoids, and phenolics (Dethier et al., 2005) can also confer significant health benefits to farmed aquatic animals.
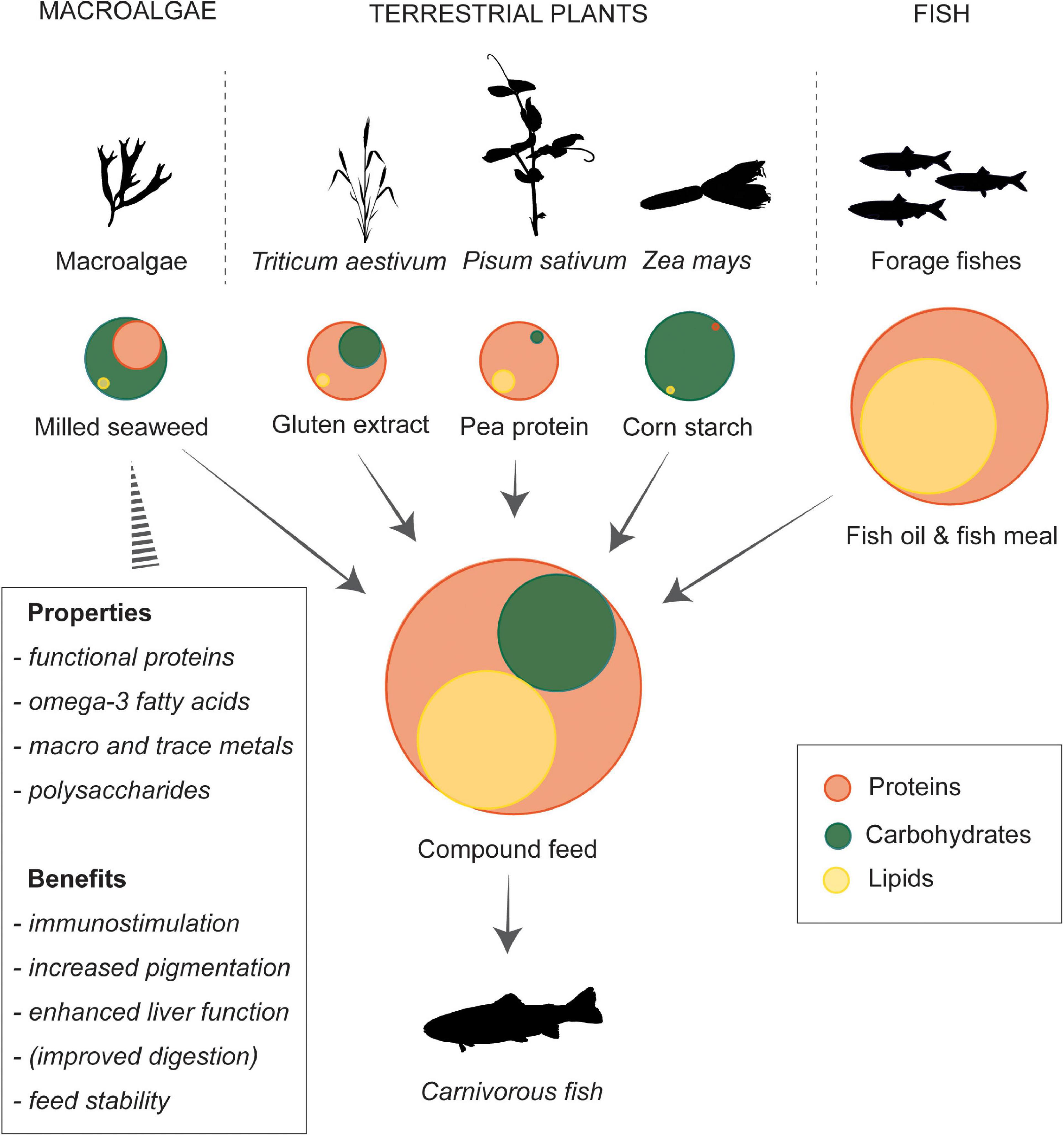
Figure 1. Schematic presentation of seaweed inclusion in compound feeds containing plant and fish derived ingredients for a carnivorous fish. The filled circles represent the relative proportion of macronutrients in each ingredient in the P10 experimental diet by Wan et al. (2016), the arrows pointing to the compound feed denote its ingredients, the arrow to the carnivorous fish indicates it feeds on the compound diet, and the striped triangle links to the box outlining key properties and benefits of milled seaweed ingredients in aquafeed. Silhouettes from PhyloPic (http://phylopic.org) under Creative Commons license.
Feeding trials that test dietary seaweed inclusions typically focus on a suite of immunological parameters in concert with fish growth performance or muscle quality (Wan et al., 2018; Hua et al., 2019; Thépot et al., 2021). Moreover, the inclusion of seaweeds in aquafeeds can lead to several nutritional benefits: Their AA composition is relatively complete and although commercially important seaweeds can be limited in their lipid content, the quality of these lipids are typically higher than those derived from terrestrial plants (Wan et al., 2018). Many macroalgal species possess a high proportion of polyunsaturated fatty acids (PUFA) compared to, e.g., terrestrial ingredients. In particular, long-chain ω-3 highly unsaturated fatty acids (HUFA, e.g., eicosapentaenoic acid, 20:5nω3), which can increase mitochondrial β-oxidation activity leading to leaner farmed fish (Todorčević et al., 2009). The levels and types of complex polysaccharides found in different macroalgae species are major factors in influencing nutrient digestibility (Gyurcsik and Nagy, 2000; Marrion et al., 2003). In addition, the natural feeding strategy and genotype in the farmed fish are important variables that determine the efficiency to which seaweeds are utilized as a nutrient source (Krogdahl et al., 2005; Kamalam et al., 2017). Even though carnivorous and omnivorous species have reduced capacity to digest and utilize complex polysaccharides, feeding trials have demonstrated that in addition to enhancing innate immune responses, seaweed inclusions in aquafeed can enhance growth and feed intake (Wan et al., 2016; Thépot et al., 2022).
Despite a suite of beneficial functional properties, the metabolic fate of algal macronutrients are generally poorly understood. This knowledge gap makes it more challenging to optimize the use of macroalgae as an aquafeed ingredient – especially for carnivorous species. Isotopic evidence shows it is unlikely that algal polysaccharides function as energy sources for Atlantic salmon (Salmo salar) (Wang et al., 2019). This is probably due to a lack or low presence of complex carbohydrate degrading enzymes, e.g., cellulase, and hemicellulose (Hidalgo et al., 1999). This lack of assimilation of macroalgal ingredients should in theory decrease the feed conversion ratio, at least for carnivorous species. Hence, to strike an optimal balance between the health benefits of seaweeds and feed utilization, it is crucial to trace the metabolic fate of seaweed ingredients in aquaculture animals. While there has been only a limited number of seaweeds tested in feeding trials to date (Wan et al., 2018; Hua et al., 2019; Thépot et al., 2021), it is also important to be mindful that seaweeds are a highly diverse group of organisms.
While molecular methods (e.g., DNA quantification and targeted metabolite profiling) are suited for characterizing aquafeed ingredients before or right after ingestion, stable isotope analyses of animal tissues are usually employed to characterize ingredients after their nutrients have been absorbed (Belghit et al., 2021; Gamboa-Delgado, 2022). The stable isotope values of total organic carbon (bulk δ13C), the most commonly analyzed element, have been used successfully for food authentication and tracing aquaculture ingredients (Molkentin et al., 2007; Anderson et al., 2010; Hassoun et al., 2020). Isotopes can be analyzed on different tissues. For example, blood and splanchnic tissues (e.g., intestine and spleen) are used to infer more recent diets because they have a higher turnover rate than structural tissues (e.g., muscle fiber and tendon) (Tieszen et al., 1983; Buchheister and Latour, 2010). It can, however, be challenging interpreting bulk isotope results because δ13C values are affected by a host of physical, chemical and physiological variables that can confound isotope values among different sources and impart variable and relatively poorly constrained isotope discrimination during trophic transfer (Casey and Post, 2011; Robinson et al., 2021). Among marine-derived ingredients, a common challenge with bulk δ13C tracers is that they lack the ability in distinguishing between, e.g., marine fish and macroalgae because of the equifinality of those sources.
Recently, stable isotope analysis in single AAs has shown considerable more precision in discerning among algae and other novel functional feed additives within test aquafeed composites (Wang et al., 2018b, 2019; Belghit et al., 2021; Xu et al., 2021). In terms of nutritional requirements, the 20 proteogenic AAs can be divided into two functional classes: the essential and the non-essential. Like many other animals, the AAs that finfish cannot synthesize de novo are referred to as essential amino acids (EAAs) and are usually passed on from one trophic level to the next with no or minor alterations of their carbon skeletons, i.e., the carboxyl group and the α-carbon to which a variety of functional groups are attached. Regarding the non-essential amino acids (NEAAs), animals can incorporate them directly from the diet or synthesize them de novo from both glycolytic and tricarboxylic acid (TCA) cycle intermediates (see Figure 2). Therefore, the differences in isotopic composition between a consumer and its diet, which is denoted Δδ13C, is greater for the NEAAs than the EAAs (McMahon et al., 2010; Barreto-Curiel et al., 2017; Liu et al., 2018; Wang et al., 2018b; Xu et al., 2021).
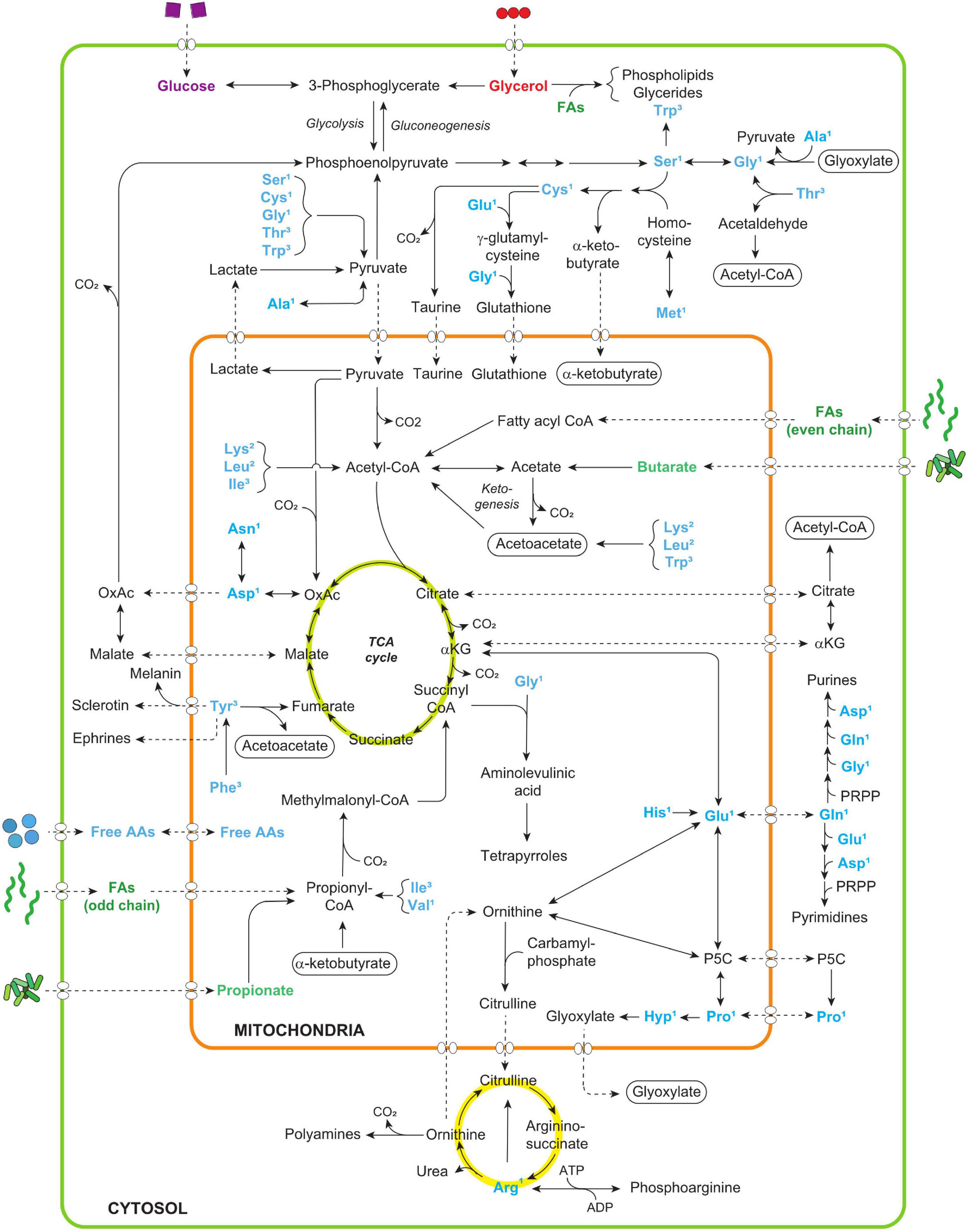
Figure 2. The chart depicts the main anabolic and catabolic amino acid (AA) pathways in vertebrates according to Berg et al. (2015) and Caspi et al. (2020) (all the proteogenic amino acids are assigned three-letter symbols according to IUPAC nomenclature). The non-essential AAs can be grouped according to their association with their main biosynthesis pathways: The glycolytic AAs are synthesized from metabolic intermediates (3-PGA, 3-phosphoglyceric acid; PEP, phosphoenolpyruvic acid) of the glycolytic pathway (in the cytosol) and the Krebs NEAAs are synthesized from intermediates of the or tricarboxylic acid cycle (TCA; αKG, α-Ketoglutaric acid; OAA, oxaloacetate) (in the mitochondria). Glucose and glycerol are sourced to the glycolytic pathway, and fatty acids (FAs) and short chain fatty acids are sourced to the TCA cycle. TCA products can also be function as intermediates for Ala via phosphoenolpyruvate and pyruvate. The catabolism of excess AAs either occurs via gluconeogenesis or ketogenesis. Gluconeogenesis is the synthesis of glucose from non-carbohydrate precursors such as the glucogenic amino acids (marked with 1) and ketogenesis is the metabolic pathway for producing ketone bodies by breaking down fatty acids and ketogenic amino acids (marked with 2). A large group of AAs can be catabolized by both processes (marked 3). PRPP and P5C signify phosphoribosyl pyrophosphate and 1-pyrroline-5-carboxylic acid, respectively.
This review will examine the analytical and theoretical underpinnings behind δ13CAA analysis, and evaluate how δ13CAA based methods can be used to understand macronutrient metabolism and trace back dietary protein origins. While this review will focus on the potential of tracing seaweed ingredients in aquafeed fed to farmed finfish, it will also evaluate the use of δ13CAA analysis in other animal species and dietary sources. Furthermore, the review will discuss the current barriers for using δ13CAA as a nutritional biomarker in farmed aquaculture animals, and the possible solutions to overcome these obstacles.
Amino Acid Abbreviations
Ala, alanine; Arg, arginine; Asn, asparagine; Asp, aspartic acid; Asx, asparagine/aspartic acid; Cys, cysteine; His, histidine; Gln, glutamine; Glu, glutamic acid; Glx, glutamine/glutamic acid; Gly, glycine; Hyp, hydroxyproline; Ile, isoleucine; Leu, leucine; Lys, lysine; Met, methionine; Phe, phenylalanine; Pro, proline; Ser, serine; Tyr, tyrosine; Thr, threonine; Val, valine.
Analytical Considerations
Gas chromatography combustion isotope ratio mass spectrometry (GC-C-IRMS) is the most common methodological approach for determining δ13CAA values. The methodology encompasses three core steps:
(1) sample cleaning and protein isolation (if needed) followed by AA extraction and purification;
(2) derivatization of the AAs to increase their volatility; and
(3) analysis with isotope ratio mass spectrometry where the analytes are separated on a GC column, combusted to CO2 and other gases before determining the isotopic composition of CO2 molecules with different molecular weights (e.g., 12C16O16O, 13C16O16O, 12C16O18O).
The most commonly used derivatization approaches for GC-C-IRMS involve esterification of the carboxylic acid group with an acidified alcohol and acylation of the amine, hydroxyl and thiol groups (Corr et al., 2007b). To account for the kinetic isotope effect and added carbon from the reagents, AA mixtures with known δ13C values are treated in parallel with the collected samples. The resulting δ13C values of these reference mixtures can then be used to correct for the isotope effect of the samples. The shift in isotope values can be reduced by using a derivatization method that adds as few carbon atoms as possible such as N-acetylmethyl esters (NACME) and selecting AA references, alcohols, and acylation agents with δ13C values that approximate those of the samples of interest (Corr et al., 2007a).
The core tenants underlying δ13CAA applications are consistency in preparation, measurement, and as mentioned above, correction for added carbon during derivatization and the use of reference standards and samples (Meier-Augenstein and Schimmelmann, 2019). The accuracy and precision of the data depend on the quality of GC separation, interface design and isotopic calibration. In short, the isotopic drift of analytical standards and reference materials should be monitored to ensure data accuracy and precision, and scale normalization should be based on two or more reference analytes (Paul et al., 2007). Operators must also ensure that the GC-C-IRMS system is regularly serviced by changing inlet liners, maintaining the integrity of the guard/main columns, and checking the combustion reactors and leakages when needed. To date, the accuracy of GC-C-IRMS produced δ13CEAA data has been somewhat inconsistent across laboratories (Arthur et al., 2014; Jarman et al., 2017; Stücheli et al., 2021). Hence, for furthering δ13CAA applications in aquaculture, it will be essential to carry out inter-laboratory comparison exercises, sharing of standardized protocols, and globally available standard reference materials, e.g., reference centers (Yarnes and Herszage, 2017). Accuracy is less of an issue for liquid chromatography–isotope ratio mass spectrometry (LC-C-IRMS) produced δ13CAA data since AAs do not need to be derivatized, but they come with the limitation that most studies report a low number of AAs (McCullagh et al., 2008; Smith et al., 2009; Dunn et al., 2011). A further consideration is that GC-C-IRMS based methods require approximately 0.3 μg of total protein per injection as opposed to 6 μg for LC-C-IRMS based methods (Smith et al., 2009; Dunn et al., 2011). Finally, the use of δ13CAA analyses as a biomarker method should be weighed against the fact that these measurements remain relatively expensive and time-consuming compared to, e.g., bulk isotope analysis. However, ongoing advances in analytical approaches and an expansion of laboratories with the capacity to measure δ13CAA are likely to increase accessibility and affordability. In-depth discussions of these topics can be found in publications authored by van Leeuwen et al. (2014), Jochmann and Schmidt (2015), and Meier-Augenstein (2018).
Digestive Physiology and Isotope Effects
To infer dietary information from δ13CAA values, it is important to consider the role of digestive and metabolic processes on trophic discrimination. The isotopic composition of a whole organism is the result of a dynamic equilibrium between nutrient assimilation and discharge of excreta (e.g., indigestible molecules) and colonic fermentation products (e.g., carbon dioxide, methane and indoles) (Butt and Volkoff, 2019). For example, a study found that the gut contents of the sand goby (Pomatoschistus minutus) were more 13C enriched in the hindgut than in the foregut, a result that in part can be ascribed to gut microbial activities (Guelinckx et al., 2008). Gut bacterial diversity and activity is generally lower in carnivores, and progressively increase from omnivores to herbivores (Wang et al., 2018a). Furthermore, carnivorous and omnivorous species usually rely far more on acid digestion than herbivorous species (Egerton et al., 2018). The intestines of carnivorous fish have evolved for processing a highly digestible, energy and nutrient-dense diet that is high in protein and low in carbohydrates. For this reason, their gut to body length is far shorter than that of detritivores and herbivores. Carnivorous fish also lacks defined gastrointestinal structures typically found in herbivorous species, e.g., gizzard, and compartmentalization of the stomachs (De Silva and Anderson, 1994), and carnivores are poor at utilizing dietary carbohydrates owing to low intestinal glucose uptake rates and limited ability to digest complex polymers (Kamalam et al., 2017). In herbivorous and detritivorous fish species, the hindgut microbiome plays a particularly important role in digesting complex polymers and providing the host with short-chain fatty acids and in some cases, de novo synthesized EAAs (Newsome et al., 2011; Clements et al., 2014). These activities incurred by the gut microbiome (Larsen et al., 2016a) and the mucous membrane of the intestinal tract (Burrin and Stoll, 2009) can lead to an increase in metabolic costs. NEAAs such as Gln, Glu, and Asp may be catabolized extensively for oxidative fuel (Box 1), and lack of these NEAAs in the diet can lead to increased catabolism of certain EAAs.
BOX 1. Overview of main anabolic and catabolic pathways of the non-essential amino acids in finfish. See Figure 2 for the visualization of the biochemical pathways and definition of key biochemical terms.
Alanine (Ala) Is the predominant amino acid (AA) catabolized by the liver where it is a main contributor to gluconeogenesis. The carbon of its precursor pyruvate derives from glucose, lactate, and other AAs. Ala and Asp are major glucogenic precursors. Ala and Gln are the main carriers of fish muscle derived nitrogen that is transported to
the liver via the blood (Felig, 1973; Okun et al., 2021).
Arginine (Arg) In fish, Arg is key for modulation of ureagenesis and ammonia detoxification, and like Pro, it is often rate limiting for growth and metabolic functions if the quantities are insufficient in the diet (Hoseini et al., 2020). It is abundant in tissue fluid as phosphoarginine, a major reservoir of ATP (Li et al., 2009).
Asparagine (Asn) and aspartate (Asp) Are major metabolic energy source for intestinal epithelial cells and have also been shown to regulate intestinal and neurological development and function (Wu, 2014). Together with Gly and Gln, Asp act as a precursor for purines and pyrimidines, which form part of the structural subunits of nucleic acids.
Cysteine (Cys) Is synthesized from methionine (Met) and serine (Ser). This semi-essential AA is a precursor for the two antioxidants, taurine and glutathione, which are key metabolites for mitochondrial functioning (Prabhu et al., 2014).
Glutamate (Glu) and glutamine (Gln) Glu is involved in diverse processes such as nitrogen assimilation and cofactor for biosynthesis (increase the rate of a chemical reaction), as well as a building for the construction of complex molecules beyond proteins (Walker and Van Der Donk, 2016). Glu and its decarboxylation product function as neurotransmitter (Li et al., 2009). Gln is one of the most abundant free α-AA in fish plasma and muscle. A large fraction of dietary Glu and Gln carbon skeletons may be degraded in the gut (Wu, 1998).
Glycine (Gly) and serine (Ser) Gly and Ser participate in fat digestion and one-carbon unit metabolism (Fang et al., 2002), and can also stimulate feed intake (Shamushaki et al., 2007). Gly is likely to regulate gene expression in fish (RileyJr., Higgs et al., 1996), and it plays critical role in the osmoregulatory responses of fishes (Powell et al., 2007). Ser is essential to maintain mitochondrial respiration (Lucas et al., 2018).
Proline (Pro) and hydroxyproline (Hyp) Pro fulfills a unique biological role in stress adaptation. The requirements of Pro for whole-body protein synthesis are the greatest among all AAs. The rates of endogenous Pro synthesis are inadequate in fish and can as such be regarded as a semi-essential AA, especially for juvenile fish (Dabrowski et al., 2005; Wu et al., 2011). Hyp is a main constituent of collagen. Although Hyp is considered a NEAA, it is a potent growth promoters for fish (Aksnes et al., 2008).
Tyrosine (Tyr) Is synthesized from phenylalanine (Phe), and is the main precursor for melanin synthesis and sclerotization (Vavricka et al., 2014). Adding Tyr to aquafeed can reduce fish requirement for Phe.
Once the macronutrients are digested into smaller molecules and absorbed into somatic cells, they are broken down for producing energy and synthesizing metabolic intermediates needed for synthesizing new molecular structures. The liver functions as the main hub for AA metabolism catabolizing most AAs, synthesizing NEAAs, and providing AAs for protein synthesis in the liver and other tissues (Figure 2). The availability of AAs at the moment of protein synthesis is critical for protein retention efficiency and protein turnover (Brezas and Hardy, 2020): If one AA is not present in sufficient amounts, the remaining AAs are alternatively catabolized for energy. Since the capacity of the organism to store free AAs is very low, it is critical that EAAs are released and assimilated approximately simultaneously to maximize the incorporation of dietary AAs into somatic tissues (Rungruangsak-Torrissen et al., 2009). Both catabolism and anabolism lead to kinetic isotope effects. This occurs when the reaction rates are affected by the isotopic composition of molecules (the isotopologues). For example, enzymatic decarboxylation reactions in the tricarboxylic acid cycle produce 13C-depleted CO2 and 13C-enriched residual acids (Takizawa et al., 2020). Thus, greater rates of biochemical reactions usually lead to greater trophic discrimination (Hayes, 2001). Sourcing of metabolic intermediates derived from macronutrients also affects δ13C values of de novo synthesized NEAAs because lipid moieties and short-chain fatty acids are 13C depleted relative to proteins and carbohydrates (Deniro and Epstein, 1977; Melzer and Schmidt, 1987; Weber et al., 1997). While not always the case for the EAAs (Newsome et al., 2020), most feeding trials show that Δδ13CEAA values usually fall within 1‰ for healthy animals feeding on nutritionally adequate diets (McMahon et al., 2010; Barreto-Curiel et al., 2017; Liu et al., 2018; Wang et al., 2018b, 2019; Takizawa et al., 2020; Xu et al., 2021). Nevertheless, some animals specialized in feeding detrital or poorly digestible diets can make up for nutritional insufficiencies by assimilating EAAs synthesized by their gut microbiomes (Newsome et al., 2011; Arthur et al., 2014; Ayayee et al., 2015; Larsen et al., 2016b). Excess of dietary proteins may cause trophic discrimination. A feeding trial with the totoaba (Totoaba macdonaldi) found that Δδ13CEAA for Ile and Leu increased linearly with higher protein levels (Ile: from 0.1‰Δδ13C at 38% protein level to 0.5‰ at 49%; Leu: 0.1‰ at 38% to 1.1‰ at 49%) probably because of isotope effects associated with EAA catabolism for energy (Barreto-Curiel et al., 2019). The study found no clear correlations between Δδ13C and dietary protein levels for the remaining EAAs (Lys, Met, Phe, and Val). Starvation, at least over a relatively short time span, appears to have little effect on Δδ13CEAA values in muscle tissues according to a study of the yellowtail (Seriola lalandi) that were starved for 35 day (Barreto-Curiel et al., 2017).
Inferring Diet and Nutrition From Amino Acid δ13C Values
Essential Amino Acids
A key source diagnostic feature of the EAAs is that algae, bacteria, fungi, and terrestrial plants have distinct δ13CEAA patterns in which the relative differences among EAAs are consistent, regardless of the actual source bulk δ13C value (Scott et al., 2006; Larsen et al., 2009a, 2013). These δ13CEAA patterns are termed fingerprints when they are unique and source characteristic. The δ13CEAA patterns of both micro- and macroalgae are diverse and appear to be more diverse compared to terrestrial vascular plants (Larsen et al., 2013; McMahon et al., 2015a; Elliott Smith et al., 2018). Brown macroalgae differ from red macroalgae (Figure 3; Larsen et al., 2013), and within the monophyletic group of cyanobacteria, diazotrophic vs. non-diazotrophic cyanobacteria appear to have distinct fingerprints (McMahon et al., 2015b). Likewise, regional differences in microalgal assemblages result in distinct δ13CEAA fingerprints in trophic chains fueled by these basal resources (Wang et al., 2018b; Larsen et al., 2020).
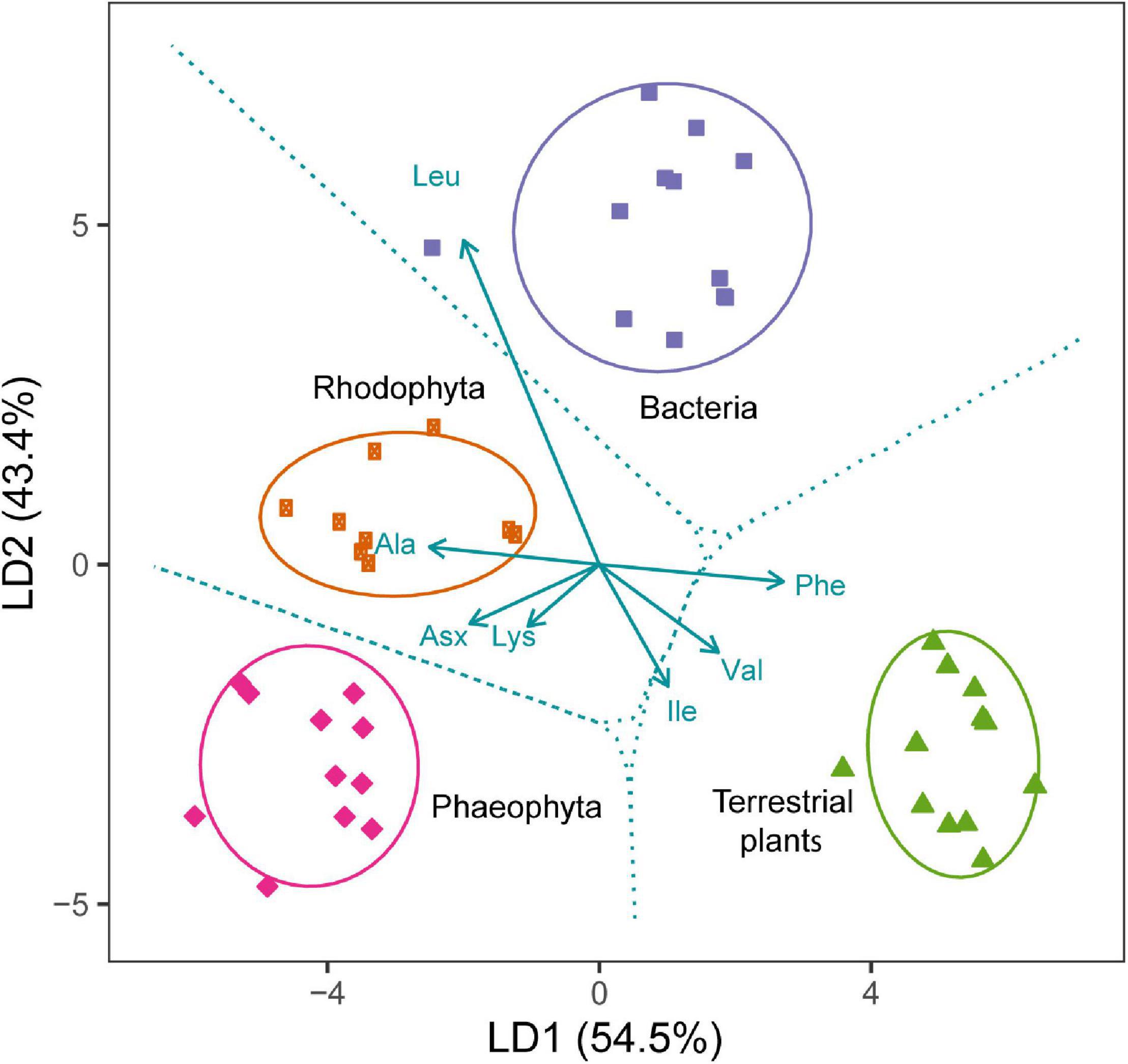
Figure 3. Linear function discriminant analysis (LDA) based on the seven most discriminate amino acid δ13C variables for separating bacteria (n = 12), Phaeophyta (n = 11), Rhodophyta (n = 9) and terrestrial plants (n = 12). Insufficient data available for Chlorophyta. Values in parentheses are the percentage variations accounted by each LD axis (54.5 and 43.4% for LD1 and LD2, respectively), the ellipses represent the 95% confidence intervals of each group, and the broken lines represent the decision boundaries. The median values of the four groups are significantly different (Pillai’s Trace = 1.90, F6,80 = 247.1; p < 0.001). The data were compiled from Larsen et al. (2013).
Non-essential Amino Acids
In contrast to the EAAs, NEAAs are less suited as source tracers because metazoans either incorporate dietary NEAAs directly into their tissue or synthesize them from metabolic intermediates sourced from lipids, carbohydrates, and proteins (Berg et al., 2015). Furthermore, dietary NEAAs are more likely to be catabolized for energy, act as metabolic precursors or be a source of nitrogen than the EAAs (Wu, 2014; McMahon et al., 2015c). While the term non-essential implies that animals can synthesize them at a rate that meets the cellular demand for protein synthesis, it is well documented that adequate amounts of dietary NEAA are required for maximum growth and optimum health (Peres and Oliva-Teles, 2006; Gaye-Siessegger et al., 2007). Hence, the rate by which the NEAAs are incorporated directly into proteinogenic tissue or synthesized de novo from metabolic intermediates varies according to physiological demands, and the quality and supply of dietary proteins. It is important to note that the term non-essential is misleading because NEAA synthesis is energetically expensive and animals have limited capacity to maintain physiological functions if their diets are NEAA limited (Borman et al., 1946; Womack and Rose, 1947; Reeds, 2000). Instead, synthesizable, and non-synthesizable AAs would be more accurate but unconventional terms for the NEAAs and EAAs (Wu, 2014; Hou and Wu, 2017). Despite the metabolic complexity of NEAA routing and synthesis (Hayes, 2001) and the variable factors affecting fractionation, controlled feeding trials have demonstrated the potential of δ13CNEAA patterns to inform about dietary ingredients and macronutrients (Newsome et al., 2014; McMahon et al., 2015c; Wang et al., 2018b, 2019; Whiteman et al., 2018).
Tracing Aquafeed Macronutrients With δ13CAa
Commercial aquafeeds have in recent years gone from a primary protein source, fishmeal, and a singular lipid, fish oil, to more than several dozen ingredients such as soy, cereals, legumes, insects, crustaceans, yeast, and algae. This development has made it more challenging to trace the origins and metabolic fate of this diverse array of ingredients and their interactions. Despite the limited development and applications of δ13CAA–based approaches in aquaculture, the few studies published to date have shown considerable promise for tracing new ingredients in aquafeed.
Wang et al. (2018b) studied Atlantic salmon to test whether δ13CAA could differentiate among fish fed aquafeed with and without seaweed inclusion. These aquafeeds contained macronutrients from up to five different marine and terrestrial sources (see Figure 1). The alternative diet substituted 15% of macroalgae, the green (Ulva sp.) or the red (Palmaria sp.), into the aquafeed by decreasing fishmeal by 5% and corn starch by 10%, while the compositions of other ingredients remain unchanged (Moroney et al., 2015; Wan et al., 2018). The three glycolytic AAs (Ala, Gly, and Ser) against four EAAs (His, Ile, Met, and Val) separated the control groups from their respective algal inclusion groups with high certainty (Figure 4). The two control groups cluster separately in part because their respective fishmeal ingredients originated from different locations and marine fish species, and in part due to the different nutritional and functional profiles of the two macroalgal species. To understand why the three glycolytic AAs could function as biomarkers of macroalgal inclusions, Wang et al. (2019) analyzed δ13CAA of salmon muscle tissue and the various protein sources in the aquafeed (fishmeal, pea protein, wheat gluten and Palmaria palmata). Macroalgal inclusion resulted in more positive Δδ13C values of the three glycolytic AAs (P < 0.01). Two factors can explain the Δδ13C shift. First, fish oil, the major source of lipids in the aquafeed, is more 13C depleted than the other macronutrients. Second, the replacement of high with low digestible carbohydrates (corn starch vs. algal carbohydrates) lowered the energy content of the aquafeed due to Atlantic salmon’s inability to digest complex carbohydrates. For this reason, we posit that salmon fed diets with macroalgal ingredients allocated a relatively higher proportion of lipids for energy than the control group. Since these catabolized lipids could not be sourced as metabolic intermediates for synthesizing NEAAs, macroalgal inclusion resulted in more positive δ13CNEAA values. This case study exemplifies the usefulness of knowing the δ13C values of individual ingredients and/or macronutrients for inferring metabolic routing from δ13CNEAA values.
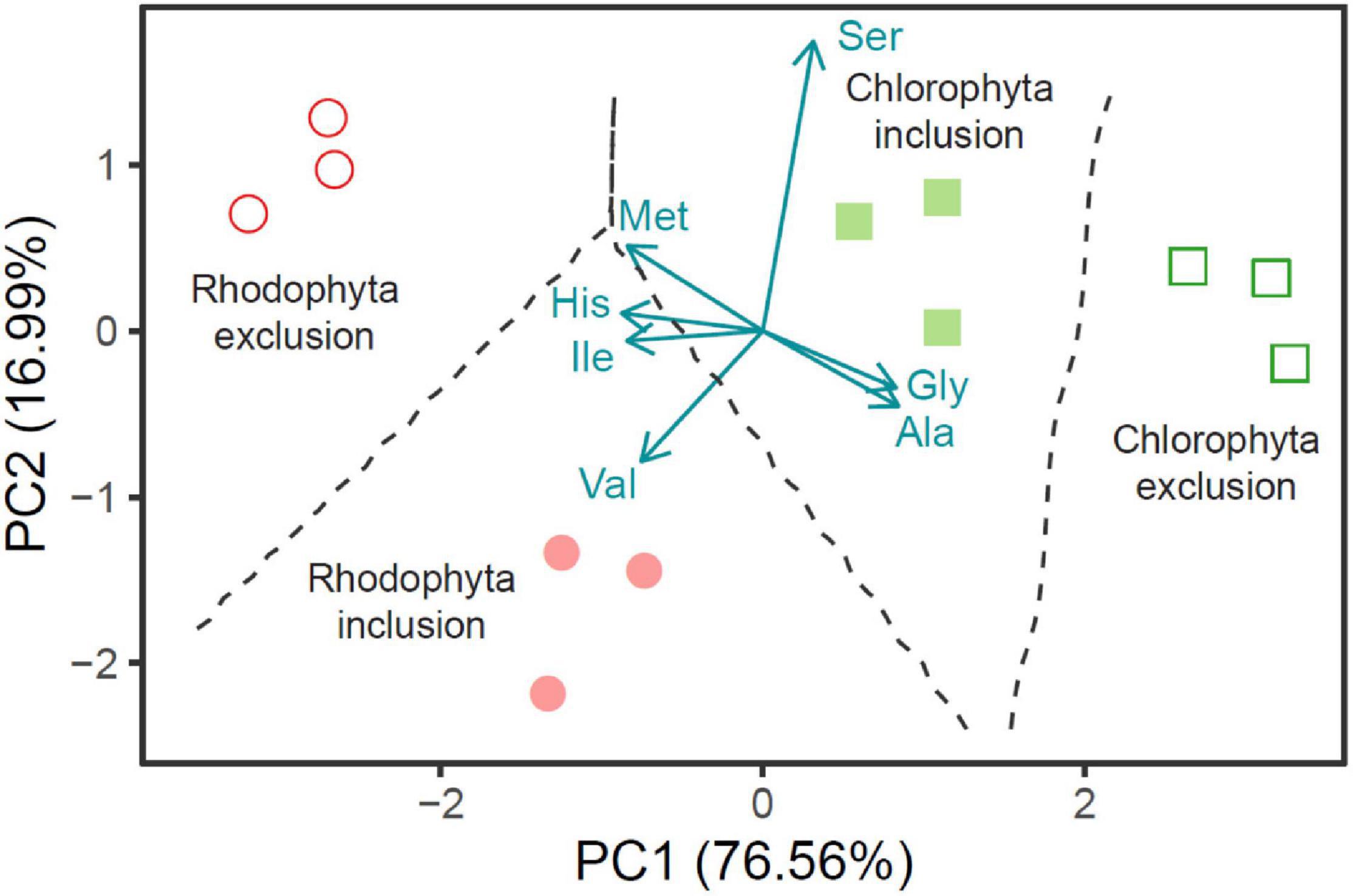
Figure 4. Principal component analyses (PCA) based on the seven most discriminate amino acid δ13C variables for separating Atlantic salmon (n = 3 in each group) fed composite diets with (15%) and without Rhodophyta and Chlorophyta ingredients. Values in parentheses are the percentage variations accounted by each PC axis (76.6 and 17.0% for PC1 and PC2, respectively), and the broken lines represent the decision boundaries. The data were compiled from Wang et al. (2019).
For algivorous aquaculture species, aquafeed diets can comprise completely of seaweeds. In a feeding trial, the sea cucumber Apostichopus japonicus was either fed the microalga Cylindrotheca fusiformis (CF; 21.1% protein, 10.8% lipid, 19.1% carbohydrate) or the brown macroalga Sargassum thunbergii (ST; 17.1% protein, 3.9% lipid, 50.0% carbohydrate) (Xu et al., 2021). CF was tested as an alternative feed source to ST because in China it is becoming increasingly difficult to meet the demands of brown macroalgae for the rapidly growing sea cucumber industry. The results showed that the growth rates of sea cucumbers were highest on the CF diet despite the relatively lower feed conversion efficiency compared to the ST diet. Between the two treatments, the Δδ13C values of Pro, Asp, and Ala were consistently more negative (from −5.0 to −2.5‰) in sea cucumbers reared on CF than ST diets. Xu et al. (2021) proposed that these differences in part can be explained by the relatively lower NEAA abundance in the CF diet. However, the abundance was only lower for some and not all NEAAs (such as Ala), and the absolute AA concentration was higher in the CF than ST diet. It is also possible that the higher lipid content of CF than ST led to more negative Δδ13C values of Pro and Asp in the CF treatment. This hypothesis is, however, at odds with the Δδ13C values of Glx being similar between the two treatments. Since Glu acts as a precursor for Pro via pyrroline-5-carboxylate (see Figure 2). We propose a third hypothesis for the Δδ13C differences, namely that the different macronutrient profiles of the two algal diets affected gut metabolic activities and therefore catabolic demands for certain NEAAs. For example, diets that cause increased osmotic stress of the intestinal microbiome is likely to surge the demand for polyamines, not only for gut bacteria but also for the enterocytes (the cells of the intestinal lining) (Wu et al., 2000; Rothe and Blaut, 2013). Although three NEAAs, Glu, Pro, and Arg, can act as precursors for polyamine synthesis via ornithine (see Figure 2), Pro appears to be the major source of ornithine (Wu et al., 2000). It is also worth noting that the Δδ13C values of Asx were more negative in the CF treatment. Asp is one of the major oxidative fuels for intestinal epithelial cells (Wu, 2014). If Asp served as an energy source, it could have decreased incorporation of dietary Asp into the body walls of the sea cucumbers (the tissue being analyzed). Whether the two diets affected gut metabolic activities differently remains speculative on our part, but the sea cucumber study illustrates the need to employ additional biomarkers of enzymatic activities and tracers such as radiocarbon to better understand Δδ13CNEAA controls.
Feeding trials can also comprise a trophic chain encompassing primary producers and primary and secondary consumers. In an elegantly designed feeding trial where the microalga Chlorella spp. (18.5% protein, 11.1% lipid, 62.7% carbohydrate) was fed to the copepod Calanus sinicus (74.3% protein, 20.4% lipid, 2.8% carbohydrate), and the copepods to the European anchovy, Engraulis encrasicolus (50.5% protein, 41.8% lipid, 1.1% carbohydrate), Liu et al. (2018) determined the Δδ13C values of the two consumers. In summary, the copepod Δδ13C values were positive for Pro, Ser, Glx, and Gly (from 6.5 to 10.3‰), but not for Ala and Asx (−4.9 and −2.3‰). The anchovy Δδ13C values were positive for Pro and Asx (2.2 and 5.4‰) but not for Gly, Ser, Ala, and Glx (from −9.7 to −3.8‰). The mostly contrasting Δδ13C results of the two consumers can in large part be ascribed to the different macromolecular compositions of their diets. The copepods fed on a low protein but high carbohydrate content diet, which would increase metabolic sourcing from carbohydrates rather than proteins (and lipids) to de novo synthesized NEAAs. The reason that the pyruvate group (Ala and Asx; see Figure 2) has more negative Δδ13C values than the α-ketoglutarate group (Glx and Pro) group is probably that the pyruvate to α-ketoglutarate pathway losses two CO2 molecules (see Figure 2). The anchovies fed on a diet almost completely devoid of carbohydrates. Like the study’s authors (Liu et al., 2018), we cannot completely explain the contrasting Δδ13C results of Asx and Pro vs. Gly, Ser, Ala, and Glx. We suppose these results are a mismatch of some NEAAs being redundantly ingested or catabolized during digestive processes (i.e., Asx and Pro), which would leave the remaining pool 13C enriched. For Gly, Ser and Ala, the negative Δδ13C values might be explained by carbon sourcing from 13C depleted glycerol via 3-phospoglycerate to de novo synthesized carbon skeletons (see Figure 2).
The feeding trials reviewed above with sea cucumbers, copepods, and anchovies show it can be complex making inferences about macronutrient routing with just one aquafeed ingredient. A study by McMahon et al. (2010) illustrates that the complexity increases when consumers are fed compound feeds with varying nutritional compositions. Juvenile mummichogs (Fundulus heteroclitus) were fed high carbohydrate Vegi-Pro (9.0% protein, 6.7% lipid, 82.0% carbohydrate partially from corn meal, 2.3% fiber) or high protein Bio-Vita (63.8% protein: 28.6 lipid: 7.2% carbohydrate, 0.4% fiber) aquafeeds. Interestingly, the two glycolytic AAs Gly and Ser had contrasting responses: Δδ13CGly values were quite positive (∼3‰) in the Vegi-Pro fish indicating carbohydrate sourcing and the very negative (∼−8‰) in the Bio-Vita fish indicating sourcing of lipid moieties. Conversely, Δδ13CSer values were slightly negative (∼−1‰) in the Vegi-Pro fish and quite positive (∼3‰) in the Bio-Vita fish indicating that metabolic routing of lipid moieties to Ser synthesis was minimal. It is possible that carbohydrates were sourced to Ser synthesis in both treatments, which leaves the question of why it was not the case for Gly in the Bio-Vita treatment. In comparison, in a more recent feeding study where hatchery-reared Chinook salmon (Oncorhynchus tshawytscha) were fed Bio-Vita, the Δδ13CGly values were positive (2.6‰; Δδ13CSer was not reported) (Rogers et al., 2019), which raises the possibility of mummichogs and salmon having species-specific metabolic responses. In the mummichog study, the Δδ13C values of the third glycolytic AA, Ala, was negative in both treatments indicating sourcing from lipids. We also want to highlight that δ13CGlu values in fish mirrored those of the Bio-Vita diets, but not among the Vegi-Pro fish (Δδ13C: ∼5‰). This might indicate that Glu was synthesized to a lesser degree in fish feeding on high than low protein diets. Moreover, gut microbial activity may have been higher for fish feeding on Vegi-Pro owing to its comparatively higher carbohydrate and fiber content, which in turn could have increased Glu and Gln degradation in the gut (Wu, 1998). Despite the multiple nutritional variables between the two aquafeeds, the study sheds light on important controls underlying macronutrient routing.
δ13CAA-based approaches can also be used to investigate the contributions of gut microbial AAs to protein synthesis. This would be particularly relevant for understanding the effects of seaweed dietary supplements in herbivorous or omnivorous fish species. For example, a feeding trial with Nile Tilapia (Oreochromis niloticus) found that gut symbiotic microbes supplemented de novo synthesized EAAs to host when dietary proteins were replaced by hardly digestible fibers (Newsome et al., 2011). While δ13CEAA fingerprinting is suited for assessing gut microbial supplementation of EAAs to host, it is much more complex understanding the controls underlying δ13CNEAA patterns. The reason is that the host also synthesize NEAAs, which means that it is necessary to consider three rather than two NEAA sources, namely the food, gut microbes and host synthesized NEAAs. In such cases, we see little scope for inferring macromolecular metabolism from δ13CNEAA patterns.
The rapid expansion of alternative ingredients in aquafeeds makes it more pressing to develop new authentication and traceability methods. In this regard, δ13CAA analysis is emerging as a complementary approach to already established tracer methods. As reviewed above, δ13CAA values could detect whether salmon fed on aquafeed containing milled seaweed ingredients (15% seaweed substituted the caloric equivalent of fishmeal and corn starch) (Wang et al., 2018b). Likewise, in a feeding trial with black soldier flies reared for aquafeed, the δ13CAA method could detect whether the flies had fed on diets spiked with non-permitted bovine blood residues (Belghit et al., 2021). This is a remarkable result because the flies were fed a control diet for 7 days after initially being fed a diet with 10% (w/w) bovine blood residues. The study also assessed the suitability of legacy molecular analysis tools such as qPCR and LC-MS/MS, but only the δ13CAA method could detect whether the flies had ingested bovine blood 1 week prior to being fed the control diets. However, both legacy and novel authentication methods displayed shortcomings. Hence, to detect particular contaminants or ingredients across two trophic levels Belghit et al. (2021) recommended a tiered combined use of complementary approaches.
Outlook and Perspectives
Given the rapid expansion of aquaculture and the need to find more sustainable and low environmental impact proteinaceous ingredients (i.e., alternatives to fish meal and soybean) for aquafeed production, macroalgae stand out as an important alternative that has the potential to improve feed efficiency and fish health (Hua et al., 2019). Globally, more than 11,500 seaweed species have been identified (Guiry and Guiry, 2022). Their early divergence and genetic diversity have led to a range of different bioactive and nutritional compounds to be evolved. Yet, only 34 different seaweed species are represented in the 142 studies reviewed for their immunological properties by Thépot et al. (2021). Probably due to low commercial interests, only two studies out of the 142 studies evaluated the effects of seaweed dietary supplements in marine herbivorous fish, i.e., species that would naturally forage on seaweed in the wild. For both carnivorous and herbivorous fish species, δ13CAA based approaches can provide further insight into how fish utilize macroalgal macronutrients. As with any other novel and relatively untested aquafeed ingredients, it is important to rely on complementary approaches to holistically assess the suitability of macroalgae through their nutritional value and potential hazards such as potential toxic metals (National Food Institute, Technical University of Denmark, Denmark, Monteiro et al., 2019).
The case studies presented in this paper have primarily focused on using δ13CAA to trace macroalgal ingredients and detect gut microbial supplementation of AAs to host. Another promising δ13CAA application would be the use of marine protist and heterotrophic microalgae as an aquafeed ingredient (Klamczynska and Mooney, 2017; Fossier Marchan et al., 2018). These promising alternative aquafeed ingredient can be cultured without light and in higher densities compared to photoautotrophic algae. Depending on the culture strain and conditions during cultivation, it is possible within a short time span to produce mixotrophic heterokonts such as Schizochytrium sp. under heterotrophic conditions that contain >60% proteins or >70% lipids. According to a cradle-to-gate assessment of whole algal protein products, heterotrophically grown microalgae (i.e., through bioreactors and fermenters) also leave a smaller CO2 footprint compared to terrestrial protein sources such as soy byproducts (Thinkstep, 2015). However, whole algae proteins contain very high levels of NEAAs compared to other proteinaceous aquafeed ingredients, e.g., animal-based proteins (Klamczynska and Mooney, 2017). For this reason, it will be important to develop biorefinery methods for augmenting the AA profiles of heterotrophically grown microalgae for aquafeed, such as altering their nutrient growing substrate (Nham Tran et al., 2020). Another possibility to augment the nutritional profile of aquafeed containing whole algae protein sources would be to mix them with other functional feed additives such as macroalgae and spent yeasts (Rakowska et al., 2017). From an analytical perspective, the addition of multiple feed additives to aquafeed increases the complexity of tracing the trophic fate of their macronutrients.
While the δ13CEAA fingerprinting method holds considerable promise for investigating the biosynthetic of EAAs from a diverse set of feed additives, the phylogenetic resolution of fingerprints beyond algae, bacteria, fungi and plants is still unknown and needs further exploration. The δ13CEAA variability within each of the broad taxonomic groups can be large, which may lead to unspecific results. Also, the ability of fingerprints to detect microbial fermentation of feed additives remains unknown. To overcome these constraints and explore the phylogenetic specificity and robustness of the fingerprinting method, a concerted transdisciplinary effort is needed to expand δ13CAA reference libraries of primary production sources. It will be key to investigate how environmental conditions and nutritional composition of growth media affect algal δ13CEAA fingerprints (Larsen et al., 2015). For example, crude protein, total lipid, and secondary metabolite (e.g., fucoxanthin and polyphenols) concentrations of algae have been found to vary considerably across seasons and geographic locations (Steinberg, 1989; Fleurence, 1999; Nomura et al., 2013). It is likely that algal internal organs with high concentrations of storage lipids and secondary metabolites can affect δ13CEAA profile due to the upregulation of these compounds may affect 13C fractionation of upstream EAA precursors (Hayes, 2001). Related to growth conditions and substrates, litter-using and humus-using ectomycorrhizal fungi appear to have distinctive δ13CEAA profiles. This could be due to the conversion of source carbon to metabolic AA precursors affecting isotopic discrimination (Pollierer et al., 2019). In microbial mats dominated by anaerobic methanotrophic archaea, δ13CEAA patterns are variable and not fixed as is the case with photoautotrophic microalgae (Takano et al., 2018; Stücheli et al., 2021). During biosynthesis of the pyruvate family AAs, 13C fractionation widens between short- and long-chain carbon AAs with increasing 13C depletion of the substrate methane (Takano et al., 2018). While microbial mats are an unlikely aquafeed ingredient, the examples above serve to show the limitations and possibilities of δ13CEAA fingerprints for tracing protein sources.
To deepen our understanding of the metabolic routing of macronutrients and their constituent molecules with δ13CAA-based methods, further, carefully designed feeding trials are warranted. In terms of assessing trophic EAA discrimination and inferring gut microbial EAA supplementation to the host, it is key that the metabolically active tissues being analyzed are in full equilibrium with the diet. Feeding trials where animals are switched to a diet with distinct isotope values consistently show memory effects of the former diet (Tieszen et al., 1983; Bauchinger and Mcwilliams, 2009; Larsen et al., 2009b; Buchheister and Latour, 2010). It is also key that feeding trials strive to vary only one nutritional or isotopic parameter at a time. The aforementioned totoaba fish feed trial kept dietary levels of aquafeed lipids and carbohydrates fixed while varying protein levels by changing the ratio of digestible to non-digestible proteins (Barreto-Curiel et al., 2019). This was done by crosslinking the proteins by exposing them to formaldehyde and heat. Another approach that simplifies the nutritional interpretation of δ13CAA data is maintaining a fixed macromolecular composition between the control and the experimental diets as demonstrated in the aforementioned salmon feeding trials with macroalgal ingredients (Wang et al., 2018b, 2019). It is also possible to keep the nutritional composition fixed and instead change the isotopic values of the constituent ingredient and/or macronutrients being used in the feed formulation. For example, substituting C3 plant ingredients (e.g., soy) with C4 plants (e.g., maize), or freshwater with marine algae. This approach based on naturally occurring isotope variability would in most cases be sufficient to trace metabolic routing of macronutrients in question, and it is less costly and laborious than using isotope labeled ingredients.
Disentangling the direct and indirect interactions between seaweed and their digested derivatives during the metabolic pathways in the animals presents a significant challenge. While δ13CAA is an emerging and promising tool in understanding metabolic routing of aquafeed ingredients and macronutrients, it cannot stand alone. Interpretations of δ13CAA data can be enhanced by merging with other biomarkers as demonstrated in a comparison of traditional molecular biomarkers (e.g., qPCR and LC-MS/MS) and δ13CAA for their ability to trace adulterated ingredients in the aquafeed chain (Belghit et al., 2021). Overall, the use of δ13CAA as a biomarker will allow feed formulations to be more nutritionally optimized and refined to specific farmed fish species. The data collected can also be used in further downstream assessments, such as life cycle analysis on fish production impact and sustainability through more accurate data in dietary amino acid contribution (Cooney et al., 2021). In addition, δ13CAA can advance our understanding of the dietary requirements of new farmed fish species and continue our exploitation of novel aquafeed ingredients, i.e., algae, bacteria, protists, fungi, annelids, and insects.
Materials and Methods
Statistical Methods
To compare δ13CAA patterns among phylogenetic groups and treatments, we applied principal component analysis (PCA) (R: prcomp) and linear discriminant function analysis (LDA) (R: MASS). PCA is commonly used for exploring δ13CAA variability and patterns because it is an unsupervised technique that seeks to maximize variability among samples while reducing the number of dimensions. LDA is a supervised technique that seeks to maximize variability among the predefined groups or classes with the goal of predicting specific protein sources with the δ13CAA fingerprinting approach. For the PCA, we used the covariance matrix approach that preserves variance as the range and scale of variables are in the same units of measure. Based on the first and second LD scores, we used 95% prediction ellipses to visualize variability relative to the group centroid. We applied Multivariate Analysis of Variance (MANOVA, R: manova) in conjunction with Pillai’s trace to test the null hypothesis that groups have a common centroid in a dependent variable vector space. A rejection of this hypothesis entails that the groups have significantly different δ13CEAA patterns or fingerprints. All data for multivariate comparisons were first assessed for homogeneity of variance by using Fligner-Killeen tests (R: fligner.test) and visually checked for departures from normality on Q-Q plots. R version 3.6.3 was used for statistical analyses (R-Development-Core-Team 2020) and ggplot2 for figure production (Wickham, 2016).
Author Contributions
TL and AW: conceptualization. TL: methodology, statistics, and visualization. TL and AW: writing and original draft preparation. TL, AW, and YW: review and editing. All authors contributed to the article and approved the submitted version.
Funding
TL was supported by Germany’s Federal Ministry of Education and Research (BMBF) via LOMVIA (03V01459). AW was supported by Grant-Aid (Agreement No. MFFRI/07/01) under the Sea Change Strategy with the support of the Marine Institute and also National Development Plan 2007–2013 grant to the Department of Agriculture, Food and the Marine, Ireland, and Science Foundation Ireland, Technology Innovation Development Award (Agreement No. 18/TIDA/6107).
Conflict of Interest
The authors declare that the research was conducted in the absence of any commercial or financial relationships that could be construed as a potential conflict of interest.
The handling editor declared a past co-authorship with several of the authors YW and TL.
Publisher’s Note
All claims expressed in this article are solely those of the authors and do not necessarily represent those of their affiliated organizations, or those of the publisher, the editors and the reviewers. Any product that may be evaluated in this article, or claim that may be made by its manufacturer, is not guaranteed or endorsed by the publisher.
References
Aksnes, A., Mundheim, H., Toppe, J., and Albrektsen, S. (2008). The effect of dietary hydroxyproline supplementation on salmon (Salmo salar L.) fed high plant protein diets. Aquaculture 275, 242–249.
Anderson, K. A., Hobbie, K. A., and Smith, B. W. (2010). Chemical profiling with modeling differentiates wild and farm-raised salmon. J. Agri. Food Chem. 58, 11768–11774. doi: 10.1021/jf102046b
Arthur, K. E., Kelez, S., Larsen, T., Choy, C. A., and Popp, B. N. (2014). Tracing the biosynthetic source of essential amino acids in marine turtles using δ13C fingerprints. Ecology 95, 1285–1293. doi: 10.1890/13-0263.1
Ayayee, P. A., Larsen, T., Rosa, C., Felton, G. W., Ferry, J. G., and Hoover, K. (2015). Essential amino acid supplementation by gut microbes of a wood-feeding cerambycid. Environ. Entomo. 45, 66–73. doi: 10.1093/ee/nvv153
Barreto-Curiel, F., Focken, U., D’abramo, L. R., Mata-Sotres, J., and Viana, M. T. (2019). Assessment of amino acid requirements for Totoaba macdonaldi at different levels of protein using stable isotopes and a non-digestible protein source as a filler. Aquaculture 503, 550–561.
Barreto-Curiel, F., Focken, U., D’abramo, L. R., and Viana, M. T. (2017). Metabolism of Seriola lalandi during starvation as revealed by fatty acid analysis and compound-specific analysis of stable isotopes within amino acids. PLoS One 12:e0170124. doi: 10.1371/journal.pone.0170124
Bauchinger, U., and Mcwilliams, S. (2009). Carbon turnover in tissues of a passerine bird: allometry, isotopic clocks, and phenotypic flexibility in organ size. Physiol. Biochem. Zool. 82, 787–797. doi: 10.1086/605548
Belghit, I., Varunjikar, M., Lecrenier, M. C., Steinhilber, A. E., Niedzwiecka, A., Wang, Y. V., et al. (2021). Future feed control – tracing banned bovine material in insect meal. Food Control 128:108183.
Berg, J. M., Stryer, L., Tymoczko, J. L., and Gatto, G. J. (2015). Biochemistry. Germany: Macmillan Learning.
Borman, A., Wood, T. R., Black, H. C., Anderson, E. G., Oesterling, M. J., Womack, M., et al. (1946). The role of arginine in growth with some observations on the effects of argininic acid. J. Biol. Chem. 166, 585–594.
Brezas, A., and Hardy, R. W. (2020). Improved performance of a rainbow trout selected strain is associated with protein digestion rates and synchronization of amino acid absorption. Sci. Rep. 10:4678. doi: 10.1038/s41598-020-61360-0
Buchheister, A., and Latour, R. J. (2010). Turnover and fractionation of carbon and nitrogen stable isotopes in tissues of a migratory coastal predator, summer flounder (Paralichthys dentatus). Can. J. Fish. Aquat. Sci. 67, 445–461.
Burrin, D. G., and Stoll, B. (2009). Metabolic fate and function of dietary glutamate in the gut. Am. J. Clin. Nutr. 90, 850S–856S. doi: 10.3945/ajcn.2009.27462Y
Butt, R. L., and Volkoff, H. (2019). Gut microbiota and energy homeostasis in fish. Front. Endocrino. 10:9. doi: 10.3389/fendo.2019.00009
Carreto, J. I., and Carignan, M. O. (2011). Mycosporine-like amino acids: relevant secondary metabolites. chemical and ecological aspects. J. Mar. Drugs 9, 387–446. doi: 10.3390/md9030387
Casey, M. M., and Post, D. M. (2011). The problem of isotopic baseline: reconstructing the diet and trophic position of fossil animals. Earth-Sci. Rev. 106, 131–148.
Caspi, R., Billington, R., Keseler, I. M., Kothari, A., Krummenacker, M., Midford, P. E., et al. (2020). The MetaCyc database of metabolic pathways and enzymes - a 2019 update. Nucleic Acids Res. 48, D445–D453. doi: 10.1093/nar/gkz862
Choi, Y. H., Lee, B.-J., and Nam, T. J. (2015). Effect of dietary inclusion of Pyropia yezoensis extract on biochemical and immune responses of olive flounder Paralichthys olivaceus. J. Aquac. 435, 347–353.
Clements, K., Angert, E., Montgomery, L., and Choat, J. (2014). Intestinal microbiota in fishes: what’s known and what’s not. Mol. ecol. 23, 1891–1898. doi: 10.1111/mec.12699
Cooney, R., Wan, A. H. L., O’donncha, F., and Clifford, E. (2021). Designing environmentally efficient aquafeeds through the use of multicriteria decision support tools. Curr. Opin. Environ. Sci. Health 23:100276.
Corr, L. T., Berstan, R., and Evershed, R. P. (2007a). Development of N-acetyl methyl ester derivatives for the determination of δ13C values of amino acids using gas chromatography-combustion-isotope ratio mass spectrometry. Ana. Chem. 79, 9082–9090. doi: 10.1021/ac071223b
Corr, L. T., Berstan, R., and Evershed, R. P. (2007b). Optimisation of derivatisation procedures for the determination of d13C values of amino acids by gas chromatography/combustion/isotope ratio mass spectrometry. Rapid Commun. Mass Spectrom. 21, 3759–3771. doi: 10.1002/rcm.3252
Dabrowski, K., Terjesen, B. F., Zhang, Y., Phang, J. M., and Lee, K.-J. (2005). A concept of dietary dipeptides: a step to resolve the problem of amino acid availability in the early life of vertebrates. J. Exp. Biol. 208, 2885–2894. doi: 10.1242/jeb.01689
Deniro, M. J., and Epstein, S. (1977). Mechanism of carbon isotope fractionation associated with lipid-synthesis. Science 197, 261–263. doi: 10.1126/science.327543
Dethier, M. N., Williams, S. L., and Freeman, A. (2005). Seaweeds under stress: manipulated stress and herbivory affect critical life–history functions. J. Ecol. Monogr. 75, 403–418.
Duarte, C. M., Wu, J., Xiao, X., Bruhn, A., and Krause-Jensen, D. (2017). Can seaweed farming play a role in climate change mitigation and adaptation? Front. Mar. Sci. 4:100. doi: 10.3389/fmars.2017.00100
Dunn, P. J. H., Honch, N. V., and Evershed, R. P. (2011). Comparison of liquid chromatography-isotope ratio mass spectrometry (LC/IRMS) and gas chromatography-combustion-isotope ratio mass spectrometry (GC/C/IRMS) for the determination of collagen amino acid δ13C values for palaeodietary and palaeoecological reconstruction. Rapid Commun. Mass Spectrom. 25, 2995–3011. doi: 10.1002/rcm.5174
Egerton, S., Culloty, S., Whooley, J., Stanton, C., and Ross, R. P. (2018). The gut microbiota of marine fish. Front. Microbiol. 9:873. doi: 10.3389/fmicb.2018.00873
Elliott Smith, E. A., Harrod, C., and Newsome, S. D. (2018). The importance of kelp to an intertidal ecosystem varies by trophic level: insights from amino acid δ13C analysis. Ecosphere 9:e02516.
Fang, Y.-Z., Yang, S., and Wu, G. (2002). Free radicals, antioxidants, and nutrition. Nutrition 18, 872–879.
Fleurence, J. (1999). Seaweed proteins: biochemical, nutritional aspects and potential uses. J. Trends Food Sci. Technol. 10, 25–28.
Fossier Marchan, L., Lee Chang, K. J., Nichols, P. D., Mitchell, W. J., Polglase, J. L., and Gutierrez, T. (2018). Taxonomy, ecology and biotechnological applications of thraustochytrids: a review. Biotechnol. Adv. 36, 26–46. doi: 10.1016/j.biotechadv.2017.09.003
Gamboa-Delgado, J. (2022). Isotopic techniques in aquaculture nutrition: state of the art and future perspectives. Rev. Aquac. 14, 456–476. doi: 10.1111/raq.12609
Gaye-Siessegger, J., Focken, U., Abel, H., and Becker, K. (2007). Influence of dietary non-essential amino acid profile on growth performance and amino acid metabolism of Nile tilapia, Oreochromis niloticus (L.). Comp. Biochem. Physiol. A Mol. Integr. Physiol. 146, 71–77. doi: 10.1016/j.cbpa.2006.09.025
Gomez-Zavaglia, A., Prieto Lage, M. A., Jimenez-Lopez, C., Mejuto, J. C., and Simal-Gandara, J. (2019). The potential of seaweeds as a source of functional ingredients of prebiotic and antioxidant value. Antioxidants 8:406. doi: 10.3390/antiox8090406
Guelinckx, J., Dehairs, F., and Ollevier, F. (2008). Effect of digestion on the δ13C and δ15N of fish-gut contents. J. Fish Biol. 72, 301–309.
Guiry, M. D., and Guiry, G. M. (2022). AlgaeBase. World-Wide Electronic Publication. Galway: National University of Ireland. Available online at: https://www.algaebase.org (accessed January 24, 2022).
Gyurcsik, B., and Nagy, L. J. C. C. R. (2000). Carbohydrates as ligands: coordination equilibria and structure of the metal complexes. Coord. Chem. Rev. 203, 81–149.
Harnedy, P. A., and Fitzgerald, R. J. (2011). Bioactive proteins, peptides, and amino acids from macroalgae 1. J. Phycol. 47, 218–232. doi: 10.1111/j.1529-8817.2011.00969.x
Hassoun, A., Måge, I., Schmidt, W. F., Temiz, H. T., Li, L., Kim, H.-Y., et al. (2020). Fraud in animal origin food products: advances in emerging spectroscopic detection methods over the past five years. J. Foods 9:1069. doi: 10.3390/foods9081069
Hayes, J. M. (2001). “Fractionation of the isotopes of carbon and hydrogen in biosynthetic processes,” in Reviews in Mineralogy and Geochemistry 43, Stable Isotope Geochemistry, ed. D. R. Cole (Washington: The Mineralogical Society of America), 225–277.
Hidalgo, M., Urea, E., and Sanz, A. (1999). Comparative study of digestive enzymes in fish with different nutritional habits. proteolytic and amylase activities. Aquaculture 170, 267–283.
Hoseini, S. M., Ahmad Khan, M., Yousefi, M., and Costas, B. (2020). Roles of arginine in fish nutrition and health: insights for future researches. Rev. Aquac. 12, 2091–2108.
Hou, Y., and Wu, G. (2017). Nutritionally nonessential amino acids: a misnomer in nutritional sciences. Adv. Nutr. 8, 137–139. doi: 10.3945/an.116.012971
Hua, K., Cobcroft, J. M., Cole, A., Condon, K., Jerry, D. R., Mangott, A., et al. (2019). The future of aquatic protein: implications for protein sources in aquac ure diets. One Earth 1, 316–329.
Jarman, C. L., Larsen, T., Hunt, T., Lipo, C., Solsvik, R., Wallsgrove, N., et al. (2017). Diet of the prehistoric population of Rapa Nui (Easter Island, Chile) shows environmental adaptation and resilience. Am. J. Biol. Anthropol. 164, 343–361. doi: 10.1002/ajpa.23273
Jochmann, M. A., and Schmidt, T. C. (2015). Compound-specific Stable Isotope Analysis. Cambridge: Royal Society of Chemistry.
Kamalam, B. S., Medale, F., and Panserat, S. (2017). Utilisation of dietary carbohydrates in farmed fishes: new insights on influencing factors, biological limitations and future strategies. J. Aquacul. 467, 3–27.
Klamczynska, B., and Mooney, W. D. (2017). “Chapter 20 - heterotrophic microalgae: a scalable and sustainable protein source,” in Sustainable Protein Sources, eds S. R. Nadathur, J. P. D. Wanasundara, and L. Scanlin (San: Academic Press), 327–339.
Krogdahl, Å, Hemre, G. I, and Mommsen, T. (2005). Carbohydrates in fish nutrition: digestion and absorption in postlarval stages. Aquacul. Nutr. 11, 103–122.
Larsen, T., Bach, L. T., Salvatteci, R., Wang, Y. V., Andersen, N., Ventura, M., and Mccarthy, M. D. (2015). Assessing the potential of amino acid 13C patterns as a carbon source tracer in marine sediments: effects of algal growth conditions and sedimentary diagenesis. Biogeosciences 12, 4979–4992. doi: 10.5194/bg-12-4979-2015
Larsen, T., Hansen, T., and Dierking, J. (2020). Characterizing niche differentiation among marine consumers with amino acid δ13C fingerprinting. Ecol. Evol. 10, 7768–7782. doi: 10.1002/ece3.6502
Larsen, T., Ventura, M., Andersen, N., O’Brien, D. M., Piatkowski, U., and Mccarthy, M. D. (2013). Tracing carbon sources through aquatic and terrestrial food webs using amino acid stable isotope fingerprinting. PLoS One 8:e73441. doi: 10.1371/journal.pone.0073441
Larsen, T., Taylor, D. L., Leigh, M. B., and O’Brien, D. M. (2009a). Stable isotope fingerprinting: a novel method for identifying plant, fungal or bacterial origins of amino acids. Ecology 90, 3526–3535. doi: 10.1890/08-1695.1
Larsen, T., Ventura, M., Damgaard, C., Hobbie, E. A., and Krogh, P. H. (2009b). Nutrient allocations and metabolism in two collembolans with contrasting reproduction and growth strategies. Funct. Ecol. 23, 745–755.
Larsen, T., Pollierer, M. M., Holmstrup, M., D’annibale, A., Maraldo, K., Andersen, N., et al. (2016a). Substantial nutritional contribution of bacterial amino acids to earthworms and enchytraeids: a case study from organic grasslands. Soil Biol. Biochem. 99, 21–27.
Larsen, T., Ventura, M., Maraldo, K., Triadó-Margarit, X., Casamayor, E. O., and Wang, Y. V. (2016b). The dominant detritus–feeding invertebrate in Arctic peat soils derives its essential amino acids from gut symbionts. J. Anim. Ecol. 85, 1275–1285. doi: 10.1111/1365-2656.12563
Li, P., Mai, K., Trushenski, J., and Wu, G. (2009). New developments in fish amino acid nutrition: towards functional and environmentally oriented aquafeeds. Amino Acids 37, 43–53. doi: 10.1007/s00726-008-0171-1
Liu, H.-Z., Luo, L., and Cai, D.-L. (2018). Stable carbon isotopic analysis of amino acids in a simplified food chain consisting of the green alga Chlorella spp., the calanoid copepod Calanus sinicus, and the Japanese anchovy (Engraulis japonicus). Can. J. Zool. 96, 23–30.
Lucas, S., Chen, G., Aras, S., and Wang, J. (2018). Serine catabolism is essential to maintain mitochondrial respiration in mammalian cells. Life Sci. Alliance 1:e201800036. doi: 10.26508/lsa.201800036
Marrion, O., Schwertz, A., Fleurence, J., Guéant, J. L., and Villaume, C. (2003). Improvement of the digestibility of the proteins of the red alga Palmaria palmata by physical processes and fermentation. J. Food Nahrung 47, 339–344. doi: 10.1002/food.200390078
McCullagh, J., Gaye-Siessegger, J., and Focken, U. (2008). Determination of underivatized amino acid δ13C by liquid chromatography/isotope ratio mass spectrometry for nutritional studies: the effect of dietary non-essential amino acid profile on the isotopic signature of individual amino acids in fish. Rapid Commun. Mass Spectrom. 22, 1817–1822. doi: 10.1002/rcm.3554
McMahon, K. W., Fogel, M. L., Elsdon, T. S., and Thorrold, S. R. (2010). Carbon isotope fractionation of amino acids in fish muscle reflects biosynthesis and isotopic routing from dietary protein. J. Anim. Ecol. 79, 1132–1141. doi: 10.1111/j.1365-2656.2010.01722.x
McMahon, K., Thorrold, S., Houghton, L., and Berumen, M. (2015a). Tracing carbon flow through coral reef food webs using a compound-specific stable isotope approach. Oecologia 180, 809–821. doi: 10.1007/s00442-015-3475-3
McMahon, K. W., Mccarthy, M. D., Sherwood, O. A., Larsen, T., and Guilderson, T. P. (2015b). Millennial-scale plankton regime shifts in the subtropical North Pacific Ocean. Science 350, 1530–1533. doi: 10.1126/science.aaa9942
McMahon, K. W., Polito, M. J., Abel, S., Mccarthy, M. D., and Thorrold, S. R. (2015c). Carbon and nitrogen isotope fractionation of amino acids in an avian marine predator, the gentoo penguin (Pygoscelis papua). Ecol. Evol. 5, 1278–1290. doi: 10.1002/ece3.1437
Meier-Augenstein, W. (2018). Stable Isotope Forensics: An Introduction to the Forensic Application of Stable Isotope Analysis. Chichester, UK: John Wiley & Sons.
Meier-Augenstein, W., and Schimmelmann, A. (2019). A guide for proper utilisation of stable isotope reference materials. Isotopes Environ. Health Stud. 55, 113–128. doi: 10.1080/10256016.2018.1538137
Melzer, E., and Schmidt, H. L. (1987). Carbon isotope effects on the pyruvate-dehydrogenase reaction and their importance for relative 13C depletion in lipids. J. Biol. Chem. 262, 8159–8164.
Molkentin, J., Meisel, H., Lehmann, I., and Rehbein, H. (2007). Identification of organically farmed Atlantic salmon by analysis of stable isotopes and fatty acids. Eur. Food Res. Technol. 224, 535–543.
Moroney, N. C., Wan, A. H., Soler-Vila, A., Fitzgerald, R. D., Johnson, M. P., and Kerry, J. P. (2015). Inclusion of Palmaria palmata (red seaweed) in Atlantic salmon diets: effects on the quality, shelf-life parameters and sensory properties of fresh and cooked salmon fillets. J. Sci. Food Agric. 95, 897–905. doi: 10.1002/jsfa.6753
National Food Institute, Technical University of Denmark, Denmark, Monteiro, M., Sloth, J., Holdt, S., et al. (2019). Analysis and risk assessment of seaweed. EFSA J. 17:e170915. doi: 10.2903/j.efsa.2019.e170915
Newsome, S. D., Feeser, K. L., Bradley, C. J., Wolf, C., Takacs-Vesbach, C., and Fogel, M. L. (2020). Isotopic and genetic methods reveal the role of the gut microbiome in mammalian host essential amino acid metabolism. J. Proc. R. Soc. B 287:20192995. doi: 10.1098/rspb.2019.2995
Newsome, S. D., Fogel, M. L., Kelly, L., and Del Rio, C. M. (2011). Contributions of direct incorporation from diet and microbial amino acids to protein synthesis in Nile tilapia. Funct. Ecol. 25, 1051–1062.
Newsome, S. D., Wolf, N., Peters, J., and Fogel, M. L. (2014). Amino Acid δ13C analysis shows flexibility in the routing of dietary protein and lipids to the tissue of an omnivore. Integr. Org. Biol. 54, 890–902. doi: 10.1093/icb/icu106
Nham Tran, T. L., Miranda, A. F., Gupta, A., Puri, M., Ball, A. S., Adhikari, B., et al. (2020). The nutritional and pharmacological potential of New Australian thraustochytrids isolated from mangrove sediments. Mar. Drugs 18:151. doi: 10.3390/md18030151
Nomura, M., Kamogawa, H., Susanto, E., Kawagoe, C., Yasui, H., Saga, N., et al. (2013). Seasonal variations of total lipids, fatty acid composition, and fucoxanthin contents of Sargassum horneri (Turner) and Cystoseira hakodatensis (Yendo) from the northern seashore of Japan. J. Appl. Phyco. 25, 1159–1169.
Okun, J. G., Rusu, P. M., Chan, A. Y., Wu, Y., Yap, Y. W., Sharkie, T., et al. (2021). Liver alanine catabolism promotes skeletal muscle atrophy and hyperglycaemia in type 2 diabetes. Nat. Metabol. 3, 394–409. doi: 10.1038/s42255-021-00369-9
Omont, A., Py, C., Gamboa-Delgado, J., Nolasco-Soria, H., Spanopoulos-Zarco, M., and Peña-Rodríguez, A. (2021). Nutritional contribution of seaweed Ulva lactuca single-cell detritus and microalgae Chaetoceros calcitrans to the growth of the Pacific oyster Crassostrea gigas. J. Aquacul. 541:736835.
Paul, D., Skrzypek, G., and Fórizs, I. (2007). Normalization of measured stable isotopic compositions to isotope reference scales – a review. Rapid Commun. Mass Spectrom. 21, 3006–3014. doi: 10.1002/rcm.3185
Peres, H., and Oliva-Teles, A. (2006). Effect of the dietary essential to non-essential amino acid ratio on growth, feed utilization and nitrogen metabolism of European sea bass (Dicentrarchus labrax). J. Genome Biol. 256, 395–402.
Pollierer, M. M., Larsen, T., Potapov, A., Brückner, A., Heethoff, M., Dyckmans, J., et al. (2019). Compound-specific isotope analysis of amino acids as a new tool to uncover trophic chains in soil food webs. Ecol. Monogr. 89:e01384.
Powell, M. D., Ransome, J., Barney, M., Duijf, R. M., and Flik, G. (2007). Effect of dietary inclusion of N–Acetyl cysteine on mucus viscosity and susceptibility of rainbow trout, Oncorhynchus mykiss, and Atlantic Salmon, Salmo salar, to Amoebic gill disease. J. World Aquac. Soc. 38, 435–442.
Prabhu, A., Sarcar, B., Kahali, S., Yuan, Z., Johnson, J. J., Adam, K.-P., et al. (2014). Cysteine catabolism: a novel metabolic pathway contributing to glioblastoma growth. Cancer Res. 74, 787–796. doi: 10.1158/0008-5472.CAN-13-1423
Rakowska, R., Sadowska, A., Dybkowska, E., and Świderski, F. (2017). Spent yeast as natural source of functional food additives. Rocz. Panstw. Zakl. Hig. 68, 115–121.
Reeds, P. J. (2000). Dispensable and indispensable amino acids for humans. J. Nutr. 130, 1835s–1840s. doi: 10.1093/jn/130.7.1835S
Riley, W. Jr., Higgs, D., Dosanjh, B., and Eales, J. (1996). Influence of dietary arginine and glycine content on thyroid function and growth of juvenile rainbow trout, Oncorhynchus mykiss (Walbaum). Aquac. Nutr. 2, 235–242.
Roberfroid, M. (2007). Prebiotics: the concept revisited. J. Nutr. 137, 830S–837S. doi: 10.1093/jn/137.3.830S
Robinson, J. R., Rowan, J., Barr, W. A., and Sponheimer, M. (2021). Intrataxonomic trends in herbivore enamel δ13C are decoupled from ecosystem woody cover. Nat. Ecol. Evol. 5, 995–1002. doi: 10.1038/s41559-021-01455-7
Rogers, M., Bare, R., Gray, A., Scott-Moelder, T., and Heintz, R. (2019). Assessment of two feeds on survival, proximate composition, and amino acid carbon isotope discrimination in hatchery-reared Chinook salmon. Fish. Res. 219:105303.
Rothe, M., and Blaut, M. (2013). Evolution of the gut microbiota and the influence of diet. Benef. Microbes 4, 31–37.
Rungruangsak-Torrissen, K., Sunde, J., Berg, A. E., Nordgarden, U., Fjelldal, P. G., and Oppedal, F. (2009). Digestive efficiency, free amino acid pools and quality of growth performance in Atlantic salmon (Salmo salar L.) affected by light regimes and vaccine types. Fish. Physiol. Biochem. 35, 255–272. doi: 10.1007/s10695-008-9206-5
Schleder, D. D., Da Rosa, J. R., Guimarães, A. M., Ramlov, F., Maraschin, M., Seiffert, W. Q., et al. (2017). Brown seaweeds as feed additive for white-leg shrimp: effects on thermal stress resistance, midgut microbiology, and immunology. J. Appl. Phycol. 29, 2471–2477.
Scott, J. H., O’Brien, D. M., Emerson, D., Sun, H., Mcdonald, G. D., Salgado, A., et al. (2006). An examination of the carbon isotope effects associated with amino acid biosynthesis. Astrobiology 6, 867–880. doi: 10.1089/ast.2006.6.867
Shamushaki, V.a.J, Kasumyan, A. O., Abedian, A., and Abtahi, B. (2007). Behavioural responses of the Persian sturgeon (Acipenser persicus) juveniles to free amino acid solutions. Mar. Freshw. Behav. Physiol. 40, 219–224.
Smith, C., Fuller, B., Choy, K., and Richards, M. (2009). A three-phase liquid chromatographic method for δ13C analysis of amino acids from biological protein hydrolysates using liquid chromatography-isotope ratio mass spectrometry. Anal. Biochem. 390, 165–172. doi: 10.1016/j.ab.2009.04.014
Steinberg, P. D. (1989). Biogeographical variation in brown algal polyphenolics and other secondary metabolites: comparison between temperate Australasia and North America. J. Oecol. 78, 373–382. doi: 10.1007/BF00379112
Stücheli, P. E., Larsen, T., Wehrli, B., and Schubert, C. J. (2021). Amino acid and chlorin based degradation indicators in freshwater systems. Geochim. Cosmochim. Acta 304, 216–233.
Takano, Y., Chikaraishi, Y., Imachi, H., Miyairi, Y., Ogawa, N. O., Kaneko, M., et al. (2018). Insight into anaerobic methanotrophy from 13C/12C- amino acids and 14C/12C-ANME cells in seafloor microbial ecology. Sci. Rep. 8:14070. doi: 10.1038/s41598-018-31004-5
Takizawa, Y., Takano, Y., Choi, B., Dharampal, P. S., Steffan, S. A., Ogawa, N. O., et al. (2020). A new insight into isotopic fractionation associated with decarboxylation in organisms: implications for amino acid isotope approaches in biogeoscience. Prog. Earth Planet. Sci. 7:50.
Thépot, V., Campbell, A. H., Rimmer, M. A., Jelocnik, M., Johnston, C., Evans, B., et al. (2022). Dietary inclusion of the red seaweed Asparagopsis taxiformis boosts production, stimulates immune response and modulates gut microbiota in Atlantic salmon. Salmo. Salar. Aquac. 546:737286.
Thépot, V., Campbell, A. H., Rimmer, M. A., and Paul, N. A. (2021). Meta-analysis of the use of seaweeds and their extracts as immunostimulants for fish: a systematic review. Rev. Aquac. 13, 907–933.
Thinkstep (2015). Cradle-to-Gate Assessment of Whole Algal Protein Products. Preliminary Analysis Pending Critical Review. Stuttgart: Thinkstep
Tieszen, L. L., Boutton, T. W., Tesdahl, K. G., and Slade, N. A. (1983). Fractionation and turnover of stable carbon isotopes in animal-tissues - implications for δ13C analysis of diet. Oecologia 57, 32–37. doi: 10.1007/BF00379558
Todorčević, M., Kjær, M. A., Djaković, N., Vegusdal, A., Torstensen, B. E., Ruyter, B. J. C. B., et al. (2009). N-3 HUFAs affect fat deposition, susceptibility to oxidative stress, and apoptosis in Atlantic salmon visceral adipose tissue. Comp. Biochem. Physiol. B Biochem. Mol. Biol. 152, 135–143. doi: 10.1016/j.cbpb.2008.10.009
van Leeuwen, K. A., Prenzler, P. D., Ryan, D., and Camin, F. (2014). Gas chromatography-combustion-isotope ratio mass spectrometry for traceability and authenticity in foods and beverages. Compr. Rev. Food Sci. Food Saf. 13, 814–837. doi: 10.1111/1541-4337.12096
Vavricka, C. J., Han, Q., Mehere, P., Ding, H., Christensen, B. M., and Li, J. (2014). Tyrosine metabolic enzymes from insects and mammals: a comparative perspective. Insect Sci. 21, 13–19. doi: 10.1111/1744-7917.12038
Walker, M. C., and Van Der Donk, W. A. (2016). The many roles of glutamate in metabolism. J. Industrial Microbiol. Biotechnol. 43, 419–430. doi: 10.1007/s10295-015-1665-y
Wan, A. H., Davies, S. J., Soler–Vila, A., Fitzgerald, R., and Johnson, M. P. (2018). Macroalgae as a sustainable aquafeed ingredient. Rev. Aquac. 11, 458–492.
Wan, A. H. L., Soler-Vila, A., O’keeffe, D., Casburn, P., Fitzgerald, R., and Johnson, M. P. (2016). The inclusion of Palmaria palmata macroalgae in Atlantic salmon (Salmo salar) diets: effects on growth, haematology, immunity and liver function. J. Appl. Phycol. 28, 3091–3100.
Wang, A. R., Ran, C., Ringø, E., and Zhou, Z. G. (2018a). Progress in fish gastrointestinal microbiota research. Rev. Aquac. 10, 626–640.
Wang, Y. V., Wan, A. H. L., Lock, E.-J., Andersen, N., Winter-Schuh, C., and Larsen, T. (2018b). Know your fish: a novel compound-specific isotope approach for tracing wild and farmed salmon. Food Chem. 256, 380–389. doi: 10.1016/j.foodchem.2018.02.095
Wang, Y. V., Wan, A. H. L., Krogdahl, Å, Johnson, M., and Larsen, T. (2019). 13C values of glycolytic amino acids as indicators of carbohydrate utilization in carnivorous fish. PeerJ 7:e7701. doi: 10.7717/peerj.7701
Weber, D., Kexel, H., and Schmidt, H. L. (1997). 13C-Pattern of natural glycerol:? origin and practical importance. J, Agric. Food Chem. 45, 2042–2046.
Whiteman, J. P., Kim, S. L., McMahon, K. W., Koch, P. L., and Newsome, S. D. (2018). Amino acid isotope discrimination factors for a carnivore: physiological insights from leopard sharks and their diet. Oecologia 188, 977–989. doi: 10.1007/s00442-018-4276-2
Wickham, H. (2016). ggplot2: Elegant Graphics for Data Analysis. Switzerland: Springer International Publishing.
Womack, M., and Rose, W. C. (1947). The role of proline, hydroxyproline, and glutamic acid in growth. J. Biol. Chem. 171, 37–50. doi: 10.1084/jem.108.5.617
Wu, G. (1998). Intestinal mucosal amino acid catabolism. J. Nutr. 128, 1249–1252. doi: 10.1093/jn/128.8.1249
Wu, G. (2014). Dietary requirements of synthesizable amino acids by animals: a paradigm shift in protein nutrition. J. Anim. Sci. Biotechnol. 5:34. doi: 10.1186/2049-1891-5-34
Wu, G., Bazer, F. W., Burghardt, R. C., Johnson, G. A., Kim, S. W., Knabe, D. A., et al. (2011). Proline and hydroxyproline metabolism: implications for animal and human nutrition. Amino acids 40, 1053–1063. doi: 10.1007/s00726-010-0715-z
Wu, G., Flynn, N. E., and Knabe, D. A. (2000). Enhanced intestinal synthesis of polyamines from proline in cortisol-treated piglets. Am. J. Physiol.-Endocrinol. Metabol. 279, E395–E402. doi: 10.1152/ajpendo.2000.279.2.E395
Xiao, X., Agusti, S., Lin, F., Li, K., Pan, Y., Yu, Y., et al. (2017). Nutrient removal from Chinese coastal waters by large-scale seaweed Aquac ure. Sci. Rep. 7:46613. doi: 10.1038/srep46613
Xu, D., Liu, J., Gu, Y., Chen, Y., Zhao, C., Sun, G., et al. (2021). Biosynthesis and isotopic routing of dietary protein by sea cucumber Apostichopus japonicus (Selenka): evidence from compound-specific carbon stable isotope analysis. J. Agric. Food Chem. 69, 14802–14809. doi: 10.1021/acs.jafc.1c04731
Xu, S.-Y., Huang, X., and Cheong, K.-L. (2017). Recent advances in marine algae polysaccharides: isolation, structure, and activities. Mar. Drugs 15:388. doi: 10.3390/md15120388
Yan, N., and Wang, Y. (2019). Catalyst: is the amino acid a new frontier for biorefineries? Chem 5, 739–741.
Keywords: macroalgae, aquafeed, metabolism, salmon, isotope fingerprinting, nutrition, essential and non-essential amino acids
Citation: Larsen T, Wang YV and Wan AHL (2022) Tracing the Trophic Fate of Aquafeed Macronutrients With Carbon Isotope Ratios of Amino Acids. Front. Mar. Sci. 9:813961. doi: 10.3389/fmars.2022.813961
Received: 12 November 2021; Accepted: 17 January 2022;
Published: 09 February 2022.
Edited by:
Ikram Belghit, Norwegian Institute of Marine Research (IMR), NorwayReviewed by:
Yiou Zhu, Norwegian Institute of Marine Research (IMR), NorwayJulián Gamboa, Autonomous University of Nuevo León, Mexico
Kelton McMahon, University of Rhode Island, United States
Copyright © 2022 Larsen, Wang and Wan. This is an open-access article distributed under the terms of the Creative Commons Attribution License (CC BY). The use, distribution or reproduction in other forums is permitted, provided the original author(s) and the copyright owner(s) are credited and that the original publication in this journal is cited, in accordance with accepted academic practice. No use, distribution or reproduction is permitted which does not comply with these terms.
*Correspondence: Thomas Larsen, bGFyc2VuQHNoaC5tcGcuZGU=