- 1Faculty of Science, Hokkaido University, Sapporo, Japan
- 2Cooperative Institute for Research in Environmental Sciences, University of Colorado, Boulder, CO, United States
- 3Physical Sciences Laboratory, National Oceanic and Atmospheric Administration (NOAA), Boulder, CO, United States
- 4Southwest Fisheries Science Center, National Oceanic and Atmospheric Administration (NOAA), Monterey, CA, United States
- 5Institute of Marine Sciences, University of California Santa Cruz, Santa Cruz, CA, United States
- 6Application Laboratory, Japan Agency for Marine-Earth Science and Technology, Yokohama, Japan
- 7Pacific Islands Fisheries Science Center, National Oceanic and Atmospheric Administration (NOAA), Honolulu, HI, United States
- 8Department of Oceanography, School of Ocean and Earth Science and Technology, University of Hawaii, Honolulu, HI, United States
This perspective paper discusses how the research community can promote enhancement of marine ecosystem forecasts using physical ocean conditions predicted by global climate models (GCMs). We review the major climate prediction projects and outline new research opportunities to achieve skillful marine biological forecasts. Physical ocean conditions are operationally predicted for subseasonal to seasonal timescales, and multi-year predictions have been enhanced recently. However, forecasting applications are currently limited by the availability of oceanic data; most subseasonal-to-seasonal prediction projects make only sea-surface temperature (SST) publicly available, though other variables useful for biological forecasts are also calculated in GCMs. To resolve the bottleneck of data availability, we recommend that climate prediction centers increase the range of ocean data available to the public, perhaps starting with an expanded suite of 2-dimensional variables, whose storage requirements are much smaller than 3-dimensional variables. Allowing forecast output to be downloaded for a selected region, rather than the whole globe, would also facilitate uptake. We highlight new research opportunities in both physical forecasting (e.g., new approaches to dynamical and statistical downscaling) and biological forecasting (e.g., conducting biological reforecasting experiments) and offer lessons learned to help guide their development. In order to accelerate this research area, we also suggest establishing case studies (i.e., particular climate and biological events as prediction targets) to improve coordination. Advancing our capacity for marine biological forecasting is crucial for the success of the UN Decade of Ocean Science, for which one of seven desired outcomes is “A Predicted Ocean”.
Introduction
Marine ecosystem forecasting, often leveraging predictions of physical ocean conditions, is an emerging research area that has rapidly attracted significant attention (Payne et al., 2017; Tommasi et al., 2017; Hobday et al., 2018; Capotondi et al., 2019a; Park et al., 2019; Jacox et al., 2020; Bolin et al., 2021). The development and improvement of marine biological forecasts are motivated by a number of ecological and socioeconomic aims, including management of fisheries and aquaculture, conservation of endangered marine species, and protection of human health. At present, most marine ecosystem predictions are in the experimental stage, but in the future, they could be operationalized with a wide range of applications.
Many different statistical and dynamical methods can be used for ecological prediction on subseasonal to decadal timescales (Tommasi et al., 2017; Jacox et al., 2020). However, perhaps the most promising approach is to start with general circulation or global climate model (GCM) predictions of the physical environment and use them as the basis for ecological prediction. GCM predictions are conducted for a range of forecast lead times (i.e., the length of time between the time of initial condition and the time for which conditions are being predicted1). Forecasts with subseasonal to seasonal lead time (i.e., several weeks to a year) are operationally produced by a number of modeling centers, and multiannual predictions (i.e., 1-10 years) are also being examined. These global climate forecasts offer a foundation to be used for an array of marine ecosystem predictions. While alternative methods may also be leveraged to generate biological predictions (for example, forecasting fish population dynamics by monitoring earlier life stages), they are beyond the scope of this paper.
Forecasting marine ecosystems using physical predictions consists of a multi-step process, typically including a GCM prediction, the dynamical or statistical downscaling of the GCM fields, and biological estimation (Figure 1) (e.g., Jacox et al., 2020). The data transfer between the tasks is an important consideration for the workflow. The most intensive data transfer is needed for dynamical downscaling, in which the three-dimensional (3D) output of a GCM prediction is needed to force a regional model (Figure 1A). A prime example of this workflow is J-SCOPE (JISAO’s Seasonal Coastal Ocean Prediction of the Ecosystem), for which dynamical downscaling using the Regional Ocean Modeling System (ROMS) is conducted using surface and lateral boundary conditions taken from version 2 of the National Oceanic and Atmospheric Administration (NOAA) Climate Forecast System (CFSv2) (Kaplan et al., 2016; Siedlecki et al., 2016). This system is supported by publicly available, 6-hourly, 3D forecast outputs of ocean variables for CFSv2. However, such data availability is exceptional; 3D GCM forecast output at higher than monthly resolution is typically not publicly available for other projects. Thus, in most cases, this workflow requires a close collaboration between the climate prediction center and the user institute.A more practical workflow for many researchers is to use two-dimensional (2D) GCM output for key fields such as SST (Figure 1B). This workflow was employed by a series of Australian studies in fisheries forecasting applications (Spillman et al., 2013; Spillman and Hobday, 2014; Eveson et al., 2015; Brodie et al., 2017). In this case, users may employ statistical downscaling rather than dynamical downscaling. A promising future extension of this workflow is to use multiple GCM outputs (Figure 1C) because a multi-model ensemble can better capture reality than a single model due to the reduction of model-specific errors, as found for SST (Hervieux et al., 2019; Yati and Minobe, 2021) and for sea-surface height (Widlansky et al., 2017; Long et al., 2021). Furthermore, the reduction of model-specific errors can lead to a better estimation of prediction uncertainty, which can be useful for applications using predictions.For marine ecosystem forecasts based on physical predictions, some bottlenecks and gaps need to be resolved. In order to address those problems, coordination across institutes is needed, and a large body of research is required. Thus, researchers, managers, and funding agencies need a strategy to work across climate and oceanographic disciplines in pursuit of the larger goal. The purpose of this perspective paper is twofold: (1) to review major ongoing activities related to climate predictions at subseasonal to decadal lead times, and (2) to outline new research opportunities for marine ecosystem forecasting.
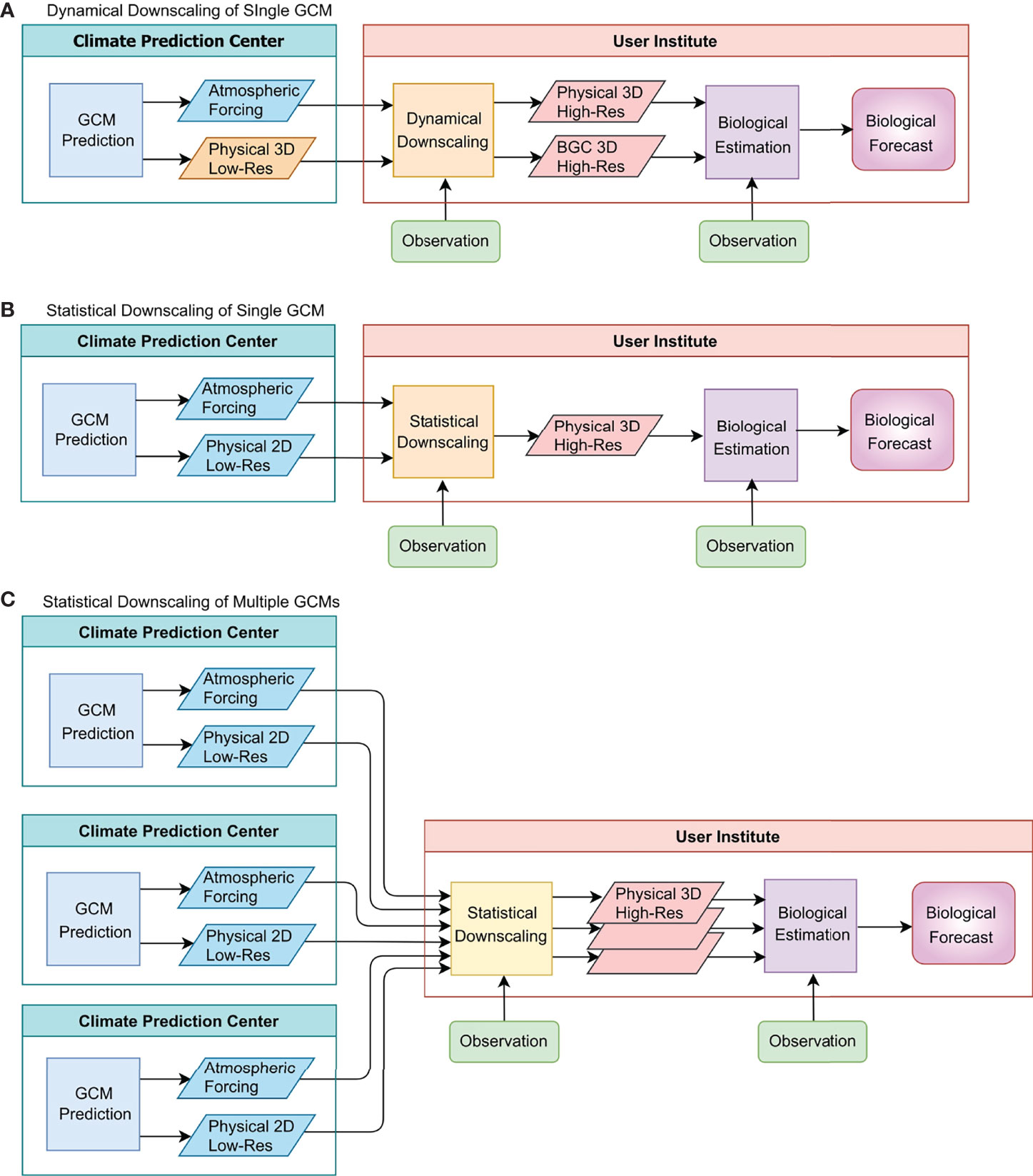
Figure 1 Typical workflows of biological forecasts based on physical GCM predictions. The squares indicate systems that produce predictions, downscaling or biological estimation, whereas the parallelograms indicate the outputs from the systems. Panel (A) and panel (B) indicate the workflows with dynamical and statistical downscalings, respectively, using outputs of a single GCM prediction, and panel (C) indicates the workflow using outputs of multiple GCMs with statistical downscaling. The stacked parallelograms in the middle of panel (C) indicate downscaling of different GCM predictions. “BGC” in the figure indicates information about biogeochemistry and lower-trophic level biology. Downscaling can be skipped if GCM predictions are of adequate resolution and acceptable bias. The physical prediction is assumed to be conducted by GCMs, but it can be made by ESMs, which also include BGC.
Present Status of Predictions of Oceanic Physical Conditions
In this section, we review how predictions of oceanic physical conditions, which are the basis of marine ecosystem prediction, are conducted from subseasonal (two weeks to two months), seasonal (from two months to one year), and to multiannual (from a year to ten years) lead times, including information on publicly available oceanic variables (Table 1). As noted above, our focus here is on physical predictions obtained using GCMs. Other prediction products may be suitable for some applications but are outside the scope of this paper (for example, forecasts produced with statistical methods such as linear inverse models2 and ocean-only model forecasts such as 10-day ocean weather forecasts around Japan3).
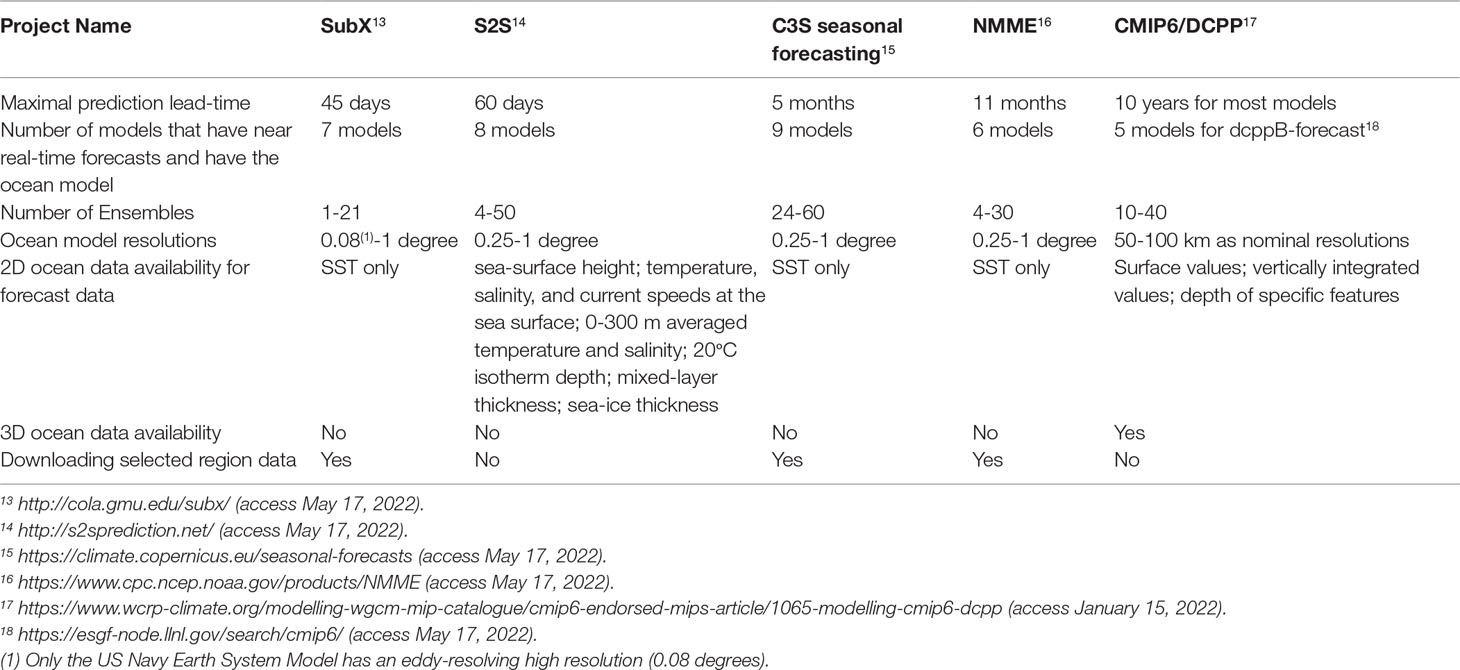
Table 1 Currently available ocean forecast output from major climate prediction projects on subseasonal to decadal timescales.
The subseasonal to seasonal (S2S) prediction project of the World Climate Research Programme (WCRP) (Vitart et al., 2017) provides S2S prediction datasets for forecast lead-times up to 60 days (Table 1). The data are available at the European Centre for Medium-Range Weather Forecasts (ECMWF)4and at the Chinese Meteorological Administration (CMA)5. Several modeling centers participating in the project provide various oceanic variables as 2D outputs, including SST, sea-surface salinity, surface currents, sea-surface height, mixed-layer depth, and 0-300 m averaged temperature and salinity.For other subseasonal-to-seasonal prediction projects, currently SST is the only oceanic variable made publicly available. Those projects include the Subseasonal Experiment (SubX) project (Pegion et al., 2019), the North American Multi-Model Ensemble (NMME) (e.g., Becker et al., 2014; Kirtman et al., 2014), and seasonal prediction by the Copernicus Climate Change Service (C3S) (Table 1). However, it should be noted that ocean output from CFSv2 seasonal forecasts, which can be obtained from NOAA6, includes a suite of 2D variables (temperature, salinity, and currents at fixed depths; isotherm depths, sea-level height, 0-300 m heat content) as well as 3D fields (temperature, salinity, and horizontal and vertical velocities) at monthly-mean or 6-hourly resolution.
Multiannual prediction, which is often called “decadal prediction” (Boer et al., 2016), is in its experimental stage. The first systematic collection of multiannual predictions was conducted in the context of the Climate Model Intercomparison Project Phase 5 (CMIP5) (Taylor et al., 2012) and has been enhanced in CMIP6 (Eyring et al., 2016). The data of CMIP5 and CMIP6 are available via the Earth System Grid Federation (ESGF)7. In CMIP6, multiannual prediction is coordinated under the Decadal Climate Prediction Project (DCPP), and DCPP experiments contain both reforecasts (i.e., forecasts simulated for a retrospective period; called dccpA) and near real-time forecasts (called dccpB) (Boer et al., 2016). Early evaluations of multiannual prediction skill have found that it mainly arises from initial conditions in the first few years, and at longer lead times is associated with the forced response to climate change, especially for temperatures (e.g.,Branstator and Teng, 2010 Yeager et al., 2018). CMIP6/DCPP provides a suite of 2D ocean variables as well as 3D ocean temperature, salinity and currents, all available as monthly or annual means. Recently, the World Meteorological Organization has established the Lead Centre for Annual to Decadal Climate Prediction, which annually issued a Global Annual to Decadal Climate Update8. The latest report documented forecasts for the target years from 2022 to 2026 (see also Hermanson et al., 2022).
Most of these prediction projects use GCMs, but a few modeling centers use Earth System Models (ESMs), i.e., GCMs coupled with a biogeochemical and lower-trophic ecosystem model. The Seasonal-to-Multiyear Large Ensemble (SMYLE) (Yeager et al., 2022) and the Decadal Prediction Large Ensemble (DPLE) (Yeager et al., 2018), both produced by the National Center for Atmospheric Research (NCAR), use the Community Earth System Model (CESM). The outputs of DPLE are publicly available on the NCAR web site9. Also, biogeochemical and biological variables for near real-time multiannual prediction under DCPP (dcppB) are available for one model (for the Canadian Earth System Model version 5) and for six models for reforecast (dcppA) at the present10.
In addition to considering the availability of data, it is also helpful to know whether a subset of the data for a selected region can be easily downloaded. The downloading of a selected region is possible for the C3S seasonal forecast data using the Application Programming Interface and for the SubX, NMME, and CFSv2 data via Open-source Project for a Network Data Access Protocol (OPeNDAP); but such an option is not available for the S2S and CMIP6/DCPP data.This summary of available output from global climate forecast systems highlights both the considerable potential in ongoing efforts and several major bottlenecks for marine ecosystem prediction, specifically the availability of already-computed data and the ability to download them efficiently.
New Opportunities
A wide range of new studies needs to be conducted to successfully develop marine ecological forecasts built on physical predictions. These studies can be broadly divided into two main categories: physical downscaling and biological prediction (Figure 1). The physical downscaling can be viewed as an intermediate goal that can be undertaken by physical researchers. An appropriate intermediate goal will allow researchers of physical oceanography to publish their own papers and obtain funding as principal investigators, and these prospects are important to attract young researchers (Minobe, 2014). To highlight the many specific opportunities for research in physical and biological aspects of marine ecosystem forecasting, we describe them separately below. But of course, even research in specialized areas can benefit from interdisciplinary collaboration.
Physical Research
GCM prediction skill should be examined for various oceanic variables that are useful for biological forecasts, because skillful ocean predictions of quantities that drive biological models are needed to achieve skillful ecological forecasts. To date, oceanic prediction skill has been examined mainly for SST (e.g., Becker et al., 2014; Doi et al., 2019; Hervieux et al., 2019) including marine heatwaves (Jacox et al., 2022), sea-surface height (e.g., Widlansky et al., 2017; Long et al., 2021; Shin and Newman, 2021; Amaya et al., 2022), and upper-layer temperatures (e.g., Yeager et al., 2018; Doi et al., 2020), because these variables are important in describing physical climate variability and relatively easy to evaluate with observation-based products. However, for biological predictions, other variables (e.g., mixed-layer depth, upwelling, salinity, bottom temperature, vertical profiles of temperature and density) can also be important, as they impact nutrient availability and the habitat of marine species, and they may be associated with a higher degree of predictability (e.g., Siedlecki et al., 2016; Capotondi et al., 2019a).
Furthermore, physical predictions from large ensembles and multi-model ensembles should be examined for their use in biological prediction. Recent studies identified an interesting bias in climate prediction systems known as the “predictability paradox” or “signal-to-noise paradox” (Eade et al., 2014; Dunstone et al., 2016; Smith et al., 2020). The basic idea of ensemble prediction is that the reality can be viewed as one member of an ensemble, and thus the difference between the ensemble mean and reality (as approximated by observations) should be similar to the differences between the ensemble mean and each ensemble member. However, when the predictability paradox occurs, the ensemble mean is more similar to reality than to other ensemble members. In this case, averaging over large ensembles is helpful to obtain a better prediction than those from smaller ensembles. To increase the size of ensembles, it is generally effective to use output from multiple models, and as discussed in Section 1, the use of multiple models also has the effect of reducing problems specific to individual models. Therefore, we suggest that using outputs of large ensembles from multiple GCMs can also have advantages for marine biological prediction (Figure 1C), and this possibility should be explored.
For regional marine ecosystem prediction efforts, the resolution of the global ocean models may not be sufficient, and thus dynamical or statistical downscaling of the predicted data at higher spatial resolutions may be necessary. Dynamical downscaling is used for J-SCOPE, as mentioned above, and various machine learning techniques are used for statistical downscaling (Stengel et al., 2020; Kashinath et al., 2021). Statistical downscaling schemes can be constructed using observations at specific sites together with coarse outputs of numerical models, but they can also be built on the results of dynamically downscaled data from the coarse model outputs (Jacox et al., 2020). The latter approach should be especially useful for variables that are not well observed. Both dynamical and statistical downscaling should be investigated in detail, as they have advantages and limitations. Dynamical downscaling can provide a complete representation of the ocean at the needed resolution, but will inherit the biases of the climate model that was used for the lateral boundary conditions and the surface forcing. The skill of downscaled forecasts should be compared to that of GCMs to quantify the added value of the downscaling procedure. Relative to dynamical downscaling, statistical downscaling can better capture observed relationships, but may be limited by data availability. Furthermore, dynamical downscaling is much more computationally expensive and slower than statistical downscaling, which can be important considerations for operational biological forecasting. Thus, depending on the specific application, different approaches may be more suitable.
Biological Research
It is important to identify which ecological variables are promising targets for prediction. The target for prediction should be relevant to species valued by society and should be sensitive to physical conditions. Candidates of target species can be identified by examining the statistical relationships between physical conditions and marine ecosystem status using the observational data as a first step. A classic example is the relation between the Pacific Decadal Oscillation (PDO) and Pacific salmon catches (Mantua et al., 1997), and that between the PDO and the Japanese sardine population (Yasuda et al., 1999). A more systematic approach using principal component analysis of a large number of marine ecosystem indicators reported that many species are influenced by climate variability and change in the North Pacific and adjacent seas (Hare and Mantua, 2000; Tian et al., 2006; Litzow and Mueter, 2014; Ma et al., 2019; Yati et al., 2020) and in the northeast Atlantic (Brunel and Boucher, 2007). Of course, such statistical analysis can only identify correlations, which do not necessarily mean causality, and the relationships may be non-stationary. Furthermore, a causal relationship is not enough for prediction, because if physical conditions that influence marine species are unpredictable, then biological targets are also not predictable (Brodie et al., 2021).
For the potential marine ecosystem targets, prediction skill should be assessed by conducting retrospective forecasts (i.e., reforecasts) evaluated against observations. Reforecasts using global ESMs have demonstrated meaningful prediction skill with lead times of a year or more for certain regions and variables, including surface pH (Brady et al., 2020), ocean carbon uptake (Lovenduski et al., 2019), aragonite saturation state (Yeager et al., 2022), chlorophyll and net primary productivity (Séférian et al., 2014; Rousseaux and Gregg, 2017: Krumhardt et al., 2020) and even annual fish catch in large marine ecosystems (Park et al., 2019). The availability of the biogeochemical model output is very important for better understanding the links between physics and biology in the model context. These links are still poorly constrained by observations due to the sparsity of biogeochemical data (Turi et al., 2018), highlighting the need for expanded and sustained biogeochemical observational networks in support of biological prediction efforts (Capotondi et al., 2019a).Ecological predictions are also challenged by shifts in the relationships between physical conditions and biological responses through time and with population sizes. Empirical relationships that appear robust for several years can decay over time (Myers, 1998; Deyle et al., 2013). Changes in climate conditions at the basin scale have been linked to shifts in relationships between local physical properties and fisheries recruitment (Litzow et al., 2019). An understanding of the underlying ecological mechanisms that explain empirical relationships between physical and biological properties and how those mechanisms may change over time is necessary to increase confidence in ecological predictions.In the coming years, regional biological reforecasts should be widely examined. Biological reforecasting is a relatively new study area, but lessons learned from climate reforecasts can be useful to guide similar efforts for biology:
1. Forecast skill should be examined for anomalies, i.e., the differences between forecasted raw values and the forecasted mean seasonal cycle (or climatology). If the forecast skill is examined with raw values, then the skill is likely to be dominated by a seasonal cycle rather than the interannual variability of interest. Furthermore, since model biases tend to grow due to model drift at longer lead times, model climatologies should be lead-time dependent.
2. For the estimation of the statistical significance of a metric, it is important to take into account the serial correlation of the data to be examined. For example, if there are annually sampled predicted and observed data for a period of N -years, and the respective time series have auto-correlation at a one-year lag of. ra and rb, then the effective degrees of freedom of the data for the Pearson’s correlation can be estimated as N (1−rarb)/(1+ rarb) (Bretherton et al., 1999). The influence becomes strong when the lag-1 autocorrelation is large. If the lag-1 autocorrelation is 0.6 (0.3) then the effective degrees of freedom are 47% (83%) of the original data samples. The serial correlation is not generally considered in widely used software packages or libraries. Therefore, the p-value obtained by such packages is inappropriate when the serial correlation cannot be ignored. In any case, how degrees of freedom were estimated should be clarified.
3. The separation of training and verification data, known as “cross-validation”, is crucial for assessing the performance of statistical estimation (e.g. Arlot and Celisse, 2010). For example, in V-fold cross validation, all data are divided into v “folds,” the prediction model is trained using data of v-1 folds, the remaining one fold is used for validation, and the fold to be used for validation is successively changed. Cross-validation is especially important when using a statistical or machine-learning technique that can substantially overfit the training data.
4. Ensembles of prediction should be used appropriately. Since the biological responses to physical conditions may be nonlinear, it is desirable to use the individual ensemble members of physical forecasts to drive biological models, rather than using the ensemble mean of the physical forecasts. A large ensemble size is especially useful to evaluate the probability of extreme events and to evaluate whether the predictability paradox occurs as mentioned above. Furthermore, if biological estimation involves uncertainty in the variables other than the physical prediction, using ensembles for these variables may be useful to understand the uncertainty originating in biological process.
Summary and Recommendations
Existing subseasonal to decadal climate predictions can potentially be very valuable for the prediction of marine ecosystems. However, availability of forecast output for ocean conditions is generally rather poor; in particular, most seasonal prediction systems do not provide ocean variables other than SST. While there are technical hurdles to providing additional large datasets, one of the reasons for the limited data availability may be that the modeling centers do not see enough demand for those data to be shared. We suggest that the demand actually exists, and we make two recommendations for enabling the uptake of physical forecasts in marine ecosystem prediction: 1) Climate prediction projects should make more ocean prediction data available to the research community. Making an expanded suite of 2D variables available, as done by the S2S project and CFSv2, would be a good starting point. Some currently unavailable 2D variables, such as eddy kinetic energy, could be useful for marine biological forecasts (e.g., Brodie et al., 2018), and thus they would be candidates of variables to be made available in the future. 2) Enable users to download data for selected regions. This capability is useful for a wide range of users who may be interested in specific regions and greatly reduces user requirements for data downloading, storage, and processing.
Combining physical and biological disciplines with the common goal of improved marine ecosystem prediction will be a fruitful area of research with clear applications to society. To facilitate this research area, it would be useful to develop a set of case studies for biological prediction. For example, a massive Northeast Pacific marine heatwave in 2013-2016 involved compound extremes of a heatwave, a low-oxygen extreme, and an ocean acidity extreme (Gruber et al., 2021). While the forecast skill and predictability of SST anomalies during this event have been explored (e.g., Hu et al., 2017; Jacox et al., 2019; Capotondi et al., 2019b; Capotondi et al., 2022), further research could investigate how much these various co-occurring extremes and their impacts on the marine ecosystem could be predicted. Since a regional phenomenon is generally studied by regional researchers, other case studies distributed across the global ocean can be identified to attract the international community’s interest.As the global community increasingly recognizes the sensitivity of marine ecosystems to climate variability and change and the potential consequences to human society, the time is ripe to enhance forecasts of marine ecosystems by pursuing the strategies proposed here. Such efforts are gaining some attraction at the international level. For example, the North Pacific Marine Science Organization (PICES) is in the process of establishing a new working group on “Climate Extremes and Coastal Impacts in the Pacific” with a focus on climate and marine ecosystem predictions11. Furthermore, the United Nations Decade of Ocean Science for Sustainable Development (UN Ocean Decade) has been launched for the 2021-2030 decade. The overarching theme of the UN Ocean Decade is “The Science We Need for the Ocean We Want.” One of its seven expected outcomes is “A Predicted Ocean.”12 To know what ocean we can have in the future, the capability of marine biological predictions is essential.
Data Availability Statement
The original contributions presented in the study are included in the article. Further inquiries can be directed to the corresponding author.
Author Contributions
SM wrote the first draft, and all authors actively contributed to its improvement. All authors contributed to the article and approved the submitted version.
Funding
SM was supported JSPS KAKENHI Grant Numbers JP18H04129, JP19H05704 and MN was by JP19H05701. MGJ was supported by NOAA Southwest Fisheries Science Center, RRR was supported by NOAA Pacific Islands Fisheries Science Center, and AC was supported by the NOAA Climate Program Office Modeling, Analysis, Predictions and Projections (MAPP) program.
Conflict of Interest
The authors declare that the research was conducted in the absence of any commercial or financial relationships that could be construed as a potential conflict of interest. The reviewer AH declared a past co-authorship with one of the authors MJ to the handling Editor.
Publisher’s Note
All claims expressed in this article are solely those of the authors and do not necessarily represent those of their affiliated organizations, or those of the publisher, the editors and the reviewers. Any product that may be evaluated in this article, or claim that may be made by its manufacturer, is not guaranteed or endorsed by the publisher.
Acknowledgments
This paper is an initiative of the Working Group on “Climate and Ecosystem Predictability”, jointly sponsored by the North Pacific Marine Science Organization (PICES) and the Climate and Oceans - Variability, Predictability, and Change (CLIVAR). We thank PICES and CLIVAR for their sponsorship and support. We appreciate two reviewers for their helpful and constructive comments.
Footnotes
- ^ This definition of lead time is widely used for research, though for practical use of forecasts, the lead time may be defined based on a time when a forecast is issued instead of the time of the initial condition..
- ^ https://psl.noaa.gov/forecasts/sstlim/(access January 15, 2022).
- ^ https://www.eorc.jaxa.jp/ptree/ocean_model/index.html(access January 15, 2022).
- ^ https://apps.ecmwf.int/datasets/data/s2s(access January 15, 2022).
- ^ http://s2s.cma.cn/index(access January 15, 2022).
- ^ https://www.ncei.noaa.gov/products/weather-climate-models/climate-forecast-system(access May 18, 2022).
- ^ https://esgf-node.llnl.gov/projects/esgf-llnl/(access May 1, 2022).
- ^ https://www.wmolc.org/(access January 10, 2022).
- ^ https://www.cesm.ucar.edu/projects/community-projects/DPLE/data-sets.html(access December 26, 2021).
- ^ https://esgf-node.llnl.gov/projects/cmip6/(access May 3, 2022).
- ^ https://meetings.pices.int/members/working-groups/wg49(access January 15, 2022).
- ^ https://www.oceandecade.org/vision-mission/(access January 10, 2021).
References
Amaya D. J., Jacox M. G., Dias J., Alexander M. A., Karnauskas K. B., Scott J. D., et al. (2022). Subseasonal-To-Seasonal Forecast Skill in the California Current System and its Connection to Coastal Kelvin Waves. J. Geophys. Res.: Ocean. 127, e2021JC017892. doi: 10.1029/2021JC017892
Arlot S., Celisse A. (2010). A Survey of Cross-Validation Procedures for Model Selection. Stat Surveys. 4, 40–79. doi: 10.1214/09-SS054
Becker E., van den Dool H., Zhang Q. (2014). Predictability and Forecast Skill in NMME. J. Clim. 27, 5891–5906. doi: 10.1175/JCLI-D-13-00597.1
Boer G. J., Smith D. M., Cassou C., Doblas-Reyes F., Danabasoglu G., Kirtman B., et al. (2016). The Decadal Climate Prediction Project (DCPP) Contribution to CMIP6. Geosci. Model. Dev. 9, 3751–3777. doi: 10.5194/gmd-9-3751-2016
Bolin J. A., Schoeman D. S., Evans K. J., Cummins S. F., Scales K. L. (2021). Achieving Sustainable and Climate-Resilient Fisheries Requires Marine Ecosystem Forecasts to Include Fish Condition. Fish. Fish. 22,1067–1084. doi: 10.1111/faf.12569
Brady R. X., Lovenduski N. S., Yeager S. G., Long M. C., Lindsay K. (2020). killful multiyear predictions of ocean acidification in the California Current System. Nat. Commun. 11, 2166. doi: 10.1038/s41467-020-15722-x
Branstator G., Teng H. (2010) Potential impact of initialization on decadal predictions as assessed for CMIP5 models. Geophys. Res. Lett. 39. doi: 10.1029/2012GL051974
Brodie S., Abrahms B., Bograd S. J., Carroll G., Hazen E. L., Muhling B. A., et al. (2021). Exploring Timescales of Predictability in Species Distributions. Ecography 44, 832–844. doi: 10.1111/ecog.05504
Brodie S., Hobday A. J., Smith J. A., Spillman C. M., Hartog J. R., Everett J. D., et al. (2017). Seasonal Forecasting of Dolphinfish Distribution in Eastern Australia to Aid Recreational Fishers and Managers. Deep. Sea. Res. Part 2. Top. Stud. Oceanogr. 140, 222–229. doi: 10.1016/j.dsr2.2017.03.004
Brodie S., Jacox M. G., Bograd S. J., Welch H., Dewar H., Scales K. L., et al. (2018). Integrating Dynamic Subsurface Habitat Metrics into Species Distribution Models. Front. Mar. Sci. 5:219. doi: 10.3389/fmars.2018.00219
Capotondi A., Jacox M., Bowler C., Kavanaugh M., Lehodey P., Barrie D., et al. (2019a). Observational Needs Supporting Marine Ecosystems Modeling and Forecasting: From the Global Ocean to Regional and Coastal Systems. Front. Mar. Sci. 6. doi: 10.3389/fmars.2019.00623
Capotondi A., Newman M., Xu T., Di Lorenzo E. (2022). An Optimal Precursor of Northeast Pacific Marine Heatwaves and Central Pacific El Niño Events. Geophys. Res. Lett. 49, e2021GL097350. doi: 10.1029/2021GL097350
Capotondi A., Sardeshmukh P. D., Di Lorenzo E., Subramanian A., Miller A. J. (2019b). Predictability of US West Coast Ocean Temperature is Not Solely Due to ENSO. Sci. Rep. 9, 10993. doi: 10.1038/s41598-019-47400-4
Deyle E. R., Fogarty M., Hsieh C.-H., Kaufman L., MacCall A. D., Munch S. B., et al. (2013). Predicting Climate Effects on Pacific Sardines. PNAS 110, 6430–6435. doi: 10.1073/pnas.1215506110
Doi T., Behera S. K., Yamagata T. (2019). Merits of a 108-Member Ensemble System in ENSO and IOD Predictions. J. Clim. 32, 957–972. doi: 10.1175/JCLI-D-18-0193.1
Doi T., Nonaka M., Behera S. (2020). Skill Assessment of Seasonal-To-Interannual Prediction of Sea Level Anomaly in the North Pacific Based on the SINTEX-F Climate Model. Front. Mar. Sci. 7, 855. doi: 10.3389/fmars.2020.546587
Dunstone N., Smith D., Scaife A., Hermanson L., Eade R., Robinson N., et al. (2016). Skilful Predictions of the Winter North Atlantic Oscillation One Year Ahead. Nat. Geosci. 9, 809–814. doi: 10.1038/ngeo2824
Eade R., Smith D., Scaife A., Wallace E., Dunstone N., Hermanson L., et al. (2014). Do Seasonal-to-Decadal Climate Predictions Underestimate the Predictability of the Real World? Geophys. Res. Lett. 41, 5620–5628. doi: 10.1002/2014GL061146
Eveson J. P., Hobday A. J., Hartog J. R., Spillman C. M., Rough K. M. (2015). Seasonal Forecasting of Tuna Habitat in the Great Australian Bight. Fish. Res. 170, 39–49. doi: 10.1016/j.fishres.2015.05.008
Eyring V., Bony S., Meehl G. A., Senior C. A., Stevens B., Stouffer R. J., et al. (2016). Overview of the Coupled Model Intercomparison Project Phase 6 (CMIP6) Experimental Design and Organization. Geosci. Model. Dev. 9, 1937–1958. doi: 10.5194/gmd-9-1937-2016
Gruber N., Boyd P. W., Frölicher T. L., Vogt M. (2021). Biogeochemical Extremes and Compound Events in the Ocean. Nature 600, 395–407. doi: 10.1038/s41586-021-03981-7
Hare S. R., Mantua N. J. (2000). Empirical Evidence for North Pacific Regime Shifts in 1977 and 1989. Prog. Oceanogr. 47, 103–145. doi: 10.1016/S0079-6611(00)00033-1
Hermanson L., Smith D., Seabrook M., Bilbao R., Doblas-Reyes F., Tourigny E., et al (2022). WMO Global Annual to Decadal Climate Update: A Prediction for 2021–25. Bull. Am. Meteorological. Soc. 103 (4), E1117–E1129. doi: 10.1175/BAMS-D-20-0311.1
Hervieux G., Alexander M. A., Stock C. A., Jacox M. G., Pegion K., Becker E., et al. (2019). More Reliable Coastal SST Forecasts From the North American Multimodel Ensemble. Clim. Dyn. 53, 7153–7168. doi: 10.1007/s00382-017-3652-7
Hobday A. J., Spillman C. M., Eveson J. P., Hartog J. R., Zhang X., Brodie S. (2018). A Framework for Combining Seasonal Forecasts and Climate Projections to Aid Risk Management for Fisheries and Aquaculture. Front. Mar. Sci. 5. doi: 10.3389/fmars.2018.00137
Hu Z.-Z., Kumar A., Jha B., Zhu J., Huang B. (2017). Persistence and Predictions of the Remarkable Warm Anomaly in the Northeastern Pacific Ocean During 2014–16. J. Clim. 30, 689–702. doi: 10.1175/JCLI-D-16-0348.1
Jacox M. G., Alexander M. A., Amaya D., Becker E., Bograd S. J., Brodie S., et al. (2022). Global Seasonal Forecasts of Marine Heatwaves. Nature 604, 486–490. doi: 10.1038/s41586-022-04573-9
Jacox M. G., Alexander M. A., Siedlecki S., Chen K., Kwon Y.-O., Brodie S., et al. (2020). Seasonal-To-Interannual Prediction of North American Coastal Marine Ecosystems: Forecast Methods, Mechanisms of Predictability, and Priority Developments. Prog. Oceanogr. 183, 102307. doi: 10.1016/j.pocean.2020.102307
Jacox M. G., Tommasi D., Alexander M. A., Hervieux G., Stock C. A. (2019). Predicting the Evolution of the 2014–2016 California Current System Marine Heatwave From an Ensemble of Coupled Global Climate Forecasts. Front. Mar. Sci. 6, 497. doi: 10.3389/fmars.2019.00497
Kaplan I. C., Williams G. D., Bond N. A., Hermann A. J., Siedlecki S. A. (2016). Cloudy With a Chance of Sardines: Forecasting Sardine Distributions Using Regional Climate Models. Fish. Oceanogr. 25, 15–27. doi: 10.1111/fog.12131
Kashinath K., Mustafa M., Albert A., Wu J.-L., Jiang C., Esmaeilzadeh S., et al. (2021). Physics-Informed Machine Learning: Case Studies for Weather and Climate Modelling. Philos. Trans. A. Math. Phys. Eng. Sci. 379, 20200093. doi: 10.1098/rsta.2020.0093
Kirtman B. P., Min D., Infanti J. M., Kinter J. L., Paolino D. A., Zhang Q., et al. (2014). The North American Multimodel Ensemble: Phase-1 Seasonal-to-Interannual Prediction; Phase-2 Toward Developing Intraseasonal Prediction. Bull. Am. Meteorol. Soc 95, 585–601. doi: 10.1175/BAMS-D-12-00050.1
Krumhardt K. M., Lovenduski N. S., Long M. C., Luo J. Y., Lindsay K., Yeager S., et al. (2020). Potential Predictability of Net Primary Production in the Ocean. Global Biogeochem. Cycles. 34, e2020GB006531. doi: 10.1029/2020GB006531
Litzow M. A., Ciannelli L., Puerta P., Wettstein J. J., Rykaczewski R. R., Opiekun M. (2019). Nonstationary Environmental and Community Relationships in the North Pacific Ocean. Ecology 100 (8), e02760. doi: 10.1002/ecy.2760
Litzow M. A., Mueter F. J. (2014). Assessing the Ecological Importance of Climate Regime Shifts: An Approach From the North Pacific Ocean. Prog. Oceanogr. 120, 110–119. doi: 10.1016/j.pocean.2013.08.003
Long X., Widlansky M. J., Spillman C. M., Kumar A., Balmaseda M., Thompson P. R., et al. (2021). Seasonal Forecasting Skill of Sea-Level Anomalies in a Multi-Model Prediction Framework. J. Geophys. Res. Ocean. 126, e2020JC017060. doi: 10.1029/2020JC017060
Lovenduski N. S., Yeager S. G., Lindsay K., Long M. C. (2019). Predicting Near-Term Variability in Ocean Carbon Uptake. J. Earth System. Dynamics. 10, 45–57. doi: 10.5194/esd-10-45-2019
Ma S., Liu Y., Li J., Fu C., Ye Z., Sun P., et al. (2019). Climate-Induced Long-Term Variations in Ecosystem Structure and Atmosphere-Ocean-Ecosystem Processes in the Yellow Sea and East China Sea. Prog. Oceanogr. 175, 183–197. doi: 10.1016/j.pocean.2019.04.008
Mantua N. J., Hare S. R., Zhang Y., Wallace J. M., Francis C. (1997). A pacific interdecadal climate oscillation with impacts on salmon production. Bull. Am. Meteorol. Soc 78, 1069–1079. doi: 10.1175/1520-0477(1997)078<1069:apicow>2.0.co;2
Minobe S. (2014). More Attractive Science Ecosystem Design for FUTURE and Beyond: A Personal View From a Researcher in a Peripheral Field. PICES. Press. 22. 2, 6–8. Retrieved from https://www.proquest.com/scholarly-journals/more-attractive-science-ecosystem-design-future/docview/1556456820/se-2?accountid=28258.
Myers R. A. (1998). When do Environment–Recruitment Correlations Work? Rev. Fish. Biol. Fish. 8, 285–305. doi: 10.1023/A:1008828730759
Park J.-Y., Stock C. A., Dunne J. P., Yang X., Rosati A. (2019). Seasonal to Multiannual Marine Ecosystem Prediction With a Global Earth System Model. Science 365, 284–288. doi: 10.1126/science.aav6634
Payne M. R., Hobday A. J., MacKenzie B. R., Tommasi D., Dempsey D. P., Fässler S. M. M., et al. (2017). Lessons From the First Generation of Marine Ecological Forecast Products. Front. Mar. Sci. 4. doi: 10.3389/fmars.2017.00289
Pegion K., Kirtman B. P., Becker E., Collins D. C., LaJoie E., Burgman R., et al, (2019). The Subseasonal Experiment (SubX): A Multimodel Subseasonal Prediction Experiment. Bull. Am. Meteorol. Soc 100, 2043–2060. doi: 10.1175/BAMS-D-18-0270.1
Rousseaux C. S., Gregg W. W. (2017). Forecasting Ocean Chlorophyll in the Equatorial Pacific. Front. Mar. Sci. 4, 236. doi: 10.3389/fmars.2017.00236
Séférian R., Bopp L., Gehlen M., Swingedouw D., Mignot J., Guilyardi E., et al. (2014). Multiyear Predictability of Tropical Marine Productivity. Proc. Natl. Acad. Sci. U. S. A. 111, 11646–11651. doi: 10.1073/pnas.1315855111
Shin S.-I., Newman M. (2021). Seasonal Predictability of Global and North American Coastal Sea Surface Temperature and Height Anomalies. Geophys. Res. Lett. 48, e2020GL091886. doi: 10.1029/2020gl091886
Siedlecki S. A., Kaplan I. C., Hermann A. J., Nguyen T. T., Bond N. A., Newton J. A., et al. (2016). Experiments With Seasonal Forecasts of Ocean Conditions for the Northern Region of the California Current Upwelling System. Sci. Rep. 6, 27203. doi: 10.1038/srep27203
Smith D. M., Scaife A. A., Eade R., Athanasiadis P., Bellucci A., Bethke I., et al. (2020). North Atlantic Climate Far More Predictable Than Models Imply. Nature 583, 796–800. doi: 10.1038/s41586-020-2525-0
Spillman C. M., Alves O., Hudson D. A. (2013). Predicting Thermal Stress for Coral Bleaching in the Great Barrier Reef Using a Coupled Ocean-Atmosphere Seasonal Forecast Model. Int. J. Climatol. 33, 1001–1014. doi: 10.1002/joc.3486
Spillman C. M., Hobday A. J. (2014). Dynamical Seasonal Ocean Forecasts to Aid Salmon Farm Management in a Climate Hotspot. Clim. Risk Manage. 1, 25–38. doi: 10.1016/j.crm.2013.12.001
Stengel K., Glaws A., Hettinger D., King R. N. (2020). Adversarial super-resolution of climatological wind and solar data. Proc. Natl. Acad. Sci.U.S.A.117, 16805–16815. doi: 10.1073/pnas.1918964117
Taylor K. E., Stouffer R. J., Meehl G. A. (2012). An Overview of CMIP5 and the Experiment Design. Bull. Am. Meteorol. Soc 93, 485–498. doi: 10.1175/BAMS-D-11-00094.1
Tian Y., Kidokoro H., Watanabe T. (2006). Long-Term Changes in the Fish Community Structure From the Tsushima Warm Current Region of the Japan/East Sea With an Emphasis on the Impacts of Fishing and Climate Regime Shift Over the Last Four Decades. Prog. Oceanogr. 68, 217–237. doi: 10.1016/j.pocean.2006.02.009
Tommasi D., Stock C. A., Hobday A. J., Methot R., Kaplan I. C., Eveson J. P., et al. (2017). Managing Living Marine Resources in a Dynamic Environment: The Role of Seasonal to Decadal Climate Forecasts. Prog. Oceanogr. 152, 15–49. doi: 10.1016/j.pocean.2016.12.011
Turi G., Alexander M. A., Lovenduski N. S., Capotondi A., Scott J., Stock C., et al. (2018). Response of O2 and pH to ENSO in the California Current System in a High-Resolution Global Climate Model. Ocean. Sci. 14, 69–86. doi: 10.5194/os-14-69-2018
Vitart F., Ardilouze C., Bonet A., Brookshaw A., Chen M., Codorean C., et al. (2017). The Subseasonal to Seasonal (S2S) Prediction Project Database. Bull. Am. Meteorol. Soc 98, 163–173. doi: 10.1175/BAMS-D-16-0017.1
Widlansky M. J., Marra J. J., Chowdhury M. R., Stephens S. A., Miles E. R., Fauchereau N., et al. (2017). Multimodel ensemble sea level forecasts for tropical Pacific sslands. J. Appl. Meteor. Climatol. 56, 849–862. doi: 10.1175/JAMC-D-16-0284.1
Yasuda I., Sugisaki H., Watanabe Y., Minobe S. S., Oozeki Y. (1999) Interdecadal variations in Japanese sardine and ocean/climate. Fish. Oceanogr.8:18–24. doi: 10.1046/j.1365-2419.1999.00089.x
Yati E., Minobe S. (2021). Sea Surface Temperature Predictability in the North Pacific From Multi-Model Seasonal Forecast. J. Oceanogr. 77, 897–906. doi: 10.1007/s10872-021-00618-1
Yati E., Minobe S., Mantua N., Ito S.-I., Di Lorenzo E. (2020). Marine Ecosystem Variations Over the North Pacific and Their Linkage to Large-Scale Climate Variability and Change. Front. Mar. Sci. 7. doi: 10.3389/fmars.2020.578165
Yeager S. G., Danabasoglu G., Rosenbloom N. A., Strand W., Bates S. C., Meehl G. A., et al. (2018). Predicting Near-Term Changes in the Earth System: A Large Ensemble of Initialized Decadal Prediction Simulations Using the Community Earth System Model. Bull. Am. Meteorol. Soc 99, 1867–1886. doi: 10.1175/BAMS-D-17-0098.1
Keywords: dynamical downscaling, statistical downscaling, biological forecast, marine ecosystem prediction, GCM prediction
Citation: Minobe S, Capotondi A, Jacox MG, Nonaka M and Rykaczewski RR (2022) Toward Regional Marine Ecological Forecasting Using Global Climate Model Predictions From Subseasonal to Decadal Timescales: Bottlenecks and Recommendations. Front. Mar. Sci. 9:855965. doi: 10.3389/fmars.2022.855965
Received: 16 January 2022; Accepted: 06 June 2022;
Published: 01 August 2022.
Edited by:
Doran M. Mason, NOAA-Great Lakes Environmental Research Laboratory, United StatesReviewed by:
Albert J. Hermann, University of Washington, United StatesClaire M. Spillman, Bureau of Meteorology, Australia
Copyright © 2022 Minobe, Capotondi, Jacox, Nonaka and Rykaczewski. This is an open-access article distributed under the terms of the Creative Commons Attribution License (CC BY). The use, distribution or reproduction in other forums is permitted, provided the original author(s) and the copyright owner(s) are credited and that the original publication in this journal is cited, in accordance with accepted academic practice. No use, distribution or reproduction is permitted which does not comply with these terms.
*Correspondence: Shoshiro Minobe, minobe@sci.hokudai.ac.jp