- 1Digital Ocean Laboratory, Shanghai Ocean University, Shanghai, China
- 2College of Electronics and Information Engineering, Shanghai University of Electric Power, Shanghai, China
- 3Institute of Deep Sea Science and Engineering, Chinese Academy of Sciences, Sanya, Hainan, China
- 4College of Information Technology, Shanghai Jian Qiao University, Shanghai, China
Underwater images play a crucial role in various fields, including oceanographic engineering, marine exploitation, and marine environmental protection. However, the quality of underwater images is often severely degraded due to the complexities of the underwater environment and equipment limitations. This degradation hinders advancements in relevant research. Consequently, underwater image restoration has gained significant attention as a research area. With the growing interest in deep-sea exploration, deep-sea image restoration has emerged as a new focus, presenting unique challenges. This paper aims to conduct a systematic review of underwater image restoration technology, bridging the gap between shallow-sea and deep-sea image restoration fields through experimental analysis. This paper first categorizes shallow-sea image restoration methods into three types: physical model-based methods, prior-based methods, and deep learning-based methods that integrate physical models. The core concepts and characteristics of representative methods are analyzed. The research status and primary challenges in deep-sea image restoration are then summarized, including color cast and blur caused by underwater environmental characteristics, as well as insufficient and uneven lighting caused by artificial light sources. Potential solutions are explored, such as applying general shallow-sea restoration methods to address color cast and blur, and leveraging techniques from related fields like exposure image correction and low-light image enhancement to tackle lighting issues. Comprehensive experiments are conducted to examine the feasibility of shallow-sea image restoration methods and related image enhancement techniques for deep-sea image restoration. The experimental results provide valuable insights into existing methods for addressing the challenges of deep-sea image restoration. An in-depth discussion is presented, suggesting several future development directions in deep-sea image restoration. Three main points emerged from the research findings: i) Existing shallow-sea image restoration methods are insufficient to address the degradation issues in deep-sea environments, such as low-light and uneven illumination. ii) Combining imaging physical models with deep learning to restore deep-sea image quality may potentially yield desirable results. iii) The application potential of unsupervised and zero-shot learning methods in deep-sea image restoration warrants further investigation, given their ability to work with limited training data.
1 Background
The ocean contains many unknown organisms and vast energy sources, which play an important role in sustaining life on earth. The exploitation of marine resources, the development of the marine economy, and the strengthening of the marine industry have become integral components of countries’ strategic planning and progress. Underwater image processing is essential for ocean exploration; however, the complexity of the marine environment often leads to severely degraded image quality. The differing rates of light attenuation at various wavelengths in the ocean cause images to predominantly appear blue–green. In addition, microorganisms and suspended particles in the water absorb most of the light energy and deflect its direction, resulting in low-contrast and blurred images. These factors significantly impact the efficacy of many underwater vision systems. Image restoration is a technique that involves reversing the imaging process used to produce low-quality images. Underwater image restoration technology aims to enhance image visibility, eliminate color casts, and stretch contrast to effectively improve the visual quality of input images, thereby increasing the efficiency of underwater operations. Furthermore, the restored images highlight scenes and objects, thus serving as a preprocessing step in underwater image research. This can facilitate advanced tasks, such as target detection, recognition, and classification, and ultimately improve the observation and processing of underwater information.
In contrast to images taken on land, images taken by underwater imaging systems often suffer from low contrast, loss of detail, color distortion, low light or non-uniform illumination, and reduced visual ranges as a result of the influence of complex underwater imaging environments and lighting environments. The degradation of underwater images has caused great inconvenience to practical applications and further research. The principle of underwater optical imaging can be seen in Figure 1. The attenuation of light under water is primarily caused by absorption and scattering effects, leading to degraded image quality such as reduced contrast and blurriness. In addition, different wavelengths of light have varying rates of attenuation when traveling underwater, which results in color distortion in the images. In clear water, red light is the first to disappear, at a depth of 5 meters, followed by orange light at 10 meters. Blue light, with the shortest visible wavelength, can travel the farthest in water, which causes underwater images to have an undesirable blue–green hue. The presence of small particles, plankton, and dissolved organic matter in the water frequently causes significant noise issues in underwater imaging and exacerbates the impact of backscattering.
The deep sea, broadly defined as the depth of the ocean where natural light does not penetrate (NOAA, 2022), is characterized by extreme conditions such as low temperatures, darkness, and high pressure, making exploration difficult (Paulus, 2021). Remote-operated vehicles (ROVs) equipped with underwater optical photography technology become an indispensable means of deep-sea exploration. However, images captured in the depths of the dark ocean using artificial light sources are subject to a combination of light attenuation, scattering interference, and uneven illumination, resulting in images with strong halo effects that are less clear than those taken in shallower waters. Therefore, improving the quality of deep-sea images and extracting more useful information from them is vital to promote deep-sea exploration and to discover new deep-sea phenomena.
In the research transition from shallow-sea to deep-sea image restoration methods, the core issue is the composition of the light source in the underwater imaging process. Although natural light alone or in combination with artificial light can serve as the light source in shallow-sea imaging, artificial light sources are essential in deep-sea imaging because of the absence of natural light. Artificial light sources have different characteristics from natural light and can result in non-uniform lighting, creating bright spots in the middle of the light source and dark spots around the edges of deep-sea images. Furthermore, inherent image degradation problems arise because of the absorption and scattering of light source propagation in artificial light sources.
Numerous studies have been developed to improve the quality of underwater images (Ancuti et al., 2018; Anwar and Li, 2020; Wang et al., 2022). A majority focused on designing direct image enhancement techniques or networks without taking the principles of underwater imaging into account. Others concentrated on developing underwater image restoration techniques that reverse the underwater imaging process to recover the original image. This study focuses on underwater image restoration rather than enhancement for two reasons. First, non-physical model-based underwater image enhancement methods can enhance the visual quality of images to some degree but do not consider the unique optical characteristics of underwater imaging, resulting in color distortions, artifacts, and increased noise. Second, the effectiveness of deep learning-based underwater image enhancement techniques depends heavily on the quality of the training data used. However, obtaining suitable datasets, particularly for deep-sea environments, remains a significant challenge owing to their scarcity. Although various reviews of underwater image enhancement (Wang et al., 2019b; Anwar and Li, 2020; Fayaz et al., 2021) exist, there is still a lack of systematic overview to bridge the gap between shallow-sea studies and deep-sea studies.
After a systematic review, this research paper summarizes the challenges and advanced solutions for shallow-sea image restoration to provide a reliable reference for researchers in the related fields. The study then shifts its focus to deep-sea image restoration, summarizing the difficulties faced in this field, examining the connections and differences between shallow-sea and deep-sea image restoration research, exploring fields, such as exposure and low-light image enhancement, and summarizing feasible methods for deep-sea image recovery. The contributions of this study are as follows.
(1) This study categorizes recent methods for restoring shallow-sea images into three groups, physical model-based methods, prior-based methods, and deep learning-based methods, which integrate physical models. It offers an in-depth analysis of the fundamental concepts and essential features of these techniques, and provide a comprehensive overview of their classification.
(2) This study provides an overview of the latest research advancements, challenges, and promising research directions in deep-sea image restoration. Considering two causes of the degradation of deep-sea images, the deep-sea environment and artificial light sources, this study reviews the related research for potential solutions to these problems. Techniques for shallow-sea image restoration provide valuable insights for addressing degradation issues arising from underwater environments, such as color cast and blur. The degradation problem caused by artificial light sources has been approached with solutions such as layer decomposition and the integration of deep learning and physical models.
(3) Experiments have been carried out extensively to assess the effectiveness of shallow-sea image restoration, low-light image enhancement, and exposure correction techniques in handling deep-sea images. The findings reveal that, although shallow-sea images have improved in color correction to some extent, the issue of image light sources has become more pronounced, and some prior techniques have not been effective in deep-sea environments. On the other hand, low-light image enhancement and exposure correction can improve uniform illumination and increase brightness; however, they also come with drawbacks such as worsening color cast. Using the results of the analysis, this study discusses the key scientific challenges that need to be addressed in the field of underwater image restoration, from shallow-sea to deep-sea image restoration, and provides insight into potential future research directions.
2 Shallow-sea image restoration methods
In general, restoration techniques model the degradation and apply an inverse process to recover the original image. Therefore, research on underwater image restoration focuses initially on the development of a physical model that conforms to the principle of underwater image formation. Although a more comprehensive imaging model can be obtained by taking into account various factors that influence the imaging process, a simpler model can often be applied to a wider range of scenarios. Underwater image restoration is based on prior knowledge from degradation principles or statistical data.
In this section, underwater image restoration methods are classified into three categories. The first category focuses on building a physical model that is aligned with the principle of underwater image formation. The second category utilizes prior knowledge from degradation principles or statistical data to make more accurate estimates of unknowns in the imaging model. The third category is a combination of an underwater imaging physical model and a deep learning approach for underwater image restoration.
2.1 Physical model-based shallow-sea image restoration methods
Currently, the image formation models (IFMs) employed in the field of underwater image restoration are one of four types: the atmospheric light scattering (Koschmieder) model (Koschmieder, 1924), the simplified underwater formation model, the revised underwater formation model, of which the Akkaynak–Treibitz model (Akkaynak et al., 2017) is the most widely used, and the Retinex model.
2.1.1 Koschmieder model
The Koschmieder model is an imaging model that accurately explains the principle of image degradation caused by atmospheric conditions through physical analysis (Koschmieder, 1924). As a result, it has been applied to various fields such as underwater image restoration, restoration of foggy images, and low-light image enhancement. The Koschmieder model can be described as:
In the Koschmieder model, and represent the degraded and undegraded underwater images captured by the camera, respectively, denotes the background light, and t denotes the transmittance.
Lu et al. (2015) developed a simplified underwater imaging model that takes into account the combined effects of both natural and artificial light sources. They used an energy attenuation model to describe the lighting, and the model can be formulated as follows:
The , , and illuminances represent the total illuminance, natural light source, and artificial light source, respectively. By incorporating the Koschmieder model, a new imaging model formula has been derived:
The Koschmieder model is a useful tool for accurately describing the physical degradation of images and has been widely applied in various fields, including low-light image enhancement, image dehazing, and underwater image restoration. However, the model has some limitations. Specifically, it considers only the effects of absorption and scattering on the imaging process, while ignoring other factors that can lead to significant image degradation, such as the absorption of different wavelengths of light by water.
2.1.2 Simplified underwater image formation model
Many physical model-based methods in underwater image restoration rely on simplified models and their derivatives. In accordance with the principle of underwater imaging, light is affected by absorption and scattering in water, resulting in the degradation of underwater images such as blue-green cast and blur. The formation of an underwater image is often considered a linear combination of direct transmission , backscattering , and forward scattering components , as described below:
In underwater image restoration research, the direct transmission component and backscattering component are typically considered the key parts, whereas the forward scattering component is usually difficult to obtain and has a relatively minor impact on the formation of underwater images, and, thus, is often neglected. A simplified underwater image formation model (IFM) (Narasimhan and Nayar, 2000; Fattal, 2008; Narasimhan and Nayar, 2008) is used to mathematically simulate the underwater degradation process, which can be expressed as:
where represents the underwater degraded image, represents the undegraded image captured by the camera, A represents the background light, c represents the red, green, and blue (RGB) color channel, and t represents the transmittance according to the light attenuation law, which can be further expressed as the attenuation index (Zhao et al., 2015):
where represents the water depth and is the attenuation coefficient. The mathematical expression of the IFM is very similar to that of the Koschmieder model. Even so, we still consider the IFM an independent part for two reasons: (1) the Koschmieder model is an “accurate” description of the imaging process in the atmosphere, whereas the IFM is a “simulation” of the underwater imaging process under the analysis of the underwater environment and certain assumptions; and (2) research based on the Koschmieder model is often used to develop a new physical model of underwater imaging, whereas research based on the IFM is used to estimate the transmission map and background light more accurately under specific prior conditions in order to obtain a restored image with enhanced quality.
Not all underwater scenes can be effectively modeled using the simplified underwater IFM. To address the issue of water types and artificial light source interference in underwater images, Chiang and Chen (2012) considered the difference between the attenuation of different light wavelength and adjusted the normalized residual energy ratio Nrer based on that of Ocean Type I (extremely clear waters) as follows:
where is the wavelength. In underwater scenes, there is a relationship between the transmittance and the normalized residual energy ratio:
Then, the underwater imaging model considering the artificial light source, blur, and wavelength attenuation can be expressed as:
Simplified underwater IFMs are widely used in shallow-sea image restoration research and have achieved satisfactory results. However, they have significant limitations in deep-sea image restoration research. The simplified underwater IFM attributes the degradation of underwater images to three factors: the absorption and scattering characteristics of water, the distance between the target and the camera, and the geometric angle between the light source, the camera, and the target. It is an approximate model derived by reverse-deriving the degradation process through computer simulation of the underwater imaging process, neglecting the forward scattering component. In the deep-sea environment, the forward scattering component is a crucial factor that cannot be ignored, and the composition of the imaging light source differs significantly from that in the shallow-sea environment. Therefore, computer simulations based on shallow-sea imaging environments cannot accurately describe the degradation of images in deep-ocean environments.
Moreover, the simplified models used in the field of underwater image restoration are based on the assumption that the light sources is parallel natural light, such as sunlight. Although a few models consider the presence of artificial light sources during the imaging process, they are often considered auxiliary light sources with negligible effects on imaging. However, in the deep-sea environment, without natural light, an artificial light source with a bright center and dark surroundings becomes the only light source for imaging, resulting in an inaccurate description of the degradation process of deep-sea images by underwater imaging models. Furthermore, the deep-sea environment is different from the shallow-sea environment, and the absorption and scattering of light in deep-sea environments differ from those in general shallow-water environments. Therefore, simplified underwater imaging models are not suitable for deep-sea image enhancement and restoration.
2.1.3 Akkaynak–Treibitz model
The Akkaynak–Treibitz model is proposed as an alternative to the IFM model currently used in underwater image restoration. Akkaynak et al. (2017) conducted in situ experiments in the Red Sea and the Mediterranean Sea, and found that attenuation coefficients of light depend on the imaging range and object reflectivity. The study also quantified the error arising from neglecting such dependencies. Building on these findings, Akkaynak and Treibitz (2018) proposed a revised underwater physical imaging model, as expressed in Equation 11. In the revised model, the attenuation coefficients of the direct transmission component and the backscattering component are different, and the relationship between the distance between the camera and the target and the direct transmission component is mainly investigated:
where and are both vectors and and , represents the distance between the camera and the target, represents the reflectivity, is the irradiance, is the camera response function, is the light scattering coefficient, and is the physical scattering attenuation coefficient of the water body.
Subsequently, Akkaynak and Treibitz identified a functional dependence between the direct transmission attenuation coefficient and the camera–target distance , as described in Equation 12. They proposed the “sea-thru” underwater image restoration method (Akkaynak and Treibitz, 2019) based on this relationship, along with a practical approach for estimating the parameters of the corrected model:
where and c are coefficients related to the type of water body and their values can be calculated based on the relevant data measured on site, and d is the depth of the water.
The Akkaynak–Treibitz model can be regarded as an enhancement of the simplified underwater IFM through optimization. This entails introducing non-uniform attenuation coefficients for the direct transmission component and backscattering transmission component and establishing distinct correlations between the two-component attenuation coefficient and the camera–target distances. Although the Akkaynak–Treibitz model has been further confirmed by many scholars in the field of shallow-sea image restoration and has led to the development of effective shallow-sea image restoration methods, it is still an approximate model simulating the imaging process of shallow-sea degradation.
2.1.4 Retinex model
The Retinex theory (Land and McCann, 1971; Land, 1977) is an effective method for addressing complex lighting issues in images. It can balance dynamic range compression, edge enhancement, and color preservation in image processing. Many researchers have applied it to the fields of underwater image enhancement and restoration. The implementation of Retinex requires certain assumptions, such as that the color of objects as seen by the human eye is the result of the object’s reflection of light under different conditions, and that all colors in nature are composed of fixed wavelengths of the three primary colors, red, green, and blue. Meanwhile, the color of objects in the real world depends solely on the object’s reflection properties and is not affected by the non-uniformity of lighting, resulting in color constancy.
Based on the Retinex theory, the Retinex model (Land and McCann, 1971; Land, 1977) is represented by the following equation:
where represents the illumination component, background information, or global information, represents the reflectance component or the attributes of the photographed object, represents the observed image, xrepresents the pixel, and the symbol “ denotes pixel multiplication.
The Retinex model has achieved good results in the fields of underwater image enhancement and low-light image enhancement. Kimmel et al. (2003) first proposed an optimized algorithm for the Retinex model based on a variational framework, which has inspired the development of methods based on a variational framework to address the problem of underwater image degradation. Zhuang et al. (2021) proposed a Bayesian optimization algorithm for a single-frame underwater imaging model based on multiorder gradient priors for reflectance and illuminance enhancement, without the need for additional prior knowledge of underwater imaging. Later, Zhuang et al. (2022), proposed a modified variational model with different reflectance and illumination priors that are independent of prior knowledge of underwater imaging.
Based on the Retinex theory, Zhang and Peng (2018) proposed to use the global background light color as the light source color to restore the underwater image color, and proposed an imaging model that considered both the underwater imaging degradation principle and the light source characteristics, as follows:
where L is the light source color and M is the surface reflectance.
The Retinex model differs significantly from the three physical imaging models mentioned earlier. Most shallow-sea image restoration methods that utilize the Retinex model achieve accurate estimation of both the illumination and reflection components through different mathematical derivations. Such methods have the advantage of being faster, but often require additional prior knowledge of underwater imaging and thus are subject to the limitations of prior knowledge. Therefore, the Retinex shallow-sea image restoration method without additional prior knowledge cannot guarantee good results in deep-sea image restoration.
To sum up, the physical imaging model applied in shallow-sea image restoration lacks generalizability in deep-sea image restoration. Therefore, it is necessary and feasible to construct deep-sea imaging physics based on the environmental characteristics of the deep sea and the light source characteristics of deep-sea imaging combined with deep-sea-collected images.
2.2 Prior-based shallow-sea image restoration methods
Based on prior knowledge, the unknown quantities in the physical model, transmission map and background light, are estimated more accurately.
He et al. (2011) introduced the dark channel prior (DCP) method for dehazing natural land images by leveraging the fog imaging model. They creatively solved the problem of dehazing natural land images by estimating background light and transmission maps. The DCP method is based on a statistical prior known as the dark channel, which is derived from the observation that, in most outdoor haze-free images, pixels in non-sky regions have at least one color channel with very low luminance values. The dark channel is defined as follows:
Based on this statistical prior, the estimation of ambient light was suggested by selecting the brightest points in the top 0.1% of the dark channel of the observed image, and the transmission map could be calculated using the following formula:
where the variable is used to make the restored image more realistic. A value of 0.95 is typically employed for .
Although the DCP method is not effective when applied directly to underwater images, it has inspired many other underwater image restoration methods (Hautière et al., 2008; Carlevaris-Bianco et al., 2010). The underwater dark channel prior (UDCP) method accounts for the fact that water absorbs different wavelengths of light differently, with the transmission distance of red light being shorter. Drews et al. (2016) found that, although the DCP method fails in the red channel of underwater images, the blue and green channels are still suitable for the DCP method. Consequently, they applied the DCP method to the blue-green channel of a degraded underwater image, resulting in significant improvement in the restored image. Galdran et al. (2015) have proposed the red channel prior (RCP) method, as shown in Equation 17, which restores the color of shortwave-related underwater images based on the red wavelength with the fastest attenuation. These methods can be considered variants of the DCP method:
As the RCP method is effective in restoring artificially illuminated areas of underwater images, Zhou et al. (2021a) combined the RCP method with a quadratic guidance filter to refine the transmission map in underwater image restoration. Chiang and Chen (2012) corrected the color of underwater images by compensating for the attenuation of different colors of light along the propagation path and used the DCP method to achieve defogging. Peng et al. (2018) proposed the generalized dark channel prior (GDCP) method, which estimates ambient light through depth-dependent color changes, and calculates the scene transmission through the difference between the observed value and the estimated value. This method applies to a wide range of scenarios. Li et al. (2016b) proposed a new underwater dark channel prior model that combines the grayscale world assumption to achieve blue-green channel dehazing and red channel color correction, and used an adaptive exposure map to adjust the color of the image. Gao et al. (2016) proposed the bright channel prior (BCP) method, which is suitable for underwater images and can restore underwater images by estimating background light and transmission map through the bright channel, drawing on prior knowledge of the dark channel.
In contrast to the DCP method, the maximum intensity prior (MIP) method (Carlevaris-Bianco et al., 2010) uses the attenuation difference between the three color channels of an underwater image to estimate the depth of the scene and restore the image. The MIP method involves comparing the maximum intensity of the red channel with the maximum intensity of the green and blue channels on a small image patch. It then calculates the difference between the maximum intensity of the red channel and the maximum intensity of the green and blue channels using the following formula:
Here, the transmission at the point x is estimated by the following formula:
Wang et al. (2017) proposed the maximum attenuation identification (MAI) method, which is based on a simple prior knowledge of underwater imaging: that the intensity of light decays as an exponential function of distance. They rewrote the simplified underwater imaging model as follows:
and, further, estimated the attenuation as:
Peng et al. (2015) observed that in underwater images the scenes that are farther away from the camera appear more blurred. Based on this observation, they proposed a blur prior (BP) to estimate the distance between the scene point and the camera in order to obtain the depth map of the underwater image and then restore the degraded image. This method is effective under different lighting conditions. Peng and Cosman (2017) later proposed a new method called image blurriness and light absorption (IBLA), which takes into account the absorption characteristics of underwater light and further optimizes the estimation of the depth map and background light. They proposed a new hypothesis that scene points that retain more red light in the red channel map are closer to the camera, which is used to estimate the depth map , as expressed in the following formula:
where is a stretching function:
where V is a vector, which can represent the red channel R, the MIP, and the BP. The final depth map of IBLA is obtained by combining the three estimated depth maps.
The principle of the minimum information loss prior (MILP) states that the underwater imaging model can be mapped from the transmission map to the undegraded image; however, the input value range is and its effective mapping range is . Li et al. (2016a) proposed an effective underwater image dehazing algorithm that combines the MILP to restore the visibility, color, and natural appearance of underwater images. They also proposed a simple but effective contrast ratio enhancement algorithm based on the histogram prior, which improves the contrast and brightness of underwater images.
Song et al. (2018) proposed the underwater light attenuation prior (ULAP) method based on the observation of a large number of underwater images. The calculation of the depth map using the ULAP method is as follows:
In this formula, m represents the maximum value of the blue-green channel intensity and v represents the intensity value of the red channel.
Inspired by the color-line algorithm for land image dehazing (Fattal, 2014), Berman et al. (2016) found that by clustering the pixels of haze-free color images using k-means, each color cluster in the RGB space was distributed along a straight line, which they called the haze line. They used this discovery to achieve image depth map estimation and haze-free image restoration. Later, Menaker et al. (2017) introduced the haze line into the field of underwater image restoration and restored the image by combining the blue-to-green and blue-to-red channel attenuation ratio and the extracted parameters in the existing water-type library. They also chose the best-restored image based on the grayscale world assumption. Berman et al. (2020) further optimized the method by automatically selecting the best-restored image based on the color distribution of the underwater image. Bekerman et al. (2020) proposed a robust underwater image restoration algorithm that estimates attenuation from image color distribution and estimates veiling light from scene objects based on the underwater optical characteristics.
Zhou et al. (2021b) proposed an underwater background light estimation model based on flatness, hue, and brightness feature priors, which adaptively selects the most obvious features according to the input image to obtain more accurate background light and transmission map estimation. This method is inspired by the underwater scene prior.
Underwater image restoration methods that combine multiple prior advantages also continue to be developed (Zhao et al., 2015; Li et al., 2016b; Peng and Cosman, 2017). For instance, Zhang and Peng (2018) used two kinds of priors, MIP and UDCP, and saliency-guided multi-feature fusion to restore salient areas of underwater images. Zhou et al. (2021c) also developed a new method for underwater depth estimation that combines the advantages of the revised physical model of underwater imaging with priors and includes image segmentation and smoothing. In Table 1, a summary of the prior-based shallow-sea image restoration methods is provided.
Currently, there are two types of prior knowledge used in the field of shallow-sea image restoration: objective principles under the environmental conditions of shallow-sea imaging, and general statistical phenomena in shallow-sea images. However, the applicability of these priors in deep-sea conditions needs to be verified. In addition, the prior knowledge used in shallow-sea image restoration should be optimized for deep-sea imaging conditions. Another approach is to extract objective principles and common phenomena from the specific imaging environment and images of the deep sea and use these to inform the development of a joint prior method that combines the advantages of different prior methods to achieve the most accurate parameter estimation for deep-sea images.
2.3 Deep learning-based shallow-sea image restoration combined with physical models
Deep learning has gained popularity in underwater image restoration and has shown promising results in recent years. Anwar and Li (2020) have classified deep learning networks into five categories, namely, encoder–decoder networks, modular design networks, multibranch designs, depth-guided networks, and dual-generator generative adversarial networks (GANs), and provided detailed introductions to these networks. Although most deep learning networks prioritize directly generating visually appealing images, a few seek to recover more realistic images by leveraging the knowledge of the image degradation process, which may overcome the lack of ground-truth underwater images. Depth-guided networks, for instance, consider the relationship between depth and the estimation of transmission ratio and background light in the underwater imaging model, making it a valuable technique for shallow-sea image restoration. Eigen et al. (2014) applied neural networks to depth estimation, and researchers have subsequently combined depth prediction with the underwater IFM to achieve significant advancements in underwater image restoration (Hou et al., 2020a). In addition to these methods, there are other ways to restore images by integrating physical imaging models with deep learning networks. This section aims to investigate various approaches that combine deep learning techniques with physical imaging models, such as the Koschmieder model, the IFM, and the Akkaynak–Treibitz model, for the restoration of shallow-sea images.
2.3.1 Koschmieder model-based approach
Kar et al. (2021) proposed a multidomain image restoration method based on the Koschmieder model and zero-shot learning. In this approach, the network is trained using the degraded image and the degraded image generated by the Koschmieder model, and then the learned mapping is used to transfer between the undegraded image and the degraded image to obtain the restored image. The network estimates the unknown parameters of background light and transmission map in the Koschmieder model separately. The projection estimation network is implemented using multiscale feature extraction and feature selection of color channels, as illustrated in Figure 2C. When applied to the field of underwater image restoration, this method requires compensation for the red channel, which is performed as follows:
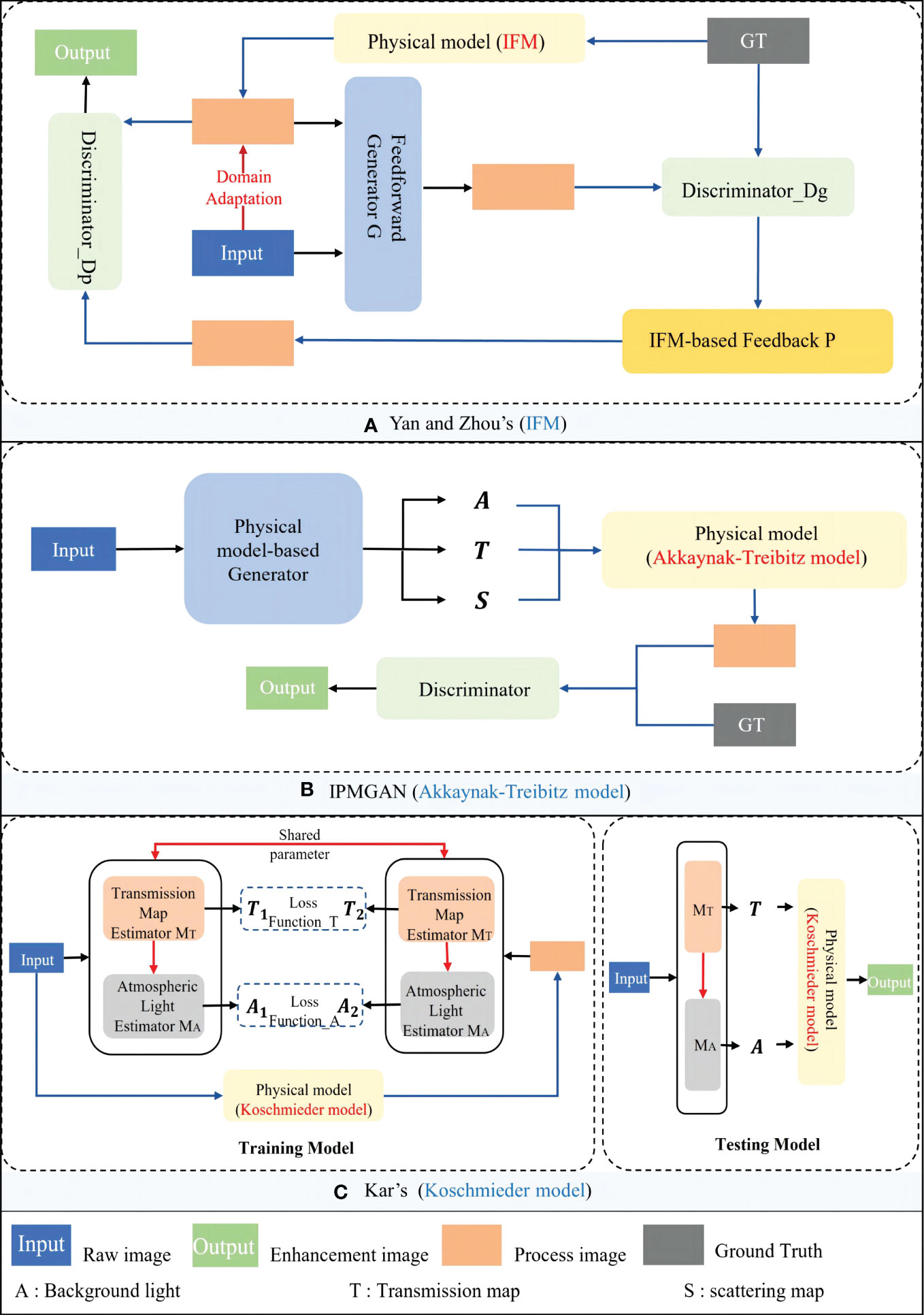
Figure 2 Shallow-sea image restoration methods based on the fusion of deep learning and physical models. (A) The deep learning network is based on the image formation model (IFM) (Yan and Zhou, 2020). (B) The deep learning network is based on the Akkaynak–Treibitz model (Liu et al., 2021). (C) The deep learning network is based on the Koschmieder model (Kar et al., 2021).
2.3.2 IFM-based approach
Lu et al. (2018) were among the first to use deep learning technology to tackle the problem of underwater image depth estimation, proposing a method based on optical cameras and deep convolutional neural networks for real-world underwater images. Ding et al. (Ding et al., 2017) used a convolutional neural network to estimate a depth map from a white balance-corrected image, which was then directly converted into a transmission map. Cao et al. (2018) proposed two network models, one for estimating the background light and the other for estimating depth. In the depth estimation network, two depth networks were overlaid to preserve both global features and local details, and the rough depth map was connected to the first layer of the refining network to preserve more detailed information. Pan et al. (2018) improved the contrast of underwater images using white balance and DehazeNet (Cai et al., 2016). They fused the two using a Laplacian pyramid and applied an edge enhancement algorithm to the fused image. DehazeNet estimated the transmission map and obtained the contrast-enhanced image based on the IFM. As shown in Figure 2A, Yan and Zhou (2020) creatively employed an imaging model as a constraint for network training, using the underwater image imaging model as a feedback controller for a GAN network to ensure that the estimation results were more realistic and consistent with the real image. In addition, a domain adaptation mechanism was introduced in the network to eliminate the domain difference between synthetic and real images.
2.3.3 Akkaynak–Treibitz model-based approach
The Akkaynak–Treibitz model integrates with deep learning methods in two ways. One is by generating synthetic image data for deep learning network training; the other is by guiding the deep learning network to estimate the physical model parameters to restore underwater images. As shown in Figure 2B, Liu et al. (2021) estimated the parameters of the revised underwater imaging physical model through an advanced global–local feature fusion network and restored the image under the guidance of the Akkaynak–Treibitz model. Desai et al. (2021) took advantage of the underwater parameter sensitivity of the Akkaynak–Treibitz model to propose reliable estimation methods for the relevant parameters. They used the reference image and its depth map as input to synthesize the underwater dataset and then used the synthetic dataset to train a conditional GAN network for underwater image restoration. Han et al. (2022) synthesized the reference images in the real underwater Heron Island coral reef dataset (HICRD) based on the new attenuation coefficient and background light estimation method. They proposed a network that uses a conditional GAN network and contrastive learning to improve the mutual information between the original image and the restored image. Lu et al. (2021) used an encoder network to extract features for the background light, backscattered transmission map, and direct transmission map based on the revised underwater IFM. Three independent decoder networks estimated these three components simultaneously. A scene attention module was designed in the network to refine the results. Finally, the estimated value was brought into the IFM to obtain the underwater restored image.
In the field of shallow-sea image restoration, combining physical models and deep learning methods has shown great potential and achieved remarkable results. Therefore, it is reasonable to explore the effectiveness of this approach in deep-sea image restoration as well. However, the shortage of deep-sea image data and the absence of reliable reference images have posed a challenge for traditional deep learning methods. Combining physical models with deep learning can reduce reliance on reference data to some extent. On the one hand, using a proper physical model to simulate the degradation process of deep-sea images we can construct deep-sea image datasets based on a large number of land images. On the other hand, physical models can serve as a constraint for the deep learning network to enable fast training with limited data. Alternatively, physical models can be integrated with deep-sea images to transform the image restoration process into a parameter estimation or linear solution problem, which can be solved more easily. Furthermore, exploring unsupervised deep learning methods, such as zero-shot learning in the field of deep-sea image restoration, is also promising. These methods could potentially improve the quality of deep-sea image restoration without relying on large numbers of labeled data.
3 Deep-sea image restoration methods
The exploration from shallow-sea to deep-sea environments presents significant challenges for imaging and observation owing to the absence of light in deeper waters. Artificial light sources must be used for imaging but result in image degradation such as low light and non-uniform illumination. Current research on illumination problems in underwater imaging is limited. Figure 3 demonstrates a transition from shallow-sea to deep-sea image restoration, highlighting other relevant approaches to exposure and low-light enhancement to address the problems caused by artificial light sources.
In this research paper, the current methods for deep-sea image restoration are divided into two categories. The first category includes general methods that can be utilized to tackle specific problems in deep-sea images and the second category consists of methods designed specifically for deep-sea images.
3.1 General image restoration models applied to deep-sea images
A general image restoration method can be applied to the field of deep-sea image restoration by taking into account the light source problem during the imaging process or by generalizing the method used to solve degradation problems in shallow-sea images. This can help to mitigate the degradation caused by light source issues in deep-sea images to some extent.
The specific degradation issue in deep-sea images, as distinguished from that in shallow-sea images, lies in the use of artificial light sources. Therefore, studies that target lighting effects, such as vignetting, halo, and uneven illumination and exposure, can achieve good results in deep-sea image restoration. The general image restoration methods that have strong generalization capabilities can be used to address specific degradation issues present in deep-sea images by considering the light source problem in the imaging process. Researchers, such as Wen et al. (2013), have achieved good results in restoring deep-sea images using the underwater optical imaging model and the underwater dark channel estimation method. Lu et al. (2016) proposed a solution to the halo problem caused by artificial light sources, rather than the more general problems of deep sea image restoration such as color correction and brightness distribution, or outside the shallow-water image restoration process, to address degradation caused by light sources. Lu et al. (2015) considered a scenario where both ambient light and artificial light sources exist in enhancing shallow-water images and proposed an ambient light estimation algorithm based on color lines, a local adaptive filtering algorithm to enhance images, and correction of color bias based on spectral features, followed by illumination compensation for dark regions of the image to achieve global contrast enhancement of underwater images. Li’s method (Li et al., 2020) took into account the improper installation of underwater light sources, lighting unevenness caused by environmental factors, and local overexposure, and proposed an adaptive filter correction to lighting and combined image segmentation and an image enhancement exponential metric to improve the adaptiveness of filter parameters.
In shallow-sea image restoration, the imaging models and prior knowledge used remain valid even when lighting conditions change. Such methods often have advantages in deep-sea image restoration. Wavelength compensation and image dehazing (WCID) proposed by Chiang and Chen (2012) determines the influence of artificial light sources on the imaging process by comparing the separated foreground and background intensity and compensates for the difference in light attenuation caused by artificial light sources. Color restoration is then done based on the residual energy ratio of different color channels and the scene depth combined with the corresponding attenuation. Li et al. (2018b) proposed a layer-wise transmission fusion method and a color-line background light estimation method to improve the illumination problem of single-input images by removing scattering. Deng’s method (Deng et al., 2019) considered attenuation under different lighting conditions based on a new scene depth estimation. The background light is estimated based on the grayscale opening and scene depth estimation to avoid pixels in white objects and artificial lighting areas being mistakenly estimated as background light, and the defogged image can be obtained based on the estimated background light and transmission map. Although DCP and MIP are often ineffective owing to underwater illumination conditions, the IBLA method (Peng and Cosman, 2017) estimates the scene depth based on image blurriness and light absorption, which is more suitable for different lighting conditions. The GDCP method (Peng et al., 2018) estimates the background light based on the color change-dependent scene depth estimation and estimates the scene transmission from the difference between the observed intensity and the estimated intensity, which is suitable for image restoration under various special environment lighting and turbid media conditions. The RCP method (Galdran et al., 2015) focuses on the problem of light spots in images caused by artificial light sources rather than the low-illumination problem of deep-sea images.
However, despite their ability to generalize, the methods that are primarily designed for shallow-sea image restoration may not fully take into account the unique differences and lighting conditions present in deep-sea environments. Although these methods can still be applied to deep-sea image restoration, they may require further optimization to fully address the specific challenges of this environment.
3.2 Specially-designed models for deep-sea image restoration
Considering deep-sea image restoration based on the knowledge of shallow-sea imaging is a solid starting point, but the methods developed for shallow-sea image restoration may not fully address the unique and complex challenges of deep-sea imaging. Therefore, it is important to research new image restoration methods specifically tailored for deep-sea environments. For example, Wen et al. (2013) proposed a new underwater imaging model and transmittance estimation method for extreme underwater environments such as deep-sea and turbid waters. This model draws inspiration from the fog image imaging model (Narasimhan and Nayar, 2000; Narasimhan and Nayar, 2003; Fattal, 2008; Tan, 2008), but takes into account the additional effects of underwater absorption and scattering on imaging. The new imaging model is described as:
where represents the proportion of scene radiation that reaches the camera directly, and represents the sum of the effects of underwater absorption and scattering.
Liu et al. (2019) addressed the issue of regional color shift caused by the use of colored or uneven artificial light sources in deep-sea imaging by focusing on the illumination characteristics of deep-sea images and incorporating them into a simplified underwater imaging model. They proposed a frequency-domain-based hue estimation method to correct global color shift and combined it with scattering correction to improve pixel-level color shift and contrast. Subsequently, Liu et al. (2022) utilized the underwater simplified IFM and illumination parameters to simulate imaging principles under different lighting conditions and synthesized the first underwater uneven illumination dataset. They then used this dataset to train a proposed multiresolution image feature reconstruction convolutional neural network for deep-sea image enhancement.
The field of deep-sea image restoration is of great research value and significance as it allows for the full utilization of information in deep-sea images, which is beneficial for further deep-sea exploration tasks. However, in comparison to shallow-sea image restoration, research in this field is lacking. The complex deep-sea imaging environment and the unique characteristics of deep-sea images urgently require further study.
3.3 Analysis of deep-sea image restoration problems
Degradation problems in deep-sea image restoration can be divided into two categories: one is the color shift, low contrast, and blur caused by underwater characteristics; the other is low light, non-uniform illumination, and noise caused by artificial light sources. The restoration of underwater images has been analyzed in detail in Section 2. To address the degradation problem caused by artificial light-assisted imaging, Cao et al. (2020) proposed NUICNet, a fully connected network suitable for deep-sea images with an illumination correction loss. NUICNet views the underwater uneven illumination image as the product of the additive combination of the ideal image and the illumination layer and solves the problem with two modules: feature fusion and illumination layer separation. The feature extraction module combines the input image with parameters trained on the benchmark dataset (ImageNet; Deng et al., 2009) as hypercolumn features; the illumination layer separation module outputs the ideal image and illumination layer through an end-to-end network using the hypercolumn features as input.
Nevertheless, many deep learning-based image enhancement methods are supervised, requiring a large number of paired training data that consist of high-quality ground-truth images with diverse content. Currently, there is a dearth of deep-sea image data and no established deep-sea benchmark dataset with reference images. The problem of degradation induced by artificial light sources in deep-sea images could be tackled by drawing inspiration from research in related fields, such as exposure image correction and low-light image enhancement. Shallow-sea image enhancement methods based on deep learning would also be beneficial for restoring deep-sea images or serve as a valuable reference, given the success of these methods in eliminating various degradations of shallow-sea images.
3.3.1 Exposure image correction
At present, exposure errors remain a primary concern in camera imaging. These errors can be divided into two categories: overexposure, where certain areas in the image appear too bright and washed out, and underexposure, where certain areas appear too dark. Both types of exposure problems can occur in the same image, and they are common issues in deep-sea images. Therefore, research in the field of exposure can be leveraged to inspire the development of methods for deep-sea image restoration.
Wang et al. (2019a) proposed a network that employs local and global feature encoders to learn the mapping from underexposed images to illumination maps in order to achieve well-exposed images based on the Retinex model. Instead of directly learning the mapping from underexposure to the corrected image, this network learns the mapping from the illumination layer to the corrected image in order to preserve global features, such as color distribution, average brightness, and scene category, as well as local features, such as contrast, sharp details, intensity, shadow, and highlights. The network is constructed with dual modules for local and global feature extraction and smooths the output illumination map to obtain a high-precision illumination map. Figure 4A illustrates the network structure and implementation process of the method.
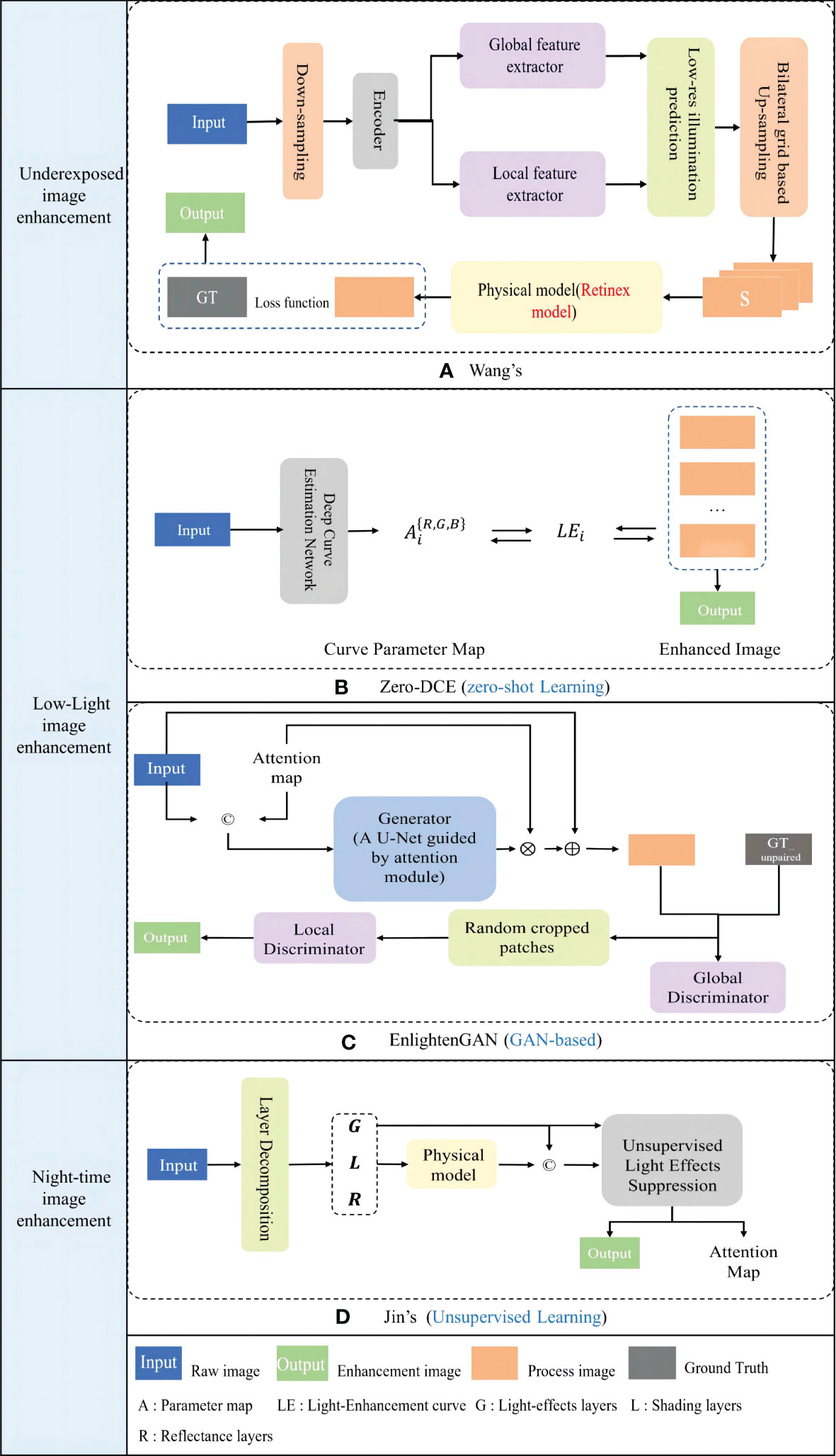
Figure 4 Representative deep learning network models. (A) The deep learning network of Wang’s method (Wang et al., 2019a). (B) The deep learning network of Zero-DCE (Guo et al., 2020). (C) The deep learning network of EnLightenGAN (Jiang et al., 2021). (D) The deep learning network of Jin’s method (Jin et al., 2022).
To address the issue of uneven exposure in deep-sea images, several methods have been proposed. Yu et al. (2018) presented a method that uses image segmentation to determine local exposure and apply it to the entire image. The resulting image is a fusion of images with different exposure levels to achieve a corrected image. Zhang et al., (2019a) considered both overexposure and underexposure in images and proposed a dual-illumination estimation network, which uses guidance to fuse corrected images with the input image to obtain a well-exposed image. Afifi et al. (2021) tackled the same problem by breaking down exposure correction into the two sub-problems of detail enhancement and color enhancement and proposed a coarse-to-fine deep network, which was trained on a constructed paired dataset and successfully solved the sub-problems.
The study of exposure correction in images, particularly those with multiple exposures, holds valuable insights for addressing the degradation caused by artificial light sources in deep-sea images. As data collection in the field of exposure research is relatively straightforward, there is an abundance of reliable paired training datasets. However, the differences between these datasets and those of the deep-sea environment make it necessary to adapt exposure correction methods to the unique characteristics of the deep sea and reduce their dependence on training data.
3.3.2 Low-light image enhancement
Research on low-light image enhancement can provide valuable insights for deep-sea image restoration, as the deep sea is also considered a low-light environment. In low-light conditions, images captured by cameras often have issues such as loss of detail, reduced contrast, poor visibility, and noise.
For low-light image enhancement, Lore et al. (2017) proposed a method that utilizes stacked sparse denoising autoencoders to learn latent features in low-light images and to obtain an output image with minimal noise and optimized contrast. Guo et al. (2017) proposed a new low-light image restoration method based on the Retinex model, which initializes an illumination map by selecting the maximum value in the pixel channel and refining it with the structure prior, ultimately producing an illumination-corrected image based on the refined illumination map. Li et al. (2018a) proposed a four-layer fully convolutional neural network, in which the first two layers focus on high-light areas, the third layer focuses on low-light areas, and the last layer is used to reconstruct the illumination map. The gamma-corrected illumination map and the original image are combined using the Retinex model to produce a well-exposed image. Fu et al. (2016) proposed a weighted variational model for estimating reflection and illumination maps from input images. This model can suppress noise and estimate more detailed reflection maps than the traditional Retinex model.
Guo et al. (2020) took into consideration low light and uneven illumination caused by different illumination conditions and proposed the zero-deep curve estimation (Zero-DCE) network, as shown in Figure 4B. This network does not rely on paired data and transforms image enhancement into a curve estimation problem, iteratively finding the best-fitting curve pair and adjusting the original image pixel by pixel to achieve image illumination correction. A lightweight network of Zero-DCE is named Zero-DCE++ (Li et al., 2021b).
Jiang et al. (2021) introduced unpaired training into the field of low-light image enhancement for the first time. The network adopts a PatchGAN-based global–local double discriminator structure to solve the problem of overexposure and underexposure simultaneously. In addition, the network incorporates a self-attention mechanism known as U-Net (Ronneberger et al., 2015) to improve the visual effect of brightness correction in regions of varying illumination. The network details are shown in Figure 4C.
For night image enhancement, Jin et al. (2022) performed layer decomposition using three independent unsupervised networks. They used the light effect layer to guide the light suppression module, reducing the influence of light effects and enhancing the dark areas. The detailed network structure is shown in Figure 4D.
In addition, Zhang et al., (2019b) proposed a KinD network to decouple the original image space into illumination components and reflection components and take images with different exposure levels as inputs for their proposed model. The illumination adjustment module in the model can adjust the illumination level according to specific needs. Later, Zhang et al. (2021) further optimized the low-light image enhancement effect by introducing a multiscale brightness attention module and abandoning the U-Net network model structure of the reflectance restoration module in the KinD network, resulting in the KinD++ network.
Research on low-light image enhancement has shown promising results in brightness correction and noise suppression through the use of the Retinex layer decomposition method. However, to apply this method to deep-sea image restoration, it is necessary to take into account the unique characteristics of the deep-sea environment and reduce reliance on training data.
3.4 Deep learning-based methods design
Deep learning-based methods are becoming mainstream in shallow-sea image quality improvement research, but their reliance on training data needs careful consideration when they are designed for deep-sea images. The following potential solutions are considered.
First, some well-trained, supervised deep learning models have demonstrated good generalization and robustness to effectively solve challenging underwater image quality enhancement problems, such as Ucolor (Li et al., 2021a) and U-shape (Peng et al., 2023). Ucolor is a multicolor space deep network model that uses the transmission map estimation output by GDCP to guide network model training, offering advantages that combine traditional and deep learning methods for richer image feature extraction. U-shape is based on the transformer network and is strengthened by a self-attention mechanism and a multicolor space loss function designed according to the human vision principle. This kind of supervised model could serve as a fundamental model for deep-sea image restoration.
Second, semisupervised and unsupervised learning methods are less dependent on data and are better suited to the current situation in which reliable reference data cannot be obtained. For instance, Semi-UIR (Huang et al., 2023), a semisupervised underwater image restoration method based on the mean teacher approach, incorporates unpaired data into the model training process and introduces pseudo-reference images and contrastive regularization to counteract network overfitting. The unsupervised method UDnet (Saleh et al., 2022) requires only degraded images, with a reference image generated by a conditional variational autoencoder with probabilistic adaptive instance normalization and a multicolor space stretching module.
Other semi-supervised and unsupervised learning methods based on GANs or zero-shot learning can help deep-sea image quality enhancement network design. The combination of imaging models and GANs, as shown in Figure 2, has produced promising results in enhancing underwater image quality. However, when integrating the Retinex model into deep learning methods for low-illumination image enhancement, several limitations must be considered. The ideal assumption used in Retinex-based low-light image enhancement methods, that reflectivity is the final enhancement result, may still impact the final outcome. In addition, despite the use of the Retinex theory, deep networks may still be at risk of overfitting (Li et al., 2021b). Similar considerations should be taken into account for deep learning-based restoration methods that integrate physical models, including the fusion strategy, the assumptions of the physical model, and the need to prevent overfitting. Refer to Table 2 for a detailed examination of some representative network models. It is worth considering whether or not supervised shallow-sea image enhancement networks, such as Ucolor and U-shape, known for their robustness, can achieve ideal results in deep-sea image enhancement. The impact of deep networks on different levels of data dependency will also be analyzed in the next section.
4 Experiment analysis
In order to extend the application of underwater image restoration to the deep sea, this section uses both the shallow-sea image dataset and the deep-sea underwater image dataset to conduct subjective and objective evaluations. The results of the experiments will be analyzed and summarized to highlight the strengths and weaknesses of each prior-based method in deep-sea image restoration. In addition, visual examples of some classic and advanced deep-sea image enhancement, low-light image enhancement, exposure image correction, and shallow-sea image enhancement methods will be applied to the OceanDark dataset to further investigate reliable techniques for deep-sea image restoration.
4.1 Experiment setup
In order to reflect the advantages and characteristics of each method, all the experiment methods adopted in this research paper are based on the open-source code from the original studies and are tested using the Linux+ NVIDIA RTX 3090 GPU experimental environment.
The experiment datasets used are the real shallow-sea underwater image enhancement benchmark dataset (UIEB) (Li et al., 2019) and the deep-sea underwater image dataset OceanDark (Porto Marques et al., 2019). Detailed information on the datasets can be found in Table 3. In the comparison experiment, the underwater image colorfulness measure (UIQM) (Panetta et al., 2016), underwater color image quality evaluation (UCIQE) (Yang and Sowmya, 2015), and the blind/reference less image spatial quality evaluator (BRISQUE) (Mittal et al., 2012) were selected as three no-reference underwater image quality evaluation indicators to quantitatively evaluate the enhancement effects of different methods on deep-sea degraded images.
The experimental methods used in this study include a selection of prior-based shallow-sea image restoration methods, including DCP (Kaiming He et al., 2011), MIP (Carlevaris-Bianco et al., 2010), IBLA (Peng and Cosman, 2017), ULAP (Song et al., 2018), UDCP (Drews et al., 2016), GDCP (Peng et al., 2018), and (Li et al., 2016a). The aim is to assess the applicability of these methods in the deep-sea environment and analyze their advantages and limitations. In addition, the experiments were also conducted with a variety of low-light image enhancement methods, such as low-light image enhancement (LIME) (Guo et al., 2017), joint enhancement and denoising (JED) (Ren et al., 2018), LightenNet (Li et al., 2018a), KinD (Zhang et al., 2019b), Wang’s method (Wang et al., 2019c), Zero-DCE (Guo et al., 2020), Zero-DCE++ (Li et al., 2021c), the robust Retinex decomposition network (RRDNet) (Zhu et al., 2020) and KinD++ (Zhang et al., 2021), nighttime image enhancement methods, such as Jin’s method (Jin et al., 2022); and underwater low-light and poor visibility methods, such as L2uwe (Marques and Branzan Albu, 2020), MLLE (Zhang et al., 2022), and hyper-laplacian reflectance priors (HLRP) (Zhuang et al., 2022). A set of deep learning-based methods that have shown excellent performance in shallow-sea image enhancement were also employed. They are divided into the supervised methods Ucolor (Li et al., 2021a) and U-shape (Peng et al., 2023), the semisupervised method Semi-UIR (Huang et al., 2023), and the unsupervised methods UDnet (Saleh et al., 2022) and Kar’s method (Kar et al., 2021). These methods aim to assist the design of new deep-sea image degradation problems. The significance of the image enhancement scheme was analyzed, with advantages and limitations in enhancing deep-sea images discussed. In total, 25 methods were compared and analyzed to determine their effectiveness in enhancing deep-sea images by addressing issues related to underwater light absorption and scattering, low light caused by artificial light sources, and uneven illumination.
4.2 Experiment results
4.2.1 Results of prior-based underwater image restoration
Deep-sea images and shallow-sea images share a common problem: color shift and blur that are caused by underwater light absorption and reflection. Thus, a natural consideration is whether or not we can apply shallow-sea image restoration methods to deep-sea images to deal with the color shift and blur problem. However, there are objective differences between deep and shallow sea environments. To verify this, experiments were conducted with prior-based shallow-sea image restoration methods using both UIEB and OceanDark datasets.
Based on the objective evaluation results in Tables 4, 5, the shallow-sea image restoration methods showed improvements in both UIQM (Panetta et al., 2016) and UCIQE (Yang and Sowmya, 2015) metrics for the UIEB and the OceanDark deep-sea dataset compared with the scores of “raw” images. UIQM is a combination of colorfulness, sharpness, and contrast, and UCIQE is also a linear combination of image characteristics such as chroma, saturation, and contrast. UIQM and UCIQE may assign high ratings to images with severely degraded naturalness (e.g., the ULAP-enhanced images score higher). In contrast, BRISQUE based on natural scene statistics is more suitable to evaluate the quality of enhanced deep-sea images, and the lower the score, the better. Comparing the metric values in Table 5 with those in Table 4, it can be concluded that these shallow-sea image restoration methods perform worse on OceanDark than on UIEB. This proves that deep-sea images suffer from more severe degradation than shallow-sea images.

Table 5 Objective evaluations of classic shallow-sea image restoration methods on OceanDark dataset.
To analyze the challenges encountered when applying shallow-sea image restoration methods to deep-sea image restoration, the visual effects of the different methods are shown in Figure 5. The DCP method produces deep-sea images with a more severe blue-green tint than other methods and fails to restore images with white targets. The deep-sea images restored using the MIP method have more bright and dark areas. Both IBLA and ULAP can effectively enhance contrast, but they each introduce false colors and are more sensitive to degradation caused by artificial light sources, resulting in over-dark and bright areas with a significant loss of image details. Although both GDCP and UDCP are based on the underwater DCP, they produce conflicting results in the restoration of deep-sea images. UDCP causes an overall decrease in image brightness, whereas GDCP overexposes deep-sea images. Li’s method, based on minimum information loss and histogram prior, has achieved the best visual effect in terms of color correction and texture detail preservation, but it makes bright areas too bright and introduces obvious blocky artifacts.
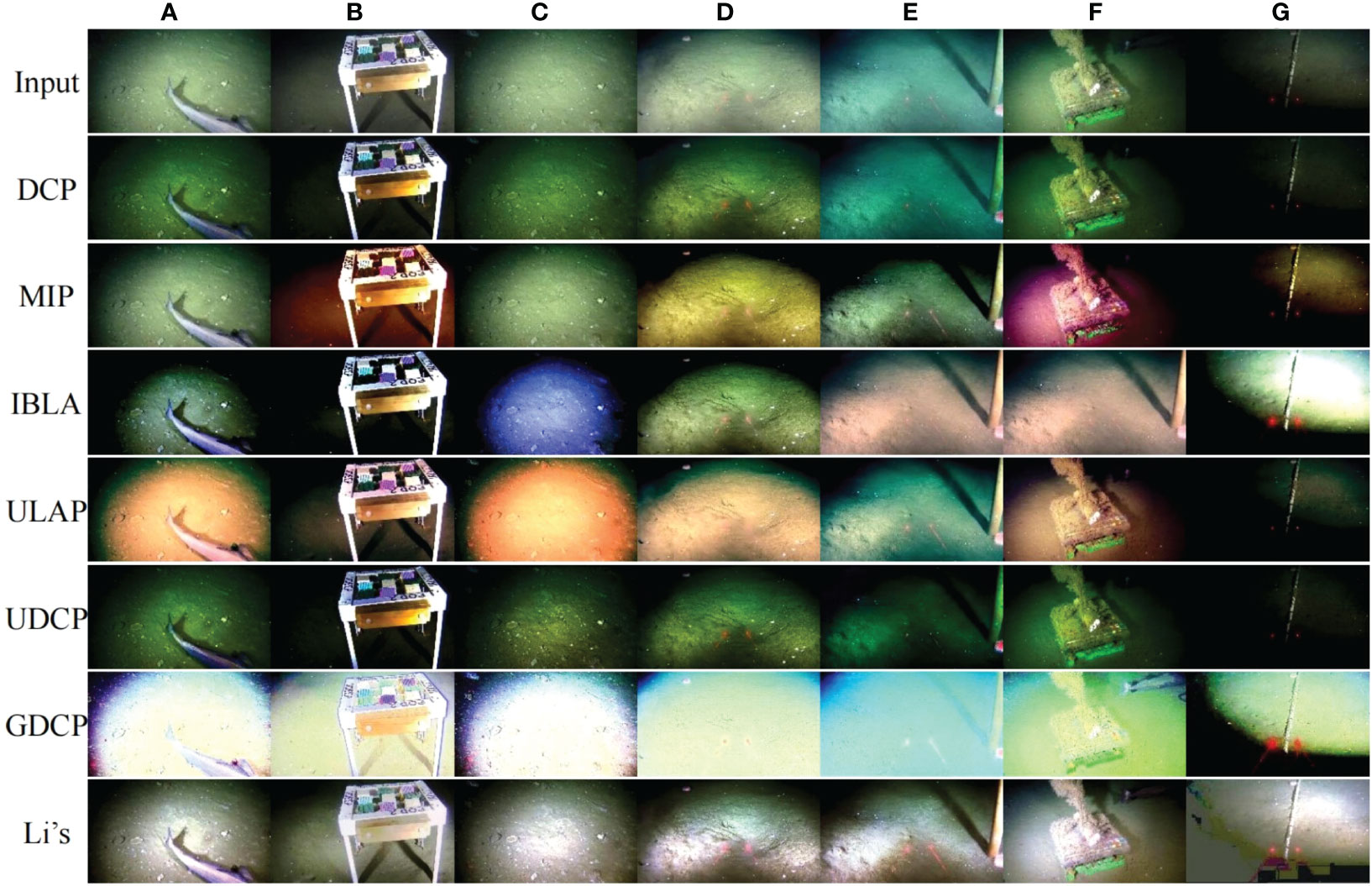
Figure 5 (A–G) represent column numbers. The visual effect of different prior-based methods on the OceanDark dataset.
Following the above analysis, it is clear, both subjectively and objectively, that the priori-based methods designed for shallow-sea images have a certain level of effectiveness; however, they cannot be directly applied to deep-sea image restoration.
4.2.2 Results of the methods for complex environmental problems
A further problem of deep-sea images is low light and uneven illumination caused by artificial light sources. As discussed in Section 3.3, the methods that are purposely designed for image exposure correction and low-light image enhancement might be useful in improving the quality of deep-sea images. To verify this idea, we performed a group of experiments and demonstrated their results using various deep-sea images.
Considering that there are few methods specifically designed for deep-sea images, we selected and compared 14 methods that might be effective in addressing some problems caused by the deep-sea environment. Listed in Table 6, these methods were originally developed for various fields, such as underwater images (e.g., L2uwe, MLLE, HLRP), low-light images [e.g., LIME, JED, LightenNet, KinD, KinD++, RRDNet, Wang’s method (Wang et al., 2019c), Kar’s method (Kar et al., 2021)], night images [e.g., Jin’s method (Jin et al., 2022)], and over/underexposed images (e.g., Zero-DCE, Zero-DCE++).
The advantages and limitations of these methods for deep-sea image restoration are analyzed in Table 7 and Figure 6, providing a reference for research in deep-sea image restoration. It is important to note that the comparisons of these methods are based on their effectiveness in deep-sea image restoration and may not reflect their overall performance in their respective fields of origin.
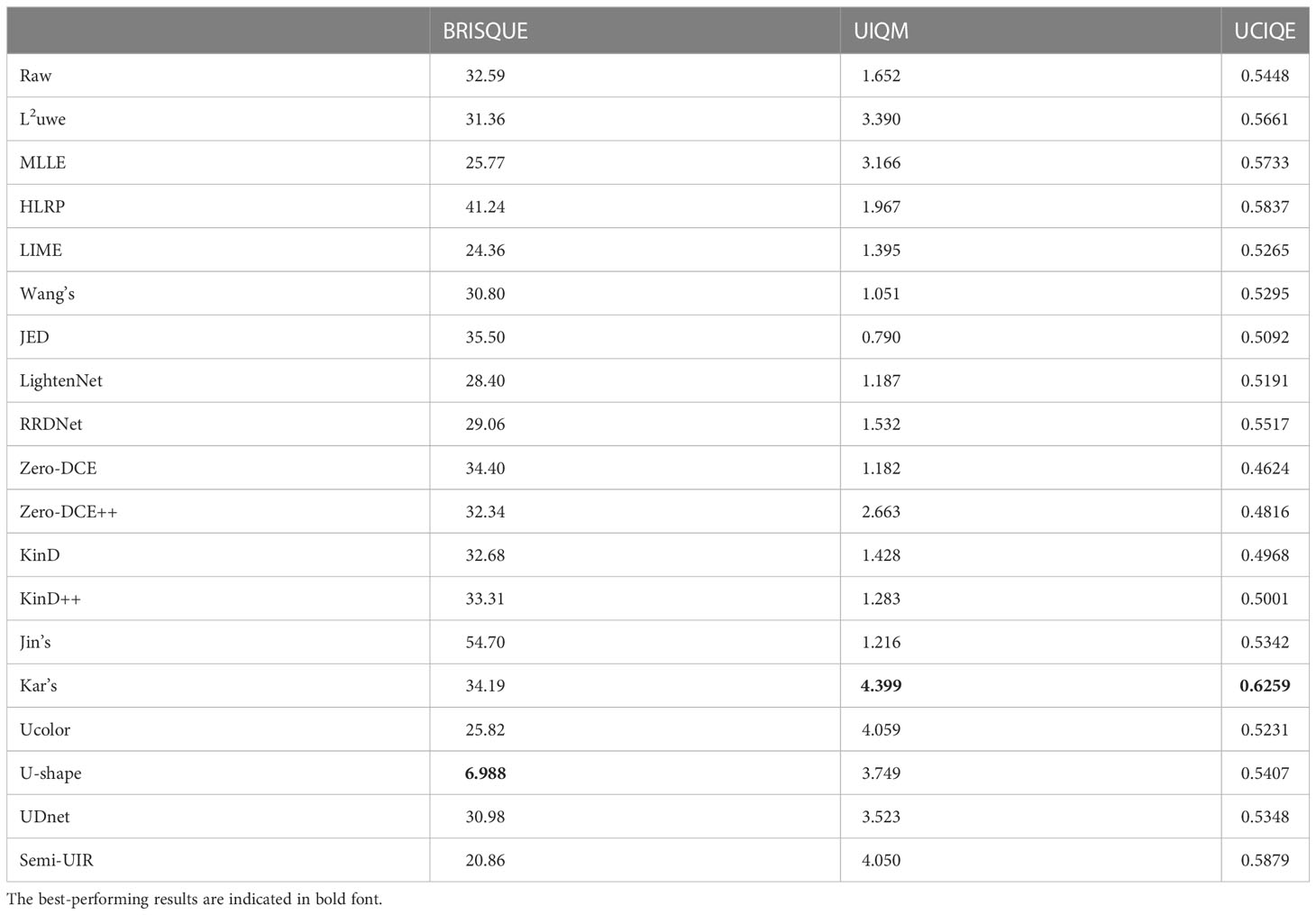
Table 7 Objective evaluations of image enhancement methods in various fields on the OceanDark dataset.
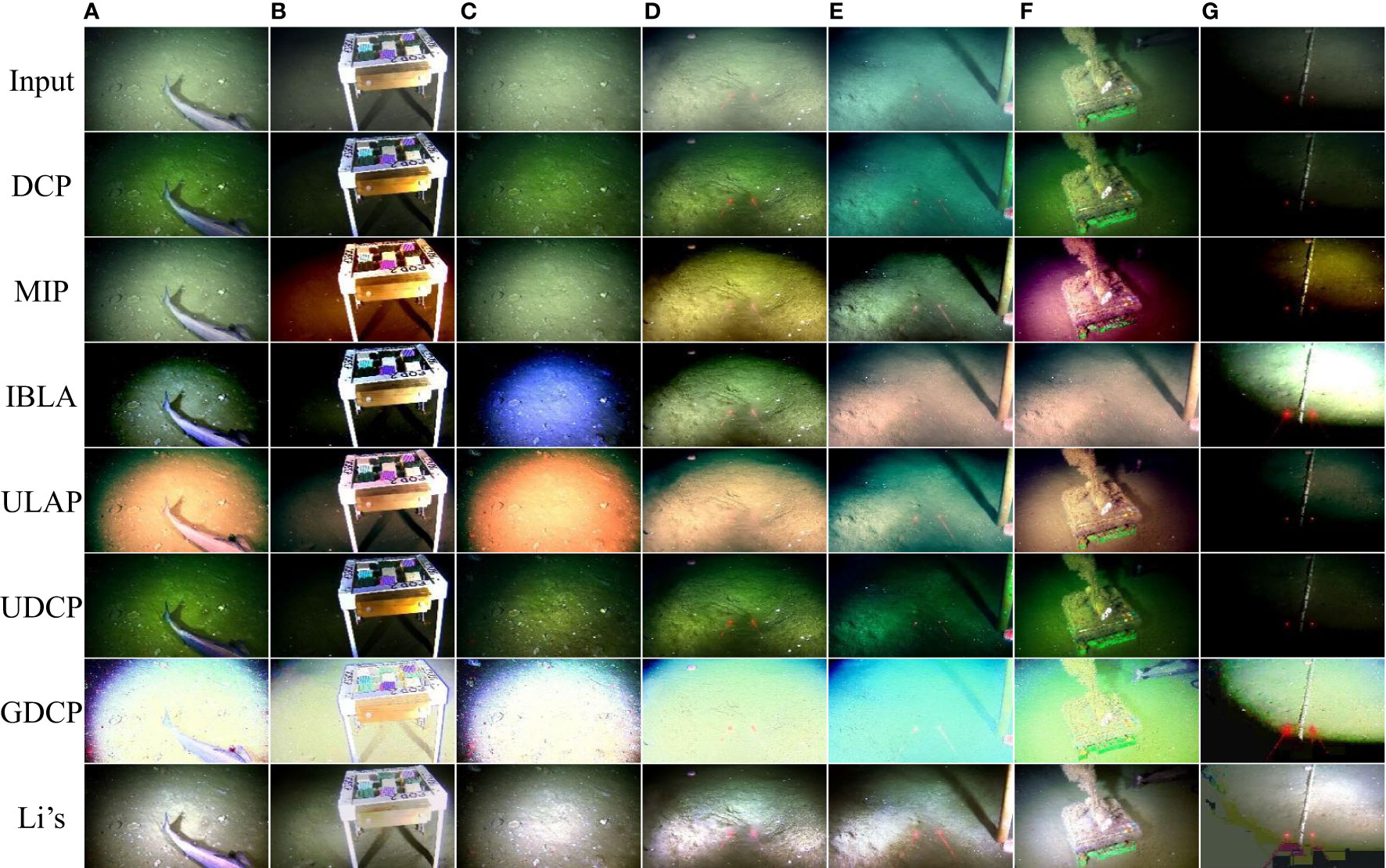
Figure 6 (A–G) represent column numbers. The visual effect of different deep learning-based methods on the OceanDark dataset.
With regard to color correction, Figure 6A demonstrates that the methods specifically designed for underwater image enhancement, such as MLLE and HLRP, perform better than those from other fields. Meanwhile, the methods from the low-light and exposure correction field, such as RRDNet, often lack a color correction process and may even introduce new color casts when addressing degradation caused by artificial light sources. When it comes to illumination correction, low-light image enhancement methods, such as LIME, Zero-DCE, Zero-DCE++, KinD, and KinD++, achieve good results, but have limitations in preserving details, correcting color cast, and reducing artifacts in deep-sea images. This highlights the need for further research that incorporates deep-sea characteristics to find solutions.
In terms of handling sudden changes in pixel values, such as the red beam in Figure 7B, methods such as HLRP and L2uwe are more effective. However, HLRP leads to overexposure in the center of the light source instead of darkening the light source area, and L2uwe results in a contrast that is too high in the processed deep-sea image. As shown in Figures 6C, D, in extreme examples of deep-sea images neither low-light enhancement nor underwater image enhancement methods have achieved satisfactory results. The severe lack of illumination and the overexposure of foreground targets in deep-sea images requires further research.
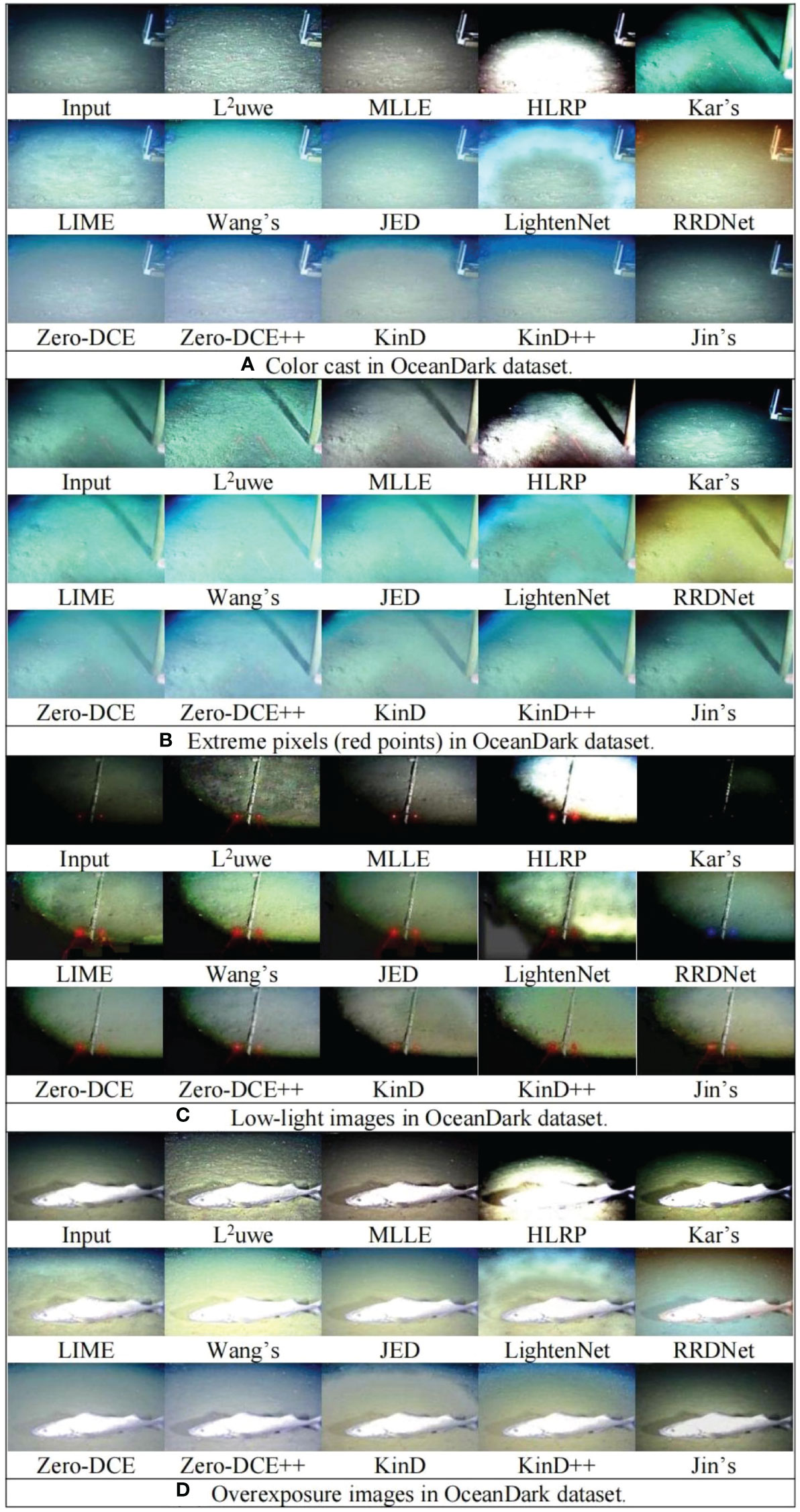
Figure 7 (A–D) different degradation types in the deep-sea environment. Comparison results of experiments in the OceanDark dataset.
According to the objective evaluation results shown in Table 7, underwater image enhancement methods show increases in both UIQM and UCIQE, whereas the low-light image enhancement and nighttime image enhancement methods have led to decreases in these two metrics. This is because UIQM and UCIQE place a greater weight on color measurement, which is not required for low-light image enhancement and nighttime image enhancement as they do not aim to correct color deviation caused by underwater light absorption. When compared with “raw” images, most image enhancement methods across various fields did not show significant improvements on the BRISQUE index. This indicates that, no matter the method of shallow-sea image restoration—low-light image enhancement, night image enhancement, or exposure image correction—they all have limitations in deep-sea image enhancement. On the BRISQUE index, however, the MLLE method for underwater improvement showed promising results. This is because the technique produces an improved image that is more realistic in terms of both color and content.
4.2.3 Results of deep learning-based underwater image enhancement
In this section, we aim to explore the potential effectiveness of the robust shallow-sea image enhancement method in addressing the degradation of deep-sea images and the influence of various data dependencies on deep learning image enhancement. The OceanDark dataset is used to experiment with supervised deep learning methods, including Ucolor and U-shape methods, the semisupervised learning method Semi-UIR, and the unsupervised deep learning method UDnet and Kar’s method. The objective evaluation results with UIQM, UCIQE, and BRISQUE are listed in Table 7.
The visual results, as illustrated in Figure 6, indicate that deep learning-based shallow-sea image enhancement methods, with the exception of Kar’s method, exhibit superior visual outcomes in deep-sea image color correction and the retention of underwater environmental details. Notably, the supervised model Ucolor demonstrates distinct advantages in color correction, also evidenced by its UIQM score in Table 7. Furthermore, the U-shape method produces remarkably robust results using the BRISQUE indicator. Compared with the unsupervised methods, the supervised deep learning approach for enhancing shallow-sea images has produced more competitive visual results, but problems remain with low light and uneven illumination created by artificial light sources, and lower lighting may decrease color correction accuracy. Kar’s method performed well using the UIQM and UICQE indicators. This is because the technique accounts for how underwater images degrade, producing a restored image with more details preserved.
In terms of implementation efficiency, it is important to note that the running time of the various deep learning methods is not always long. As shown in Table 8, methods such as KinD, Zero-DCE, Zero-DCE++, and Jin’s method have relatively shorter running times, making them more suitable for real-time applications. Shallow-sea image restoration methods that utilize deep learning techniques. These methods do not provide a processing time advantage due to the inherent complexity involved in transforming from image to image. However, KinD and KinD++ address the complexity of the image problem by dividing it into two simpler sub-problems. Similarly, Zero-DCE and Zero-DCE++ tackle the problem by estimating curves from the image. As a result, these methods effectively reduce the time cost.
5 Conclusion
This study provides an overview of the current state of research on underwater image restoration, focusing on research gaps between shallow-sea image restoration and deep-sea image restoration. It identifies the causes of degradation in underwater images, classifies and examines existing restoration methods, and evaluates their strengths and weaknesses. By comparing the results of classic shallow-sea image restoration techniques applied to both shallow-sea and deep-sea datasets, and the results of the latest methods for underwater image enhancement, exposure correction, and low-light enhancement using the deep-sea dataset, this study concludes that existing methods in the related fields are insufficient to address the deep-sea image degradation problem. Following an analysis of the similarities and differences between shallow-sea and deep-sea image degradation and the experimental results, we suggest the following research directions to guide future research on underwater image restoration.
(1) Combining an underwater formation physical model with deep learning techniques has great potential in the domain of deep-sea image restoration. The combination aims to retain two advantages: producing more realistic and naturally restored images and improving the robustness and adaptability of the methods. However, two major challenges must be addressed. (i) The physical model for the deep-sea environment is not well studied. In particular, the existing underwater imaging model cannot accurately express the deep-sea lighting conditions, resulting in a significant reduction of visual areas; and (ii) different underwater scenarios and types of degraded images require high adaptability of the models to meet the demands of practical applications.
(2) Given the current scarcity of deep-sea image datasets, future research in deep-sea image restoration should explore the potential application of unsupervised learning and zero-shot learning. However, the relationship between these learning strategies and deep-sea image restoration is not well understood, and further research is needed to evaluate the effectiveness of unsupervised learning and zero-shot learning in deep-sea image restoration.
(3) To be applicable in real-world environments, methods for deep-sea image restoration should be optimized for real-time performance. However, most existing methods for underwater image restoration require significant processing time. Inspired by the application fields and requirements of low-light image enhancement, improving the real-time performance of deep learning-based underwater image restoration methods can simplify complex image processing procedures, such as estimating curve parameters (Guo et al., 2020) or splitting into multiple sub-problems that are easier to handle (Zhang et al., 2019b).
(4) The establishment of an underwater image quality evaluation system is important. There is a lack of publicly available datasets that can support training deep learning-based deep-sea image restoration methods, and the evaluation systems are not optimal. This hinders the progression of research in this field and the selection of appropriate methods for practical applications.
(5) Aside from what has been mentioned in this research paper, there are more issues related to deep-sea images that are rarely studied. When collecting deep-sea images, the landing of equipment on the seabed can cause an influx of seabed dust, microorganisms, and suspended particles, which often lasts for a long time (even hours) and leads to red-yellowish and blurry images. Developing solutions to address this problem is crucial for practical applications. Although much of the research in underwater image restoration focuses on single images, the practical application of underwater images also extends to videos. However, there is a lack of attention given to the restoration of underwater videos. This research gap needs to be addressed, as underwater videos play a significant role in practical applications. Urgent attention is needed to address processing efficiency and frame-to-frame consistency in underwater video restoration.
Data availability statement
Publicly available datasets were analyzed in this study. These data can be found here:.
OceanDark dataset: https://sites.google.com/view/oceandark/home
UIEB dataset:
Raw: https://drive.google.com/file/d/12W_kkblc2Vryb9zHQ6BfGQ_NKUfXYk13/view?pli=1
References: https://drive.google.com/file/d/1cA-8CzajnVEL4feBRKdBxjEe6hwql6Z7/view
Challenging: https://drive.google.com/file/d/1Ew_r83nXzVk0hlkfuomWqsAIxuq6kaN4/view.
Author contributions
Conceptualization—WS, YL, and HX. Methodology—WS, YL, DH, ZS, and BZ. Original draft—YL, and HX. Experiments—YL and WS. Review, editing, and supervision—WS, DH, BZ, and HX. Investigation and visualization—YL and ZS. Funding acquisition—WS, DH, BZ, and HX. All authors contributed to the article and approved the submitted version.
Funding
This work was funded by the National Natural Science Foundation of China (61972240), and the program for the capacity development of Shanghai local universities by the Shanghai Science and Technology Commission (20050501900).
Conflict of interest
The authors declare that the research was conducted in the absence of any commercial or financial relationships that could be construed as a potential conflict of interest.
Publisher’s note
All claims expressed in this article are solely those of the authors and do not necessarily represent those of their affiliated organizations, or those of the publisher, the editors and the reviewers. Any product that may be evaluated in this article, or claim that may be made by its manufacturer, is not guaranteed or endorsed by the publisher.
References
Afifi M., Derpanis K. G., Ommer B., Brown M. S. (2021). “Learning multi-scale photo exposure correction,” in Proceedings of the IEEE/CVF Conference on Computer Vision and Pattern Recognition (Nashville, TN, USA: IEEE). 9157–9167. doi: 10.1109/CVPR46437.2021.00904
Akkaynak D., Treibitz T. (2019)Sea-Thru: a method for removing water from underwater images (IEEE) (Accessed 22 Aug. 2021).
Akkaynak D., Treibitz T., Shlesinger T., Loya Y., Tamir R., Iluz D. (2017)What is the space of attenuation coefficients in underwater computer vision? (IEEE) (Accessed 22 Aug. 2021).
Ancuti C. O., Ancuti C., De Vleeschouwer C., Bekaert P. (2018). Color balance and fusion for underwater image enhancement. IEEE Trans. Image Process. 271, 379–393. doi: 10.1109/TIP.2017.2759252
Anwar S., Li C. (2020). Diving deeper into underwater image enhancement: a survey. Signal Processing: Image Communication 89, 115978. doi: 10.1016/j.image.2020.115978
Bekerman Y., Avidan S., Treibitz T. (2020). “Unveiling optical properties in underwater images,” in 2020 IEEE International Conference on Computational Photography (ICCP). (St. Louis, MO, USA). 1–12.
Berman D., Levy D., Avidan S., Treibitz T. (2020). Underwater single image color restoration using haze-lines and a new quantitative dataset. IEEE Trans. Pattern Anal. Mach. Intell. 438, 2822–2837. doi: 10.1109/TPAMI.2020.2977624
Cai B., Xu X., Jia K., Qing C., Tao D. (2016). DehazeNet: an end-to-End system for single image haze removal. IEEE Trans. Image Process. 2511, pp.5187–5198. doi: 10.1109/TIP.2016.2598681
Cao K., Peng Y.-T., Cosman P. C. (2018)Underwater image restoration using deep networks to estimate background light and scene depth (IEEE) (Accessed 19 Jun. 2022).
Cao X., Rong S., Liu Y., Li T., Wang Q., He B. (2020). NUICNet: non-uniform illumination correction for underwater image using fully convolutional network. IEEE Access 8, 109989–110002. doi: 10.1109/ACCESS.2020.3002593
Carlevaris-Bianco N., Mohan A., Eustice R. M. (2010)Initial results in underwater single image dehazing (IEEE) (Accessed 7 Mar. 2022).
Chiang J. Y., Chen Y.-C. (2012). Underwater image enhancement by wavelength compensation and dehazing. IEEE Trans. Image Process. 214, 1756–1769. doi: 10.1109/TIP.2011.2179666
Deng J., Dong W., Socher R., Li L., Li K., Fei-Fei L. (2009). ImageNet: a large-scale hierarchical image database. In: 2009 IEEE Computer Society Conference on Computer Vision and Pattern Recognition Workshops (CVPR Workshops). 248–255. doi: 10.1109/CVPR.2009.5206848
Deng X., Wang H., Liu X. (2019). Underwater image enhancement based on removing light source color and dehazing. IEEE Access 7, 114297–114309. doi: 10.1109/ACCESS.2019.2936029
Desai C., Tabib R. A., Reddy S. S., Patil U., Mudenagudi U. (2021)RUIG: realistic underwater image generation towards restoration (IEEE) (Accessed 31 Oct. 2022).
Ding X., Wang Y., Zhang J., Fu X. (2017)Underwater image dehaze using scene depth estimation with adaptive color correction (IEEE) (Accessed 19 Jun. 2022).
Drews P. L. J., Nascimento E. R., Botelho S. S. C., Montenegro Campos M. F. (2016). Underwater depth estimation and image restoration based on single images. IEEE Comput. Graphics Appl. 362, 24–35. doi: 10.1109/MCG.2016.26
Eigen D., Puhrsch C., Fergus R. (2014)Depth map prediction from a single image using a multi-scale deep network. In: Advances in neural information processing systems (Curran Associates, Inc). Available at: https://proceedings.neurips.cc/paper/2014/hash/7bccfde7714a1ebadf06c5f4cea752c1-Abstract.html (Accessed 19 Jun. 2022).
Fayaz S., Parah S. A., Qureshi G. J., Kumar V. (2021). Underwater image restoration: a state-of-the-art review. IET Image Process. 152, 269–285. doi: 10.1049/ipr2.12041
Fu X., Zeng D., Huang Y., Zhang X.-P., Ding X. (2016). A weighted variational model for simultaneous reflectance and illumination estimation (IEEE) (Accessed 27 Sep. 2022).
Galdran A., Pardo D., Picón A., Alvarez-Gila A. (2015). Automatic red-channel underwater image restoration. J. Visual Communication Image Representation 26, 132–145. doi: 10.1016/j.jvcir.2014.11.006
Gao Y., Li H., Wen S. (2016). Restoration and enhancement of underwater images based on bright channel prior. Math. Problems Eng. 2016, 1–15. doi: 10.1155/2016/3141478
Guo C., Li C., Guo J., Loy C. C., Hou J., Kwong S., et al. (2020)Zero-reference deep curve estimation for low-light image enhancement (IEEE) (Accessed 25 Apr. 2022).
Guo X., Li Y., Ling H. (2017). LIME: low-light image enhancement via illumination map estimation. IEEE Trans. Image Process. 262, 982–993. doi: 10.1109/TIP.2016.2639450
Han J., Shoeiby M., Malthus T., Botha E., Anstee J., Anwar S., et al. (2022). Underwater image restoration via contrastive learning and a real-world dataset. Remote Sens. 1417, 4297. doi: 10.3390/rs14174297
Hautière N., Tarel J.-P., DIDIER A., Dumont E. (2008). Blind contrast enhancement assessment by gradient ratioing at visible edges. Image Anal. Stereology 27, 87–95. doi: 10.5566/ias.v27.p87-95
He K., Sun J., Tang X. (2011). Single image haze removal using dark channel prior. IEEE Trans. Pattern Anal. Mach. Intell. 33(12), 2341–2353. doi: 10.1109/TPAMI.2010.168
Hou G., Li J., Wang G., Yang H., Huang B., Pan Z. (2020a). A novel dark channel prior guided variational framework for underwater image restoration. J. Visual Communication Image Representation 66, 102732. doi: 10.1016/j.jvcir.2019.102732
Hou G., Zhao X., Pan Z., Yang H., Tan L., Li J. (2020b). Benchmarking underwater image enhancement and restoration, and beyond. IEEE Access 8, 122078–122091. doi: 10.1109/ACCESS.2020.3006359
Huang S., Wang K., Liu H., Chen J., Li Y. (2023) Contrastive semi-supervised learning for underwater image restoration via reliable bank. Available at: http://arxiv.org/abs/2303.09101 (Accessed 27 Apr. 2023).
Jiang Y., Gong X., Liu D., Cheng Y., Fang C., Shen X., et al. (2021). Enlightengan: deep light enhancement without paired supervision. IEEE Trans. image Process. 30, 2340–2349. doi: 10.1109/TIP.2021.3051462
Jin Y., Yang W., Tan R. T. (2022). Unsupervised night image enhancement: when layer decomposition meets light-effects suppression. In: Computer Vision – ECCV 2022. eds., Avidan S., Brostow G., Cissé M., Farinella G.M., Hassner T., Cham: Springer Nature Switzerland, pp.404–421.
Kar A., Dhara S. K., Sen D., Biswas P. K. (2021). Zero-shot single image restoration through controlled perturbation of koschmieder’s model (IEEE) (Accessed 14 May 2022).
Kimmel R., Elad M., Shaked D., Keshet R., Sobel I. (2003). A variational framework for retinex. Int. J. Comput. Vision 52, 7–23. doi: 10.1023/A:1022314423998
Koschmieder H. (1924). Theorie der horizontalen sichtweite. Beitrage zur Physik der freien Atmosphare, 33–53.
Land E. H. (1977). The retinex theory of color vision. Sci. Am. 2376, 108–128. doi: 10.1038/scientificamerican1277-108
Land E. H., McCann J. J. (1971). Lightness and retinex theory. J. Optical Soc. America 611, 1. doi: 10.1364/JOSA.61.000001
Li C., Anwar S., Hou J., Cong R., Guo C., Ren W. (2021a). Underwater image enhancement via medium transmission-guided multi-color space embedding. IEEE Trans. Image Process. 30, 4985–5000. doi: 10.1109/TIP.2021.3076367
Li C., Guo J., Cong R., Pang Y., Wang B. (2016a). Underwater image enhancement by dehazing with minimum information loss and histogram distribution prior. IEEE Trans. Image Process. 2512, 5664–5677. doi: 10.1109/TIP.2016.2612882
Li C., Guo C., Han L., Jiang J., Cheng M.-M., Gu J., et al. (2021b). Low-light image and video enhancement using deep learning: a survey. IEEE Trans. Pattern Anal. Mach. Intell. 4412, 9396–9416. doi: 10.1109/TPAMI.2021.3126387
Li C., Guo C., Loy C. C. (2021c). Learning to enhance low-light image via zero-reference deep curve estimation. IEEE Trans. Pattern Anal. Mach. Intell. 448, 4225–4238. doi: 10.1109/TPAMI.2021.3063604
Li C., Guo J., Pang Y., Chen S., Wang J. (2016b). Single underwater image restoration by blue-green channels dehazing and red channel correction (IEEE) (Accessed 2 Feb. 2023).
Li C., Guo J., Porikli F., Pang Y. (2018a). LightenNet: a convolutional neural network for weakly illuminated image enhancement. Pattern Recognition Lett. 104, 15–22. doi: 10.1016/j.patrec.2018.01.010
Li C., Guo C., Ren W., Cong R., Hou J., Kwong S., et al. (2019). An underwater image enhancement benchmark dataset and beyond. IEEE Trans. Image Process. 29, 4376–4389. doi: 10.1109/TIP.2019.2955241
Li Y., Lu H., Li K.-C., Kim H., Serikawa S. (2018b). Non-uniform de-scattering and de-blurring of underwater images. Mobile Networks Appl. 232, 352–362. doi: 10.1007/s11036-017-0933-7
Li T., Rong S., Cao X., Liu Y., Chen L., He B. (2020). Underwater image enhancement framework and its application on an autonomous underwater vehicle platform. Optical Eng. 5908, 1. doi: 10.1117/1.OE.59.8.083102
Liu X., Gao Z., Chen B. M. (2021). IPMGAN: integrating physical model and generative adversarial network for underwater image enhancement. Neurocomputing 453, 538–551. doi: 10.1016/j.neucom.2020.07.130
Liu Y., Xu H., Shang D., Li C., Quan X. (2019). An underwater image enhancement method for different illumination conditions based on color tone correction and fusion-based descattering. Sensors 1924, 5567. doi: 10.3390/s19245567
Liu Y., Xu H., Zhang B., Sun K., Yang J., Li B., et al. (2022). Model-based underwater image simulation and learning-based underwater image enhancement method. Information 134, 187. doi: 10.3390/info13040187
Lore K. G., Akintayo A., Sarkar S. (2017). LLNet: a deep autoencoder approach to natural low-light image enhancement. Pattern Recognition 61, 650–662. doi: 10.1016/j.patcog.2016.06.008
Lu H., Li Y., Uemura T., Kim H., Serikawa S. (2018). Low illumination underwater light field images reconstruction using deep convolutional neural networks. Future Generation Comput. Syst. 82, 142–148. doi: 10.1016/j.future.2018.01.001
Lu H., Li Y., Xu X., Li J., Liu Z., Li X., et al. (2016). Underwater image enhancement method using weighted guided trigonometric filtering and artificial light correction. J. Visual Communication Image Representation 38, 504–516. doi: 10.1016/j.jvcir.2016.03.029
Lu H., Li Y., Zhang L., Serikawa S. (2015). Contrast enhancement for images in turbid water. J. Optical Soc. America A 325, 886. doi: 10.1364/JOSAA.32.000886
Lu J., Yuan F., Yang W., Cheng E. (2021). An imaging information estimation network for underwater image color restoration. IEEE J. Oceanic Eng. 464, 1228–1239. doi: 10.1109/JOE.2021.3077692
Marques T. P., Branzan Albu A. (2020)L 2 UWE: a framework for the efficient enhancement of low-light underwater images using local contrast and multi-scale fusion (IEEE) (Accessed 21 Dec. 2021).
Menaker D., Treibitz T., Avidan S. (2017). Color restoration of underwater images. In Proceedings of the British machine vision conference (BMVC), Eds., T.K. Kim, S. Zafeiriou, G. Brostow and K. Mikolajczyk (Durham, UK: BMVA Press.) 44.1-44.12.
Mittal A., Moorthy A. K., Bovik A. C. (2012). No-reference image quality assessment in the spatial domain. IEEE Trans. Image Process. 2112, 4695–4708. doi: 10.1109/TIP.2012.2214050
Narasimhan S. G., Nayar S. K. (2000). Chromatic framework for vision in bad weather (IEEE Comput. Soc) (Accessed 19 Jun. 2022).
Narasimhan S. G., Nayar S. K. (2003). Contrast restoration of weather degraded images. IEEE Trans. ON Pattern Anal. AND Mach. Intell. 256, 12. doi: 10.1109/TPAMI.2003.1201821
Narasimhan S. G., Nayar S. K. (2008). Vision and the atmosphere (ACM Press) (Accessed 19 Jun. 2022).
NOAA (2022) What is the “deep” ocean?: ocean exploration facts: NOAA office of ocean exploration and research. Available at: https://oceanexplorer.noaa.gov/facts/deep-ocean.html (Accessed 2 Feb. 2023). doi: 10.6119/JMST.201808_26(4).0006
Pan P., Yuan F., Cheng E. (2018). Underwater image de-scattering and enhancing using dehazenet and HWD. J. Mar. Sci. Technol. 264, 6. doi: 10.6119/JMST.201808_26(4).0006
Panetta K., Gao C., Agaian S. (2016). Human-Visual-System-Inspired underwater image quality measures. IEEE J. Oceanic Eng. 413, 541–551. doi: 10.1109/JOE.2015.2469915
Paulus E. (2021). Shedding light on deep-Sea biodiversity–a highly vulnerable habitat in the face of anthropogenic change. Front. Mar. Sci. 8, 667048. doi: 10.3389/fmars.2021.667048
Peng Y.-T., Cao K., Cosman P. C. (2018). Generalization of the dark channel prior for single image restoration. IEEE Trans. Image Process. 276, 2856–2868. doi: 10.1109/TIP.2018.2813092
Peng Y.-T., Cosman P. C. (2017). Underwater image restoration based on image blurriness and light absorption. IEEE Trans. Image Process. 264, 1579–1594. doi: 10.1109/TIP.2017.2663846
Peng Y.-T., Zhao X., Cosman P. C. (2015). Single underwater image enhancement using depth estimation based on blurriness (IEEE) (Accessed 30 Aug. 2021).
Peng L., Zhu C., Bian L. (2023). “U-Shape transformer for underwater image enhancement,” in Computer Vision–ECCV 2022 Workshops, L. Karlinsky, T. Michaeli and K. Nishino, eds., (Cham: Springer Nature Switzerland). 290–307. doi: 10.1007/978-3-031-25063-7_18
Porto Marques T., Branzan Albu A., Hoeberechts M. (2019). A contrast-guided approach for the enhancement of low-lighting underwater images. J. Imaging 510, 79. doi: 10.3390/jimaging5100079
Ren X., Li M., Cheng W.-H., Liu J. (2018). “Joint enhancement and denoising method via sequential decomposition,” in 2018 IEEE international symposium on circuits and systems (ISCAS) (Florence, Italy: IEEE), 1–5. doi: 10.1109/ISCAS.2018.8351427
Ronneberger O., Fischer P., Brox T. (2015). U-Net: convolutional networks for biomedical image segmentation. In: Medical image computing and computer-assisted intervention – MICCAI 2015, lecture notes in computer science (Cham: Springer International Publishing). Available at: http://link.springer.com/10.1007/978-3-319-24574-4_28 (Accessed 27 Oct. 2021).
Saleh A., Sheaves M., Jerry D., Azghadi M. R. (2022). Adaptive uncertainty distribution in deep learning for unsupervised underwater image enhancement. Available at: http://arxiv.org/abs/2212.08983 (Accessed 27 Apr. 2023).
Song W., Wang Y., Huang D., Tjondronegoro D. (2018). A rapid scene depth estimation model based on underwater light attenuation prior for underwater image restoration. In: Advances in multimedia information processing – PCM 2018, lecture notes in computer science (Cham: Springer International Publishing). Available at: http://link.springer.com/10.1007/978-3-030-00776-8_62 (Accessed 31 Mar. 2022).
Tan R. T. (2008). “Visibility in bad weather from a single image.,” in Computer Vision and Pattern Recognition, 2008. CVPR 2008. IEEE Conference on (Anchorage, AK, USA: IEEE). 1–8. doi: 10.1109/CVPR.2008.4587643
Wang Z., Cun X., Bao J., Zhou W., Liu J., Li H. (2022). Uformer: a general U-shaped transformer for image restoration (IEEE) (Accessed 11 Feb. 2023).
Wang Y.-F., Liu H.-M., Fu Z.-W. (2019c). Low-light image enhancement via the absorption light scattering model. IEEE Trans. Image Process. 2811, 5679–5690. doi: 10.1109/TIP.2019.2922106
Wang Y., Song W., Fortino G., Qi L.-Z., Zhang W., Liotta A. (2019b). An experimental-based review of image enhancement and image restoration methods for underwater imaging. IEEE Access 7, 140233–140251. doi: 10.1109/ACCESS.2019.2932130
Wang R., Zhang Q., Fu C.-W., Shen X., Zheng W.-S., Jia J. (2019a)Underexposed photo enhancement using deep illumination estimation (IEEE) (Accessed 2 Feb. 2023).
Wang N., Zheng H., Zheng B. (2017). Underwater image restoration via maximum attenuation identification. IEEE Access 5, 18941–18952. doi: 10.1109/ACCESS.2017.2753796
Wen H., Tian Y., Huang T., Gao W. (2013). Single underwater image enhancement with a new optical model (IEEE) (Accessed 25 Mar. 2022).
Yan K., Zhou Y. (2020)Underwater image processing by an adversarial network with feedback control. In: Pattern recognition and computer vision, lecture notes in computer science (Cham: Springer International Publishing). Available at: http://link.springer.com/10.1007/978-3-030-60633-6_38 (Accessed 19 Mar. 2023).
Yang M., Sowmya A. (2015). An underwater color image quality evaluation metric. IEEE Trans. Image Process. 2412, 6062–6071. doi: 10.1109/TIP.2015.2491020
Yu R., Liu W., Zhang Y., Qu Z., Zhao D., Zhang B. (2018)DeepExposure: learning to expose photos with asynchronously reinforced adversarial learning. In: Advances in neural information processing systems (Curran Associates, Inc). Available at: https://proceedings.neurips.cc/paper/2018/hash/a5e0ff62be0b08456fc7f1e88812af3d-Abstract.html (Accessed 2 Nov. 2022).
Zhang Y., Guo X., Ma J., Liu W., Zhang J. (2021). Beyond brightening low-light images. Int. J. Comput. Vision 1294, 1013–1037. doi: 10.1007/s11263-020-01407-x
Zhang Q., Nie Y., Zheng W.-S. (2019a). “Dual illumination estimation for robust exposure correction,” in Computer graphics forum (England: Wiley Online Library), 243–252. doi: 10.1111/cgf.13833
Zhang M., Peng J. (2018). Underwater image restoration based on a new underwater image formation model. IEEE Access 6, 58634–58644. doi: 10.1109/ACCESS.2018.2875344
Zhang Y., Zhang J., Guo X. (2019b). Kindling the darkness: a practical low-light image enhancer (Accessed 27 Sep. 2022).
Zhang W., Zhuang P., Sun H.-H., Li G., Kwong S., Li C. (2022). Underwater image enhancement via minimal color loss and locally adaptive contrast enhancement. IEEE Trans. Image Process. 31, 3997–4010. doi: 10.1109/TIP.2022.3177129
Zhao X., Jin T., Qu S. (2015). Deriving inherent optical properties from background color and underwater image enhancement. Ocean Eng. 94, 163–172. doi: 10.1016/j.oceaneng.2014.11.036
Zhou J., Liu Z., Zhang W., Zhang D., Zhang W. (2021a). Underwater image restoration based on secondary guided transmission map. Multimedia Tools Appl. 805, 7771–7788. doi: 10.1007/s11042-020-10049-7
Zhou J., Wang Y., Zhang W., Li C. (2021b). Underwater image restoration via feature priors to estimate background light and optimized transmission map. Optics Express 2918, 28228. doi: 10.1364/OE.432900
Zhou J., Yang T., Ren W., Zhang D., Zhang W. (2021c). Underwater image restoration via depth map and illumination estimation based on a single image. Optics Express 29 (19), 29864. doi: 10.1364/OE.427839
Zhu A., Zhang L., Shen Y., Ma Y., Zhao S., Zhou Y. (2020). Zero-shot restoration of underexposed images via robust retinex decomposition (IEEE) (Accessed 9 Aug. 2022).
Zhuang P., Li C., Wu J. (2021). Bayesian Retinex underwater image enhancement. Eng. Appl. Artif. Intell. 101, 104171. doi: 10.1016/j.engappai.2021.104171
Keywords: shallow-sea image restoration, deep-sea image restoration, image formation, physical model, prior, deep learning
Citation: Song W, Liu Y, Huang D, Zhang B, Shen Z and Xu H (2023) From shallow sea to deep sea: research progress in underwater image restoration. Front. Mar. Sci. 10:1163831. doi: 10.3389/fmars.2023.1163831
Received: 11 February 2023; Accepted: 09 May 2023;
Published: 31 May 2023.
Edited by:
Haiyong Zheng, Ocean University of China, ChinaCopyright © 2023 Song, Liu, Huang, Zhang, Shen and Xu. This is an open-access article distributed under the terms of the Creative Commons Attribution License (CC BY). The use, distribution or reproduction in other forums is permitted, provided the original author(s) and the copyright owner(s) are credited and that the original publication in this journal is cited, in accordance with accepted academic practice. No use, distribution or reproduction is permitted which does not comply with these terms.
*Correspondence: Huifang Xu, MTcwNjlAZ2VuY2guZWR1LmNu