- 1Ocean Discovery League, Saunderstown, RI, United States
- 2MIT Media Lab, Cambridge, MA, United States
- 3Continental Scientific Drilling Facility, University of Minnesota, Minneapolis, MN, United States
- 4SpeSeas, D’Abadie, Trinidad and Tobago
- 5Marine Science Institute, University of California Santa Barbara, Santa Barbara, CA, United States
- 6Sharks Pacific, Avarua, Cook Islands
- 7Australian Research Council Centre of Excellence for Coral Reef Studies, James Cook University, Townsville, QLD, Australia
- 8Department of Biology, Boston University, Boston, MA, United States
- 9Voyaging, Polynesian Voyaging Society, Honolulu, HI, United States
- 10Oceanswell, Colombo, Sri Lanka
- 11Oceans Institute, University of Western Australia, Crawley, WA, Australia
- 12Nekton Foundation, Oxford, United Kingdom
- 13Talma Consultancy, Mahe, Seychelles
- 14Charles Darwin Research Station, Charles Darwin Foundation, Puerto Ayora, Ecuador
- 15Department of Geography, University of British Columbia, Vancouver, BC, Canada
- 16Fish ‘N Fins Inc. Clubhouse, Little Bay, Montserrat
- 17Department of Zoology, Nelson Mandela University, Port Elizabeth, South Africa
- 18Department of Environment, Forestry, and Fisheries, Pretoria, South Africa
- 19Education Department, Bermuda Institute of Ocean Sciences, Saint George, Bermuda
- 20Diversity in Aquatics, Alexandria, VA, United States
- 21Expedition Department, Lindblad Expeditions, Seattle, WA, United States
- 22CoLAB +ATLANTIC, Cascais, Portugal
- 23Organismic and Evolutionary Biology, Harvard University, Cambridge, MA, United States
- 24Laboratório de Sistemas e Tecnologias Subaquáticas, University of Porto, Porto, Portugal
- 25Kamehameha Schools, Honolulu, HI, United States
- 26School of Science, Engineering And Environment, University of Salford, Manchester, United Kingdom
- 27Marine Environmental Program, Bermuda Institute of Ocean Sciences, Saint George, Bermuda
- 28Louisiana Universities Marine Consortium, Chauvin, LA, United States
- 29Biology Department, University of Louisiana at Lafayette, Lafayette, LA, United States
A minuscule fraction of the deep sea has been scientifically explored and characterized due to several constraints, including expense, inefficiency, exclusion, and the resulting inequitable access to tools and resources around the world. To meet the demand for understanding the largest biosphere on our planet, we must accelerate the pace and broaden the scope of exploration by adding low-cost, scalable tools to the traditional suite of research assets. Exploration strategies should increasingly employ collaborative, inclusive, and innovative research methods to promote inclusion, accessibility, and equity to ocean discovery globally. Here, we present an important step toward this new paradigm: a collaborative design study on technical capacity needs for equitable deep-sea exploration. The study focuses on opportunities and challenges related to low-cost, scalable tools for deep-sea data collection and artificial intelligence-driven data analysis. It was conducted in partnership with twenty marine professionals worldwide, covering a broad representation of geography, demographics, and domain knowledge within the ocean space. The results of the study include a set of technical requirements for low-cost deep-sea imaging and sensing systems and automated image and data analysis systems. As a result of the study, a camera system called Maka Niu was prototyped and is being field-tested by thirteen interviewees and an online AI-driven video analysis platform is in development. We also identified six categories of open design and implementation questions highlighting participant concerns and potential trade-offs that have not yet been addressed within the scope of the current projects but are identified as important considerations for future work. Finally, we offer recommendations for collaborative design projects related to the deep sea and outline our future work in this space.
1 Introduction
The deep seafloor (>200 m) represents 92.6% of the global seabed (Figure 1; Eakins & Sharman, 2012) but only a tiny fraction of this percentage has been scientifically explored and characterized1,2. Yet, the deep sea provides regulating, provisioning, and cultural services, including many that support life on our planet, such as the cycling of ocean water and nutrients and the regulation of the Earth’s climate by acting as a carbon and heat sink (Thurber et al., 2014; Le et al., 2017). The deep sea is also a growing source of living and non-living resources, including fisheries, conventional and non-conventional energy resources, and genetic resources (Ramirez-Llodra et al., 2010; Ramirez-Llodra et al., 2011; Armstrong et al., 2012; Jouffray et al., 2020). In addition, it has the potential to be a source of minerals, although there are significant questions about the sustainability and responsibility of deep-sea mining (Rogers et al., 2014; Levin et al., 2020; Amon et al., 2022b; Amon et al., 2022c). While it is clear that deep-sea exploration is vital to our understanding of planetary biodiversity and function and how to mitigate impacts on them, studying these remote environments has thus far been limited by insufficient technological development, inequitable global access to available resources, and the concentration of expertise in only a few regions.
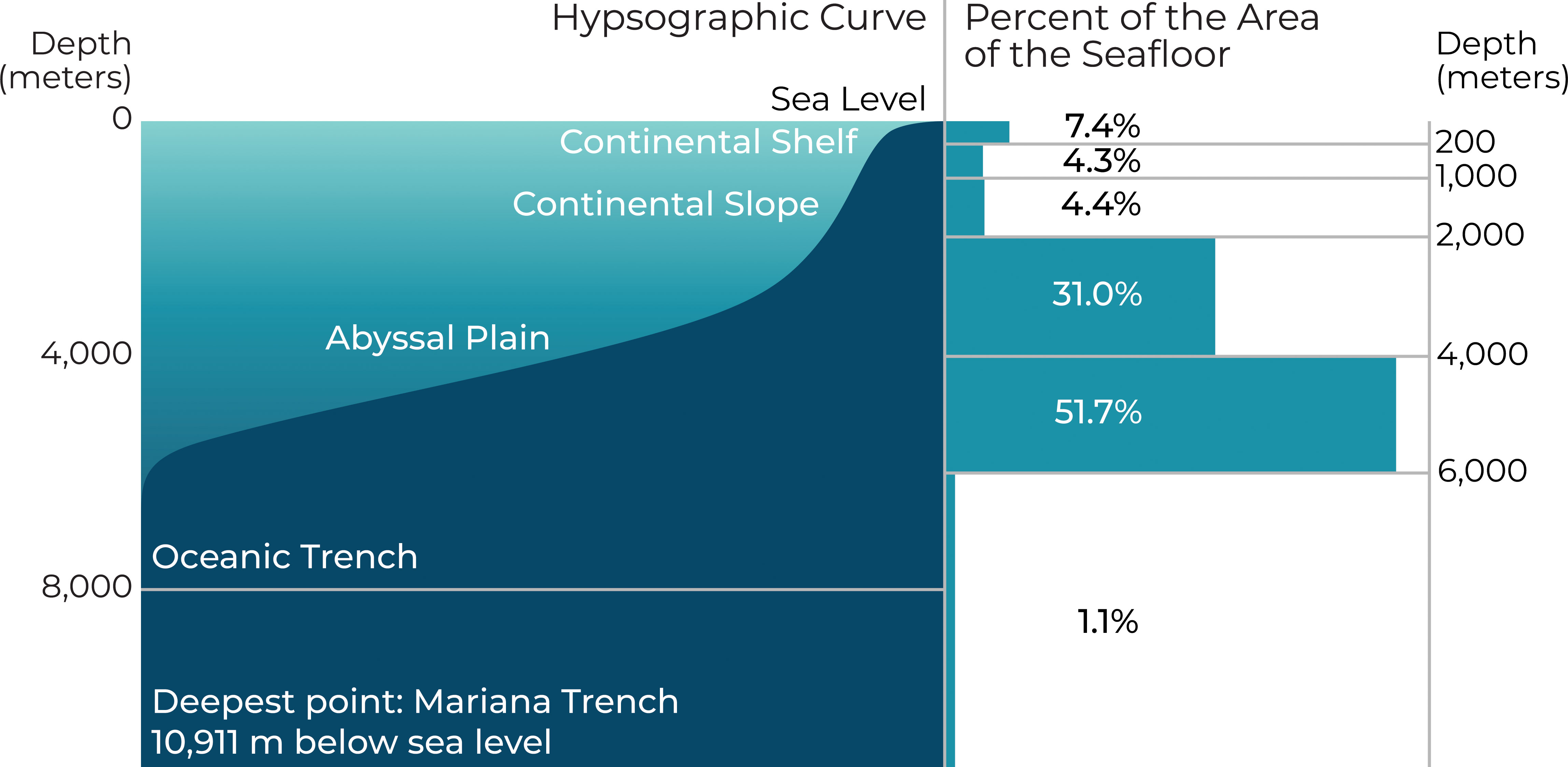
Figure 1 The deep seafloor greater than 200 meters below sea level (mbsl) covers 92.6% of the seabed, 82.7% of which lies between 2,000 to 6,000 mbsl (Eakins & Sharman, 2012).
A critical component of characterizing and understanding the deep seafloor is imaging (Katija et al., 2021), a non-invasive method for observing habitats, identifying organisms, and understanding interactions between organisms and their environment (Huvenne, 2022). Imaging also provides a way to connect humans with remote and inaccessible environments which are therefore “out of sight, out of mind” to most people (Fundis & Bell, 2014; Katija et al., 2021; Genda et al., 2022). Despite the importance of imaging for understanding the deep sea, the tools necessary to undertake this research, as well as the collection of basic parameters such as salinity, temperature, and depth (CTD) to understand environmental conditions, are not available to many researchers around the world (IOC-UNESCO, 2020; Amon et al., 2022d; Bell et al., in prep). For example, preliminary analysis of data from the 2022 Global Deep-Sea Capacity Assessment shows that 19-48% of survey respondents for Africa, Oceania, Latin America, and the Caribbean have access to imaging tools and CTDs, while 48-90% of respondents for Asia, Europe, and Northern America have access to the same tools (Bell et al., in prep). Similar trends are exhibited in access to deployment methods, such as ROVs, AUVs, benthic landers, drifters, towsleds, and HOVs, showing large disparities in access to these deep submergence systems between different regions of the world (Bell et al., in prep).
Our limited understanding of the deep sea is primarily a consequence of not prioritizing the development of affordable, efficient, and equitable approaches to deep-sea exploration and characterization. Many sensors, vessels, and deployment systems can cost tens of thousands to millions of dollars to develop, purchase, and/or operate. Because of their high expense and low availability, the technologies that exist today are more accessible to scientists in wealthy nations, biasing regions explored and motivations for exploration, and creating massive knowledge gaps (IOC-UNESCO, 2020; Amon et al., 2022d; Bell et al., in prep). These tools are still relatively slow at exploring and characterizing the deep sea, especially given the urgent need for robust science to inform management decisions related to increasing exploitation pressures. Furthermore, existing data lack sufficient standardization, formatting, aggregation, and access (Brett et al., 2020; Katija et al., 2021), rendering global synthesis and understanding extremely difficult, if not impossible. Finally, even within nations with the tools necessary to conduct deep-sea exploration and characterization, the field has historically been overwhelmingly white and male (Orcutt & Cetinić, 2014; NSF, 2018; Bell, 2019), potentially resulting in biases and gaps due to homogeneity and/or homophily.Today, humankind is sitting at an inflection point. New technologies, research methods, and communities of people have the potential to transform what it means to explore and characterize the ocean in the 21st century. It is now possible and necessary to accelerate the pace and broaden the scope of exploration by adding low-cost, scalable tools for data collection and AI-driven methods for data analysis to the traditional suite of research assets. Exploration strategies should increasingly employ collaborative research methods to promote inclusion, accessibility, and equity to ocean discovery globally.Here, we present one step toward this new paradigm: a participatory and collaborative design study on technical capacity needs for deep-sea exploration and characterization. This work was conducted in partnership with twenty marine professionals from around the world representing very different domains, including educators, divers, navigators, scientists, engineers, indigenous peoples, and conservation practitioners. The study focused on opportunities and challenges related to deep-sea exploration and research in developed and developing areas worldwide.These findings informed our development of a low-cost, deep-sea imaging and sensing system called Maka Niu and a forthcoming artificial intelligence (AI) video analysis tool. We report on the broader collaborative design process and our early steps towards technology prototypes that are currently being tested in nine countries. We close with lessons learned and recommendations for future participatory and collaborative design work in ocean exploration and characterization and outline plans for the Maka Niu and automated video analysis development process. We hope, by presenting our process and learnings, to (1) inform the research and design agendas of others working toward advancing equity in deep-sea exploration; (2) provide insight on the design needs for low-cost sensing and imaging systems and AI-driven image and data analysis; (3) encourage others to utilize collaborative design methods to build low-cost, accessible tools that enable their fields to become more inclusive and equitable; and, (4) increase familiarity and exposure to the deep sea for local communities to encourage literacy, advocacy, sustainable economic opportunities, and effective stewardship.
2 Background
In this section, we explain the history and emerging role of participatory design (PD), the current status of low-cost, deep-sea technology development, and share the process that facilitated this collaborative research.
2.1 Participatory and Collaborative Design Approaches
The field of Participatory Design (PD) emerged alongside workplace democracy movements led by Scandinavian trade unions in the 1970s. As workplaces modernized with new technologies, early practitioners of PD argued that workers ought to have a say in the design and management of their changing working conditions (Simonsen & Robertson, 2013). PD (also called co-operative design or co-design) argues for direct participation by stakeholders in design activities—from setting the initial terms for collaboration to scoping and framing design challenges to making decisions about proposed solutions.
Over the past fifty years, technology designers have begun to embrace and apply participatory and collaborative approaches around the world to contexts well beyond the workplace (Simonsen & Robertson, 2013; Vines et al., 2013; Emilson et al., 2014; Bannon et al., 2018). Researchers in the field of Information and Computing Technology for Development commonly leverage participatory and collaborative approaches to design digital technologies that can lead to socio-economic development for marginalized communities in low- and middle-income countries (Kendall & Dearden, 2020). The growth of participatory methods in this field responds to a history of failed initiatives, including power dynamics in development projects whereby control of funding and decision-making rests in the hands of those in wealthier, so-called “developed” regions and not those with lived experience of problems to be solved (Brown & Mickelson, 2019). As Irani et al. (2010) argue, many “well-intentioned efforts to ‘migrate’ technologies from industrialized contexts to other parts of the world have foundered either on infrastructural differences or on social, cultural, political, or economic assumptions that do not hold.”Many technology development efforts seek “universal” design solutions that are uniform and scalable across cultures and contexts, often with the laudable goal of making interfaces accessible to all people no matter their ability level (Shneiderman & Plaisant, 2009). However, Bardzell (2010) suggests that a universal approach to design can also “quietly and usually unintentionally impose—without transparent or rational justification—Western technological norms and practices.”In contrast with universalist approaches that flatten difference and encourage conformity, Bardzell suggests using pluralist design approaches. Unlike universalist design approaches, pluralist approaches to design “foreground questions of cultural difference, encourage a constructive engagement with diversity, and embrace the margins both to be more inclusive and to benefit from the marginal as resources for design solutions” (Bardzell, 2010). In other words, pluralist approaches are not intended to be “one size fits all,” and are thus more likely to produce culturally-relevant and sustainable solutions.Participatory design approaches embrace the philosophy of pluralism and are offered as a way forward for intercultural collaborations with diverse stakeholders. Such approaches involve building relationships of trust and mutual benefit, respecting and building on local knowledge, and challenging power dynamics often present in top-down collaborations where groups with more funding and power direct priorities, often at the expense of local partners.In ocean science, participatory approaches have also been applied to the practice of natural resource management. Co-management—an approach where governments and stakeholders work together to manage natural resources by incorporating local knowledge of resources and different stakeholder priorities—was proposed in response to “increasing criticism of the traditional model of top-down management as a method of governance” (Smith, 2012). According to Smith, co-management requires stakeholders (e.g., scientists, researchers, industry representatives, conservation organizations, community members, and more) to be involved in “making decisions about the resources in question in some capacity, and thus involves significant sustained participation.”Community-driven capacity development work aligns with a new global effort—the UN Decade of Ocean Science for Sustainable Development, which launched in 2021 (United Nations, 2018; IOC-UNESCO, 2021). The Ocean Decade aims to transform ocean science by providing a collaborative framework that can account for different disciplines, sectors, and stakeholder communities. This framework supports the co-creation of knowledge about science and capacity needs. In addition to defining globally-set objectives and priorities among research and development areas, a series of regional consultation workshops helped establish Ocean Decade’s strategies based on locally- and regionally-defined objectives, priorities, and needs promoting the use of bottom-up processes from its conception (IOC-UNESCO, 2021).
2.2 Toward Low-Cost in the Deep Sea
Until recently, the most sophisticated and reliable equipment for deep-sea environments have been ROVs, AUVs, and HOVs that cost ~$100k-10M USD to purchase, develop, and/or operate from comparably expensive vessels (Kohnen, 2013; Teague et al., 2018). Increasingly, emerging technologies for ocean exploration and research cost ~$10k-100k USD and are more portable, easier to operate, and offer a variety of capabilities, accuracy levels, and robustness (Sheehan et al., 2016; Dominguez-Carrió et al., 2021; Giddens et al., 2021). For the past few years, “do-it-yourself” and open-sourced shallower tools (<300 m) have been developed using microcontrollers, single-board computers, and commercially available components to create camera and/or sensor systems within ~$100-$1000 USD (Simoncelli et al., 2019; Greene et al., 2020; Lertvilai, 2020; Mouy et al., 2020; Bilodeau et al., 2022; Butler and Pagniello, 2022). Two low-cost camera systems are designed for depths of 5,500-6,000 m (Phillips et al., 2019; Purser et al., 2020), and commercially available cameras such as GoPros can be after-market housed to ~3,000 m; none of these options, however, include sensors such as depth or temperature, which are critical for scientific understanding of the environment.
There is room for innovation in this space, and the collaborative design approach introduced in this paper is an example of how to build upon this movement. By aligning the earliest development stages of ocean technology to the requirements of a diversity of users–for example, the intersection of imaging, sensing, affordability, and ease of use we–can establish a collaborative process and design community to create a new system that meets the community’s needs.
2.3 Our Approach
The Open Ocean Initiative incubated the work presented here at the MIT Media Lab in collaboration with individuals and organizations around the world, several of whom are interviewees, test users, and co-authors of this research. Co-development and co-production of knowledge were essential to this study, allowing us to surface interconnected challenges related to ocean exploration across various domains, emphasized as an important approach in the recent work of Woodall et al. (2021). Several other recent publications about capacity building also call for more knowledge sharing in deep-ocean science (Markus et al., 2018; Miloslavich et al., 2018; Howell et al., 2020b).
In 2018, Open Ocean facilitated and participated in the launch of two pilot projects: My Deep Sea, My Backyard, which aimed to grow deep-sea capacity in two Small Island Developing States (SIDS) (Amon et al., 2022d), and FathomNet, an open-source image database that AI algorithms can use to help us understand our ocean and its inhabitants (Katija et al., 2021). Nascent at the time, both efforts have since become critical components of making deep-sea exploration and research less expensive, more efficient, and more equitable (Márquez, 2018). Parallel to these initiatives, a network of researchers and stakeholders was coalescing, building a community of research and practice via two events held at the MIT Media Lab: Here Be Dragons (Bell et al., 2021) and the 2018 National Ocean Exploration Forum: All Hands on Deck (Bell et al., 2019; Bell et al., 2020a). These events and projects provide context for building diverse communities from which collaborative, transdisciplinary research and design projects have emerged organically, including this participatory design study to further Maka Niu and AI tool development. In 2021, Open Ocean spun out of MIT as the non-profit Ocean Discovery League (ODL), aiming to accelerate deep-sea exploration by developing accessible systems to broaden the community of those who explore and understand the deep sea.
2.3.1 Maka Niu
Maka Niu, loosely translated as “coconut eye” in Hawaiian, was conceived in February 2020 as an educational tool in collaboration between Open Ocean/ODL, the Polynesian Voyaging Society (PVS), and the MIT Future Ocean Lab (now Oceanic Labs). Maka Niu was envisioned to be a tool that could go deep in the water column to illuminate what is underneath the waʻa (canoe), allowing the community to see and safeguard what extends beyond the loko i’a (fishponds) of the ahupua’a (watershed). Due to COVID-19, the design process took longer than anticipated. However, the delay allowed the Design Research and Engineering Teams to incorporate learnings from the summer 2020 interviews into the design and implementation of the camera systems. While initially conceived as a system for educational use, a broader range of marine users and applications became apparent throughout the interview and engineering design process. Today, Maka Niu is ‘a low-cost, modular imaging and sensor platform that leverages off-the-shelf commodity hardware along with the efficiencies of mass production to decrease the price per unit and allow more global communities to explore previously unseen regions of the ocean’ (Novy, Kawasumi et al., in prep).
2.3.2 Automated Artificial Intelligence Video Analysis Tools
Our automated ocean video analysis product strategy builds on four years of work on FathomNet, an open-source, expertly annotated database of underwater imagery (Katija et al., 2021). A new effort will take this work further by creating an easy-to-use platform that enables users to analyze their video data with AI algorithms without prior computer programming experience.
The platform aims to create an accessible online tool for holistically analyzing deep-sea video and environmental data using machine learning. Algorithms will rapidly analyze visual ocean data, observations, and associated environmental metadata to automatically localize and classify marine species and features. By dramatically accelerating the ability to analyze ocean video and creating a collaborative environment for open data sharing of discoveries, we will dramatically expand our understanding of global ocean biodiversity and habitats.
3 Methods
Since July 2020, the Design Research Team has conducted a collaborative design study with our growing global network of colleagues. Our overarching goals are to (1) collect feedback on feature and capability requirements from potential users of the new technologies before and while they were developed; and (2) assemble interested users from the study to test prototypes of the tools created in an interactive, collaborative way.
3.1 Interview Goals and Participants
We used qualitative methods to conduct the interview phase of the collaborative design study from 27 July to 7 August 2020. Interview invitations were extended to project collaborators and network colleagues. A total of twenty people were interviewed during nineteen semi-structured virtual sessions; two participants were interviewed in the same session. Nineteen of the twenty interviewees are co-authors of this manuscript. The purpose of the interviews was to seek feedback on Maka Niu and AI analysis tools. Interview input was reviewed, coded, and analyzed by the interview team. Its synthesis was reviewed and edited by the interview participants in a shared online document such that our findings were collaboratively established. This follows established practices in participatory-collaborative design processes where lead researchers assume the role of facilitators of knowledge production rather than acting as translators between interview subjects and designers (Scariot et al., 2012).
The twenty interview participants were marine professionals representing a broad cross-section of domain expertise such as education, diving, traditional navigation, science, engineering, indigenous knowledge, and conservation. Interviewees were located in ten countries and conducted fieldwork in every ocean basin (Figure 2). One-third of the interviewees live in countries or territories with developing economies3, including 21% who live in SIDS. Of the nineteen interviewees who self-reported their demographic backgrounds, 63% are female, and 37% are male (Figure 3A). At the time of the interviews, 37% were between the ages of 30-39 years old, 58% were 40-49, and 5% were 50-59 (Figure 3B); and 16% had completed a bachelor’s degree, while 37% had a master’s degree, and 47% had a doctoral degree (Figure 3C). In terms of ethnic/racial origin, seven (37%) of the nineteen interviewees identified as White or Caucasian, two (11%) as Black/African-American, and one each as Native Hawaiian/Pacific Islander, Asian, Afro Caribbean/Latina/White, Asian/Native Hawaiian/White, Black Caribbean, Indian Ocean Islander/African, Mixed, and Jewish/White. One each selected ‘A race/ethnicity not listed here’ and ‘Prefer not to answer’ (Figure 3D).
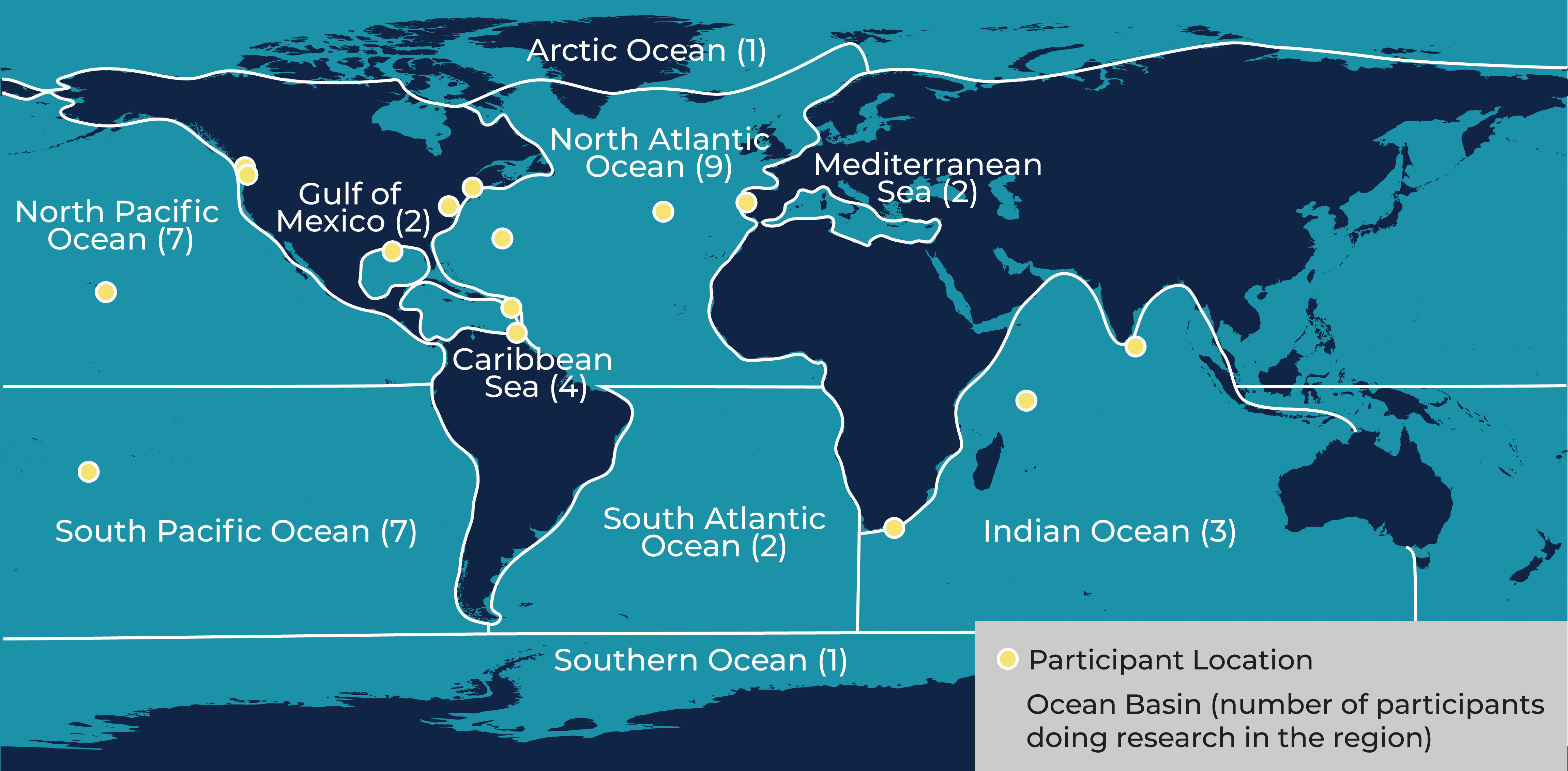
Figure 2 Nineteen out of twenty interviewees self-reported their location of residence (yellow dot) and fieldwork location(s) (ocean basins). Locations of residence include Bermuda (2), Canada, Cook Islands, Montserrat, Portugal (2), Seychelles, South Africa, Sri Lanka, Trinidad and Tobago, and the United States (8). Many participants conduct fieldwork in different ocean basins from their home location.
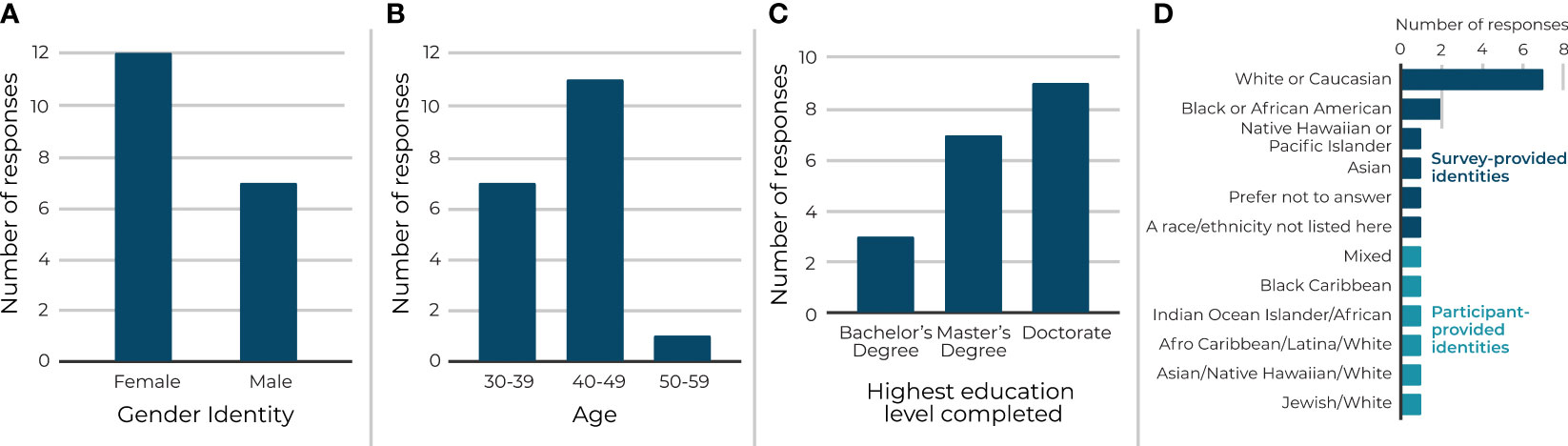
Figure 3 Nineteen out of twenty interviewees self-reported their: (A) gender identity, (B) age, (C) highest level of education completed, and (D) race/ethnicity/origin identity.
3.2 Interview Set Up
Before the interview, interviewers acquainted themselves with the work of each interviewee, and interviewees were provided with background materials on Maka Niu and AI analysis tools and the Open Ocean value-guided design principles (Hope et al., 2019).
3.3 Interview Protocol
All interviews were conducted over two weeks in English via video teleconference calls, recorded with permission from each interviewee. The typical interview duration was one hour. Interviewers worked in pairs, with an interviewer and a rapporteur, both among the authors of this paper. A universal set of eighteen questions were asked of each interviewee (see Supplementary Material), allowing for qualitative data analysis that yielded comprehensive input to the Maka Niu and AI design teams. The interviews covered four main topics: (1) the interviewee’s ocean-related background, interests, and community; (2) low-cost deep-sea imaging tools, with a focus on the capabilities of Maka Niu; (3) AI-driven data analysis, with a focus on capabilities of AI tools; and, (4) the interest and availability for user testing of Maka Niu and/or future AI tools.
Because of its exploratory nature, a semi-structured interview methodology (e.g., Blandford, 2013) was used to conduct this collaborative design study. This approach allowed for emergent data and the identification of broadly-shared challenges in deep-sea exploration and individual mission-critical requirements for each interviewee. Instrumental to developing the interview questionnaire and protocols was having one member of the interview team with experience working in participatory and collaborative design projects in other domains to train and orient the other interview team members.Each interview began with introductions, followed by a brief description of Maka Niu and the AI tools. The interviewer mainly engaged with the interviewee, guided by the questions while adaptively tuning the conversation flow to listen, acknowledge, and interact responsively to the interviewee’s input. The questionnaire was designed to situate the interviewee and their network in the global marine community and determine their interest and needs to explore the deep sea with Maka Niu and automated video analysis tools. At the conclusion of the interview, the interview team provided the interviewees with additional information as requested.
3.4 Data Analysis
Using the Background Materials, the research team created a preliminary a priori codebook (Glaser & Strauss, 1999). The team then followed an open coding process, adding emergent codes to the a priori codebook. At least two researchers independently coded each interview to increase researchers’ exposure to data, prompting new connections and discoveries and supporting team discussion of emergent codes. Emergent codes were discussed as a group. Themes were then generated from codes, and connections were noted between themes. After the themes were generated, the team drafted an initial “Interview Synthesis” document (Bell et al., 2020b) and shared it with all interview participants for their feedback on how their needs and ideas were represented in our dataset. In some cases, the analysis was modified to reflect clarifications provided by interviewees to move toward a collaborative model of developing the study.
4 Results
4.1 Interviewee Archetypes
As part of our data analysis process, we identified different archetypes to represent the interviewees’ professional domains, experiences, motivations, and requirements (Table 1). Archetypes can be a valuable tool to synthesize concerns and identify differences between stakeholder priorities and requirements. We shared these archetypes with the interviewees to solicit their feedback on how well they reflected their motivations and requirements and made modifications as needed. Archetypes are not meant to be conclusive or dogmatic but rather are offered as a starting point for talking about different perspectives and needs.
Eighteen out of twenty interviewees self-identified up to three archetypes they consider to represent themselves (Figure 4). The most frequently represented archetypes were Scientist/Researcher and Formal or Informal Educator (78% each). These were followed by: Policy Maker or Manager (28%), person in Aquatics/Recreation (22%), Engineer (17%), and Traditional Knowledge Holder (17%). Individuals identified as different combinations of these archetypes (Figure 4), resulting in specific motivations and requirements that guided ideation and decision-making processes (Table 1).
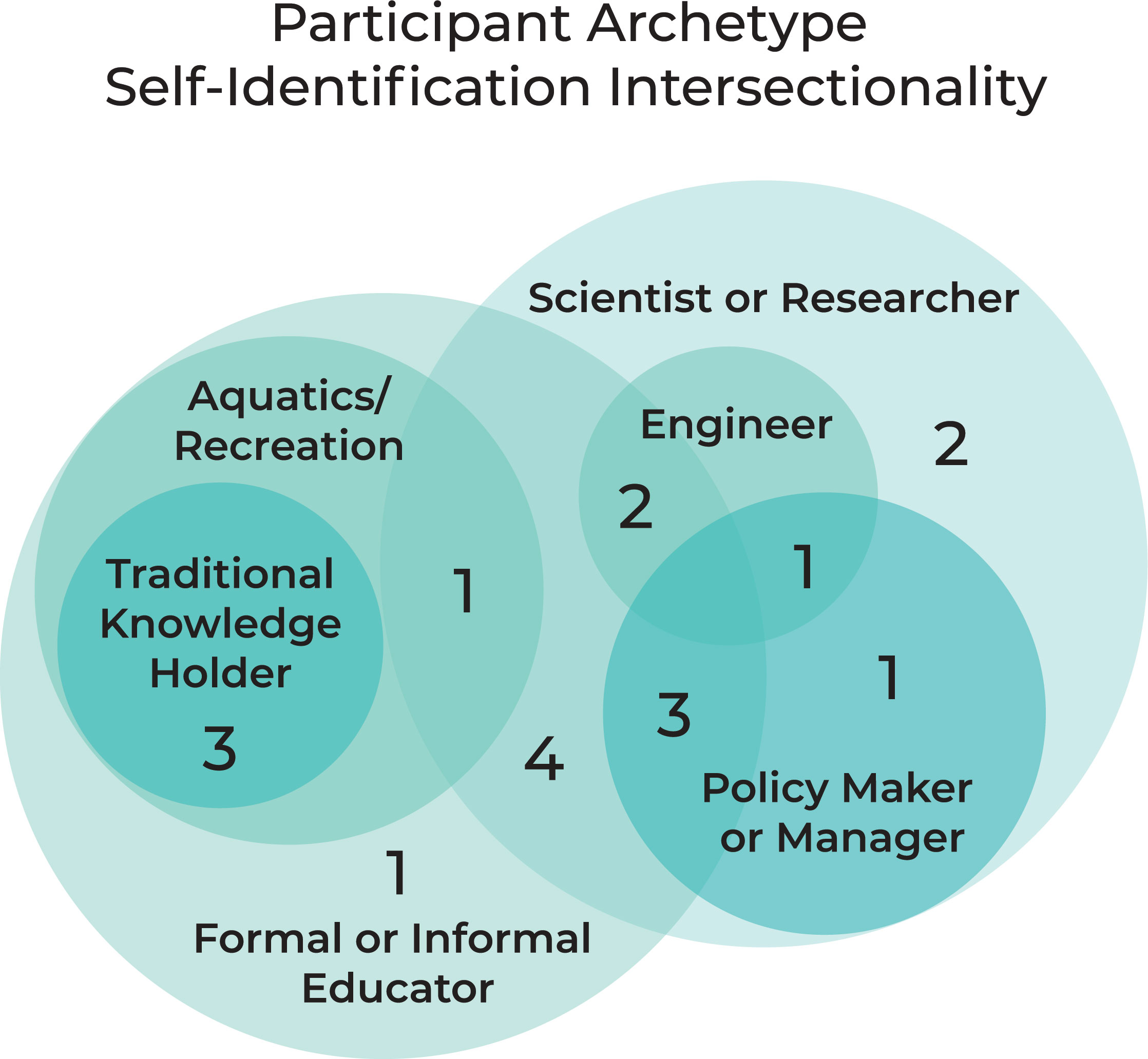
Figure 4 Participant archetype self-identification. Interviewees were asked to identify up to three out of six domains/communities which they represented the most. Eighteen out of twenty interviewees responded.
Furthermore, 42% of the interviewees live and/or work in countries or territories with developing economies, highlighting the need for low-cost, low-logistics tools for deep-sea exploration and research. Motivations of those who live and/or work in these areas include: enabling locally-led science while dissolving “parachute science”; sharing local ocean knowledge with people to encourage them to conserve and protect it; engaging populations not usually engaged in scientific research (e.g., fishers, youth, tourists); and, preparing local people for marine jobs. These motivations resulted in specific requirements for deep-sea tools, including:
•low-cost, easy-to-use, and robust;
•no dependence on big boats or internet access;
•ability to deal with maintenance and repair issues locally;
•and,additional language support for software and training.
While we were not able to incorporate all of these requirements into the first iteration of Maka Niu and AI tool development, we took as many into consideration as possible and aim to include others in future work.
4.2 Community Involvement: How Access to This Technology May Differ by Sector
The ocean community includes a wide range of disciplines, levels of expertise, and stakeholder groups such as researchers, engineers, policymakers, indigenous peoples, NGOs, students, fishers, tourists, and offshore industries. Each of these groups uses the ocean for a variety of reasons, including exploration, exploitation, recreation, and conservation. Interviewees are local experts who belong to different combinations of archetypes and regularly interact with different stakeholders in their regions (Table 2). They were therefore suited to advise how various communities can play a role in ocean exploration, including testing, improvement, and use of Maka Niu and AI analysis tools, once available. In some cases, financial and other support would be required for involvement. The tailoring of technology and skillshare training to each archetype’s research and mission objectives with systems like Maka Niu and AI analysis tools could allow for a more inclusive co-creation of ocean knowledge with different sectors of the community.
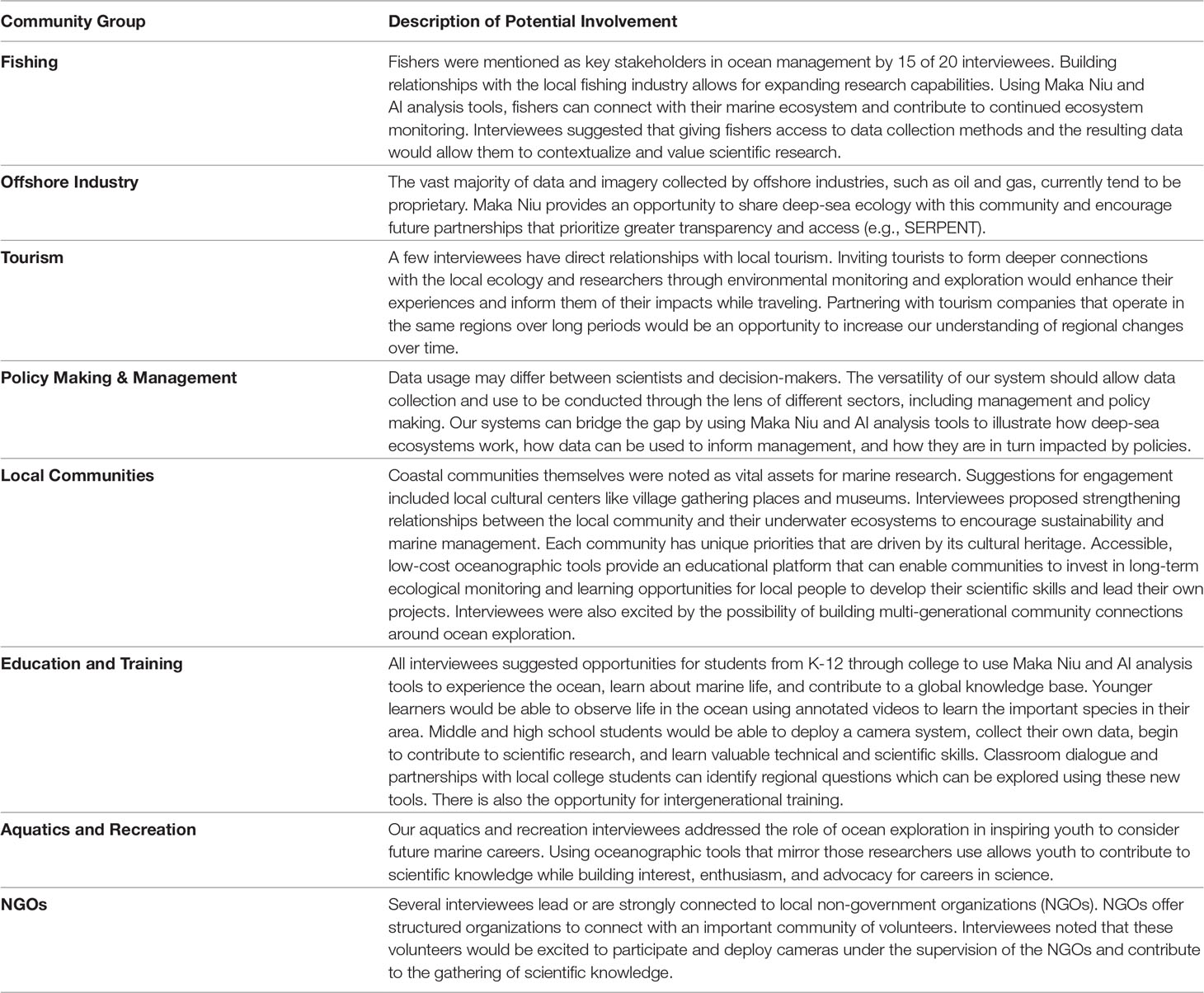
Table 2 Communities and areas of potential involvement in low-cost, deep-sea exploration and research.
4.3 Maka Niu: Data Collection Design Considerations and Implementation
Low-cost imaging and sensing system development is critical for increased efficiency of deep-sea exploration and equitable access to the deep sea. The first technological topic of discussion during the interviews focused on recommendations and requirements for the Maka Niu imaging and sensing system. The interviews pointed to various considerations, including sensor development, features, and capabilities that would ensure the usability of a low-cost system and deployment scenarios to support the interviewees’ work. The priority levels in each section below reflect the relative consensus amongst participants about the need for these capabilities.
4.3.1 Sensor Recommendations
The following are the highest priority deep-sea sensing capabilities that interviewees identified as important for their work:
• 1st Priority: Temperature, Imaging, Depth, Salinity
• 2nd Priority: GPS, Oxygen, pH, Acoustic tags, Light attenuation, eDNA
● Imaging Recommendations: High definition, Stereo, 360°
Various types of water-quality indicators were also noted, but less consistently than the 1st and 2nd Priority measurements. These included chlorophyll, methane, nitrates, phosphates, alkalinity, and turbidity. Additional work could be done to further refine and prioritize sensing capabilities for the deep sea, similar to the Essential Ocean Variables defined by Miloslavich et al. (2018) and Exploration Variables identified by the NOAA Office of Ocean Exploration (Egan et al., 2021).
4.3.2 Feature Requests
In addition to specific sensing capabilities, interviewees also discussed other kinds of features that would make the design of Maka Niu easy to use. These included:
• 1st Priority: Easy to access video/database, depth capability of hundreds to thousands of meters
• 2nd Priority: Long duration (days to months), access to live stream3rd Priority: Easy to use and fix, modular, programmable missions
4.3.3 Deployment Scenarios
The Maka Niu system was initially envisioned as a standalone imaging and sensing system that could deploy from various platforms. We discussed some deployment scenarios, listed in order of capacity and interest, and aggregated them into categories:
• On a deployed benthic structure (e.g., lander, elevator)
• Deployed from a small boat (e.g., kayak, fishing vessel, wa`a)
• On a fixed structure (e.g., buoy, mooring)By people (e.g., SCUBA diver, snorkeler)
• On a tethered system (e.g., ROV, fishing line)
These deployment scenarios are not mutually exclusive; for example, one might deploy a lander from a small boat. Some additional features of a standalone deployable system could include the ability to: A) deploy/retrieve quickly and easily; B) deploy as a drifting system; C) work without the need for an anchor; D) be baited and, E) deployed as an array of units to simultaneously image/sense larger areas of seafloor.
4.3.4 Maka Niu Prototype Imaging & Sensing System
Following the interviews in the summer of 2020, the Maka Niu Engineering Team designed and built a deep-sea imaging and sensing system over six months (Novy, Kawasumi et al., in prep; see Supplementary Material). The Maka Niu Design Research and Engineering Teams considered as many of the design requirements and considerations listed above as possible, particularly the 1st Priority capabilities (Table 3). Several 2nd and 3rd Priority items were also incorporated, including GPS, ease of use, and easily programmable missions. Finally, we experimented with the design and prototyping additional modules with different capabilities, such as a light module, through student design projects at MIT and the University of Porto, Portugal, demonstrating the system’s modularity. Many of the current Maka Niu system components were designed to be extendible and reusable such that additional Maka Niu modules with different capabilities can be driven by its modular parts, allowing users to address their specific research and exploration needs. Additional details on the design, engineering, and modularity of Maka Niu, including student projects, can be found in Novy, Kawasumi et al. (in prep).
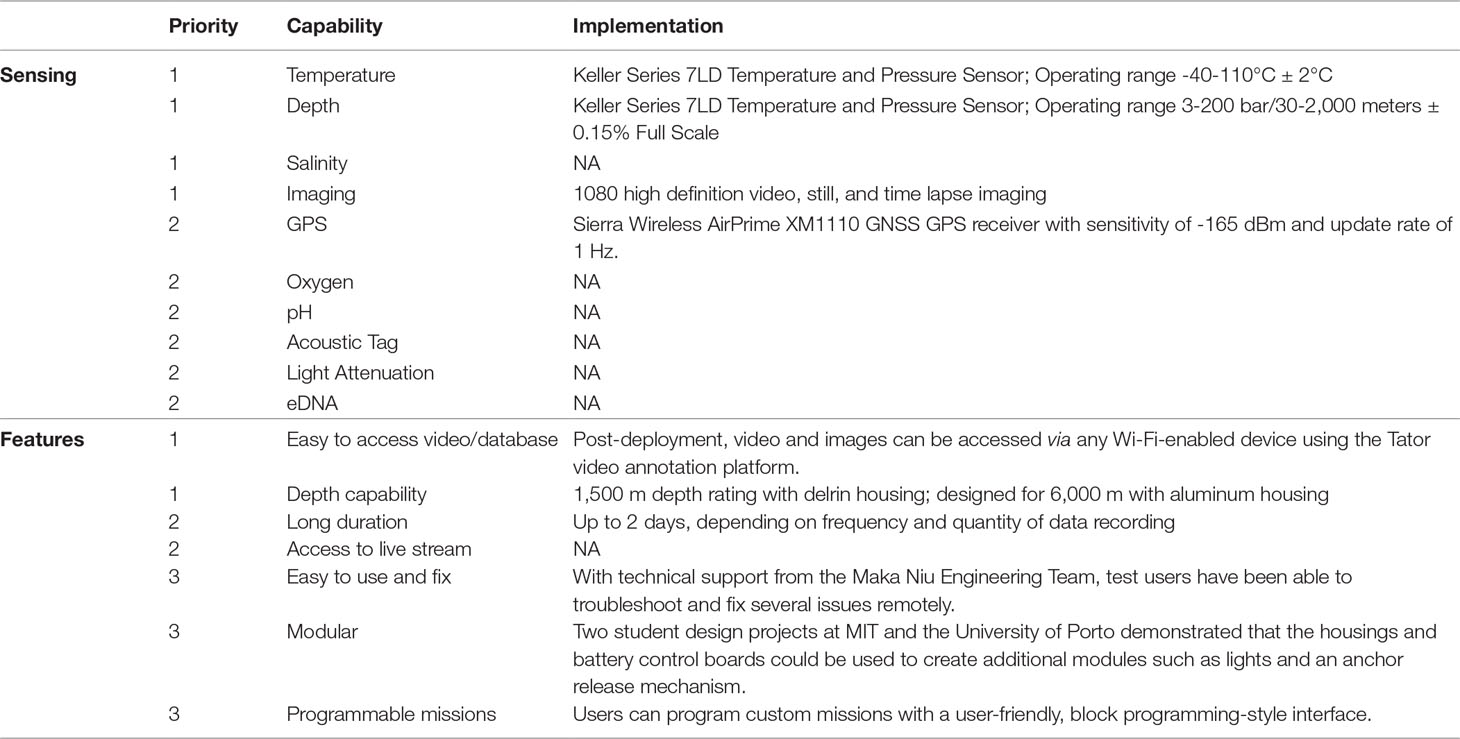
Table 3 Implementation of sensing and feature capabilities for Maka Niu identified during interviews, listed by priority.
Seventeen Maka Niu deep-sea imaging and sensing systems were built in the spring of 2021 (Figure 5). The systems are roughly the size of a large flashlight (Figures 5A, B): they are 261 mm long, 64 mm in diameter (76 mm including the button), and weigh 870 g in air (150 g in water). The Delrin housings are rated to 1,500 m water depth and have been designed to increase the operational depth to 6,000 m with aluminum housings. Maka Niu uses a Raspberry Pi single-board computer for controls with an 8-megapixel Pi Camera Module V2 for still, video, and timelapse image recording. The sensing suite includes temperature, depth, GPS, and 9-axis motion tracking. The control collar enables easy switching between six modes: Off, Wi-Fi, Still Capture, Video Capture, Mission 1 (user-programmed video), and Mission 2 (user-programmed time-lapse). The user can modify missions using a graphical programming interface, allowing easy customization of the mission to their operational needs (Figure 5C). After retrieval, users can access recorded imagery on any Wi-Fi-enabled device (e.g., smartphone, tablet) while in the field, enabling them to verify their data before returning to shore without internet access. Once data capture is complete, the user can download data directly to their device via Wi-Fi or upload it to the online, open-source video annotation web platform, Tator4 (Figure 5D), where they can annotate their images for scientific analysis and/or contribute them to FathomNet5.
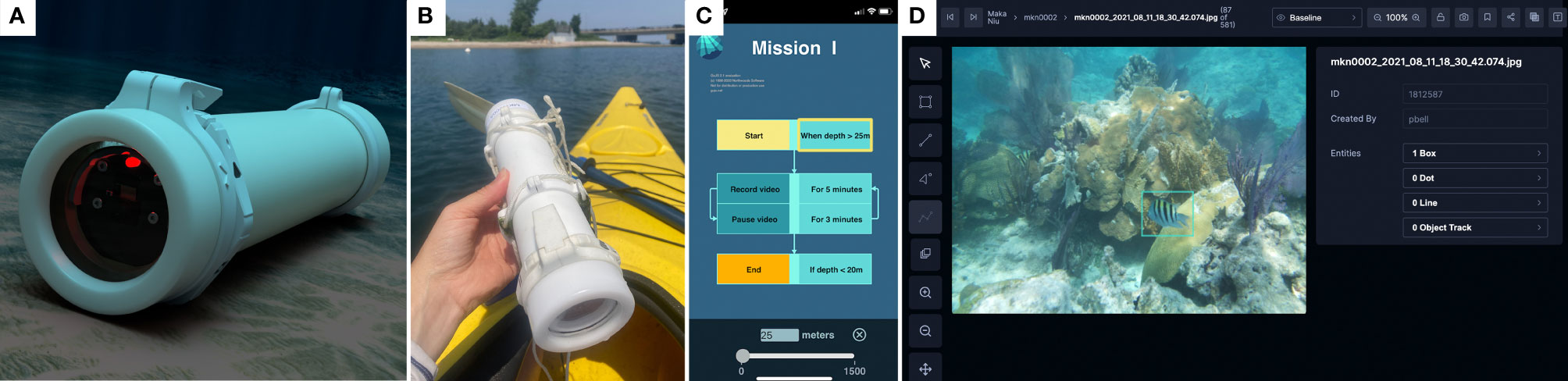
Figure 5 (A, B) Maka Niu is small, lightweight, and easy to deploy. It is roughly the size of a flashlight and weighs 870 g in air (<1 lb). The control collar allows easy switching between modes, and the button triggers actions, for example, starting and stopping video recording. LED flash patterns indicate status information, such as video recording, satellite connection, and battery life. (C) The user can modify missions using a graphical programming interface, allowing the user to easily tailor their mission to their operational needs. (D) In the online, open-source video annotation platform, Tator, users can localize (green box) and identify the organisms and features observed in their video and still images for analysis and optionally submit them to FathomNet. Image (B) by KLC Bell deploying a Maka Niu from a kayak in Narragansett Bay, Rhode Island, USA. Image in (D) by T. & K. Noyes using a test Maka Niu system in Bermuda.
4.3.5 Current and Next Steps
Of the twenty interviewees, thirteen were shipped a Maka Niu system in 2021 to test in eleven locations: Bermuda, Cook Islands, Montserrat, Portugal (2), Seychelles, South Africa, Sri Lanka, Trinidad & Tobago, and the United States (Hawai’i and Louisiana). Systems were provided at no cost to the test user; however, test users assisted with customs fees and logistics in some cases. There were limited systems available; priority was given to interviewees who had time and interest to train and test in winter 2021/2022, had access to seawater, and were geographically distributed worldwide. Members of the Engineering and Design Research Teams also have systems for deployment and testing (see Supplementary Material for sample video). Here we highlight key aspects of the testing and iterative technology development phase.
First, the test users were provided with a brief description of our testing goals and an online User Manual, written and continually updated by the Maka Niu Engineering Team. Soon after receiving the systems, virtual training sessions were offered to introduce test users to the hardware and software. Throughout the testing phase thus far, we have maintained technical and administrative support to the test users through various online communication tools.
The collaborative components of the technology development process involve soliciting test user feedback, offering quick and individualized technical support, identifying common issues among test users, and reworking the hardware and software to address these issues. The goals of our testing phase include feedback on the user experience; data on the sensor and camera system accuracy; feedback on the camera and sensor system performance at depth and in various environmental situations; establishing a community among the test users for direct technical support with the Maka Niu system and career and personal support while in pursuit of their organizations’ mission. In the future, we intend to report on the test users’ assessment of the Maka Niu system and the strengths and weaknesses of this iterative technology development process.
4.4 Image & Data Analysis Design Considerations
In parallel with the development of accessible data collection systems, we must also consider the volume of data collected and plan for an easy-to-use way to train and enable efficient and automated video and data analysis. The second technical discussion focused on developing an online platform that would use machine learning and AI to quickly and easily analyze imagery and associated environmental data. Interview questions focused on what features would be helpful for users, what kinds of people might need to use it, accuracy requirements, and technical requirements such as connectivity.
4.4.1 Key Feature and Capability Requirements
The most important feature identified for an image and data-analysis platform is utility: ease of use and accessibility. Interviewees emphasized the simplicity of design as a high priority, keeping in mind that this system will be implemented with users from different cultures, educational backgrounds, and age groups.
The data-analysis experience must be designed with different user groups in mind, and the level of data access and user experience should therefore vary. The interface used by classroom students should look and function differently than the interface for marine researchers. The entire toolset must also be developed for both desktop and mobile devices, internet-limited use, and real-time capabilities.Interviewees noted that the value of AI is greatly dependent on accuracy; therefore, higher accuracy identification at coarser taxonomic levels (phylum/class) is of greater importance than lower accuracy identification at finer (genus/species) levels. Compatibility with existing databases and collaboration with other image identification efforts may expedite the development of AI-enabled video analysis products and prevent the creation of yet another data silo. Access, storage, and data flow management were also common concerns among interviewees.Key Features and Requirements include:
• Software must be easy to use; assume no computer programming background.
• Participants desire to combine different data sets (e.g., imaging and environmental sensing).
• Higher accuracy at coarser taxonomic levels is more useful.
•Assume low/no bandwidth situations.
• Consider different user groups, what their experiences are, and what their level of access to data could/should be, including youth, teachers, researchers, and policymakers.
• Governance concerns include data management, access, storage, and ownership.
4.4.2 Current and Next Steps
Maka Niu users can upload their imagery and sensor data wirelessly to the online video annotation platform, Tator. Users can then use Tator to annotate (localize and characterize) their observations directly on the video and still images. The resulting annotations can be used for their own research and/or submitted to FathomNet, an open, online, expertly annotated underwater image database. Each step in this process is optional, and up to the user to decide which are appropriate for their purposes.
The FathomNet database contributes to algorithm development for ocean AI analysis tools. This study’s key features and requirements have informed further user interviews in the design and development process of these products.An online, AI-enabled video analysis prototype is currently in development with numerous partners and organizations, including federal agencies, academic institutions, and non-profit organizations, funded by National Geographic Society/Microsoft AI for Earth (PI: Author KLCB) and NSF Convergence Accelerator Track E (PI: K. Katija, MBARI).
4.5 Open Design Questions and Considerations
Our interviews revealed several design and implementation questions that highlight participant concerns and potential trade-offs. These questions have not yet been addressed, but doing so will be necessary to ensure that these systems have long-term impact where intended. Six major categories of these open design questions include deployment, sensor development, software, data sharing, funding, and storytelling (Table 4).
5 Discussion & Recommendations
The future of scientific deep-sea exploration will require radical and creative solutions to accelerate the pace of discovery. Low-cost tools and smaller, low-logistics technologies for the deep sea are being developed (Hardy et al., 2013; Cazenave et al., 2014; Phillips et al., 2019; Purser et al., 2020; Giddens et al., 2021) and cited as one solution to accelerating deep-sea research and broadening its participation (Amon et al., 2022d; Hand & German, 2018; United Nations, 2018; Bell, 2019; Howell et al., 2020a; Howell et al., 2020b; Pizarro & Pace, 2021). At the same time, the challenge of increasing volumes of underwater video and image data is being addressed with systematic and automated annotation and analysis systems (e.g. Langenkämper et al., 2017; Katija et al., 2021).
Given these technological movements, we sit at an exciting time in oceanographic history—it is now technically possible to lower the cost to these tools and therefore increase access to the deep sea. In doing so, we must also take a participatory and collaborative design approach to ensure that these low-cost data-collection technologies and AI-driven data analysis tools will indeed be transformative and lead to both acceleration of discovery and equitable access to the deep ocean. Below, we share six recommendations for deep-sea collaborative design projects and outline our future work in this space.
5.1 Build Balanced Relationships: Cross-Cultural and Trans-Disciplinary Exchange Is Essential for Conceptualizing and Implementing Innovative, Inclusive Projects
The Maka Niu project was the direct result of long-term engagement and relationship building between Open Ocean/ODL and PVS. Actions that led to this collaboration experience include: (1) investing time in relationship building and cultural exchange; (2) constraining technology development to that which is feasible and mutually beneficial; and (3) respecting each others’ knowledge systems through action, such as conscious effort applied to learning and engaging in multiple perspectives (i.e., knowledge pluralism; Parsons et al., 2016; Bingham et al., 2021). As our organizations’ relationship deepened, our ways of knowing expanded, and the quality of project ideas evolved towards those that better intertwined shared goals and had tangible outcomes. Working across intersecting differences—those of culture, gender, geography, institution, and sector—requires time, trust, and respect built through a commitment to a shared set of values and the principle of mutual learning (Bratteteig, 1997; Lang et al., 2012; Parsons et al., 2016; Bingham et al., 2021; Trisos et al., 2021; Woodall et al., 2021).
5.2 Expect to Pivot Your Priorities: Viewing Expectations as a Complex Path as Opposed to a Firm Resolution Allows for Flexibility and Promotes Equitable Collaboration
The results of the interview study (Section 4) affected research and design timelines, the allocation of funds, and redirected team responsibilities. For example, less technical staff time was spent on developing additional sampling system modules for Maka Niu, while more time was devoted to technical training and support. In addition, the detailed feedback on what criteria an AI video analysis platform must satisfy to be ultimately adopted by the field of ocean exploration resulted in a pause of research and prototyping, then subsequently a reorganization of the approach (Section 4.4.2), which involves new funding and the collaboration of numerous partners and organizations. To arrive at a locally sustainable project, where all partners feel ownership and gain some benefit, early action should be taken to include individuals having a variety of different perspectives and critically reflect on assumptions that underlie your priorities. Open dialogue around individuals’ or groups’ priorities and expectations can facilitate project planning that aptly addresses social equity, feasibility, necessary compromise, accountability, and resilience to unexpected and unanticipated course corrections (Tebes, 2018).
5.3 Consider the Accessibility of Low-Cost Tools : The Low Financial Risk and Ease of Deploying Low-Cost, Low-Logistics Oceanographic Tools Make Them a Powerful Driver for Capacity Development, Technical Training, and Novel Field Deployment Opportunities
Building on the experience of My Deep Sea, My Backyard (Amon et al., 2022d), the priority for Maka Niu was to create a high-fidelity prototype of a deep-sea camera and sensor system, quickly getting the system in its early phase of development into the hands of as many test users as possible for feedback (Novy, Kawasumi, et al., in prep). Unanticipated, the collaborative-design research revealed that the potential impact of such a low-cost system appeared tied to the tools’ dollar value. Several interview participants reported that Maka Niu, with a material cost of <$1000 USD, will be in a low-stakes realm where it is seemingly more approachable, experimental, and versatile. For example, the low-logistics design and low-cost build make it easier for test users to view the Maka Niu prototype as an opportunity to take on new hardware and software skills and deploy it in new conditions and locations, without fear of great financial risk. Participants explicitly stated their intention to try deploying Maka Niu in areas they’ve long been interested in exploring and sampling, but the conditions were not conducive with their existing larger and more expensive equipment.
5.4 Create Opportunities for Capacity Development: Consider the Engineering Design Process and Open Hardware as Opportunities for Capacity Development
To accomplish the Ocean Decade’s challenge of “skills, knowledge, and technology for all” (IOC-UNESCO, 2020; IOC-UNESCO, 2021), the transfer of marine technology—including data collection, analysis, and management tools—and the skills necessary for development, operation, and maintenance of those tools are required. Currently, Maka Niu test users participate in the engineering design cycle—lab and field testing, collecting data, identifying issues, and iterating to improve the system. Through this exposure, test users new to engineering may build valuable hardware and software skills that could translate to the in-country maintenance of these tools, a need identified by multiple interviewees. In addition, our goal is to make these designs open source so that anyone can use them to build–and modify–their own systems. By including partners in the engineering process and making designs and data openly available, we hope that a new model of technology capacity building will emerge, leading to locally-led community of practice that eliminates the dependency on outsider expertise or technical support and development (de Vos, 2020; Stefanoudis et al., 2021; Asase et al., 2022; de Vos, 2022; Harden-Davies et al., 2022; Johnson et al., 2022). These efforts go beyond collaboration and help avoid “parachute science” by responding to the realities on the ground and to what skills people would like to acquire (Genda et al., 2022; Asase et al., 2022).
5.5 Dedicate Sufficient Resources: Ensure Appropriate Time, Funding, and Other Resources Are Allocated to All Steps Above and for the Long-Term, as Needed and Desired by Collaborators
While pressures from research and funding timelines can accelerate the pace of design and development work, we have learned that a slow and thoughtful approach results in increased trust between partners and allows for pivoting in response to what is learned along the way. This shift in mindset also requires funders’ understanding that the co-design process takes time and does not always proceed linearly. Unfortunately, much funding is short-term and project-based versus long-term and visionary, forcing work to be completed within an arbitrary timetable that may not be appropriate, particularly for those projects that require long-term relationship-building. It is also critical to compensate people for their time and expertise in the participatory design process. While some design projects expect people to participate simply because they care about the challenge, not compensating participants is unrealistic about the demands on human time and attention and can lead to exploitation. Finally, time spent on the personal growth and development of researchers and organizations is well spent on cross-cultural collaboration. All organizations should consider taking additional time to educate themselves on issues related to exploitation, marginalization, and colonization, as well as positions of privilege, power, and access (Bennett et al., 2021; Trisos et al., 2021; Amon et al., 2022a).
5.6 Follow Through on Commitments: Focusing on the Ideal, Far-Future Outcomes of Co-Design, Co-Development, and Co-Management Projects Will Demand Accountability Among Partners and Operational Planning for a Long-Term Thriving That Grows and Evolves Under the Leadership of Local Ocean Experts
Common causes of failure in collaborative design projects are the lack of sustainable funding, mismatch in stakeholder priorities and benefits gained from the work, and competing demands of other commitments (IOC-UNESCO, 2021). Relationship building helps create a culture of long-term collaboration. However, establishing a code of conduct or a framework to assess the fairness and sustainability of the project’s time and resource demands on each partner is essential for overcoming challenges to its success. This framework may take the form of measuring impacts and progress toward capacity development and the transparent documentation of success and failures, as well as conscientious monitoring of ongoing funding, staffing time, needs being met, and project milestones (Bennett et al., 2021; Harden-Davies et al., in review). Ultimately, an inclusive plan should be developed for the project to become financially sustainable, and entirely locally run as it evolves per the needs of local ocean heroes and communities (WWF, 2015; de Vos, 2020; de Vos, 2022; WWF, 2020).
6 Conclusion
Historically, deep-sea technologies have been inefficient, expensive, and inequitably distributed around the globe. Deep-sea data are siloed, controlled, unstandardized, and fragmented (Brett et al., 2020). Now, it is not only possible, but critical, to create powerful, low-cost, robust deep-sea sensing systems and share, aggregate, and analyze data on a massive scale. Tremendous changes to the system are not only possible but are on the near horizon.
We are at a critical moment in time. The emergence and expected proliferation of low-cost sensors and systems, combined with the power of cloud computing and AI-driven analysis, could widen the gap between those who have access to the deep sea and those who do not, thus exacerbating the existing inequities in deep-sea exploration and research. Or, if undertaken with an intentional and collaborative design approach, these technological changes could usher in an inclusive and equitable future for deep-sea exploration and research. By building balanced relationships with each other and remaining open and flexible to the perspectives and requirements of others, we can successfully design and deploy new systems–both technological and human–to enable new opportunities for exploration, research, collaboration, and discovery.
We challenge the deep-sea community to address the issues with access to the field of deep-ocean exploration by breaking down the barriers, both technical and human. Only by making the tools and systems significantly more accessible, efficient, and inexpensive will we make meaningful progress toward exploring the deep sea, thereby better understanding this critical biosphere and our impact on it. The data and learnings presented here are our first steps along a long-term path of continued co-development of accessible technologies and holistic capacity development activities dedicated to increasing access to and broadening participation in deep-ocean exploration.
Data Availability Statement
The raw data supporting the conclusions of this article, with the exception of identifying information, will be made available by the authors, without undue reservation.
Ethics Statement
Ethical review and approval was not required for the study on human participants in accordance with the local legislation and institutional requirements. Written informed consent for participation was not required for this study in accordance with the national legislation and the institutional requirements.
Author Contributions
KB, JSC, AH, MQ, KC conceived and designed the study. KB, JSC, AH, MQ, KC, DA, RR, LK, AV, ST, JEC, VW, ZF, KN, ML, SB, AK, JS, CB, NL, BK, CM, TN, PG participated in and executed the study. KB, JSC, AH, MQ, KC, DA, RR, LK drafted the article. AV, ST, JC, VW, ZF, KN, ML, SB, AK, NL, PG critically revised the article. All authors gave approval of the submitted version and agree to be accountable for all aspects of the work.
Funding
The participatory design study was funded by the MIT Media Lab Open Ocean Initiative, MIT Portugal Program, Oceankind, and National Philanthropic Trust. The development of Maka Niu was funded by the MIT Portugal Program, MIT Media Lab Open Ocean Initiative, MIT Future Ocean Lab, and Oceanic Labs. User testing and deployments are supported in part by the National Philanthropic Trust. Funding for RR and BK was provided by the National Geographic Society's grant for My Deep Sea, My Backyard (NGSBU-PFA-2018-03). Support for AV was provided by the Schmidt Foundation. Participation of numerous co-authors was supported by their respective organizations. Seed funding for FathomNet was provided by the National Geographic Society (#518018), National Oceanic and Atmospheric Administration (NA18OAR4170105), and the Monterey Bay Aquarium Research Institute through support from the David and Lucile Packard Foundation. Additional funding for AI-enabled video analysis has been provided by the National Geographic Society (NGS-86951T-21) and the National Science Foundation (OTIC #1812535 & Convergence Accelerator #2137977). The Ocean Discovery League supported open-access publication fees.
Conflict of Interest
Author AK is employed by Lindblad Expeditions.
The remaining authors declare that the research was conducted in the absence of any commercial or financial relationships that could be construed as a potential conflict of interest.
Publisher’s Note
All claims expressed in this article are solely those of the authors and do not necessarily represent those of their affiliated organizations, or those of the publisher, the editors and the reviewers. Any product that may be evaluated in this article, or claim that may be made by its manufacturer, is not guaranteed or endorsed by the publisher.
Acknowledgments
We thank one anonymous interviewee for their participation and thoughtful contributions to this study. We thank the Maka Niu Concept Development Team: Nainoa Thompson, Daniel Novy, Allan Adams, Sonja Swenson Rogers, and Noelani Kamalu; the Maka Niu Engineering Team: Daniel Novy, Lui Kawasumi, Allan Adams, Kat Cantner, Jon Ferguson, Margaret Sullivan, and Peter Bell; and, additional Maka Niu test users who worked with the co-authors: Osei Agyapong, João Andrade, Corie Boolukos, João Costa, Noelani Kamalu, Andreas Ratteray, Caroline Schio. Thanks to the E/V Nautilus and Mesobot teams for deploying a Maka Niu unit on cruise NA131. Thank you to the Ecole Flottant organizers and funders, especially IFREMER, IRD, WIOMSA, and University of Reunion Island, for the successful deployment of the Maka Niu on the towed camera, SCAMPI. A special thank you to Simon Tranvouez and Renaud Quinquis of Genavir for their support, advice, and enthusiasm during deployments of the Maka Niu. We also acknowledge the FathomNet team, particularly Kakani Katija and Benjamin Woodward. Finally, sincere thanks Susan Poulton and Jessica Sandoval, as well as reviewers Ana Hilário and David A Bowden, who provided thoughtful and challenging reviews that greatly improved this manuscript.
Supplementary Material
The Supplementary Material for this article can be found online at: https://www.frontiersin.org/articles/10.3389/fmars.2022.873700/full#supplementary-material
Footnotes
- ^ We use the following definitions from OSTS, 2020: Ocean exploration provides a multidisciplinary first look at an unknown or poorly understood area of the seafloor, sub-bottom, and/or water column and an initial assessment of an area’s physical, chemical, and biological characteristics. Ocean characterization provides comprehensive data and interpretations for a specific area of interest of the seafloor, sub-bottom, and/or water column in direct support of specific research, resource management, policymaking, or applied mission objectives.
- ^ Developing Economies and SIDS are identified by the UN Statistics Division M49 Standard (https://unstats.un.org/unsd/methodology/m49/).
- ^ Developing Economies and SIDS are identified by the UN Statistics Division M49 Standard (https://unstats.un.org/unsd/methodology/m49/).
- ^ Tator Online. https://www.tator.io/
- ^ FathomNet. http://fathomnet.org/
References
Amon D. A., Filander Z., Harris L., Harden-Davies H. (2022a). Safe Working Environments are Key to Improving Inclusion in Open-Ocean, Deep-Ocean, and High-Seas Science. Mar. Policy. 137, 104947. doi: 10.1016/j.marpol.2021.104947
Amon D. J., Gollner S., Morato T., Smith C. R., Chen C., Christensen S., et al. (2022b). Assessment of Scientific Gaps Related to the Effective Environmental Management of Deep-Seabed Mining. Mar. Policy. 138, 105006 doi: 10.1016/j.marpol.2022.105006
Amon D. J., Levin L. A., Metaxas A., Mudd G. M., Smith C. R. (2022c). Heading to the Deep End Without Knowing How to Swim: Do We Need Deep-Seabed Mining? One Earth. 5 (3), 220−223. doi: 10.1016/j.oneear.2022.02.013
Amon D. J., Rotjan R. D., et al. (2022d). My Deep Sea, My Backyard: A Pilot Study to Build Capacity for Global Deep-Ocean Exploration and Research. Philos. Trans. R. Soc. London Ser. B: Biol. Sci. doi: 10.1098/rstb.2021.0121
Armstrong C. W., Foley N. S., Tinch R., van den Hove S. (2012). Services From the Deep: Steps Towards Valuation of Deep Sea Goods and Services. Ecosystem Serv. 2, 2–13. doi: 10.1016/j.ecoser.2012.07.001
Asase A., Mzumara-Gawa T. I., Owino J. O., Peterson A. T., Saupe E. (2022). Replacing “Parachute Science” With “Global Science” in Ecology and Conservation Biology. Conserv. Sci. Practice. 4.5, e517. doi: 10.1111/csp2.517
Bannon L., Bardzell J., Bodker S. (2018). Reimagining Participatory Design. Interactions 26.1, 26–32. doi: 10.1145/3292015
Bardzell S. (2010). “Feminist HCI: Taking Stock and Outlining an Agenda for Design,” in Proceedings of the SIGCHI Conference on Human Factors in Computing Systems, Association for Computing Machinery, New York, NY, USA vol. 2. , 1301–1310.
Bell K. L. C. (2019). “Envisioning the Future of Ocean Exploration,” in Ocean Exploration: Diving to New Depths and Discoveries, Hearings Before the Subcommittee on Environment, Committee on Science, Space, and Technology, 116th Cong 25. Washington, DC Available at: https://science.house.gov/imo/media/doc/Bell%20Testimony.pdf.
Bell K. L. C., Cantner K. A., Chow J. S., Hope A., Quinzin M. (2020b). “Co-Designing for Deep Ocean Imaging and Analysis: Interview Synthesis, Fall 2020,” in Open Ocean Initiative ( MIT Media Lab). Cambridge, MA doi: 10.21428/a680be9a.5afa6279
Bell K. L. C., Chow J. S., Hope A., Novy D. (2021). “Here Be Dragons 2018 Report,” in Open Ocean Initiative ( MIT Media Lab). Cambridge, MA, doi: 10.21428/a680be9a.207c346e
Bell K. L. C., Copeland A., Chow J. S., Wiener C., Hope A., McKinnie D. (2019). “All Hands on Deck: The 2018 National Ocean Exploration Forum,” in New Frontiers in Ocean Exploration: The E/V Nautilus, NOAA Ship Okeanos Explorer, and R/V Falkor 2018 Field Season, vol. 32 . Eds. Raineault N. A., Flanders J., 4–5. doi: 10.5670/oceanog.2019.supplement.01
Bell K. L. C., McKinnie D., Copeland A., Wiener C., Chow J. S., Novy D., et al. (2020a). 2018 National Ocean Exploration Forum: All Hands on Deck (Cambridge, Massachusetts: MIT Media Lab and NOAA).
Bell K. L. C., Quinzin M. A., Sarti O., Canete T., Smith A., Chung T.-R., et al. (in prep). Results of the Global Deep-Sea Capacity Assessment.
Bennett N. J., Katz L., Yadao-Evans W., Ahmadia G. N., Atkinson S., Ban N. C., et al. (2021). Advancing Social Equity in and Through Marine Conservation. . Front. Mar. Sci. 8, 711538 doi: 10.3389/fmars.2021.711538
Bilodeau S. M., Schwarts A. W. H., Xu B., Pauca V. P., Silman M. R. (2022). A Low-Cost, Long-Term Underwater Camera Trap Network Coupled With Deep Residual Learning Image Analysis. PloS One 17.2, e0263377. doi: 10.1371/journal.pone.0263377
Bingham J. A., Milne S., Murray G., Dorward T. (2021). Knowledge Pluralism in First Nations’ Salmon Management. Front. Mar. Sci. vol: 8, pp: 671112 doi: 10.3389/fmars.2021.671112
Blandford A. E. (2013). “Semi-Structured Qualitative Studies,” in The Encyclopedia of Human-Computer Interaction. Eds. Soegaard M., Dam R. (Denmark: Interaction Design Foundation).
Bratteteig T. (1997). Mutual Learning. Enabling cooperation insystems design. Proc. IRIS. 20, 1–20. https://www.academia.edu/1036805/Mutual_learning_Enabling_cooperation_in_systems_design
Brett A., Leape J., Abbott M., Sakaguchi H., Cao L., Chand K., et al. (2020). Ocean Data Need a Sea Change to Help Navigate the Warming World. Nature. 582, 181–183. doi: 10.1038/d41586-020-01668-z
Brown S., Mickelson A. R. (2019). Why Some Well-Planned and Community-Based ICTD Interventions Fail. Inf. Technol. Int. Dev. 15, 13. https://itidjournal.org/index.php/itid/article/view/1706.html
Butler J., Pagniello C. M. L. S. (2022). Emerging, Low-Cost Ocean Observing Technologies to Democratize Access to the Ocean. Oceanography. 34.4, 94–95. doi: 10.5670/oceanog.2021.supplement.02-35
Cazenave F., Kecy C., Risi M., Haddock S. H. D. (2014). SeeStar: A Low-Cost, Modular and Open-Source Camera System for Subsea Observations. In 2014 Oceans - St. John’s, Vol. 1–7. 2014. 2014 Oceans, MTS/IEEE, St. John's, Newfoundland doi: 10.1109/OCEANS.2014.7003077
de Vos A. (2020). The Problem of ‘Colonial Science.’Sci. Am. https://www.scientificamerican.com/article/the-problem-of-colonial-science/
de Vos A. (2022). Stowing Parachutes, Strengthening Science. Conserv. Sci. Practice. 4.5, e12709. doi: 10.1111/csp2.12709
Dominguez-Carrió C., Fontes J., Morato T. (2021). A Cost-Effective Video System for a Rapid Appraisal of Deep-Sea Benthic Habitats: The Azor Drift-Cam. Methods Ecol. Evolution. 12.8, 1379–1388. doi: 10.1111/2041-210X.13617
Eakins B. W., Sharman G. F. (2012). Hypsographic Curve of Earth’s Surface From Etopo1 (Boulder, CO: NOAA National Geophysical Data Center).
Egan K. E., Le J. T., Murphy J. W. A., Netburn A. N., Bohan M., Copeland A., et al. (2021). “Exploration Variables Identified by the NOAA Ocean Exploration,” in NOAA Ocean Exploration (Silver Spring, MD: NOAA Technical Memorandum OAR OER), 136. doi: 10.25923/m37w-8b55
Emilson A., Hillgren P.-A., Seravalli A., Marttila S., Hobye M., Cuartielles D., et al. (2014). Making Futures: Marginal Notes on Innovation, Design, and Democracy ( MIT Press). Cambridge, MA
Fundis A., Bell K. L. C. (2014). Inspiring, Engaging, and Educating the Next Generation of STEM Learners. J. Ocean Technology. 9.3, 73–78. https://www.thejot.net/archive-issues/?id=42
Genda P. A., Ngoteya H. C., Caro T., Mulder M. B. (2022). Looking Up and Down: Strong Collaboration is Only the First Step in Tackling Parachute Science. Conserv. Sci. Practice. 4.5, e12677. doi: 10.1111/csp2.12677
Giddens J., Turchik A., Goodell W., Rodriguez M., Delaney D. (2021). The National Geographic Society Deep-Sea Camera System: A Low-Cost Remote Video Survey Instrument to Advance Biodiversity Observation in the Deep Ocean. Front. Mar. Sci. vol: 7, pp: 601411 doi: 10.3389/fmars.2020.601411
Glaser B. G., Strauss A. L. (1999). Discovery of Grounded Theory: Strategies for Qualitative Research. (1st ed.) Routledge. doi: 10.4324/9780203793206
Greene A., Forsman Z., Toonen R. J., Donahue M. J. (2020). CoralCam: A Flexible, Low-Cost Ecological Monitoring Platform. HardwareX. 7, e00089. doi: 10.1016/j.ohx.2019.e00089
Hand K. P., German C. R. (2018). Exploring Ocean Worlds on Earth and Beyond. Nat. Geosci. volume 11, 2–4. doi: 10.1038/s41561-017-0045-9
Harden-Davies H., Amon D. J., Vierros M., Bax N. J., Hanich Q., Hills J. M. (2022). Capacity Development in the Ocean Decade and Beyond: Key Questions About Meanings, Motivations, Pathways, and Measurements. Earth System Governance. 12, 100138. doi: 10.1016/j.esg.2022.100138
Harden-Davies H., Amon D. J., Vierros M., Bax N., Hanich Q., Hills J. M., et al. In Review. Meaning, Motivation and Measurement: Critical Thinking for Capacity Building in the Ocean Decade and Beyond, Earth System Governance.
Hardy K., Cameron J., Herbst L., Bulman T., Pausch S. (2013). Hadal Landers: The DEEPSEA CHALLENGE Ocean Trench Free Vehicles. In 2013 OCEANS - San Diego 1–10, 2013. doi: 10.23919/OCEANS.2013.6741368
Hope A., Chow J. S., Bell K. L. C. (2019). “North Star Design Principles,” in Open Ocean Initiative, MIT Media Lab. Open Ocean Initiative, MIT Media Lab, Cambridge, MA doi: 10.21428/a680be9a.82c44a5e/0b7b143a
Howell K. L., Hilário A., Allcock A. L., Bailey D., Baker M., Clark M. R., et al. (2020a). A Blueprint for an Inclusive, Global Deep-Sea Ocean Decade Field Program. Front. Mar. Sci. vol: 7, pp: 584861 doi: 10.3389/fmars.2020.584861
Howell K. L., Hilário A., Allcock A. L., Bailey D., Baker M., Clark M. R., et al. (2020b). A Decade to Study Deep-Sea Life. Nat. Ecol. Evolution. 5, 265–267. doi: 10.1038/s41559-020-01352-5
Huvenne V. (2022). “The Big Picture: Using Imagery in Marine Science. Imaging the Ocean: New Technology to Reveal the Secrets of Life in the Deep Sea,” in UN Ocean Decade Laboratory: An Accessible Ocean. Available at: https://www.iatlantic.eu/imaging-the-ocean-event.
IOC-UNESCO (2020). Global Ocean Science Report 2020–Charting Capacity for Ocean Sustainability. Ed. Isensee K. (Paris: UNESCO Publishing).
IOC-UNESCO (2021). Co-Designing the Science We Need for the Ocean We Want: Guidanceand Recommendations for Collaborative Approaches to Designing & Implementing Decade Actions Vol. 29 (Paris: UNESCO. (The Ocean Decade Series).
Irani L., Vertesi J., Dourish P., Philip K., Grinter R. E. (2010). “Postcolonial Computing: A Lens on Design and Development,” in Proceedings of the SIGCHI Conference on Human Factors in Computing Systems, Association for Computing Machinery, New York, NY, USA vol. 2. , 1311–1320. doi: 10.1145/1753326.1753522
Johnson A., Saypanya S., Hansel T., Rao M. (2022). More Than an Academic Exercise: Structuring International Partnerships to Build Research and Professional Capacity for Conservation Impact. Conserv. Sci. Practice. 4.5, e539. doi: 10.1111/csp2.539
Jouffray J.-B., Blasiak R., Norström A. V., Österblom H., Nyström M. (2020). The Blue Acceleration: The Trajectory of Human Expansion Into the Ocean. One Earth 2 (1), 43–54. doi: 10.1016/j.oneear.2019.12.016
Katija K., Orenstein E., Schlining B., Lundsten L., Barnard K., Sainz G., et al. (2021). FathomNet: A Global Underwater Image Training Set for Enabling Artificial Intelligence in the Ocean. arXiv, 2109.14646v2. doi: 10.48550/arXiv.2109.14646
Kendall L., Dearden A. (2020). The Politics of Co-Design in ICT for Sustainable Development. CoDesign 16 (1), 81–95. doi: 10.1080/15710882.2020.1722176
Kohnen W. (2013). Review of Deep Ocean Manned Submersible Activity in 2013. MTS J. 47.5, 56–68. doi: 10.4031/MTSJ.47.5.6
Langenkämper D., Zurowietz M., Schoening T., Nattkemper T. W. (2017). BIIGLE 2.0 - Browsing and Annotating Large Marine Image Collections. Front. Mar. Sci. vol: 4, pp: 83 doi: 10.3389/fmars.2017.00083
Lang D. J., Wiek A., Bergmann M., Stauffacher M., Martens P., Moll P., et al. (2012). Transdisciplinary Research in Sustainability Science: Practice, Principles, and Challenges. Sustain Sci. 7, 25–43. doi: 10.1007/s11625-011-0149-x
Le J. T., Levin L. A., Carson R. T. (2017). Incorporating Ecosystem Services Into Environmental Management of Deep-Seabed Mining. Deep Sea Res. Part II: Topical Stud. Oceanography 137, 486–503. doi: 10.1016/j.dsr2.2016.08.007
Lertvilai P. (2020). The In Situ Plankton Assemblage Explorer (IPAX): An Inexpensive Underwater Imaging System for Zooplankton Study. Methods Ecol. Evolution. 11.9, 1042–1048. doi: 10.1111/2041-210X.13441
Levin L. A., Amon D. J., Lily H. (2020). Challenges to the Sustainability of Deep-Seabed Mining. Nat. Sustainability 3, 784–794. 10.1038/s41893-020-0558-x
Markus T., Hillebrand H., Hornidge A.-K., Krause G., Schlüter A. (2018). Disciplinary Diversity in Marine Sciences: The Urgent Case for an Integration of Research. ICES J. Mar. Sci. 75, 502–509. doi: 10.1093/icesjms/fsx201
Márquez M. C. (2018). “My Deep Sea, My Backyard: Empowering Nations To Study The Deep,” in Forbes. Jersey City, Available at: https://www.forbes.com/sites/melissacristinamarquez/2018/11/21/my-deep-sea-my-backyard-empowering-nations-to-study-the-deep/.
Miloslavich P., Bax N. J., Simmons S. E., Klein E., Appeltans W., Aburto-Oropeza O. (2018). Essential Ocean Variables for Global Sustained Observations of Biodiversity and Ecosystem Changes. Glob Change Biol. 24, 2416–2433. doi: 10.1111/gcb.14108
Mouy X., Black M., Cox K., Qualley J., Mireault C., Dosso S., et al. (2020). FishCam: A Low-Cost Open Source Autonomous Camera for Aquatic Research. HardwareX. 8, e00110. doi: 10.1016/j.ohx.2020.e00110
Novy D., Kawasumi L., Ferguson J., Sullivan M., Bell P., Chow J. S., et al. (in prep). Maka Niu: A Low-Cost, Modular Imaging and Sensor Platform to Increase Observation Capabilities of the Deep Ocean. Front. Mar. Res.
NSF (2018) National Center for Science and Engineering Statistics (NCSES). Doctorate Recipients From U.S. Universities: 2017. Alexandria, VA. NSF 19-301. December 04, 2018. Available at: https://ncses.nsf.gov/pubs/nsf19301/data.
Ocean Science and Technology Subcommittee (OSTS) (2020). “National Strategy for Mapping, Exploring, and Characterizing the United Stated Exclusive Economic Zone,” in Subcommittee of the Ocean Policy Committee. Washington, DC, USA Available at: https://oeab.noaa.gov/wp-content/uploads/2021/01/2020-national-strategy.pdf.
Orcutt B. N., Cetinić I. (2014). Women in Oceanography: Continuing Challenges. Oceanography 27 (4), 5–13. doi: 10.5670/oceanog.2014.106
Parsons M., Fisher K., Nalau J. (2016). Alternative Approaches to Co-Design: Insights From Indigenous/Academic Research Collaborations. Curr. Opin. Environ. Sustainability. 20, 99–105. doi: 10.1016/j.cosust.2016.07.001
Phillips B. T., Licht S., Haiat K. S., Bonney J., Allder J., Chaloux N., et al. (2019). DEEPi: A Miniaturized, Robust, and Economical Camera and Computer System for Deep-Sea Exploration. Deep Sea Res. Part I: Oceanographic Res. Papers. 153, 103136. doi: 10.1016/j.dsr.2019.103136
Pizarro O., Pace L. (2021). Editorial: Emerging Technologies With High Impact for Ocean Sciences, Ecosystem Management, and Environmental Conservation. Front. Mar. Sci. vol: 8, pp: 671877 doi: 10.3389/fmars.2021.671877
Purser A., Hoge U., Lemburg J., Wenzhofer F., Dreutter S., Dorschel B. (2020). “Deep Sea Biodiversity Hotspots: Time Series Image Data Acquisition From Challenging Ecosystems With Affordable Open Source Hardware and Software,” in Ocean Sciences Meeting 2020 (San Diego) Purser: ASLO/TOSVines: Association for Computing Machinery.
Ramirez-Llodra E., Brandt A., Danovaro R., De Mol B., Escobar E., German C. R., et al. (2010). Deep, Diverse and Definitely Different: Unique Attributes of the World’s Largest Ecosystem. Biogeosciences 7, 2851–2899. doi: 10.5194/bg-7-2851-2010
Ramirez-Llodra E., Tyler P. A., Baker M. C., Bergstad O. A., Clark M. R., Escobar Briones E., et al. (2011). Man and the Last Great Wilderness: Human Impact on the Deep Sea. PLoS One 6 (7), e22588. doi: 10.1371/journal.pone.0022588
Rogers A., Sumaila U., Hussain S., Baulcomb C. (2014). The High Seas and Us: Understanding the Value of High Seas Ecosystems (Oxford: Global Ocean Commission).
Scariot C., Heemann A., Padovani S. (2012). Understanding the Collaborative-Participatory Design. Work 41 (Suppl 1), 2701–2705. doi: 10.3233/WOR-2012-0656-2701
Sheehan E. V., Vaz S., Pettifer E., Foster N. L., Nancollas S. J., Cousens S. (2016). An Experimental Comparison of Three Towed Underwater Video Systems Using Species Metrics, Benthic Impact and Performance. Methods Ecol. Evolution. 7.7, 843–852. doi: 10.1111/2041-210X.12540
Simoncelli S., Kirillin G., Tolomeev A. P., Grossart H.-P. (2019). A Low-Cost Underwater Particle Tracking Velocimetry System for Measuring in Situ Particle Flux and Sedimentation Rate in Low-Turbulence Environments. Limnology Oceanography Methods 17.12, 665–681. doi: 10.1002/lom3.10341
Simonsen J., Robertson T. (2013). Routledge International Handbook of Participatory Design (New York: Routledge).
Smith S. L. (2012). Toward Inclusive Co-Management: Factors Influencing Stakeholder Participation. Coast. Manage. 40, 327–337. doi: 10.1080/08920753.2012.677642
Stefanoudis P. V., Licuanan W. Y., Morrison T. H., Talma S., Veitayaki J., Woodall L. C. (2021). Turning the Tide of Parachute Science. Curr. Biol. 31, R184–R185. doi: 10.1016/j.cub.2021.01.029
Teague J., Allen M. J., Scott T. B. (2018). The Potential of Low-Cost ROV for Use in Deep-Sea Mineral, Ore Prospecting and Monitoring. Ocean Engineering. 147, 333–339. doi: 10.1016/j.oceaneng.2017.10.046
Tebes J. K. (2018). Team Science, Justice, and the Co-Production of Knowledge. Amer. J. @ Commun. Psych. 62, 1–2. doi: 10.1002/ajcp.12252
Thurber A. R., Sweetman A. K., Narayanaswamy B. E., Jones D. O. B., Ingels J., Hansman R. L. (2014). Ecosystem Function and Services Provided by the Deep Sea. Biogeosciences 11, 3941–3963. doi: 10.5194/bg-11-3941-2014
Trisos C. H., Auerbach J., Katti M. (2021). Decoloniality and Anti-Oppressive Practices for a More Ethical Ecology. Nat. Ecol. Evol. 5, 1205−1212 doi: 10.1038/s41559-021-01460-w
United Nations (2018) Revised Roadmap for the UN Decade of Ocean Science for Sustainable Development. Available at: http://www.fao.org/3/CA0463EN/ca0463en.pdf (Accessed 13 Dec 2021).
Vines J., Clarke R., Wright P., McCarthy J., Olivier P. (2013). “Configuring Participation: On How We Involve People in Design,” in In Proceedings of the SIGCHI Conference on Human Factors in Computing Systems (CHI ‘13) Association for Computing Machinery(ACM, New York, NY, USA), 429–438. doi: 10.1145/2470654.2470716
Woodall L. C., Talma S., Steeds O., Stefanoudis P., Jeremie-Muzungaile M.-M., Comarond de (2021). Co-Development, Co-Production and Co-Dissemination of Scientific Research: A Case Study to Demonstrate Mutual Benefits. Biol. Lett. 17, 20200699. doi: 10.1098/rsbl.2020.0699
WWF (2015). Project Finance for Permanence: Key Outcomes and Lessons Learned ( WWF - World Wildlife Fund for Nature). Washington, DC
Keywords: ocean exploration, marine science, technology, capacity development, artificial intelligence, machine learning, co-design, participatory design
Citation: Bell KLC, Chow JS, Hope A, Quinzin MC, Cantner KA, Amon DJ, Cramp JE, Rotjan RD, Kamalu L, de Vos A, Talma S, Buglass S, Wade V, Filander Z, Noyes K, Lynch M, Knight A, Lourenço N, Girguis PR, de Sousa JB, Blake C, Kennedy BRC, Noyes TJ and McClain CR (2022) Low-Cost, Deep-Sea Imaging and Analysis Tools for Deep-Sea Exploration: A Collaborative Design Study. Front. Mar. Sci. 9:873700. doi: 10.3389/fmars.2022.873700
Received: 11 February 2022; Accepted: 15 June 2022;
Published: 11 August 2022.
Edited by:
Paolo Favali, ERIC foundation, ItalyReviewed by:
Ana Hilário, University of Aveiro, PortugalDavid A Bowden, National Institute of Water and Atmospheric Research, New Zealand
Copyright © 2022 Bell, Chow, Hope, Quinzin, Cantner, Amon, Cramp, Rotjan, Kamalu, de Vos, Talma, Buglass, Wade, Filander, Noyes, Lynch, Knight, Lourenço, Girguis, de Sousa, Blake, Kennedy, Noyes and McClain. This is an open-access article distributed under the terms of the Creative Commons Attribution License (CC BY). The use, distribution or reproduction in other forums is permitted, provided the original author(s) and the copyright owner(s) are credited and that the original publication in this journal is cited, in accordance with accepted academic practice. No use, distribution or reproduction is permitted which does not comply with these terms.
*Correspondence: Katherine L. C. Bell, Y3JvZmZAYWx1bS5taXQuZWR1
†These authors have contributed equally to this work and share first authorship