- 1Bigelow Laboratory for Ocean Sciences, Tandy Center for Ocean Forecasting, East Boothbay, ME, United States
- 2Maine Department of Marine Resources, Boothbay, ME, United States
- 3Bigelow Analytical Services, Bigelow Laboratory for Ocean Sciences, East Boothbay, ME, United States
- 4Biology Department Southern Maine Community College, South Portland, ME, United States
- 5Bangs Island Mussels, Portland, ME, United States
- 6Lovepoint Oysters, South Freeport, ME, United States
Paralytic shellfish poison (PSP) is a human health concern for shellfish aquaculture and wild harvest. This paper discusses lessons learned from a forecasting program for PSP in coastal Maine, USA, designed based on stakeholder input, and run in an operational mode for the 2021 season. The forecast uses a deep learning algorithm to make site-specific, probabilistic forecasts at a weekly forecast range for toxin levels measured in shellfish tissue. Forecasts had high accuracy in the 2021 season, correctly predicting closure events and locations despite a highly unusual season. Stakeholders reported a positive view of the forecast system, and stakeholder input continues to be of key importance as further modifications are made to the system. There are benefits and challenges to the stakeholder-based design of the system.
Introduction
Paralytic shellfish poison (PSP) has long been a concern in coastal Maine (Hurst and Yentsch, 1981), as in other parts of the world (Jin et al., 2020). With aquaculture representing the fastest growing food production sector globally (Tacon, 2020), the demand for early warning of high toxicity levels in shellfish has grown as well. Previously, forecasting programs for PSP toxin-producing species like Alexandrium catenella focused on broad-scale seasonal dynamics, and water column and cyst bed abundance and distribution, generally driven by hydrodynamic modeling (McGillicuddy et al., 2011 Valbi et al., 2019). Meetings and conversations with local stakeholders (growers/harvesters, managers), however, have recently identified different emerging needs. Here we report on a forecasting program designed based on these expressed needs, with the following properties: (1) ongoing communication with stakeholders to incorporate feedback; (2) predictions of toxicity in shellfish, rather than predictions of algal abundance variables; (3) site-specific within the complex, near-shore sites of shellfish production and harvesting; and (4) near-term (1-2 week) forecasting range. The shift to a stakeholder-driven approach has benefits as well as new challenges as compared with more traditional forecasting paradigms. Historically, most harmful algal bloom forecasts have been developed for densely populated areas (Ralston and Moore, 2020). Maine has the highest rural population proportion in the United States (U.S. Census Bureau, 2010) and can serve as a model for how to approach forecasting system design as the technology spreads to less populated communities.
Benefits and Challenges
Maine has a rapidly growing shellfish aquaculture industry with careful toxin monitoring. Paralytic shellfish toxins (PST) are sampled in shellfish tissue weekly at multiple sites by the Maine Department of Marine Resources (DMR) and are processed by Bigelow Analytical Services (BAS). The toxin testing dataset dates back to 2014 and follows a chemical analytical technique that uses high-performance liquid chromatography (HPLC) approved for regulatory purposes (Rourke et al., 2008), generating information on 12 congeners of saxitoxin that contribute to a total toxicity value for each sample (µg SAX eq 100 g-1 shellfish). Prior to the 2021 season, we had developed and evaluated a forecasting algorithm for the weekly PST measurements. The forecast used a deep learning neural network to predict probabilities that toxin levels would exceed the closure threshold (>80 µg SAX eq 100 g-1 shellfish), and showed high skill in a simulated forecast mode (Grasso et al., 2019). In 2021, we conducted the first operational deployment of the forecasting system, gathering feedback from a focus group of stakeholders to inform development of the system. There were some minor modifications from the tested model – evaluation and inclusion of environmental covariates, and refinement of the neural network topology – but the core approach was the same. The first live forecasting season was generally successful, with a few lessons to carry forward.
Lesson 1: Ongoing communication with stakeholders shaped the forecasting system in real time. In the design of the system, we used a knowledge co-production approach (Norström et al., 2020), which is iterative and collaborative, and allows for ongoing learning. For example, with accessibility in mind, we developed a simple, text-based communication system, delivered via email. Feedback from industry and management stakeholders, however, indicated a preference for a more complex graphical display of the forecast. As a response, in collaboration with the DMR, we developed a map-based communication tool (Figure 1). This tool has been integrated with the DMR’s other data access tools and has since received positive feedback in surveys of the stakeholders. Similarly, feedback from stakeholders helped to guide modifications in the forecasting window (the span of days for which a forecast was valid) and the timing of forecast delivery, in order to better fit with decision making timelines.
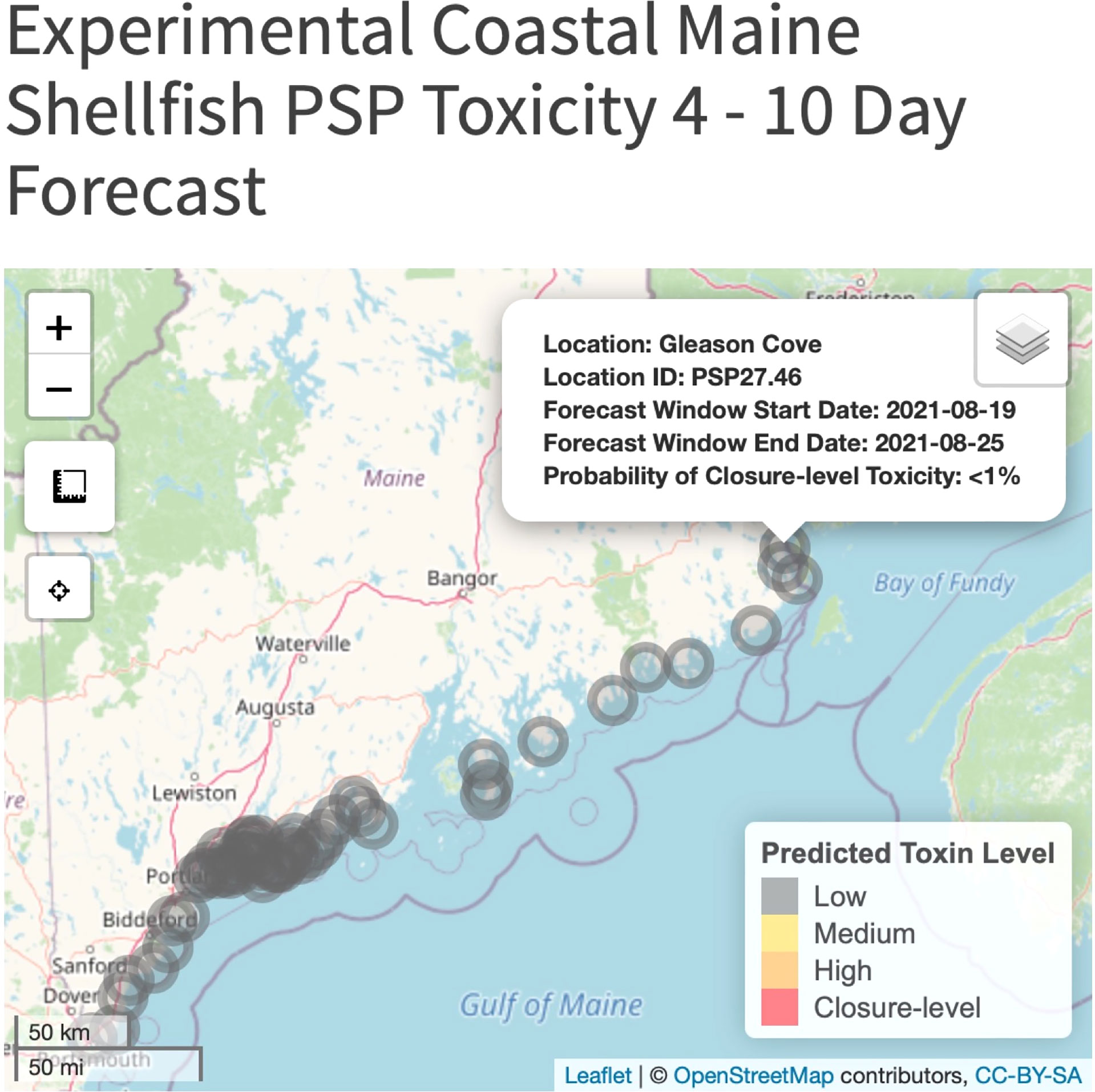
Figure 1 PSP toxicity forecast communication tool, developed by the Maine DMR. The tool provides a simple site-clickable map with closure-level toxicity prediction expressed as a probability (%). Forecasts are available here: https://mainedmr.shinyapps.io/bph_phyto/.
In some cases, ongoing communication and feedback reaffirmed aspects of the forecasting system design. For example, regardless of the communication approach, stakeholders from industry and management emphasized that communicating the forecast as a probability was a useful aspect. This is notable, as other ecological forecasting programs have found that users do not have the same intuition for probabilistic forecasts that they might have when interpreting weather forecasts (Pershing et al., 2018). Here, because the closure-level toxicity is provided as a probability, stakeholders have the opportunity to develop their own strategies over time regarding how to manage different levels of risk. As a second example, stakeholders reaffirmed that a 1-2 week forecast range and site-specific spatial scales were useful for decision making, for both industry (operations decisions like harvesting amounts and locations) and management (prioritizing monitoring resources). As the pool of users grows, further feedback is expected to guide refinements of the system design and its communication. The tradeoff to having on-the-water knowledge and feedback is that responding in real time can be difficult. With the potential for such frequent and rapid changes to the system, it helps to have clear criteria for evaluating changes and their impacts before implementing them.
Lesson 2: Preparations for low- or no-analogue conditions saved the forecasting season. The stakeholder need for location-specific and near term predictions across many sites exceeded the current capabilities of process-based models in the region. To meet this need, we used newer deep learning algorithms. One drawback to these algorithms is that because they are largely empirical, they often do not perform as well in novel conditions. The 2021 monitoring season (March-October) was one of the lowest PSP seasons on record, with only two closure-level events measured out of 741 samples taken, or < 0.3%. This is outside the standard deviation of the long-term pattern (200 ± 185 closure-level measurements per year, and 43 ± 28 stations per year registering closure-level toxicity, since 1975), and occurred despite temperatures being consistently warmer than the long term average in coastal Maine, both at the surface and at depth. Because the algorithm incorporates two weeks of autocorrelation, it was able to make forecasts for 533 of the measurements (72%)–thus the two closure-level events represent <0.4% of forecasts. This represents an extreme class imbalance problem, where the most crucial class (i.e. closure-level) occurred far less frequently than the others. There was minimal analogue in the training data to such an extreme imbalance, providing a very challenging test for a learning algorithm. To illustrate this challenge: a hypothetical algorithm that had predicted zero closures through all of 2021 could still have had an accuracy > 99% but would not have had any practical use and could have hurt the perceived reliability of the forecast among stakeholders.
The extreme conditions of the 2021 season provided a valuable test case for the forecasting algorithm in that it pushed the algorithm beyond the type of season it had been trained on. Because there had been a focus on the class imbalance problem during development, both of the closure-level events, which occurred at the Bear Island site in western Maine, were predicted correctly (Figure 2). There were two other occasions where closure-level toxicity was predicted above 50%, but where subsequent measured values were below the closure level. One instance was during the week following the closure on Bear Island, where closure-level toxicity was predicted at a 56% probability, but the measured value had declined just below the closure level–the sort of near miss that is common in a probabilistic forecast. The other was at the Gurnet site, also in western Maine, where the prediction was 95%, but the subsequent measurement was significantly below the closure threshold. This outlier case illustrates one drawback to an empirical, machine learning forecast: it is difficult to trace a missed forecast to a known mechanism the way one could with a process-based forecast. Given the challenge of the extreme class imbalance of the season, the forecast performed remarkably well. Along the eastern half of the coast, measured toxicity levels never increased above classification level zero, and the forecasting system correctly predicted every case. Nevertheless, in an environment that is rapidly changing, there is an increased possibility that novel conditions will challenge a forecast.
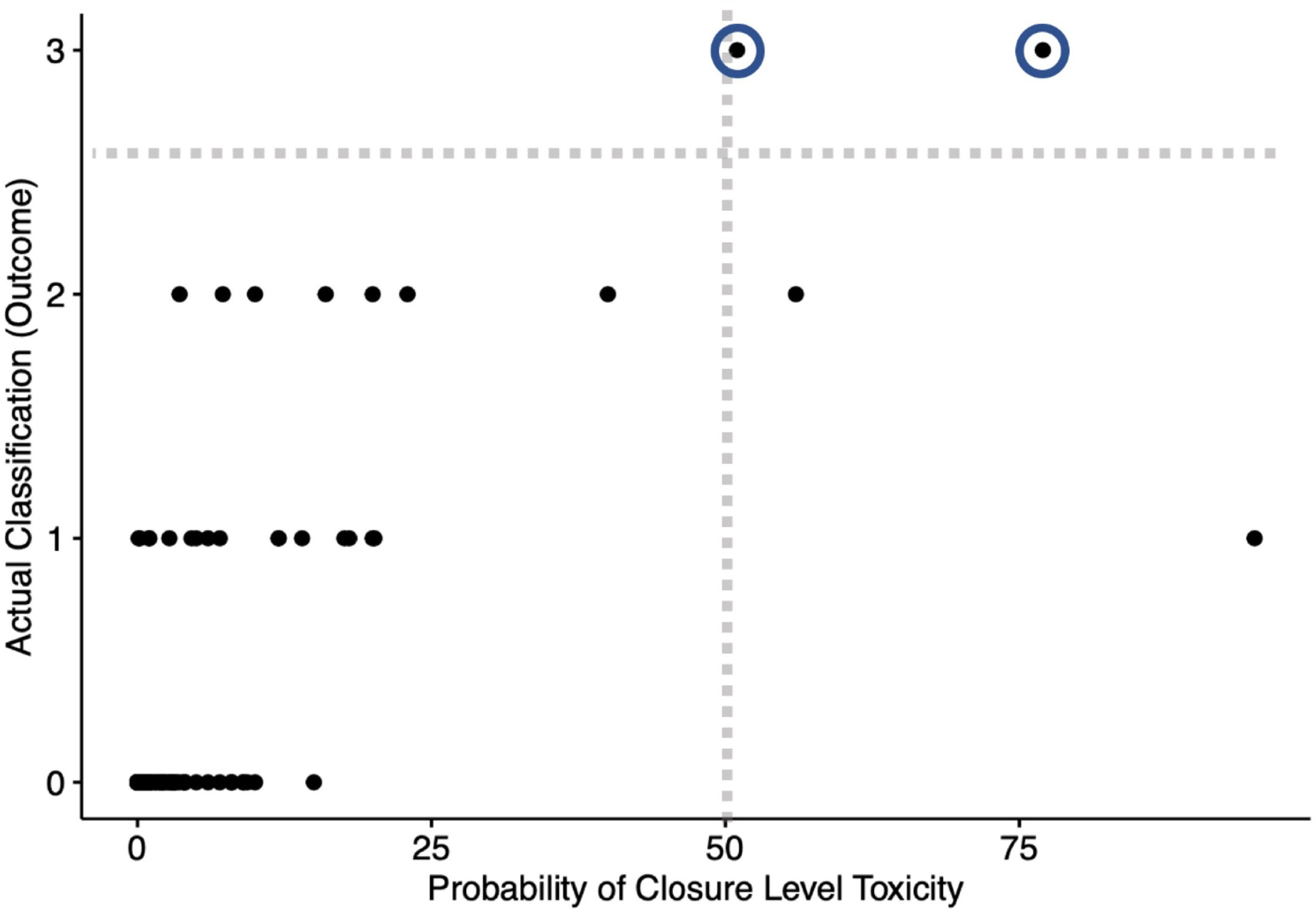
Figure 2 Toxicity classification levels at all sites/times where a forecast was made for the 2021 season, compared to the probability of a closure predicted one week ahead of time. Classifications represent toxicity levels measured in shellfish, with level 3 representing a closure-level toxicity (toxicity for classification levels 0-3 are, respectively, 0-10, 10-30, 30-80, and >80 µg SAX eq 100 g-1 shellfish). The circled points represent the two closure-level events that occurred, both correctly predicted.
Lesson 3: Following a Technology Readiness Levels (TRL) framework (Héder, 2017) helped to avoid pitfalls. The TRL approach offers a stepwise sequence for transitioning a technology from the basic or conceptual level to operational deployment. In this case, taking a gradual approach to open access, including a pilot season where stakeholder feedback is incorporated, has strengthened both the forecast and its perception among users. Importantly, this approach can help to avoid some of the pitfalls of forecasting such as reflexivity and confirmation bias (Record and Pershing, 2021), unintended consequences (Hobday et al., 2019), or out-of-scope uses (Grasso et al., 2020). The TRL framework is not specifically designed with forecasts in mind but can provide helpful scaffolding. The 2021 season would fall around TRL level 7, “demonstration in a relevant environment”, where the forecast is run in an operational mode for a subset of the stakeholder groups. Moving forward, the 2022 season forecasts will transition to TRL level 8, “demonstration in the actual environment”–that is, full access by the stakeholder communities. Because the forecast will be advertised to stakeholders across the industry and management communities with feedback collected, further modifications are expected as the system develops toward TRL level 9, “regular operational use.” Even at this final stage, a forecasting system shouldn’t be considered complete, as feedback and input from stakeholders can continue to improve the system as the environment, the science, and the needs of stakeholders change.
Discussion
Environmental forecasting is often presented as a multi-step iterative cycle, somewhat similar to a TRL framework, where new knowledge is periodically incorporated to improve the system. There are multiple paradigms, and most center a model- or data-driven approach, with stakeholder input coming in later on, and/or placed in a separate stage of the cycle (Dietze et al., 2018, Bauer et al., 2021). An alternative approach centers stakeholder input and knowledge and couples them with the development and refinement of the forecasting system from inception (Jones et al., 2021). Involving stakeholders from inception, and at each stage, can slow the TRL process and publicly expose vulnerabilities in the models or approach. On the other hand, this level of collaboration can build trust, help incorporate local knowledge, and help guide the forecast design to align with the needs and perceptions of users. As the field of Ocean Science becomes more deliberate and thoughtful about modes of engaging and centering human communities (Schreiber et al., 2022, Liboiron et al., 2021), it is worth putting thought into the tradeoffs between these frameworks in the design of ocean forecasting programs. In coastal Maine, where both the environment and the aquaculture industry are changing rapidly, involving stakeholders at each step has so far been a net benefit.
Data Availability Statement
The datasets presented in this study can be found in online repositories. Historical data can be found at the public repository here: https://github.com/BigelowLab/pspforecast. Live data is served at the following link: https://mainedmr.shinyapps.io/bph_phyto/.
Author Contributions
NR led the study and composition of the manuscript. NR, JE, KK, CrB, CC, BL, JM, BT, DM, AT, SA, CW, MM, BH, CaB contributed to the design of the forecasting program and editing of the manuscript. All authors contributed to the article and approved the submitted version.
Funding
Funding was provided by a NOAA MERHAB grant (NA19NOS4780187) to Bigelow Laboratory for Ocean Sciences.
Conflict of Interest
The authors declare that the research was conducted in the absence of any commercial or financial relationships that could be construed as a potential conflict of interest.
Publisher’s Note
All claims expressed in this article are solely those of the authors and do not necessarily represent those of their affiliated organizations, or those of the publisher, the editors and the reviewers. Any product that may be evaluated in this article, or claim that may be made by its manufacturer, is not guaranteed or endorsed by the publisher.
References
Bauer P., Dueben P. D., Hoefler T., Quintino T., Schulthess T. C., Wedi N. P. (2021). The Digital Revolution of Earth-System Science. Nat. Comput. Sci. 1 (2), 104–113. doi: 10.1038/s43588-021-00023-0
Dietze M. C., Fox A., Beck-Johnson L. M., Betancourt J. L., Hooten M. B., Jarnevich C. S. (2018). Iterative Near-Term Ecological Forecasting: Needs, Opportunities, and Challenges. Nat. Comput. Sci. 115 (7), 1424–1432.
Grasso I., Archer S. D., Burnell C., Tupper B., Rauschenberg C., Kanwit K., et al. (2019). The Hunt for Red Tides: Deep Learning Algorithm Forecasts Shellfish Toxicity at Site Scales in Coastal Maine. Ecosphere 10 (12), e02960. doi: 10.1002/ecs2.2960
Grasso I., Russell D., Matthews A., Matthews J., Record N. R. (2020). “Applying Algorithmic Accountability Frameworks With Domain-Specific Codes of Ethics: A Case Study in Ecosystem Forecasting for Shellfish Toxicity in the Gulf of Maine,” in Proceedings of the 2020 ACM-IMS on Foundations of Data Science Conference. 83–91.
Héder M. (2017). From NASA to EU: The Evolution of the TRL Scale in Public Sector Innovation. Innovation J. 22 (2), 1–23.
Hobday A. J., Hartog J. R., Manderson J. P., Mills K. E., Oliver M. J., Pershing A. J., et al. (2019). Ethical Considerations and Unanticipated Consequences Associated With Ecological Forecasting for Marine Resources. ICES J. Mar. Sci. 76 (5), 1244–1256. doi: 10.1093/icesjms/fsy210
Hurst J. W., Yentsch C. M. (1981). Patterns of Intoxication of Shellfish in the Gulf of Maine Coastal Waters. Can. J. Fish. Aquat. Sci. 38 (2), 152–156. doi: 10.1139/f81-020
Jin D., Moore S., Holland D., Anderson L., Lim W. A., Kim D., et al. (2020). 2 Evaluating the Economic Impacts of Harmful Algal Blooms: Issues, Methods, and Examples. PICES Sci. Rep. 59), 5–41.
Jones C. M., Jones S., Petrasova A., Petras V., Gaydos D., Skrip M. M., et al. (2021). Iteratively Forecasting Biological Invasions With PoPS and a Little Help From Our Friends. Front. Ecol. Environ. 19 (7), 411–418. doi: 10.1002/fee.2357
Liboiron M., Zahara A., Hawkins K., Crespo C., de Moura Neves B., Wareham-Hayes V., et al. (2021). Abundance and Types of Plastic Pollution in Surface Waters in the Eastern Arctic (Inuit Nunangat) and the Case for Reconciliation Science. Sci. Total Environ. 782, 146809. doi: 10.1016/j.scitotenv.2021.146809
McGillicuddy D. J., Townsend D. W., He R., Keafer B. A., Kleindinst J. L., Li Y., et al. (2011). Suppression of the 2010 Alexandrium Fundyense Bloom by Changes in Physical, Biological, and Chemical Properties of the Gulf of Maine. Limnol. Oceanog. 56 (6), 2411–2426. doi: 10.4319/lo.2011.56.6.2411
Norström A. V., Cvitanovic C., Löf M. F., West S., Wyborn C., Balvanera P., et al. (2020). Principles for Knowledge Co-Production in Sustainability Research. Nat. sustainability 3 (3), 182–190. doi: 10.1038/s41893-019-0448-2
Pershing A. J., Mills K. E., Dayton A. M., Franklin B. S., Kennedy B. T. (2018). Evidence for Adaptation From the 2016 Marine Heatwave in the Northwest Atlantic Ocean. Oceanography 31 (2), 152–161. doi: 10.5670/oceanog.2018.213
Ralston D. K., Moore S. K. (2020). Modeling Harmful Algal Blooms in a Changing Climate. Harmful Algae 91, 101729. doi: 10.1016/j.hal.2019.101729
Record N. R., Pershing A. J. (2021). “Facing the Forecaster’s Dilemma: Reflexivity in Ocean System Forecasting,” in Oceans 2 (4), 738–751.
Rourke W. A., Murphy C. J., Pitcher G., van de Riet J. M., Burns B. G., Thomas K. M., et al. (2008). Rapid Postcolumn Methodology for Determination of Paralytic Shellfish Toxins in Shellfish Tissue. J. AOAC Int. 91 (3), 589–597. doi: 10.1093/jaoac/91.3.589
Schreiber M. A., Chuenpagdee R., Jentoft S. (2022). Blue Justice and the Co-Production of Hermeneutical Resources for Small-Scale Fisheries. Mar. Policy 137, 104959. doi: 10.1016/j.marpol.2022.104959
Tacon A. G. (2020). Trends in Global Aquaculture and Aquafeed Production: 2000–2017. Rev. Fish. Sci. Aquacult. 28 (1), 43–56. doi: 10.1080/23308249.2019.1649634
U.S. Census Bureau (2010) Percent Urban and Rural in 2010 by State. Available at: https://www.census.gov/programs-surveys/geography/guidance/geo-areas/urban-rural/2010-urban-rural.html.
Keywords: forecast, harmful algal bloom, Maine, neural network, paralytic shellfish toxin, stakeholder
Citation: Record NR, Evanilla J, Kanwit K, Burnell C, Cartisano C, Lewis BJ, MacLeod J, Tupper B, Miller DW, Tracy AT, White C, Moretti M, Hamilton B, Barner C and Archer SD (2022) Benefits and Challenges of a Stakeholder-Driven Shellfish Toxicity Forecast in Coastal Maine. Front. Mar. Sci. 9:923738. doi: 10.3389/fmars.2022.923738
Received: 19 April 2022; Accepted: 24 May 2022;
Published: 14 July 2022.
Edited by:
Wei-Bo Chen, National Science and Technology Center for Disaster Reduction (NCDR), TaiwanReviewed by:
Andrew M. Fischer, University of Tasmania, AustraliaJose Bustillos, Centro de Investigación Biológica del Noroeste (CIBNOR), Mexico
Copyright © 2022 Record, Evanilla, Kanwit, Burnell, Cartisano, Lewis, MacLeod, Tupper, Miller, Tracy, White, Moretti, Hamilton, Barner and Archer. This is an open-access article distributed under the terms of the Creative Commons Attribution License (CC BY). The use, distribution or reproduction in other forums is permitted, provided the original author(s) and the copyright owner(s) are credited and that the original publication in this journal is cited, in accordance with accepted academic practice. No use, distribution or reproduction is permitted which does not comply with these terms.
*Correspondence: Nicholas R. Record, bnJlY29yZEBiaWdlbG93Lm9yZw==