- 1Center of Deep-Sea Research, Institute of Oceanology, Chinese Academy of Sciences, Qingdao, China
- 2University of Chinese Academy of Sciences, Beijing, China
- 3Laboratory for Marine Ecology and Environmental Science, Qingdao National Laboratory for Marine Science and Technology, Qingdao, China
Single-cell RNA-sequencing (scRNA-seq) is the genomic approach that directly examines gene expression information from individual cells and provides a higher resolution of cellular differences than bulk RNA-seq. In the past decade, with the rapid development of microfluid, barcoding, and next-generation sequencing (NGS) technologies, scRNA-seq has revolutionized transcriptomic studies and gained great success and broad prospects. However, compared with the wide use in the few model animals, the application of scRNA-seq in marine organisms is limited due to the high cost of early scRNA-seq and the immature of single-cell methods for marine organisms. Recently, with the increase of genomic data, maturation of scRNA-seq platform and downstream bioinformatics algorithms, the scRNA-seq has been successfully applied in several marine model animals with great success, which demonstrated that the scRNA-seq could be the ideal and powerful tool to extend our understanding of marine organisms’ evolutionary and physiological processes and their adaptation to ecological niches. Here, we review the state-of-the-art improvements of single-cell sequencing techniques and new studies that apply single-cell methods to marine organisms. We also summarize the opportunities and challenges scientists may face in further single-cell research and propose several appealing prospects that may benefit from the combination of single-cell techniques and marine organisms.
1 Introduction
Single-cell sequencing examines the sequence information from individual cells, provding a higer resolution of cellular differences and a better understanding of the function of an individual cell in its microenvironment (Eberwine et al., 2014). With the advances in molecular biology, microfluidics and nanotechnology, single-cell sequencing methods have experienced rapid development (Stuart and Satija, 2019). From the in-depth analysis of gene expressions in only a few precious cells (Tang et al., 2009), to the establishment of high-throughput single-cell sequencing platforms (Zheng et al., 2017), the single-cell sequencing technology has gradually become a practical and efficient way to unravel the gene expression shifts among different cell subpopulations and was selected as the “Method of the Year for 2013” by Nature Methods for its potential in understanding life one cell at a time (Nature Method Editorial Board, 2014). Among the single-cell techniques (Figure 1A), single-cell RNA sequencing (scRNA-seq) is one of the most widely used, with a range of protocols for sensitive and accurate sequencing being introduced in the last decade (Svensson et al., 2017). The unique advantage of scRNA-seq over typical RNA sequencing methods is the ability to eliminate the influence of averaging signals caused by the cell homogenate procedures and distinguish expression changes due to gene regulations from those due to shifts among cell types as well (Trapnell, 2015).
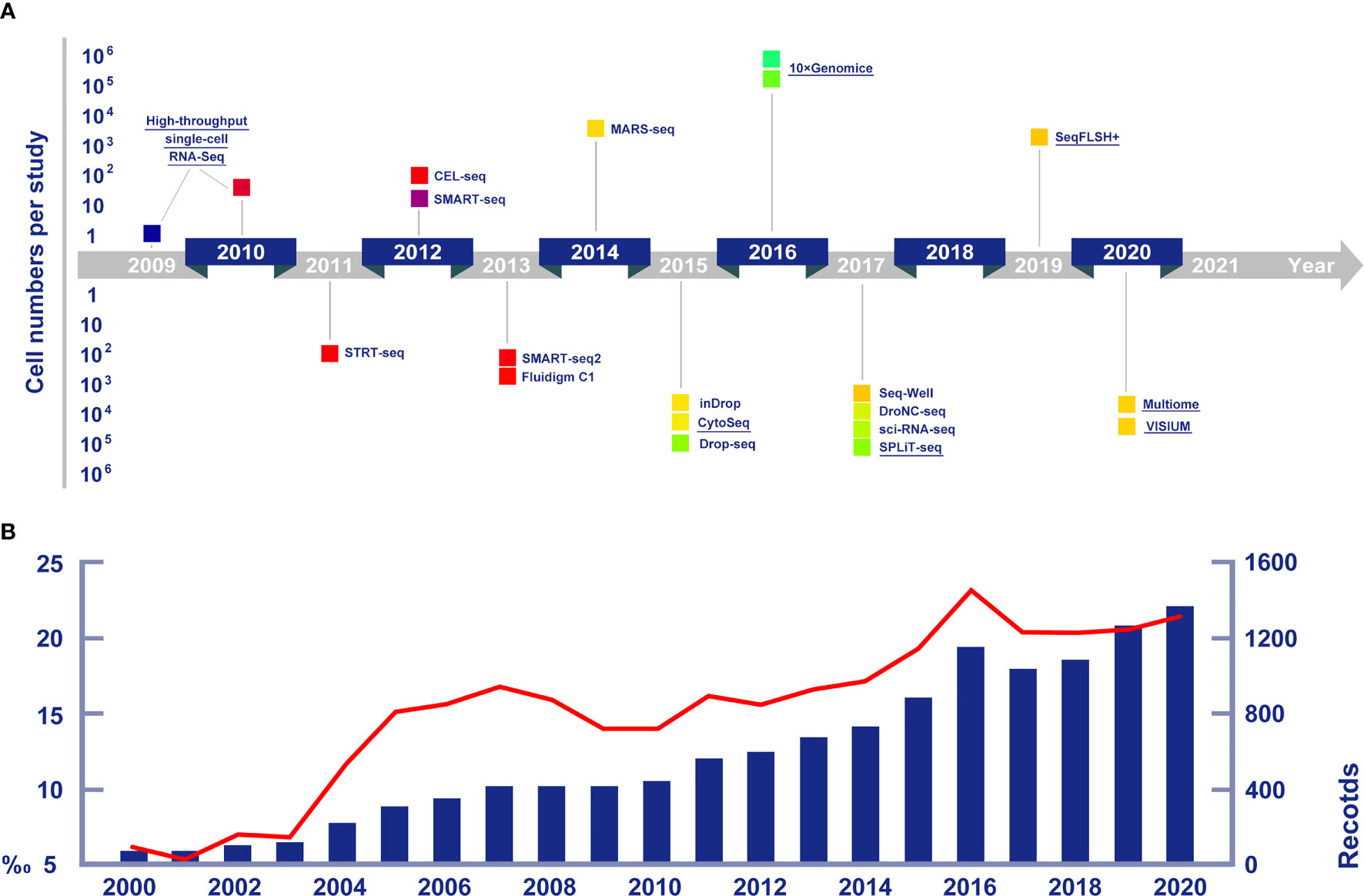
Figure 1 (A) Cell numbers reported in publications and crucial single cell sequencing technologies in chronological arrangement. Technologies mentioned in detail in this article are highlighted by underlines. Data of new technologies are collected from Eng et al. (2019, seqFISH+), Bravo et al. (2020, 10x Multiome) and Longo et al. (2021, VISIUM). (B) The increasing trends of publications on marine life RNA sequencing research (the blue histogram), and the growing proportions of marine life research among all RNA sequencing studies (the red line chart).
Typical RNA sequencing methods generally inform about the average gene expression across the overall cell population and this expression level of RNA is estimated across all cells of each subpopulation. Hence, this approach has missed the fact that individual cells could have patterns of expression that are very different from the average expression (Hong et al., 2020). Such cell-to-cell variability have important biological implications that can be deciphered by scRNA-seq data, which provide information about the degree of significance and meaning of a specific proportion of cells with a specific gene expression pattern (Gupta and Kuznicki, 2020).Therefore, scRNA-seq is an ideal method to better understand the function of multicellular tissues or organisms.
Understanding the cellular heterogeneity in diseased tissue can significantly promote the identification of precise drug targets and possibly develop new therapeutic approaches, single-cell sequencing was first put into practice in pharmaceutical and medical science (Tang et al., 2009). The possible applications of scRNA-seq are wide-ranging, including identifying stem cells, discovering new biomarkers, and detecting transcriptomic trajectories within the cell population. Consequently, numbers of articles concerning the application of scRNA-seq have greatly increased. Hitherto, scRNA-seq has been widely used not only in medicine, but also in biology, ecology and other fields (Figure 1B).
Compared to these disciplines above, marine biology has remained a brand new area for single-cell studies. As the ocean covers 70% of the earth surface and provides about 90% of the living space on the planet, marine lives are characterized by the incomparable diversity of both species and genes (Poore and Wilson, 1993). Recently, with the rapid development of scRNA-seq techniques, single-cell sequencing on marine organisms has promising perspectives,such as the application on studying marine life evolutionalry-development, symbiosis, stem cell and cell regeneration mechanism (Cao et al., 2019; Siebert et al., 2019; Hu et al., 2020; Zhang et al., 2020; Chari et al., 2021; Levy et al., 2021). Here, we review the development of scRNA-seq techniques, the single-cell data analysis methods, and highlight some recent improvements. We then discuss the opportunities of applying scRNA-seq techniques on marine organisms by summarizing the recent subcellular studies of marine multicellular organisms. Furthermore, we also argue the perspectives and possible challenges scientists might face in further studies of single-cell sequencing of marine organisms.
2 Progresses of Single-Cell Sequencing
2.1 Development of Single-Cell Sequencing Techniques
The expression of individual genes from single cells was measured for the first time in 1992 by Eberwine et al. (1992). This study uses a sophisticated approach based on in vivo reverse transcription (RT) followed by amplification through in vitro transcription (IVT). Subsequently, simpler methods based on polymerase chain reaction (PCR) emerged, leading to scaling up of the number of cells and genes assayed over the years (Lambolez et al., 1992; Sheng et al., 1994; Peixoto et al., 2004). Eventually, untargeted single-cell mRNA amplification techniques were developed, which allowed researchers to perform transcriptome-wide studies using microarrays (Kurimoto et al., 2006; Esumi et al., 2008; Kurimoto et al., 2007; Tietjen et al., 2003). Building upon this, Tang et al. (2009) adapted the technologies to make them compatible with high-throughput DNA sequencing, which allowed a completely unbiased transcriptome-wide investigation of the mRNA in a single cell for the first time. During the past decade, advances in molecular biology, microfluidics and nanotechnology have given rise to a multitude of single-cell sequencing technologies.
2.1.1 Single-Cell Sequencing Methods
The scRNA-seq is one of the most widely used single-cell sequencing approaches, with a range of technologies for sensitive, highly multiplexed, or combinatorically barcoded profiling (Hashimshony et al., 2012; Ramsköld et al., 2012; Jaitin et al., 2014; Macosko et al., 2015). The collection of single cells is fundamental to scRNA-seq, and the cell separation techniques are becoming increasingly convenient and efficient thanks to the developments in molecular biology, microfluidics and nanotechnology (Stuart and Satija, 2019). Meanwhile, scRNA-seq has inspired a variety of complementary single-cell genomic, epigenomic and proteomic profiling technologies (Shapiro et al., 2013). Recently, scRNA-seq techniques have been widely used to differentiate cell populations (Plass et al., 2018; Koiwai et al., 2021), explore the dynamics of developmental biological processes (Farrell et al., 2018; Cao et al., 2019; Pijuan-Sala et al., 2019; Soria et al., 2020), and identify gene regulatory networks (Wagner et al., 2018). Alternatively, Grindberg et al. (2013) have promoted another set of single-cell sequencing technical procedures called the single-nuclei RNA-seq (snRNA-seq). This method focuses on the transcriptomic expression in single nuclei instead of whole cells, in order to solve the problem that some cells are too large to be manipulated by microfluids. In addition, the advantage of snRNA-seq is the application of a rapid dissociation protocol. This protocol can reduce the likelihood of aberrant transcription, and minimize abnormal gene expression due to the absence of ribosomes that are located in the cytoplasm (Lacar et al., 2016).
Besides single cell transcriptome sequencing, epigenomes of cells can also be obtained by sequencing the non-coding genomic regions in chromatins (Baek and Lee, 2020). An assay for Transposase Accessible Chromatin with high-throughput sequencing (ATAC-seq) was designed to identify open chromatin regions in the genome and to depict the epigenomes of cell bulks (Buenrostro et al., 2013). Using a novel sequencing method named single-cell ATAC-seq (scATAC-seq), the chromatin accessibility landscape at the single-cell level is profiled. The sequencing result can be used to uncover the mechanisms regulating cell-to-cell heterogeneity (Chen et al., 2019). Conventional ATAC-seq is an ensemble measure of open chromatin that uses the prokaryotic Tn5 transposase to tag regulatory regions by inserting sequencing adapters into accessible regions of the genome (Buenrostro et al., 2013). In scATAC-seq, individual cells are captured and assayed using a programmable microfluidics platform, and after being amplified and sequenced, the DNA accessibility maps of each individual cell can be generated. In contrast to bulk ATAC-seq data, data from scATAC-seq include more information, such as the fragment-size periodicity corresponding to integer multiples of nucleosomes and strong enrichment of fragments within regions of accessible chromatin (Buenrostro et al., 2015). Combined with scRNA-seq, scATAC-seq has been launched on a new single cell manipulation platform called 10x Multiome®. Multiomic studies based on the conjoint analysis of transcriptomic and epigenetic data have become practical since then, and recently, Bravo González-Blas et al. (2020) firstly used this platform to achieve multiomic analysis of Drosophila eye-antennal disc cells.
2.1.2 Single-Cell Sequencing Platforms
The most challenging issue in successful application scRNA-seq is to isolate high quality single cells with minimal disruption of the cell’s native expression profile (Valihrach et al., 2018). As a result, many cell isolation protocols were introduced to isolate individual cells for sample preparation, after which the cells should become well dispersed and intact. Traditional single cell isolation technologies include micromanipulation (Woyke et al., 2009), fluorescence-activated cell sorting (FACS, Stepanauskas and Sieracki, 2007), and laser capture microdissection (LCM) (Ishii et al., 2010). Micromanipulation is the most conventional method of isolating single cells and relies on visual control to obtain pure cell populations. The main obstacle of micromanipulation is its inefficiency and low magnification due to limited resolution (Hohnadel et al., 2018). FACS measures the interactions of light with characteristics such as cell size, granularity and fluorescent structures or stains. LCM can isolate single cells, not in fluid suspension but cutting single cells in tissue sample sections.
In the past decade, many high-throughput platforms implementing single-cell sequencing have emerged (Figure 2), leading to an exponential increase in the number of cells assayed in one experiment and the expected boost of single-cell multi-omics data (Svensson et al., 2018).The most widely used modern cell isolation method is the droplet microfluidic technology. This technology typically involves the steps of encapsulating single cells in droplets in an inert carrier oil. The usage of carrier oil allows the droplets to be moved, merged and stored (Hwang et al., 2018). Performing on up to thousands of cells in seconds, the high-throughput and efficiency of this technology make it ideal for large-scale application (Choi et al., 2020). Based on this droplet microfluidic technology, the Chromium System (10x Genomics®) was launched in 2016 as a scalable platform for the characterization and profiling of hundreds to millions of cells (Zheng et al., 2017). The gel beads contain barcoded oligo-nucleotides that are mixed with reversed transcription (RT) reagents and cells in oil environment that create the droplets, wherein the cDNA is synthesized. Droplets are then pooled, dissolved, and a cDNA library containing unique molecular identifiers (UMI) is prepared. It is currently the most popular instrument for high-throughput single-cell analysis judging from the high number of publications on its application.
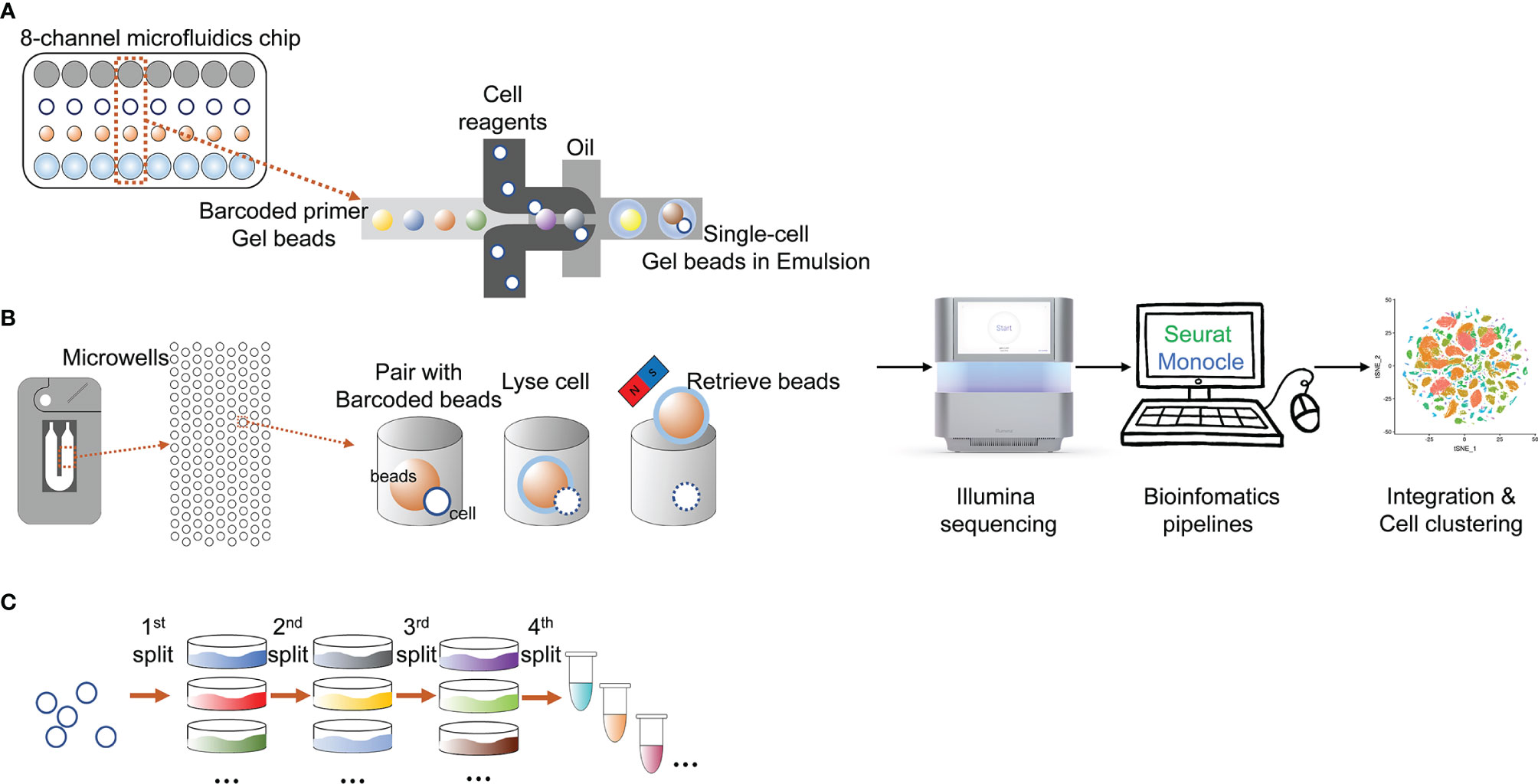
Figure 2 State-of-the-art cell isolation methods and the main procedures of different scRNA-seq technologies. (A) microfluids; (B) microchips; (C) SPLiT-seq.
Another modern cell isolation method is derived from nanowell technologies. Compared to droplet multifluidic technologies, nanowell technologies have advantages including a short cell-loading period, low reagent and sample volumes and compatibility with optical imaging (Valihrach et al., 2018). The ability to perform optical imaging allows users to examine and tune cell loading density, identify multiplets, and determine cell viabilities (Choi et al., 2020). The Rhapsody Single-Cell Analysis System (BD®) is a new instrument based on nanowell technologies, and is currently the only commercial high-throughput system that is not based on droplet technology (Valihrach et al., 2018). The Rhapsody system is based on arrays of 200,000 microwells with UMI-barcoded magnetic beads capable of capturing up to 20,000 single cells. Captured cells are lysed, and mRNA is bound to the beads. Due to the optical imaging ability, it can help users to decide how many beads are optimal for library preparation and if the quality of the sample is sufficient for downstream analysis. The remaining beads may be stored for later use (Valihrach et al., 2018).
Split-pool is an efficient and economical tool to isolate and individually barcode cells. Accordingly, Split-Pool Ligation-based Transcriptome sequencing (SPLiT-seq) is a low-cost, scRNA-seq platform that enables transcriptional profiling of hundreds of thousands of fixed cells or nuclei in a single experiment (Rosenberg et al., 2018). The most representative feature of SPLiT-seq is that no other instruments are required when applying this technique (Rosenberg et al., 2018). SPLiT-seq was recently introduced by Rosenberg et al. (2018) in their research on the development of the mouse brain and spinal cord. In SPLiT-seq, individual transcriptomes are uniquely labelled by passing a suspension of formaldehyde-fixed cells or nuclei through four rounds of combinatorial barcoding. After sequencing, each transcriptome is assembled by combining reads containing the same four-barcode combination. Four rounds of combinatorial barcoding can yield about 2×107 barcode combinations, enough to uniquely label over 1 million cells. By applying SPLiT-seq rather than conventional scRNA-seq platforms, the cellular origin of RNA in multicellular systems was successfully labelled.
The past decades witnessed the sustained efforts that have been made in developing single-cell sequencing techniques and single cell manipulating platforms, which has greatly enhanced researchers’ ability to handle a large number of single cells from diverse sources. Integrating these advancements together, single-cell sequencing achieves breakthroughs in numerous research areas and finally draws great public attention. As a result, the application of scRNA-seq or other substitute single-cell sequencing techniques in marine organisms is pressing and promising.
2.2 Development of ScRNA-Seq Data Analysis Methods
Recent advances in single-cell isolation and barcoding technologies have enabled DNA and mRNA profiles to be measured at single-cell resolution. The boost of single-cell transcriptome and genome databases calls for multiple analysis methods to characterize cell types and their functions regarding pathophysiological processes based on molecular signatures (Lee and Hwang, 2020). ScRNA-seq analysis provides abundant information of cell populations, regulatory networks, and cellular trajectories at the single-cell level (Hwang et al., 2018, Figure 3). Different methods have been developed for each kind of information to achieve diverse goals based on the corresponding information.
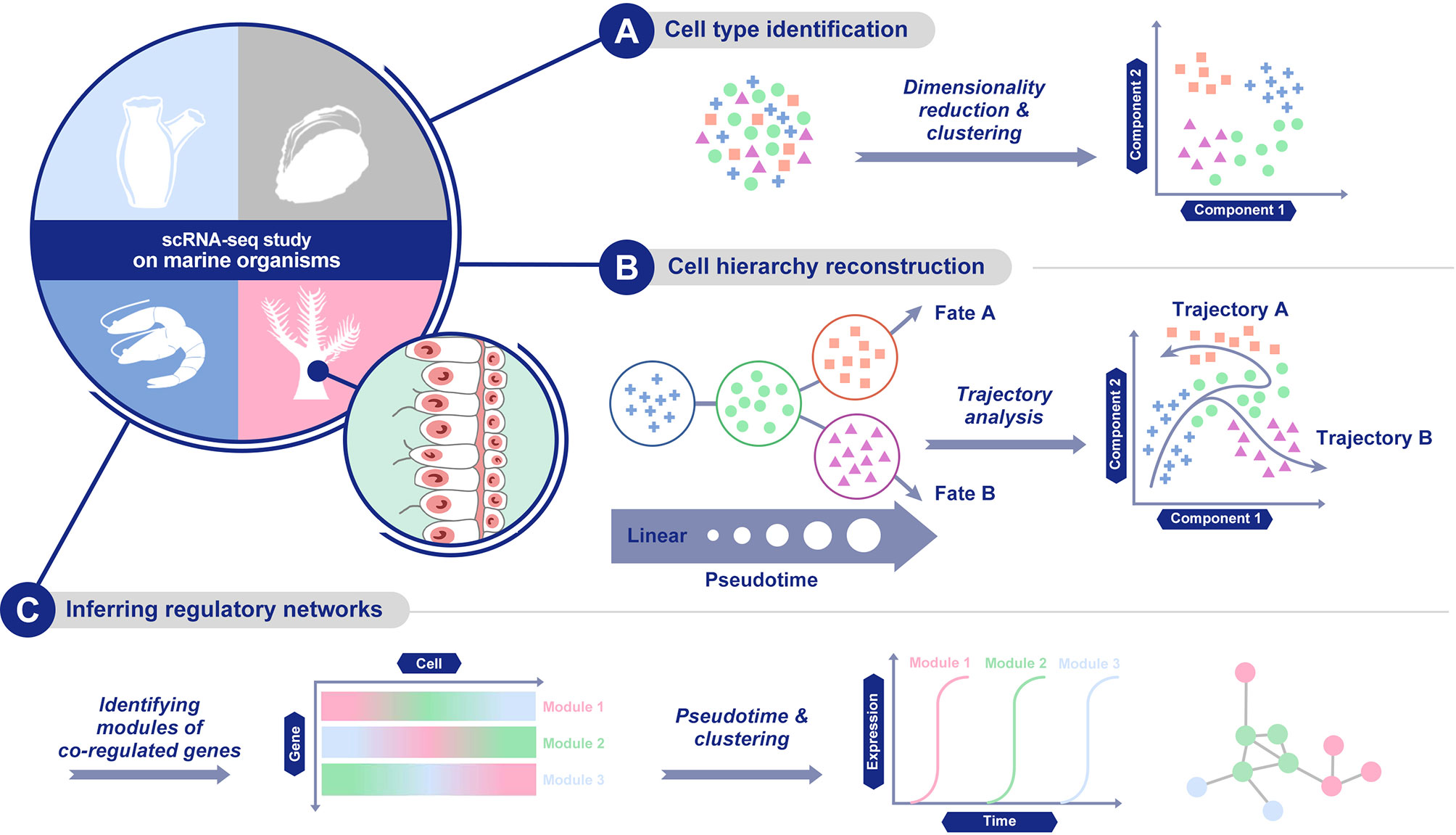
Figure 3 Main scRNA-seq data analysis methods. (A) cell population characterization via dimensionality reduction and clustering; (B) cellular trajectory reconstruction by trajectory analysis; and (C) gene regulatory network establishment.
First, for cell population characterization, the methods cluster cells based on the similarity of the expression profile and identify marker genes that are predominantly expressed in each cell cluster. Cell population characterization is based on the combination of dimensionality reduction and clustering analysis. As for dimensionality reduction methods, a linear method, principal component analysis (PCA, Wold et al., 1987), and a nonlinear method, t-distributed stochastic neighbour embedding (t-SNE, Van der Maaten and Hinton, 2008), have both been widely used. Similar to t-SNE, uniform manifold approximation and projection (UMAP) is another nonlinear dimensionality reduction method, with more preservation of the global data structure and faster computing runtime (Becht et al., 2019). Recently, methods dealing with zero-inflated data or based on neural network models have also been developed (Qi et al., 2020).
Clustering methods aim to group data objects into multiple classes or clusters so that objects in the same cluster are similar and different from objects in other clusters. They are categorized into two types based on the quantification of similarity. Clustering based on distance, such as K-means (Wong and Hartigan, 1979) and X-means (Ishioka, 2000), clusters similar objects close to each other, while clustering based on a probability distribution model (EM algorithm, Dempster et al., 1977) finds a set of objects in a group of objects that conform to a specific distribution model. Popular clustering analysis methods include Seurat (Pollen et al., 2014), BackSPIN70 (Guo and Xu, 2018), and SNN-cliq (Guo et al., 2015).
Second, another set of methods are able to infer regulatory networks. By delineating regulatory relationships among marker genes, this set of methods can show co-expression across different cells in a cell population. The analysis of gene expression and the establishment of regulatory networks in a single cell come with a variety of computational challenges (Chen and Mar, 2018). Compared with bulk sample data, single-cell gene expression data has higher rates of zero values. This can be attributed to both the true biological effects that a transcript of a gene is not expected to be produced in every cell and to technical variations, which are associated with single cell assays due to the limited amounts of biological material. Recently, several methods specialized in the inference of regulatory networks were developed, such as SCENIC (Aibar et al., 2017), SCODE (Matsumoto et al., 2017) and PIDC (Chan et al., 2017).
Finally, there is a set of methods for building the cellular trajectory describing the temporal evolution of cells, which is estimated through the transition analysis of expression profiles. Early trajectory inference methods fixed the topology of the trajectory and focused on correctly ordering the cells along with the fixed topology, but recently developed methods infer the topology of the trajectory and the order of cells on the individual branches at the same time (Lee and Hwang, 2020). When picking up suitable analysis methods for the cellular trajectory, the accuracy, scalability, stability and usability of each method should be considered carefully (Saelens et al., 2019). Nowadays, frequently-used methods include Monocle (Qiu et al., 2017), DPT (Haghverdi et al., 2016) and Wishbone (Setty et al., 2016).
2.3 Applying ScRNA-Seq on Marine Organisms
The scRNA-seq technique has been attached to significant meaning and promising perspectives once it was developed in the last century due to its unique ability to provide a detailed understanding of spatial organization and transcriptomic programs of individual cells. Especially in the last five years, numerous studies applying single-cell methods on multicellular organisms were accomplished to reveal the genome expression of individual cells, quantify the relative abundance of different cell types, and understand the cellular lineages across the life cycle (Farrell et al., 2018; Plass et al., 2018; Wagner et al., 2018; Pijuan-Sala et al., 2019; Soria et al., 2020). Predictably, because of both the rapid growth of whole-genome sequencing data and the rapid development of single cell RNA-seq technologies, the implementation of single-cell sequencing on marine multicellular organisms remains in its ascendant, and shall catch the attention of more biological scientists in the near future. Hence, here we focused on the up-to-date research about the application of scRNA-seq on marine multicellular organisms, in order to discuss the opportunities and challenges that may be encountered in future single-cell related studies (Figure 4).
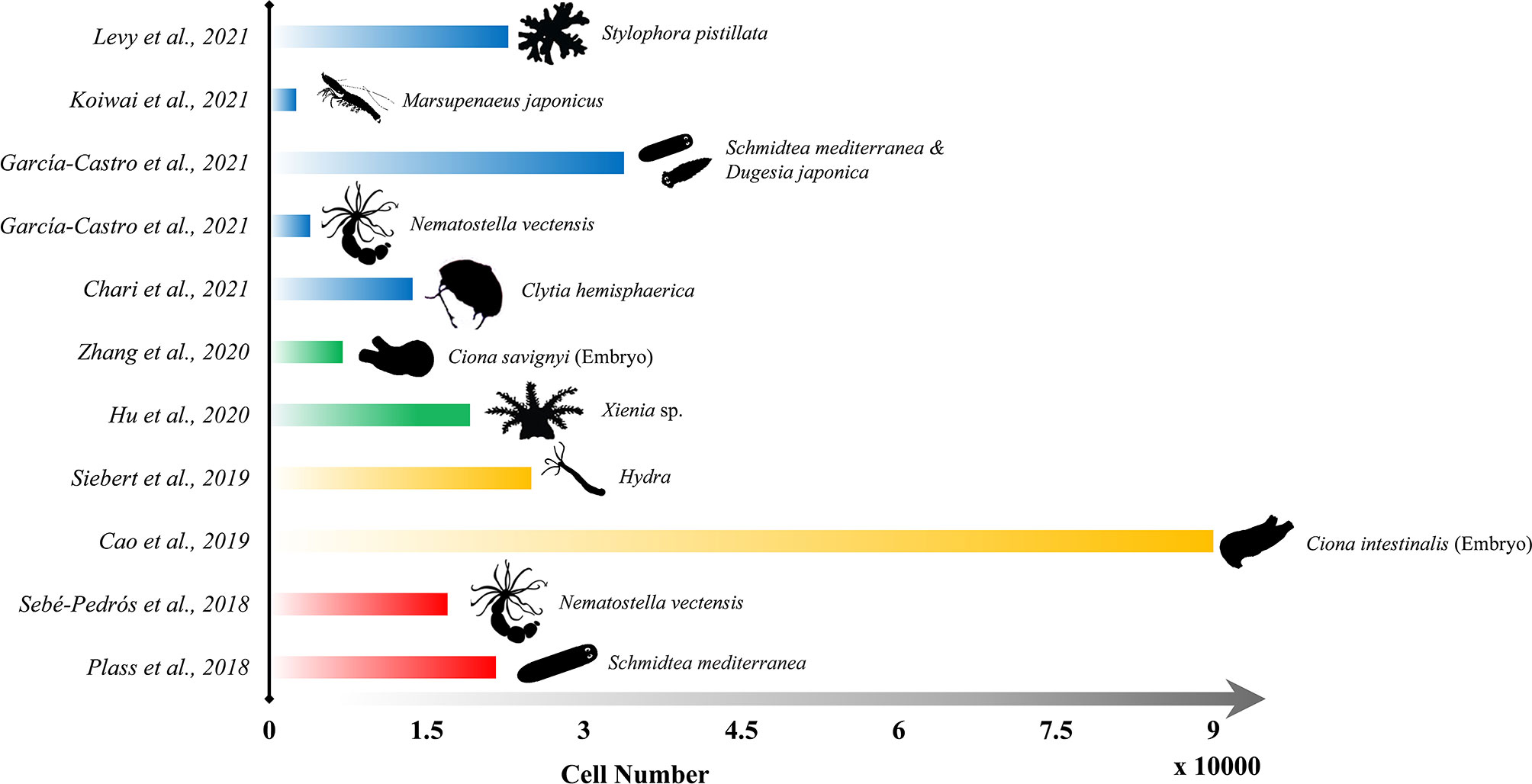
Figure 4 Representative publications of the application of scRNA-seq on marine organisms since 2018. Length of each bar refers to the sequenced cell numbers, and the outline of each species are shown on the right end of the bar.
2.3.1 ScRNA-Seq Study of Evolutionalry-Development in Marine Organisms
As one of the closest living relatives of vertebrates, the ascidian serves a valuable model in understanding developmental and physiological processes that are comparable to those of vertebrates (Delsuc et al., 2006). Ascidian embryos are simple with fewer cells, making them the perfect subject to study the developmental processes of vertebrate embryos. Using a droplet-based high-throughput scRNA-seq method, Cao et al. (2019) obtained comprehensive coverage of every cell population during the development of ascidian embryos. Single-cell transcriptomes for more than 90,000 cells spanning the entire development stage (from the onset of gastrulation to swimming tadpoles) in the ascidian Ciona intestinalis were determined, and the comprehensive transcriptome trajectories, as well as the gene regulatory networks, for over 60 cell types (including nearly 40 neuronal subtypes) comprising the Ciona tadpole were presented. This study revealed the dual properties of the Ciona notochord and the expansion of the vertebrate forebrain, providing insights into the evolutionary transition between invertebrates and vertebrates.
Furthermore, Zhang et al. (2020) applied scRNA-seq on embryos of the ascidian Ciona savignyi and acquired comparable cell population clustering results. Their work systematically examined the lineage specification in early Ciona embryogenesis. 47 cell types over eight cell cycles in the wild-type embryo were identified. And by building gene regulatory networks (GRN) of embryonic lineage development, the research indicates that Brachyury mutants, a key regulator of notochord fate in Ciona, might not be a strictly defined master regulator, and that Brachyury together with Brachyury-independent genes act in parallel in notochord GRN for notochord specification. Moreover, by matching to mouse embryo cell types, their study discovered conserved expression of a limited number of transcription factors, similar to the results between Ciona and zebrafish, or Ciona and Xenopus.
2.3.2 ScRNA-Seq Analysis of Symbiosis in Marine Organisms
Inhabitating ologotrophic tropical or subtropical oceans, many corals take up dinoflagellate algae of the Symbiodiniaceae family into their gastrodermis to maintain metabolic processes (Baker, 2003). The dinoflagellate alga resides within a lysosomal-like organelle inside the host cell and transfers diverse photosynthetic products to the coral, which in turn provides the symbiont with inorganic carbon (Davy et al., 2012). Based on single-cell sequencing technologies, it becomes practical to derive cell types and depict differentiated gene expression of the symbiotic cells in corals. Hu et al. (2020) identified 16 cell clusters out of 19,134 individual Xenia sp. cells, including gastrodermal cells and cnidocytes. Cluster 16 was attached to the endosymbiotic cells and its marker genes were predicted to encode proteins like receptors, extracellular matrix proteins, immune response proteins, phagocytosis and/or endocytosis proteins, or nutrient transporters. The cellular lineage dynamics of Cluster 16 were also examined by surgically cutting away all tentacles from Xenia polyps and observing the regeneration. The result indicated five progressive states between homeostatic conditions and the regeneration process, showing substantial changes of gene expression along pseudotime.
Recently, Levy et al. (2021) reported similar alga-hosting cell types in Stylophora pistillata, a stony coral species, and compared them to Xenia sp., both highlighted by positive gene expressions related to lipid metabolism. In this research, more than 40 cell types across the life cycle (larva, primary polyp and adult colony) of S. pistillata were identified by scRNA-seq. Among these cell types, one cell type in polyps related to skeleton-producing and two putative immune cell types were characterized by specialized gene overexpression. Moreover, comparative analysis with other cnidarian organisms provided systematic evidence of the evolutionary conservation of major cnidarian cell type programs, as well as novelties of calicoblasts and alga-hosting cell types in the stony coral.
2.3.3 ScRNA-Seq Study of Stem Cell and Cell Regeneration Mechanism in Marine Organisms
Due to the continual self-renewal and the capability of whole-body regeneration from a small piece of tissue, the cnidarian polyp Hydra keeps attracting researchers’ attention. Siebert et al. (2019) have recently generated 24,985 single-cell transcriptomes from Hydra using Drop-seq, clustered the cells and annotated cell states from stem cells to terminally differentiated cells. Three previously uncharacterized subtypes of 12 neuronal cell populations were identified: two in the ectoderm and one in the endoderm, likely composed of ganglion neurons connected throughout the Hydra body. Also, in studies on the differentiation trajectories, a new marker gene specific to the multipotent stem cell population was identified, and a previously omitted cell state was discovered to be shared by the neuron and gland cell trajectory, indicating a shared evolutionary history of these cell types.
In contrast to the research on the cnidarian polyp, new scRNA-seq applications focused on the cnidarian hydrozoan medusa (Chari et al., 2021). A new workflow, so called whole animal multiplexed single-cell sequencing (WHAM-seq), was proposed and applied on Clytia hemisphaerica, and the Clytia cell types’ response to starvation was determined. The results indicate that there is greater cell type diversity in the Clytia medusa than in its polyp-only hydrozoan cousin Hydra. The starvation experiment analysis reveals that the gastrodermal cell populations related to digestive compartments were maintained operationally as distinct ‘cell types’ rather than ‘cell states’ between starved and controlled conditions.
2.3.4 ScRNA-Seq Analysis of Marine Invertebrate’s Cellular and Molecular Immunity
Another up-to-date research using scRNA-seq focused on the subpopulation of penaeid shrimp hemocytes in order to achieve more comprehensive understanding of its immune system (Koiwai et al., 2021). ScRNA-seq was performed on Marsupenaeus japonicus hemocytes using the custom-built Drop-seq platform. Six hemocyte types (Hem1-6) were classified and their functions in cell differentiation and immune system were characterized. The result indicates that Hem1 can be categorized into oligopotent hemocytes and located upstream in the differentiation process due to the strong expression of TGase, cell proliferation, and G2/M state-related genes, while Hem1 only accounts for a very small fraction of the analyzed cells. By analyzing the expression patterns of Antimicrobial peptide (AMP) and virus responsible protein- (VRP) encoding genes, Hem3-4 was attached to importance of maintaining immune response against viruses whereas Hem5-6 are thought to be responsible for bacterial defense.
3 Future Directions of Single-Cell Sequencing on Marine Organisms
Single-cell sequencing has been regarded as among the most remarkable biological technique enhancements in the 21st century, and especially in the past decade, the rapidly-increasing sequencing scales and the great success in commercial scRNA-seq technologies have accelerated the application of single-cell sequencing in various research fields. The total number of marine oganism species reaches about 226,000, occupying a large part in the zoology (Appeltans et al., 2012). Hot research fields, such as evolutionary-developmental studies of transitional species and the environmental adaptation studies of organisms, also have urgent needs in single cell sequencing data (Jager and Manuel, 2016; Cao et al., 2019; Schenkelaars et al., 2019; Zhang et al., 2020). As a result, the single-cell studies on marine organisms, focusing on either the cell type recognition or mapping cell lineage and gene regulatory networks, are just unfolding.
New techniques capable of controlling cell quality before analysis, such as the application of optical imaging systems and user-interfaces in sequencing platforms, will improve the reliability of scRNA-seq results (Valihrach et al., 2018). Applying multiomic single-cell sequencing is also a future direction for scRNA-seq further developing as well as integrative analyzing for multiomic data. Measurements of multiomes (genome, transcriptome, epigenome and proteome) are valuebale to describe the complexity of cells (Macaulay et al., 2017; Yuan et al., 2017). However, technologies for single-cell isolation, barcoding and sequencing to measure multiomes from the same cell, as well as integrative analysis methods for multiomic data, are altoghter in urgent need to be perfected (Lee and Hwang, 2020).
Furthermore, the analysis of single-cell gene expression variations in the special context of tissue is also a crucial perspective in the development of scRNA-seq technology, since the spatial variations of transcriptomic expressions in single cells throughout certain tissues are important evidence to unravel how cellular expressions change in response to the presence and proximity to other cells (Ståhl et al., 2016). The spacial analysis could be achieved by gene expression patterning technologies such as the application of in situ hybridization (ISH) or fluorescence in situ hybridization (FISH) to highlight marker genes and visualize cell morphology and gene expression locations (Horie et al., 2018; Soria et al., 2020; Chari et al., 2021; García-Castro et al., 2021). New techniques merging scRNA-seq and marker gene localization (e.g., spatial transcriptome techniques like SeqFISH+ and VISIUM) are urgently needed in the study of marine organisms (Ståhl et al., 2016; Longo et al., 2021).
4 Conclusion
The last decade has witnessed the vigorous development of single-cell sequencing technologies, and the application of these state-of-the-art techniques on marine organisms has both vital scientific significance and promising prospects. With the improvements of whole-genome amplification methods, automated cell isolation and barcoding strategies, commercial single-cell manipulation platforms met great success, bringing high efficiency, high accuracy and relatively low costs to the application of single-cell sequencing. The development of single-cell data analysis methods has made it possible to differentiate cell groups, map cell lineage history and decipher gene regulatory networks of target organisms. Hitherto, applications of single-cell sequencing mainly focused on marine microorganisms due to the smaller gene capacity and convenience of sampling, but creative single-cell studies on marine multicellular organisms have rapidly emerged.
While the advancement of sequencing techniques and the ongoing establishment of gene reference libraries have expedited the application of single-cell sequencing on marine organisms, challenges also exist due to the difficulties in sampling and preserving marine organism samples and the scarcity of high-quality genome sequencing databases. However, three prospects of applying single-cell sequencing (cell population characterization, regulatory network establishment and cellular trajectory analysis) on marine organisms are still appealing.
Author Contributions
JL wrote all sections of the manuscript. HW and CL contributed to manuscript revision, read, and approved the submitted version. All authors contributed to the article and approved the submitted version.
Funding
This study was supported by the National Key RandD Program of China (Project Number 2018YFC0310702) and National Natural Science Foundation of China (Grant No. 42030407).
Conflict of Interest
The authors declare that the research was conducted in the absence of any commercial or financial relationships that could be construed as a potential conflict of interest.
Publisher’s Note
All claims expressed in this article are solely those of the authors and do not necessarily represent those of their affiliated organizations, or those of the publisher, the editors and the reviewers. Any product that may be evaluated in this article, or claim that may be made by its manufacturer, is not guaranteed or endorsed by the publisher.
References
Aibar S., González-Blas C. B., Moerman T., Imrichova H., Hulselmans G., Rambow F., et al. (2017). SCENIC: Single-Cell Regulatory Network Inference and Clustering. Nat. Methods 14 (11), 1083–1086. doi: 10.1038/nmeth.4463
Appeltans W., Ahyong S. T., Anderson G., Angel M. V., Artois T., Bailly N., et al. (2012). The Magnitude of Global Marine Species Diversity. Curr. Biol. 22 (23), 2189–2202. doi: 10.1016/j.cub.2012.09.036
Baek S., Lee I. (2020). Single-Cell ATAC Sequencing Analysis: From Data Preprocessing to Hypothesis Generation. Comput. Struct. Biotechnol. J. 18, 1429–1439. doi: 10.1016/j.csbj.2020.06.012
Baker A. C. (2003). Flexibility and Specificity in Coral-Algal Symbiosis: Diversity, Ecology, and Biogeography of Symbiodinium. Annu. Rev. Ecol Evolution System 34 (1), 661–689. doi: 10.1146/annurev.ecolsys.34.011802.132417
Becht E., McInnes L., Healy J., Dutertre C.-A., Kwok I. W., Ng L. G., et al. (2019). Dimensionality Reduction for Visualizing Single-Cell Data Using UMAP. Nat. Biotechnol. 37 (1), 38–44. doi: 10.1038/nbt.4314
Bravo González-Blas C., Quan X. J., Duran-Romaña R., Taskiran I. I., Koldere D., Davie K., et al. (2020). Identification of Genomic Enhancers Through Spatial Integration of Single-Cell Transcriptomics and Epigenomics. Mol. Syst. Biol. 16 (5), e9438. doi: 10.15252/msb.20209438
Buenrostro J. D., Giresi P. G., Zaba L. C., Chang H. Y., Greenleaf W. J. (2013). Transposition of Native Chromatin for Fast and Sensitive Epigenomic Profiling of Open Chromatin, DNA-Binding Proteins and Nucleosome Position. Nat. Methods 10 (12), 1213. doi: 10.1038/nmeth.2688
Buenrostro J. D., Wu B., Litzenburger U. M., Ruff D., Gonzales M. L., Snyder M. P., et al. (2015). Single-Cell Chromatin Accessibility Reveals Principles of Regulatory Variation. Nature 523 (7561), 486–490. doi: 10.1038/nmeth.2688
Cao C., Lemaire L. A., Wang W., Yoon P. H., Choi Y. A., Parsons L. R., et al. (2019). Comprehensive Single-Cell Transcriptome Lineages of a Proto-Vertebrate. Nature 571(7765), 349–354. doi: 10.1038/s41586-019-1385-y
Chan T. E., Stumpf M. P., Babtie A. C. (2017). Gene Regulatory Network Inference From Single-Cell Data Using Multivariate Information Measures. Cell Syst. 5 (3), 251–267.e253. doi: 10.1016/j.cels.2017.08.014
Chari T., Weissbourd B., Gehring J., Ferraioli A., Leclère L., Herl M., et al. (2021). Whole Animal Multiplexed Single-Cell RNA-Seq Reveals Plasticity of Clytia Medusa Cell Types. bioRxiv doi: 10.1101/2021.01.22.427844
Chen H., Lareau C., Andreani T., Vinyard M. E., Garcia S. P., Clement K., et al. (2019). Assessment of Computational Methods for the Analysis of Single-Cell ATAC-Seq Data. Genome Biol. 20 (1), 241. doi: 10.1186/s13059-019-1854-5
Chen S., Mar J. C. (2018). Evaluating Methods of Inferring Gene Regulatory Networks Highlights Their Lack of Performance for Single Cell Gene Expression Data. BMC Bioinf. 19 (1), 1–21. doi: 10.1186/s12859-018-2217-z
Choi J. R., Yong K. W., Choi J. Y., Cowie A. C. (2020). Single-Cell RNA Sequencing and Its Combination With Protein and DNA Analyses. Cells 9 (5), 1130. doi: 10.3390/cells9051130
Davy S. K., Allemand D., Weis V. M. (2012). Cell Biology of Cnidarian-Dinoflagellate Symbiosis. Microbiol. Mol. Biol. Rev. 76 (2), 229–261. doi: 10.1128/MMBR.05014-11
Delsuc F., Brinkmann H., Chourrout D., Philippe H. (2006). Tunicates and Not Cephalochordates Are the Closest Living Relatives of Vertebrates. Nature 439 (7079), 965–968. doi: 10.1038/nature04336
Dempster A. P., Laird N. M., Rubin D. B. (1977). Maximum Likelihood From Incomplete Data via the EM Algorithm. J. R. Stat. Society: Ser. B (Methodological) 39 (1), 1–22. doi: 10.1111/j.2517-6161.1977.tb01600.x
Eberwine J., Sul J. Y., Bartfai T., Kim J. (2014). The Promise of Single-Cell Sequencing. Nat. Methods 11, 25–27. doi: 10.1038/nmeth.2769
Eberwine J., Yeh H., Miyashiro K., Cao Y., Nair S., Finnell R., et al. (1992). Analysis of Gene Expression in Single Live Neurons. Proc. Natl. Acad. Sci. 89 (7), 3010–3014. doi: 10.1073/pnas.89.7.3010
Eng C. H. L., Lawson M., Zhu Q., Dries R., Koulena N., Takei Y., et al. (2019). Transcriptome-Scale Super-Resolved Imaging in Tissues by RNA seqFISH+. Nature 568 (7751), 235–239. doi: 10.1038/s41586-019-1049-y
Esumi S., Wu S.-X., Yanagawa Y., Obata K., Sugimoto Y., Tamamaki N. (2008). Method for Single-Cell Microarray Analysis and Application to Gene-Expression Profiling of GABAergic Neuron Progenitors. Neurosci. Res. 60 (4), 439–451. doi: 10.1016/j.neures.2007.12.011
Farrell J. A., Wang Y., Riesenfeld S. J., Shekhar K., Regev A., Schier A. F. (2018). Single-Cell Reconstruction of Developmental Trajectories During Zebrafish Embryogenesis. Science 360 (6392), eaar3131. doi: 10.1126/science.aar3131
García-Castro H., Kenny N. J., Iglesias M., Alvarez-Campos P., Mason V., Elek A., et al. (2021). ACME Dissociation: A Versatile Cell Fixation-Dissociation Method for Single-Cell Transcriptomics. Genome Biol. 22 (1), 89. doi: 10.1186/s13059-021-02302-5
Grindberg R. V., Yee-Greenbaum J. L., McConnell M. J., Novotny M., O’Shaughnessy A. L., Lambert G. M., et al. (2013). RNA-Sequencing From Single Nuclei. Proc. Natl. Acad. Sci. 110 (49), 19802–19807. doi: 10.1073/pnas.1319700110
Guo M., Wang H., Potter S. S., Whitsett J. A., Xu Y. (2015). SINCERA: A Pipeline for Single-Cell RNA-Seq Profiling Analysis. PLoS Comput. Biol. 11 (11), e1004575. doi: 10.1371/journal.pcbi.1004575
Guo M., Xu Y. (2018). “Single-Cell Transcriptome Analysis Using SINCERA Pipeline,” in Transcriptome Data Analysis (Cham, Switzerland: Springer), 209–222.
Gupta R. K., Kuznicki J. (2020). Biological and Medical Importance of Cellular Heterogeneity Deciphered by Single-Cell RNA Sequencing. Cells 9 (8), 1751. doi: 10.3390/cells9081751
Haghverdi L., Büttner M., Wolf F. A., Buettner F., Theis F. J. (2016). Diffusion Pseudotime Robustly Reconstructs Lineage Branching. Nat. Methods 13 (10), 845. doi: 10.1038/nmeth.3971
Hashimshony T., Wagner F., Sher N., Yanai I. (2012). CEL-Seq: Single-Cell RNA-Seq by Multiplexed Linear Amplification. Cell Rep. 2 (3), 666–673. doi: 10.1016/j.celrep.2012.08.003
Hohnadel M., Maumy M., Chollet R. (2018). Development of a Micromanipulation Method for Single Cell Isolation of Prokaryotes and Its Application in Food Safety. PLoS One 13 (5), e0198208. doi: 10.1371/journal.pone.0198208
Hong M., Tao S., Zhang L., Diao L. T., Huang X., Huang S., et al. (2020). RNA Sequencing: New Technologies and Applications in Cancer Research. J. Hematol. Oncol. 13 (1), 166. doi: 10.1186/s13045-020-01005-x
Horie R., Hazbun A., Chen K., Cao C., Levine M., Horie T. (2018). Shared Evolutionary Origin of Vertebrate Neural Crest and Cranial Placodes. Nature 560 (7717), 228–232. doi: 10.1038/s41586-018-0385-7
Hu M., Zheng X., Fan C. M., Zheng Y. (2020). Lineage Dynamics of the Endosymbiotic Cell Type in the Soft Coral Xenia. Nature 582 (7813), 534–538. doi: 10.1038/s41586-020-2385-7
Hwang B., Lee J. H., Bang D. (2018). Single-Cell RNA Sequencing Technologies and Bioinformatics Pipelines. Exp. Mol. Med. 50 (8), 1–14. doi: 10.1038/s12276-018-0071-8
Ishii S., Tago K., Senoo K. (2010). Single-Cell Analysis and Isolation for Microbiology and Biotechnology: Methods and Applications. Appl. Microbiol. Biotechnol. 86 (5), 1281–1292. doi: 10.1007/s00253-010-2524-4
Ishioka T. (2000). Extended K-Means With an Efficient Estimation of the Number of Clusters. Ouyou toukeigaku 29 (3), 141–149. doi: 10.5023/jappstat.29.141
Jager M., Manuel M. (2016). Ctenophores: An Evolutionary-Developmental Perspective. Curr. Opin. Genet. Dev. 39, 85–92. doi: 10.1016/j.gde.2016.05.020
Jaitin D. A., Kenigsberg E., Keren-Shaul H., Elefant N., Paul F., Zaretsky I., et al. (2014). Massively Parallel Single-Cell RNA-Seq for Marker-Free Decomposition of Tissues Into Cell Types. Science 343 (6172), 776–779. doi: 10.1126/science.1247651
Koiwai K., Koyama T., Tsuda S., Toyoda A., Kikuchi K., Suzuki H., et al. (2021). Single-Cell RNA-Seq Analysis Reveals Penaeid Shrimp Hemocyte Subpopulations and Cell Differentiation Process. Elife 10, e66954. doi: 10.7554/eLife.66954
Kurimoto K., Yabuta Y., Ohinata Y., Ono Y., Uno K. D., Yamada R. G., et al. (2006). An Improved Single-Cell cDNA Amplification Method for Efficient High-Density Oligonucleotide Microarray Analysis. Nucleic Acids Res. 34 (5), e42. doi: 10.1093/nar/gkl050
Lambolez B., Audinat E., Bochet P., Crépel F., Rossier J. (1992). AMPA Receptor Subunits Expressed by Single Purkinje Cells. Neuron 9 (2), 247–258. doi: 10.1016/0896-6273(92)90164-9
Lacar B., Linker S. B., Jaeger B. N., Krishnaswami S. R., Barron J. J., Kelder M. J. E., et al. (2016). Nuclear RNA-Seq of Single Neurons Reveals Molecular Signatures of Activation. Nat. Commun. 7, 11022. doi: 10.1038/ncomms11022
Lee J., Hwang D. (2020). Single-Cell Multiomics: Technologies and Data Analysis Methods. Exp. Mol. Med. 52 (9), 1428–1442. doi: 10.1038/s12276-020-0420-2
Levy S., Elek A., Grau-Bové X., Menéndez-Bravo S., Iglesias M., Tanay A., et al. (2021). A Stony Coral Cell Atlas Illuminates the Molecular and Cellular Basis of Coral Symbiosis, Calcification, and Immunity. Cell 184 (11), 2973–2987. doi: 10.1016/j.cell.2021.04.005
Longo S. K., Guo M. G., Ji A. L., Khavari P. A. (2021). Integrating Single-Cell and Spatial Transcriptomics to Elucidate Intercellular Tissue Dynamics. Nat. Rev. Genet. 22 (10), 1–18. doi: 10.1038/s41576-021-00370-8
Macaulay I. C., Ponting C. P., Voet T. (2017). Single-Cell Multiomics: Multiple Measurements From Single Cells. Trends Genet. 33 (2), 155–168. doi: 10.1016/j.tig.2016.12.003
Macosko E. Z., Basu A., Satija R., Nemesh J., Shekhar K., Goldman M., et al. (2015). Highly Parallel Genome-Wide Expression Profiling of Individual Cells Using Nanoliter Droplets. Cell 161 (5), 1202–1214. doi: 10.1016/j.cell.2015.05.002
Matsumoto H., Kiryu H., Furusawa C., Ko M. S., Ko S. B., Gouda N., et al. (2017). SCODE: An Efficient Regulatory Network Inference Algorithm From Single-Cell RNA-Seq During Differentiation. Bioinformatics 33 (15), 2314–2321. doi: 10.1093/bioinformatics/btx194
Mosmann T. R., Cherwinski H., Bond M. W., Giedlin M. A., Coffman R. L. (1986). Two Types of Murine Helper T Cell Clone. I. Definition According to Profiles of Lymphokine Activities and Secreted Proteins. J. Immunol. 136 (7), 2348–2357.
Nature Method Editorial Board (2014). Method of the Year 2013. Nat. Methods 11 (1), 1–1. doi: 10.1038/nmeth.2801
Peixoto A., Monteiro M., Rocha B., Veiga-Fernandes H. (2004). Quantification of Multiple Gene Expression in Individual Cells. Genome Res. 14 (10a), 1938–1947. doi: 10.1101/gr.2890204
Pijuan-Sala B., Griffiths J. A., Guibentif C., Hiscock T. W., Jawaid W., Calero-Nieto F. J., et al. (2019). A Single-Cell Molecular Map of Mouse Gastrulation and Early Organogenesis. Nature 566 (7745), 490–495. doi: 10.1038/s41586-019-0933-9
Plass M., Solana J., Wolf F. A., Ayoub S., Misios A., Glažar P., et al. (2018). Cell Type Atlas and Lineage Tree of a Whole Complex Animal by Single-Cell Transcriptomics. Science 360 (6391), eaaq1723. doi: 10.1126/science.aaq1723
Pollen A. A., Nowakowski T. J., Shuga J., Wang X., Leyrat A. A., Lui J. H., et al. (2014). Low-Coverage Single-Cell mRNA Sequencing Reveals Cellular Heterogeneity and Activated Signaling Pathways in Developing Cerebral Cortex. Nat. Biotechnol. 32 (10), 1053. doi: 10.1038/nbt.2967
Poore G. C. B., Wilson G. D. F. (1993). Marine Species Richness. Nature 361 (6413), 597–598. doi: 10.1038/361597a0
Qi R., Ma A., Ma Q., Zou Q. (2020). Clustering and Classification Methods for Single-Cell RNA-Sequencing Data. Briefings Bioinf. 21 (4), 1196–1208. doi: 10.1093/bib/bbz062
Qiu X., Mao Q., Tang Y., Wang L., Chawla R., Pliner H. A., et al. (2017). Reversed Graph Embedding Resolves Complex Single-Cell Trajectories. Nat. Methods 14 (10), 979. doi: 10.1038/nmeth.4402
Ramsköld D., Luo S., Wang Y.-C., Li R., Deng Q., Faridani O. R., et al. (2012). Full-Length mRNA-Seq From Single-Cell Levels of RNA and Individual Circulating Tumor Cells. Nat. Biotechnol. 30 (8), 777–782. doi: 10.1038/nbt.2282
Rosenberg A. B., Roco C. M., Muscat R. A., Kuchina A., Sample P., Yao Z., et al. (2018). Single-Cell Profiling of the Developing Mouse Brain and Spinal Cord With Split-Pool Barcoding. Science 360 (6385), 176–182. doi: 10.1126/science.aam8999
Saelens W., Cannoodt R., Todorov H., Saeys Y. (2019). A Comparison of Single-Cell Trajectory Inference Methods. Nat. Biotechnol. 37 (5), 547–554. doi: 10.1038/s41587-019-0071-9
Schenkelaars Q., Vernale A., Fierro-Constaín L., Borchiellini C., Renard E. (2019). “A Look Back Over 20 Years of Evo-Devo Studies on Sponges: A Challenged View of Urmetazoa,” in Evolution, Origin of Life, Concepts and Methods (Springer), 135–160.
Setty M., Tadmor M. D., Reich-Zeliger S., Angel O., Salame T. M., Kathail P., et al. (2016). Wishbone Identifies Bifurcating Developmental Trajectories From Single-Cell Data. Nat. Biotechnol. 34 (6), 637–645. doi: 10.1038/nbt.3569
Shapiro E., Biezuner T., Linnarsson S. (2013). Single-Cell Sequencing-Based Technologies Will Revolutionize Whole-Organism Science. Nat. Rev. Genet. 14 (9), 618–630. doi: 10.1038/nrg3542
Sheng H. Z., Lin P. X., Nelson P. G. (1994). Analysis of Multiple Heterogeneous mRNAs in Single Cells. Analytical Biochem. 222 (1), 123–130. doi: 10.1006/abio.1994.1463
Siebert S., Farrell J. A., Cazet J. F., Abeykoon Y., Primack A. S., Schnitzler C. E., et al. (2019). Stem Cell Differentiation Trajectories in Hydra Resolved at Single-Cell Resolution. Science 365 (6451), eaav9314. doi: 10.1126/science.aav9314
Soria C. L. D., Lee J., Chong T., Coghlan A., Tracey A., Young M. D., et al. (2020). Single-Cell Atlas of the First Intra-Mammalian Developmental Stage of the Human Parasite Schistosoma Mansoni. Nat. Commun. 11 (1), 1–16. doi: 10.1038/s41467-020-20092-5
Ståhl P. L., Salmén F., Vickovic S., Lundmark A., Navarro J.F., Magnusson J., et al. (2016). Visualization and Analysis of Gene Expression in Tissue Sections by Spatial Transcriptomics. Science 353 (6294), 78–82. doi: 10.1126/science.aaf2403
Stepanauskas R., Sieracki M. E. (2007). Matching Phylogeny and Metabolism in the Uncultured Marine Bacteria, One Cell at a Time. Proc. Natl. Acad. Sci. 104 (21), 9052. doi: 10.1073/pnas.0700496104
Stuart T., Satija R. (2019). Integrative Single-Cell Analysis. Nat. Rev. Genet. 20 (5), 257–272. doi: 10.1038/s41576-019-0093-7
Svensson V., Natarajan K. N., Ly L.-H., Miragaia R. J., Labalette C., Macaulay I. C., et al. (2017). Power Analysis of Single-Cell RNA-Sequencing Experiments. Nat. Methods 14 (4), 381–387. doi: 10.1038/nmeth.4220
Svensson V., Vento-Tormo R., Teichmann S. A. (2018). Exponential Scaling of Single-Cell RNA-Seq in the Past Decade. Nat. Protoc. 13 (4), 599–604. doi: 10.1038/nprot.2017.149
Tang F., Barbacioru C., Wang Y., Nordman E., Lee C., Xu N., et al. (2009). mRNA-Seq Whole-Transcriptome Analysis of a Single Cell. Nat. Methods 6 (5), 377–382. doi: 10.1038/nmeth.1315
Tietjen I., Rihel J. M., Cao Y., Koentges G., Zakhary L., Dulac C. (2003). Single-Cell Transcriptional Analysis of Neuronal Progenitors. Neuron 38 (2), 161–175. doi: 10.1016/S0896-6273(03)00229-0
Trapnell C. (2015). Defining Cell Types and States With Single-Cell Genomics. Genome Res. 25 (10), 1491–1498. doi: 10.1101/gr.190595.115
Valihrach L., Androvic P., Kubista M. (2018). Platforms for Single-Cell Collection and Analysis. Int. J. Mol. Sci. 19 (3), 807. doi: 10.3390/ijms19030807
Van der Maaten L., Hinton G. (2008). Visualizing Data Using T-SNE. J. Mach. Learn. Res. 9 (11). doi: 10.48550/arXiv.2108.01301
Wagner D. E., Weinreb C., Collins Z. M., Briggs J. A., Megason S. G., Klein A. M. (2018). Single-Cell Mapping of Gene Expression Landscapes and Lineage in the Zebrafish Embryo. Science 360 (6392), 981–987. doi: 10.1126/science.aar4362
Weigand H., Beermann A. J., Čiampor F., Costa F. O., Csabai Z., Duarte S., et al. (2019). DNA Barcode Reference Libraries for the Monitoring of Aquatic Biota in Europe: Gap-Analysis and Recommendations for Future Work. Sci. Total Environ. 678, 499–524. doi: 10.1016/j.scitotenv.2019.04.247
Wold S., Esbensen K., Geladi P. (1987). Principal Component Analysis. Chemometrics Intelligent Lab. Syst. 2 (1-3), 37–52. doi: 10.1016/0169-7439(87)80084-9
Wong M. A., Hartigan J. (1979). Algorithm as 136: A K-Means Clustering Algorithm. J. R. Stat. Society Ser. C (Applied Statistics) 28 (1), 100–108. doi: 10.2307/2346830
Woyke T., Xie G., Copeland A., Gonzalez J. M., Han C., Kiss H., et al. (2009). Assembling the Marine Metagenome, One Cell at a Time. PLoS One 4 (4), e5299. doi: 10.1371/journal.pone.0005299
Yuan G.-C., Cai L., Elowitz M., Enver T., Fan G., Guo G., et al. (2017). Challenges and Emerging Directions in Single-Cell Analysis. Genome Biol. 18 (1), 1–8. doi: 10.1186/s13059-017-1218-y
Zhang T., Xu Y., Imai K., Fei T., Wang G., Dong B., et al. (2020). A Single-Cell Analysis of the Molecular Lineage of Chordate Embryogenesis. Sci. Adv. 6 (45), eabc4773. doi: 10.1126/sciadv.abc4773
Keywords: scRNA-seq, marine organism, snRNA-seq, future directions, cell isolation method
Citation: Li J, Wang H and Li C (2022) Single-Cell Sequencing on Marine Life: Application and Future Development. Front. Mar. Sci. 9:906267. doi: 10.3389/fmars.2022.906267
Received: 28 March 2022; Accepted: 18 May 2022;
Published: 21 June 2022.
Edited by:
Taewoo Ryu, Okinawa Institute of Science and Technology Graduate University, JapanReviewed by:
Yi Lan, Hong Kong University of Science and Technology, Hong Kong, SAR ChinaJack Chi-Ho Ip, Hong Kong Baptist University, Hong Kong, SAR China
Copyright © 2022 Li, Wang and Li. This is an open-access article distributed under the terms of the Creative Commons Attribution License (CC BY). The use, distribution or reproduction in other forums is permitted, provided the original author(s) and the copyright owner(s) are credited and that the original publication in this journal is cited, in accordance with accepted academic practice. No use, distribution or reproduction is permitted which does not comply with these terms.
*Correspondence: Chaolun Li, bGNsQHFkaW8uYWMuY24=
†These authors share first authorship