- Department of Physical and Technological Oceanography, Instituto de Ciencias del Mar (ICM) – Consejo Superior de Investigaciones Científicas (CSIC), Barcelona, Spain
For the first time, an accurate and complete picture of Mixed Layer (ML) water mass dynamics can be inferred at high spatio-temporal resolution via the material derivative derived from Sea Surface Salinity/Temperature (SSS/T) and Currents (SSC). The product between this satellite derived material derivative and in-situ derived Mixed Layer Depth (MLD) provides a satellite based kinematic approach to the water mass (trans)formation framework (WMT/F) above ML. We compare this approach to the standard thermodynamic approach based on air-sea fluxes provided by satellites, an ocean state estimate and in-situ observations. Southern Hemisphere surface density flux and water mass (trans)formation framework (WMT/F) were analysed in geographic and potential density space for the year 2014. Surface density flux differences between the satellite derived thermodynamic and kinematic approaches and ECCO (an ocean state estimate) underline: 1) air-sea heat fluxes dominate variability in the thermodynamic approach; and 2) fine scale structures from the satellite derived kinematic approach are most likely geophysical and not artefacts from noise in SSS/T or SSC—as suggested by a series of smoothing experiments. Additionally, ECCO revealed surface density flux integrated over ML are positively biased as compared to similar estimates assuming that surface conditions are homogeneous over ML—in part owing to the e-folding nature of shortwave solar radiation. Major differences between the satellite derived kinematic and thermodynamic approaches are associated to: 1) lateral mixing and mesoscale dynamics in the kinematic framework; 2) vertical excursions of, and vertical velocities through the ML base; and 3) interactions between ML horizontal velocities and ML base spatial gradients.
1 Introduction
Walin (1982) initially established a framework in which interior circulation could be deduced from knowledge of atmosphere-ocean thermal interactions and sea surface thermal state. This process-based framework was further developed by Tziperman (1986) to include Sea Surface Salinity (SSS) and air-sea freshwater fluxes in a buoyancy-based thermodynamic water mass (trans)formation (WMT/F) framework. Another WMT/F framework is based on material changes of ocean variables, such as temperature, salinity and density (kinematic framework) (Iudicone et al., 2008; Li et al., 2017; Groeskamp et al., 2019; Nurser and Griffies, 2019-04). As this framework obviates the need for air-sea flux data, it is perfectly suited to take advantage of the high spatio-temporal resolution data now offered by satellites.
Piracha et al. (2019) applied the thermodynamic WMT/F framework in density, SSS/Sea Surface Temperature (SST) and geographic space to identify and trace winter cooling associated water masses in the Southern Ocean, North Pacific and North Atlantic. Through in-situ datasets of net heat fluxes (HF), obtained by Voluntary Observing Ships (VOS) and satellite datasets of net freshwater fluxes (FWF), SSS and SST, they found that satellite-derived datasets showed a salinification and warming in all water masses studied as compared to in-situ data from ARGO, linked to increases in the water cycle over the two years (2011-2012) studied.
Recent studies have shown two sources of uncertainties in air-sea flux estimation; the bulk algorithms used; and variables taken as the algorithms input (Brunke et al., 2011). Despite many advancements in the field of deriving specific humidity from satellite observations (Tomita et al., 2018), uncertainties in their estimates are numerous (Cerovečki et al., 2011-12). As evaporation depends on Latent HF (LHF), errors in LHF will propagate to evaporation (Gutenstein et al., 2021). Furthermore, the estimation of air-sea HF is sensitive to wind speed. Through an inter-comparison of 11 HF products, Brunke et al. (2011) showed that in the presence of high wind speeds, such as those found near the Western Boundary Currents (WBC) HF estimates from the various sources tended to disagree considerably.
We aim to show that the WMT/F framework can be more accurately and completely estimated, in terms of geophysical effects, when utilised in a kinematic rather than thermodynamic definition. To accomplish this, we first compare thermodynamic density flux between HF and FWF from satellites, in-situ observations and an ocean state estimate described in section 2 to understand dominant term(s) in density flux variability. The mathematics underlying the thermodynamic and kinematic WMT/F framework are described in detail. Futhermore, the modifications of our kinematic framework with respect to Iudicone et al. (2008) and the underlying assumptions are stated where necessary, paying close attention to the physical processes being resolved (section 3).
How uncertainties in air-sea and sea surface satellite observations and underlying assumptions made in the kinematic framework influence the density flux over the Southern Hemisphere (SH) is assessed in a series of comparisons with an ocean state estimate (section 4). The advantage of the kinematic over the thermodynamic WMT/F framework based purely on satellite observations is assessed with respect to physical processes and geophysical phenomena in section 5. The impacts of the current study with respect to SH water masses are discussed along with a summary of the main findings (section 6).
2 Datasets
All datasets are remapped to 0.25˚ using a Gaussian inverse-distance weighted interpolation scheme, in which the weights decrease exponentially with distance.
The weighting function has the following form.
Where, λ and ϕ are the longitude and latitude, respectively, d is the distance in Km from a point in the new (interpolated) grid from each point in a 3x3 window of the original grid where data values are known. σ is the resolution of the target grid (i.e. 0.25˚) in Km. The sum of the products between the data values in the original grid and weights (equation 1) for each respective point in the 3x3 window in the original grid (centred on the point in the interpolated grid) is calculated. This sum is then divided by the sum of the weights within the respective 3x3 window in the original grid. Thus, providing the interpolated value at each respective point in the new grid.
All datasets are subsequently converted to a monthly temporal resolution covering SH to the annual maximum sea-ice edge.
2.1 Satellite products
2.1.1 Sea surface temperature and salinity
The Level 4 Optimal Sea Surface Temperature and Sea Ice Analysis (OSTIA) SST product from the UK Met Office was constructed using objective analysis applied to a blend of satellite and in-situ sources as well as information on sea ice from the European Organisation for the Exploitation of Meteorological Satellites (EUMETSAT) (Donlon et al., 2012). The dataset is operationally produced at daily temporal resolution and at a spatial resolution of 0.05˚. The datasets are hosted on the ftp servers of the Copernicus Marine Environmental Monitoring Service (CMEMS; ftp://nrt.cmems-du.eu).
The Barcelona Expert Center (BEC) produces level 4 SSS maps at daily temporal resolution from the Microwave radiometer data aboard the European Space Agency Soil Moisture and Ocean Salinity satellite (SMOS) (Olmedo et al., 2021). The product has global coverage over the ice-free global oceans with spatial resolution identical to that of the OSTIA level 4 SST. Datasets were constructed using a debiased non-Bayesian approach at daily time scales (Olmedo et al., 2017). The SMOS level 4 SSS from BEC is quoted in Practical Salinity Units (PSU) which were converted to Absolute Salinity Units. The SSS dataset is available from the BEC ftp server at becftp.icm.csic.es
2.1.2 Sea surface current
Global near-surface currents were provided by the NASA Jet Propulsion Laboratory (JPL) Ocean Surface Current Analysis Real-time (OSCAR) project using a blend of satellite and in-situ sources to achieve daily mean fields at 0.25˚, making use of a simplified turbulent surface Mixed Layer (ML) model (Bonjean and Lagerloef, 2002). Sea surface currents are averaged over the top 30m of the ocean in units of ms–1. The data is hosted on the NASA Physical Oceanography Data Access and Archiving Center (PODAAC) servers and can be found at https://podaac.jpl.nasa.gov/.
2.1.3 Heat fluxes
The Japanese Ocean Flux (JOFURO) project uses satellite datasets to derive the turbulent and radiative components of the HF (Tomita et al., 2019). HF is calculated with satellite-derived SST, surface wind speed and specific humidity, and provided as daily means at 0.25˚. Furthermore, the COARE3.0 bulk algorithm was used to estimate surface turbulent HF. The radiative HF were taken from other projects. The datasets were downloaded from the JOFURO project page at https://j-ofuro.isee.nagoya-u.ac.jp/en/.
2.1.4 Freshwater fluxes
Net FWF is the difference between Objectively Analysed Air-sea Flux (OAFLUX) evaporation and Global Precipitation Climatology Project (GPCP) precipitation datasets (Yu et al., 2008; Nelkin et al., 2021). Daily mean fields of precipitation (P) were obtained from NASA’s GPCP project [GPCP Precipitation data can be found at https://disc.gsfc.nasa.gov/datasets (Huffman et al., 2015)]. Global P fields were calculated using satellite retrievals from the Global Precipitation Measurement (GPM) mission and in struments aboard the Television InfraRed Operational Satellite (TIROS). The final product has a spatial resolution of 0.5˚. The GPCP data are downloaded from https://measures.gesdisc.eosdis.nasa.gov/.
The Woods Hole Oceanographic Institute’s (WHOI) OAFlux project provides datasets of evaporation (E) calculated from latent HF derived using the COARE3.0 bulk formula (Yu et al., 2008). OAFLUX is a synthesis of satellite observations from scatterometers (wind speed), the Special Sensor Microwave Imager (for specific humidity [SSM/I]) and radiometers for high resolution SST fields. OAFLUX is available as daily mean 1˚ fields of evaporation over the ice-free global ocean. OAFLUX evaporation fields were downloaded from the project page at https://oaflux.whoi.edu/.
2.2 In-situ observations
2.2.1 Heat fluxes
The Southampton National Oceanography Center (NOCS) provides turbulent and radiative components of HF at 1˚ spatial and monthly temporal resolution (Berry and Kent, 2009). The dataset was constructed as an optimal interpolation of atmospheric and sea state observations via VOS from the International Ocean-Atmosphere Comprehensive DatasetS (ICOADS) version 2.4/5. NOCSv2 uses the bulk formulas from Smith (1988) for the turbulent fluxes and Reed (1977) for radiative fluxes.
2.2.2 Freshwater fluxes
We calculate in-situ E from NOCS LHF utilising the following relation.
Henderson-Sellers (1984) put forward an equation in which the latent heat of evaporation of water (LE ) in Jkg–1 is dependent on SST.
E calculated this way will have units of kgm–2s–1. The data can be found on the Research Data Archives (RDA) of the University Centers for Atmospheric Research (UCAR) at https://rda.ucar.edu/datasets/.
The NASA Integrated Multi-satellitE Retrievals for GPM (IMERG) dataset is an assimilation of P data from multiple sources including satellites and rain-gauges. It integrates various passive microwave and infrared sensor P retrievals aboard the GPM virtual constellation of satellites (Huffman et al., 2015). Gridded P fields were constructed from the 2017 Goddard Profiling algorithm globally and daily from 2000-2021 over ice-free regions of the ocean at 0.5˚ and can be found on the Copernicus Climate Data Servers (CDS) at https://cds.climate.copernicus.eu.
2.2.3 Mixed layer depth
The Japanese Agency for Marine-Earth Science and Technology (JAMSTEC) provided 1˚ datasets for ML depth (MLD) at 10-day averages derived from Argo float profiling data (Hosoda et al., 2010). The MLD was defined as the depth with which density becomes 0.03kgm–3 denser than at 10m depth. Being based on ARGO, and due to the spatial coverage of ARGO floats, certain 1˚ bins had more data than others (some areas also had no data due to an absence of floats). To mitigate this effect an objective analysis following the method of Zweng et al. (2010) was performed, however, with influence radii of 888, 666 and 444 km, to obtain complete global coverage.
2.3 Ocean state estimate
Reconstruction of the 3-D time dependent ocean and sea-ice state, Estimating the Circulation and Currents of the Ocean (ECCO) provides data of Salinity, Temperature, HF, FWF, Currents and MLD globally (Fukumori et al., 2017). The state estimate covers the period 1992 to 2018 and provides datasets on approximately a 1˚ spatial resolution (20 to 110 km in length) with 50 vertical levels (from the surface to 6145m) of varying widths from 10m to 456.5m. ECCO both reproduces remotely sensed and in-situ datasets within their respective uncertainties and satisfies the laws of physics and thermodynamics, thereby, providing data which is dynamically consistent. The state estimate derives from a global configuration of a 1˚ general circulation model provided by the Massachusetts Institute of Technology (MITgcm) (Dataset et al., 2017). ECCO is, therefore, perfect for application to analysis of budgets within the ocean (Forget et al., 2015).
Satellite forcings from various sensors are assimilated into the ECCO state estimate, including altimeters for sea surface height, radiometers for SSS (from NASA’s Aquarius mission (Entekhabi et al., 2010)) and SST, as well as scatterometers for sea surface wind speed. In-situ sources such as from ARGO floats, Conductivity-Temperature-Depth (CTD) casts, marine mammals and autonomous gliders were used for temperature and salinity profiles.
MITgcm calculates turbulent fluxes following Bryan et al. (1996) using atmospheric state variables (temperature, humidity and saturated water vapour pressure) and SST. Additionally, ECCO derives ML depth (MLD) from temperature profiles following the threshold criteria by Kara et al. (2000).
ECCO data are hosted by the NASA PODAAC servers and can be found at https://podaac.jpl.nasa.gov/.
3 Methodology
The thermodynamic density flux describes the density change of surface waters by air-sea fluxes (Walin, 1982; Tziperman, 1986; Speer and Tziperman, 1992). Whereas, this thermodynamic approach only includes HF and E-P, the kinematic approach accounts for additional geophysical forcings (ice-sea/land-sea fluxes of heat and salt and turbulent processes). Furthermore, by considering MLD, the kinematic approach additionally resolves entrainment through the base of ML (MLB).
Both relations hinge on accurate definitions of Sea Surface Density which is here defined as the locally referenced potential density ρl defined by McDougall et al. (2014) in kgm–3.
The current state of water mass analysis is reviewed in depth by Groeskamp et al. (2019).
3.1 Thermodynamic density flux
The thermodynamic relation for density flux in discrete form is
Where f is the density flux in latitude-longitude (ϕ - λ , respectively) coordinates. ℋ is the sum of turbulent and radiative components of HF and Q is E-P. They have units of Wm −2 and ms −1, respectively. Cp is the specific heat capacity of water (assuming constant of 4000 Jkg −1∘C−1 ). Therefore, f has units of kgm −2 s −1 . α and β are thermal contraction and haline expansion coefficients, respectively. They are computed at each λ−ϕ point using the 75-term polynomial expression detailed in Roquet et al. (2015).
We evaluate the thermodynamic relation using SSS and SST from satellite sources (section 3.2.2), respectively. In addition, we consider multiple sources of HF and FWF from; a state estimate (section 3.2.2); in-situ sources (sections 2.2.1 and 2.2.2, respectively); and satellite datasets (sections 2.1.3 and 2.1.4, respectively).
4.2 Kinematic density flux
4.2.1 Material evolution of scalar ocean variables
Following Iudicone et al. (2008), the material evolution of an ocean tracer can be discussed in terms of physical processes (interior mixing and surface forcings) which alter tracer properties.
Where γ is an arbitrary ocean tracer, dγ and fγ describe the evolution of γ due to mixing and surface forcings. The mixing and forcing term describe interior mixing and air-sea forcings, respectively. When γ is replaced by ρl equation 5 becomes
Where, κ is the eddy diffusivity in m2s−1 . When considering ML, an additional term is introduced as hρl —representing both the vertical excursion of MLB and interactions between the background flow and MLB spatial gradients. Equation 6 then becomes.
Therefore, the residual between and f becomes.
Where h is MLD and subscript −h denotes measurement taken at the MLB. The control volume considered is ML. represents the 3-dimensional velocity vector.
An analysis of the right hand terms in equation 8 is not performed in the current study (see Kim et al. (2006) for an evaluation of entrainments effect on ML temperature budgets). Nevertheless, we try to describe the results by the physical processes they represent.
3.2.2 Numerical approximation
We average terms without temporal derivatives over two time-steps to keep consistent temporal grids. Spatially, we perform an extrapolation before taking a centred spatial derivative to avoid grid shifts or loss of coverage. In one spatial (x ) and time (t ) dimension, the numerical material derivative becomes.
Where ux,t is velocity in the x direction. However, equation 9 makes no reference to observations below the surface. This highlights that we assume surface observations are homogeneous over ML (see section 4.3). Thus, the thermodynamic and kinematic approaches are equated in the following way.
The material derivative of ρl has units of kgm −3 s −1 as opposed to kgm −2 s −1 for the thermodynamic approach (equation 4). This discrepancy in units is resolved by taking the product of the material derivative with MLD.
To evaluate the kinematic approach, we use a satellite based approach, utilising SSS, SST and SSC (sections and, respectively) and a state estimate based approach (section 3.2.2, which contains all variables needed to compute the kinematic framework). For the satellite based approach we used in-situ derived MLD (section 2.2.3). The state estimate additionally contains all variables (salinity, temperature, currents) on 50 pressure levels (section 2.3) which were exploited to understand the impact of a surface definition of the kinematic approach (i.e. assuming that ML is homogeneous).
3.3 Water mass (trans)formation in density and geographic space
Transformation is defined as the volume transport through each respective density class. In practice, transformation requires a discretisation in terms of ρl . Convergences of transformation result in the WMF of water within a specific density range; allowing for interior circulation to be inferred.
However, geographic information is lost in discretising the ocean with respect to ρl . Discrete isopycnals have widths of 0.05kgm −3 (Δρl ) between 1020-1030 kgm −3 .
Where A is the outcropping isopycnal area (m 2 ). The boxcar function (∏) is 1 in discrete outcropping isopycnal regions () and zero otherwise. Trans(formation) is defined in Sverdrup (Sv) (1 Sv = 10 6 m 3 s −1 ).
WMF (given by M in Sv) occurs via a net down-welling (subduction) as opposed to up-welling (destruction) through MLB. This allows interior circulation to be inferred via only surface conditions.
The density class between two consecutive density classes is (i.e. ).
To disentangle geographic information from equation (12), we follow the technique outlined in Brambilla et al. (2008) and Maze et al. (2009). Annually averaged maps of formation are subsequently estimated via a sum over all time steps considered—in our case 12 monthly time steps.
Where A represents the discrete outcropping isopycnal region (m2 ) and t refers to the month number.
To ρl -space (trans)formation estimates, a 2-point running window is applied to reduce noise related from calculating the material derivative.
4 Sensitivity analysis
We compare satellite and ECCO derived thermodynamic and kinematic density flux to assess differences arising from using satellite datasets. Furthermore, to highlight the impact of assuming that surface variables are homogeneous over ML, we compare kinematic density flux from ECCO defined at the surface and integrated over ML.
4.1 Sensitivity of the thermodynamic approach to heat and freshwater fluxes
Figure 1 shows the thermodynamic density flux calculated using ECCO and satellite SSS/T with satellite, in-situ and ECCO HF and FWF. Overall, a purely satellite based thermodynamic density flux shows strong signals of density gain being restricted to SH western boundary currents (the Agulhas at 40S-35E, Brazil Current 40S-50W and East Australia Current at 35S-140E) as well as significant positive density flux being associated to the Lewin Current off the west coast of Australia (20S-110E). In contrast, ECCO shows more widespread density gain over SH. Especially in the temperature component, satellites show much stronger gains in buoyancy—with differences being especially striking in the Indian Ocean (IO). Therefore, implying satellite HF are stronger than ECCO HF.
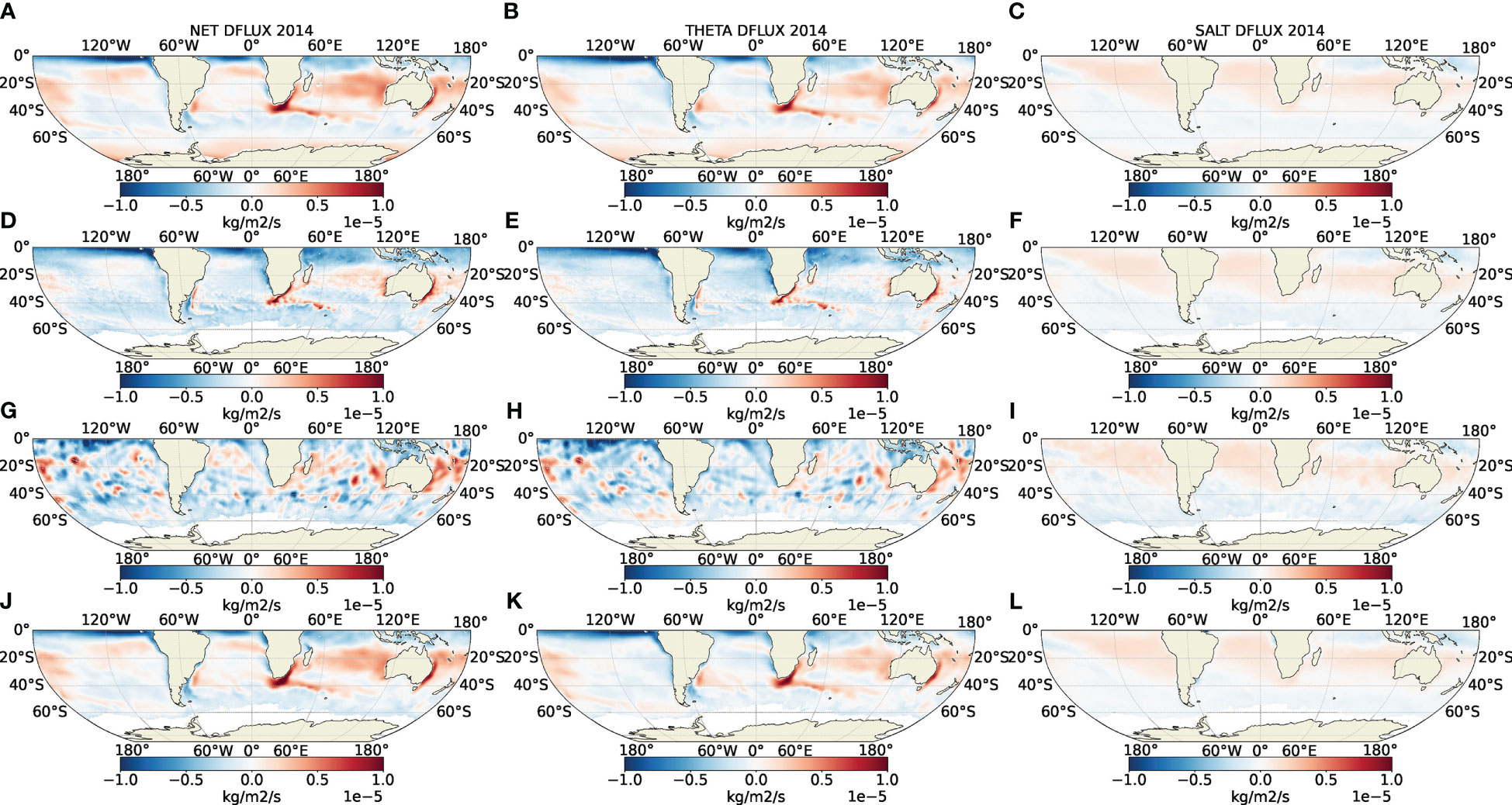
Figure 1 Annually averaged thermodynamic density flux (equation 4) derived from all ECCO inputs (first row), all Satellite inputs (second row), satellite SSS,SST along with in-situ fluxes (third row), satellite SSS,SST with ECCO fluxes (fourth row). Net density fluxes (left column) are split into a thermal (middle column) and haline (right column) forced density flux.
This becomes apparent when density flux is derived using ECCO fluxes and satellite SSS/T and compared to a pure ECCO derived density flux. The results are almost identical and, furthermore, the haline forced density flux is similar between both. Suggesting that differences in HF affect density flux disproportionately (as compared to FWF).
As the in-situ fluxes are based on observations from VOS, interpolations performed in generating the flux datasets result in differences with respect to the other approaches. Looking at the thermal and haline components, it becomes clear that the thermal component of density flux is where most differences with respect to ECCO originate; the haline component is very similar to the purely ECCO based thermodynamic density flux.
4.2 Kinematic approach sensitivity to noise in satellite observations
Figure 2 aims to show how a purely satellite and ECCO definition of the kinematic density flux compare. Our first goal is to show areas of significant differences. We then try to analyse whether those differences were an effect of noise in the satellite datasets or a resolution of some geophysical processes. To achieve the latter goal we spatially average satellite kinematic density flux with a series of increasing bin widths, therefore, significantly dampening the effect of noise uncovering geophysical signals.
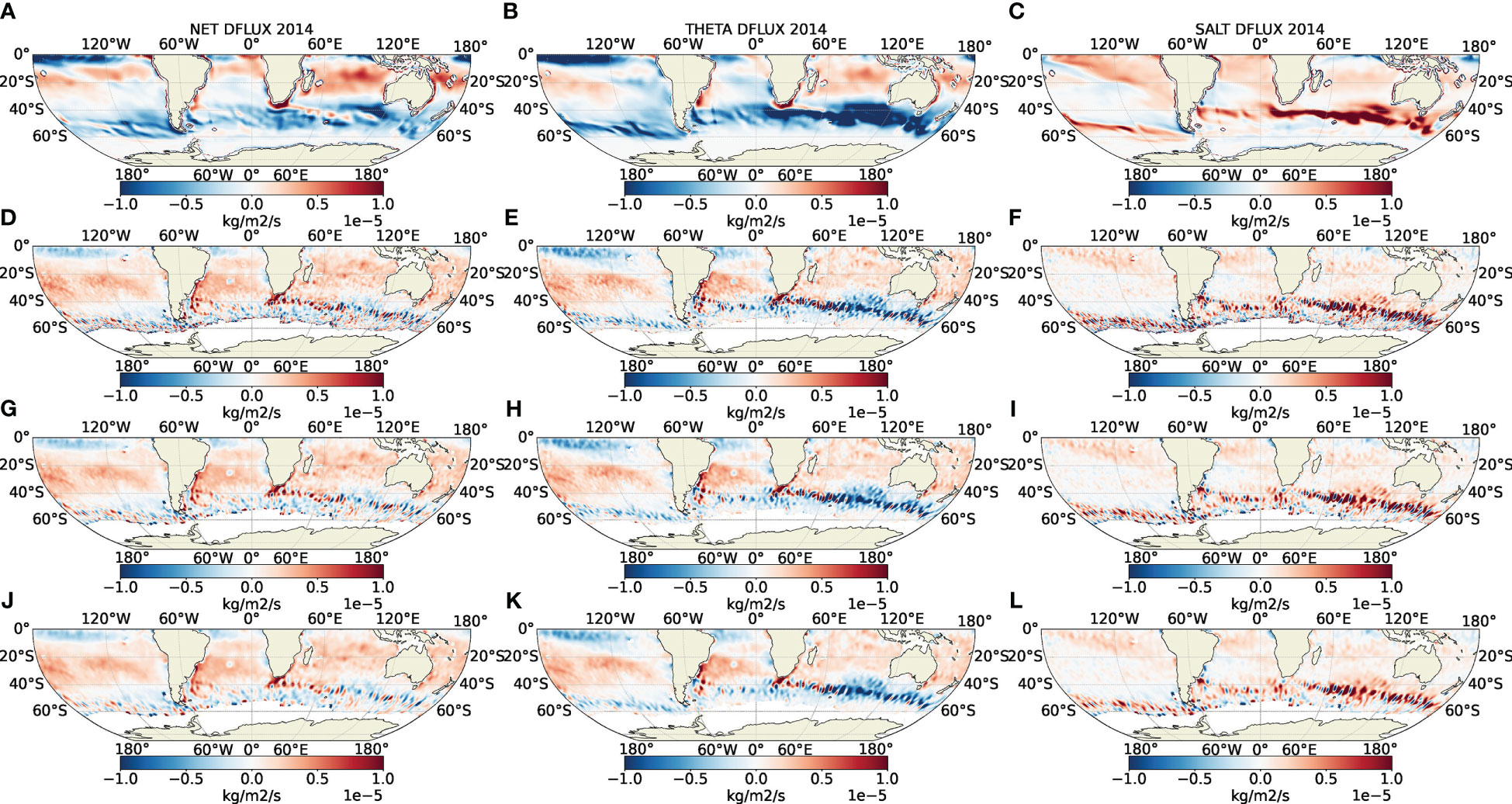
Figure 2 Annually averaged kinematic density flux (equation 10) derived from all ECCO inputs (first row), all Satellite inputs (second row) with no smoothing applied, binned using a 7x7 window (third row), binned using an 11x11 window (fourth row). Net density fluxes (left column) are split into a thermal (middle column) and haline (right column) forced density flux.
ECCO based density flux is also much smoother than similar results based on satellites, which contain more granularity. This granularity is mostly seen south of the Sub-Antarctic Front (SAF), where they are noted to be dynamically active regions of mesoscale eddy activity. Furthermore, interactions between the strong westerlies associated with the Antarctic Circumpolar Current (ACC) and mesoscale surface currents affect large scale ocean circulation (Song et al., 2020).
To assess the source of this granularity, we smooth the satellite kinematic density flux with two window lengths each of increasing size.
Although, some of the granularity is reduced when considering the largest bin width, significant alternating signals of density flux remain throughout the ACC (south of 40S). However, this signal is smoothed, regular and defined, suggesting a geophysical origin. Thus, it is likely that the signal seen is more a resolution of geophysical processes than noise from the satellite datasets propagating to kinematic density flux estimates.
4.3 Sensitivity of the kinematic approach to mixed layer depth
What is the consequence of assuming that surface conditions are representative of ML. This question is fundamental in our satellite based approach to the WMT/F framework.
The analysis employed consists of comparing two versions of an ECCO derived kinematic framework (Figure 3). These are; 1) computing the product between the material derivative at surface level (5m depth) and MLD; and 2) computing the material derivative at each level within, and integrating over, MLD with respect to the depth of each layer.
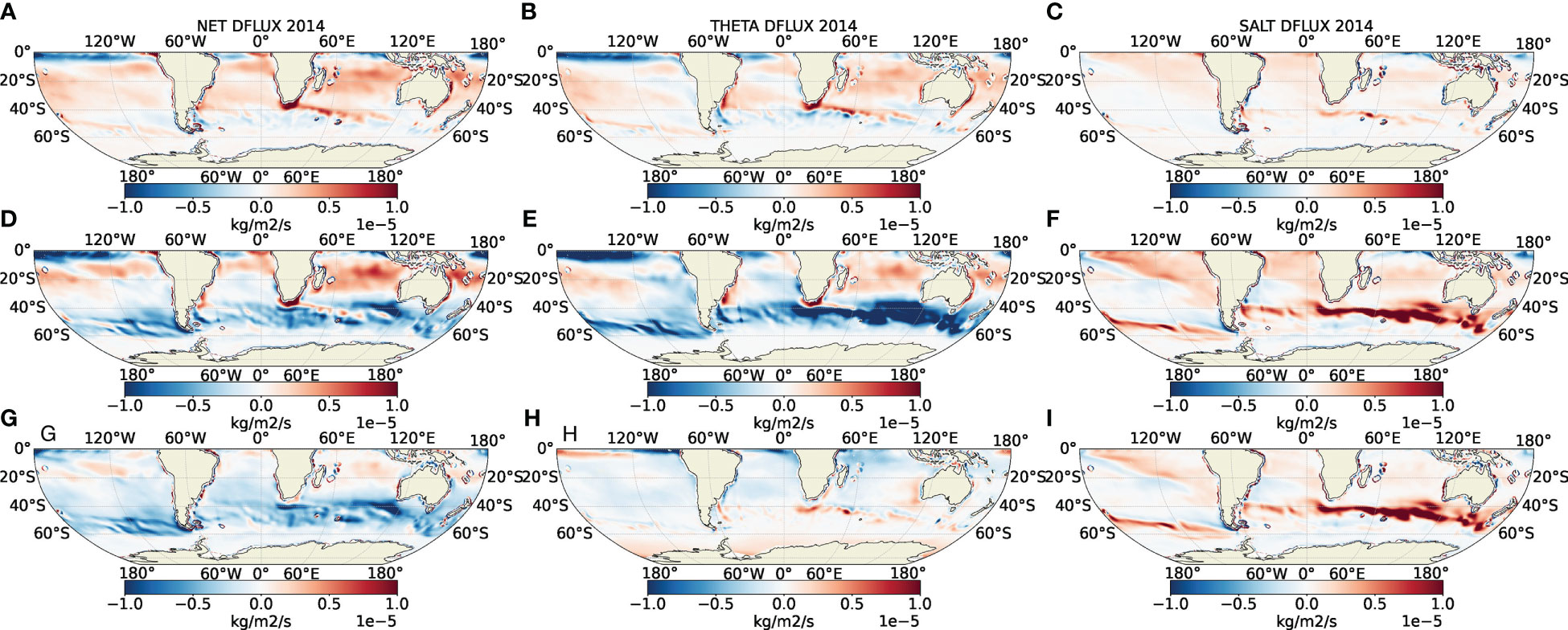
Figure 3 Annually averaged kinematic density flux derived using all ECCO inputs integrated throughout ML (first row) (equation 15) and at the surface layer (second row) (equations 10). The difference between the surface and ML integrated net, thermal and haline kinematic density flux is also shown (third row). Net density fluxes (left column) are split into a thermal (middle column) and haline (right column) forced density flux.
Where i is the depth index, δz is the width of each layer, z is the depth and n is the number of depth layers—which is 50 for ECCO.
The boxcar function ([symbol]) is 1 within MLD and 0 otherwise.
Equatorial (North of 15S) and mid-latitude (between 15-40S) differences are most pronounced in the thermal component, where surface results are similar and negative compared to ML integrated results, respectively. Especially for HF, their penetrative nature with depth is shown to have a non-negligible impact on WMT/F and, therefore, density flux (Iudicone et al., 2008). South of the SAF, negative/positive differences between surface and ML integrated definitions appear in the thermal/haline component. This region is marked by enhanced mesoscale activity, eddy dynamics and strong current-wind interaction resulting in the signal of strong density flux. These signals dampen rapidly with depth explaining the weakly negative ML integrated density fluxes.
ML integrated results are generally much weaker and less defined than a surface definition, suggesting physical processes occurring at the surface becoming weaker with depth. Specifically, Iudicone et al. (2008) has shown that in estimating WMT/F, ignoring the penetrative nature of shortwave solar radiation results in an overestimation of internal mixing.
5 Results
5.1 Density flux
The kinematic net density flux shows stronger density gains over SH than the thermodynamic approach from satellite fluxes (Figure 4). Significant differences arise in SST contributions, where the kinematic buoyancy forcing shows major density gains throughout much of SH compared to the thermodynamic thermal density flux.
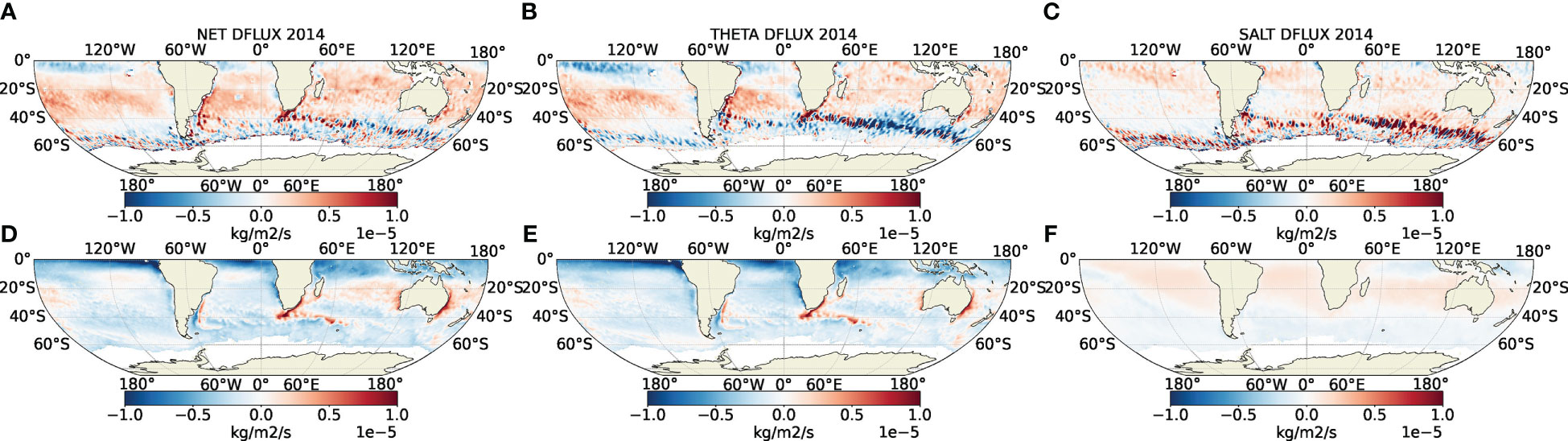
Figure 4 Annually averaged density flux during 2014 across SH from the equator to 70S. Density fluxes have been derived from a kinematic (first row) (equation 10) and an thermodynamic approach based on satellite (second row) (equation 4) derived air-sea heat and freshwater fluxes. The net density flux (left column) is split into a thermal (middle column) and a haline (right column) component.
For example, one such place of significant overestimation of the kinematic approach relative to the thermodynamic approach is the Sub-Tropical Gyre in SA. Here, the buoyancy gain in the thermodynamic approach is most likely caused by a signal of surface heating—as HF have previously shown as the dominant term in setting the net thermodynamic density flux. Thus, air-sea fluxes have the effect of reinforcing this signal of buoyancy gain seen in the thermodynamic approach. With the kinematic approach we have the additional resolution of lateral mixing through MLB combined with ML dynamics plus lateral induction of fluid into ML (right hand side of equation 8). In other words, lateral mixing and diffusion in general act downgradient (i.e. opposing the signal of buoyancy established through the heat fluxes) and in doing so result in a signal of increasing density.
Furthermore, the salinity contributions to density flux generally match between both approaches. Major differences between both approaches are visible as a much stronger signal of density flux around Southern Ocean close to the SAF from 45-65S in the salinity contribution. Furthermore, the westerlies south of 40S that drive ACC cause increased mesoscale turbulence (in the form of eddies), and along with interactions between the sloping MLB and the northward Ekman transport (driven by the westerlies) cause an induction of warm, saline waters into ML—as suggested by the positive and negative density flux seen in the haline and thermal driven density flux south of 40S in the kinematic approach, respectively. These processes are additionally resolved in the kinematic approach, which is also evident by the granular nature of the density flux south of 40S; which is of coarse partly due to noise in the satellite retrievals in polar regions, however, as suggested by section 4.2, the majority of the signal is a resolution of geophysical effects.
In terms of zonal averages (Figure 5), the equatorial regions in the net and thermal kinematic density flux show much less buoyancy gain then their thermodynamic counterparts. The most likely explanation is that air-sea heat fluxes over the equatorial regions up to the mid-latitudes reinforce a signal of density loss over ML (through surface heating). However, when lateral mixing is taken into account in the kinematic density flux, the gradient of increasing density from the equator to the mid-latitudes is significantly reduced with respect to the thermodynamic approach—owning to the down-gradient nature of lateral mixing acting to smooth gradients reinforced by air-sea fluxes.

Figure 5 Annual zonally averaged density flux for 2014. Showing the net density flux (A) made up of thermal (B) and haline (C) contributions. The kinematic (equation 10) and thermodynamic density flux (equation 4) is shown as a black and red solid line, respectively.
Moreover, in the mid-latitudes, net and thermal kinematic density flux are much stronger than from the thermodynamic approach using satellite derived fluxes. This is due to mid-latitude WBC experience strong cooling and thus a rapid deepening of MLB—the formation mechanisms for mode waters. This process is responsible for entrainment through the winter MLB and has been cited as a major factor responsible for the ventilation of ML (Williams et al., 1995). However, the thermodynamic approach shows that instead of positive density flux the mid-latitudes experience buoyancy gain, suggesting that satellite heat fluxes are positively biased, resulting in negative density flux biases with respect to the kinematic approach.
Interestingly, zonal averages of the haline density flux approximately match north of 35S with both approaches showing a haline driven density gain around 15S ± 10 which is probably a resolution of the salinity maximum region which is driven by positive E-P resolved both in the thermodynamic and kinematic approaches. However, south of 35S both approaches diverge with the kinematic approach estimating another peak in the haline driven surface density gain around 45-50S close to the SAF. At the same location, the thermodynamic approach shows a completely different picture with a weak haline driven surface density loss. This can be explained by the westerlies and the wind-driven currents south of 40S result in increased mesoscale turbulence generated through eddies, which results in increased lateral mixing in ML. As this is resolved in the kinematic approach unlike the thermodynamic approach, part of the stronger density gain signal is geophysical in nature. However, the erratic nature of the kinematic density flux poleward of 60S in the kinematic haline density flux seems to be a manifestation of the granular nature of the geographic kinematic density flux close to the sea-ice edge (Figure 4). In which case, the zonal average of the haline kinematic density flux resolves both a signal of noise and the geophysical effect of entrainment through the MLB, caused by interactions between the westerly driven northward Ekman transport interacting with spatial gradients of ML across the ACC frontal region.
5.2 (Trans)formation in density space
Transformation rates (F(ρl) ) are estimated from equation 11 over SH from 30S-70S and shown in Figures 6A–C. Overall, thermodynamic net transformation rates from all flux sources show a similar pattern to the kinematic approach, however, with marked differences. Namely, both satellite and in-situ fluxes show negative transformations in all regions of SH and throughout all isopycnals, whereas the kinematic approach shows clear positive (negative) transformation peaks lighter (denser) than ρl = 1026.4 kgm −3 .
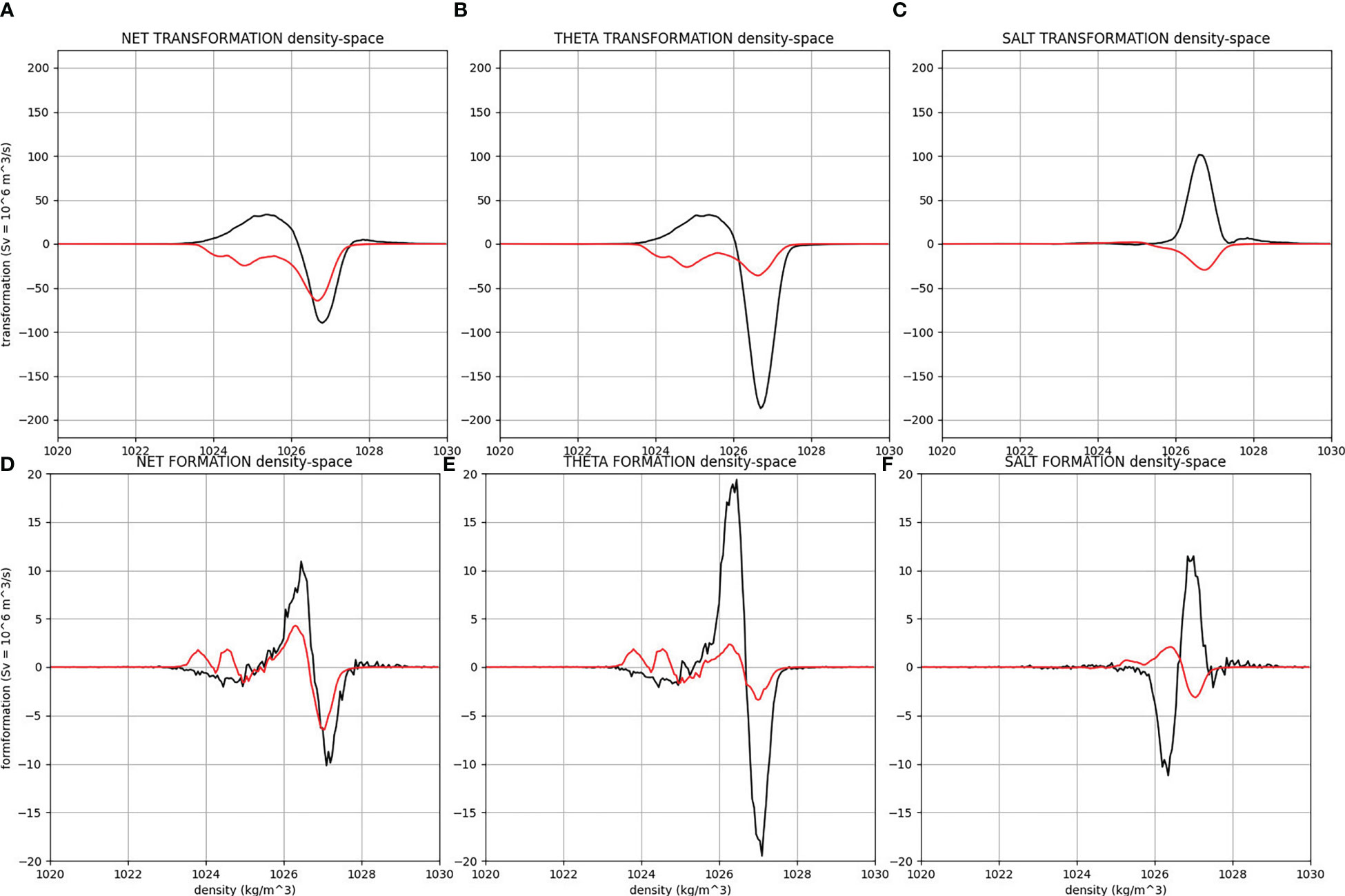
Figure 6 Annually averaged transformation (first row) (equation 11) and formation (second row) (equation 12) in density (ρl ) space. The net (trans)formation (left column) is broken down into a thermal (middle column) and a haline component (right column). The solid-black and red curves represent the kinematic (equation 10) and thermodynamic approaches (equation 4), respectively. Where the thermodynamic approach is estimated from satellite (solid-red curve) fluxes.
Across the entire SH (30-70S), positive formation (Figures 6D–F) generally peaks between 1026-1026.5 kgm −3 with the densest and highest peak occurring as water from ML passes into the interior ocean at a rate of 10 Sv according to the kinematic approach, whereas a similar 10 Sv of fluid ventilates ML in isopycnals denser than 1027 kgm −3 . Net kinematic formation is seen to be similar but weaker to the thermal kinematic formation, owing to the weakly compensating kinematic haline formation. Thus, the kinematic net formation shows a formation and subduction of a warm, salty water mass between 1026-1026.5 kgm −3 and an upwelling of cold freshwater in isopycnals denser than 1027 kgm −3 .
On the other hand, although a similar picture is painted by the net thermodynamic formation with slightly weaker positive formations driving less subduction—which gives more weight to the argument that the heat fluxes are positively biased. This is made up of a similar contribution of thermal and haline thermodynamic formation. This suggests that the air-sea freshwater flux forced formation is strongly opposed by upwelling due to ML dynamics and westerly driven turbulence inducing lateral mixing. Furthermore, these isopycnals (denser than 1026 kgm −3 ) outcrop within the region of the ACC frontal system, where the influence of the westerlies, both with its ability to induce turbulence in ML and through its induced northward Ekman transport causes significant transfer of fluid through MLB.
5.3 Formation in geographic space
Regional formation within a specific range of outcropping isopycnals is mapped in Figure 7. These isopycnal ranges reflect the densification of the Sub Antarctic Mode Water westward from the lightest outcropping densities in IO (1026.45-1026.75 kgm −3 ) getting progressively denser in SA (1026.55-1026.85 kgm −3 ) and finally reaching their maximum density in SP (1026.75-1027.05 kgm −3 ). The density ranges of the various versions of the Sub Antarctic Mode Water are from Li et al. (2021)).
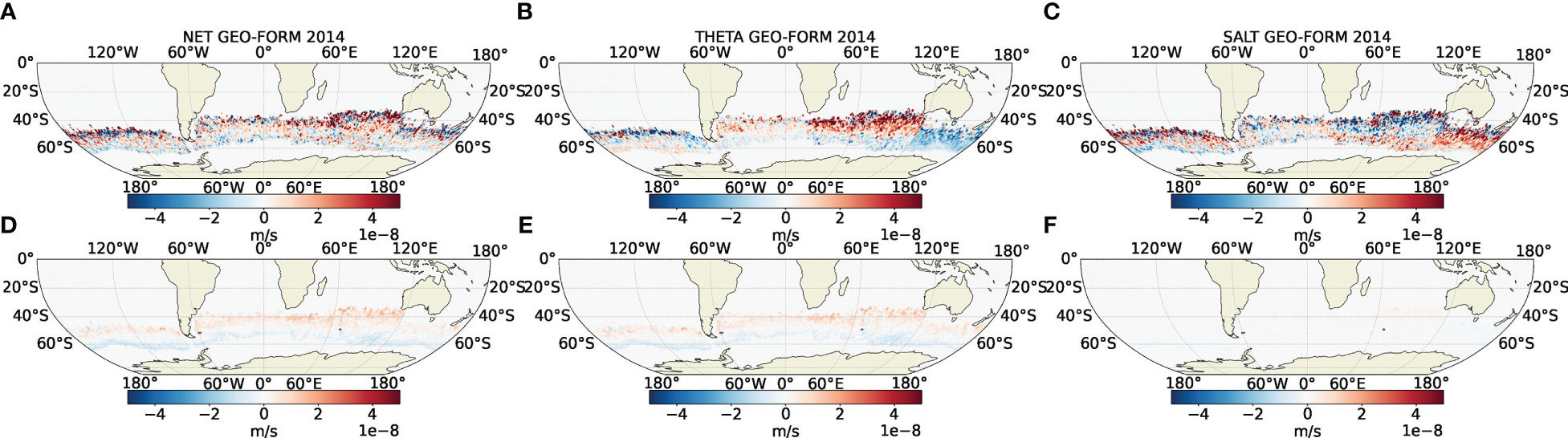
Figure 7 Annually averaged geographic distribution of formation (equation )?? across the outcropping region of the 1027 kgm−3 isopycnal. Formation has been derived from a kinematic (first row) (equation 9) and a thermodynamic approach (equation 4) based on satellite (second row) fluxes. The net geographic distribution (left column) is split into a thermal (middle column) and a haline (right column) component.
There is a prevailing bi-modal pattern with positive/negative formation north/south of ~ 40S from both the thermodynamic and kinematic approaches to net geographic formation. This suggests that in IO at around 40S a cool, fresh water mass is subducted below ML in the kinematic geographic formation. Furthermore, south of 45S the ML is ventilated by upwelling of warm, haline water. A possible hypothesis is northward Ekman transport driven by the westerlies around the latitude of the Drake Passage cause water to leave ML forming a cool fresh water mass. South of around 45S, forced by ML dynamics, warm, salty water is entrained into ML.
A striking finding is that, although the net kinematic and thermodynamic geographic formation tend to agree qualitatively, this is almost entirely influenced by thermal formation according to the thermodynamic approach as opposed to a joint thermal and haline influence from the kinematic approach. Therefore, we can assume that haline formation is entirely the result of ML entrainment and dynamics between the northward Ekman transport and the sloping MLB near within the ACC frontal system as the surface flux forced formation is negligible—as shown by thermodynamic haline formation.
6 Discussion and conclusion
We have highlighted the differences between the different approaches to calculate water mass dynamics throughout SH in 2014 with the aim to show the enhanced resolution of water mass dynamics offered by satellite observations. Surface density flux and WMT/F were estimated with two different methodologies: on one hand, the traditional air-sea flux approach, which is termed the thermodynamic approach using HF and FWF (E-P) (Tziperman, 1986) derived from three different sources of data (in situ, satellite and numerical models); on the other hand, we have used a kinematic approach based on the material evolution of sea surface variables, which is a modified version of the approach by Iudicone et al. (2008) taking into account the varying MLB and applied for the first time to satellite observations.
Overall, our approach is a modified version of the framework outlined by Iudicone et al. (2008). This is due to the definition of MLD and the evolving ML base which results in the additional contribution from entrainment—driven both by vertical velocities at the MLB and the vertical excursion of MLB throughout the year and interactions between horizontal velocities and spatial gradients of MLB which act to laterally induct fluid into the ML, respectively.
When looking at the geographic distribution of formation, we see that these isopycnals are associated to the SAF and more generally the ACC. Furthermore, this area of the ACC frontal system is marked both by the westerly driven ACC and the northward Ekman transport it induces, which, by equation 8, suggest that entrainment through MLB caused by vertical movements of ML driven by mesoscale eddy induced turbulent mixing (a result of interactions between the westerlies over the ACC and the eastward current it drives) and induced entrainment caused by the northward Ekman transport interacting with the sloping MLB are significant contributors to WMF.
SSS within the ACC is highly variable and brightness temperatures have low sensitivities to SSS (Martínez et al., 2022). Therefore, the signal of noise becomes a greater concern for satellite SSS retrievals in cold water regions (Fournier et al., 2019; Martínez et al., 2022) such as the ACC region. More than this, the OSCAR surface current product is known to suffer from issues related to velocity variance poleward of 10˚ with respect to in-situ data (Johnson et al., 2007) and as surface currents is needed for the calculation of the material derivative this could adversely impact kinematic estimates of the WMT/F framework.
On the other hand, through comparing a purely satellite based approach to an approach based on the dynamically consistent output provided by the ECCO numerical model, we aimed to highlight the extent to which noise from a combination of the uncertainty in the satellite data and the inconsistency of datasets in the satellite based kinematic approach affects kinematic estimates of density flux. Through a series of smoothing experiments we show that, although uncertainties in the datasets considered for the satellite based kinematic approach affect density flux—seen by the smoothing of some of the granularity seen in the satellite kinematic density flux without smoothing applied (Figure 2)—the majority of the signal is geophysical in nature as suggested by the alternating regions of gains and losses of surface density which persist even after averaging (Figure 2).
Recently, Li et al. (2021) performed a two part study on the WMT/F within Southern Ocean, being 1) an analysis of WMF based on the thermodynamic approach of Tziperman (1986) and 2) a Lagrangian approach based on the material evolution of ML. The authors used both approaches to complement each other and draw a more detailed picture on the fate of water masses in SO. They found that there exist peaks of oceanic subduction linked to the Sub Antarctic Mode Water. Similar to our results, they show these peaks being located in IO and ESP. This finding is significant as the biggest differences in geographic WMF between both approaches are found in ESP and IO sectors of SO where Sub Antarctic Mode Water and Antarctic Intermediate Water are noted to form in their largest volumes (McCartney, 1977).
The kinematic approach also has a stronger signal of density flux as compared to the other sources of fluxes used in the thermodynamic approach. Furthermore, Zhou et al. (2020) concluded from inter-comparing 20 numerical model sources of HF that they have positive biases in Latent HF and Sensible HF of, respectively, 20 and 5 Wm −2 , which would also subsequently lead to the greater buoyancy gain of surface waters seen in the thermodynamic buoyancy forcings. Providing more evidence for our finding of negative density flux bias being driven by a positive bias in the satellite derived heat fluxes.
However, the kinematic approach hinges on an accurate definition of MLD. As of now, no satellite based MLD product exists and we have therefore used an MLD dataset based on in-situ data from ARGO which suffers from under-coverage in the SO (Hosoda et al., 2010). As such, future efforts should aim at constructing a satellite based MLD product. Furthermore, in order to derive truly meaningful WMT/F estimates via the kinematic approach further work must be done in constraining and obtaining quantitative estimates of the implicitly included terms associated to lateral mixing in ML and ML dynamics in the kinematic approach.
In conclusion, we associate differences between a kinematic and a thermodynamic approach to WMT/F to; 1) the inclusion of lateral mixing and mesoscale dynamics in the kinematic framework; 2) the annual vertical excursion of ML causing transport of fluid through MLB (resolved by the kinematic approach); and 3) interactions between the background flow and spatial gradients of MLB which induces transport through MLB in a process known as lateral induction.
Data availability statement
The raw data supporting the conclusions of this article will be made available by the authors, without undue reservation.
Author contributions
AP is a Predoc-Trainee and PhD student contracted by ICM (CSIC) under an FPI grant by the (CSIC). EO and AT are AP’s PhD supervisors. MP is the PI of the project under which the paper was written. CG-H assisted in accumulating and understanding some of the datasets. All authors contributed to the article and approved the submitted version.
Funding
This work was supported in part by the Spanish RD project L-BAND (ESP2017-89463-C3-1-R), which is funded by MCIN/AEI/10.13039/501100011033 and “ERDF A way of making Europe” under Grant FPI PRE2018-086106, and in part by the project INTERACT (PID2020-114623RB-C31), which is funded by MCIN/AEI/10.13039/501100011033. We also acknowledge funding from the Spanish government through the ‘Severo Ochoa Centre of Excellence’ accreditation (CEX2019-000928-S). This work has also been carried out as part of the SOFRESH project (A0/1-10461/20/I-NB), funded by the European Space Agency. This work is moreover a contribution to CSIC PTI Teledetect. The publication fee was partially supported by the CSIC Open Access Publication Support Initiative through its Unit of Information Resources for Research (URICI). Discussions with Dr. Lisan Yu, Dr. Sylvia Cole, Dr. Nick Foukal and Dr. Gael Forget at WHOI were invaluable for the derivation of the kinematic approach. Discussions with Dr. Ivana Cerovecki also helped immensely in the interpretation of results.
Conflict of interest
The authors declare that the research was conducted in the absence of any commercial or financial relationships that could be construed as a potential conflict of interest.
Publisher’s note
All claims expressed in this article are solely those of the authors and do not necessarily represent those of their affiliated organizations, or those of the publisher, the editors and the reviewers. Any product that may be evaluated in this article, or claim that may be made by its manufacturer, is not guaranteed or endorsed by the publisher.
References
Berry D. I., Kent E. C. (2009). A new air–sea interaction gridded dataset from ICOADS with uncertainty estimates. Bulletin of the American Meteorological Society 90, 645–656. doi: 10.1175/2008bams2639.1
Bonjean F., Lagerloef G. S. (2002). Diagnostic model and analysis of the surface currents in the tropical pacific ocean. J. Phys. Oceanography 32, 2938–2954. doi: 10.1175/1520-0485(2002)032<2938:DMAAOT>2.0.CO;2
Brambilla E., Talley L. D., Robbins P. E. (2008). Subpolar mode water in the northeastern atlantic: 2. origin and transformation. Journal of Geophysical Research 113, 1–16. doi: 10.1029/2006jc004063
Brunke M. A., Wang Z., Zeng X., Bosilovich M., Shie C.-L. (2011). An assessment of the uncertainties in ocean surface turbulent fluxes in 11 reanalysis, satellite-derived, and combined global datasets. Journal of Climate 24, 5469–5493. doi: 10.1175/2011JCLI4223.1
Bryan F. O., Kauffman B. G., Large W. G., Gent P. R. (1996). Ncar csm flux coupler. technical note. national center for atmospheric research, boulder, CO (United states). Climate Global Dynamics Div.
Cerovečki I., Talley L. D., Mazloff M. R. (2011-12). A comparison of southern ocean air–sea buoyancy flux from an ocean state estimate with five other products. Journal of Climate 24, 6283–6306. doi: 10.1175/2011jcli3858.1
Donlon C. J., Martin M., Stark J., Roberts-Jones J., Fiedler E., Wimmer W. (2012). The operational sea surface temperature and sea ice analysis (OSTIA) system. Remote Sensing of Environment 116, 140–158. doi: 10.1016/j.rse.2010.10.017
Entekhabi D., Njoku E. G., ONeill P. E., Kellogg K. H., Crow W. T., Edelstein W. N., et al. (2010). The soil moisture active passive (SMAP) mission. Proceedings of the IEEE 98, 704–716. doi: 10.1109/jproc.2010.2043918
Forget G., Campin J.-M., Heimbach P., Hill C. N., Ponte R. M., Wunsch C. (2015). Ecco version 4: an integrated framework for non-linear inverse modeling and global ocean state estimation. Geoscientific Model. Dev. 8, 3071–3104. doi: 10.5194/gmd-8-3071-2015
Fournier S., Lee T., Tang W., Steele M., Olmedo E. (2019). Evaluation and intercomparison of smos, aquarius, and smap sea surface salinity products in the arctic ocean. Remote Sens. 11, 3043. doi: 10.3390/rs11243043
Fukumori I., Wang O., Fenty I., Forget G., Heimbach P., Ponte P., et al. (2017). Ecco version 4 release 3.
Groeskamp S., Griffies S. M., Iudicone D., Marsh R., Nurser A. G., Zika J. D. (2019). The water mass transformation framework for ocean physics and biogeochemistry. Annual Review of Marine Science 11, 271–305. doi: 10.1146/annurev-marine-010318-095421
Gutenstein M., Fennig K., Schröder M., Trent T., Bakan S., Roberts J. B., et al. (2021). Intercomparison of freshwater fluxes over ocean and investigations into water budget closure. Hydrology Earth System Sci. 25, 121–146. doi: 10.5194/hess-25-121-2021
Henderson-Sellers B. (1984). A new formula for latent heat of vaporization of water as a function of temperature. Q. J. R. Meteorological Soc. 110, 1186–1190. doi: 10.1002/qj.49711046626
Hosoda S., Ohira T., Sato K., Suga T. (2010). Improved description of global mixed-layer depth using argo profiling floats. J. oceanography 66, 773–787. doi: 10.1007/s10872-010-0063-3
Huffman G. J., Bolvin D. T., Braithwaite D., Hsu K., Joyce R., Xie P., et al. (2015). Nasa global precipitation measurement (gpm) integrated multi-satellite retrievals for gpm (imerg). Algorithm Theor. Basis Document (ATBD) Version 4 26.
Iudicone D., Madec G., McDougall T. J. (2008). Water-mass transformations in a neutral density framework and the key role of light penetration. Journal of Physical Oceanography 38, 1357–1376. doi: 10.1029/2006jc003878
Johnson E. S., Bonjean F., Lagerloef G. S. E., Gunn J. T., Mitchum G. T. (2007). Validation and error analysis of oscar sea surface currents. J. Atmospheric Oceanic Technol. 24, 688–701. doi: 10.1175/JTECH1971.1
Kara A. B., Rochford P. A., Hurlburt H. E. (2000). An optimal definition for ocean mixed layer depth. J. Geophysical Research: Oceans 105, 16803–16821. doi: 10.1029/2000JC900072
Kim S.-B., Fukumori I., Lee T. (2006). The closure of the ocean mixed layer temperature budget using level-coordinate model fields. J. Atmospheric Oceanic Technol. 23, 840–853. doi: 10.1175/JTECH1883.1
Li H., Xu F., Zhou W., Wang D., Wright J. S., Liu Z., et al. (2017). Development of a global gridded argo data set with barnes successive corrections. Journal of Geophysical Research: Oceans 122, 866–889. doi: 10.1002/2016jc012285
Li Z., England M. H., Groeskamp S., Cerovečki I., Luo Y. (2021). The origin and fate of subantarctic mode water in the southern ocean. J. Phys. Oceanography 51, 2951–2972. doi: 10.1175/JPO-D-20-0174.1
Martínez J., Gabarró C., Turiel A., González-Gambau V., Umbert M., Hoareau N., et al. (2022). Improved bec smos arctic sea surface salinity product v3.1. Earth System Sci. Data 14, 307–323. doi: 10.5194/essd-14-307-2022
Maze G., Forget G., Buckley M., Marshall J., Cerovecki I. (2009). Using transformation and formation maps to study the role of air–sea heat fluxes in north atlantic eighteen degree water formation. Journal of Physical Oceanography 39, 1818–1835. doi: 10.1175/2009jpo3985.1
McDougall T. J., Groeskamp S., Griffies S. M. (2014). On geometrical aspects of interior ocean mixing. J. Phys. Oceanography 44, 2164–2175. doi: 10.1175/JPO-D-13-0270.1
Nelkin E., Huffman G. J., Bolvin D. T., Behrangi A., Adler R. F. (2021). “Introducing the gpcp version 3.1 daily precipitation dataset,” in AGU fall meeting 2021 (AGU).
Nurser A. J. G., Griffies S. M. (2019-04). Relating the diffusive salt flux just below the ocean surface to boundary freshwater and salt fluxes. Journal of Physical Oceanography 49, 2365–2376. doi: 10.1175/JPO-D-19-0037.1
Olmedo E., Gonzalez-Haro C., Hoareau N., Umbert M., Gonzalez-Gambau V., Martinez J., et al. (2021). Nine years of smos sea surface salinity global maps at the barcelona expert center. Earth System Sci. Data 13, 857–888. doi: 10.5194/essd-13-857-2021
Olmedo E., Martínez J., Turiel A., Ballabrera-Poy J., Portabella M. (2017). Debiased non-bayesian retrieval: A novel approach to smos sea surface salinity. Remote Sens. Environ. 193, 103–126. doi: 10.1016/j.rse.2017.02.023
Piracha A., Sabia R., Klockmann M., Castaldo L., Fernández D. (2019). Satellite-driven estimates of water mass formation and their spatio-temporal evolution. Frontiers in Marine Science 6. doi: 10.3389/fmars.2019.00589
Reed R. (1977). On estimating insolation over the ocean. J. Phys. Oceanography 7, 482–485. doi: 10.1175/1520-0485(1977)007<0482:OEIOTO>2.0.CO;2
Roquet F., Madec G., McDougall T. J., Barker P. M. (2015). Accurate polynomial expressions for the density and specific volume of seawater using the teos-10 standard. Ocean Modelling 90, 29–43. doi: 10.1016/j.ocemod.2015.04.002
Smith S. D. (1988). Coefficients for sea surface wind stress, heat flux, and wind profiles as a function of wind speed and temperature. J. Geophysical Research: Oceans 93, 15467–15472. doi: 10.1029/JC093iC12p15467
Song H., Marshall J., McGillicuddy D. J. Jr., Seo H. (2020). Impact of current-wind interaction on vertical processes in the southern ocean. J. Geophysical Research: Oceans 125, e2020JC016046. doi: 10.1029/2020JC016046.E2020JC01604610.1029/2020JC016046
Speer K., Tziperman E. (1992). Rates of water mass formation in the north atlantic ocean. Journal of Physical Oceanography 22, 93–104. doi: 1175/1520-0485(1992)022<0093:ROWMFI>2.0.CO;2
Tomita H., Hihara T., Kako S., Kubota M., Kutsuwada K. (2019). An introduction to j-ofuro3, a third-generation japanese ocean flux data set using remote-sensing observations. J. Oceanography 75, 171–194. doi: 10.1007/s10872-018-0493-x
Tomita H., Tsutomu H., Masahisa K. (2018). Improved satellite estimation of near-surface humidity using vertical water vapor profile information. Geophysical Research Letters 45, 899–906. doi: 10.1002/2017GL076384
Tziperman E. (1986). On the role of interior mixing and air-sea fluxes in determining the stratification and circulation of the oceans. Journal of Physical Oceanography 16, 680–693. doi: 10.1175/1520-0485(1986)016<0680:OTROIM>2.0.CO;2
Walin G. (1982). On the relation between sea-surface heat flow and thermal circulation in the ocean. Tellus 34, 187–195. doi: 10.1111/j.2153-3490.1982.tb01806.x
Williams R. G., Spall M. A., Marshall J. C. (1995). Does stommel’s mixed layer “demon” work? Journal of Physical Oceanography 25, 3089–3102. doi: 10.1175/1520-0485(1995)025<3089:DSMLW>2.0.CO;2
Yu L., Jin X., Weller R. A. (2008). Multidecade global flux datasets from the objectively analyzed air-sea fluxes (oaflux) project: Latent and sensible heat fluxes, ocean evaporation, and related surface meteorological variables 74.
Zhou X., Ray P., Barrett B. S., Hsu P. (2020). Understanding the bias in surface latent and sensible heat fluxes in contemporary agcms over tropical oceans. Climate Dynamics 55, 2957–2978. doi: 10.1007/s00382-020-05431-y
Keywords: water mass transformation, SMOS, satellite observations, atmosphere-ocean interaction, ocean circulation
Citation: Piracha A, Olmedo E, Turiel A, Portabella M and González-Haro C (2023) Using satellite observations of ocean variables to improve estimates of water mass (trans)formation. Front. Mar. Sci. 10:1020153. doi: 10.3389/fmars.2023.1020153
Received: 15 August 2022; Accepted: 27 February 2023;
Published: 15 March 2023.
Edited by:
Paul Myers, University of Alberta, CanadaReviewed by:
Kenneth Hughes, Oregon State University, United StatesShinya Kouketsu, Japan Agency for Marine-Earth Science and Technology (JAMSTEC), Japan
Copyright © 2023 Piracha, Olmedo, Turiel, Portabella and González-Haro. This is an open-access article distributed under the terms of the Creative Commons Attribution License (CC BY). The use, distribution or reproduction in other forums is permitted, provided the original author(s) and the copyright owner(s) are credited and that the original publication in this journal is cited, in accordance with accepted academic practice. No use, distribution or reproduction is permitted which does not comply with these terms.
*Correspondence: Aqeel Piracha, cGlyYWNoYS5hcWVlbDFAZ21haWwuY29t