- 1Programa de Postgrados en Oceanografía, Universidad de Concepción, Concepción, Chile
- 2Instituto Milenio de Oceanografía, Concepción, Chile
- 3Departamento de Zoología, Facultad de Ciencias Naturales y Oceanográficas, Universidad de Concepción, Concepción, Chile
- 4College of Earth, Ocean, and Atmospheric Sciences, Oregon State University, Corvallis, Oregon, OR, United States
- 5Departamento de Física, Facultad de Ciencias, Universidad del Bío-Bío, Concepción, Chile
- 6Instituto Milenio en Socio-Ecología Costera (SECOS), Santiago, Chile
- 7Centro de Investigación Oceanográfica COPAS COASTAL, Universidad de Concepción, Concepción, Chile
- 8Departamento de Oceanografía y Medio Ambient, Instituto de Fomento Pesquero, Valparaíso, Chile
Euphausiids (hereafter “krill”) are one of the main components of the pelagic communities of the Humboldt Current System (HCS). Their community dynamics have been well studied in central-southern Chile where upwelling is strongly seasonal, but little is known about the permanent-upwelling area of the HCS, which yields the largest fishery in the world, the Peruvian anchovy. We applied hierarchical generalized additive models with environmental and biological predictors to determine the main drivers of krill abundance, adjusting species-specific functions. We used a time series of 16 bi-annual surveys to study annual, seasonal, and spatial scales of variability of the four numerically dominant taxa: Euphausia mucronata (Humboldt krill), E. eximia, Stylocheiron affine, and Nematoscelis spp. The spatial pattern of the Humboldt krill (the dominant species) proved it is an upwelling-associated species, with higher abundances within 10 km from the coast. The other 3 taxa showed opposite spatial patterns with higher abundances offshore. The main covariates explaining krill abundances were the depth of the upper limit of the oxygen minimum zone (dOMZ) and the mean temperature of the water column. Humboldt krill was negatively correlated to both drivers, and the opposite effect was observed for the other taxa. Although many krill species are metabolically adapted to cope with the severe hypoxic conditions of this system, the Humboldt krill was the only species with higher modeled abundances when dOMZ was shallower. Chlorophyll-a remained high during all sampling periods, and it was an insignificant predictor for all taxa, suggesting food is not a limitation for krill in this highly productive system. The acoustic biomass of the Peruvian anchovy had a negative non-linear effect on the abundances of the Humboldt krill, and higher Humboldt krill abundances were found in areas with no anchovy hotspots. Our results indicate that krill in this system are susceptible to changes in temperature, oxygen, and upwelling conditions. Extreme events (e.g. heatwaves and ENSO events) are expected to increase in frequency and intensity, while climate change scenarios show a potential intensification of upwelling. These conditions could lead to distribution displacements and alter trophic interactions by modifying the distribution and biomass of the predator.
Introduction
The Humboldt Current System (HCS) is the most productive eastern boundary upwelling system (EBUS) regarding fish productivity (Montecino and Lange, 2009). Here, coastal upwelling, driven by equatorward winds, sustains high levels of primary productivity that ultimately support abundant zooplankton and pelagic communities (Medellín-Mora et al., 2016; Espinoza et al., 2017). As in other cold and temperate ecosystems, krill are an essential link in the food webs of the HCS, transferring energy from phytoplankton and microzooplankton to higher trophic levels (Espinoza et al., 2017; Massing et al., 2022). Their large size in comparison to other mesozooplankton groups, and their ability to form large and dense swarms, make them one of the most diversely predated animals in upwelling systems (Pillar et al., 1992; Antezana, 2010), being a critical prey item for many marine mammals, seabirds, and fishes of the HCS. The recruitment and standing stocks of some of the most important biological resources in the eastern South Pacific, such as the Peruvian anchovy, the most globally productive fishery (FAO, 2020), the south Pacific hake, and jack mackerel, rely on krill populations (Espinoza and Bertrand, 2008; Antezana, 2010). Hence, understanding all sources of temporal and spatial variability influencing krill dynamics is critical to improving ecosystem and fisheries management.
Krill communities of the HCS are strongly influenced by the hydrographic structure and advective processes associated with upwelling dynamics. The cross-shore distribution of krill life stages in northern Chile suggests that, during spring months, early stages are advected offshore through the Ekman layer (Díaz-Astudillo et al., 2022). Similar observations have been made in central Chile, where upwelling is highly seasonal, and smaller individuals are usually found farther offshore (Riquelme-Bugueño et al., 2012). The cross-shore gradients in temperature, dissolved oxygen concentration, and chlorophyll-a modulate the community structure and distribution of the upwelling-associated, transition, and oceanic krill ensembles off central Chile (Riquelme-Bugueño et al., 2012). In contrast, the upwelling is permanent year-round off northern Chile with the presence of a quasi-permanent oxygen minimum zone (OMZ) that can act as a biological barrier for organisms (Manríquez et al., 2009; Escribano et al., 2009). Highly migratory krill can cross or even inhabit the OMZ, while others are restricted to oxygenated waters (Escribano et al., 2009; Riquelme-Bugueño et al., 2020). Despite being one of the most important trophic links, relatively low efforts have focused on the effect of other environmental and biological forcings (e.g., climate oscillations and predator abundance) on krill population dynamics in the HCS, compared to other highly productive systems.
In addition to mesoscale variability, basin-scale low-frequency physical processes, such as marine heat waves and climate events, also influence the distribution of species by altering the environmental conditions of the water column and circulation patterns, which causes changes in krill body length and biomass (Brinton and Townsend, 2003; Sydeman et al., 2013; Robertson and Bjorstedt, 2020; Killeen et al., 2022). In the California Current System (CCS), the onshore advection of warmer water masses during El Niño events causes changes in the distribution of tropical, subtropical, and subarctic euphausiid species, decreasing the overall contribution of krill to total zooplankton carbon biomass (Ambriz-Arreola et al., 2018). Warm events can also modify the phenology and intensity of primary productivity peaks (Thomas et al., 2009). Consequently, the abundance of cold-water species decreases, and reproductive processes are delayed and/or altered (Díaz-Astudillo et al., 2022; Killeen et al., 2022). In the HCS, climate events affect net primary productivity and zooplankton communities by modifying species composition and increasing species diversity (Aronés et al., 2009; Thomas et al., 2009; Escribano et al., 2012). These changes in community traits, caused by environmental anomalies, escalate through the trophic web ultimately modifying ecological prey-predator interactions (Orlova et al., 2015).
Modeling the relationships between krill and their predators has been a common approach to studying the complex and non-linear effect of predation on krill populations, while incorporating environmental variability. In the Eastern Bering Sea, krill are a main component of the ecosystem and a key prey for several trophic levels, including many commercial fishes, such as cod, capelin, and the walleye pollock (Hunt et al., 2016). Several studies have shown a moderate effect, though statistically significant, of the density of these fishes on krill abundance, suggesting the occurrence, to different degrees, of top-down control of krill populations (Hunt and McKinnell, 2006; Hunt et al., 2016; Simonsen et al., 2016). Similar observations have been made in the Barents Sea (Orlova et al., 2015), where fish predation pressure on zooplankton has been well studied (Frank et al., 2007). The extent and intensity of trophic interactions depend on local and remote environmental proxies (Orlova et al., 2015); thus, they vary in space and time. Krill also dominates the zooplankton community in the CCS, being associated with the distribution of predators such as whales (Rockwood et al., 2020) and seabirds (Santora et al., 2014). Long-term temporal correlations between euphausiid dominance and anchovy biomass (Ayón et al., 2011), and small-scale spatial correlations with jack mackerel (Bertrand et al., 2004), both in the HCS, hint that some extent of trophic control between krill and pelagic fish might occur in the HCS. Although krill is one of the most abundant zooplankton groups in the HCS, regression models determining the effect of predation on krill abundance in this highly productive region have not been applied. This study aims to identify the main environmental drivers of krill abundance at interannual, seasonal and spatial scales, and to determine the effect of predator biomass (the Peruvian anchovy Engraulis ringens, hereafter anchovy) on the abundance of krill in the permanent-upwelling area off northern Chile.
Methods
Study area and sampling design
The study area comprised the region off northern Chile from 20°S to 25°S and 70°W to 72°W (Figure 1). The area presents a generally narrow continental shelf, with no river discharges. Along-shore winds are dominant and sustain intense upwelling year-round, which produces a very shallow oxygen minimum zone (OMZ) and chlorophyll-a filaments that extend several kilometers offshore (for a more detailed description of the study area please refer to Díaz-Astudillo et al., 2022). The in situ data used in this study (i.e., euphausiid abundance, anchovy biomass and CTD-O (conductivity, temperature, depth and, oxygen) data) were collected during 16 research cruises, conducted every austral fall (8 in April each year) and austral spring (8 in December each year) from 2010 to 2017 onboard the R/V Abate Molina by the Instituto de Fomento Pesquero (IFOP).
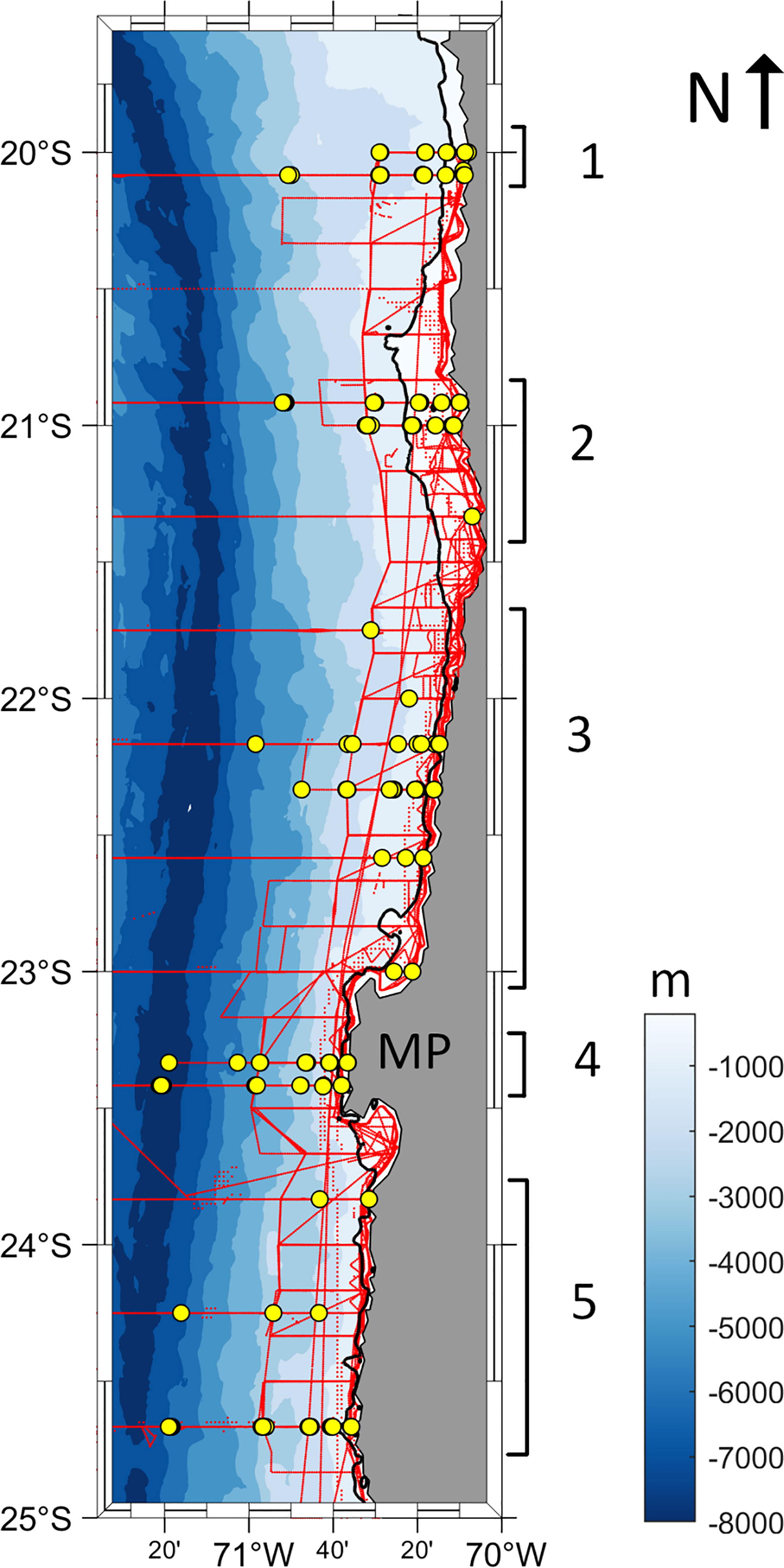
Figure 1 Map of the study area showing the bathymetry (color coded) and 200 m isobath as a black line. Yellow dots represent the zooplankton and CTD-O sampling stations. Red dots (seen as red lines because of the high spatial resolution of the data) represent the anchovy acoustic sampling records. Samples were grouped in 5 latitudinal groups (numbers 1-5, defined in the text).
The navigation track of the ship during the cruises was composed of parallel along-shore transects each separated by 10 nm, in which in situ biological and physical sampling was conducted. The sampling stations were positioned at 1, 5, 10, 20, and 40 nm from the coast along each transect (the 40 nm station was available only during the fall cruises) by categorizing the stations into 5 latitudinal groups according to topographic and bathymetric features. Using this categorization, we incorporate the variability in the continental shelf’s width and the coastline’s shape, which can influence krill distribution and trophic interactions (Ayón et al., 2011; Santora et al., 2017). Group 1 includes the stations north of 21°S where the continental shelf is narrow; Group 2, the stations at ~21°S, where the continental shelf is wider; Group 3, the stations north of Mejillones Peninsula (MP), a major upwelling point at 23.3°S, with a narrow continental shelf; Group 4, the stations near MP; and Group 5, the stations south of MP (Figure 1).
Environmental data
Temperature, salinity, and dissolved oxygen data were collected using a SeaBird 911 CTD-O. Sampling stations were classified according to their distance from the coast (‘DC’, 5 levels, 1, 5, 10, 20 and 40 nm), latitudinal group (‘LG’, 5 groups), season (fall and spring), and year (8 levels, from 2010-2017). A total of 240 samples were used in this study.
Daily satellite surface chlorophyll-a concentration (SSC, 4x4 km resolution) data were obtained from the merged Copernicus Global Ocean Colour products (https://resources.marine.copernicus.eu/). Daily satellite surface temperature (SST) (0.025°x0.025° resolution) data were obtained from MODIS-Aqua (https://oceancolor.gsfc.nasa.gov/), and daily satellite winds (0.25°x0.25° resolution) from CMEMS IFREMER CERSAT multi-sensor blended wind field (https://resources.marine.copernicus.eu/). Ekman transport vectors for each sampling period, and the zonal Ekman transport (ET, m2 s-1) of the previous 5 days of each sampling date, were calculated using satellite winds.
Krill data
At each station, nighttime zooplankton sampling was performed with a 60 cm mouth-diameter double-framed Bongo net with 300 µm mesh. The Bongo net was equipped with a calibrated T.S.K. flowmeter to measure the volume of water filtered. The tows were oblique and integrated from 100 m to the surface, and samples were immediately fixed onboard with 5% borax-buffered formaldehyde. In the laboratory, adult krill were sorted, counted, identified to the lowest taxonomic level possible following Baker et al. (1990), and standardized to individuals per 1000 cubic meters (ind. 1000 m-3). Due to difficulties identifying the genus Nematoscelis (~50% of all individuals had missing or broken appendages), the genus species were pooled together. Because only 4 taxa accumulated 96% of the total abundance, only these were included in subsequent analyses: Euphausia mucronata (Humboldt krill), E. eximia, Stylocheiron affine, and Nematoscelis spp.
Anchovy data
Peruvian anchovy acoustic biomass data were continuously recorded during the navigation track using a SIMRAD EK 60 multi-frequency echo sounder operating at 18, 38, 120 and 200 kHz. Acoustic data were georeferenced with a GPS and discretized in 0.5 nm sampling intervals. Fishing catches were performed at several random stations to validate acoustic categorizations. Anchovy target strength estimations were carried out when >80% of the catches corresponded to anchovy and were adjusted to the observed size structure and modal sizes. Anchovy identification was then made by visually examining the echograms and the species-specific mean volume backscattering strength (SV in dB). Acoustic data were processed using Echoview software (Sonardata, Pty. Ltd.). The Nautical Area Scattering Coefficient (NASC, m2 nmi-2), an acoustic proxy for anchovy biomass, was determined by:
where k = conversion factor from m to nm, z1/z2 = lower/upper limit of the echo-integrated stratum, SV = mean volume backscattering strength (dB), Δz = stratum depth (MacLennan et al., 2002). Specific details about acoustic data processing and fishing methods can be found in Leiva et al. (2018). This standard methodology has been previously used with anchovy data by Murase et al. (2009) and Gutiérrez et al. (2007).
Data analysis
Environmental and biological analysis
We constructed climatologies (April and December) of SST, SSC, and Ekman transport to characterize the study area and the study period, and to obtain indices for each sampling date and position. We used 1 mg m-3 in the satellite chlorophyll-a composites as a limit to visualize upwelling extension, Letelier et al. (2009), who found that the upwelling front usually coincided with the 1 mg m-3 boundary off central Chile. In situ CTD-O data were used to calculate the mean temperature (MeanTemp), salinity (MeanSal), and dissolved oxygen (MeanDO) of the first 100 m of the water column, and the depth of the upper limit of the OMZ (<1.4 m L-1, dOMZ). We calculated mean values for each station, and then the mean for every LG and DC group, for every cruise, to explore across- and along-shore spatial variability through years and seasons. Interannual, seasonal, latitudinal, and longitudinal (distance from the coast) comparisons of the environmental variables were carried out using univariate Kruskal Wallis tests.
To explore spatial and temporal differences in the community, non-metric permutational analysis of variance (PERMANOVAs with Bray-Curtis similarity matrix and 9999 permutations) was performed with log(X+1)-transformed abundances. The effects of the year (2010-2017), season (fall and spring), DC, LG, and the interaction between year and season, and DC and LG (two-way analysis), were tested. The contribution percentage to total abundance was calculated to observe changes in species proportions through time.
Spatial hotspots of krill and anchovy aggregations were identified using the Getis-Ord statistic (Gi*, Getis and Ord, 1992). This index, commonly used to identify and describe krill aggregations (Santora et al., 2017; Rockwood et al., 2020), identifies statistically significant spatial clusters of an entity (in this study log(Abundance+1) and log(NASC +1)). The local sum for a feature and its neighborhood is proportionally compared with the sum of all features, to determine whether the local sum is significantly different from that randomly expected using the Z score statistic. Significant values of Z>0 provide evidence for hotspots, while values of Z<0 provide evidence for groups of features that are lower than expected by chance (i.e., ‘coldspots’). The analysis resolution was ~100 km² considering equal-sized hexagons. The Gi* statistic was mapped to identify significant spatial patterns in krill and anchovy distribution, and their potential coupling. Hotspot analyses were performed in the ArcGIS 10.5 software (ESRI, 2019).
Modeling euphausiid abundance in the HCS
To explore the physical and biological mechanisms controlling euphausiid abundance, we applied Hierarchical Generalized Additive Models (HGAMs). As a form of Generalized Additive Models (GAMs), HGAMs allow the construction of flexible models that fit non-linear responses using smooth curves (Pedersen et al., 2019). By being “hierarchical”, HGAMs can account for intergroup variability when data are grouped into categories (e.g., species or taxa). Hence, they are suitable when the relationship between the response and explanatory variables is expected to vary across groups (Pedersen et al., 2019). We modeled the response of krill [log(Abundance+1)] to 7 predictors that account for spatial variability (latitude and longitude), environmental variability (MeanTemp, MeanSal, dOMZ, SSC and ET), and predator biomass [log(NASC +1)]. The spatial term was constructed with the geographic position of each sample in order to obtain the modeled distribution of each taxon in a continuous spatial gradient. Abundance variables were log-transformed to approach a normal distribution of the residuals. The hydrographic variables were selected to be incorporated in the analysis after evaluating multicollinearity by calculating variance inflation factors (VIF) and exploring correlograms using the ‘AlleleShift’ R package (Kindt, 2021). The original pool of variables was composed of in situ surface temperature, salinity and dissolved oxygen (first 20 m of the water column), MeanTemp, MeanSal, MeanDO, dOMZ, SST, SSC, and the zonal (cross-shore) Ekman transport (ET). The variables with VIF<7 and Spearman correlation coefficient less than 0.7 were selected as predictor variables in the models (Table 1).
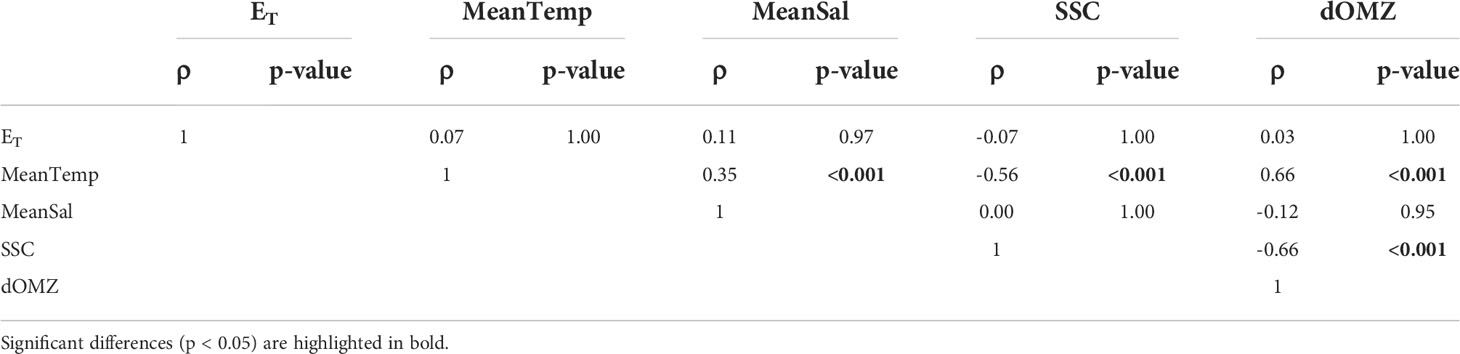
Table 1 Spearman correlations among the environmental variables selected as covariates for the HGAM models.
The response variable was categorized according to taxa to observe specific responses of E. mucronata, E. eximia, S. affine, and Nematoscelis spp. After careful evaluating the model outcomes and fittings and taking into consideration the contrasting distribution and ecological role of the selected taxa (Riquelme-Bugueño et al., 2012; Massing et al., 2022), we decided not to incorporate a global smoother in the model. By this, the specific response curves are not penalized if they deviate from the global smoother. The general model structure is:
Where Y is the response value to be modeled, g-1 is the inverse link function, β0 is the intercept, f(xi) is the smooth function of the covariates, ζ is the random effect of the group-specific intercepts, and ϵ is the error term. The spatial term was constructed as a Gaussian process smooth to deal with spatial autocorrelation.(i.e., the interaction between the latitudinal and longitudinal position of each sample is a spherical correlation model with a maximum range of 1) (Wood, 2017). This term was included to incorporate any spatial trend that is unrelated to the predictors (Ressler et al., 2014). Several model structures and designs were tested, and the model with the lowest Akaike Information Criterion (AIC), highest deviance explained, and all terms being statistically significant for at least one taxon was selected as the best. Smoothing parameters and model coefficients were estimated using restricted maximum likelihood (REML). Because anchovy data were unavailable for the fall cruise of 2010, 2011 and 2013, these periods were not included in the models, hence the number of observations used for the model was 189 (by using 4 taxa the pseudo-n is 756). HGAMs were constructed in R (R Core Team, 2021) using the ‘mgcv’ package (Wood, 2017) and plots with the ‘gratia’ package (Simpson, 2022).
Results
Temporal and spatial changes in environmental conditions
The region presented high environmental variability at interannual, seasonal, and spatial scales. The fall of 2015 was the warmer period (21.96 ± 0.3°C), followed by the spring of 2016 (21.74 ± 0.25°C), both periods coinciding with the highest values of the Multivariate El Niño Index for the study period (see Díaz-Astudillo et al., 2022). In contrast, the coldest periods were the spring of 2010 (20.02 ± 0.26°C) and 2014 (20.06 ± 0.33°C). Large SST anomalies were found during the fall of 2010, 2012 and 2015 (+0.63°C, +1.12°C and +1.11°C, respectively), and the spring of 2010 and 2014 (-0.83°C and -0.69°C, respectively) (Figure 2). During fall of 2010 and spring of 2015, positive anomalies of ~2°C were observed in a coastal band of ~40 km, while positive anomalies affected the whole study area during the fall of 2012 and the spring of 2015. The cross-shore Ekman transport remained negative during the whole study period (Figure 2). The fall of 2010 was characterized by the highest ET values (-0.6 m2 s-1), followed by the fall of 2016 (-0.52 m2 s-1), while the spring of 2011 and 2013 were the periods with the lowest values (-0.3 and -0.28 m2 s-1, respectively). In general, ET was less intense near the coast (-0.33 ± 0.4 m2 s-1 at 1 nm) and increased in intensity offshore (-0.44 ± 0.43 m2 s-1 at 40 nm), although differences were unsignificant (H=5.77, p=0.22). ET was significantly lower (higher in intensity) during fall (-0.44 ± 0.03 m2 s-1) than spring (-0.38 ± 0.02 m2 s-1) (H=51.79, p<0.001).
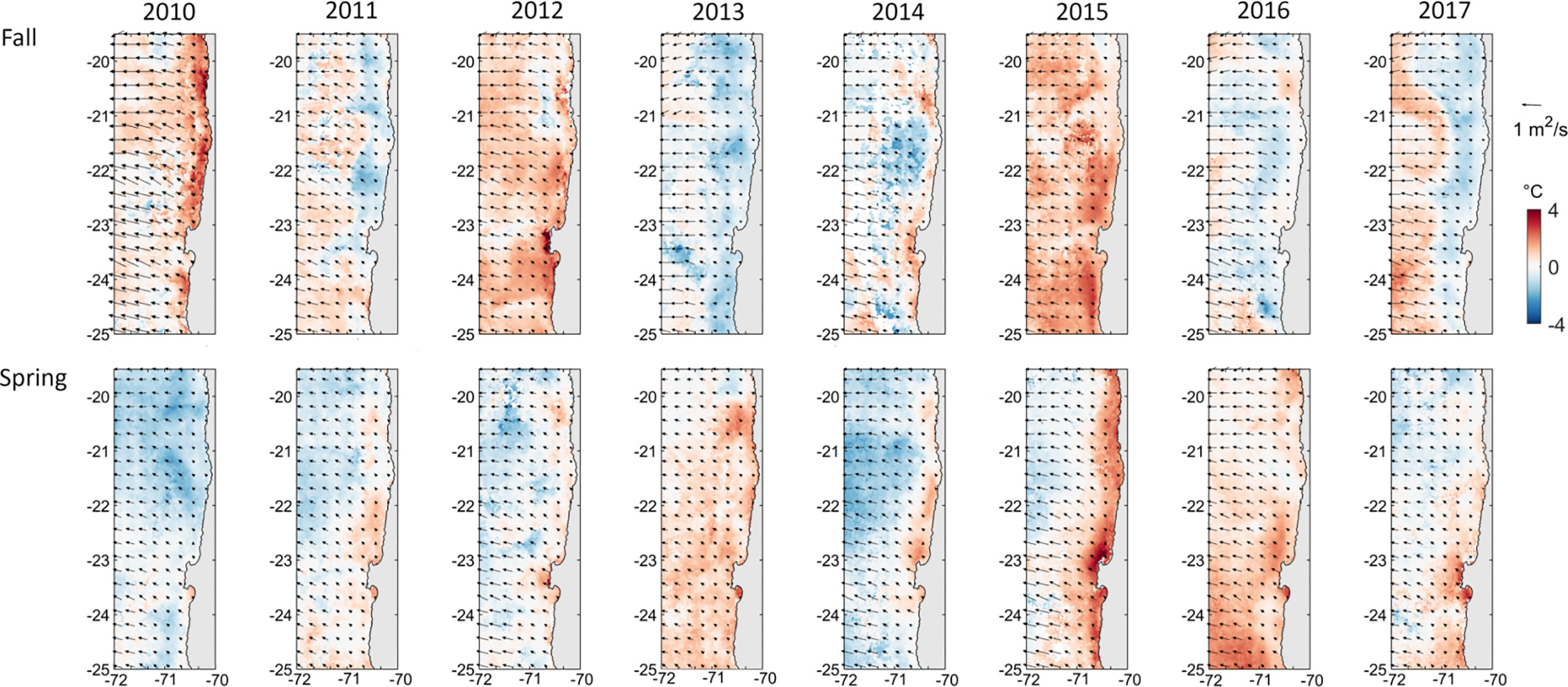
Figure 2 Monthly SST anomalies (°C) of every April (representative of fall, upper panels) and December (representative of spring, lower panels). The vectors correspond to the mean Ekman transport vectors (m2 s-1).
The presence of a coastal band with satellite chlorophyll-a concentration of >1 and up to 16 mg m-3 during all the sampling periods (Figure 3), in addition to the negative ET, confirms the average pattern of permanent upwelling in this area. The offshore extension of chlorophyll-a filaments suggests high mesoscale activity. Mean SSC was higher near the coast (5.87± mg m-3 at the 1 nm stations) and decreased offshore (0.43± mg m-3 at the 40 nm stations), with significant differences in chlorophyll-a concentration among the stations at 1-5 nm, 10 nm, and 20-40 nm (H=109.7, p<0.001). The coastal band with values >1 mg m-3 was usually wider near 21°S, where the continental shelf gets wider, and north of the Mejillones Peninsula (LG 2 and 3), exhibiting significant latitudinal differences in SSC (H=15.9, p=0.003). There were no significant interannual differences in SSC (H=7.19, p=0.41), but the upwelling band was wider between 2010-2013 and 2017 than in 2014-2016. Fall of 2011 and 2017 were the periods with higher mean SSC (of 0.98 ± 0.91 and 1.25 ± 0.59 mg m-3, respectively), which agreed with negative temperature anomalies near the coast (Figure 2). In contrast, the fall of 2014 was the period with the lowest mean SSC (0.5 ± 0.91 mg m-3). There were no seasonal differences in chlorophyll-a concentration (H=0.83, p=0.42).
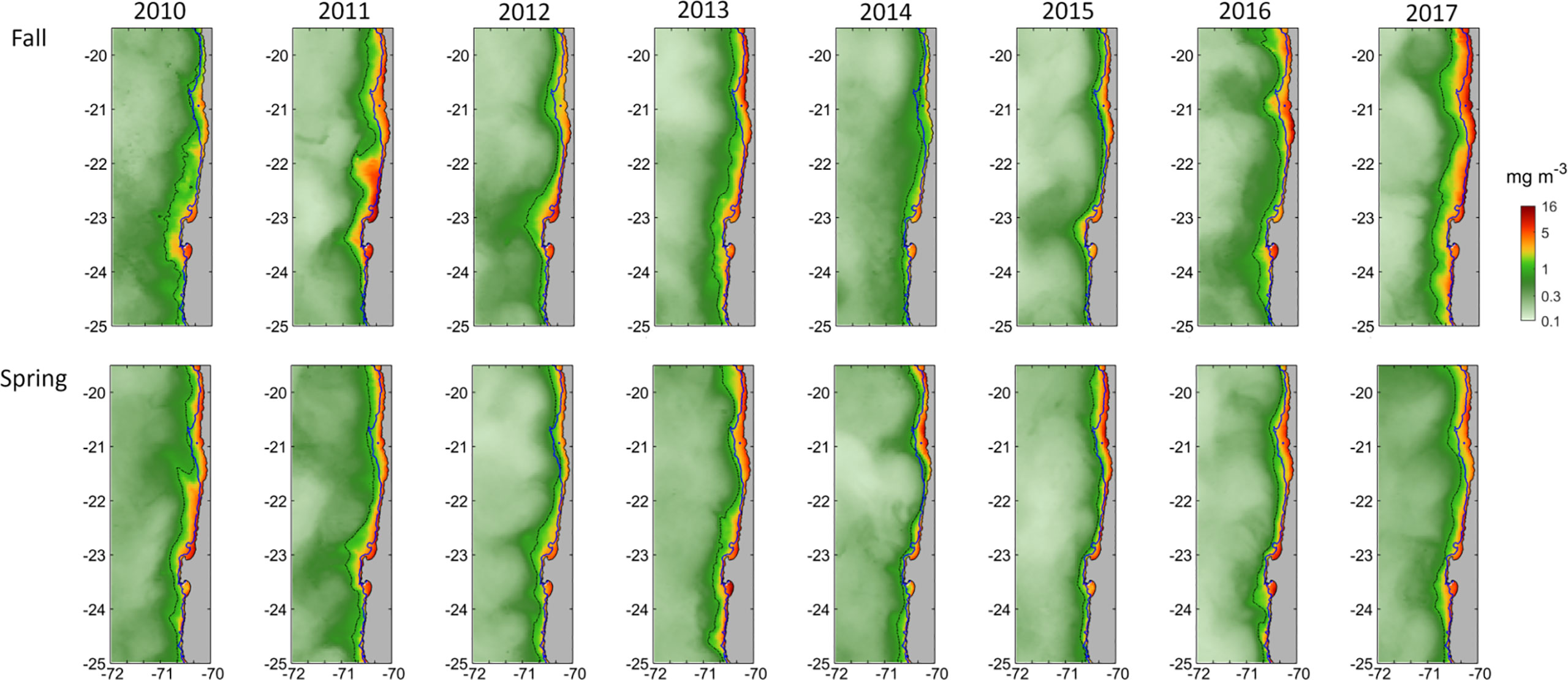
Figure 3 Monthly mean satellite chlorophyll-a (mg m-3) of every April (representative of fall, upper panels) and December (representative of spring, lower panels). The black dotted line indicates the 1 mg m-3 isoline, whereas the blue line indicates the 200 m isobath.
The in situ environmental data confirmed the upwelling pattern observed with satellite chlorophyll-a and temperature data. MeanTemp, MeanDO, and dOMZ were significantly lower near the coast (14.5 ± 0.9°C, 1.9 ± 0.8 ml L-1 and 24 ± 12 m at 1 nm, respectively) than in offshore stations (17.7 ± 1.6°C, 4.09 ± 1.17 ml L-1 and 73 ± 42 m at 40 nm, respectively) (H=102.4, p<0.001, H=88.1, p<0.001, and H=217, p<0.001, respectively). MeanSal tended to decrease toward the coast, although cross-shore differences were not statistically significant (H=5, p=0.29).During the study period, all DC and LG groups observed an increase in MeanTemp and MeanSal from 2014 to the spring of 2016. MeanDO increased in all DC groups during 2011-2012 and in 2014-2016. Similarly, dOMZ showed an increase in the same periods that was more noticeable in 2014-2015, from 10-40 nm and in LG 3-5. In the latitudinal range, only MeanSal presented a distinctive pattern of higher salinity towards the north, with significantly higher salinity in LG 1 (34.91 ± 0.11) and 2, versus LG 5 (34.68 ± 0.12) (H=65.6, p<0.001) (Figure 4).
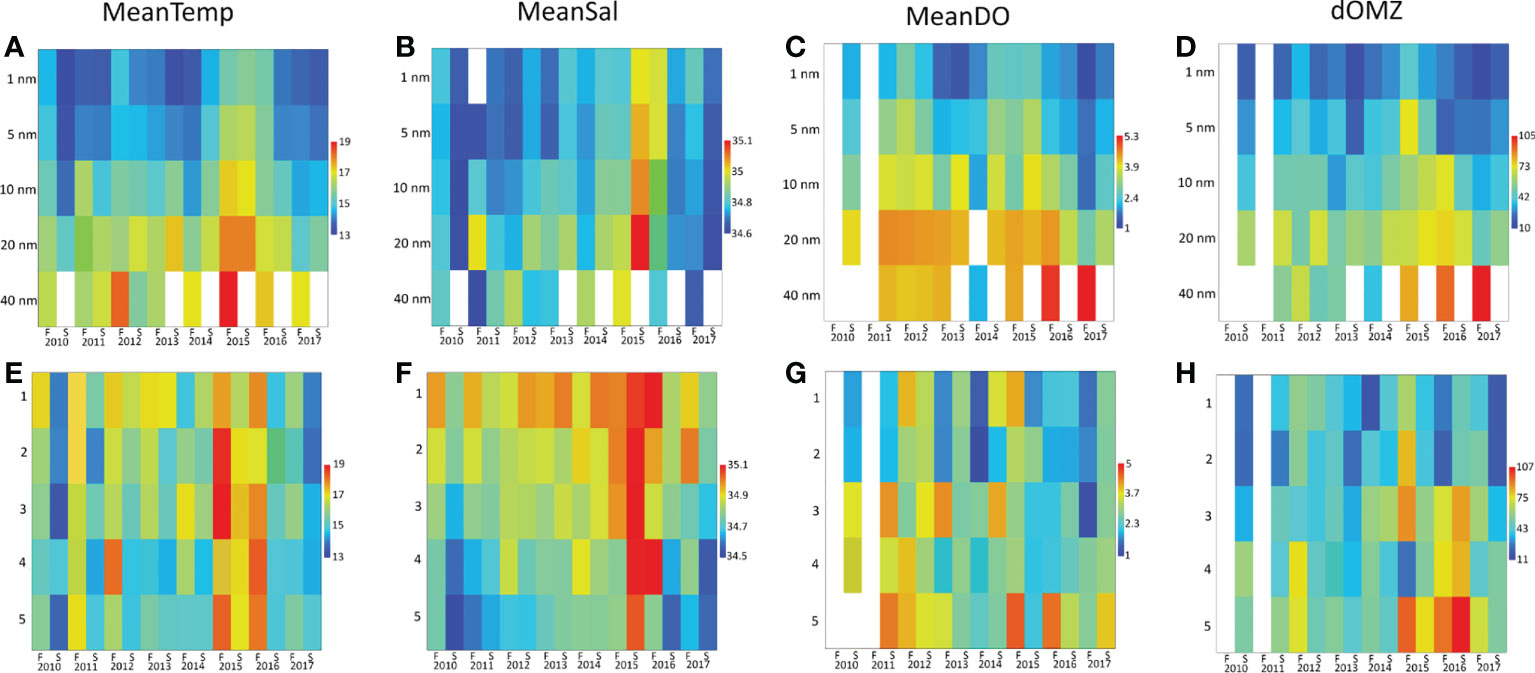
Figure 4 Heatmaps of CTD-O derived variables across sampling periods and as a function of distance from the coast (A–D) and latitudinal group (E–H). F, fall survey; S, spring survey.
Euphausiid abundance variability by taxa
Out of the 4 taxa that dominated the community, Humboldt krill was the most abundant (1222 ± 2312 ind. 1000 m-3) and accounted for 73% of the total abundance, followed by E. eximia (15%, 236 ± 631 ind. 1000 m-3), S. affine (9%, 181 ± 487 ind. 1000 m-3) and Nematoscelis spp. (3%, 46 ± 109 ind. 1000 m-3). Taxa contributions to total abundance showed large fluctuations among sampling periods (Figure 5). E. mucronata accounted for 17% (spring of 2012) to 96% (fall of 2014) of the total abundance, while the highest contribution of E. eximia (68%), S. affine (39%), and Nematoscelis spp. (8%) was observed during the fall of 2011, spring of 2011 and fall of 2012, respectively. E. mucronata was negatively correlated to the abundance of S. affine (ρ=-0.31, p<0.001) and Nematoscelis spp. (ρ=-0.18, p=0.03), while E. eximia was positively related to the same taxa (ρ=0.33, p<0.001 and ρ=0.58, p<0.001, respectively).
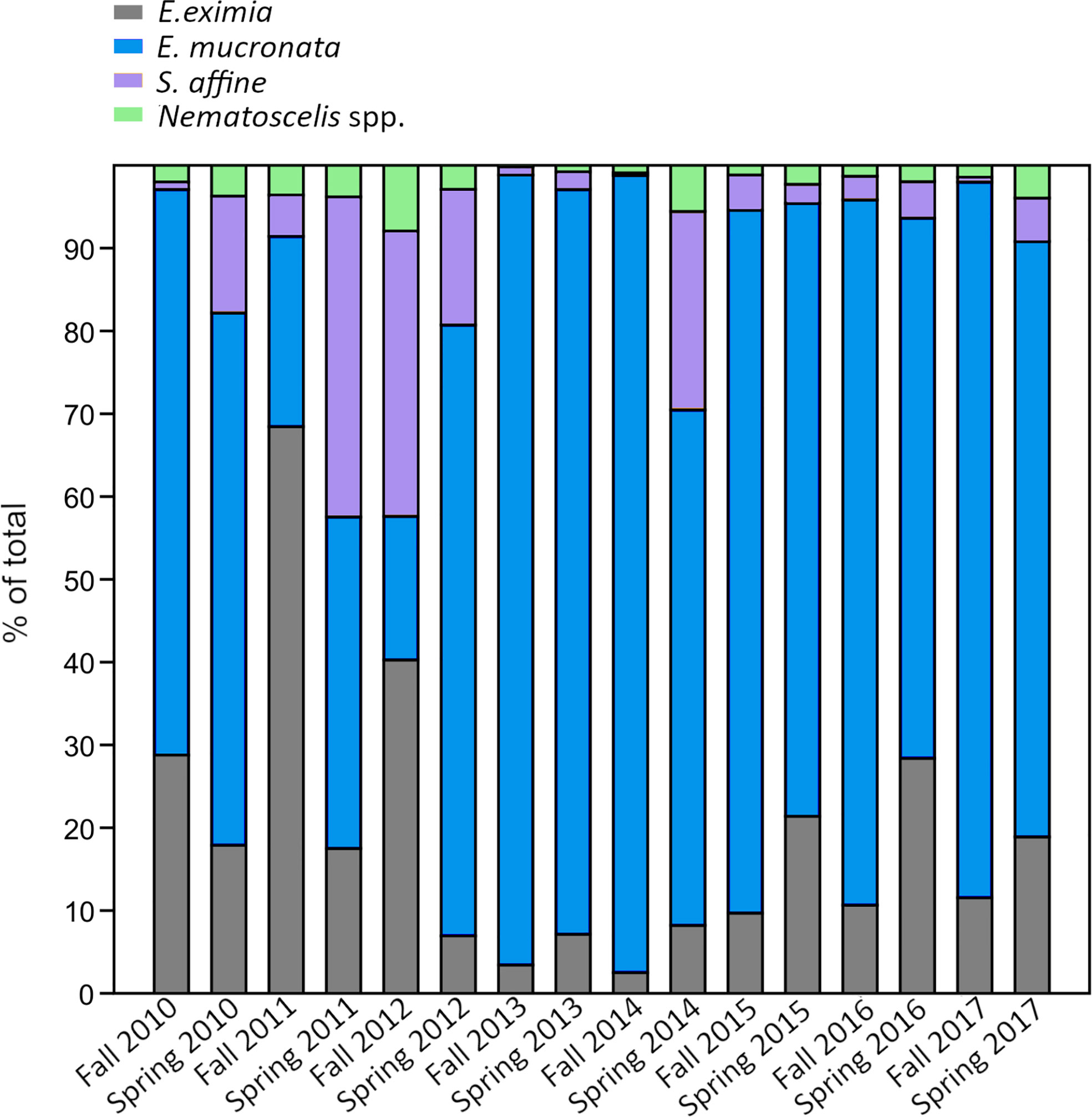
Figure 5 Percentage of contribution of each euphausiid taxon to total abundance, for each sampling period.
Significant interannual differences (Pseudo-F=1.59, p=0.002) were found in all taxa (Figure 6; Table 2). The years with positive temperature anomalies, such as 2012 and 2015, had lower mean abundances than years with negative anomalies, such as 2014 and 2017. The abundances caused the highest differences in the contrasting consecutive years of 2014 and 2015. The mean abundances of E. mucronata, S. affine, and Nematoscelis spp. decreased significantly by one order of magnitude in 2015 (771 ± 1841, 31 ± 56 and 17 ± 40 ind. 1000 m-3, respectively) compared to 2014 (1531 ± 2149, 435 ± 741 and 105 ± 219 ind. 1000 m-3, respectively). At a seasonal scale, spring abundances of E. eximia, S. affine and Nematoscelis spp. were higher than fall abundances in all taxa (Pseudo-F=4.39, p<0.001), with the highest differences observed for S. affine (269 ± 598 and 38 ± 97 ind. 1000 m-3, in spring and fall respectively). E. mucronata did not show a seasonal abundance pattern. We did not find a significant effect of the interaction between year and season on krill abundances (Pseudo-F=-2.93, p=0.33).
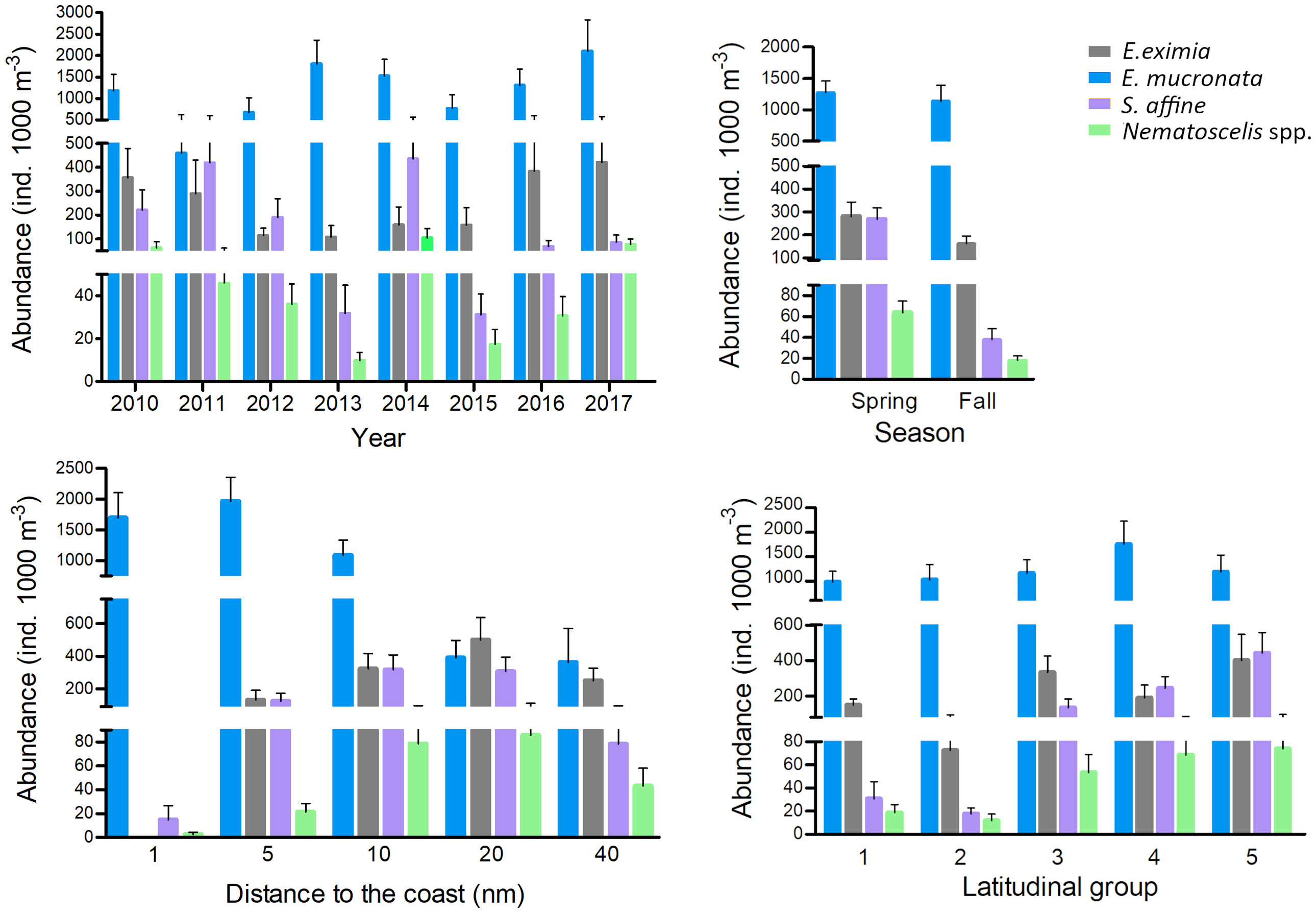
Figure 6 Interannual, seasonal, longitudinal (distance from the coast) and latitudinal (latitudinal groups as defined in the Methods section) variability in mean abundances (ind. 1000 m-3) and standard error, of the 4 most abundant taxa.
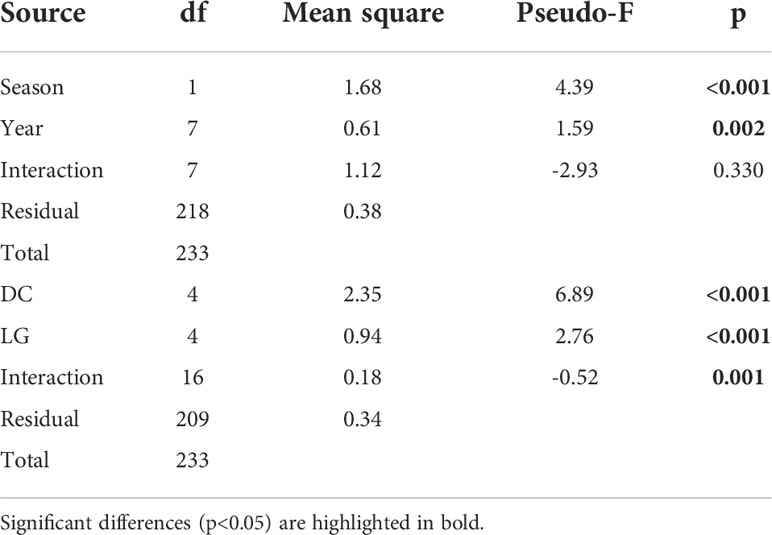
Table 2 PERMANOVA results for the 4 krill taxa, considering season, year, distance from the coast (DC) and latitudinal group (LG) as potential structuring factors.
The cross-shore variability in krill abundance showed significant differences in relation to the distance from the coast (H=6.89, p<0.001), and contrasting patterns were found according to the taxa (Figure 6). Humboldt krill, the only species with higher abundances nearshore, had the highest mean abundances at 1 and 5 nm from the coast (1697 ± 3033 and 1968 ± 2825 ind. 1000 m-3 respectively) and the lowest at 40 nm (363 ± 906 ind. 1000 m-3). E. eximia exhibited the opposite pattern, with the lowest mean abundances at 1 nm (0 ind. 1000 m-3 respectively), and the highest at 20 and 40 nm from the coast (501 ± 964 and 250 ± 209 ind. 1000 m-3 respectively), evidencing their offshore habitat preferences. Both S. affine and Nematoscelis spp. showed the highest abundances at 10 and 20 nm (317 ± 690 and 308 ± 602 ind. 1000 m-3 respectively for S. affine, and 78 ± 125 and 89 ± 168 ind. 1000 m-3 for Nematoscelis spp.), and the lowest at 1 nm (15 ± 88 and 3 ± 11 ind. 1000 m-3 respectively), suggesting a tendency to inhabit the upwelling front.
Latitude also significantly affected the abundance of krill (Pseudo-F=2.76, p<0.001). The general pattern showed a decrease in the abundances to the north (Figure 6). For E. eximia, S. affine and Nematoscelis spp., Group 2 (area with the widest continental shelf) was the one with lower abundances (73 ± 149, 18 ± 35, and 12 ± 38 ind. 1000 m-3 respectively), indicating there could be a negative effect of the width of the continental shelf on krill abundances. Their highest mean abundances were observed in LG 5 (400 ± 1053, 441 ± 833 and 74 ± 163 ind. 1000 m-3, respectively). Humboldt krill had the lowest abundances in Group 1 (977 ± 1446 ind. 1000 m-3) and the highest in Group 4, which corresponds to the Mejillones Peninsula upwelling center (1754 ± 3199 ind. 1000 m-3). The interaction between DCxLG was also significant (Pseudo-F=-0.52, p=0.001), and it was mainly driven by the high abundances of Humboldt krill in the coastal stations in LG 4 and 5.
Model results: environmental drivers of euphausiid abundance
We ran several models with different combinations of the original 7 predictor variables derived from satellite, in situ, and survey data. The best model explained 55.3% of the deviance and included 5 covariates: the spatial term, the mean temperature of the upper 100 m of the water column, cross-shore Ekman transport, the depth of the upper limit of the OMZ, and anchovy acoustic biomass (Table 3). The contribution of salinity and satellite chlorophyll-a to the overall explained variance was negligible in every combination, and not significant in the full model, so they were dropped in the construction of the final model. Grouping by species revealed contrasting responses among the 4 taxa (Figure 7). The spatial term (a smooth Gaussian process between longitude and latitude), MeanTemp, and dOMZ were the only terms that were significant for all taxa when being the only smooth function in the model. The spatial term explained 39.7% of the deviance, while the depth of the OMZ explained 42.4%, and temperature, 27.9% (in single-term models) (Table 3). In contrast, ET and anchovy acoustic biomass were only significant for S. affine and Humboldt krill, respectively. biomass. In the final model all terms were significant for at least 1 taxon, and at the same time, AIC was the lowest.
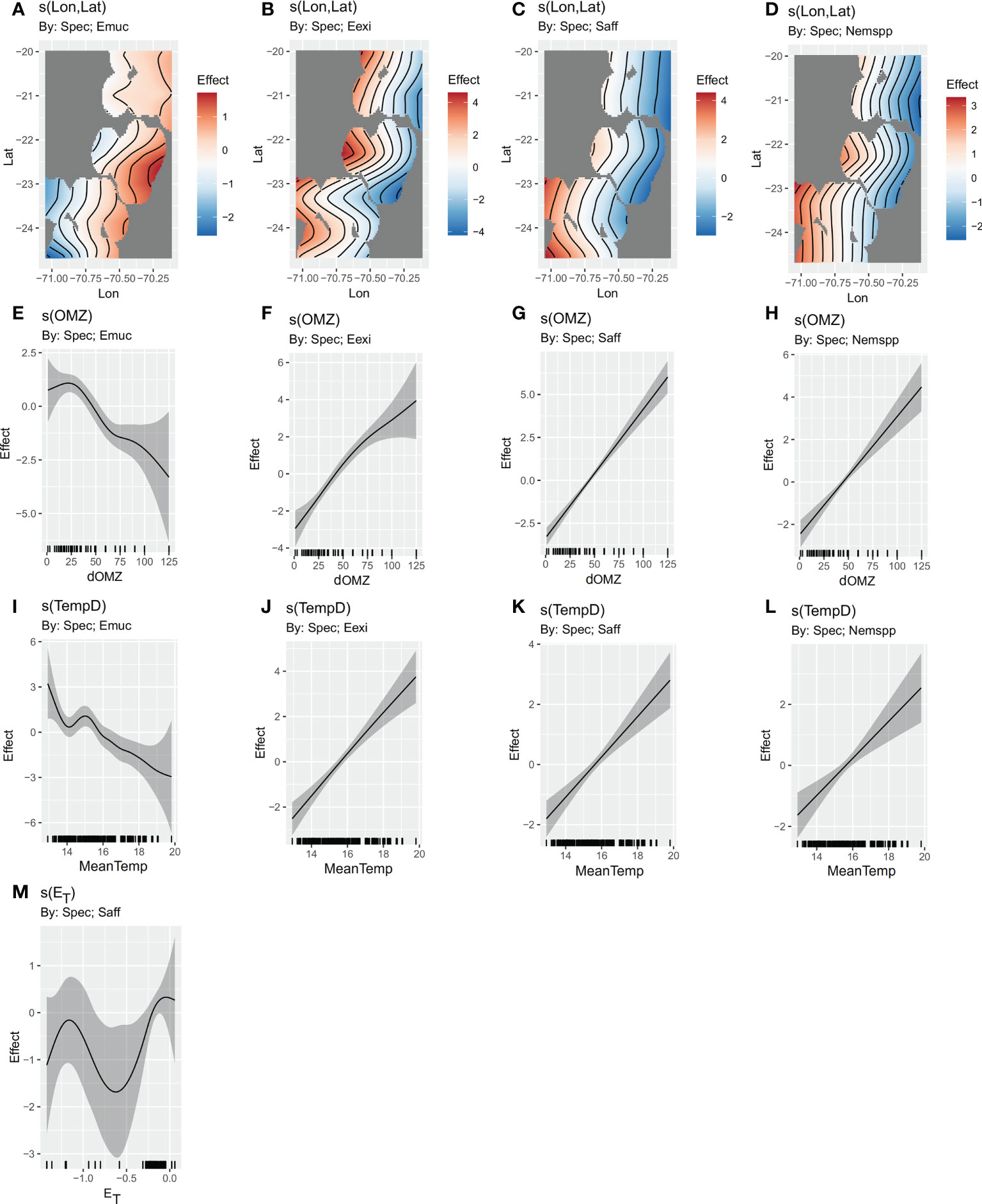
Figure 7 HGAM models, showing function smooths with 95% confidence bands of the selected covariates, in different models. (A–D) Spatial model, (E–H) dOMZ model, (I–L) MeanTemp model, and (M) effect of ET from final model. Only significant terms are shown. Emuc, E. mucronata, Eexi, E. eximia, Saff, S. affine, and Nemspp, Nematoscellis spp.
The spatial model revealed contrasting modeled distribution patterns between Humboldt krill and the other taxa. The modeled distribution of E. mucronata showed higher abundances near the coast, between 22° and 23°S, while E. eximia had higher abundances in the offshore zone, near ~22°S. Both S. affine and Nematoscelis spp. showed higher abundances in the offshore area south of 23°S (Figure 7). In the dOMZ model, a strong, negative, and non-linear effect was observed for Humboldt krill, in which the highest abundances are observed when dOMZ is ~ 25 m. A weak non-linear positive effect was observed with E. eximia, and a linear positive effect with S. affine and Nematoscelis spp. (Figure 7). In the MeanTemp model, the temperature had a negative non-linear effect over the abundance of Humboldt krill, with a peak near ~15°C. E. eximia, S. affine and Nematoscelis spp. showed a positive linear relationship with temperature. In the full model, the effect of the spatial covariate was significant for E. eximia and S. affine, the effect of MeanTemp was significant on Humboldt krill, and the effect of dOMZ, on all taxa. In addition, there was a significant non-linear relationship between ET and S. affine abundances, with lower values of ET (i.e., more intense offshore transport) having a negative effect on the abundances. However, this result needs to be analyzed carefully, because the scarce amount of data with ET<-0.5 creates large confidence intervals.
Because dOMZ and MeanTemp were the only environmental variables that were highly significant for all taxa in single-term models, their abundances were plotted against these variables to observe patterns across these environmental gradients (Figure 8). E. eximia, S. affine, and Nematoscelis spp. had a similar pattern of higher abundances when the depth of the OMZ was >50 m, and when the temperature of the upper 100 m was >14°C. E. mucronata had the opposite pattern, presenting the highest abundances within 13° and 15°C, and<60 m depth of the OMZ.
Model results: the effect of anchovy on euphausiid abundance
Even though the acoustic biomass of anchovy, included as a predatory term, had only a marginal effect in the final selected model, it was a significant predictor term for the endemic and numerically abundant species E. mucronata in every model that incorporated it. Anchovy acoustic biomass had a positive nonlinear effect on this species’ abundance up to biomass of ~30 m2 nm-2. Biomasses above that threshold had a negative effect, observed as a decrease in the abundance of E. mucronata as the anchovy biomass reached ~900 m2 nm-2. Above that level, the negative effect started to decrease (Figure 9).
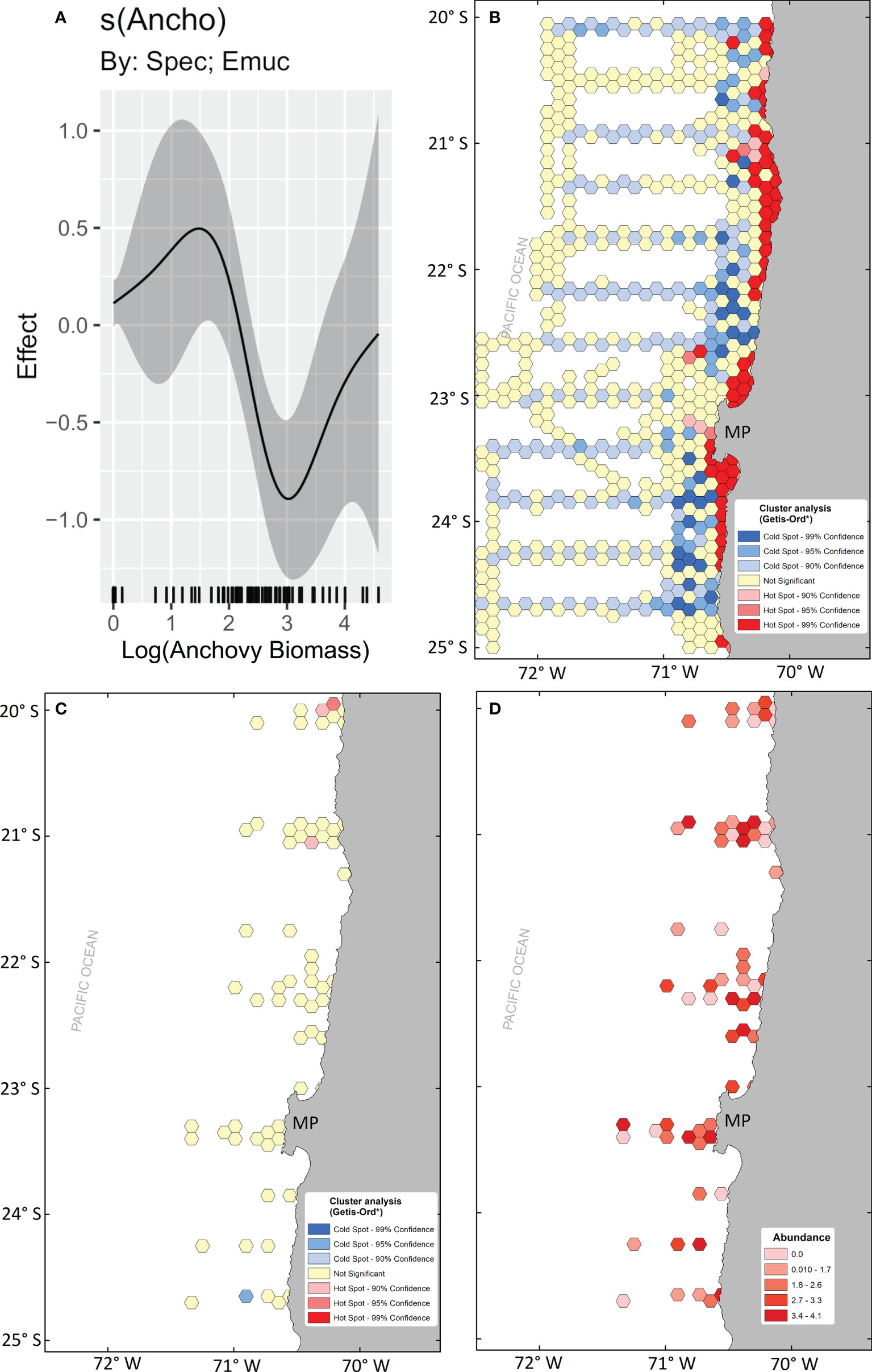
Figure 9 Effect of anchovy biomass (m2 nm-2) on E mucronata abundances (smooth function obtained from the HGAM final model) (A), Getis-Ord local Gi* cluster analysis for anchovy (B), and E mucronata (C), and mean abundance map of E mucronata (D).
We tested potential distribution overlap between anchovy and Humboldt krill by identifying the distribution of spatial hotspots. Significant clusters of anchovy biomass were found throughout the study area in the coastal zone (Figure 9). The aggregation of anchovy hotspots followed bathymetry, with more significant clusters where the continental shelf was wider (e.g., near 21°S, and north and south of the MP), and almost no hotspots and several coldspots outside the 200 m isobath. The Gi* statistic calculated with log-transformed abundance data for the whole study period did not show significant Humboldt krill aggregations, probably due to the relatively low number of sampling points and resolution compared to the anchovy dataset. The mean distribution of E. mucronata showed its presence in the whole study area, although abundances >2000 ind. 1000 m-3 were found in 3 main areas. A cluster of high abundances was found ~21°S, where the continental shelf is wider, a second cluster was found south of 22°S, and the third cluster was found in the continental shelf off the MP. The first cluster overlapped with significant anchovy hotspots, but the other two were found in areas with no anchovy hotspots.
Discussion
Impacts of thermal fluctuations, food availability, advection and oxygen concentration on krill abundances
Several modeling studies in cold and temperate systems have proved that temperature has a strong influence on euphausiid population dynamics (Dorman et al., 2015; Orlova et al., 2015; Hunt et al., 2016; Robertson and Bjorkstedt, 2020; Killeen et al., 2022; Phillips et al., 2022). Accordingly, our results provide evidence that temperature is one of the main drivers of krill abundance off northern Chile and plays a significant role in modulating krill spatial and temporal distribution. The HCS is subject to climate perturbations that account for most of the interannual environmental variability (Thomas et al., 2009; Di Lorenzo et al., 2013). During the study period, both ENSO and PDO indices shifted from negative (2010-2013) to positive (2014-2016), and then to neutral values (2017), with consequent fluctuations in water temperature, along-shore wind stress, and satellite chlorophyll-a (Díaz-Astudillo et al., 2022). Thermal fluctuations associated with climate oscillations and/or heatwaves tend to modulate interannual changes in euphausiid assemblages of upwelling systems (Parés-Escobar et al., 2018; Lilly and Ohman, 2021; Phillips et al., 2022). For example, the 2014-2015 marine heatwave off the west coast of the US and Canada caused a decline in krill abundance (Phillips et al., 2022) and modified the size structure of Euphausia pacifica (Robertson and Bjorkstedt, 2020), a numerically important species in the CCS. We observed that the years with the highest variations in krill abundances were also the years with the highest temperature anomalies. However, those years did not exhibit proportional changes in productivity. In particular, the abundance of the upwelling-associated Humboldt krill species (Riquelme-Bugueño et al., 2012; González et al., 2021) had significantly higher abundances during the years with the most negative temperature anomalies (late 2010, 2014, and 2017). These interannual abundance fluctuations were probably caused by distribution displacements driven by poleward water-mass advection and/or by coastal trapped waves linked to ENSO events, as it has been proved in the CCS (Lilly and Ohman, 2021). Although there is still conflicting evidence about the future of EBUS, research suggests that the temperature of the coastal areas of upwelling systems will decrease due to intensified winds and upwelling (Sydeman et al., 2014; García-Ramos et al., 2015; Seabra et al., 2019). Extreme El Niño (Cai et al., 2018) and La Niña (Cai et al., 2015) events are also expected to increase in frequency due to climate change. Marine heatwaves (spanning 30-100 days) also show an increase in intensity and duration in the last decades in the HCS (Pietri et al., 2021). It is plausible to expect shifts in species distribution associated with these extreme events. Warm events might be especially detrimental, since the Humboldt krill as keystone species would probably be displaced towards the south or restricted to coastal pools.
The model results showed that temperature is a better predictor of krill abundance than primary productivity. An exhaustive analysis of the spatial patterns of the krill community variability in central-southern Chile found strong associations between coastal species and chlorophyll-a (Riquelme-Bugueño et al., 2012). Nonetheless, the study area of that study covered a larger longitudinal range, and hence a larger productivity range, in an area influenced by seasonal upwelling. The survey area of the present study presents (on average) permanent upwelling conditions, as observed in the Ekman transport and SSC climatology. The SSC climatology confirmed high productivity during spring and fall, even during years with lower ET or during positive ENSO and PDO phases (e.g., 2015). Although the coastal upwelling band was narrower in these periods, chlorophyll-a concentration remained high. Previous studies on zooplankton production have suggested that primary productivity off central Chile allows copepod communities to grow without food limitations (Escribano et al., 2016). At a basin scale, a study about the main drivers of the abundance of euphausiid species in the Pacific Ocean found that chlorophyll-a had a marginal effect on the explained variance of the tested models (Letessier et al., 2011). However, the authors did not distinguish upwelling from equatorial or high seas dynamics. The lack of association between Humboldt krill and chlorophyll-a, whose growth and abundance have been directly associated with primary productivity (Riquelme-Bugueño et al., 2012; Riquelme-Bugueño et al., 2013) hints that the species population dynamics off central Chile differs from northern Chile. A short-term study in northern Chile did not find any association between phytoplankton biomass and any euphausiid species, but it did find significant and species-specific relationships with dissolved oxygen and water density (Fernández et al., 2002). In general, our results suggest that chlorophyll-a concentration remains sufficiently high to support large euphausiid populations off northern Chile and is not a controlling factor of krill species abundances and distribution, at least at the spatial and temporal scales covered in this study. González et al. (2021) found no relationship between chlorophyll-a concentration and Humboldt krill developmental stages. However, to properly determine whether there is food limitation, it is necessary to incorporate food ingestion rates (Antezana, 2010), growth rates (Riquelme-Bugueño et al., 2016), and biomass production (Riquelme-Bugueño et al., 2013) in future studies, to observe changes in these population parameters under different productivity scenarios.
The study of the mesoscale structure of krill hotspots in the highly advective CCS revealed that krill aggregations were inversely correlated to maximum upwelling points, suggesting that upwelling could act as an optimal window, where both high and low values of Ekman transport are detrimental for krill abundance and growth (Santora et al., 2011; Dorman et al., 2015; Riquelme-Bugueño et al., 2016). Our results showed a significant effect of Ekman transport on the abundance of S. affine only, an offshore epipelagic species (Gómez-Gutiérrez et al., 2005; Riquelme-Bugueño et al., 2012). Highly migratory euphausiid species might avoid cross-shore transport by inhabiting deep and less advective layers during the day (Barange, 1990; Escribano et al., 2009). The non-migratory behavior of S. affine, its vertical distribution within the upper 100 m of the water column (Escribano et al., 2009), and the fact of being one of the smallest subtropical krill species of the HCS (8.5 mm of maximum adult length, Baker et al., 1990), could be favoring its offshore advection through the Ekman layer, as it has been observed with early non-migratory life stages (Lu et al., 2003; Dorman et al., 2005; Díaz-Astudillo et al., 2022). The expected intensification of upwelling due to an intensification of alongshore winds (Sydeman et al., 2014) might be detrimental to small epipelagic species and early life stages because of increased offshore advection and transport to less productive waters, as it has been observed in the CCS (Santora et al., 2011; Dorman et al., 2015).
The permanent coastal upwelling off northern Chile generates a practically continuous upwards transport of hypoxic Equatorial Subsurface Water, which together with the biogeochemical consumption of oxygen, creates and maintains a quasi-permanent and shallow OMZ (Paulmier et al., 2006). Several pelagic coastal organisms show vertical habitat partitioning to cope with oxygen depletion (Sameoto et al., 1987; Castro et al., 2007; Hoving et al., 2020). In the case of krill, some species inhabit the upper oxygenated layer, while others inhabit the OMZ or are regularly crossing it during their diurnal vertical migrations (Antezana, 2009; Escribano et al., 2009; Riquelme-Bugueño et al., 2020). We observed significant and opposite responses of krill abundance to dOMZ. Humboldt krill presented higher modeled abundances when the OMZ was shallower (and by association dissolved oxygen was lower), proving to be an upwelling associated species with physiological capabilities to inhabit and perform metabolically efficiently under hypoxic conditions (Antezana, 2002; Tremblay and Abele, 2016; Kiko and Hauss (2019). The other 3 taxa had the opposite pattern, suggesting a habitat preference for more oxygenated waters. The known distribution of these species shows that E. mucronata and several Nematoscelis species normally inhabit the core of the OMZ during the daytime, while S. affine occupies the surface oxygenated layer, and E. eximia regularly crosses the OMZ, but does not stay in the core (Antezana, 2009; Escribano et al., 2009). Both E. mucronata and E. eximia are large and highly migratory species with metabolic adaptations to survive in hypoxic or even anoxic conditions (e.g., through antioxidant enzyme activity and metabolism suppression (Tremblay et al., 2010; Tremblay et al., 2020)). However, vertical distribution descriptions and our model’s results indicate that higher abundances of E. eximia should be expected at higher oxygen concentrations. The adaptation of Humboldt krill to low oxygen is probably the adaptative advantage explaining its large abundance in this ecosystem. Tremblay and Abele (2016) found oxyconforming pO2-dependent respiration below 80% air saturation in E. mucronata, and Kiko et al. (2016), reported a strong reduction of respiration and ammonium excretion at low oxygen levels. Antezana (2002) on the other hand, found constant respiration rates in Humboldt krill when exposed to different O2 concentrations, suggesting oxyregulation. A larger gills/cephalotorax ratio have been found in E. mucronata and E. eximia, increasing the surface area for oxygen uptake suggesting enhanced O2 extraction capabilities in these species (Antezana, 2002). More recently, Tremblay et al. (2020) described Humboldt krill as a metabolic suppressor species based on the “Regulation Index” (RI) which ranges from RI = 0 (perfect oxyconformity) to RI = 1 (perfect regulation).
Prey-predator interactions
The study of temporal correlations between anchovy and krill (Ayón et al., 2011) and anchovy and zooplankton (Ayón et al., 2008) in the HCS have suggested that mesozooplankton can directly affect anchovy populations by bottom-up trophic control. Here, without discarding that hypothesis, we show the opposite effect, with anchovy having a negative effect on krill abundance, adding predation as a potential ecological process controlling krill populations in the HCS at local scales. Trophic processes depend on many aspects. Top-down control prevails in ecosystems with low temperatures and species diversity (Frank et al., 2007), such as the continental shelf of eastern boundary upwelling systems. Top-down control influences populations at local, but not regional scales (Hunt and McKinnell, 2006; Atkinson et al., 2014). Orlova et al. (2015) fitted different regression models to specific areas of the Barents Sea, finding that predation pressure on krill abundance varied among areas with contrasting oceanographic conditions. They found a quadratic relationship with cod and a negative linear regression with capelin, which together with environmental variability explained between 30% and 64% of the total euphausiid abundance variance. Nonetheless, a significant effect of a covariate does not necessarily imply trophic control. Ressler et al. (2014) found a significant effect of walleye pollock (estimated with trawl and acoustic surveys) on krill biomass (acoustically estimated) in the Bering Sea, but the relationship was relatively flat, and the explained power of the model containing the predator abundance did not improve much compared to a model without it. Hence, Ressler et al. (2014) discarded top-down control as the main process controlling krill populations in the Bering Sea. The effect of anchovy on Humboldt krill abundance was fitted as a complex non-linear curve showing a negative effect. Nonetheless, the wide confidence bands at the opposite ends of the covariate axis suggest that the positive effect at both low and high anchovy abundances should be analyzed with care, especially because they were not estimated using the same approach (Bongo nets versus acoustic estimations). Model approaches are useful for exploring temporal and spatial relationships and functional responses between prey and predator. However, food consumption rates must be included to confirm feeding habits and properly evaluate the existence of trophic control (Abrams and Ginzburg, 2000).
It has been described that, while being an opportunistic omnivorous feeder, anchovy selects the largest available prey from the community (Espinoza and Bertrand, 2008). In the HCS, Humboldt krill is one of the largest species in the mesozooplankton community, which can measure up to 30 mm (Díaz-Astudillo et al., 2022 unpublished data) and form highly dense aggregations, swarms, and schools. Humboldt krill is, therefore, the main prey item in the diet of anchovy, as it has been shown with stomach content (Espinoza and Bertrand, 2008) and trophic biomarkers (Massing et al., 2022) studies. Off Perú, Ayón et al. (2011) found that anchovy was mostly distributed near the coast, while the highest abundances of krill were found offshore and claimed there could be a spatial mismatch between both taxa. In this study, we describe how both Peruvian anchovy and Humboldt krill are mainly distributed near the coast, nonetheless, high Humboldt krill abundances were mostly found in areas without anchovy hotspots. The exception was found in areas where the continental shelf was wider, specifically in the coastal area ~21°C. There, significant anchovy hotspots overlapped with high Humboldt krill abundances, suggesting a potential effect of shelf width on trophic interactions. The interaction and overlap between both groups are complex, and they highlight the need to improve the resolution of the krill datasets for future analysis, for example, by using acoustic biomass estimations.
Peruvian anchovy abundances fluctuate with temperature, chlorophyll-a, and oxygen changes, similar to Humboldt krill, presenting higher abundances towards the coast (Bertrand et al., 2011; Silva et al., 2016; Silva et al., 2019). Anchovy distribution is closely associated with hypoxic coastal waters, where prey upon zooplankton aggregations in the shallow normoxic layer (Bertrand et al., 2011). Recent studies have shown that under climate change IPCC scenarios, anchovy habitat suitability off northern and central Chile will be reduced because of an increase in SST and upwelling intensity, and a decrease in chlorophyll-a concentration (Silva et al., 2016; Silva et al., 2018). Summer months were the exception, since anchovy modeled habitat suitability showed an increase near the coast (<50 km from the coast) in that season (Silva et al., 2016). An increase in anchovy abundances near the coast could modify trophic interactions by increasing the predatory pressure on krill, favoring top-down trophic control at a local scale. Nonetheless, this would only apply to anomalously warmer periods. An intensification of upwelling and a decrease in SST, the potential future conditions for the HCS (García-Reyes et al., 2015; Seabra et al., 2019), could lead to either higher productivity or offshore advection, which could benefit or harm krill populations, respectively. Therefore, future studies in the HCS, and EBUS in general, should (1) test the effect of increased/decreased upwelling and higher/lower predator biomass on krill populations, 2) incorporate anchovy food consumption rates and stomach content to confirm top-down associations, and (3) model the influence of climate regimes and local oceanography on krill-anchovy associations, considering that both temperature and oxygen dynamics, especially the depth of the OMZ (Gilly et al., 2013) can modify trophic interactions.
Conclusion
We fitted an HGAM model to determine the main abiotic and biotic predictors of krill abundance, fitting taxa-specific flexible responses. We found that dOMZ and MeanTemp were the main predictors of abundance, and were positively related to E. eximia, S. affine and Nematoscelis spp. In contrast, the Humboldt krill (E. mucronata), the numerically dominant species of this ecosystem, showed a non-linear negative relationship with dOMZ and MeanTemp, confirming its association to hypoxic and cold upwelling waters. Ekman transport was a significant predictor of S. affine abundances, suggesting this small species could be advected offshore under intense upwelling conditions.
Satellite chlorophyll-a was not a significant predictor, for any taxa, and SSC climatologies showed high levels of primary productivity throughout the study period. These results, along with the known omnivore feeding behavior of krill, suggest the absence of “bottom-up” trophic control of krill populations of the HUS. Anchovy acoustic biomass was a significant predictor of E. mucronata abundances, although its predictive power was lower than the environmental covariates. We explored the spatial distribution of anchovy and Humboldt krill and found that they both distribute near the coast, but the clusters of high krill abundance were mainly decoupled to anchovy hotspots.
This study arises evidence about the importance of the depth of the OMZ and temperature as controlling factors of krill abundance and distribution. Extreme events that alter hydrographic conditions in the HCS, such as heat waves and El Niño events, could get more intense and frequent with climate change. Krill could be particularly sensitive to these changes. Trophic interactions could also change with these extreme events since both prey and predator distributions can be modified. Future studies should focus on improving the resolution of krill datasets and model the effect of remote variability on trophic interactions.
Data availability statement
The raw data supporting the conclusions of this article will be made available by the authors, without undue reservation.
Author contributions
MD-A: conceptualization and design of the study, laboratory analysis, data analysis, and writing of original and final draft; KB: support with HGAM analyses, interpretation and discussion of results, revision, and draft editing. RR-B: conceptualization, analysis supervision, interpretation and discussion of results, revision, and draft editing. GS: interpretation and discussion of results, revision, and draft editing. RR: support spatial analysis and draft editing. JL: provided the datasets and revision of the draft. All authors contributed to the article and approved the submitted version.
Funding
This work was funded by the Chilean Agency for Research and Development (ANID/CONICYT-PFCHA/Doctorado Nacional/2018–21180600) and by the UCO 1866 program of Universidad de Concepción (MD-A). MD-A and RR-B are supported by the ANID Millennium Science Initiative Program (Millennium Institute of Oceanography ICN12_019), and project VRID 219.113.097-INV. GS is supported by FONDECYT grant N° 1220167, the Millennium Science Initiative Program Code ICN2019_015 (Coastal Social-Ecological Millennium Institute, SECOS), and by COPAS COASTAL ANID FB210021.
Acknowledgments
We want to thank José Córdova from Instituto de Fomento Pesquero for providing some of the anchovy datasets, and Dr. Pamela Hidalgo for providing lab space and materials used in the krill analyses.
Conflict of interest
The authors declare that the research was conducted without any commercial or financial relationships that could be construed as a potential conflict of interest.
Publisher’s note
All claims expressed in this article are solely those of the authors and do not necessarily represent those of their affiliated organizations, or those of the publisher, the editors and the reviewers. Any product that may be evaluated in this article, or claim that may be made by its manufacturer, is not guaranteed or endorsed by the publisher.
References
Abrams P. A., Ginzburg L. R. (2000). The nature of predation: Prey dependent, ratio dependent or neither? Trends Ecol. Evol. 15, 337–341. doi: 10.1016/S0169-5347(00)01908-X
Ambriz-Arreola I., Gómez-Gutiérrez J., Franco-Gordo M., del C., Plascencia-Palomera V., Gasca R., et al. (2018). Seasonal succession of tropical community structure, abundance, and biomass of five zooplankton taxa in the central Mexican pacific. Cont. Shelf Res. 168, 54–67. doi: 10.1016/j.csr.2018.08.007
Antezana T. (2002). “Adaptive behavior of Euphausia mucronata in relation to the oxygen minimum layer of the Humboldt current,” in Oceanography of the Eastern pacific. Ed. Färber-Lorda J. (Ensenada: CICESE), 29–40.
Antezana T. (2009). Species-specific patterns of diel migration into the oxygen minimum zone by euphausiids in the Humboldt current ecosystem. Prog. Oceanogr. 83, 228–236. doi: 10.1016/j.pocean.2009.07.039
Antezana T. (2010). Euphausia mucronata: A keystone herbivore and prey of the Humboldt current system. Deep. Res. Part II 57, 652–662. doi: 10.1016/j.dsr2.2009.10.014
Aronés K., Ayón P., Hirche H. J., Schwamborn R. (2009). Hydrographic structure and zooplankton abundance and diversity off paita, northern peru (1994 To 2004) - ENSO effects, trends and changes. J. Mar. Syst. 78, 582–598. doi: 10.1016/j.jmarsys.2009.01.002
Atkinson A., Hill S. L., Barange M., Pakhomov E. A., Raubenheimer D., Schmidt K., et al. (2014). Sardine cycles, krill declines, and locust plagues: Revisiting “wasp-waist” food webs. Trends Ecol. Evol. 29, 309–316. doi: 10.1016/j.tree.2014.03.011
Ayón P., Swartzman G., Bertrand A., Gutiérrez M., Bertrand S. (2008). Zooplankton and forage fish species off Peru: Large-scale bottom-up forcing and local-scale depletion. Prog. Oceanogr. 79, 208–214. doi: 10.1016/j.pocean.2008.10.023
Ayón P., Swartzman G., Espinoza P., Bertrand A. (2011). Long-term changes in zooplankton size distribution in the Peruvian Humboldt current system: Conditions favouring sardine or anchovy. Mar. Ecol. Prog. Ser. 422, 211–222. doi: 10.3354/meps08918
Baker A. C., Boden B. P., Brinton E. (1990). A practical guide to the euphausiids of the world (London: Natural History Museum Publications).
Barange M. (1990). Vertical migration and habitat partitioning of six euphausiid species in the northern benguela upwelling system. J. Plankton Res. 12, 1223–1237. doi: 10.1093/plankt/12.6.1223
Bertrand A., Barbieri M. A., Córdova J., Hernández C., Gómez F., Leiva F. (2004). Diel vertical behaviour, predator-prey relationships, and occupation of space by jack mackerel (Trachurus murphyi) off Chile. ICES J. Mar. Sci. 61, 1105–1112. doi: 10.1016/j.icesjms.2004.06.010
Bertrand A., Chaigneau A., Peraltilla S., Ledesma J., Graco M., Monetti F., et al. (2011). Oxygen: A fundamental property regulating pelagic ecosystem structure in the coastal southeastern tropical pacific. PloS One 6, 2–9. doi: 10.1371/journal.pone.0029558
Brinton E., Townsend A. (2003). Decadal variability in abundances of the dominant euphausiid species in southern sectors of the California current. Deep. Res. Part II 50, 2449–2472. doi: 10.1016/S0967-0645(03)00126-7
Cai W., Wang G., Dewitte B., Wu L., Santoso A., Takahashi K., et al. (2018). Increased variability of eastern pacific El niño under greenhouse warming. Nature 564, 201–206. doi: 10.1038/s41586-018-0776-9
Cai W., Wang G., Santoso A., Mcphaden M. J., Wu L., Jin F. F., et al. (2015). Increased frequency of extreme la niña events under greenhouse warming. Nat. Clim. Change 5, 132–137. doi: 10.1038/nclimate2492
Castro L. R., Troncoso V. A., Figueroa D.R. (2007). Fine-scale vertical distribution of coastal and offshore copepods in the golfo de arauco, central Chile, during the upwelling season. Prog. Oceanogr. 75, 486–500. doi: 10.1016/j.pocean.2007.08.012
Core Team R. (2021). R: A language and environment for statistical computing. r foundation for statistical computing (Vienna, Austria). Available at: https://www.R-project.org/.
Díaz-Astudillo M., Saldías G. S., Letelier J., Riquelme-Bugueño R. (2022). Spatial and interannual variability in the distribution of euphausiid life stages in the permanent upwelling system off northern Chile. ICES J. Mar. Sci. 79, 61–75. doi: 10.1093/icesjms/fsab241
Di Lorenzo E., Combes V., Keister J. E., Strub P. T., Thomas A. C., Franks P. J. S., et al. (2013). Synthesis of pacific ocean climate and ecosystem dynamics. Oceanography 26, 68–81. doi: 10.5670/oceanog.2013.76
Dorman J. G., Bollens S. M., Slaughter A. M. (2005). Population biology of euphausiids off northern California and effects of short time-scale wind events on Euphausia pacifica. Mar. Ecol. Prog. Ser. 288, 183–198. doi: 10.3354/meps288183
Dorman J. G., Sydeman W. J., García-Reyes M., Zeno R. A., Santora J. A. (2015). Modeling krill aggregations in the central-northern California current. Mar. Ecol. Prog. Ser. 528, 87–99. doi: 10.3354/meps11253
Escribano R., Bustos-Ríos E., Hidalgo P., Morales C. E. (2016). Non-limiting food conditions for growth and production of the copepod community in a highly productive upwelling zone. Cont. Shelf Res. 126, 1–14. doi: 10.1016/j.csr.2016.07.018
Escribano R., Hidalgo P., Fuentes M., Donoso K. (2012). Zooplankton time series in the coastal zone off Chile: Variation in upwelling and responses of the copepod community. Prog. Oceanogr. 97–100, 174–186. doi: 10.1016/j.pocean.2011.11.006
Escribano R., Hidalgo P., Krautz C. (2009). Zooplankton associated with the oxygen minimum zone system in the northern upwelling region of Chile during march 2000. Deep. Res. 56, 1083–1094. doi: 10.1016/j.dsr2.2008.09.009
Espinoza P., Bertrand A. (2008). Revisiting Peruvian anchovy (Engraulis ringens) trophodynamics provides a new vision of the Humboldt current system. Prog. Oceanogr. 79, 215–227. doi: 10.1016/j.pocean.2008.10.022
Espinoza P., Lorrain A., Ménard F., Cherel Y., Tremblay-Boyer L., Argüelles J., et al. (2017). Trophic structure in the northern Humboldt current system: new perspectives from stable isotope analysis. Mar. Biol. 164, 1–15. doi: 10.1007/s00227-017-3119-8
FAO. (2020). “The state of world fisheries and aquaculture 2020,” in Sustainability in action (Rome: FAO). doi: 10.4060/ca9229en
Fernández D., Escribano R., Hidalgo P. (2002). Distribución de eufáusidos en el sistema de surgencia frente a la península de mejillones (23°S) asociada a condiciones previas y durante El niño 1997-98. Investig. Mar. 30, 25–43. doi: 10.4067/s0717-71782002000100002
Frank K. T., Petrie B., Shackell N. L. (2007). The ups and downs of trophic control in continental shelf ecosystems. Trends Ecol. Evol. 22, 236–242. doi: 10.1016/j.tree.2007.03.002
García-Reyes M., Sydeman W. J., Schoeman D. S., Rykaczewski R. R., Black B. A., Smit A. J., et al. (2015). Under pressure: Climate change, upwelling, and Eastern boundary upwelling ecosystems. Front. Mar. Sci. 2. doi: 10.3389/fmars.2015.00109
Getis A., Ord J. K. (1992). The analysis of spatial association by use of distance statistics. Geogr. Anal. 24, 189–206. doi: 10.1111/j.1538-4632.1992.tb00261.x
Gilly W. F., Michael Beman J., Litvin S. Y., Robison B. H. (2013). Oceanographic and biological effects of shoaling of the oxygen minimum zone. Ann. Rev. Mar. Sci. 5, 393–420. doi: 10.1146/annurev-marine-120710-100849
Gómez-Gutiérrez J., Peterson W. T., Miller C. B. (2005). Cross-shelf life-stage segregation and community structure of the euphausiids off central oregon (1970-1972). Deep. Res. Part II Top. Stud. Oceanogr. 52, 289–315. doi: 10.1016/j.dsr2.2004.09.023
González P., Mujica A., Nava M. (2021). Distribution and abundance of Euphausia mucronata: development stages and its relationship with temperature and chlorophyll-a concentration. Lat. Am. J. Aquat. Res. 49 (4), 640–648. doi: 10.3856/vol49-issue4-fulltext-2509
Gutiérrez M., Swartzman G., Bertrand A., Bertrand S. (2007). Anchovy (Engraulis ringens) and sardine (Sardinops sagax) spatial dynamics and aggregation patterns in the Humboldt current ecosystem, per , from 1983-2003. Fish. Oceanogr. 16, 155–168. doi: 10.1111/j.1365-2419.2006.00422.x
Hoving H. J. T., Neitzel P., Hauss H., Christiansen S., Kiko R., Robison B. H., et al. (2020). In situ observations show vertical community structure of pelagic fauna in the eastern tropical north Atlantic off cape Verde. Sci. Rep. 10, 1–14. doi: 10.1038/s41598-020-78255-9
Hunt G. L. Jr., McKinnell S. (2006). Interplay between top-down, bottom-up, and wasp-waist control in marine ecosystems. Prog. Oceanogr. 68, 115–124. doi: 10.1016/j.pocean.2006.02.008
Hunt G. L. Jr., Ressler P. H., Gibson G. A., De Robertis A., Aydin K., Sigler M. F., et al. (2016). Euphausiids in the eastern Bering Sea: A synthesis of recent studies of euphausiid production, consumption and population control. Deep. Res. Part II Top. Stud. Oceanogr. 134, 204–222, . doi: 10.1016/j.dsr2.2015.10.007
Kiko R., Hauss H. (2019). On the estimation of zooplankton-mediated active fluxes in oxygen minimum zone regions. Front. Mar. Sci. 6. doi: 10.3389/fmars.2019.00741
Kiko R., Hauss H., Buchholz F., Melner F. (2016). Ammonium excretion and oxygen respiration of tropical copepods and euphausiids exposed to oxygen minimum zone conditions. Biogeosci.fmars-09-979984-g003.tif 13, 2241–2255. doi: 10.5194/bg-13-2241-2016
Killeen H., Dorman J., Sydeman W., Dibble C., Morgan S. (2022). Effects of a marine heatwave on adult body length of three numerically dominant krill species in the California current ecosystem. ICES J. Mar. Sci. 79, 761–774. doi: 10.1093/icesjms/fsab215
Kindt R. (2021). AlleleShift: an r package to predict and visualize population-level changes in allele frequencies in response to climate change. PeerJ 9, e11534. doi: 10.7717/peerj.11534
Leiva F., Pizarro M., Bustamantes A., Cifuentes U., Grendi C., Leiva B., et al. (2018). Informe final convenio de desempeño 2017: Evaluación hidroacústica del reclutamiento de la anchoveta en la XV, I y II regiones, año 2017 (Chile: Subsecretaría de Economía y EMT/IFOP), 301.
Letelier J., Pizarro O., Nuñez S. (2009). Seasonal variability of coastal upwelling and the upwelling front off central Chile. J. Geophys. Res. Ocean. 114, 1–16. doi: 10.1029/2008JC005171
Letessier T. B., Cox M. J., Brierley A. S. (2011). Drivers of variability in euphausiid species abundance throughout the pacific ocean. J. Plankton Res. 33, 1342–1357. doi: 10.1093/plankt/fbr033
Lilly L. E., Ohman M. D. (2021). Euphausiid spatial displacements and habitat shifts in the southern California current system in response to El niño variability. Prog. Oceanogr. 193, 102544. doi: 10.1016/j.pocean.2021.102544
Lu B., Mackas D. L., Moore D. F. (2003). Cross-shore separation of adult and juvenile euphausiids in a shelf-break alongshore current. Prog. Oceanogr. 57, 381–404. doi: 10.1016/s0079-6611(03)00107-1
Manríquez K., Escribano R., Hidalgo P. (2009). The influence of coastal upwelling on the mesozooplankton community structure in the coastal zone off Central/Southern Chile as assessed by automated image analysis. J. Plankton Res. 31, 1075–1088. doi: 10.1093/plankt/fbp053
Massing J. C., Schukat A., Auel H., Auch D., Kittu L., Pinedo E. L., et al. (2022). Toward a solution of the "Peruvian puzzle”: Pelagic food-web structure and trophic interactions in the northern Humboldt current upwelling system off Peru. Front. Mar. Sci. 8. doi: 10.3389/fmars.2021.759603
Medellín-Mora J., Escribano R., Schneider W. (2016). Community response of zooplankton to oceanographic changes (2002-2012) in the central/southern upwelling system of Chile. Prog. Oceanogr. 142, 17–29. doi: 10.1016/j.pocean.2016.01.005
Montecino V., Lange C. (2009). The Humboldt current system: Ecosystem components and processes, fisheries, and sediment studies. Prog. Oceanogr. 83, 65–79. doi: 10.1016/j.pocean.2009.07.041
Murase H., Nagashima H., Yonezaki S., Matsukura R., Kitakado T. (2009). Application of a generalized additive model (GAM) to reveal relationships between environmental factors and distributions of pelagic fish and krill: A case study in Sendai bay, Japan. ICES J. Mar. Sci. 66, 1417–1424. doi: 10.1093/icesjms/fsp105
Orlova E., Dolgov A. V., Renaud P. E., Greenacre M., Halsband C., Ivshin V. A. (2015). Climatic and ecological drivers of euphausiid community structure vary spatially in the barents Sea: relationships from a long time series (1952-2009). Front. Mar. Sci. 1. doi: 10.3389/fmars.2014.00074
Parés-Escobar F., Lavaniegos B. E., Ambriz-Arreola I. (2018). Interannual summer variability in oceanic euphausiid communities off the Baja California western coast during 1998-2008. Prog. Oceanogr. 160, 53–67. doi: 10.1016/j.pocean.2017.11.009
Pedersen E. J., Miller D. L., Simpson G. L., Ross N. (2019). Hierarchical generalized additive models in ecology: An introduction with mgcv. PeerJ 2019. doi: 10.7717/peerj.6876
Phillips E. M., Chu D., Gauthier S., Parker-Stetter S. L., Shelton A. O., Thomas R. E. (2022). Spatiotemporal variability of euphausiids in the California current ecosystem: insights from a recently developed time series. ICES J. Mar. Sci. 1–15 doi: 10.1093/icesjms/fsac055
Pietri A., Colas F., Mogollon R., Tam J., Gutierrez D. (2021). Marine heatwaves in the Humboldt current system: from 5-day localized warming to year-long El niños. Sci. Rep. 11, 1–12. doi: 10.1038/s41598-021-00340-4
Pillar S. C., Stuart V., Barange M., Gibbons M. J. (1992). Community structure and trophic ecology of euphausiids in the benguela ecosystem. South Afr. J. Mar. Sci. 12, 393–409. doi: 10.2989/02577619209504714
Ressler P. H., De Robertis A., Kotwicki S. (2014). The spatial distribution of euphausiids and walleye pollock in the eastern Bering Sea does not imply top-down control by predation. Mar. Ecol. Prog. Ser. 503, 111–122. doi: 10.3354/meps10736
Riquelme-Bugueño R., Escribano R., Gómez-Gutiérrez J. (2013). Somatic and molt production in Euphausia mucronata off central-southern Chile: The influence of coastal upwelling variability. Mar. Ecol. Prog. Ser. 476, 39–57. doi: 10.3354/meps10142
Riquelme-Bugueño R., Núñez S., Jorquera E., Valenzuela L., Escribano R., Hormazábal S. (2012). The influence of upwelling variation on the spatially-structured euphausiid community off central-southern Chile in 2007-2008. Prog. Oceanogr. 92–95, 146–165. doi: 10.1016/j.pocean.2011.07.003
Riquelme-Bugueño R., Pérez-Santos I., Alegría N., Vargas C. A., Urbina M. A., Escribano R. (2020). Diel vertical migration into anoxic and high-pCO2 waters: acoustic and net-based krill observations in the Humboldt current. Sci. Rep. 10, 1–11. doi: 10.1038/s41598-020-73702-z
Riquelme-Bugueño R., Silva-Aburto J., Escribano R., Peterson W. T., Schneider W. (2016). Growth of the Humboldt current krill in the upwelling zone off central Chile. J. Mar. Syst. 163, 1–11. doi: 10.1016/j.jmarsys.2016.06.001
Robertson R. R., Bjorkstedt E. P. (2020). Climate-driven variability in Euphausia pacifica size distributions off northern California. Prog. Oceanogr. 188, 102412. doi: 10.1016/j.pocean.2020.102412
Rockwood R. C., Elliott M. L., Saenz B., Nur N., Jahncke J. (2020). Modeling predator and prey hotspots: Management implications of baleen whale co-occurrence with krill in central California. PLoS One 15, 1–30. doi: 10.1371/journal.pone.0235603
Sameoto D., Guglielmo L., Lewis M. K. (1987). Day/night vertical distribution of euphausiids in the eastern tropical pacific. Mar. Biol. 96, 235–245. doi: 10.1007/BF00427023
Santora J. A., Schroeder I. D., Field J. C., Wells B. K., Sydeman W. J. (2014). Spatio-temporal dynamics of ocean conditions and forage taxa reveal regional structuring of seabird-prey relationships. Ecol. Appl. 24, 1730–1747. doi: 10.1890/13-1605.1
Santora J. A., Sydeman W. J., Schroeder I. D., Field J. C., Miller R. R., Wells B. K. (2017). Persistence of trophic hotspots and relation to human impacts within an upwelling marine ecosystem. Ecol. Appl. 27, 560–574. doi: 10.1002/eap.1466
Santora J. A., Sydeman W. J., Schroeder I. D., Wells B. K., Field J. C. (2011). Mesoscale structure and oceanographic determinants of krill hotspots in the California current: Implications for trophic transfer and conservation. Prog. Oceanogr. 91, 397–409. doi: 10.1016/j.pocean.2011.04.002
Seabra R., Varela R., Santos A. M., Gómez-Gesteira M., Meneghesso C., Wethey D. S., et al. (2019). Reduced nearshore warming associated with Eastern boundary upwelling systems. Front. Mar. Sci. 6. doi: 10.3389/fmars.2019.00104
Silva C., Andrade I., Yáñez E., Hormazabal S., Barbieri M. A., Aranis A., et al. (2016). Predicting habitat suitability and geographic distribution of anchovy (Engraulis ringens) due to climate change in the coastal areas off Chile. Prog. Oceanogr. 146, 159–174. doi: 10.1016/j.pocean.2016.06.006
Silva C., Leiva F., Lastra J. (2019). Predicting the current and future suitable habitat distributions of the anchovy (Engraulis ringens) using the maxent model in the coastal areas off central-northern Chile. Fish. Oceanogr. 28, 171–182. doi: 10.1111/fog.12400
Simonsen K. A., Ressler P. H., Rooper C. N., Zador S. G. (2016). Spatio-temporal distribution of euphausiids: an important component to understanding ecosystem processes in the gulf of Alaska and eastern Bering Sea. ICES J. Mar. Sci. 73, 2020–2036. doi: 10.1093/icesjms/fsv272
Simpson G. (2022). Graceful ggplot-Based Graphics and Other Functions for GAMs Fitted using mgcv. R package version 0.7.3.18. Available at: https://gavinsimpson.github.io/gratia/.
Sydeman W. J., Santora J. A., Thompson S. A., Marinovic B., Lorenzo E. (2013). Increasing variance in north pacific climate relates to unprecedented ecosystem variability off California. Glob. Change Biol. 19, 1662–1675. doi: 10.1111/gcb.12165
Sydeman W. J., Schoeman D. S., Rykaczewski R. R., Thompson S. A., Black B. A., Bograd S. J., et al. (2014). Climate change and wind intensification in coastal upwelling ecosystems. Sci. (80-. ) 345, 77–80. doi: 10.1126/science.1251635
Thomas A. C., Brickley P., Weatherbee R. (2009). Interannual variability in chlorophyll concentrations in the Humboldt and California current systems. Prog. Oceanogr. 83, 386–392. doi: 10.1016/j.pocean.2009.07.020
Tremblay N., Abele D. (2016). Response of three krill species to hypoxia and warming: an experimental approach to oxygen minimum zones expansion in coastal ecosystems. Mar. Ecol. 37, 179–199. doi: 10.1111/maec.12258
Tremblay N., Go J., Zenteno-savı T., Robinson C. J., Sa L. (2010). Role of oxidative stress in seasonal and daily vertical migration of three krill species in the gulf of California. Limnol. Oceanogr 55, 2570–2584. doi: 10.4319/lo.2010.55.6.2570
Tremblay N., Hünerlage K., Werner T. (2020). Hypoxia tolerance of 10 euphausiid species in relation to vertical temperature and oxygen gradients. Front. Physiol. 11. doi: 10.3389/fphys.2020.00248
Keywords: euphausiids, upwelling, El Niño, generalized additive models, trophic interactions, planktivorous fish
Citation: Díaz-Astudillo M, Riquelme-Bugueño R, Bernard KS, Saldías GS, Rivera R and Letelier J (2022) Disentangling species-specific krill responses to local oceanography and predator’s biomass: The case of the Humboldt krill and the Peruvian anchovy. Front. Mar. Sci. 9:979984. doi: 10.3389/fmars.2022.979984
Received: 28 June 2022; Accepted: 21 September 2022;
Published: 13 October 2022.
Edited by:
Shin-ichi Ito, The University of Tokyo, JapanReviewed by:
Kui Zhang, South China Sea Fisheries Research Institute, (CAFS), ChinaEndar Widiah Ningrum, University of Indonesia, Indonesia
Copyright © 2022 Díaz-Astudillo, Riquelme-Bugueño, Bernard, Saldías, Rivera and Letelier. This is an open-access article distributed under the terms of the Creative Commons Attribution License (CC BY). The use, distribution or reproduction in other forums is permitted, provided the original author(s) and the copyright owner(s) are credited and that the original publication in this journal is cited, in accordance with accepted academic practice. No use, distribution or reproduction is permitted which does not comply with these terms.
*Correspondence: Ramiro Riquelme-Bugueño, cnJpcXVlbG1AdWRlYy5jbA==