- 1Department of Biology, HADAL & Nordcee, University of Southern Denmark, Odense, Denmark
- 2Marine Biological Section, Department of Biology, University of Copenhagen, Helsingør, Denmark
- 3Department of Microbiology and Immunology, University of British Columbia, Vancouver, BC, Canada
- 4Department of Earth, Marine and Environmental Sciences, University of North Carolina, Chapel Hill, Chapel Hill, NC, United States
- 5Danish Institute for Advanced Study, University of Southern Denmark, Odense, Denmark
- 6Tokyo University of Marine Science and Technology, Tokyo, Japan
- 7Graduate Program in Bioinformatics, University of British Columbia, Vancouver, BC, Canada
- 8Genome Science and Technology Program, University of British Columbia, Vancouver, BC, Canada
- 9Life Sciences Institute, University of British Columbia, Vancouver, BC, Canada
- 10ECOSCOPE Training Program, University of British Columbia, Vancouver, BC, Canada
Extracellular enzyme activity is a well-established parameter for evaluating microbial biogeochemical roles in marine ecosystems. The presence and activity of extracellular enzymes in seawater provide insights into the quality and quantity of organic matter being processed by the present microorganisms. A key challenge in our understanding of these processes is to decode the extracellular enzyme repertoire and activities of natural communities at the single-cell level. Current measurements are carried out on bulk or size-fractionated samples capturing activities of mixed populations. This approach – even with size-fractionation – cannot be used to trace enzymes back to their producers, nor distinguish the active microbial members, leading to a disconnect between measured activities and the producer cells. By targeting extracellular enzymes and resolving their activities at the single-cell level, we can investigate underlying phenotypic heterogeneity among clonal or closely related organisms, characterize enzyme kinetics under varying environmental conditions, and resolve spatio-temporal distribution of individual enzyme producers within natural communities. In this perspective piece, we discuss state-of-the-art technologies in the fields of microfluidic droplets and functional screening of prokaryotic cells for measuring enzyme activity in marine seawater samples, one cell at a time. We further elaborate on how this single-cell approach can be used to address research questions that cannot be answered with current methods, as pertinent to the enzymatic degradation of organic matter by marine microorganisms.
Introduction
Through their heterotrophic activities, marine microorganisms play key roles in regulating the balance between the ∼700 Pg of organic carbon stored in the oceanic dissolved organic matter (DOM) pool and the roughly equivalent amount of carbon stored as carbon dioxide (CO2) in the atmosphere. Microbial control of organic matter remineralization ranges from the initial enzymatic breakdown of polymers to their final respiration to inorganic carbon. To catalyze the initial degradation of high molecular weight organic compounds, microorganisms produce extracellular enzymes (EE), which may be localized on the cell surface or detached and released into the extracellular matrix. The activities of EEs are generally substrate-specific and produce molecules of sufficiently small sizes (ca. <600 Da) to be imported into the cell (Weiss et al., 1991), transformed, and used for biomass production or respiration. Thus, microbial enzymatic capabilities act as a gatekeeper for early diagenesis by regulating the bioavailability of organic molecules from its production in surface waters and ultimately down to the fraction of organic matter that escapes degradation, which can potentially be sequestered over longer timescales within marine sediments.
Extracellular enzyme activities in marine systems are most commonly measured using commercially available substrate proxies labeled with fluorogenic molecules that have changed little in terms of structure and complexity since their first usage almost four decades ago (Hoppe, 1983). EEs hydrolyze the fluorophore-substrate bond, and activity is inferred from measuring the increase in fluorescence in a sample over time. A different method uses fluorescently labeled versions of naturally occurring polysaccharides to better estimate EE activities on complex substrates; however, as these labeled substrates constantly fluoresce, their hydrolysis is measured through changes in substrate molecular weight using gel permeation chromatography (Arnosti, 2003). Similarly, another method makes use of fluorescent lucifer yellow derivatives to measure peptidase activities in natural samples (Pantoja et al., 1997). The use of these methods across numerous marine habitats (e.g., water column, particles, sediments, sea-ice) has yielded significant information on the diverse enzymatic capabilities of bacterial isolates (Bong et al., 2013) and natural microbial communities (Arnosti et al., 2005; Mahmoudi et al., 2020; Balmonte et al., 2021). However, since measured rates reflect the activities of complex communities when used with environmental samples, they only provide correlation-based answers to questions about functional diversity, enzymatic regulation or identifying producer cells and relevant genes for this process. Addressing such questions requires direct measurements at the single-cell level in order to better link genotype to phenotype, a key goal for the field of microbial ecology and coined as “next-generation physiology” (Hatzenpichler et al., 2020). The importance of this approach is emphasized by recent work (Reintjes et al., 2017), which discovered a selfish uptake and hydrolysis mechanism in specific bacterial taxa by using fluorescently labeled polysaccharides. This uptake mechanism, however, was only used by a subset of the microbial community; developing a method that can link enzyme activities to a wider range of cells at the population and community levels of biological organization therefore remains a high priority.
Beyond the identification of active cells, linking measured activities to their encoding genes remains a challenge, especially when the information is obtained from natural communities. This challenge is due in part to the high abundance of marine pelagic microbes, on the order of 103 to 106 cells mL–1 (Wigington et al., 2016), as well as to their little understood genomic diversity and their limited representation in experimentally validated reference databases. Progress in single-cell technology – from cell capture, to sorting, to genome sequencing (e.g., Woyke et al., 2009; Martinez-Garcia et al., 2012; Doud et al., 2020) – has provided new insights into the phylogenetic diversity and functionality of individual taxa that comprise complex bacterial communities in surface seawater (Pachiadaki et al., 2019) without relying on culture-dependent methods. Furthermore, single-cell analyses can be cost-effective and efficient by taking a targeted approach that relies on identifying characteristic features (e.g., physiological or functional) on which to sort cells of interest. For example, the coupled use of fluorescent substrates and single-cell capture, sorting, and sequencing successfully identified microbial taxa that likely participate in polysaccharide degradation in seawater (Martinez-Garcia et al., 2012); the combination of fluorescently labeled polysaccharides and flow cytometric sorting is currently being used to identify “selfish” bacteria (see below) that hydrolyze specific polysaccharides (Giljan et al., in review). Another, related approach is activity-based protein profiling, which uses a three component probe: a reactive group designed to covalently bind to the active site on the targeted protein, a spacer group guiding the probe toward the protein, and a tag for detection (Sadler and Wright, 2015; Whidbey and Wright, 2019). This method has been used to identify bacterial cells with various glycoside hydrolase activities among pure cultures (Chauvigné-Hines et al., 2012), but has yet to be used to detect enzymatic capabilities of marine microbial communities. Work applying a single cell perspective brings to light the underlying cell-cell variability that is fundamental to understanding the manner in which microorganisms function at different levels of biological organization.
By leveraging state of the art methods in single-cell technology and microfluidics, process rate measurements at the individual cell level are within reach, particularly those necessary for our understanding of enzymatic hydrolysis of organic matter. We contend that microfluidic droplets offer a tractable platform for developing and standardizing a single-cell enzyme activity assay specifically adapted to handle the high diversity and heterogeneity of marine microbial communities (Figure 1). Single-cell level investigations of microbial enzyme-catalyzed degradation of organic matter, in turn, can provide new insights into the identities of EE producers in nature and the regulation of EE synthesis and secretion, and the range of cell-specific rates comprising measured bulk rates. This information will help parameterize trait-based models for predicting ecosystem process shifts with changing environmental conditions. Thus, developing single-cell enzyme assays paves the way for new research avenues that help resolve fundamental questions about the ecology, biogeochemistry, and evolution of microbial communities.
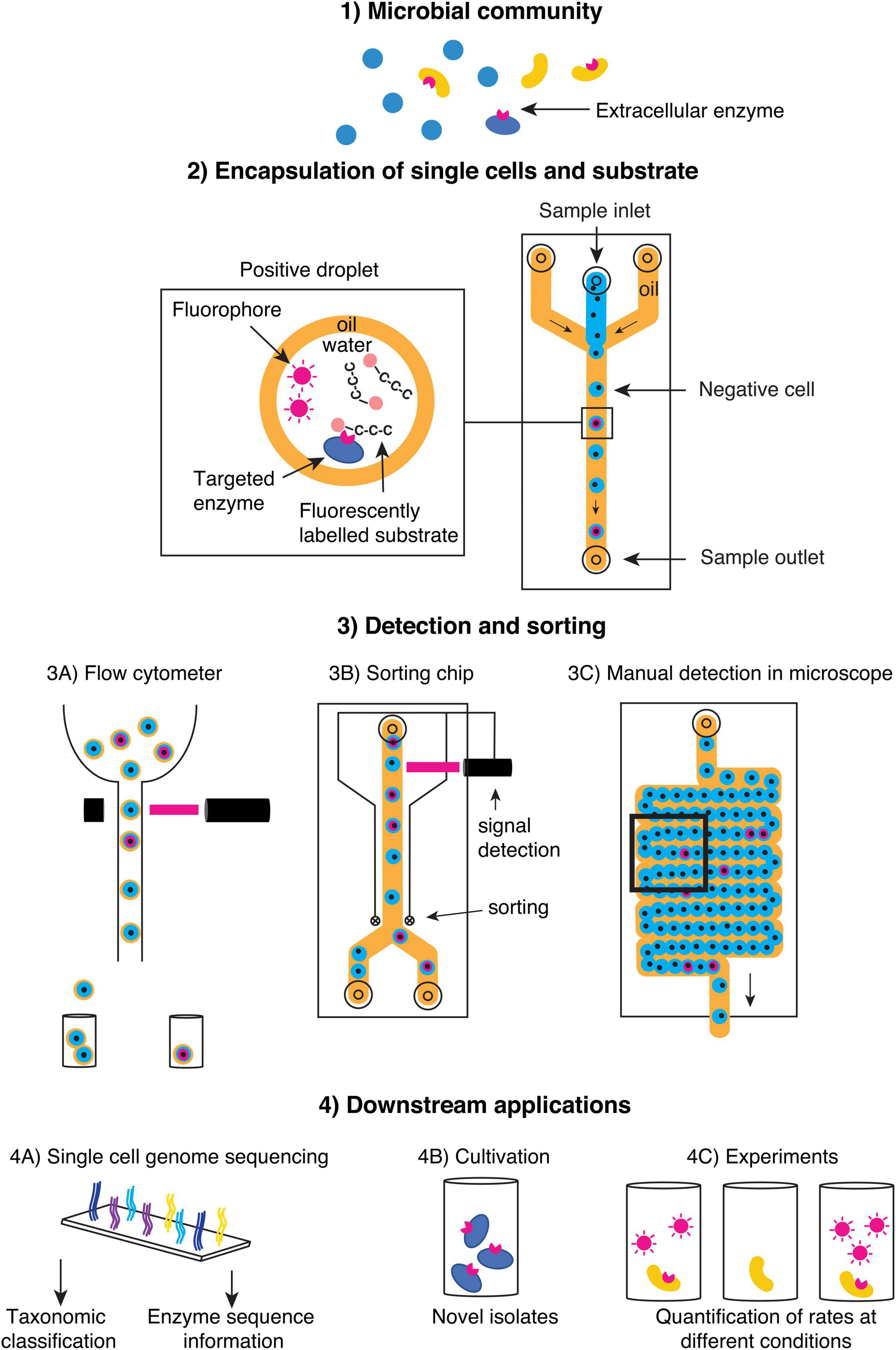
Figure 1. Schematic of a suggested workflow to screen single cells in (1) natural microbial communities using an extracellular enzyme assay in microfluidic droplets. (2) Single cells are encapsulated in droplets together with a labeled substrate, and the presence of extracellular enzyme activity can be detected by the release of fluorophores, which increases fluorescence in the droplet. Depending on the droplet type (oil-water as depicted in 3B,C or water-oil-water in 3A), various screening and sorting platforms can be applied. (4) Microfluidic droplets are biocompatible with a range of downstream applications such as sequencing (4A) or experiments requiring viable cells (4B,C).
An Emerging Technology for Single-Cell Enzyme Assays
Droplet-based microfluidics is an emerging technology with successful applications in enzymology, chemical engineering (Colin et al., 2015; Ma et al., 2016), molecular evolution (Tawfik and Griffiths, 1998), and cell cultivation (see also Joensson and Svahn, 2012 and references therein). Nakamura et al. (2016) has demonstrated the utility of droplets in screening marine microorganisms for specific enzyme activities with industrial potential, and developing this method to measure single-cell process rates offers substantial promise.
The basic premise of the method is that single cells can be encapsulated in picolitre to nanolitre sized water-oil droplets, along with any necessary reagents or substrate. Each droplet can be considered a separate screening “experiment,” with typically 105–107 droplets per sample. Considering the vast diversity and abundance of microorganisms, high throughput offered by the droplets approach is necessary if a microbial community is to be adequately represented within a screen. The small volume of each droplet dramatically reduces reagent use and is critical for assay sensitivity to enable detection at low enzyme concentration (Köster et al., 2008). After cell encapsulation, EEs stay in droplets, remaining associated with their producing cells (Agresti et al., 2010; Kintses et al., 2012).
Microfluidic chips reliably produce uniform water-oil droplets by pressure driven injection of an aqueous phase into a continuously flowing oil phase in a flow focusing (Figures 1,2) or T-junction geometry, and generally reach droplet generation frequencies between 0.1 and 10 kHz (Joensson and Svahn, 2012). Microfluidic chips are commonly manufactured in polydimethylsiloxane (PDMS), a soft elastomeric material (Joensson and Svahn, 2012). Polydimethylsiloxane chip designs are inexpensive to produce and can be created quickly from a master mold using soft lithography (Duffy et al., 1998). Water-oil droplets are generally made with either mineral or fluorinated oils, to which biocompatible surfactants are added to improve emulsion stability after droplet generation; several surfactants are biocompatible with different biological uses (Joensson and Svahn, 2012). As a caveat, small hydrophobic molecules from the aqueous phase can escape into the oil and be exchanged between droplets, as they are not impenetrable compartments.
Despite diverse applications of microfluidic droplets in chemistry and biology (Joensson and Svahn, 2012), including work illustrating viable uses for activity-based screening, droplet-based enzyme assays are still in early stages of development (Guan et al., 2014; Ma et al., 2016; Nakamura et al., 2016; Terekhov et al., 2017). Adapting EE assays to a droplet-based platform can follow the same broad principles of current methods for measuring bulk activity: A seawater sample is mixed with a fluorescently labeled substrate before each cell is encapsulated and screened. The number of cells in each droplet is determined by the Poisson distribution; hence, by controlling cell concentration, droplets can be tuned to contain single cells. Droplets showing the desired activity can be retrieved for later analysis by fluorescence activated droplet sorting (Baret et al., 2009), allowing information (i.e., activity) and material (e.g., cell, enzyme, DNA) to be captured from the same sample. In the case of double emulsion, water-oil-water droplets, sorting can be accomplished using FACS. Otherwise, dedicated microfluidic droplet sorters can be used (Sciambi and Abate, 2015). It is also possible to study the kinetics of reactions by incubating the droplets in a storage chip, such as the one described by Labanieh et al. (2015) and observing fluorescence changes over time on a microscope. Droplets can later be retrieved from storage chips and sorted as described above.
The approach of capturing single cells in microfluidic droplets comes with several caveats. First, compartmentalizing single cells limits insights into multi-cell dependent interactions, such as coordination of degradation induced by quorum sensing (Hmelo et al., 2011; Krupke et al., 2016). Moreover, the microdroplet assays proposed here are predisposed to some of the same limitations as the methods that use microplates or cuvettes, including questions about the extent to which activity measurements under substrate saturation accurately represent EE activities in nature.
Droplet-Based Extracellular Enzyme Assays can Address Unsolved Questions
The single-cell approach enables direct investigations of the manner in which genes, physiology, and environment act concertedly on individual cells, bypassing limitations inherent to bulk community measurements, and advancing our understanding of microbial control of organic matter degradation at different levels of biological organization. The resolution afforded by single-cell enzyme assays also provides the opportunity to investigate cell-cell variability within populations, including inherent cellular plasticity among individual cells, due to genomic, transcriptomic, and phenotypic heterogeneity even among clonal populations (encompassed within the field of “quantal microbiology”; Bridson and Gould, 2000). This multi-level heterogeneity facilitates microbial adaptation to and survival under changing conditions, but also challenges our current understanding of the roles played by microbes in many biogeochemical processes, including the enzymatic degradation of organic matter. Thus, we identify and discuss several questions about microbial enzymatic activities that have persisted due to challenges related to microbial heterogeneity, but which may be resolved using droplet-based screening. These questions include the following: (i) How is the diversity in EE encoding genotypes reflected in enzymatic activities? (ii) How many cells in a microbial community actively express genes for EEs, and what environmental conditions control EE production at the cellular level? (iii) What fraction of measurable enzymatic activities comes from cell-free EEs, and what factors regulate the switch to dissolved EE production? (iv) What is the distribution of cell-specific rates of EE activities, and how do these vary along environmental gradients or among different phylogenetic groups?
How Is the Diversity in Extracellular Enzyme Genotypes Reflected in Enzymatic Activities?
While EEs may exhibit varying degrees of substrate promiscuity (Khersonsky and Tawfik, 2010; Steen et al., 2015; Srivastava et al., 2021), the ability to detect EE activity in single cells enables direct coupling to a labeled organismal reference genome and facilitates high-throughput identification of the gene or gene cassette encoding the activity of interest. Resulting data sets have the potential to improve reference databases against which (meta)genomic and (meta)transcriptomic data are annotated (Quince et al., 2017; Forster et al., 2019). This effort is especially necessary for marine microbial communities, as they harbor high sequence complexity, and specifically with regards to EE genes, which have high genotypic diversity within and across microbial phylogenetic groups (Elifantz et al., 2008; Martinez-Garcia et al., 2012; Zimmerman et al., 2013). Investigating how EE gene diversity relates to different ecosystems (Berlemont and Martiny, 2016), including the quantity and quality of available organic matter, advances our understanding of the functional biogeography of microbial EEs. Integrating single-cell genomic to phenotypic information also contributes to our understanding of the manner in which different genotypes of functionally similar EEs yield different enzyme kinetics, substrate specificities (Steen et al., 2015; Srivastava et al., 2021), and temperature optima (Huston et al., 2000). Finally, by linking EE activities more closely to specific microbial genotypes, a functional interpretation may be possible for the massive amounts of publicly available amplicon datasets covering a wide range of systems, experiments, and spatiotemporal scales.
How Many Cells in a Microbial Community Actively Express Extracellular Enzymes, and What Environmental Conditions Control Extracellular Enzyme Production at the Cellular Level?
Droplet-based screening can be used to quantify the proportion of microbial cells that actively produce specific enzymes. Information on the fraction of cells contributing to bulk activity is of critical importance for understanding patterns of activity (Arnosti, 2008; Arnosti et al., 2011) and to provide data for biogeochemical models that incorporate features of microbial communities (Zakem et al., 2021). Delving into the underlying factors controlling expression, additional insights into critical questions may be gained by coupling single-cell activity detection with transcriptomic sequencing. First, what is the link between gene transcription and detectable phenotype? More specifically, does the expression of genes for enzyme production directly result in measurable rates of enzymatic activities, or do post-transcriptional or translational modifications preclude a direct connection and interpretation between transcription and measurable activity? A weak relationship between the abundance of genes that encode proteins and measurable rates suggests that direct correlations between transcription and phenotype interpretations may be problematic (Rocca et al., 2015) but the extent to which this situation applies to EEs and their activities remains unconstrained.
Applying this coupled approach to clonal populations can provide insights into a second critical question: what are the varying degrees of population-level heterogeneity in gene transcription and measurable phenotypes for diverse enzymatic processes? Studies indicate substantial transcriptional heterogeneity that leads to differences in microbial phenotype (Ackermann, 2015), and recent advances in single-cell bacterial RNA sequencing for bacterial cells in culture (Imdahl et al., 2020) can be leveraged to investigate similar questions applicable to enzymatic degradation of organic matter. As extracellular enzymes in many cases can be considered as public goods, particularly when released into the environment, studies on the evolution of cooperation and cheating (Reintjes et al., 2019) can guide investigations on whether similar dynamics are applicable to the production of enzymes among clonal populations (Baty et al., 2000a,b).
Changes in environmental conditions, cell density, and resource availability and complexity can have profound consequences on transcription, and within the context of the degradation of organic matter, can shift the predominant form of enzymatic hydrolysis of different substrates (reviewed in Arnosti et al., 2021). A “biphasic phenotype” – switching transcription of genes for polysaccharide utilization loci and enzyme production based on the availability of preferred substrates – demonstrates that enzymatic strategies are finely tuned to environmental stimuli (Koch et al., 2019). During a phytoplankton bloom, microbial community and functional succession (Teeling et al., 2012) co-occurs with a shift in the primary mode of substrate hydrolysis, dominated by low “selfish” activity in the early bloom phase, to increased “selfish” uptake of substrates approaching the peak of the bloom, to increased external hydrolysis of substrates in the late bloom phase (Reintjes et al., 2019). Resolving these processes at the single-cell level would reveal whether individual cells possess the flexibility to shift from one dominant form of substrate hydrolysis to another – and therefore can transcend boundaries of “life strategies” of substrate use (e.g., selfish bacteria, external hydrolyzers, and scavengers) (Arnosti et al., 2021) – or whether a community change is necessary for this process shift (Buchan et al., 2014). This information, consequently, also reveals the range of metabolic plasticity for organic matter degradation exhibited by individual cells and the extent to which this plasticity varies across phylogenetic groups.
What Fraction of Measurable Enzymatic Activities Comes From Cell-Free Extracellular Enzymes, and What Factors Regulate the Switch to Dissolved Extracellular Enzyme Production?
Secreted cell-free or dissolved enzymes can freely diffuse into the environmental matrix, potentially becoming decoupled from their producers, but they may nevertheless remain functional for some time. In marine systems, these enzymes may have lifetimes of days to weeks (Ziervogel et al., 2010; Steen and Arnosti, 2011), and theoretical estimates suggest potentially extended lifetimes in deep subsurface sediments (Schmidt et al., 2021). Bulk measures of enzymatic activities therefore integrate the activity of dissolved enzymes whose producer may not necessarily be present in the sample of interest. In the deep ocean, dissolved enzymes may account for the majority of measured enzymatic activity (Baltar et al., 2010). The transition from producing cell-attached to dissolved enzymes, or vice versa, may in part be determined by substrate concentration and the environment. Low diffusion environments and high concentrations of substrates, especially particulate matter, which require hydrolytic enzymes prior to microbial use, promote the production of dissolved enzymes (Vetter et al., 1998; Traving et al., 2015).
Current methodologies to investigate the presence, importance, and contribution of dissolved enzymes leave open unanswered questions. Previous studies have used size-fractionation facilitated by gentle vacuum filtration to separate and operationally define dissolved enzymes (<0.22 μm) from those that remain attached to cells (Baltar et al., 2010). However, even at low pressures, vacuum filtration may burst cells and release intracellular enzymes into the extracellular matrix, artefactually increasing dissolved enzyme contribution. Microdroplet encapsulation of single cells as well as freely dissolved enzymes would yield estimates of dissolved enzyme contributions unbiased by either intracellular or cell-attached enzymes. Another method of inferring dissolved enzyme production is through detection of transcripts encoding secretory enzymes (Zhao et al., 2020). But, as mentioned in the previous section, the extent to which gene expression results in a measurable phenotype may be complicated by post-transcriptional or post-translational processing. Leveraging the ability to capture and sequence single cell transcripts would help confirm that transcription of genes for secretory enzymes results in a measurable output. Expanding the analysis of transcripts beyond those for secretory enzymes could also provide insights into the extent to which other factors may help contribute to a switch in the mode of substrate hydrolysis. Investigating the dynamics and regulation of dissolved enzymes on a single-cell level would help advance our knowledge regarding this evolutionary conundrum of producing energetically costly enzymes that may decouple investment from returns.
What Is the Distribution of Cell-Specific Rates of Extracellular Enzyme Activities, and How Do These Vary Along Environmental Gradients or Different Phylogenetic Groups?
Cell-specific activities provide insights into the range of physiological states of individual cells in the environment. In the case of cell-specific enzymatic activity rates, these values are currently calculated by taking bulk enzymatic activities and normalizing these rates to cell abundance. However, this approach assumes that all cells produce extracellular enzymes and in equal quantities. In reality, even closely-related EE-producing cells can have distinct enzymatic capabilities (e.g., Xing et al., 2015). Moreover, the heterogeneity of physiological states in microbial cells, as well as potential contributions of dissolved enzymes from non-resident populations, can lead to deviations of calculated cell-specific rates from true cell-specific rates. The presence of dormant or metabolically inactive cells in a community would lead to underestimations of calculated cell-specific enzymatic activities. However, high contributions of dissolved enzymes in a water sample – which may have originated from non-resident taxa within a sample – may lead to an overestimation of cell-specific enzymatic activities. Inaccurate measures of cell-specific activities present a significant problem, especially when relating these rates to other cell-specific measures of heterotrophic activity, such as those for bacterial production and respiration, because of differences in the proportion of the community that carry out these distinct processes.
Developing a microfluidics approach to enable measurements of single cell enzymatic activities would provide more accurate measurements and reduce the problems of uninvolved, inactive, or dormant cells, and diffused dissolved enzymes. Whereas calculating a cell-specific enzymatic activity based on bulk measurements results in a single value, the true range of cell-specific enzymatic activities may encompass one or several orders of magnitude, typical of cell-specific respiration or bacterial production rates (Del Giorgio and Gasol, 2008). A feature that could emerge is a bimodal distribution of cell-specific enzymatic activities (Baty et al., 2000a,b), reflecting the broad categories of high nucleic acid and low nucleic acid cells typically observed among microbial communities (Bouvier et al., 2007; Del Giorgio and Gasol, 2008). Resolving the distribution of cell-specific enzymatic activities provides a better understanding of how microbial physiological states and resource acquisition strategies change in response to environmental shifts. For example, a hypothetical bimodal distribution may have a density peak of cell-specific activities in the lower range, reflecting the dominance of low-activity microorganisms that characterize much of the oceans. However, upon an environmental shift resulting in resource abundance for the community, changes in physiological states and resource acquisition strategies may alter the density peak toward the high end of the distribution, characteristic of a community succession driven by the dominance of fast-growing, opportunistic taxa.
Future and Broader Implications: An Example With Microbial Extracellular Enzymes in Marine Carbon Cycle Models
As EEs are necessary catalysts for marine microorganisms to access energy and nutrients derived from complex organic matter, single-cell information related to substrate-specific producers – including (de)coupling of genes and phenotypes, factors controlling enzyme production, contributions and lifetimes of dissolved enzymes, and cell-specific rates – can be used to refine and constrain models of processes within the marine carbon cycle. Additionally, coupling cell-specific enzymatic activity rates with other physiological data (e.g., cell size, volume, cell-specific bacterial production and respiration, etc.) advances efforts to parameterize trait-based models that may help project how microorganisms respond to environmental shifts and effect changes in carbon transformation and transport at local, regional, and global scales (Follows et al., 2007; Litchman et al., 2015). Interpreting and integrating the role of EEs from single cells to ecosystems will help establish a modeling framework applicable to other biogeochemical processes and in the recovery of useful enzymes with industrial applications.
Data Availability Statement
The original contributions presented in the study are included in the article/supplementary material, further inquiries can be directed to the corresponding authors.
Author Contributions
ST, JB, DS, CA, RG, SH, and MM all contributed to developing the work and ideas presented. All authors have contributed to the writing of this manuscript.
Funding
This work was funded by the Danish National Research Foundation through the Danish Center for Hadal Research, HADAL (No. DNRF145). CA was supported by NSF OCE-1736772 and OCE-2022952 as well as DOE DESC0019012. DS and SH were supported by Natural Sciences and Engineering Research Council (NSERC) of Canada, Genome British Columbia, the Canada Foundation for Innovation (CFI), and the U.S. Department of Energy (DOE) Joint Genome Institute (JGI) and the Facilities Integrating Collaborations for User Science (FICUS) JGI-EMSL (Environmental Molecular Sciences Laboratory) project (50967) supported by the Office of Science of US DOE Contract DE-AC02-05CH11231 with essential automation support through the Biofactorial High-Throughput Biology Facility in the Life Sciences Institute at the University of British Columbia.
Conflict of Interest
SH is a co-founder of Koonkie Inc., a bioinformatics consulting company that designs and provides scalable algorithmic and data analytics solutions in the cloud.
The remaining authors declare that the research was conducted in the absence of any commercial or financial relationships that could be construed as a potential conflict of interest.
Publisher’s Note
All claims expressed in this article are solely those of the authors and do not necessarily represent those of their affiliated organizations, or those of the publisher, the editors and the reviewers. Any product that may be evaluated in this article, or claim that may be made by its manufacturer, is not guaranteed or endorsed by the publisher.
References
Ackermann, M. (2015). A functional perspective on phenotypic heterogeneity in microorganisms. Nat. Rev. Microbiol. 13, 497–508. doi: 10.1038/nrmicro3491
Agresti, J. J., Antipov, E., Abate, A. R., Ahn, K., Rowat, A. C., Baret, J.-C., et al. (2010). Ultrahigh-throughput screening in drop-based microfluidics for directed evolution. Proc. Natl. Acad. Sci. U. S. A. 107, 4004–4009. doi: 10.1073/pnas.0910781107
Arnosti, C. (2003). Fluorescent derivatization of polysaccharides and carbohydrate-containing biopolymers for measurement of enzyme activities in complex media. J. Chromatogr. B Anal. Technol. Biomed. Life. Sci. 793, 181–191. doi: 10.1016/s1570-0232(03)00375-1
Arnosti, C. (2008). Functional differences between Arctic seawater and sedimentary microbial communities: contrasts in microbial hydrolysis of complex substrates. FEMS Microbiol. Ecol. 66, 343–351. doi: 10.1111/j.1574-6941.2008.00587.x
Arnosti, C., Durkin, S., and Jeffrey, W. H. (2005). Patterns of extracellular enzyme activities among pelagic marine microbial communities: implications for cycling of dissolved organic carbon. Aquat. Microb. Ecol. 38, 135–145. doi: 10.3354/ame038135
Arnosti, C., Steen, A. D., Ziervogel, K., Ghobrial, S., and Jeffrey, W. H. (2011). Latitudinal gradients in degradation of marine dissolved organic carbon. PLoS One 6:e28900. doi: 10.1371/journal.pone.0028900
Arnosti, C., Wietz, M., Brinkhoff, T., Hehemann, J.-H., Probandt, D., Zeugner, L., et al. (2021). The biogeochemistry of marine polysaccharides: sources, inventories, and bacterial drivers of the carbohydrate cycle. Annu. Rev. Mar. Sci. 13, 81–108. doi: 10.1146/annurev-marine-032020-012810
Balmonte, J. P., Simon, M., Giebel, H.-A., and Arnosti, C. (2021). A sea change in microbial enzymes: heterogeneous latitudinal and depth-related gradients in bulk water and particle-associated enzymatic activities from 30°S to 59°N in the Pacific Ocean. Limnol. Oceanogr. 66, 3489–3507. doi: 10.1002/lno.11894
Baltar, F., Arístegui, J., Gasol, J. M., Sintes, E., van Aken, H. M., and Herndl, G. J. (2010). High dissolved extracellular enzymatic activity in the deep central Atlantic Ocean. Aquat. Microb. Ecol. 58, 287–302. doi: 10.3354/ame01377
Baret, J.-C., Miller, O. J., Taly, V., Ryckelynck, M., El-Harrak, A., Frenz, L., et al. (2009). Fluorescence-activated droplet sorting (FADS): efficient microfluidic cell sorting based on enzymatic activity. Lab. Chip 9, 1850–1858. doi: 10.1039/B902504A
Baty, A. M., Eastburn, C. C., Diwu, Z., Techkarnjanaruk, S., Goodman, A. E., and Geesey, G. G. (2000a). Differentiation of Chitinase-active and non-Chitinase-active subpopulations of a marine bacterium during chitin degradation. Appl. Environ. Microbiol. 66, 3566–3573. doi: 10.1128/AEM.66.8.3566-3573.2000
Baty, A. M., Eastburn, C. C., Techkarnjanaruk, S., Goodman, A. E., and Geesey, G. G. (2000b). Spatial and temporal variations in chitinolytic gene expression and bacterial biomass production during Chitin degradation. Appl. Environ. Microbiol. 66, 3574–3585. doi: 10.1128/AEM.66.8.3574-3585.2000
Berlemont, R., and Martiny, A. C. (2016). Glycoside Hydrolases across environmental microbial communities. PLoS Comput. Biol. 12:e1005300. doi: 10.1371/journal.pcbi.1005300
Bong, C. W., Obayashi, Y., and Suzuki, S. (2013). Succession of protease activity in seawater and bacterial isolates during starvation in a mesocosm experiment. Aquat. Microb. Ecol. 69, 33–46. doi: 10.3354/ame01618
Bouvier, T., Del Giorgio, P. A., and Gasol, J. M. (2007). A comparative study of the cytometric characteristics of High and Low nucleic-acid bacterioplankton cells from different aquatic ecosystems. Environ. Microbiol. 9, 2050–2066. doi: 10.1111/j.1462-2920.2007.01321.x
Bridson, E. Y., and Gould, G. W. (2000). Quantal microbiology. Lett. Appl. Microbiol. 30, 95–98. doi: 10.1046/j.1472-765x.2000.00673.x
Buchan, A., LeCleir, G. R., Gulvik, C. A., and González, J. M. (2014). Master recyclers: features and functions of bacteria associated with phytoplankton blooms. Nat. Rev. Microbiol. 12, 686–698. doi: 10.1038/nrmicro3326
Chauvigné-Hines, L. M., Anderson, L. N., Weaver, H. M., Brown, J. N., Koech, P. K., Nicora, C. D., et al. (2012). Suite of activity-based probes for cellulose-degrading enzymes. J. Am. Chem. Soc. 134, 20521–20532. doi: 10.1021/ja309790w
Colin, P.-Y., Kintses, B., Gielen, F., Miton, C. M., Fischer, G., Mohamed, M. F., et al. (2015). Ultrahigh-throughput discovery of promiscuous enzymes by picodroplet functional metagenomics. Nat. Commun. 6:10008. doi: 10.1038/ncomms10008
Del Giorgio, P. A., and Gasol, J. M. (2008). “Physiological structure and single-cell activity in marine bacterioplankton,” in Microbial Ecology of the Oceans, ed. D. L. Kirchman (Hoboken: John Wiley & Sons, Inc.), 243–298. doi: 10.1002/9780470281840.ch8
Doud, D. F. R., Bowers, R. M., Schulz, F., De Raad, M., Deng, K., Tarver, A., et al. (2020). Function-driven single-cell genomics uncovers cellulose-degrading bacteria from the rare biosphere. ISME J. 14, 659–675. doi: 10.1038/s41396-019-0557-y
Duffy, D. C., McDonald, J. C., Schueller, O. J. A., and Whitesides, G. M. (1998). Rapid Prototyping of Microfluidic Systems in Poly(dimethylsiloxane). Anal. Chem. 70, 4974–4984. doi: 10.1021/ac980656z
Elifantz, H., Waidner, L. A., Cottrell, M. T., and Kirchman, D. L. (2008). Diversity and abundance of glycosyl hydrolase family 5 in the North Atlantic Ocean. FEMS Microbiol. Ecol. 63, 316–327. doi: 10.1111/j.1574-6941.2007.00429.x
Follows, M. J., Dutkiewicz, S., Grant, S., and Chisholm, S. W. (2007). Emergent biogeography of microbial communities in a model ocean. Science 315, 1843–1846. doi: 10.1126/science.1138544
Forster, S. C., Kumar, N., Anonye, B. O., Almeida, A., Viciani, E., Stares, M. D., et al. (2019). A human gut bacterial genome and culture collection for improved metagenomic analyses. Nat. Biotechnol. 37, 186–192. doi: 10.1038/s41587-018-0009-7
Giljan, G., Arnosti, C., Kirstein, I. V., Amann, R., and Fuchs, B. (in review). Environmental Microbiology.
Guan, Z., Zou, Y., Zhang, M., Lv, J., Shen, H., Yang, P., et al. (2014). A highly parallel microfluidic droplet method enabling single-molecule counting for digital enzyme detection. Biomicrofluidics 8:014110. doi: 10.1063/1.4866766
Hatzenpichler, R., Krukenberg, V., Spietz, R. L., and Jay, Z. J. (2020). Next-generation physiology approaches to study microbiome function at single cell level. Nat. Rev. Microbiol. 18, 241–256. doi: 10.1038/s41579-020-0323-1
Hmelo, L. R., Mincer, T. J., and Van Mooy, B. A. S. (2011). Possible influence of bacterial quorum sensing on the hydrolysis of sinking particulate organic carbon in marine environments. Environ. Microbiol. Rep. 3, 682–688. doi: 10.1111/j.1758-2229.2011.00281.x
Hoppe, H.-G. (1983). Significance of exoenzymatic activities in the ecology of brackish water: measurements by means of methylumbelliferyl-substrates. Mar. Ecol. Prog. Ser. 11, 299–308. doi: 10.3354/meps011299
Huston, A. L., Krieger-Brockett, B. B., and Deming, J. W. (2000). Remarkably low temperature optima for extracellular enzyme activity from Arctic bacteria and sea ice. Environ. Microbiol. 2, 383–388. doi: 10.1046/j.1462-2920.2000.00118.x
Imdahl, F., Vafadarnejad, E., Homberger, C., Saliba, A.-E., and Vogel, J. (2020). Single-cell RNA-sequencing reports growth-condition-specific global transcriptomes of individual bacteria. Nat. Microbiol. 5, 1202–1206. doi: 10.1038/s41564-020-0774-1
Joensson, H. N., and Svahn, H. A. (2012). Droplet microfluidics—a tool for single-cell analysis. Angew. Chem. Int. Ed. 51, 12176–12192. doi: 10.1002/anie.201200460
Khersonsky, O., and Tawfik, D. S. (2010). Enzyme promiscuity: a mechanistic and evolutionary perspective. Annu. Rev. Biochem. 79, 471–505. doi: 10.1146/annurev-biochem-030409-143718
Kintses, B., Hein, C., Mohamed, M. F., Fischlechner, M., Courtois, F., Lainé, C., et al. (2012). Picoliter cell lysate assays in microfluidic droplet compartments for directed enzyme evolution. Chem. Biol. 19, 1001–1009. doi: 10.1016/j.chembiol.2012.06.009
Koch, H., Dürwald, A., Schweder, T., Noriega-Ortega, B., Vidal-Melgosa, S., Hehemann, J.-H., et al. (2019). Biphasic cellular adaptations and ecological implications of Alteromonas macleodii degrading a mixture of algal polysaccharides. ISME J. 13, 92–103. doi: 10.1038/s41396-018-0252-4
Köster, S., Angilè, F. E., Duan, H., Agresti, J. J., Wintner, A., Schmitz, C., et al. (2008). Drop-based microfluidic devices for encapsulation of single cells. Lab. Chip 8, 1110–1115. doi: 10.1039/B802941E
Krupke, A., Hmelo, L. R., Ossolinski, J. E., Mincer, T. J., and Van Mooy, B. A. S. (2016). Quorum sensing plays a complex role in regulating the enzyme hydrolysis activity of microbes associated with sinking particles in the ocean. Front. Mar. Sci. 3:55. doi: 10.3389/fmars.2016.00055
Labanieh, L., Nguyen, T. N., Zhao, W., and Kang, D.-K. (2015). Floating droplet array: an ultrahigh-throughput device for droplet trapping, real-time analysis and recovery. Micromachines 6, 1469–1482. doi: 10.3390/mi6101431
Litchman, E., de Tezanos Pinto, P., Edwards, K. F., Klausmeier, C. A., Kremer, C. T., and Thomas, M. K. (2015). Global biogeochemical impacts of phytoplankton: a trait-based perspective. J. Ecol. 103, 1384–1396. doi: 10.1111/1365-2745.12438
Ma, F., Fischer, M., Han, Y., Withers, S. G., Feng, Y., and Yang, G.-Y. (2016). Substrate engineering enabling fluorescence droplet entrapment for IVC-FACS-based ultrahigh-throughput screening. Anal. Chem. 88, 8587–8595. doi: 10.1021/acs.analchem.6b01712
Mahmoudi, N., Hagen, S. M., Hazen, T. C., and Steen, A. D. (2020). Patterns in extracellular enzyme activity and microbial diversity in deep-sea Mediterranean sediments. Deep Sea Res. Part Oceanogr. Res. Pap. 158:103231. doi: 10.1016/j.dsr.2020.103231
Martinez-Garcia, M., Brazel, D. M., Swan, B. K., Arnosti, C., Chain, P. S. G., Reitenga, K. G., et al. (2012). Capturing single cell genomes of active polysaccharide degraders: an unexpected contribution of verrucomicrobia. PLoS One 7:e35314. doi: 10.1371/journal.pone.0035314
Nakamura, K., Iizuka, R., Nishi, S., Yoshida, T., Hatada, Y., Takaki, Y., et al. (2016). Culture-independent method for identification of microbial enzyme-encoding genes by activity-based single-cell sequencing using a water-in-oil microdroplet platform. Sci. Rep. 6:22259. doi: 10.1038/srep22259
Pachiadaki, M. G., Brown, J. M., Brown, J., Bezuidt, O., Berube, P. M., Biller, S. J., et al. (2019). Charting the complexity of the marine microbiome through single-cell genomics. Cell 179, 1623–1635.e11. doi: 10.1016/j.cell.2019.11.017
Pantoja, S., Lee, C., and Marecek, J. F. (1997). Hydrolysis of peptides in seawater and sediment. Mar. Chem. 57, 25–40. doi: 10.1016/S0304-4203(97)00003-0
Quince, C., Walker, A. W., Simpson, J. T., Loman, N. J., and Segata, N. (2017). Shotgun metagenomics, from sampling to analysis. Nat. Biotechnol. 35, 833–844. doi: 10.1038/nbt.3935
Reintjes, G., Arnosti, C., Fuchs, B., and Amann, R. (2019). Selfish, sharing and scavenging bacteria in the Atlantic Ocean: a biogeographical study of bacterial substrate utilisation. ISME J. 13, 1119–1132. doi: 10.1038/s41396-018-0326-3
Reintjes, G., Arnosti, C., Fuchs, B. M., and Amann, R. (2017). An alternative polysaccharide uptake mechanism of marine bacteria. ISME J. 11, 1640–1650. doi: 10.1038/ismej.2017.26
Rocca, J. D., Hall, E. K., Lennon, J. T., Evans, S. E., Waldrop, M. P., Cotner, J. B., et al. (2015). Relationships between protein-encoding gene abundance and corresponding process are commonly assumed yet rarely observed. ISME J. 9, 1693–1699. doi: 10.1038/ismej.2014.252
Sadler, N. C., and Wright, A. T. (2015). Activity-based protein profiling of microbes. Curr. Opin. Chem. Biol. 24, 139–144. doi: 10.1016/j.cbpa.2014.10.022
Schmidt, J. M., Royalty, T. M., Lloyd, K. G., and Steen, A. D. (2021). Potential activities and long lifetimes of organic carbon-degrading extracellular enzymes in deep subsurface sediments of the Baltic sea. Front. Microbiol. 12:2546. doi: 10.3389/fmicb.2021.702015
Sciambi, A., and Abate, A. R. (2015). Accurate microfluidic sorting of droplets at 30 kHz. Lab. Chip 15, 47–51. doi: 10.1039/C4LC01194E
Srivastava, A., Saavedra, D. E. M., Thomson, B., García, J. A. L., Zhao, Z., Patrick, W. M., et al. (2021). Enzyme promiscuity in natural environments: alkaline phosphatase in the ocean. ISME J. 15, 3375–3383. doi: 10.1038/s41396-021-01013-w
Steen, A. D., and Arnosti, C. (2011). Long lifetimes of β-glucosidase, leucine aminopeptidase, and phosphatase in Arctic seawater. Mar. Chem. 123, 127–132. doi: 10.1016/j.marchem.2010.10.006
Steen, A. D., Vazin, J. P., Hagen, S. M., Mulligan, K. H., and Wilhelm, S. W. (2015). Substrate specificity of aquatic extracellular peptidases assessed by competitive inhibition assays using synthetic substrates. Aquat. Microb. Ecol. 75, 271–281. doi: 10.3354/ame01755
Tawfik, D. S., and Griffiths, A. D. (1998). Man-made cell-like compartments for molecular evolution. Nat. Biotechnol. 16, 652–656. doi: 10.1038/nbt0798-652
Teeling, H., Fuchs, B. M., Becher, D., Klockow, C., Gardebrecht, A., Bennke, C. M., et al. (2012). Substrate-controlled succession of marine bacterioplankton populations induced by a phytoplankton bloom. Science 336, 608–611. doi: 10.1126/science.1218344
Terekhov, S. S., Smirnov, I. V., Stepanova, A. V., Bobik, T. V., Mokrushina, Y. A., Ponomarenko, N. A., et al. (2017). Microfluidic droplet platform for ultrahigh-throughput single-cell screening of biodiversity. Proc. Natl. Acad. Sci. U. S. A. 114, 2550–2555. doi: 10.1073/pnas.1621226114
Traving, S. J., Thygesen, U. H., Riemann, L., and Stedmon, C. A. (2015). A model of extracellular enzymes in free-living microbes: which strategy pays off? Appl. Environ. Microbiol. 81, 7385–7393. doi: 10.1128/AEM.02070-15
Vetter, Y. A., Deming, J. W., Jumars, P. A., and Krieger-Brockett, B. B. (1998). A predictive model of bacterial foraging by means of freely released extracellular enzymes. Microb. Ecol. 36, 75–92. doi: 10.1007/s002489900095
Weiss, M. S., Abele, U., Weckesser, J., Welte, W., Schiltz, E., and Schulz, G. E. (1991). Molecular architecture and electrostatic properties of a bacterial porin. Science 254, 1627–1630. doi: 10.1126/science.1721242
Whidbey, C., and Wright, A. T. (2019). “Activity-based protein profiling—enabling multimodal functional studies of microbial communities,” in Activity-Based Protein Profiling Current Topics in Microbiology and Immunology, eds B. F. Cravatt, K.-L. Hsu, and E. Weerapana (Cham: Springer International Publishing), 1–21. doi: 10.1007/82_2018_128
Wigington, C. H., Sonderegger, D., Brussaard, C. P. D., Buchan, A., Finke, J. F., Fuhrman, J. A., et al. (2016). Re-examination of the relationship between marine virus and microbial cell abundances. Nat. Microbiol. 1:15024. doi: 10.1038/nmicrobiol.2015.24
Woyke, T., Xie, G., Copeland, A., González, J. M., Han, C., Kiss, H., et al. (2009). Assembling the marine metagenome, one cell at a time. PLoS One 4:e5299. doi: 10.1371/journal.pone.0005299
Xing, P., Hahnke, R. L., Unfried, F., Markert, S., Huang, S., Barbeyron, T., et al. (2015). Niches of two polysaccharide-degrading Polaribacter isolates from the North Sea during a spring diatom bloom. ISME J. 9, 1410–1422. doi: 10.1038/ismej.2014.225
Zakem, E. J., Cael, B. B., and Levine, N. M. (2021). A unified theory for organic matter accumulation. Proc. Natl. Acad. Sci. U. S. A. 118:e2016896118. doi: 10.1073/pnas.2016896118
Zhao, Z., Baltar, F., and Herndl, G. J. (2020). Linking extracellular enzymes to phylogeny indicates a predominantly particle-associated lifestyle of deep-sea prokaryotes. Sci. Adv. 6:eaaz4354. doi: 10.1126/sciadv.aaz4354
Ziervogel, K., Steen, A. D., and Arnosti, C. (2010). Changes in the spectrum and rates of extracellular enzyme activities in seawater following aggregate formation. Biogeosciences 7, 1007–1015. doi: 10.5194/bg-7-1007-2010
Keywords: extracellular enzymes, single-cell, microfluidic droplet, carbon cycle, microbial ecology
Citation: Traving SJ, Balmonte JP, Seale D, Arnosti C, Glud RN, Hallam SJ and Middelboe M (2022) On Single-Cell Enzyme Assays in Marine Microbial Ecology and Biogeochemistry. Front. Mar. Sci. 9:846656. doi: 10.3389/fmars.2022.846656
Received: 31 December 2021; Accepted: 24 January 2022;
Published: 25 February 2022.
Edited by:
Rachel Ann Foster, Stockholm University, SwedenReviewed by:
Roland Hatzenpichler, Montana State University, United StatesCopyright © 2022 Traving, Balmonte, Seale, Arnosti, Glud, Hallam and Middelboe. This is an open-access article distributed under the terms of the Creative Commons Attribution License (CC BY). The use, distribution or reproduction in other forums is permitted, provided the original author(s) and the copyright owner(s) are credited and that the original publication in this journal is cited, in accordance with accepted academic practice. No use, distribution or reproduction is permitted which does not comply with these terms.
*Correspondence: Sachia J. Traving, c2p0cmF2aW5nQGJpb2xvZ3kuc2R1LmRr; John Paul Balmonte, anAuYmFsbW9udGVAZ21haWwuY29t