- 1Institute for Marine and Antarctic Studies, University of Tasmania, Hobart, TAS, Australia
- 2Commonwealth Scientific and Industrial Research Organisation (CSIRO), Oceans and Atmosphere, Hobart, TAS, Australia
- 3Department of Agriculture, Water and the Environment, Australian Antarctic Division, Kingston, TAS, Australia
Human activity puts our oceans under multiple stresses, whose impacts are already significantly affecting biodiversity and physicochemical properties. Consequently, there is an increased international focus on the conservation and sustainable use of oceans, including the protection of fragile benthic biodiversity hotspots in the deep sea, identified as vulnerable marine ecosystems (VMEs). International VME risk assessment and conservation efforts are hampered because we largely do not know where VMEs are located. VME distribution modelling has increasingly been recommended to extend our knowledge beyond sparse observations. Nevertheless, the adoption of VME distribution models in spatial management planning and conservation remains limited. This work critically reviews VME distribution modelling studies, and recommends promising avenues to make VME models more relevant and impactful for policy and management decision making. First, there is an important interplay between the type of VME data used to build models and how the generated maps can be used in making management decisions, which is often ignored by model-builders. Overall, there is a need for more precise VME data for production of reliable models. We provide specific guidelines for seven common applications of VME distribution modelling to improve the matching between the modelling and the user need. Second, the current criteria to identify VME often rely on subjective thresholds, which limits the transparency, transferability and effective applicability of distribution models in protection measures. We encourage scientists towards founding their models on: (i) specific and quantitative definitions of what constitute a VME, (ii) site conservation value assessment in relation to VME multi-taxon spatial predictions, and (iii) explicitly mapping vulnerability. Along with the recent increase in both deep-sea biological and environmental data quality and quantity, these modelling recommendations can lead towards more cohesive summaries of VME’s spatial distributions and their relative vulnerability, which should facilitate a more effective protection of these ecosystems, as has been mandated by numerous international agreements.
1 Introduction
Deep sea biodiversity is highly diverse, poorly understood (Ramirez-Llodra et al., 2010; Thurber et al., 2014) but threatened by a combination of multiple stresses as a result of human activity (Clark et al., 2016a; Danovaro et al., 2017). Since 2006, the UN General Assembly (UNGA) adopted a series of resolutions dedicated to the protection of fragile benthic biodiversity hotspots in the deep sea, collectively called vulnerable marine ecosystems (VMEs). Resolution 61/105 (UNGA, 2006) calls upon States and Regional Fisheries Management Organisations/Arrangements (RFMO/As) to identify areas beyond national jurisdiction where VMEs occur, or are likely to occur, in order to prevent significant adverse impacts from damaging fishing practices. In 2009, the Food and Agriculture Organization (FAO) established five criteria (FAO, 2009) that define VMEs: (i) uniqueness or rareness, (ii) functional significance of the habitat, (iii) fragility, (iv) life-history of species that makes recovery difficult, (v) structural complexity, see Table 1. Overall, the vulnerability refers to the likelihood that an ecosystem will be irreversibly altered by a short-term or chronic disturbance. VMEs include hydrothermal vents, coral reefs, xenophyophore communities, sponge grounds, seamounts, which host endemic and extraordinary biodiversity with organisms adapted to live in these specific environments. Coral communities (e.g. bamboo coral, Isididae) are very diverse, slow growing, and have been associated with populations of commercial fish, while being fragile and vulnerable to fishing gear (Maynou and Cartes, 2012; Lockhart and Hocevar, 2021). Forming structurally complex and functional habitats, VME indicator taxa have been depicted as “ecosystem engineers” (Kenchington et al., 2014; Lockhart and Hocevar, 2021) and have a critical influence on marine macrofaunal assemblages, through strengthened links between pelagic and benthic food webs (Ashford et al., 2019).
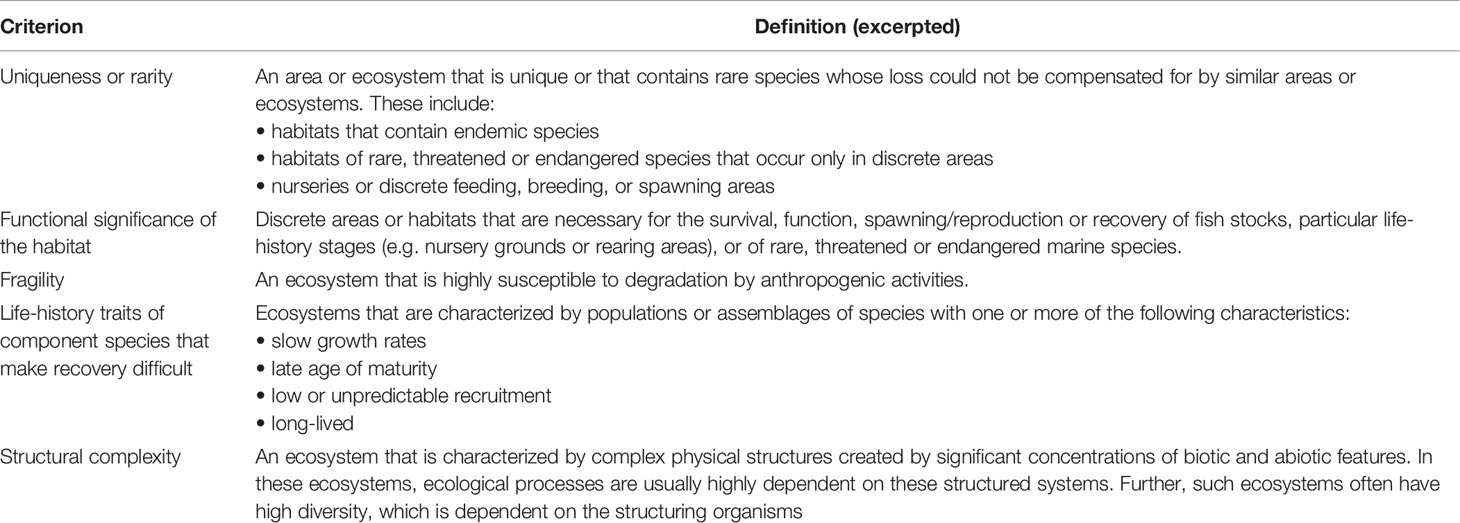
Table 1 FAO VME identification criteria, from (FAO, 2009).
Periodic reviews of the implementation of the 61/105 UNGA resolution have noted systematic failures to properly map VMEs, and judged their protection as insufficient (Rogers and Gianni, 2010; Gianni et al., 2016; Thompson et al., 2016; Bell et al., 2019). VMEs protection is directly hampered by the fact that the vast majority of the deep-sea has not been sampled (Clark et al., 2016b), hence its benthic composition remains mostly unknown (Weaver et al., 2011). Increasing our knowledge about VMEs, including their spatial distribution, composition, vulnerability and environmental requirements, is crucial in order to provide more effective protection (Chimienti et al., 2018). Although many studies urged for highly precautionary management of deep-sea benthic ecosystems (Clark et al., 2016a; Watling and Auster, 2017), its implementation remains, to date, highly limited (Norse et al., 2012; Thompson et al., 2016; Bell et al., 2019). Therefore, frameworks that can make the best of the information we have (e.g., highlighting where the key uncertainties lie, improve our VMEs knowledge into poorly sampled areas) are valuable.
What we do know about the location of VMEs typically derives from when VME indicator taxa are caught as a bycatch in fisheries gear (Ardron et al., 2014; Lauria et al., 2017; Carbonara et al., 2020; Blicher and Arboe, 2021) or, to a lesser extent when they are captured by scientific surveys (Dautova et al., 2019; Baco et al., 2020; Du Preez et al., 2020; Durán Muñoz et al., 2020; Long et al., 2020; Salinas-de-León et al., 2020). Because the former is destructive and has a limited sampling ability (Watling and Auster, 2017) and the latter is very expensive, these techniques are not suitable to be used at large spatial scales. To extend our knowledge beyond these sparse observations, VME distribution modelling has been increasingly used (Ardron et al., 2014; Vierod et al., 2014; Thompson et al., 2016; Lauria et al., 2021). Essentially, VME distribution modelling aims at predicting the suitability of a location for a VME, based on their observed relationship with environmental conditions. By collating all available information, distribution modelling provides a coherent analysis framework to highlight unsampled, and often remote, areas that may harbour VME. Apart from very recent works in the North Atlantic and South Pacific RFMOs e.g., (ICES, 2019; Rowden and Anderson, 2019), VME distribution models have yet to realise any substantial impact on conservation and management measures, and translating modelling insights to policy-decisions remains relatively limited (Ardron et al., 2014; Vierod et al., 2014; Gianni et al., 2016; Thompson et al., 2016; Bell et al., 2019; Rowden et al., 2020). The main reasons for this are: (i) the overall disagreement about what constitutes a VME, (ii) the inherent uncertainty of model predictions, especially since most are fitted on observations from fishery bycatch data with limited reliability and accuracy, and (iii) model outputs are not always easily understandable or relevant for environmental managers and conservationists.
In this study, we provide a comprehensive and critical review of VME distribution modelling and some perspectives to guide future modelling efforts towards a better integration into policy and management. We highlight modelling challenges related to the type of data used to build VME models (section Connections Between the Type of VME Data and Model Use). We underline how the lack of quantitative standards of what constitutes a VME limits the models’ applicability (section Limited Translation of the FAO Criteria Into Modelling), and provide guidelines for seven typical applications of VME distribution modelling in order to ensure that the user groups are appropriately informed (section Guidelines to Match the Modelling to the Need). Besides, we recommend a multi-criteria and -taxa modelling approach to map the vulnerability of marine ecosystems, to produce VME mappings that are more cohesive and relevant for environmental managers and conservationists (section Making VME Distribution Models More Relevant for Conservation and Management Decisions).
2 Connections Between the Type of VME Data and Model Use
This section details important connections between the type of VME data and the model use, which are often ignored by model-builders. VME observations mainly come from two sampling methods: (i) by physical sampling, e.g. bycatch of fishing gear and scientific benthic survey, or (ii) using underwater imagery, e.g. towed cameras, Remotely Operated Vehicle or Autonomous Underwater Vehicle. Different gear will have different selectivity. Gear selectivity combined with differences in species catchability can create species-specific sampling artefacts which are a source of modelling uncertainty (Parker and Bowden, 2010; Williams et al., 2020). For example, the low measured prevalence of a species can be due to its rareness in the sampled areas, or due to its limited catchability because of its size/morphology, or due to the fact that the sampling gear isn’t efficient in retaining it. In this section, we discuss the consequences of using a certain type of data on the VME modelling and on its interpretability in regard to the FAO guidelines.
The probability of detecting a VME, P, at a given location can be expressed by:
where P(0) is the probability of not detecting a VME when present, P(>0) is the probability of detecting a VME when present (also written P(1) for presence-absence data), PP is probability of VME occurrence (which may be expressed as a Poisson distribution), and PS is the probability of detecting that a VME is present (which may be a function of a number of variables). VME data may be a function of the full joint Poisson distribution (i.e., abundance data), truncated as a joint Bernoulli distribution [i.e., presence/absence data, P(1) and P(0)], or truncated so that only presence is recorded [i.e., presence-only data as there is no information on P(0)]. As more information is lost (i.e., going from abundance to presence-only), model estimation becomes more difficult and the internal variability of both response and covariate data may be such that robust estimation is no longer possible.
2.1 Data-Characteristics Need to Match the Model Application
The interpretability of VME model predictions directly depends on the underlying data used for model building, meaning the data-characteristics need to match their application (Guillera-Arroita et al., 2015). provide a clear overview of how data characteristics determine how the predictions from species distribution models should be (but often are not) interpreted. Building on (Guillera-Arroita et al., 2015), Figure 1 illustrates how model interpretation varies depending on the type of estimates yielded by the model, i.e. rank suitability, relative likelihood, true probability of occurrence, or density, which is in turn influenced by the data type. In simple terms, when Ps decreases, our confidence in being able to have reliable Presence-Absence data decreases. Rank suitability estimation provides a ranked order of suitable sites for harbouring a VME, which is not proportional to the actual probability of occurrence, i.e. does not indicate how suitable a site is for harbouring a VME, with PS unreliable. In contrast to rank suitability, the relative likelihood is proportional to the probability of occurrence Pp. Both rank suitability and relative likelihood can be used to discriminate sites, i.e. distinguish between occupied and unoccupied sites better than random. However, to estimate the relative likelihood is not sufficient for comparing across multiple modelled taxa or habitats and/or regions. In order to estimate the true probability of occurrence for a VME, models need to be fitted on presence-absence data with reliable detectability of taxa Ps (Guillera-Arroita et al., 2015). Further, when abundance data are available, the model can estimate density or percentage cover of indicator taxa.
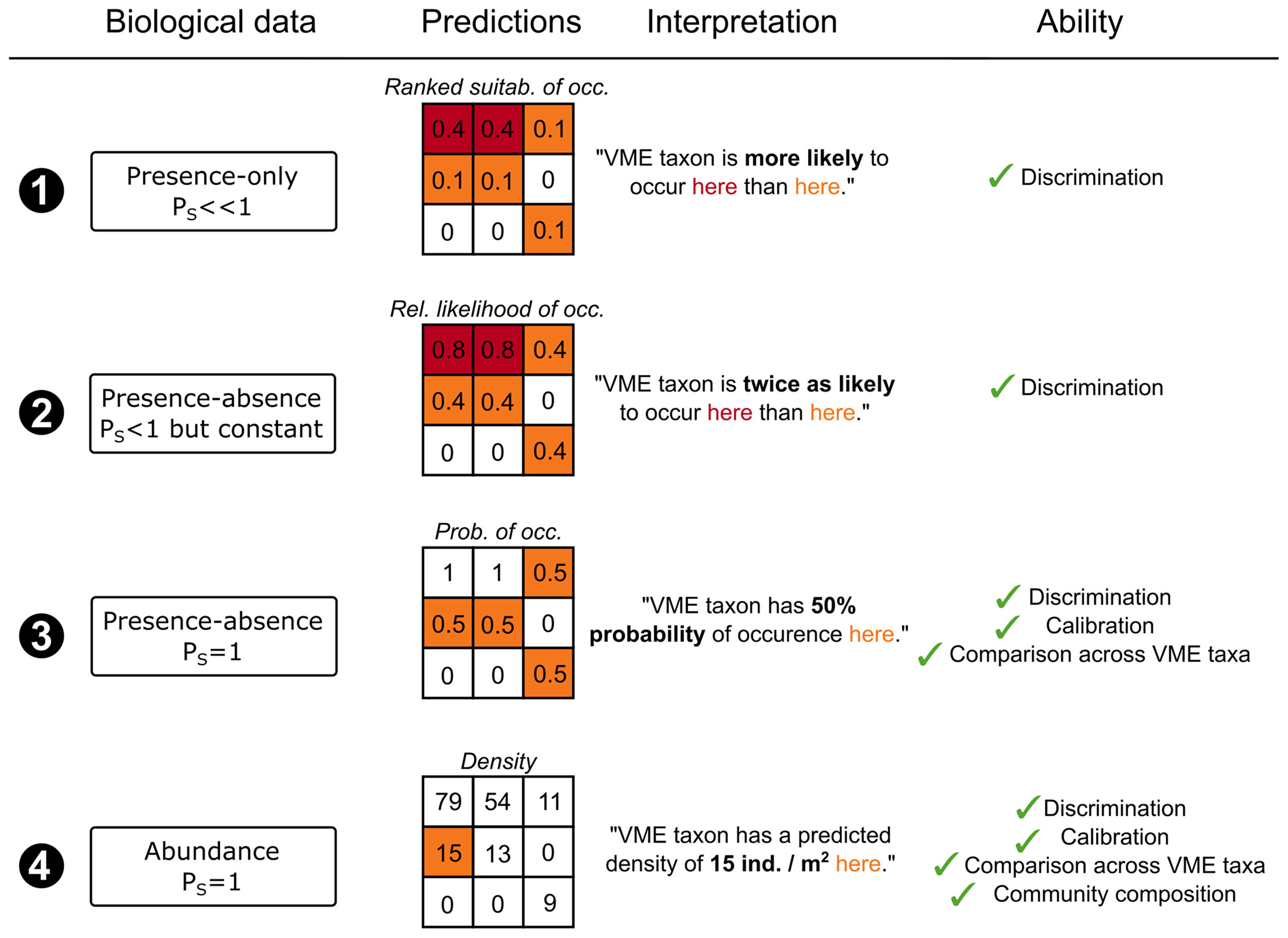
Figure 1 Influence of the VME data characteristics on the interpretability of the model’s predictions. The probability of detectability Ps directly impacts the characteristics of the model predictions, and therefore their interpretability. ind., individuals; occ., occurrence; Prob., probability; Rel., Relative; suitab., suitability; VME, Vulnerable Marine Ecosystem.
2.2 Physical Sampling
Fishing gears have generally a low catchability for benthic organisms, especially for small, fragile and brittle VME taxa (Auster et al., 2011; Watling and Auster, 2017). Fishing gears are typically designed to catch or attract motile species, as opposed to providing representative samples of VME indicator taxa: PS is small and unreliable. Moreover, there is a lack of consistency across fisheries management agencies regarding data collection of VME indicator taxa (Thompson et al., 2016). As a result, taxa may be present (and impacted) by fishing gears, but not all are necessarily caught, brought to the surface and/or reported (Knudby et al., 2013; Watling and Auster, 2017; Du Preez et al., 2020). Therefore, VME data acquired with fishing gear is widely considered as “Presence-only” data (i.e., no “true absence” data, P(0), available) with highly imperfect detection (i.e., PS << 1). With poor confidence in estimating PS, we are marginalising over PS to give a relative measure (see Figure 1). Besides fisheries bycatch data, some scientific physical sampling gear have been specifically designed for benthic ecosystems on soft substrates (Lauria et al., 2017), such as benthic sleds, yielding “Presence-absence” or “Abundance” data with reliable benthic species detectability Ps (Williams et al., 2015). However, physical sampling gear typically only allows indicator taxa collection, since the identification of habitats generally requires direct observation, e.g. via in situ imagery. Although VME data to date have been largely collected with physical sampling gear, these aforementioned restrictions, both on model predictions and the modelled biotic entity, impact the interpretation against the FAO criteria. Further, because physical sampling methods can permanently impact individuals and assemblages, these methods should be avoided for sampling vulnerable ecosystems (Williams et al., 2015).
2.3 Underwater Imagery Sampling
Underwater imagery allows sampling the seafloor with a reliable detectability of most taxa PS (Williams et al., 2015; Long et al., 2020). It is generally considered that “Presence-absence” data can be derived from these images since most VME taxa are sessile or with a limited mobility. This kind of data can be used to create models that are: (i) discriminative (i.e., occupied vs. unoccupied sites), (ii) well-calibrated (i.e., exact probability, not relative), and (iii) comparable among VME entities and/or regions (see Figure 1). Importantly, habitat identification and/or accurate VME taxa abundance can be extracted from images (Williams et al., 2015), which is important for addressing the FAO criteria. Underwater imagery allows finer model resolution, e.g. 25 m2 in (Rowden et al., 2017). Nevertheless, scientific surveys that utilise imagery are rare in the deep sea which restricts the scale of those datasets compared to the ones derived from fishing vessels. Taxonomic identification from images is also more prone to errors and uncertainties (e.g. taxonomic groupings) than when relying on physical samplings. Additionally, the resolution of environmental predictors needs to match the biological data, and high resolution environmental data has limited availability, which is another limitation to the usability of fine-resolution imagery data (Rengstorf et al., 2012). Overall, the sampling method limitations should be clearly communicated to the model’s users to guarantee a cautious interpretation of the modelling results.
3 Limited Translation of the FAO Criteria Into Modelling
The FAO guidelines are generic and non-quantitative, which makes their interpretation prone to expert-subjectivity (Howell et al., 2011; Watling and Auster, 2017). The UNGA explicitly left to the RFMO/As the responsibility to refine the VME definition relatively to their jurisdiction, which has led to differing interpretations by model-builders and experts in separate RFMO/As (Thompson et al., 2016; Bell et al., 2019; Long et al., 2020). Comparing the FAO VME identification criteria to the ones used in the Southern Ocean RFMO/A (CCAMLR), Ardron et al. (2014) showed that similar criteria have been defined differently (see their Table 1), and can therefore lead to different modelling interpretation. While the original rationale for the UNGA resolutions was to halt the impact to structurally complex, habitat forming, slow growing and brittle assemblages of deep-sea benthos, the lack of agreement as to what “vulnerability” means and what a VME is, has been a substantial time sink that has potentially delayed progress in evaluating and implementing management measures.
3.1 Lack of Consensus About How to Model a VME
In distribution modelling studies, VMEs have most often been considered as either assemblages dominated by a single indicator taxon or co-dominated by multiple indicator taxa. Within these two categories, different modelling approaches have been proposed, each with different implications for identifying VMEs using the FAO criteria. Figure 2 illustrates the different approaches based on how VMEs have been defined by model-builders.
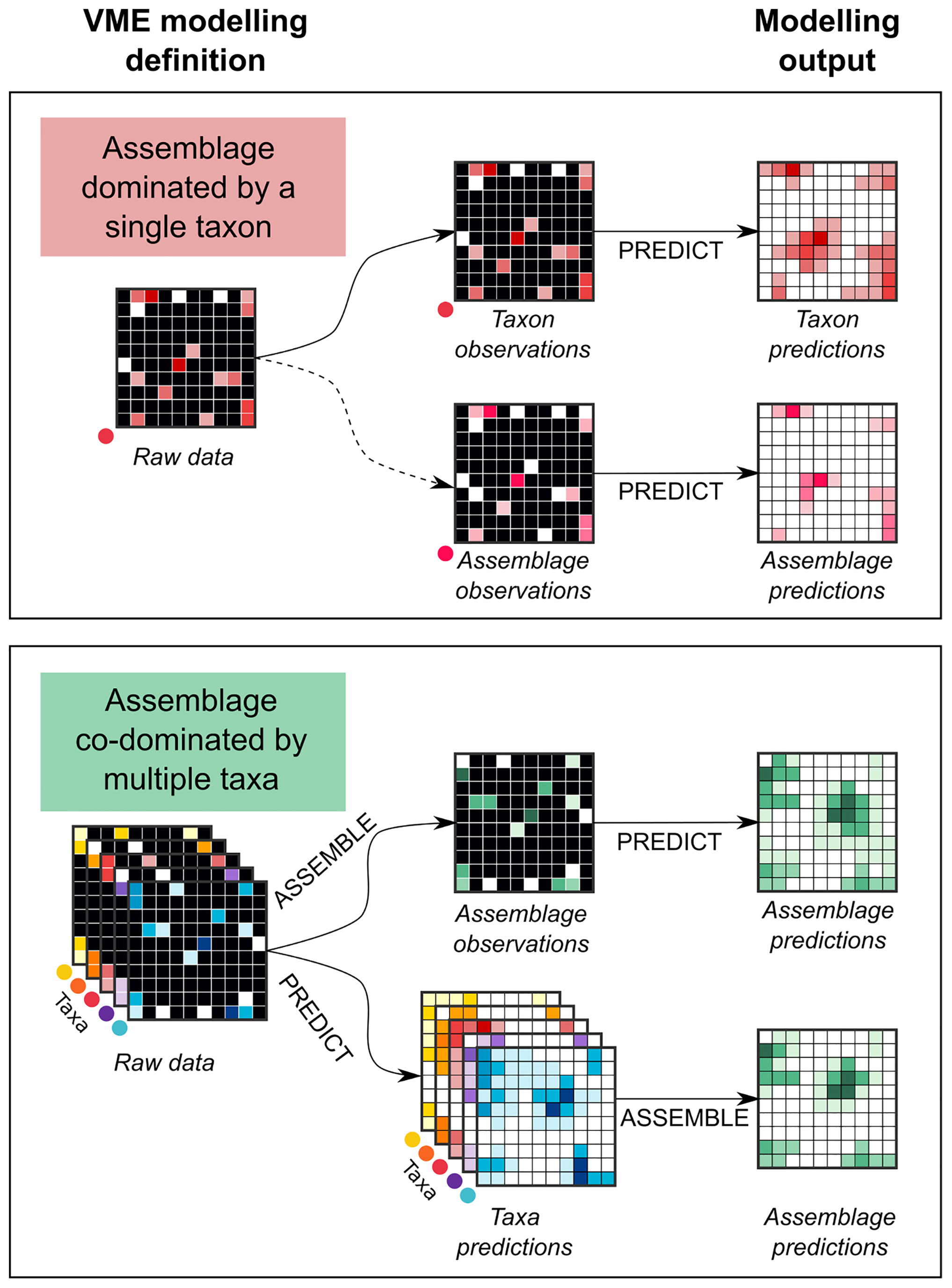
Figure 2 VME definition used in distribution modelling. VMEs can be modelled either considering them as an assemblage either dominated by a single taxon (top), or co-dominated by multiple taxa (bottom). In the first case, the modelling uses the indicator taxon distribution (e.g., cold-water coral Lophelia pertusa), or the assemblage dominated by this taxon (e.g., coral reef). When using the multiple taxa approach, the assemblage is modelled with either the “Assemble then Predict” or the “Predict then Assemble” approach.
When VMEs are defined as an assemblage dominated by a single indicator taxon, two approaches are usually employed: either by modelling the distribution of the indicator taxon, as a proxy for the associated assemblage, or by modelling the distribution of the assemblage itself (see Figure 2, top panel). Most studies model the distribution of the VME indicator taxon instead of the associated assemblage. However, the occurrence of a VME indicator taxon alone may not be sufficient to properly identify a VME (FAO, 2009; Howell et al., 2011; Watling and Auster, 2017), as it does not provide evidence of a habitat with important functional significance. The distribution of a VME assemblage often represents only a small subset of the distribution of its associated dominating indicator taxon, especially when the assemblage requires environmental factors that are more restrictive than for the occurrence of the taxon. For instance, Howell et al. (2011) model and compare the distribution of a cold-water coral (Lophelia pertusa, VME indicator taxon) vs. the reef it forms (assemblage forming a VME habitat), and show that the predicted area of high suitability for the taxon is much broader than for the assemblage (7.17% vs. 0.56% of the studied area). To model VMEs using assemblage data is however limited by the scarce availability of this data type, since it generally requires underwater imagery sampling (see section Connections Between the Type of VME Data and Model Use), and by the lack of consensus around the quantitative definition of VME assemblages, e.g. composition or density of taxa (Rowden et al., 2020).
When VMEs are defined as an assemblage of multiple indicator taxa, there are two components to the modelling approach: defining the assemblage (“Assemble”) and making predictions (“Predict”). These generally occur separately and can be in either order, i.e. “Assemble first then Predict” or “Predict first then Assemble”, as defined in (Ferrier and Guisan, 2006), see Figure 2 bottom panel. The “Assemble first then Predict” aims at predicting the occurrence of predefined benthic assemblages, e.g. circalittoral vertical rocky walls with Caryophyllia cyathus and Corallium rubrum in (Torriente et al., 2019). These assemblages can be defined by: (i) prior knowledge, e.g. biotopes known to occur in the area of interest (Bo et al., 2021), (ii) expert judgement using phylogenetic and functional diversity metrics, or (iii) cluster and ordination analysis (Barrio Froján et al., 2016; Torriente et al., 2019). Importantly, this approach cannot provide insights on environmental requirements of each taxon and can lead to an artificially wide niche description (Yesson et al., 2012; Brewin et al., 2020). The “Predict first then Assemble” approach models and predicts the spatial distribution of each VME taxon before assembling model outputs. It allows assemblage characterisation, e.g. species composition and their environmental requirements (Hill et al., 2020). For instance, (Chu et al., 2019) combined predictions from individual models developed for cold-water coral and sponge groups to identify areas as suitable assemblage for multiple biogenic habitat-forming species. In VME studies, the individual taxon predictions have mostly been assembled based on a prior beliefs about how assemblages are structured [e.g. grouping by higher taxonomic level (Yesson et al., 2012) or functionality (Davies and Guinotte, 2011)], which may not reflect real distribution patterns or confer a limited ecological interpretability (Jansen et al., 2018).
3.2 Lack of Quantitative Standards of What Constitutes a VME
To identify a VME in accordance with the FAO guidelines, the five criteria need to be encoded into the modelling approach (Table 1). However, most VME distribution modelling studies focused on predicting suitable locations for VME indicator taxa, which provides an incomplete assessment according to the FAO multi-criteria guidelines. Nevertheless, the translation of the FAO criteria into modelling terms remains unclear, especially for the “functionality” and “structural complexity” criteria. The “functionality” of an ecosystem cannot be easily modelled given the current FAO VME definition and the available knowledge of these remote ecosystems. The “structural complexity” of a habitat can potentially be assessed if abundance data with excellent detectability is available, e.g. by characterising the density and/or the morphologies of the biotic and abiotic features.
The FAO guidelines do not specify what constitutes a “significant concentration” (Auster et al., 2011; Vierod et al., 2014; Rowden et al., 2020). A quantitative criterion is needed to distinguish dense aggregations of VME taxa that form a “structurally complex habitat” from low frequency occurrences of VME indicator taxa. Most RFMO/As have established operational definitions based on thresholds of VME indicator taxa caught as fisheries bycatch (Bell et al., 2019), which then triggers precautionary management actions, e.g. CCAMLR conservation measure CM 22-07 (CCAMLR, 2013). However, the relevance of these operational encounter thresholds has been questioned (Auster et al., 2011; Ardron et al., 2014; Watling and Auster, 2017; Geange et al., 2020) because they (i) have undergone limited scientific validation and, (ii) are not taxon specific, and therefore do not take into account the differential catchability of taxa and their differential vulnerability, distribution or life history characteristics. In addition, VME extent is operationally defined in many RFMO/As by an arbitrary distance around the location where a certain amount of VME indicator taxa has been encountered, e.g. CCAMLR conservation measure CM 22-07 (CCAMLR, 2013), often without further scientific investigation. Further work is then needed to establish quantitative references, scientifically validated, of what constitutes a VME, both in terms of taxon abundance and VME extent.
4 Guidelines to Match the Modelling to the Need
The challenges of VME distribution modelling related to the type of data used (section Connections Between the Type of VME Data and Model Use) can be handled in different ways depending on the intended application. In this section, we critically review published VME distribution modelling studies and provide guidelines for seven common applications, in order to help design studies fit for their purposes.
4.1 Locate Undiscovered VME to Guide Scientific Surveys
With the vast majority of the deep-sea being unsampled (Clark et al., 2016b), distribution modelling has the potential to play an important role in guiding scientific surveys aiming to locate undiscovered VME (Chu et al., 2019; Baco et al., 2020; Long et al., 2020). For this application, models with large spatial scale and coarse resolution can be employed to broadly identify areas that may harbour VME (Vierod et al., 2014). Presence-only data from unstructured and opportunistic datasets can be used to identify the most suitable sites for a particular VME taxon occurrence. For instance, (Yesson et al., 2017) model the rank suitability of black corals using presence-only data with PS<<1 or inconsistent PS across the collated datasets (see Figure 1 case 1, “Presence-only PS<<1”), at a global scale and with a 1 km2 resolution. At a smaller scale, distribution modelling can also be used to enhance sampling programs in areas where data availability is limited, e.g. by filling gaps in survey coverage (Kenchington et al., 2014).
4.2 Appraise Spatial Closures Effectiveness for VME Protection
Spatial closures are widely recognised as the most effective method for avoiding serious adverse impacts on VME (Bell et al., 2019). Distribution modelling has increasingly been employed to assess their effectiveness and relevance for VME protection, e.g. (Lagasse et al., 2015; Rowden et al., 2017; Buhl-Mortensen et al., 2019; Georgian et al., 2019; Torriente et al., 2019) or the recent protection effectiveness assessment by the Northwest Atlantic Fisheries Organization (Kenchington et al., 2019b). To assess whether the policy driven conservation targets are met or not, a common metric is the percentage of VME spatial distribution included in protected areas. For this application, modelling the relative likelihood of occurrence, e.g. using Presence-absence data with PS < 1 but constant, can be sufficient, see section Connections Between the Type of VME Data and Model Use. For instance, (Howell et al., 2016) model the relative likelihood of sponge grounds to quantify the percentage of these that are protected by reserves. Ross et al. (2015) study the influence of the model resolution on the estimation of this metric and draw two important conclusions: (i) low resolution models are sufficient for the assessment of marine reserve effectiveness at regional scales, e.g. to appraise the progression towards protection targets, (ii) high resolution models are recommended for detailed reserve design at local scales, because of their better accuracy in detecting key habitat occurrences. Alternatively, another relevant metric for this application is the absolute VME spatial extent contained in the reserve system (Williams et al., 2020), for which the estimation of the true probability of occurrence is needed, see model requirements in section Connections Between the Type of VME Data and Model Use. Even when the data exist to model the true probability of occurrence, Ross et al. (2015) show that predicted VME spatial extents vary with model resolution without consistent direction and proportion across habitats (i.e., different taxa respond differently to a change in model resolution). This means that absolute extent predictions should be interpreted with caution. For a more robust VME extent estimation, we recommend the use of an abundance-based model (instead of probability of occurrence), e.g. using underwater imagery data and the method proposed by Williams et al. (2020).
4.3 Describe the Spatial Connectivity Between VME
Structural and functional connectivity among protected areas is an important aspect of conservation management and planning. Often used as a proxy for resilience, the analysis of population connectivity is especially valuable for VME indicator taxa because of their late maturity, low fecundity and with uncertain recruitment sources (Kenchington et al., 2019a; Rowden et al., 2019). For this application, it is important to consider the entire VME indicator taxon distribution (i.e., all species occurrences, not only the associated VME habitat distribution) since the connectivity between habitats (e.g., coral reefs) is likely to be maintained by the wider species distribution (Howell et al., 2011). Taxa distribution and dispersal models have been recently compared to investigate connectivity between VME, in order to study how many interbreeding populations are present within a given metapopulation (Ross et al., 2019) or to analyse oceanographic features that modulate larval dispersal connectivity (Kenchington et al., 2019a). Although highly relevant for conservation assessments, the feasibility of these studies remains hampered by our limited knowledge on ecological processes influencing these rare and remote communities (Parker and Bowden, 2010).
4.4 Characterize the Community Composition of VME
Distribution modelling can be used to describe the community composition of VME, e.g. the diversity and abundance of VME indicator taxa or the association with other macrofauna. Community characterisation is highly relevant during site prioritisation of management planning. In the early stages of management planning, models estimating relative likelihood of occurrence can be valuable to identify sites that are entirely unsuitable for VME (where risks from a proposed activity are minimal), or to rank vulnerable sites within the same modelled region (Baker et al., 2021). However, to prioritize sites with a high VME taxa biodiversity, e.g. based on species richness mapping, the use of probability or abundance-based models is recommended (Gonzalez-Mirelis et al., 2021), see requirements in section Connections Between the Type of VME Data and Model Use. Besides the VME protection, some studies examine spatial correlations between VME and other macrofauna presence or abundance, e.g. (Stone, 2006; Tissot et al., 2006; Kenchington et al., 2013). For instance, Ashford et al. (2019) map the relative biomass of several VME indicator taxa to analyse the community structure and their influence on precacarid assemblages. More community-oriented studies are needed to better understand the functionality of VME taxa, such as their association with commercially important deep-sea fishes.
4.5 Assess the Impact of Anthropogenic Activities on VME
The reduction of significant adverse impacts from human activities on VME, as urged by the UNGA 61/105 resolution (UNGA, 2006), is reliant upon a comprehensive impact assessment, for which distribution modelling can play a key role (Gianni et al., 2016; Bell et al., 2019). For this purpose, we recommend comparing the predicted distribution of VME taxa with fishing footprint, as done by Brewin et al. (2020) which assessed the impact of fishing activities on VME across adjacent areas with different management policies. Using species distribution modelling with environmental and fisheries variables, Lauria et al. (2017) showed that two VME indicator taxa have a very different vulnerability to fishing trawlers, since the predicted distribution of one studied taxon overlapped with fishing activities while the other is estimated to only occurs where the fishing is low or absent. Besides fisheries pressure, distribution modelling can also be used to assess impact of natural disasters on VME (Georgian et al., 2020). Ideally, the damage or threat needs to be characterised against different factors, such as intensity, timing and duration of the impact, its spatial extent, and the VME’s ability to recover (Penney and Guinotte, 2013). From these characteristics, the ecosystem’s intactness (i.e. naturalness: lack of or low level of human induced disturbance or degradation) can be derived by adjusting the model outputs with the recorded human activity, e.g. by discounting the VME predictions as per the bottom-trawling impact (Ardron et al., 2014). VME intactness mapping can guide decision-makers towards maximising the protection of VME that are still intact but fall within fishing depths, or monitoring the recovery of VME that have already been significantly damaged.
4.6 Understand the Environmental Drivers of VME
Besides the spatial distribution, some user groups are also interested in understanding the relationship of VME with environmental conditions. For this application, distribution modelling is mainly used to synthesize the available knowledge (Etnoyer et al., 2018; Lauria et al., 2021), for instance to define what are the primary drivers of a functional VME unit, e.g. (Williams et al., 2020). Importantly, VME characterisation in terms of terrain parameters is highly scale and resolution dependent (Davies and Guinotte, 2011; Rengstorf et al., 2012), which emphasizes the importance of correct spatial matching between response data and predictors for this application (Ross et al., 2015).
4.7 Assess the Impact of Climate Change on VME
Distribution modelling can also deepen our understanding on how climate change is leading to shifts in VME distributions (Morato et al., 2020; Tittensor et al., 2010), which is critically important when developing management plans (Levin and Bris, 2015; Pecl et al., 2017; Sweetman et al., 2017; Johnson et al., 2018). To achieve this, VME distribution models can be fitted with current environmental conditions, and be used to infer distribution changes under future climate projections. If the model-users are only interested in relative changes, models estimating the relative likelihood of VME occurrence can be suitable, see requirements in section Connections Between the Type of VME Data and Model Use. In contrast, models able to estimate the true probability of occurrence are required in order to compare the VME taxa persistence or the changes in VME spatial extent across habitats. Further, the “Assemble first then Predict” approach is not suitable for this application since taxa may be expected to respond differently to future environmental conditions. As an example for this application, (Morato et al., 2020) recently inferred the rank suitability of six cold-water coral species occurrence under the business-as-usual emissions trajectory RCP8.5 for the period 2081–2100, aiming to identify key refugia areas for VME taxa. Their results suggest an alarming decrease in suitable habitat, ranging from 28% to 100% depending on the VME taxa. For these analyses, we however recommend acknowledging the various sources of uncertainty that can limit their accuracy, such as species evolutionary changes and acclimation (Morato et al., 2020).
5 Making VME Distribution Models More Relevant for Conservation and Management Decisions
VME distribution models have had limited impact on conservation and management measures to date, partly because their outputs cannot be directly assessed against the FAO criteria and because quantitative standards of what constitutes a VME are lacking (Rowden et al., 2017; Morato et al., 2018; Rowden et al., 2020). To tackle these issues, our recommendations for the future generation VME models are threefold: VME identification from models should (i) be ruled by a specific and quantitative definition of what constitutes a VME, (ii) assess the entire ecosystem conservation value instead of focusing on a single indicator taxon distribution, and (iii) model the vulnerability of an area instead of its suitability to harbour a vulnerable species. It is important to note that these avenues are still under active research and there is currently no clear consensus on how to best tackle these issues. The following subsections give an overview of promising recent approaches, which will need further validation. In doing so, we are encouraging future modelling studies to explore these perspectives.
5.1 Establish a Quantitative and More Specific Definition of VME
The FAO VME identification guidelines are policy-oriented and their translation into modelling is not straightforward (see section Lack of Quantitative Standards of What Constitutes a VME). There is a need for VME identification criteria which are both model-oriented and user-specific. Therefore, we recommend early and iterative communication between model-users and -builders to converge on quantitative VME identification criteria that are suitable to the user’s need. To find a multi-country and multi-stakeholder consensus is often challenging. Therefore, the involvement of as many parties as possible during the different steps of the modelling process increases the likelihood of models’ acceptance and of a successful spatial management planning outcome (Ardron et al., 2014; Rowden et al., 2019).
Unlike the occurrence or abundance of a species which are absolute measures, vulnerability is relative and qualitative, i.e. it is a spectrum, from hardy and resilient to fragile and vulnerable, and dependent on a specific threat, specific region, etc. To ensure an adequate and precise interpretation of the model’s outputs, VME distribution models should be based on criteria that are specific and a priori clearly communicated to model-users. Vulnerability criteria should be defined relative to specific threat (e.g., fishing vs. deep sea mining) and to the characteristics of the VME data available for modelling (e.g., model cut-off values should consider the detectability of the sampling method used to collect the training data). For regional models, the VME policy-oriented criteria defined by some RFMO/As for their area of jurisdiction constitute a relevant starting point. For instance, CCAMLR considers the motility and the larval dispersal as additional VME criteria, as relevant aspects for the Southern Ocean context and the longline fishery (CCAMLR, 2009). To date, VMEs have been mainly modelled in regard to the fishing threat. For applications not only focusing on fisheries management, the seven criteria developed by the Convention on Biological Diversity to identify Ecologically or Biologically Significant Areas (EBSA) should be taken into consideration (Dunn et al., 2014). As shown by (Ardron et al., 2014), the EBSA identification criteria complement the FAO VME criteria with the assessment of the area naturalness (or ecosystem intactness, i.e., lack of or low level of human induced disturbance or degradation), the biological productivity and diversity [see (Gonzalez-Mirelis et al., 2021)].
Establishing quantitative-based criteria of what constitutes a VME is needed to impede subjectivity in VME identification. To reach a consensual definition of an ecosystem vulnerability, the establishment of cut-off values, data driven and scientifically validated, is needed (Kenchington et al., 2014; Geange et al., 2020; Rowden et al., 2020). To this end, Rowden et al. (2020) model the relationships between the density of a habitat-forming taxon and the number of other epifauna taxa, i.e. correlation between the amount of structurally complex habitat and the associated species richness. This analysis facilitates the translation of the subjective “Structural complexity” FAO criterion into a quantitative model-oriented criterion by determining percentage cover thresholds that are representative of structurally complex habitats. Such methodology has the potential to operationalise FAO’s criteria while providing distribution models with a data-driven and scientifically validated reference to identify VMEs.
5.2 Assess Site Conservation Value in Relation to VME Multi-Taxa Spatial Predictions
While most VME modelling studies focus on the distribution of a single indicator taxon, epibenthic community composition analyses would provide a more relevant assessment of both the ecological functionality and structural complexity of an ecosystem, as per key FAO VME criteria. Therefore, we recommend modelling the full list of VME taxa instead of focusing on a single taxon or habitat, and to assess the conservation value of the predicted hotspots by characterizing the whole epibenthic community in relation to the predicted VME taxa distributions.
To model the distribution of multiple species, approaches with joint responses among the species, such as Hierarchical Modelling of Species Communities (Tikhonov et al., 2020), Species Archetype Model (Dunstan et al., 2011; Hui et al., 2013) or Regions of Common Profiles model (Foster et al., 2013), should be investigated as they can directly reveal the VME’ community structure (Chu et al., 2019). Besides the assemblage approaches commonly used in the VME literature (see bottom panel of Figure 2), future studies could consider the “Analyse simultaneously” approach which concurrently correlates biological with environmental data to generate regions containing relative homogenous and distinct assemblages within a single model (Hill et al., 2020).
Once hotspots of VME taxa are identified, the ecological value of a site can be evaluated in respect to the biodiversity and productivity of the entire epibenthic community, e.g. by computing the total epibenthic megafauna richness and abundance (Dunstan et al., 2012; Gonzalez-Mirelis et al., 2021). The total epibenthic megafauna richness and abundance can arguably be used as relevant quantitative indicators to assess the “structural complexity” and “functionality” FAO criteria (Gonzalez-Mirelis et al., 2021). Further, since biodiversity and productivity are part of the EBSA identification process, their quantification in VME distribution studies supports a better incorporation of VME protection within management tools across sectoral authorities and international agreements.
The use of abundance data extracted from underwater imagery is preferable for conducting the community-oriented modelling described above. Imagery-based abundance data provides a significantly more reliable, comprehensive and non-destructive characterization of benthic communities than other sampling methods (see section Connections Between the Type of VME Data and Model Use). Besides, the visual assessment allowed by images is more compelling for non-specialists such as policymakers. Rapid advances in imaging technologies have recently reduced costs and thus increased underwater imagery coverage (Morato et al., 2018). Further, there has been a substantial global effort to make these images publicly available, e.g. MAREANO video database (Buhl-Mortensen et al., 2015). To increase the spatial and environmental coverage of the training dataset, we encourage model-builders to collate all available data that have been already acquired across the studied region, even when not annotated yet (Etnoyer et al., 2018; Lauria et al., 2021). Noteworthy recent efforts have been made to standardize protocols for VME identification (Baco-Taylor et al., 2020) and marine reference catalogues (Howell et al., 2019; Horton et al., 2021), both from underwater imagery. While indicator taxa are often defined with a low taxonomic resolution by RFMO/As, the use of images opens the door to higher resolution identifications, which can provide a finer characterization of ecosystems. In addition, taxonomic groups can be split based on morphological characteristics, e.g. using morpho-taxonomic labels (Gonzalez-Mirelis et al., 2021), or functional traits in order to further appraise the ecosystem vulnerability, e.g. “Fragility” FAO criterion. All in all, the increase of both quantity and quality of abundance data is likely to underpin the next generation VME distribution models.
5.3 Model the Vulnerability of Marine Ecosystems
Most published VME distribution models have focused on mapping the occurrence of indicator taxa, which only provides a partial assessment of the entire ecosystem vulnerability. VMEs should instead be identified on the basis of the full suite of vulnerability criteria outlined in the FAO Guidelines (Gianni et al., 2016). Therefore, we recommend future studies to model the vulnerability of an area, rather than its suitability to harbour an indicator taxon. To map the vulnerability of an area, Morato et al. (2018) propose weighting the VME taxa abundance data by taxa-specific scores for each of the five FAO criteria. This multi-criteria assessment method results in the mapping of a single composite metric, the “VME index”, which represents where a particular location lies on the vulnerability spectrum. Some drawbacks are however inherent to such composite score calculation (Ardron et al., 2014), such as sometimes leading to prioritizing sites harbouring a large number of taxa with low vulnerability, over a habitat dominated by a highly vulnerable taxon. There is also no global consensus on what would be the optimal integration method for the criteria to provide a single conservation value to an area (Yamakita et al., 2017). Nevertheless, this methodology has been adopted by the International Council for the Exploration of the Sea (ICES) in the North-East Atlantic (ICES, 2019), and has been recently used to generate the “North Atlantic Ocean basin-scale VME index dataset” (Morato et al., 2020). Building on Morato et al. (2018) method, Burgos et al. (2020) map the “VME index” beyond observed sites using the predictions of VME taxa distribution models. Avenues of improvement include: (i) to use joint-distribution modelling approaches, as detailed in section Appraise Spatial Closures Effectiveness for VME Protection, and (ii) to derive vulnerability scores for each taxon from imagery-based analyses that are less prone to expert subjectivity because data-driven and scientifically validated, see section Locate Undiscovered VME to Guide Scientific Surveys.
6 Conclusion
Distribution modelling will have an increasingly important role to play in underpinning the next generation of targeted VME management and conservation measures. VME distribution modelling will benefit from the recent increase in both VME data quality and quantity, but several challenges will need to be addressed to make modelling efforts relevant and impactful. First, it is crucial that model-builders understand the connection between the type of data used to build models and what such models are able to estimate to ensure they are appropriate for the intended application. Second, a limitation in the general applicability of VME models occurs partly because VME identification often relies on subjective standards and quantitative standards of what constitutes a VME are lacking (Rowden et al., 2017; Morato et al., 2018; Rowden et al., 2020). We strongly recommend that scientists and RFMO/As work towards generating quantitative definitions and modelling approaches that (i) consider the whole ecosystem instead of focusing on a single indicator taxon, and (ii) model the vulnerability of an area instead of just its suitability to harbour a vulnerable species. To this end, the combination of models that simultaneously quantify the distribution of a suite of VME taxa with a tailored VME index that considers multiple aspects of the FAO criteria appears a promising way forward. The present work comes at a critical time where there is increased international focus on the sustainable use of oceans, particularly in Areas Beyond National Jurisdiction. International negotiations in Convention on Biological Diversity and Biodiversity Beyond National Jurisdiction are reaching a stage of maturity where having a science- and data-based framework is important, wherein VME distribution modelling has a key role to play.
Author Contributions
CG: Conceptualization, Investigation, Writing – original draft preparation; JJ: Writing – review and editing, Supervision; PD: Writing – review and editing; DW: Writing – review and editing; NH: Conceptualization, Writing – review and editing, Supervision. All authors contributed to the article and approved the submitted version.
Funding
CG has a scholarship from IMAS 'Quantitative Marine Science' program. This research was supported by the Australian Government through the Australian Research Council’s Discovery Projects funding scheme (project DP190101858).
Conflict of Interest
The authors declare that the research was conducted in the absence of any commercial or financial relationships that could be construed as a potential conflict of interest.
Publisher’s Note
All claims expressed in this article are solely those of the authors and do not necessarily represent those of their affiliated organizations, or those of the publisher, the editors and the reviewers. Any product that may be evaluated in this article, or claim that may be made by its manufacturer, is not guaranteed or endorsed by the publisher.
Acknowledgments
We acknowledge that the work that led to this article was carried out in the country of the palawa pakana people, and pay our respects to them as Traditional Owners and ongoing custodians of lutruwita (Tasmania). We would like to thank the editor for handling the manuscript, and the three reviewers for their very insightful comments and suggestions, which greatly improved the quality of this manuscript.
References
Ardron J. A., Clark M. R., Penney A. J., Hourigan T. F., Rowden A. A., Dunstan P. K., et al. (2014). A Systematic Approach Towards the Identification and Protection of Vulnerable Marine Ecosystems. Mar. Policy 49, 146–154. doi: 10.1016/j.marpol.2013.11.017
Ashford O. S., Kenny A. J., Barrio Froján C. R. S., Downie A.-L., Horton T., Rogers A. D. (2019). On the Influence of Vulnerable Marine Ecosystem Habitats on Peracarid Crustacean Assemblages in the Northwest Atlantic Fisheries Organisation Regulatory Area. Front. Mar. Sci. 6. doi: 10.3389/fmars.2019.00401
Auster P. J., Gjerde K., Heupel E., Watling L., Grehan A., Rogers A. D. (2011). Definition and Detection of Vulnerable Marine Ecosystems on the High Seas: Problems With the “Move-On” Rule. ICES J. Mar. Sci. 68, 254–264. doi: 10.1093/icesjms/fsq074
Baco A. R., Morgan N. B., Roark E. B. (2020). Observations of Vulnerable Marine Ecosystems and Significant Adverse Impacts on High Seas Seamounts of the Northwestern Hawaiian Ridge and Emperor Seamount Chain. Mar. Policy 115, 103834. doi: 10.1016/j.marpol.2020.103834
Baco-Taylor A., Ross R., Althaus F., Bridges A., Brix S., Colaço A., et al. (2020). “A Community Consensus on Designating Vulnerable Marine Ecosystems From Imagery,” in Ocean Sciences Meeting 2020 (San Diego, California: AGU).
Baker D. J., Maclean I. M., Goodall M., Gaston K. J. (2021). Species Distribution Modelling Is Needed to Support Ecological Impact Assessments. J. Appl. Ecol. 58, 21–26. doi: 10.1111/1365-2664.13782
Barrio Froján C., Downie A.-L., Sacau Cuadrado M., Kenchington E., Kenny A. (2016). Evaluation of Benthic Assemblage Structure in the NAFO Regulatory Area With Regard to the Protection of VME. ICES J. Mar. Sci. 73, 405–419. doi: 10.1093/icesjms/fsv186
Bell J., Guijarro-García E., Kenny A. (2019) Scientific Approaches for the Assessment and Management of Deep-Sea Fisheries and Ecosystems in RFMOs and RFB : Final Report (Publications Office of the European Union). Available at: http://op.europa.eu/en/publication-detail/-/publication/0f2b559b-4610-11e9-a8ed-01aa75ed71a1 (Accessed April 28, 2020).
Blicher M. E., Arboe N. H. (2021). Atlas of Vulnerable Marine Ecosystem (VME) Indicators Observed on Bottom Trawl Surveys in Greenland Waters During 2015-2019. (2016) 113. Greenland Institute of Natural Resources. doi: 10.13140/RG.2.2.35637.81120
Bo M., Coppari M., Betti F., Enrichetti F., Bertolino M., Massa F., et al. (2021). The High Biodiversity and Vulnerability of Two Mediterranean Bathyal Seamounts Support the Need for Creating Offshore Protected Areas. Aquat. Conserv. Mar. Freshw. Ecosyst. 31, 543–566. doi: 10.1002/aqc.3456
Brewin P. E., Farrugia T. J., Jenkins C., Brickle P. (2020). Straddling the Line: High Potential Impact on Vulnerable Marine Ecosystems by Bottom-Set Longline Fishing in Unregulated Areas Beyond National Jurisdiction. ICES J. Mar. Sci. 78:2132-45. doi: 10.1093/icesjms/fsaa106
Buhl-Mortensen L., Buhl-Mortensen P., Dolan M. F. J., Holte B. (2015). The MAREANO Programme – A Full Coverage Mapping of the Norwegian Off-Shore Benthic Environment and Fauna. Mar. Biol. Res. 11, 4–17. doi: 10.1080/17451000.2014.952312
Buhl-Mortensen L., Burgos J. M., Steingrund P., Buhl-Mortensen P., Ólafsdóttir S. H., Ragnarsson S. Á. (2019). Vulnerable Marine Ecosystems (VMEs) (2019:519). Nordic. Counc. Minist. doi: 10.6027/TN2019-519
Burgos J. M., Buhl-Mortensen L., Buhl-Mortensen P., Ólafsdóttir S. H., Steingrund P., Ragnarsson S. Á., et al. (2020). Predicting the Distribution of Indicator Taxa of Vulnerable Marine Ecosystems in the Arctic and Sub-Arctic Waters of the Nordic Seas. Front. Mar. Sci. 7. doi: 10.3389/fmars.2020.00131
Carbonara P., Zupa W., Follesa M. C., Cau A., Capezzuto F., Chimienti G., et al. (2020). Exploring a Deep-Sea Vulnerable Marine Ecosystem: Isidella Elongata (Esper 1788) Species Assemblages in the Western and Central Mediterranean. Deep. Sea. Res. Part Oceanogr. Res. Pap. 166, 103406. doi: 10.1016/j.dsr.2020.103406
CCAMLR (2009). Report of the Workshop on Vulnerable Marine Ecosystems (La Jolla, California, USA: CCAMLR).
CCAMLR (2013) Conservation Measure 22-07 (CCAMLR). Available at: https://www.ccamlr.org/en/measure-22-07-2013 (Accessed May 4, 2021).
Chimienti G., Bo M., Mastrototaro F. (2018). Know the Distribution to Assess the Changes: Mediterranean Cold-Water Coral Bioconstructions. Rendiconti. Lincei Sci. Fis. E. Nat. 29, 583–588. doi: 10.1007/s12210-018-0718-3
Chu J. W. F., Nephin J., Georgian S., Knudby A., Rooper C., Gale K. S. P. (2019). Modelling the Environmental Niche Space and Distributions of Cold-Water Corals and Sponges in the Canadian Northeast Pacific Ocean. Deep. Sea. Res. Part Oceanogr. Res. Pap. 151, 103063. doi: 10.1016/j.dsr.2019.06.009
Clark M. R., Althaus F., Schlacher T. A., Williams A., Bowden D. A., Rowden A. A. (2016a). The Impacts of Deep-Sea Fisheries on Benthic Communities: A Review. ICES J. Mar. Sci. 73, i51–i69. doi: 10.1093/icesjms/fsv123
Clark M. R., Consalvey M., Rowden A. A. (2016b). Biological Sampling in the Deep Sea (Hoboken, New Jersey, USA: John Wiley and Sons).
Danovaro R., Corinaldesi C., Dell’Anno A., Snelgrove P. V. R. (2017). The Deep-Sea Under Global Change. Curr. Biol. 27, R461–R465. doi: 10.1016/j.cub.2017.02.046
Dautova T. N., Galkin S. V., Tabachnik K. R., Minin K. V., Kireev P. A., Moskovtseva A. V., et al. (2019). The First Data on the Structure of Vulnerable Marine Ecosystems of the Emperor Chain Seamounts: Indicator Taxa, Landscapes, and Biogeography. Russ. J. Mar. Biol. 45, 408–417. doi: 10.1134/S1063074019060026
Davies A. J., Guinotte J. M. (2011). Global Habitat Suitability for Framework-Forming Cold-Water Corals. PloS One 6 (4), e18483. doi: 10.1371/journal.pone.0018483
Dunn D. C., Ardron J., Bax N., Bernal P., Cleary J., Cresswell I., et al. (2014). The Convention on Biological Diversity’s Ecologically or Biologically Significant Areas: Origins, Development, and Current Status. Mar. Policy 49, 137–145. doi: 10.1016/j.marpol.2013.12.002
Dunstan P. K., Bax N. J., Foster S. D., Williams A., Althaus F. (2012). Identifying Hotspots for Biodiversity Management Using Rank Abundance Distributions. Divers. Distrib. 18, 22–32. doi: 10.1111/j.1472-4642.2011.00838.x
Dunstan P. K., Foster S. D., Darnell R. (2011). Model Based Grouping of Species Across Environmental Gradients. Ecol. Model. 222, 955–963. doi: 10.1016/j.ecolmodel.2010.11.030
Du Preez C., Swan K. D., Curtis J. M. R. (2020). Cold-Water Corals and Other Vulnerable Biological Structures on a North Pacific Seamount After Half a Century of Fishing. Front. Mar. Sci. 7. doi: 10.3389/fmars.2020.00017
Durán Muñoz P., Sacau M., García-Alegre A., Román E. (2020). Cold-Water Corals and Deep-Sea Sponges by-Catch Mitigation: Dealing With Groundfish Survey Data in the Management of the Northwest Atlantic Ocean High Seas Fisheries. Mar. Policy 116, 103712. doi: 10.1016/j.marpol.2019.103712
Etnoyer P. J., Wagner D., Fowle H. A., Poti M., Kinlan B., Georgian S. E., et al. (2018). Models of Habitat Suitability, Size, and Age-Class Structure for the Deep-Sea Black Coral Leiopathes Glaberrima in the Gulf of Mexico. Deep. Sea. Res. Part II. Top. Stud. Oceanogr. 150, 218–228. doi: 10.1016/j.dsr2.2017.10.008
FAO (2009). International Guidelines for the Management of Deep-Sea Fisheries in the High Seas (Rome, Italy: Food and Agriculture Organization of the United Nations).
Ferrier S., Guisan A. (2006). Spatial Modelling of Biodiversity at the Community Level. J. Appl. Ecol. 43, 393–404. doi: 10.1111/j.1365-2664.2006.01149.x
Foster S. D., Givens G. H., Dornan G. J., Dunstan P. K., Darnell R. (2013). Modelling Biological Regions From Multi-Species and Environmental Data. Environmetrics 24, 489–499. doi: 10.1002/env.2245
Geange S. W., Rowden A. A., Nicol S., Bock T., Cryer M. (2020). A Data-Informed Approach for Identifying Move-On Encounter Thresholds for Vulnerable Marine Ecosystem Indicator Taxa. Front. Mar. Sci. 7. doi: 10.3389/fmars.2020.00155
Georgian S. E., Anderson O. F., Rowden A. A. (2019). Ensemble Habitat Suitability Modeling of Vulnerable Marine Ecosystem Indicator Taxa to Inform Deep-Sea Fisheries Management in the South Pacific Ocean. Fish. Res. 211, 256–274. doi: 10.1016/j.fishres.2018.11.020
Georgian S. E., Kramer K., Saunders M., Shedd W., Roberts H., Lewis C., et al. (2020). Habitat Suitability Modelling to Predict the Spatial Distribution of Cold-Water Coral Communities Affected by the Deepwater Horizon Oil Spill. J. Biogeogr. 47 (7), 1455–1466. doi: 10.1111/jbi.13844
Gianni M., Fuller S. D., Currie D. E. J., Schleit K., Goldsworthy L., Pike B., et al. (2016). How Much Longer Will It Take? A Ten-Year Review of the Implementation of United Nations General Assembly Resolutions 61/105, 64/72 and 66/68 on the Management of Bottom Fisheries in Areas Beyond National Jurisdiction. Deep Sea Conservation Coalition 61, 64.
Gonzalez-Mirelis G., Ross R. E., Albretsen J., Buhl-Mortensen P. (2021). Modeling the Distribution of Habitat-Forming, Deep-Sea Sponges in the Barents Sea: The Value of Data. Front. Mar. Sci 1098. doi: 10.3389/fmars.2020.496688
Guillera-Arroita G., Lahoz-Monfort J. J., Elith J., Gordon A., Kujala H., Lentini P. E., et al. (2015). Is My Species Distribution Model Fit for Purpose? Matching Data and Models to Applications. Glob. Ecol. Biogeogr. 24, 276–292. doi: 10.1111/geb.12268
Hill N., Woolley S. N. C., Foster S., Dunstan P. K., McKinlay J., Ovaskainen O., et al. (2020). Determining Marine Bioregions: A Comparison of Quantitative Approaches. Methods Ecol. Evol. 11, 1258–1272. doi: 10.1111/2041-210X.13447
Horton T., Marsh L., Bett B. J., Gates A. R., Jones D. O. B., Benoist N. M. A., et al. (2021). Recommendations for the Standardisation of Open Taxonomic Nomenclature for Image-Based Identifications. Front. Mar. Sci. 8. doi: 10.3389/fmars.2021.620702
Howell K. L., Davies J. S., Allcock A. L., Braga-Henriques A., Buhl-Mortensen P., Carreiro-Silva M., et al. (2019). A Framework for the Development of a Global Standardised Marine Taxon Reference Image Database (SMarTaR-ID) to Support Image-Based Analyses. PloS One 14, e0218904. doi: 10.1371/journal.pone.0218904
Howell K. L., Holt R., Endrino I. P., Stewart H. (2011). When the Species Is Also a Habitat: Comparing the Predictively Modelled Distributions of Lophelia Pertusa and the Reef Habitat it Forms. Biol. Conserv. 144, 2656–2665. doi: 10.1016/j.biocon.2011.07.025
Howell K.-L., Piechaud N., Downie A.-L., Kenny A. (2016). The Distribution of Deep-Sea Sponge Aggregations in the North Atlantic and Implications for Their Effective Spatial Management. Deep. Sea. Res. Part I.: Oceanogr. Res. Pap. 115, 309–320. doi: 10.1016/j.dsr.2016.07.005
Hui F. K. C., Warton D. I., Foster S. D., Dunstan P. K. (2013). To Mix or Not to Mix: Comparing the Predictive Performance of Mixture Models vs. Separate Species Distribution Models. Ecology 94, 1913–1919. doi: 10.1890/12-1322.1
ICES (2019). Working Group on Marine Habitat Mapping (WGMHM). Copenhagen, Denmark: International Council for the Exploration of the Sea. doi: 10.17895/ICES.PUB.5578
Jansen J., Hill N. A., Dunstan P. K., Eléaume M. P., Johnson C. R. (2018). Taxonomic Resolution, Functional Traits, and the Influence of Species Groupings on Mapping Antarctic Seafloor Biodiversity. Front. Ecol. Evol. 6. doi: 10.3389/fevo.2018.00081
Johnson D., Adelaide Ferreira M., Kenchington E. (2018). Climate Change Is Likely to Severely Limit the Effectiveness of Deep-Sea ABMTs in the North Atlantic. Mar. Policy 87, 111–122. doi: 10.1016/j.marpol.2017.09.034
Kenchington E., Lirette C., Murillo F. J., Beazley L., Downie A. L. (2019b). Vulnerable Marine Ecosystems in the NAFO Regulatory Area: Updated Kernel Density Analyses of Vulnerable Marine Ecosystem Indicators (Scientific Council Meeting No. N7030; P. 68). Northw. Atlant. Fish. Org. doi: 10.1016/j.dsr.2018.11.007
Kenchington E., Murillo F. J., Lirette C., Sacau M., Koen-Alonso M., Kenny A., et al. (2014). Kernel Density Surface Modelling as a Means to Identify Significant Concentrations of Vulnerable Marine Ecosystem Indicators. PloS One 9, e109365. doi: 10.1371/journal.pone.0109365
Kenchington E., Power D., Koen-Alonso M. (2013). Associations of Demersal Fish With Sponge Grounds on the Continental Slopes of the Northwest Atlantic. Mar. Ecol. Prog. Ser. 477, 217–230. doi: 10.3354/meps10127
Kenchington E., Wang Z., Lirette C., Murillo F. J., Guijarro J., Yashayaev I., et al. (2019a). Connectivity Modelling of Areas Closed to Protect Vulnerable Marine Ecosystems in the Northwest Atlantic. Deep. Sea. Res. Part I.: Oceanogr. Res. Pap. 143, 85–103. doi: 10.1016/j.dsr.2018.11.007
Knudby A., Kenchington E., Murillo F. J. (2013). Modeling the Distribution of Geodia Sponges and Sponge Grounds in the Northwest Atlantic. PloS One 8, e82306. doi: 10.1371/journal.pone.0082306
Lagasse C. R., Knudby A., Curtis J., Finney J. L., Cox S. P. (2015). Spatial Analyses Reveal Conservation Benefits for Cold-Water Corals and Sponges From Small Changes in a Trawl Fishery Footprint. Mar. Ecol. Prog. Ser. 528, 161–172. doi: 10.3354/meps11271
Lauria V., Garofalo G., Fiorentino F., Massi D., Milisenda G., Piraino S., et al. (2017). Species Distribution Models of Two Critically Endangered Deep-Sea Octocorals Reveal Fishing Impacts on Vulnerable Marine Ecosystems in Central Mediterranean Sea. Sci. Rep. 7 (1), 8049. doi: 10.1038/s41598-017-08386-z
Lauria V., Massi D., Fiorentino F., Milisenda G., Cillari T. (2021). Habitat Suitability Mapping of the Black Coral Leiopathes Glaberrima to Support Conservation of Vulnerable Marine Ecosystems. Sci. Rep. 11 (1), 15661. doi: 10.1038/s41598-021-95256-4
Levin L. A., Bris N. L. (2015). The Deep Ocean Under Climate Change 350, 766–768. doi: 10.1126/science.aad0126
Lockhart S. J., Hocevar J. (2021). Combined Abundance of All Vulnerable Marine Ecosystem Indicator Taxa Inadequate as Sole Determiner of Vulnerability, Antarctic Peninsula. Front. Mar. Sci., 1238. doi: 10.3389/fmars.2021.577761
Long S., Sparrow-Scinocca B., Blicher M. E., Hammeken Arboe N., Fuhrmann M., Kemp K. M., et al. (2020). Identification of a Soft Coral Garden Candidate Vulnerable Marine Ecosystem (VME) Using Video Imagery, Davis Strait, West Greenland. Front. Mar. Sci. 7. doi: 10.3389/fmars.2020.00460
Maynou F., Cartes J. E. (2012). Effects of Trawling on Fish and Invertebrates From Deep-Sea Coral Facies of Isidella Elongata in the Western Mediterranean. J. Mar. Biol. Assoc. Unite. Kingd. 92 (7), 1501–1507. doi: 10.1017/S0025315411001603
Morato T., González-Irusta J.-M., Dominguez-Carrió C., Wei C.-L., Davies A., Sweetman A. K., et al. (2020). Climate-Induced Changes in the Suitable Habitat of Cold-Water Corals and Commercially Important Deep-Sea Fishes in the North Atlantic. Glob. Change Biol. 26, 2181–2202. doi: 10.1111/gcb.14996
Morato T., Pham C. K., Pinto C., Golding N., Ardron J. A., Durán Muñoz P., et al. (2018). A Multi Criteria Assessment Method for Identifying Vulnerable Marine Ecosystems in the North-East Atlantic. Front. Mar. Sci. 5. doi: 10.3389/fmars.2018.00460
Norse E. A., Brooke S., Cheung W. W. L., Clark M. R., Ekeland I., Froese R., et al. (2012). Sustainability of Deep-Sea Fisheries. Mar. Policy 36, 307–320. doi: 10.1016/j.marpol.2011.06.008
Parker S. J., Bowden D. A. (2010). Identifying Taxonomic Groups Vulnerable to Bottom Longline Fishing Gear in the Ross Sea Region. CCAMLR Sci. 17, 105–127.
Pecl G. T., Araújo M. B., Bell J. D., Blanchard J., Bonebrake T. C., Chen I.-C., et al. (2017). Biodiversity Redistribution Under Climate Change: Impacts on Ecosystems and Human Well-Being. Science 355 (6332):958–61. doi: 10.1126/science.aai9214
Penney A. J., Guinotte J. M. (2013). Evaluation of New Zealand’s High-Seas Bottom Trawl Closures Using Predictive Habitat Models and Quantitative Risk Assessment. PloS One 8 (12). doi: 10.1371/journal.pone.0082273
Ramirez-Llodra E., Brandt A., Danovaro R., De Mol B., Escobar E., German C. R., et al. (2010). Deep, Diverse and Definitely Different: Unique Attributes of the World’s Largest Ecosystem. Biogeosciences 7, 2851–2899. doi: 10.5194/bg-7-2851-2010
Rengstorf A. M., Grehan A., Yesson C., Brown C. (2012). Towards High-Resolution Habitat Suitability Modeling of Vulnerable Marine Ecosystems in the Deep-Sea: Resolving Terrain Attribute Dependencies. Mar. Geodes. 35 (4), 343–361. doi: 10.1080/01490419.2012.699020
Rogers A. D., Gianni M. (2010). The Implementation of UNGA Resolutions 61/105 and 64/72 in the Management of Deep-Sea Fisheries on the High Seas. Report Prepared for the Deep-Sea Conservation Coalition. International Programme on the State of the Ocean. London, United Kingdom: Food Agric. Organ. Unite. Nation. 97.
Ross L. K., Ross R. E., Stewart H. A., Howell K. L. (2015). The Influence of Data Resolution on Predicted Distribution and Estimates of Extent of Current Protection of Three ‘Listed’ Deep-Sea Habitats. PloS One 10 (10), e0140061. doi: 10.1371/journal.pone.0140061
Ross R. E., Wort E. J. G., Howell K. L. (2019). Combining Distribution and Dispersal Models to Identify a Particularly Vulnerable Marine Ecosystem. Front. Mar. Sci. 6. doi: 10.3389/fmars.2019.00574
Rowden A., Anderson O. (2019). SC7-DW12 New Data Compilation for VME Indicator Taxa and Implications for the Review of VME Habitat Suitability Models in the SPRFMO Area. SPRFMO.
Rowden A. A., Anderson O. F., Georgian S. E., Bowden D. A., Clark M. R., Pallentin A., et al. (2017). High-Resolution Habitat Suitability Models for the Conservation and Management of Vulnerable Marine Ecosystems on the Louisville Seamount Chain, South Pacific Ocean. Front. Mar. Sci. 4. doi: 10.3389/fmars.2017.00335
Rowden A. A., Pearman T. R. R., Bowden D. A., Anderson O. F., Clark M. R. (2020). Determining Coral Density Thresholds for Identifying Structurally Complex Vulnerable Marine Ecosystems in the Deep Sea. Front. Mar. Sci. 7. doi: 10.3389/fmars.2020.00095
Rowden A. A., Stephenson F., Clark M. R., Anderson O. F., Guinotte J. M., Baird S. J., et al. (2019). Examining the Utility of a Decision-Support Tool to Develop Spatial Management Options for the Protection of Vulnerable Marine Ecosystems on the High Seas Around New Zealand. Ocean. Coast. Manage. 170, 1–16. doi: 10.1016/j.ocecoaman.2018.12.033
Salinas-de-León P., Martí-Puig P., Buglass S., Arnés-Urgellés C., Rastoin-Laplane E., Creemers M., et al. (2020). Characterization of Deep-Sea Benthic Invertebrate Megafauna of the Galapagos Islands. Sci. Rep. 10, 13894. doi: 10.1038/s41598-020-70744-1
Stone R. P. (2006). Coral Habitat in the Aleutian Islands of Alaska: Depth Distribution, Fine-Scale Species Associations, and Fisheries Interactions. Coral. Reef. 25 (2), 229–238. doi: 10.1007/s00338-006-0091-z
Sweetman A. K., Thurber A. R., Smith C. R., Levin L. A., Mora C., Wei C.-L., et al. (2017). Major Impacts of Climate Change on Deep-Sea Benthic Ecosystems. Element.: Sci. Anthropo. 5. doi: 10.1525/elementa.203
Thompson A., Sanders J., Tandstad M., Carocci F., Fuller J. (2016). Vulnerable Marine Ecosystems - Processes and Practices in the High Seas (Rome: Food and Agriculture Organization of the United Nations).
Thurber A. R., Sweetman A. K., Narayanaswamy B. E., Jones D. O., Ingels J., Hansman R. L. (2014). Ecosystem Function and Services Provided by the Deep Sea. Biogeosciences 11, 3941–3963. doi: 10.5194/bg-11-3941-2014
Tikhonov G., Opedal Ø.H., Abrego N., Lehikoinen A., de M. M. J., Oksanen J., et al. (2020). Joint Species Distribution Modelling With the R-Package Hmsc. Methods Ecol. Evol. 11, 442–447. doi: 10.1111/2041-210X.13345
Tissot B. N., Yoklavich M. M., Love M. S., York K., Amend M. (2006). Benthic Invertebrates That Form Habitat on Deep Banks Off Southern California, With Special Reference to Deep Sea Coral. Fish. Bull. 104 (2), 167–181. Available From: http://fishbull.noaa.gov/1042/tissot.pdf.
Torriente A., González-Irusta J. M., Aguilar R., Fernández-Salas L. M., Punzón A., Serrano A. (2019). Benthic Habitat Modelling and Mapping as a Conservation Tool for Marine Protected Areas: A Seamount in the Western Mediterranean. Aquat. Conserv. Mar. Freshw. Ecosyst. 29, 732–750. doi: 10.1002/aqc.3075
UNGA (2006). 61/105. Sustainable Fisheries, Including Through the 1995 Agreement for the Implementation of the Provisions of the United Nations Convention on the Law of the Sea of 10 December 1982 Relating to the Conservation and Management of Straddling Fish Stocks and Highly Migratory Fish Stocks, and Related Instruments (New York, NY, USA: UNGA).
Vierod A. D. T., Guinotte J. M., Davies A. J. (2014). Predicting the Distribution of Vulnerable Marine Ecosystems in the Deep Sea Using Presence-Background Models. Deep. Sea. Res. Part II. Top. Stud. Oceanogr. 99, 6–18. doi: 10.1016/j.dsr2.2013.06.010
Watling L., Auster P. J. (2017). Seamounts on the High Seas Should Be Managed as Vulnerable Marine Ecosystems. Front. Mar. Sci. 4. doi: 10.3389/fmars.2017.00014
Weaver P. P. E., Benn A., Arana P. M., Ardron J. A., Bailey D. M., Baker K., et al. (2011). “The Impact of Deep-Sea Fisheries and Implementation of the UNGA Resolutions 61/105 and 64/72,” in Report of an International Scientific Workshop, National Oceanography Centre. (Southampton), 45.
Williams A., Althaus F., Green M., Maguire K., Untiedt C., Mortimer N., et al. (2020). True Size Matters for Conservation: A Robust Method to Determine the Size of Deep-Sea Coral Reefs Shows They Are Typically Small on Seamounts in the Southwest Pacific Ocean. Front. Mar. Sci. 7. doi: 10.3389/fmars.2020.00187
Williams A., Althaus F., Schlacher T. A. (2015). Towed Camera Imagery and Benthic Sled Catches Provide Different Views of Seamount Benthic Diversity. Limnol. Oceanogr. Methods 13, e10007. doi: 10.1002/lom3.10007
Yamakita T., Sudo K., Jintsu-Uchifune Y., Yamamoto H., Shirayama Y. (2017). Identification of Important Marine Areas Using Ecologically or Biologically Significant Areas (EBSAs) Criteria in the East to Southeast Asia Region and Comparison With Existing Registered Areas for the Purpose of Conservation. Mar. Policy 81, 273–284. doi: 10.1016/j.marpol.2017.03.040
Yesson C., Bedford F., Rogers A. D., Taylor M. L. (2017). The Global Distribution of Deep-Water Antipatharia Habitat. Deep. Sea. Res. Part II.: Top. Stud. Oceanogr. 145, 79–86. doi: 10.1016/j.dsr2.2015.12.004
Keywords: vulnerable marine ecosystems, species distribution model, habitat suitability model, marine conservation, environmental impact assessment
Citation: Gros C, Jansen J, Dunstan PK, Welsford DC and Hill NA (2022) Vulnerable, but Still Poorly Known, Marine Ecosystems: How to Make Distribution Models More Relevant and Impactful for Conservation and Management of VMEs? Front. Mar. Sci. 9:870145. doi: 10.3389/fmars.2022.870145
Received: 06 February 2022; Accepted: 12 May 2022;
Published: 27 June 2022.
Edited by:
Lorenzo Angeletti, National Research Council (CNR), ItalyReviewed by:
Fabio Fiorentino, National Research Council (CNR), ItalyMar Sacau Cuadrado, Spanish Institute of Oceanography (IEO), Spain
Copyright © 2022 Gros, Jansen, Dunstan, Welsford and Hill. This is an open-access article distributed under the terms of the Creative Commons Attribution License (CC BY). The use, distribution or reproduction in other forums is permitted, provided the original author(s) and the copyright owner(s) are credited and that the original publication in this journal is cited, in accordance with accepted academic practice. No use, distribution or reproduction is permitted which does not comply with these terms.
*Correspondence: Charley Gros, Y2hhcmxleS5ncm9zQHV0YXMuZWR1LmF1