- 1CNR-ISMAR, Istituto di Scienze Marine, Consiglio Nazionale delle Ricerche, Rome, Italy
- 2AEQUORA LDA, Lisboa, Portugal
- 3Finnish Environment Institute (SYKE), Helsinki, Finland
- 4Commonwealth Scientific and Industrial Research Organization (CSIRO) Environment, Brisbane, QLD, Australia
The Baltic Sea is characterized by large gradients in salinity, high concentrations of colored dissolved organic matter, and a phytoplankton phenology with two seasonal blooms. Satellite retrievals of chlorophyll-a concentration (chl-a) are hindered by the optical complexity of this basin and the reduced performance of the atmospheric correction in its highly absorbing waters. Within the development of a regional ocean color operational processing chain for the Baltic Sea based on Sentinel-3 Ocean and Land Colour Instrument (OLCI) full-resolution data, the performance of four atmospheric correction processors for the retrieval of remote-sensing reflectance (Rrs) was analyzed. Assessments based on three Aerosol Robotic Network-Ocean Color (AERONET-OC) sites and shipborne hyperspectral radiometers show that POLYMER was the best-performing processor in the visible spectral range, also providing a better spatial coverage compared with the other processors. Hence, OLCI Rrs spectra retrieved with POLYMER were chosen as input for a bio-optical ensemble scheme that computes chl-a as a weighted sum of different regional multilayer perceptron neural nets. This study also evaluated the operational Rrs and chl-a datasets for the Baltic Sea based on OC-CCI v.6. The chl-a retrievals based on OC-CCI v.6 and OLCI Rrs, assessed against in-situ chl-a measurements, yielded similar results (OC-CCI v.6: R2 = 0.11, bias = −0.22; OLCI: R2 = 0.16, bias = −0.03) using a common set of match-ups for the same period. Finally, an overall good agreement was found between chl-a retrievals from OLCI and OC-CCI v.6 although differences between Rrs were amplified in terms of chl-a estimates.
1 Introduction
The Baltic Sea (Figure 1) is a brackish shallow semi-enclosed basin characterized by large inputs of pollutants and nutrients from natural and anthropogenic sources combined with a limited water exchange with the open ocean through the Danish Straits in the southwest, causing large latitudinal gradients of salinity and dissolved organic matter (Omstedt et al., 2004; Leppäranta and Myrberg, 2009). As a consequence of the strong anthropogenic pressure, pollution (HELCOM, 2018), eutrophication episodes (Andersen et al., 2011; Fleming-Lehtinen et al., 2015; Heiskanen et al., 2019), and/or phytoplankton blooms (Wasmund et al., 2011; Kahru et al., 2018; Hjerne et al., 2019) threaten its fragile ecosystems. Growing concern about the basin’s health is raised by the Baltic Marine Environment Protection Commission (Helsinki Commission, HELCOM) (HELCOM, 2007). The Baltic Sea is characterized by high concentrations of colored dissolved organic matter (CDOM). Rivers are the main CDOM source, which follows a general dilution gradient from north to south with a large spatiotemporal variability driven by processes such as ice melting, rainfall, phytoplankton blooms, or photodegradation (Berthon and Zibordi, 2010; Ylöstalo et al., 2016; Simis et al., 2017; Kratzer and Moore, 2018).
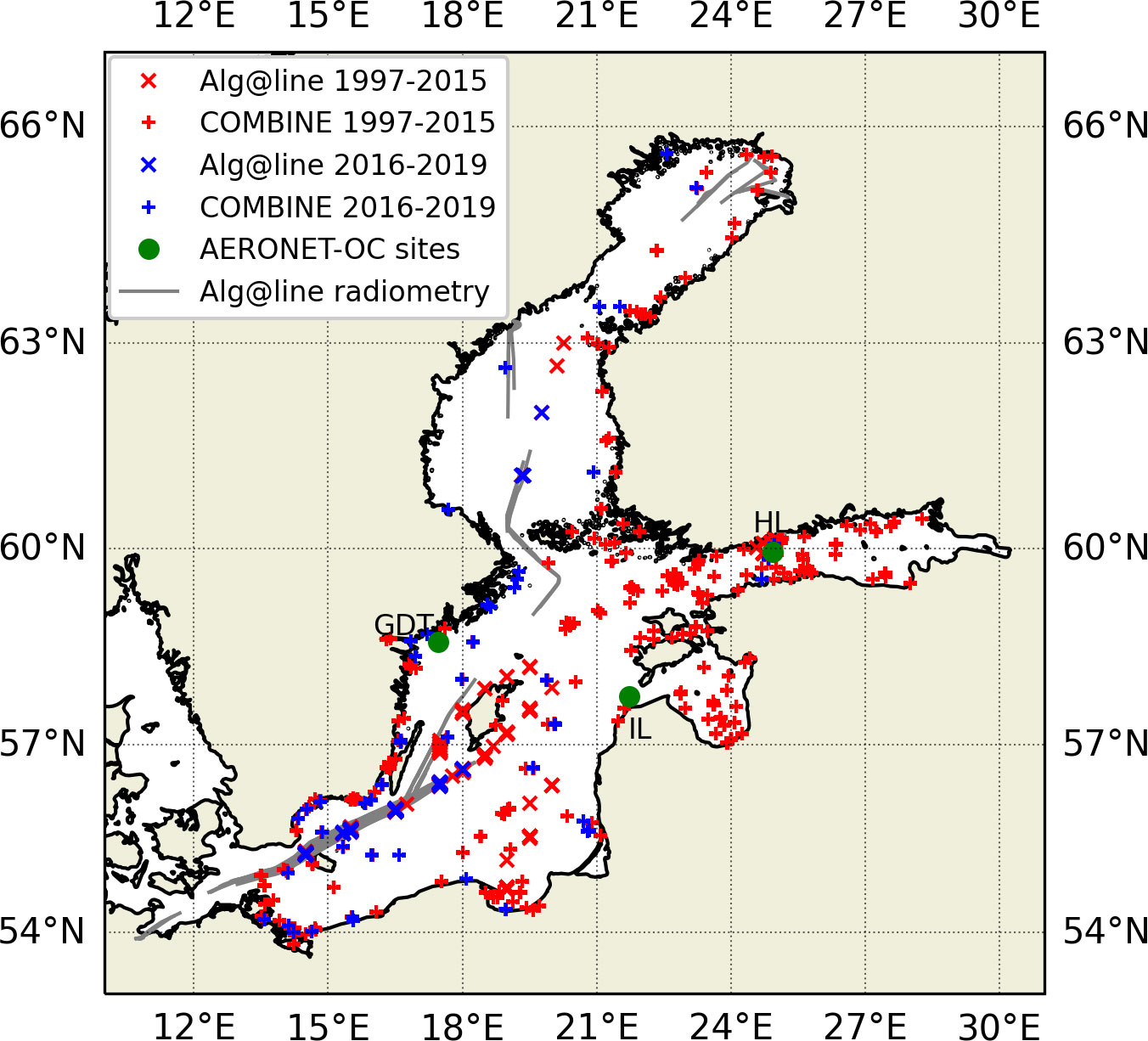
Figure 1 Study area showing the location of the in-situ measurements. The crosses identify the in-situ Alg@line chl-a data collected by the SYKE, whereas the pluses are chl-a data extracted from the COMBINE database (red points: 1997–2015; blue points: OLCI period from 2016 to 2019) (Section 2.2.2). The green dots mark the location of the AERONET-OC sites (GDT, Gustav Dalen Tower; HL, Helsinki Lighthouse; IL, Irbe Lighthouse; Section 2.2.1). Gray lines show the trajectories of the ships during the collection of the Alg@line shipborne radiometry data by SYKE in 2016 (Section 2.2.1).
Two seasonal phytoplankton blooms are usually observed in most areas of the Baltic Sea (Wasmund et al., 2011; Kahru et al., 2018; Brando et al., 2021). Firstly, a strong spring bloom dominated by diatoms and dinoflagellates is responsible for most of the annual primary production in the area (Simis et al., 2017; Zhang et al., 2018). This spring bloom progresses from south to north due to light and nitrogen limitation, and it can cause anoxia and hypoxia events in the bottom layer because of the fast diatom sedimentation (Hjerne et al., 2019). After a minimum production in early summer (May–June), phosphorus excess and increasing surface water temperature lead to the blooming of nitrogen-fixing cyanobacteria, causing extensive and prolongated surface and near-surface accumulations of filamentous species during calm weather periods in July and August (Kahru et al., 1994; Finni et al., 2001; Kahru et al., 2007; Kahru et al., 2018).
Chlorophyll-a (chl-a) concentration (measured in mg m−3) is one of the most relevant indicators for water quality monitoring within the Baltic Sea Action Plan implemented by HELCOM, as it is useful for assessing the eutrophication status and a good proxy for phytoplankton blooms (HELCOM, 2017; HELCOM, 2019; Ahlman et al., 2020). Compared with chl-a data from sampling stations, in-situ platforms, or automated ship measurements, chl-a maps derived from ocean color (OC) satellite images provide a synoptic view of the phytoplankton spatial distribution. Although data availability in terms of spatial coverage and temporal resolution is limited by the cloud cover, it can be significantly enhanced by merging data from different sensors (Groom et al., 2019; O’Reilly and Werdell, 2019; Sathyendranath et al., 2019).
Accuracy and reliability of chl-a retrievals from OC data depend on two related factors: 1) the optical characteristics of the water and 2) the performance of the atmospheric correction (AC) algorithms converting the spectral top-of-atmosphere (TOA) radiances measured by the satellite sensors to spectral remote-sensing reflectance (Rrs, defined as the ratio of the water-leaving radiance and the downwelling irradiance and measured in sr−1) used as input in chl-a estimation algorithms (Brewin et al., 2015; Sathyendranath et al., 2019). Despite the good results in open-ocean and high-scattering coastal areas (Blondeau-Patissier et al., 2014; O’Reilly and Werdell, 2019), retrieval of reliable OC products from high-absorbing waters such as the Baltic Sea is a challenging task. High absorption coefficients related to the high CDOM concentrations (with aCDOM values exceeding 1.0 m−1 at 440 nm, Ylöstalo et al., 2016) in combination with relative low sun elevation lead to low Rrs values, especially in the blue part of the spectrum. Therefore, AC algorithms show a limited performance producing inaccurate Rrs spectra with low and even negative values (Attilla et al., 2013; Beltrán-Abaunza et al., 2014; Alikas et al., 2020; Brando et al., 2021; Tilstone et al., 2022).
Regarding chl-a retrieval, standard blue-green band-ratio algorithms have been reported as not suitable for the Baltic Sea because they tend to a significant overestimation (Darecki and Stramski, 2004; Odermatt et al., 2012; D’Alimonte et al., 2016; Pitarch et al., 2016; Ligi et al., 2017; Kratzer and Moore, 2018). Better results have been achieved with regionalized blue-green ratios (Darecki and Stramski, 2004; Attilla et al., 2013; Ligi et al., 2017), red-edge bands (Ligi et al., 2017), or neural network (NN) algorithms based on different sets of Rrs values (Kratzer and Vinterhav, 2010; Hieronymi et al., 2017; Toming et al., 2017; Kyryliuk and Kratzer, 2019), although accuracy is still hampered by the optical complexity of the basin and the low performance of the AC processors.
Within the Copernicus Marine Service (CMEMS, Le Traon et al., 2019; von Schuckmann et al., 2022), two operational chl-a data streams are available for the Baltic Sea based on OLCI and on merged multisensor time series. These data streams are based on sensor merging to improve the daily spatial coverage at 300 m and 1 km resolutions to support the operational oceanography users and environmental reporting needs (Le Traon et al., 2019; Sathyendranath et al., 2019; von Schuckmann et al., 2022).
Brando et al. (2021) proposed a new ensemble approach based on multilayer perceptron neural network (MLP) bio-optical algorithms (ENS-MLP), with results outperforming those based on other methods reported in the literature. This new approach was implemented in a fully reprocessed multisensor time series of Rrs and chl-a data at ~1 km spatial resolution (OCEANCOLOUR_BAL_BGC_L3_MY_009_133, 2023).
This work documents the implementation of the new Rrs and chl-a level-3 datasets for the Baltic Sea based on the complete Sentinel-3 A and B OLCI time series (2016 to present) of OC images at full resolution (300 m), using the same ENS-MLP approach for chl-a retrievals. To this aim, the following steps were carried out: 1) the selection of the best AC processor to obtain OLCI level-2 Rrs from level-1 data, 2) the assessment of the new OLCI level-3 Rrs dataset and comparison with the CMEMS multisensor dataset at 1 km resolution, and 3) the comparative validation analysis of the multisensor and OLCI level-3 chl-a datasets based on the ENS-MLP approach. The validation results were based on several in-situ data sources: automated radiometry from the Aerosol Robotic Network-Ocean Color (AERONET-OC) sites in the Baltic Sea, shipborne hyperspectral radiometry collected by the Finnish Environment Institute (SYKE), and chl-a concentrations from Alg@line and COMBINE datasets.
The remainder of this document is structured as follows: Section 2 introduces the data and methods used in this work, describing the validation exercises; the results presented in Section 3 include a match-up summary, validation results for level-2 OLCI Rrs, level-3 Rrs and chl-a, and a comparison between CMEMS-OLCI level-3 and OC-CCI v.6 datasets. The discussion and concluding remarks are addressed in Sections 4 and 5, respectively.
2 Materials and methods
2.1 Satellite Rrs
2.1.1 Level-2 reflectance datasets
OLCI Rrs spectra were extracted from Sentinel-3 level-2 products processed by four different atmospheric correction algorithms: OLCI level-2 Water Full Resolution (WFR), POLYMER, Case 2 Regional CoastColour (C2RCC), and S3 FUB-CSIRO Coastal Water Processor (hereafter S3 FUB-CSIRO). All the level-2 products were derived from a set of Sentinel-3 level-1b Full Resolution (FR) images at 300 m resolution acquired over the Baltic Sea for both Sentinel-3A (between April 2016 and September 2022) and Sentinel-3B (between May 2018 and September 2022) missions.
The OLCI WFR products were available from the Ocean Colour baseline collection OL_L2M.003, processed by EUMETSAT using the OLCI L2 processor IPF-OL-2 version 07 (EUMETSAT, 2021; Zibordi et al., 2022). This product provides water-leaving reflectance data at the OLCI spectral bands between 400 nm and 1,020 nm except those bands dedicated to atmospheric measurements (Table 1). It also contains the pixel classification band Water Quality and Science Flags (WQSFs), providing information about invalid and/or suspicious pixels (Table 2).
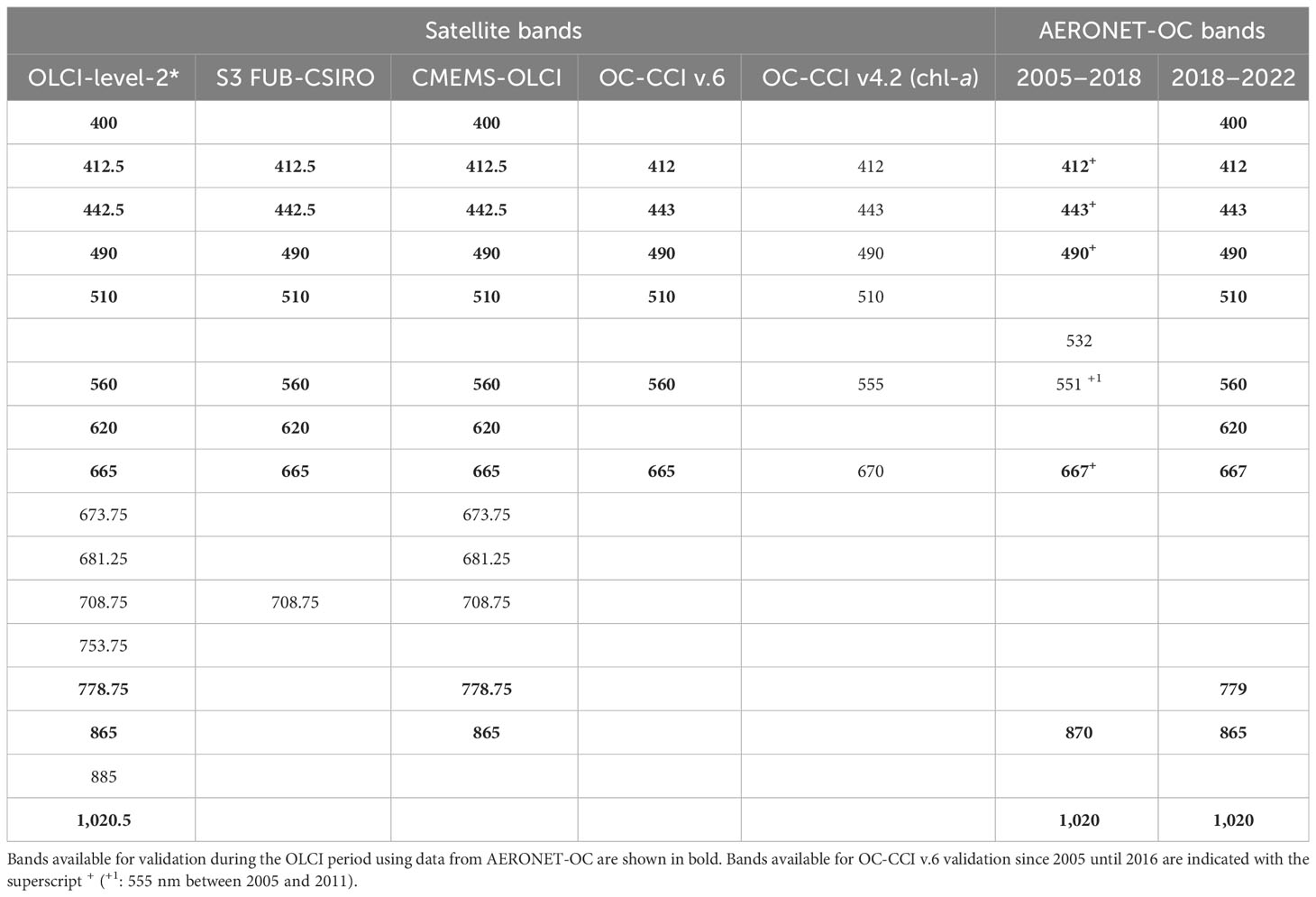
Table 1 Available wavelengths from OLCI level-2 (*: OLCI WFR, C2RCC, and POLYMER) and level-3 products, multisensor OC-CCI products, and AERONET-OC sites.
POLYMER is a coupled ocean–atmosphere algorithm that applies polynomial functions to model the (TOA) spectral reflectance and sun glint, and it applies a forward bio-optical model for the water component. It was originally developed for MERIS from an atmospheric correction processor for case-1 waters that is able to deal with sun glint (Steinmetz et al., 2011; Steinmetz and Ramon, 2018). In this study, we applied POLYMERv.4.14 (https://forum.hygeos.com/viewtopic.php?f=5&t=155), which is already adapted to OLCI, providing fully normalized water-leaving reflectance data for 16 bands between 400 nm and 1,020.5 nm (Table 1), as well as a flag band (bitmask) with pixel classification (Table 2).
C2RCC applies parametrized radiative transfer models based on the successive order of scattering (SOS) technique to obtain a large database of simulated TOA radiances, which is then used as input to train a set of neural networks (NN) for the retrieval of water-leaving reflectance as well as other water products (Doerffer and Schiller, 2007; Brockmann et al., 2016). In this work, we applied C2RCC v.2 (https://c2rcc.org/neural-nets/), providing, among other outputs, Rrs data for 16 OLCI bands between 400 nm and 1,020.5 nm (Table 1) and two flag bands (c2rcc_flag and quality_flags) with pixel identification information (Table 2).
The S3 FUB-CSIRO Coastal Water Processor is an ensemble neural network inversion trained with extensive coupled ocean–atmosphere radiative transfer simulations (Schroeder et al., 2022). The algorithm was adapted to an approach previously developed by Schroeder et al. (2007); Schroeder et al. (2003) for MERIS. The S3 FUB-CSIRO processor version 1.0.0.0.5.3 used in this study, therefore, does not provide outputs for OLCI bands at 400 nm, 673.75 nm, 681.25 nm, and above 708.75 nm (Table 1). The algorithm performs a pixel-per-pixel direct inversion of the TOA radiance signal into spectral remote sensing reflectance at mean sea level and selected water quality parameters. It also provides per-pixel sensor and inverse model uncertainties, which were not further evaluated in this study. Quality control was applied by using the flag band quality_flags for masking (Table 2), which are based on the level-1b flags but not the additional neural network-specific input/output out-of-range flags.
2.1.2 Level-3 reflectance datasets
Within CMEMS, two operational ocean color time series are available for the Baltic Sea: merged OLCI (Sentinel-3A and Sentinel-3B) at 300 m spatial resolution and merged multisensor at 1 km resolution.
The CMEMS-OLCI level-3 Rrs dataset for the Baltic Sea merges OLCI level-2 Rrs spectra retrieved from level-1b FR images for both Sentinel-3A and Sentinel-3B missions using POLYMERv.4.14. Rrs values are remapped using the “nearest value” interpolation on an equi-rectangular grid at 300 m resolution. As in the EUMETSAT v.3.0.1 reprocessing the System Vicarious Calibration gains that have been implemented by EUMETSAT for both sensors, Rrs values are merged without any bias correction. The dataset has been produced operationally by CNR as daily data since April 2016 to present for 11 OLCI bands between 400 nm and 708.74 nm (Table 1) and is available at the Copernicus Marine Service in near real-time (OCEANCOLOUR_BAL_BGC_L3_NRT_009_131, Baltic Sea Ocean Colour Plankton, Reflectances, Transparency and Optics L3 NRT daily observations, 2023) and as a fully reprocessed multiyear time series (OCEANCOLOUR_BAL_BGC_L3_MY_009_133, 2023).
The CMEMS multisensor level-3 Rrs dataset for the Baltic Sea is derived from the Ocean Color (OC)-Climate Change Initiative (CCI) v.6 processor (OC-CCI v.6) implemented by Plymouth Marine Laboratory (PML) (Sathyendranath et al., 2019; OC-CCI, 2022; Sathyendranath et al., 2022). Data were obtained from different sensors and processed with specific atmospheric correction algorithms to obtain L2 Rrs spectra: NASA standard atmospheric correction was applied to the SeaWiFS NASA R2018.0 reprocessed dataset, while POLYMER was selected for MERIS (ESA 4th reprocessing) MODIS-AQUA and VIIRS (NASA R2018.0, included only until the end of 2019) and both OLCI sensors (EUMETSAT v.3.0.1 reprocessing). Rrs output values from each sensor were band-shifted using the inverse and direct application of the Quasi-Analytical Algorithm (QAA) algorithm (Lee et al., 2014; Mélin and Sclep, 2015) to six MERIS bands (Table 1) and then bias-corrected and merged at 1 km resolution, providing a consistent time series from 1997 to 2022.
2.2 In situ data for validation
2.2.1 In situ automated radiometry
For the validation of satellite-derived Rrs, this study relies on two sources of in-situ automated above-water radiometry: the AERONET-OC and the Alg@line hyperspectral datasets were collected following the same above-water radiometry approach (Zibordi et al., 2009; Simis and Olsson, 2013).
In-situ radiometry data were available from the automated measurements collected by AERONET-OC at three sites in the Baltic Sea: Gustav Dalen Tower (58.594°N, 17.467°E), with data from 2005 to 2022; Helsinki Lighthouse (59°949°N, 24.925°E), from 2006 to 2019; and Irbe Lighthouse (57.751°N, 21.723°E), from 2018 to 2022. Due to the illumination conditions of the Baltic Sea, data are usually available between May and September, with some single days in March, April, or October. We used level-2 data available from the AERONET-OC web page (https://aeronet.gsfc.nasa.gov), consisting of quality-controlled measurements of normalized water-leaving radiances (LwN) corrected for bidirectional effects and referred to nadir (Zibordi et al., 2009, Zibordi et al., 2020). AERONET-OC provides multispectral data (Table 1): 8 bands between 412 nm and 1,020 nm until 2018 (adapted to MERIS) and 11 bands between 400 nm and 1,020 nm since 2019 when instruments were modified for OLCI validation (Zibordi et al., 2009, 2020).
The Alg@line hyperspectral dataset was collected by SYKE within the BONUS FerryScope project (Simis and Olsson, 2013; Simis et al., 2021) from April to September 2016. Data were acquired every 15 ss using a three-spectroradiometer system mounted approximately 7 m from the sea surface on board the merchant vessels Finnmaid (Finnlines) and Transpaper (Transatlantic). Downwelling irradiance (Ed) was collected using a TriOS RAMSES-ACC unit with a cosine collector, and sky (Ld) and water radiance (Ls) were measured with RAMSES-ARC sensors with a 7° field of view. The dataset was filtered to eliminate measurements with an obstructed view of the sea or affected by underexposure or oversaturation. Rrs spectra were derived by correcting for the reflection of sky radiance at the water surface using Ed, Lt, and Ls measurements. More details about data collection, processing, and quality control are available from Simis et al. (2021); Qin et al. (2017), and Warren et al. (2019).
2.2.2 In situ chl-a datasets
We used two in-situ datasets in order to validate the chl-a concentrations retrieved from satellite Rrs for the Baltic Sea: Alg@line and COMBINE.
The Alg@line dataset is derived from a set of water samples collected by SYKE from 1997 to 2017 using an acquisition system installed on board ferries operating in the Helsinki–Travemünde, Helsinki–Stockholm, and Kemi–Travemünde transects. Water samples (from surface to 5 m depth) were filtered using glass fiber filters (Whatman GF/F, 0.7-μm nominal pore size), chlorophyll-a was extracted with ethanol, and concentrations were determined by fluorometry using a Jasco FP-750 spectrofluorometer or a Perkin-Elmer LS2-b fluorometer with an excitation wavelength of 413 nm and emission wavelength of 668 nm (Fleming and Kaitala, 2006; Kaitala et al., 2008).
The COMBINE dataset, available from the International Council for the Exploration of the Sea (ICES) oceanographic database, includes chl-a measurements gathered by several institutions from the 1970s to the present within the HELCOM marine monitoring program (HELCOM, 2017; HELCOM, 2019). Chl-a concentrations were obtained using different analytical protocols and techniques, from fluorimetry to spectrophotometry and HLPC, but always meeting the quality requirements established by the program. We excluded from the analysis data acquired in the Skagerrak and Kattegat regions since these basins are characterized by physical and optical water properties deemed different from the actual Baltic Sea (Ligi et al., 2017; Simis et al., 2017).
2.3 Chl-a retrieval algorithm from satellite Rrs
Retrieval of chl-a concentrations from satellite Rrs in the Baltic Sea was based on the methodological approach proposed by Brando et al. (2021). It builds on a bio-optical ensemble scheme in which chl-a concentrations are estimated as a weighted sum of the outputs of different regional multilayer perceptron neural net (MLP) bio-optical algorithms developed using in-situ data available from the JRC/EC BiOMaP program (D’Alimonte et al., 2011; Zibordi et al., 2011). Weights defining the contribution of each individual MLP algorithm are dynamically established through the novelty index (D’Alimonte et al., 2014; Kajiyama et al., 2019; Brando et al., 2021).
Each MLP bio-optical algorithm uses as input Rrs values at a different subset of wavelengths, considering all the SeaWiFS bands implemented in the OC-CCI v4.2 product (Table 1): chl-aMLP6b (Rrs values at 412, 443, 490, 510, 555, and 670 nm), chl-aMLP_5b (Rrs values at 443, 490, 510, 555, and 670 nm), chl-aMLP_4b (Rrs values at 490, 510, 555, and 670 nm), and chl-aMLP_3b (Rrs values at 490, 510, and 555 nm).
Brando et al. (2021) tested two ensemble solutions based on four (chl-aENS4: chl-aMLP_6b, chl-aMLP_5b, chl-aMLP_4b, chl-aMLP_3b) and three (chl-aENS3: chl-aMLP_5b, chl-aMLP_4b, chl-aMLP_3b) MLP algorithms, evaluating their performance through a match-up analysis of chl-a retrievals from OC-CCI v4.2 time series against in-situ chl-a concentrations. Results showed that both ensemble solutions outperformed all the band-ratio regression algorithms based on Rrs spectral slopes instead of Rrs values at different bands and that chl-aENS3 outperformed chl-aENS4.
In this work, the performance of both chl-aENS3 and chl-aENS4 was evaluated through a match-up analysis of chl-a retrievals from satellite Rrs extracted from the CMEMS-OLCI level-3 Rrs dataset and OC-CCI v.6 datasets (see Section 2.1.2), against in-situ chl-a measurements from Alg@Line and COMBINE datasets (see Section 2.2.2). Only results for the best-performing solution, i.e., chl-aENS3, are shown in this document.
Chl-aENS3 was also the selected algorithm for the implementation of the new CMEMS-OLCI level-3 chl-a dataset for the Baltic Sea (OCEANCOLOUR_BAL_BGC_L3_MY_009_133, 2023; OCEANCOLOUR_BAL_BGC_L3_NRT_009_131, 2023), in which chl-a concentrations are retrieved from the CMEMS-OLCI level-3 Rrs dataset merging Rrs outputs from POLYMERv.4.14 (see Section 2.2.1). The new dataset was produced by CNR for the complete OLCI time series (from April 2016 to the present).
As chl-aENS3 was developed considering the SeaWiFS spectral bands available in the OC-CCI v.4.2 product, in this work, chl-a retrievals using Rrs values from CMEMS-OLCI or OC-CCI v.6 required a previous band shift to the SeaWiFS spectral bands. The band shift was performed through the inverse and direct application of the QAA algorithm (Mélin and Sclep, 2015; Lee et al., 2009) modified to ensure non-negative phytoplankton at any band (Brando et al., 2021).
2.4 Validation exercises
2.4.1 Workflow
Figure 2 summarizes the workflow for the validation exercises carried out in this work, all of them implemented using a Match-up Database File (MDB) infrastructure (EUMETSAT, 2019; González Vilas et al., submitted; González Vilas et al., 2023).
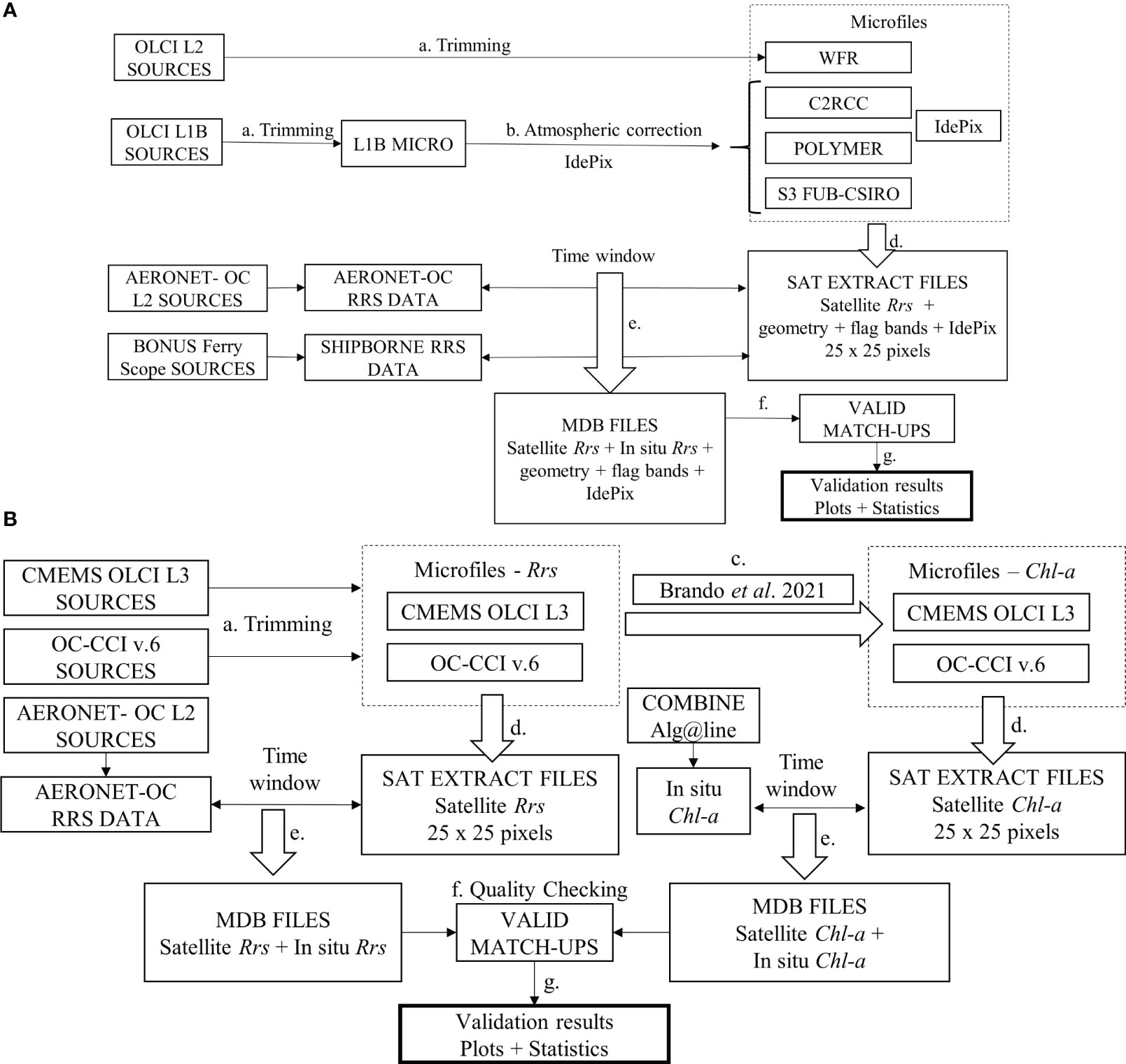
Figure 2 (A) Workflow for the comparative validation analysis of the four AC processors from OLCI level-1b to level-2 Rrs. (B) Workflow for the validation of CMEMS-OLCI and OC-CCI v.6 level-3 Rrs and chl-a datasets.
The top panel (Figure 2A) depicts the steps for a round-robin comparison of four AC algorithms (i.e., WFR, C2RCC, POLYMER, and S3 FUB-CSIRO) to select the best processor for deriving OLCI level-2 Rrs spectra from level-1 data. In-situ radiometry data for this comparative validation exercise were available from both the AERONET-OC and Alg@line hyperspectral datasets. The bottom panel of Figure 2B shows the validation procedure for the Rrs and chl-a level-3 datasets, comparing the new CMEMS-OLCI and OC-CCI v.6 datasets. In this case, only radiometric data from AERONET-OC were used for Rrs validation.
We also run the Identification of Pixels Properties (IdePix) to obtain a common flag band for all the processors. IdePix is a multisensor pixel identification tool available as a plugin for Sentinel Application Platform (SNAP) implementing pixel identification algorithms for different sensors including Sentinel-3 (https://www.brockmann-consult.de/portfolio/idepix/). It classifies pixels certainly or ambiguously affected by clouds and provides also other flags as white or bright (Table 2).
The main steps are the following ones (note that some steps are common for both analyses):
a) Trimming: With the aim of reducing the AC computational time, level-1b FR images were first trimmed into microgranules keeping the OLCI data format (SENTINEL-SAFE). In the case of AERONET-OC or chl-a measurements, microgranules cover an area of 2° by 2° around the site location. For shipborne radiometry, granules were trimmed to cover the daily transect. The same protocol was also adopted for trimming the level-2 WFR and level-3 files.
b) Atmospheric correction (Figure 2A): POLYMER, C2RCC, S3 FUB-CSIRO, and IdePix were run on the level-1b microgranules using default options. POLYMER is available from the HYGEOS website, and it is run directly in Python. C2RCC and IdePix are available as SNAP, while the S3 FUB-CSIRO processor is available as a Python/C plugin for SNAP at https://github.com/s3tbx-fub-csiro/s3tbx-fub-csiro.git. C2RCC, IdePix, and S3 FUB-CSIRO were processed using the SNAP Graph Processing Tool (GPT).
c) Chl-a processing (Figure 2B): Satellite chl-a was retrieved from level-3 microgranules using the method proposed by Brando et al. (2021) (Section 2.3).
d) Generation of satellite extract files: Level-2 extract files (Figure 2A) were created for the Sentinel-3A or Sentinel-3B mission and each AC processor (WFR, POLYMER, C2RCC, and S3 FUB-CSIRO) starting from the corresponding output microfiles. Each extract file contains 25 × 25 pixels of satellite data centered at the site location (for AERONET-OC) or transect point(s) (for shipborne radiometry). These files include always Rrs for all the available bands, geometry (zenith and azimuth observation and sun angles), the flag band corresponding to the processor (see Section 2.1.1), and the IdePix results. If output values are defined as water-leaving reflectance (WFR and POLYMER), they are converted to Rrs by dividing by pi. The satellite overpass time and band wavelengths are also included in the files.
In the case of shipborne radiometry, to reduce the number of extracts associated with a single satellite image, extract files were only created if the time difference between the satellite overpass and the transect points within a given central pixel was lower than 15 min.
Level-3 extract files (Figure 2B) produced from level-3 microfiles include a window of 25 × 25 pixels of Rrs for all the bands around the AERONET-OC site or chl-a concentrations around the measurement location. Unlike level-2 extract files, flag bands are not required since invalid pixels were already masked in the source datasets during the level-3 creation. Moreover, as data with different acquisition times and observation geometries are merged, satellite time is limited to the date, and geometry information is not available.
e) Generation of MDB files: An MDB file is created as a NetCDF file including all the potential match-ups, i.e., spatiotemporal collocations between satellite and in-situ data, in this case, Rrs spectra or chl-a concentrations. MDB files are built by associating the satellite data from each extract file with the corresponding in-situ spectra or chl-a measurements. For level-3 extracts merging sensors with different acquisition times, satellite time is set to 9:30 UCT, which is approximately the average overpass time in the Baltic Sea considering all the sensors (Brando et al., 2021). By default, the maximum time difference between satellite and in-situ acquisitions is set to 3 h for AERONET-OC and chl-a measurements and 15 min for shipborne radiometry (see Section 2.4.3). Normalized water-leaving radiances available in the AERONET-OC level-2 source files (see Section 2.2.1) were transformed to Rrs by dividing by the extra-solar irradiance spectrum and then band-shifted to the OLCI or OC-CCI v.6 spectral wavelengths using inverse and direct application of the QAA algorithm (Mélin and Sclep, 2015; Lee et al., 2009). Shipborne radiometry data (already available as Rrs) and chl-a concentrations (in mg m−3) were incorporated directly into the MDB files.
f) Quality checking: Starting from the MDB file with all the potential match-ups, we applied the quality control protocols to obtain valid match-ups for the statistical analysis (details are provided in Sections 2.4.2 and 2.4.3).
g) Statistical analysis: Plot generation and computation of validation metrics (see Section 2.4.4) using the valid match-ups.
2.4.2 Satellite quality control
Satellite quality control was based on the recommendations for OLCI or other medium-resolution satellites (Concha et al., 2021; Cazzaniga et al., 2022; Zibordi et al., 2022).
In this study, we used extraction windows of 3 × 3 pixels centered on the site only with 100% of valid pixels (9 pixels). Satellite Rrs for each band or chl-a concentrations were computed as the mean excluding outliers, considering a single pixel as an outlier if out of the range defined as the mean ± 1.5 standard deviations (EUMETSAT, 2022).
Pixels were masked according to two flag bands: IdePix and the specific flag band for each atmospheric correction algorithm. IdePix is used as a common framework for obtaining a set of common match-ups, whereas the specific flag band is useful for evaluating the spatial coverage of each AC processor in case of being applied operationally. Flag lists are summarized in Table 2.
Geometry was also considered for validation of level-2 Rrs, masking pixels with an observation zenith angle greater than 60° and a sun zenith angle greater than 70°.
We applied a spatial homogeneity test for Rrs validation by excluding match-ups with a coefficient variation (CV) at 560 nm higher than 20%. Note that CV is computed after the removal of out-of-range pixels (defined as the mean ± 1.5 standard deviations).
2.4.3 In situ quality control
As AERONET-OC systems acquire spectral measurements several times per day (usually every 20 min), MDB files can include up to 30 in-situ valid spectra for each satellite acquisition within the default 3-h time window. The validation was based on the closest spectrum in time with respect to the satellite overpass with a maximum time difference of 2 h.
For the Alg@line hyperspectral shipborne radiometry, the number of spectra in the central pixel within the 15-min time window varies from 1 to 3. Validation was also based on the closest spectrum in time.
In the case of chl-a, measurements collected between 7:00 UTC and 16 UTC on the same day as the satellite overpass were considered for obtaining valid match-ups.
2.4.4 Validation metrics
As validation metrics, we used the determination coefficient (R2), the absolute percent differences (APD), the root mean square deviation (RMSD), the relative percent differences (RPD), and the bias parameter between the in-situ (x) and satellite measurements (expected y):
The determination coefficient R2 (unitless) assesses the agreement between both variables ranging from 0 (no agreement) to 1 (perfect agreement). The bias (in Rrs units: sr−1, or chl-a units: mg m−3) is useful for determining if there is overestimation (positive values) or underestimation (negative values). RMSD and APD (%) measure the absolute error in absolute units or percentage, respectively. Likewise, RPD (%) is a measurement of the relative error, but measured in percentage. In the case of chl-a, R2, RMSD, and bias are computed on log-transformed data, whereas RPD and APD are based on non-transformed values. As error measurement, APD is preferred for the chl-a because RMSD is more difficult to interpret since it is based on log-transformed data, where RMSD is used for Rrs validation.
2.5 Comparison between CMEMS-OLCI level-3 and OC-CCI v.6 datasets
As within CMEMS two operational Rrs and chl-a datasets are available for the Baltic Sea, the consistency of the times series with spatial resolutions at 300 m and 1 km was assessed. To this aim, the CMEMS-OLCI level-3 and multisensor datasets were compared through the extraction of a set of co-collocated data points. Values were extracted from an image every 10 days from 1 May 2016 to 31 December 2022. These daily images were sampled based on a regular grid of 10 km × 10 km (115 × 119 longitude–latitude points), using the corresponding pixel value for the multisensor 1-km dataset and the mean on a 3 × 3 window for OLCI (only cases with 9 valid values were considered). The extraction was carried out for the five Rrs bands involved in the chl-a retrieval (i.e., 443 nm, 490 nm, 510 nm, 555 nm, and 670 nm) and for the chl-a concentration itself. Then, we obtained the scatter plots and computed the validation metrics (Section 2.4.4).
3 Results
3.1 Match-up summary and flagging analysis
Table 3 shows the number of total and valid match-ups available for the validation of level-2 (OLCI) and level-3 (CMEMS-OLCI and OC-CCI v.6) Rrs datasets, using both AERONET-OC and SYKE data.
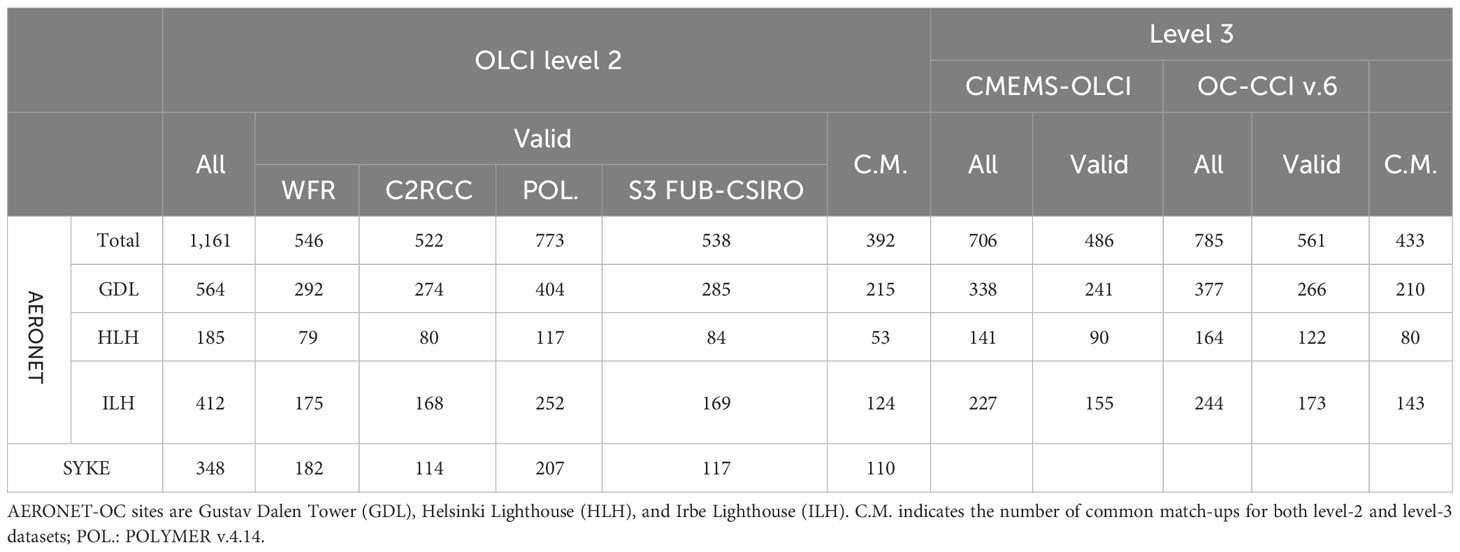
Table 3 Total and valid number of match-ups available for Rrs validation of OLCI level-2 and level-3 (CMEMS-OLCI and OC-CCI v.6) datasets.
When considering in-situ data from AERONET-OC sites, POLYMER is able to generate a higher number of valid match-ups for OLCI level-2 Rrs validation as compared with other AC processors (~65% of valid match-ups against ~45%), which show comparable figures. The percentage of valid match-ups is lower using SYKE data, but with a similar pattern: POLYMER shows the highest validity rate (~60%), followed by WFR (~52%) and finally C2RCC and S3 FUB-CSIRO (~35%).
Regarding the level-3 Rrs validation, both CMEMS-OLCI and OC-CCI v.6 show similar numbers with percentages of valid match-ups of approximately 70%, as expected considering that both datasets are mainly based on POLYMER.
As for the distribution across the AERONET-OC sites, the number of total (and valid) match-ups reveals the data availability, with more match-ups from Gustav Dalen Tower (data from 2016 to 2022), followed by Irbe Lighthouse (data from 2018 to 2022) and finally Helsinki Lighthouse (data from 2016, 2017, and 2019). Temporally, the number of match-ups has increased since 2018 (e.g., from 45 POLYMER valid match-ups in 2017 to 98 in 2018) with the launch of Sentinel-3B, being 2019 the year with the highest number as in-situ spectra were available from the three sites (e.g., 202 valid match-ups using POLYMER). The number of match-ups was also smaller in 2020 because the instruments were operational for a limited period (mainly between July and August) due to the COVID restrictions (56 valid POLYMER match-ups against 150 in 2021 or 171 in 2022).
Validation results shown in the following sections are based on common match-ups, i.e., those valid for all the AC processors in the case of level-2 Rrs validation and for both datasets (OLCI and OC-CCI v.6) in the case of level-3 Rrs validation.
Figure 3 shows a summary of the potential match-ups classified as invalid because at least one pixel in the 3 × 3 extraction window over the AERONET-OC sites was flagged using IdePix and/or other specific flag bands (Table 2).
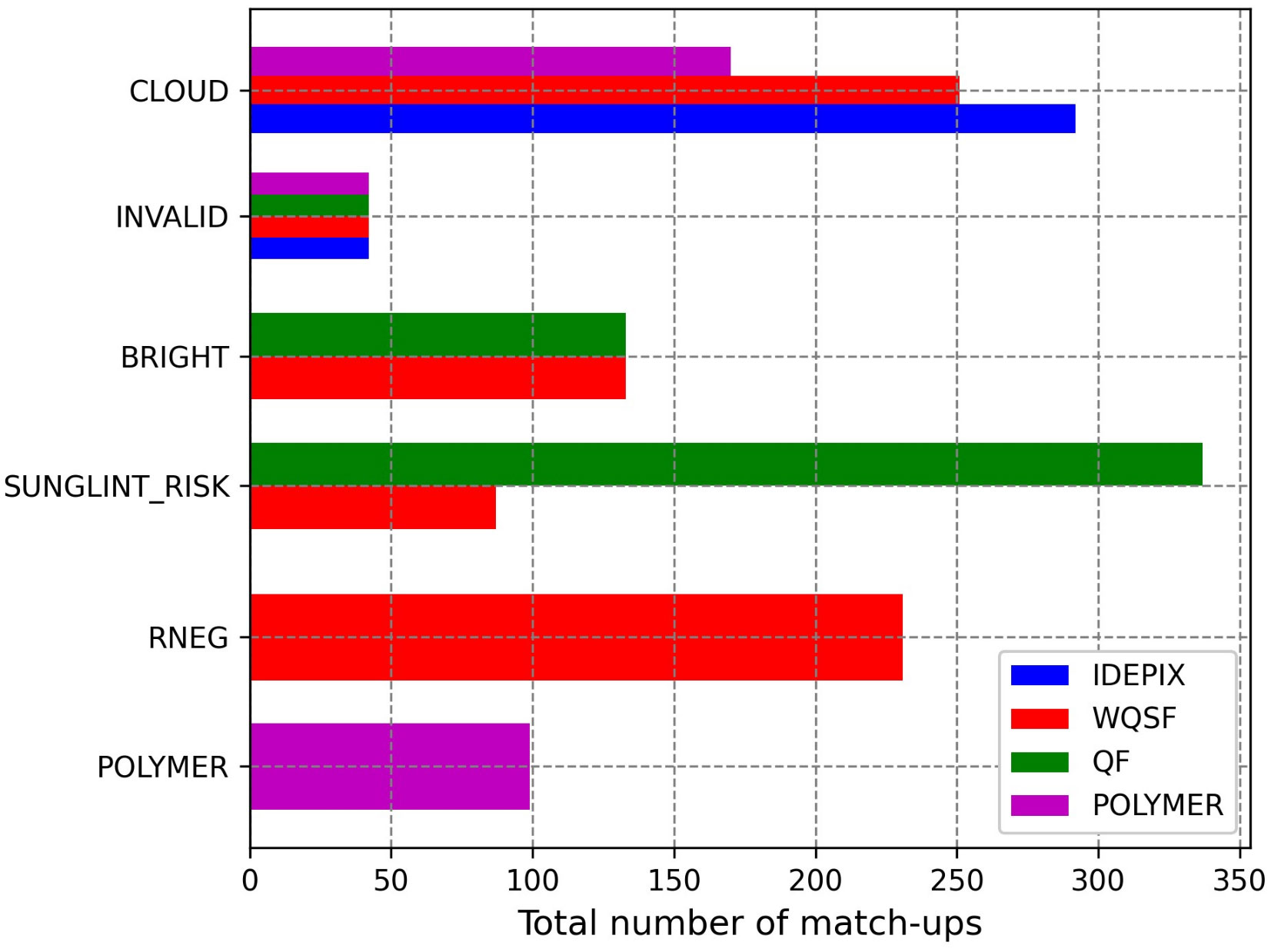
Figure 3 Total number of invalid match-ups with at least one pixel flagged in the 3 × 3 extraction window over the AERONET-OC sites. Legend colors indicate specific flag bands (IdePix: pixel_classif_flag; WQSF: wqsf; QF: quality_flags; POLYMER: bitmask). Specific flags defining each flag output are indicated according to the subscripts of Table 2 (1: CLOUD; 2: INVALID; 3: BRIGHT; 4: SUNGLINT_RISK; 5: RNEG; 6: POLYMER).
The number of match-ups affected by cloud cover varies from 150 to almost 300 match-ups, depending on the flag band specific to each processor (Table 2). As in-situ data and potential match-ups are mainly available from April to September, cloud coverage percentage is relatively low (between 10% and 30%) when compared with sites located in mid-latitudes.
Sun glint risk was the most frequent flag based on quality_flags (C2RCC and S3 FUB-CSIRO) with more than 330 match-ups, although this number is limited to only 87 match-ups using wqsf (WFR).
A common problem in the CDOM-dominated Baltic Sea waters affecting more than 230 potential match-ups is the negative reflectance retrievals (RNEGs), mainly in the blue part of the spectrum, i.e., rneg_02 (412 nm) and rneg_03 (443 nm). Note that rneg_ flags included in wqsf (WFR) allow negative values up to a threshold (EUMETSAT, 2021) so that some valid match-ups could include slightly negative reflectance values.
A remarkable number of match-ups were also flagged as invalid (42 match-ups in all the flag bands) and bright (133 match-ups using IdePix and quality_flags). Other flags (not shown in Figure 3) were identified in a small number of match-ups (less than 5), including the suspect, dubious, whitecaps, or ac_fail cases.
Approximately 100 match-ups were flagged by one of the flags in POLYMER bitmask (out_of_bounds, thick_aerosol, high_air_mass, external_mass, inconsistency, anomaly_rwmod_blue), joining to the match-ups classified as cloud (cloud_base) or invalid (l1_invalid). However, approximately 74 match-ups flagged as SUNGLINT_RISK or 146 as RNEG are considered valid using bitmask or IdePix, explaining the higher number of valid POLYMER match-ups as compared with other AC processors.
3.2 Level-2 Rrs validation
This section reports the validation results for OLCI level-2 Rrs processed using the four AC processors: WFR (standard AC), C2RCC, POLYMER, and S3 FUB-CSIRO (see Section 2.1.1). Satellite Rrs were validated against in-situ radiometric data from AERONET-OC (Section 3.2.1) and Alg@line shipborne radiometry (Section 3.2.2) based on common sets of 392 and 100 valid match-ups, respectively (Table 3).
3.2.1 AERONET-OC
Figure 4 shows the comparison between in-situ Rrs spectra from AERONET-OC sites and OLCI level-2 Rrs spectra processed using the four AC processors. Note that in-situ data for OLCI bands between 673.75 nm and 753.75 nm, as well as 885 nm, are not available using the multispectral AERONET-OC radiometers, as we limited the band shifting to a maximum of 5 nm (Table 2). Data for 1,020 nm were also excluded from the analysis because they are affected by high uncertainties in these highly absorbing waters and are not included in the OLCI level-3 dataset.
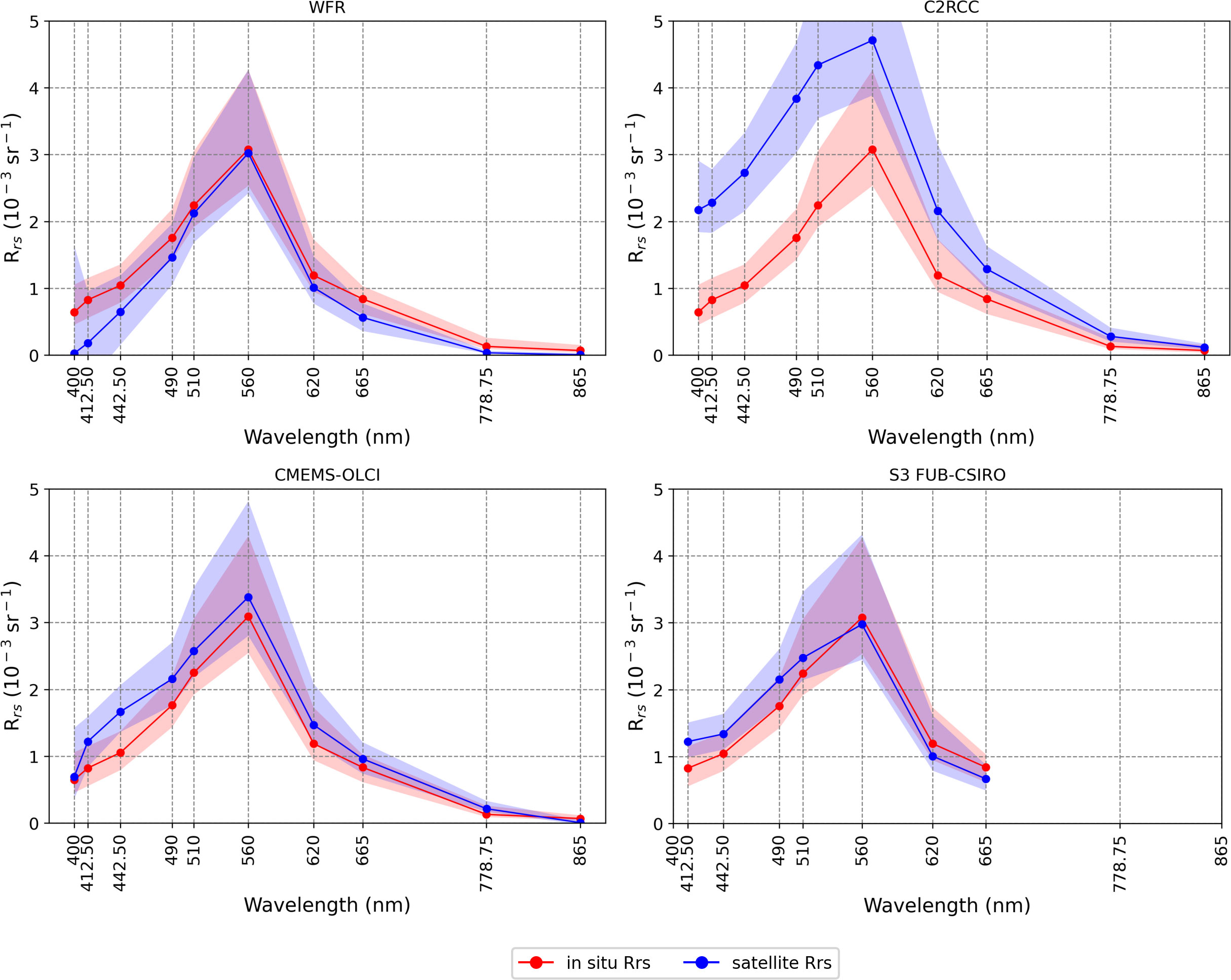
Figure 4 Comparison between in-situ Rrs from AERONET-OC sites and OLCI level-2 Rrs obtained using four AC processors: WFR, C2RCC, POLYMER, and S3 FUB-CSIRO. Distribution is based on the set of common match-ups (N = 392). Lines and shadowed areas represent the median values and the interquartile ranges, respectively.
In the case of WFR, both in-situ and satellite median spectra keep a similar shape across the whole range, with a remarkable overlapping of the interquartile areas between 520 nm and 620 nm. In other wavelengths, WFR tends to underestimate, showing high negative deviations and higher variability in the 400–490-nm spectral range. POLYMER also shows similar spectral shapes, although it tends to overestimate across the whole spectra (except at 865 nm), with higher positive deviations between 400 nm and 490 nm. Regarding C2RCC, although satellite and in-situ Rrs spectra keep similar shapes, there is a substantial overestimation, even without any overlapping between the interquartile areas at wavelengths lower than 510 nm. Finally, despite the acceptable overlapping, the main problem of S3 FUB-CSIRO is the spectral shape, as it tends to overestimate at wavelengths lower than 510 nm but shows negative deviations in the red part of the spectrum.
The match-up scatter plots between satellite and in-situ AERONET-OC Rrs for nine OLCI bands (between 400 nm and 778.75 nm) grouped by AC processor are shown in Figure 5. The results include all the available bands for validation using AERONET-OC data except for 865 nm and 1,020 nm (Table 2). The number of valid match-ups is lower for bands 400 nm, 510 nm, 560 nm, 620 nm, and 778.75 nm as in-situ data at these spectral bands were only available after 2018 (Table 2). Note also that the S3 FUB-CSIRO reflectance is not available at 400 nm and 778.75 nm.
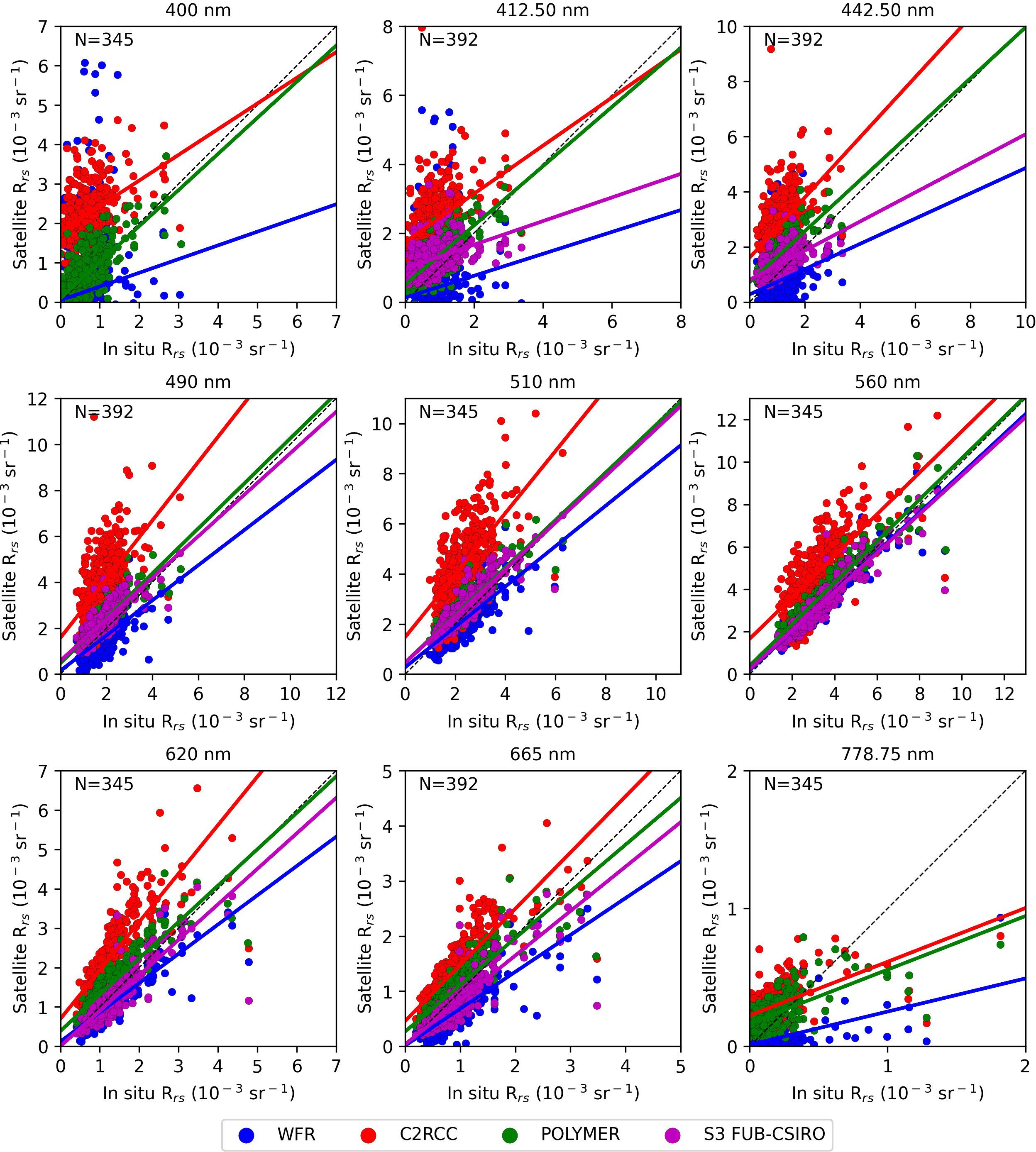
Figure 5 Scatter plots of common match-ups between satellite (OLCI level-2) and in-situ (AERONET-OC) Rrs measurements for nine OLCI bands between 400 and 778.75 nm. Data points and regression lines are color-coded by AC. The dash line represents the identity line (1:1 ratio).
In the blue bands (i.e., 400, 412.5, and 442.5 nm), POLYMER displays a better agreement with the in-situ data than other AC processors, which are affected by a marked deviation of their regression lines from the identity line (1:1). At 490 nm and 510 nm, POLYMER and S3 FUB-CSIRO perform better, whereas C2RCC and WFR tend to over- and underestimate, respectively. A good agreement is obtained at 560 nm using all the ACs except for C2RCC, which shows a positive bias. In the red part of the spectrum (i.e., 620 nm, 665 nm), the POLYMER regression line in agreement with the identity line is better than that of other ACs. Finally, at 778.75 nm, there are higher uncertainties and none of the AC processors seem to perform adequately. Nevertheless, POLYMER seems to give the best results when limiting the match-ups to low Rrs values (<0.5 10−3 sr−1).
Figure 6 shows the spectral variation of some validation metrics per wavelength and AC algorithm, including also results in the infrared (i.e., 865 nm and 1,020 nm). Metric values confirm the tendencies observed in Figures 4, 5 in the visible spectral range. The WFR performance in the blue spectral region is inadequate with negative bias and RPD values and higher RMSD and lower correlation coefficients compared with other processors. However, statistical figures improve between 510 nm and 778.75 nm, being the best-performing algorithm at 560 nm. Broadly speaking, C2RCC performs worse than other AC algorithms clearly overestimating the in-situ Rrs (Figures 4, 5). It shows higher RMSD values, lower determination coefficients, and very high positive RPD and bias values (up to 0.002 sr−1), especially between 400 nm and 620 nm. On the other hand, POLYMER is the best-performing AC processor: it provides the best fitting (higher R2) and lower error (RMSD) values (except for 442.5 nm). As observed in Figure 4, its main issue is the overestimation, showing positive RPD and bias values (lower than 0.0005 sr−1 except for 442.5 nm) across the visible spectral range. Finally, S3 FUB-CSIRO performs well in terms of error (RMSD) and fitting, with determination coefficients only lower than those obtained using POLYMER. Its main drawback is the spectral shape (Figure 4), with bias and RPD varying from positive to negative values with increasing wavelengths. Overall, metrics in the infrared spectral region are worse in comparison with those obtained for the visible spectral range. At 865 nm, WFR provides better results, whereas C2RCC performs better at 1,020.5 nm.
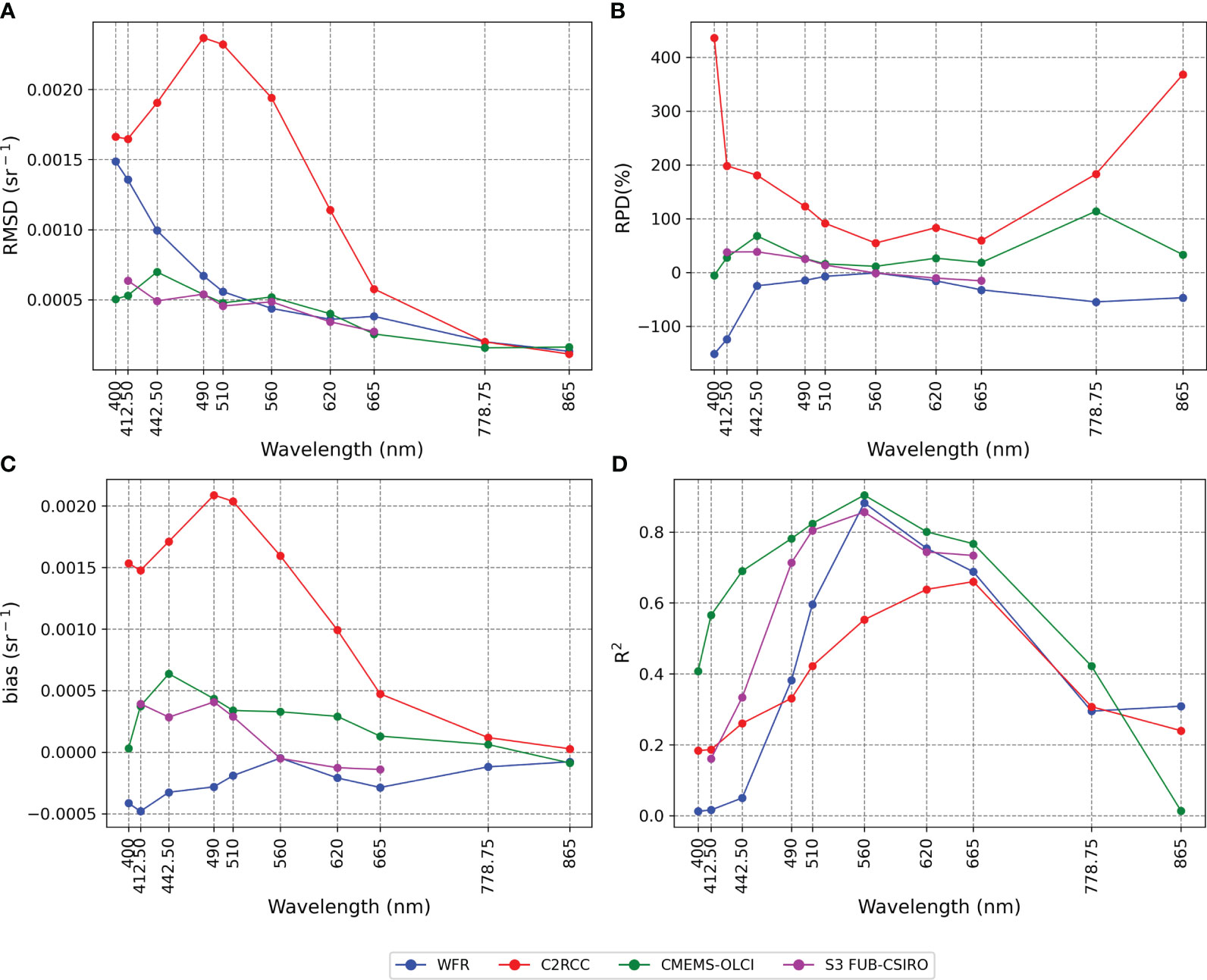
Figure 6 Spectral variation of the validation metrics computed for each AC from match-ups of OLCI level-2 and AERONET-OC in-situ Rrs data. (A) RMSD (in Rrs units: sr−1). (B) Relative percent differences (%). (C) Bias (in Rrs units: sr−1). (D) Determination coefficient (R2, unitless).
3.2.2 Shipborne radiometry
A comparison between in-situ Rrs spectra from Alg@line shipborne radiometry and OLCI level-2 Rrs spectra processed using the four AC processors is shown in Figure 7. In this case, as the shipborne radiometric data are hyperspectral measurements, in-situ data are available for all the OLCI bands. Although patterns are similar to those observed in Figure 4, some differences exist.
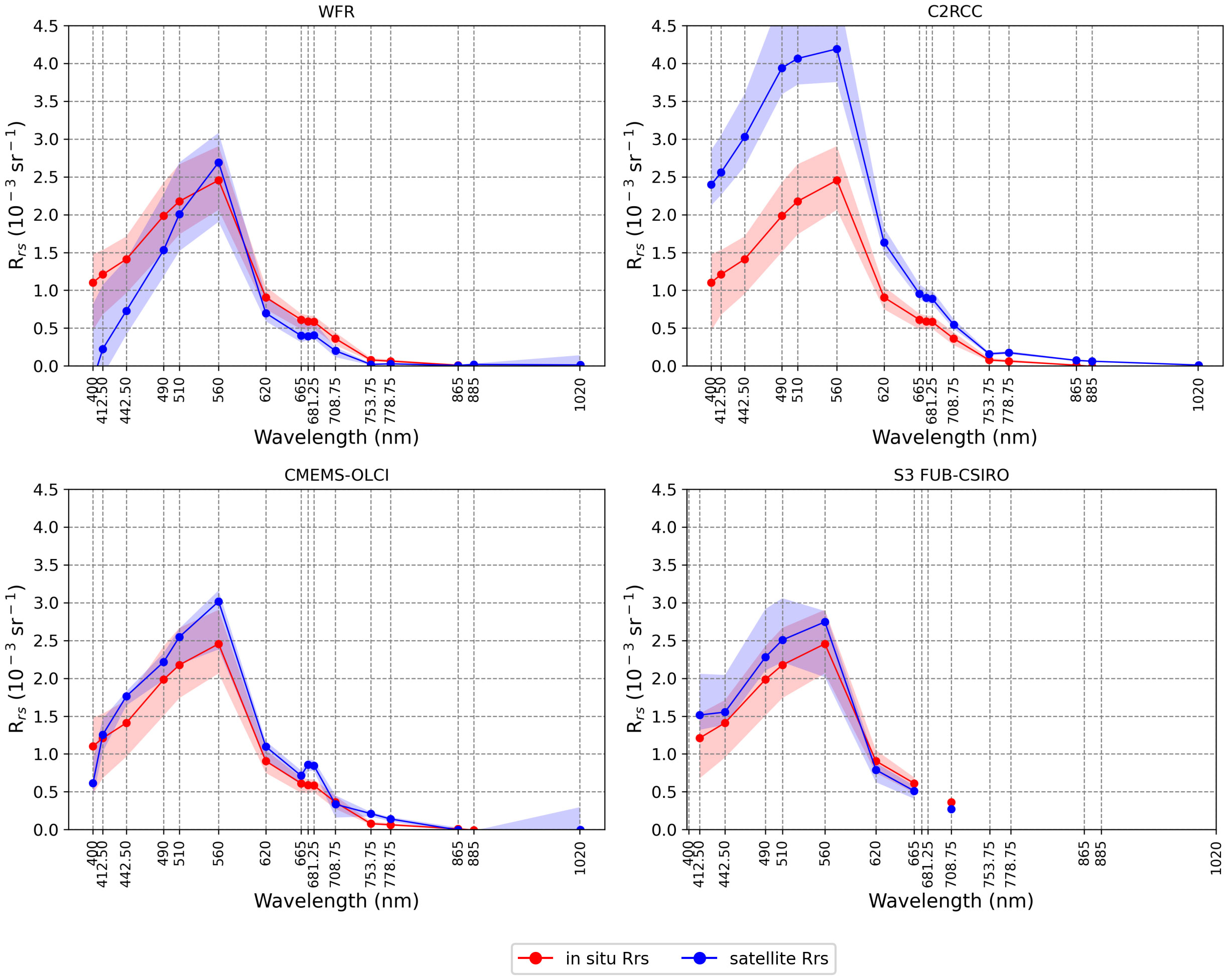
Figure 7 Comparison between in-situ Rrs from automated shipborne radiometry and OLCI level-2 Rrs obtained using four AC processors: WFR, C2RCC, POLYMER, and S3 FUB-CSIRO. Lines and shadowed areas represent the median values and the interquartile ranges, respectively.
In detail, WFR can express well the median spectral shape, but with a higher negative bias across the bands and limited overlapping of the interquartile areas between 400 nm and 442 nm. In the case of C2RCC, it also shows extreme positive deviations for all the wavelengths, with hardly any overlapping between both distributions. S3 FUB-CSIRO follows the same pattern with a transition from positive to negative bias toward the red, but with higher uncertainties and less overlapping in the blue part of the spectrum.
Regarding POLYMER, the divergence from the reference data is more pronounced than in Figure 4: it shows negative deviations at 400 nm and 412 nm and an anomalous spectral shape between 673.75 nm and 708 nm in the three bands that are acquired at AERONET-OC sites (and hence not shown in Figure 4). For the remaining bands, they display a spectral shape agreement with a lower positive bias.
Figure 8 shows the match-up scatter plots between satellite and in-situ shipborne Rrs grouped by AC processor for the same nine OLCI bands between 400 nm and 778.75 nm shown in Figure 5. Overall, the results are worse than those obtained with the AERONET-OC sites, with the regression lines clearly deviating from the identity line (1:1). This behavior could be due to the presence of some in-situ spectra with very high Rrs values, possibly unflagged glint.
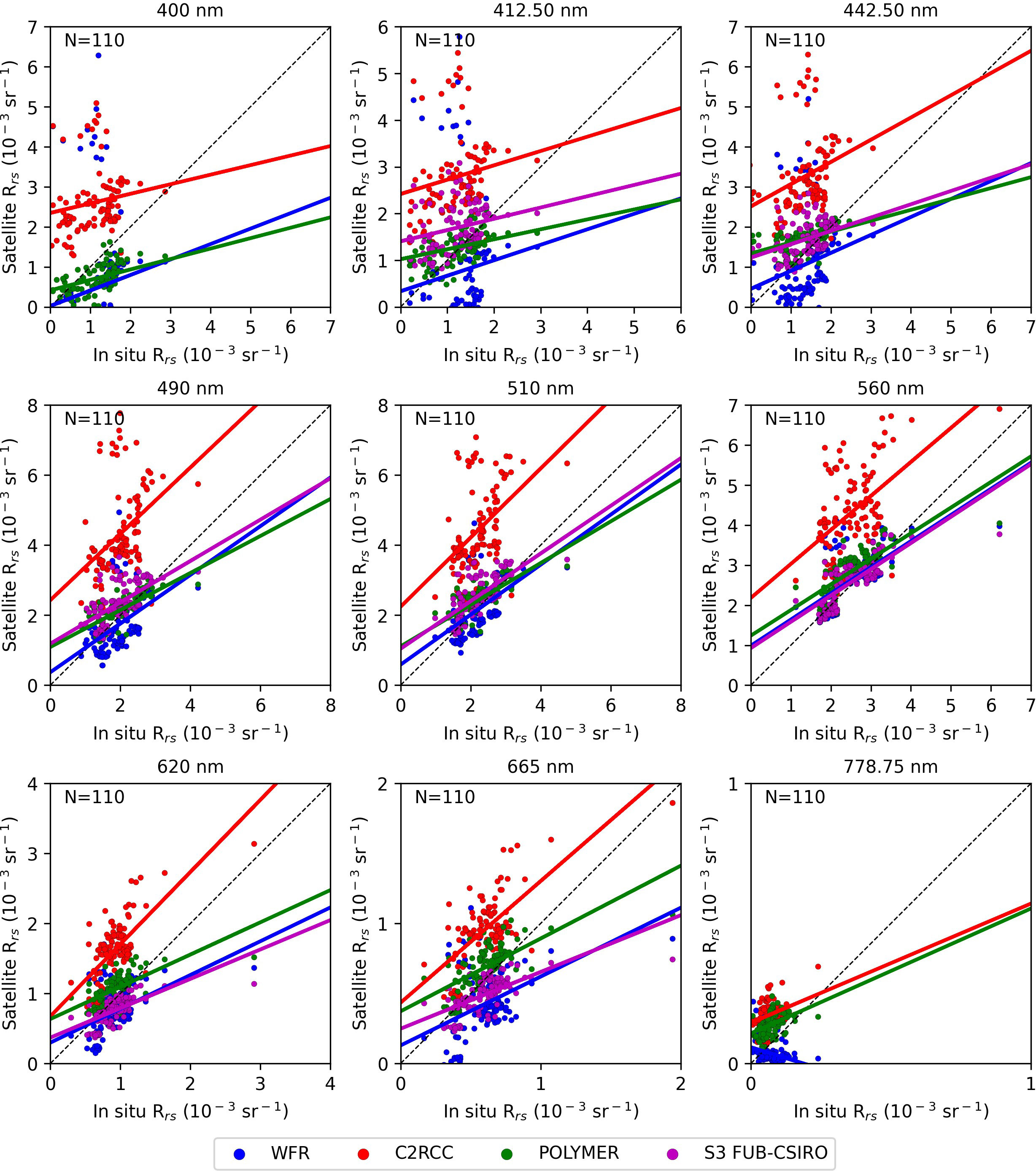
Figure 8 Scatter plots of common match-ups between satellite (OLCI level-2) and in-situ (shipborne radiometry) Rrs measurements, for nine OLCI bands between 400 nm and 778.75 nm. Data points and regression lines are color-coded by AC. The dash line represents the identity line (1:1 ratio).
If we consider the distribution of the data points, POLYMER seems to perform better with a higher density over the identity line for all the wavelengths, although S3 FUB-CSIRO also shows good results, especially in the central wavelengths. Like AERONET-OC validation results, and as observed in Figure 7, WFR presents higher variability in the blue (wavelengths lower than 490 nm) and a better agreement in the green and red parts of the spectrum, whereas C2RCC tends to overestimate with high positive deviations across the spectra.
The spectral metrics for each processor (Figure 9) confirm the patterns observed in Figures 7, 8, as well as the validation results based on AERONET-OC in-situ Rrs. POLYMER is again the best-performing processor in the visible spectral range in terms of error (RMSD, RPD) and fitness (R2), showing a positive bias lower than 0.0005 sr−1 across the spectra (except for 400 nm). As observed in Figure 7, performance is worse between 673.75 nm and 708.75 nm for the bands not validated using AERONET-OC, with lower R2 and higher bias values. WFR does not perform well in the blue part of the spectrum, showing higher RMSD values and high negative bias compared with POLYMER or S3 FUB-CSIRO. The S3 FUB-CSIRO shows similar results to POLYMER between 560 nm and 708.75 nm, even with a better agreement (higher R2 value) at 708.75 nm and a lower RMSD at 560 nm. However, the results are worse in terms of correlation and RMSD with wavelengths lower than 510 nm, and it cannot match the spectral shape as indicated by the decreasing bias (changing from positive to negative values) with increasing wavelengths. C2RCC, as seen in Figures 7, 8, shows a high positive bias across the whole spectra and high RMSD values with wavelengths lower than 620 nm. Overall, it performs worse than other ACs at all the wavelengths and metrics except for its higher determination coefficients between 620 nm and 708 nm. Performance metrics in the infrared (865 nm, 885 nm, and 1,020 nm) are worse than those obtained for the visible range in terms of correlation and RPD, although bias and RMSD show comparable values due to their lower Rrs range. WFR appears as the best-performing AC in this spectral range, with higher R2 and lower RPD values.
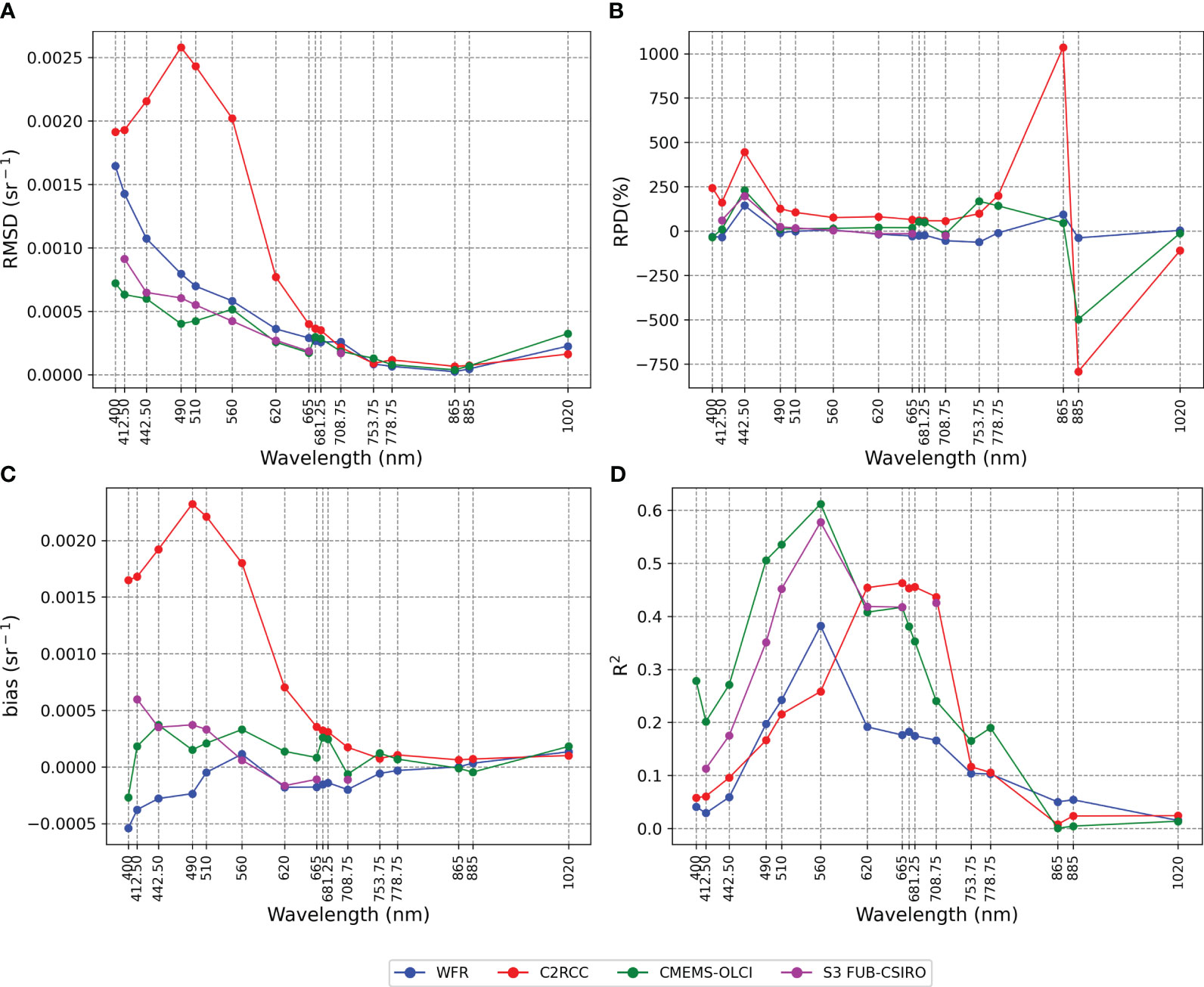
Figure 9 Spectral variation of the validation metrics computed for each AC from match-ups of OLCI level-2 and SYKE in-situ Rrs data. (A) RMSD (in Rrs units: sr−1). (B) Relative percent differences (%). (C) Bias (in Rrs units: sr−1). (D) Determination coefficient (R2, unitless).
3.3 Level-3 Rrs validation
The OLCI level-3 Rrs dataset was processed by merging OLCI level-2 Rrs obtained using POLYMER v.4.14, as it was the best-performing AC algorithm in the Baltic Sea according to the results shown in Section 3.2. In this section, we summarize the validation results of this dataset in comparison with the multisensor level-3 Rrs dataset derived from OC-CCI v.6 (see Section 2.1.2) using in-situ radiometric measurements from AERONET-OC as reference. In addition to a common set of 433 match-ups acquired between 2016 and 2022, the results were obtained for the complete OC-CCI v.6 time series (2005–2022). Figure 10 shows the comparison between satellite and in-situ spectra using all the datasets and including the six bands available in OC-CCI v.6.
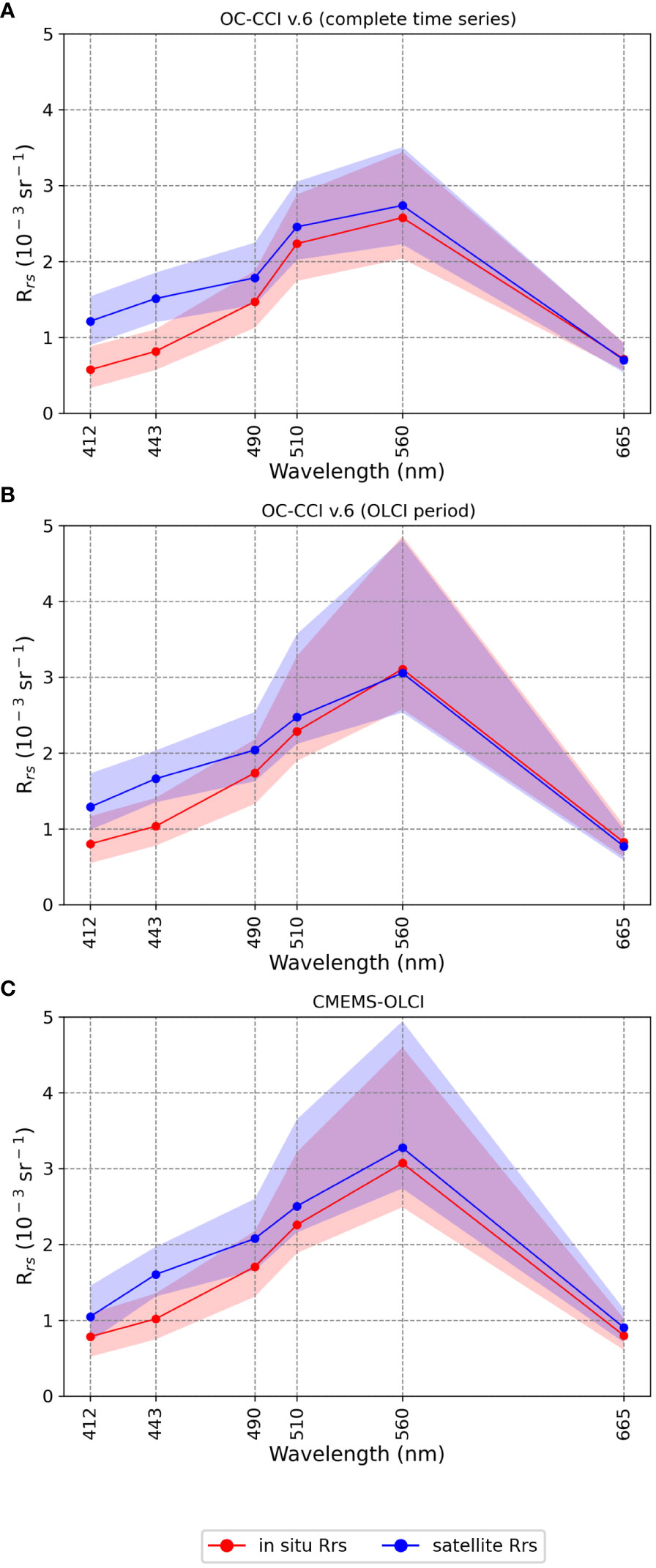
Figure 10 Comparison between in-situ Rrs from AERONET-OC sites and level-3 Rrs datasets. (A) OC-CCI v.6 (complete time series: 2005–2022). (B) OC-CCI v.6 (OLCI period: 2016–2022). (C) CMEMS-OLCI. Lines and shadowed areas represent the median values and the interquartile ranges, respectively.
Using the complete time series (Figure 10A), OC-CC1 v.6 captures the spectral shape across the complete spectral range with positive deviations for all the bands except for 665 nm. Similar results are observed with data from the OLCI period (Figure 10B), although the bias is close to zero or slightly negative at 560 nm with a larger overlap of the interquartile regions. CMEMS-OLCI level 3 also matches the spectral shape with positive deviations at all the bands (including 665 nm) and a relatively higher bias value at 443 nm.
Scatter plots between satellite and AERONET-OC for the same six bands comparing CMEMS-OLCI and OC-CCI v.6 (complete time series and OLCI period) are shown in Figure 11. Overall, all the datasets perform adequately in terms of fitting, especially between 490 nm and 560 nm.
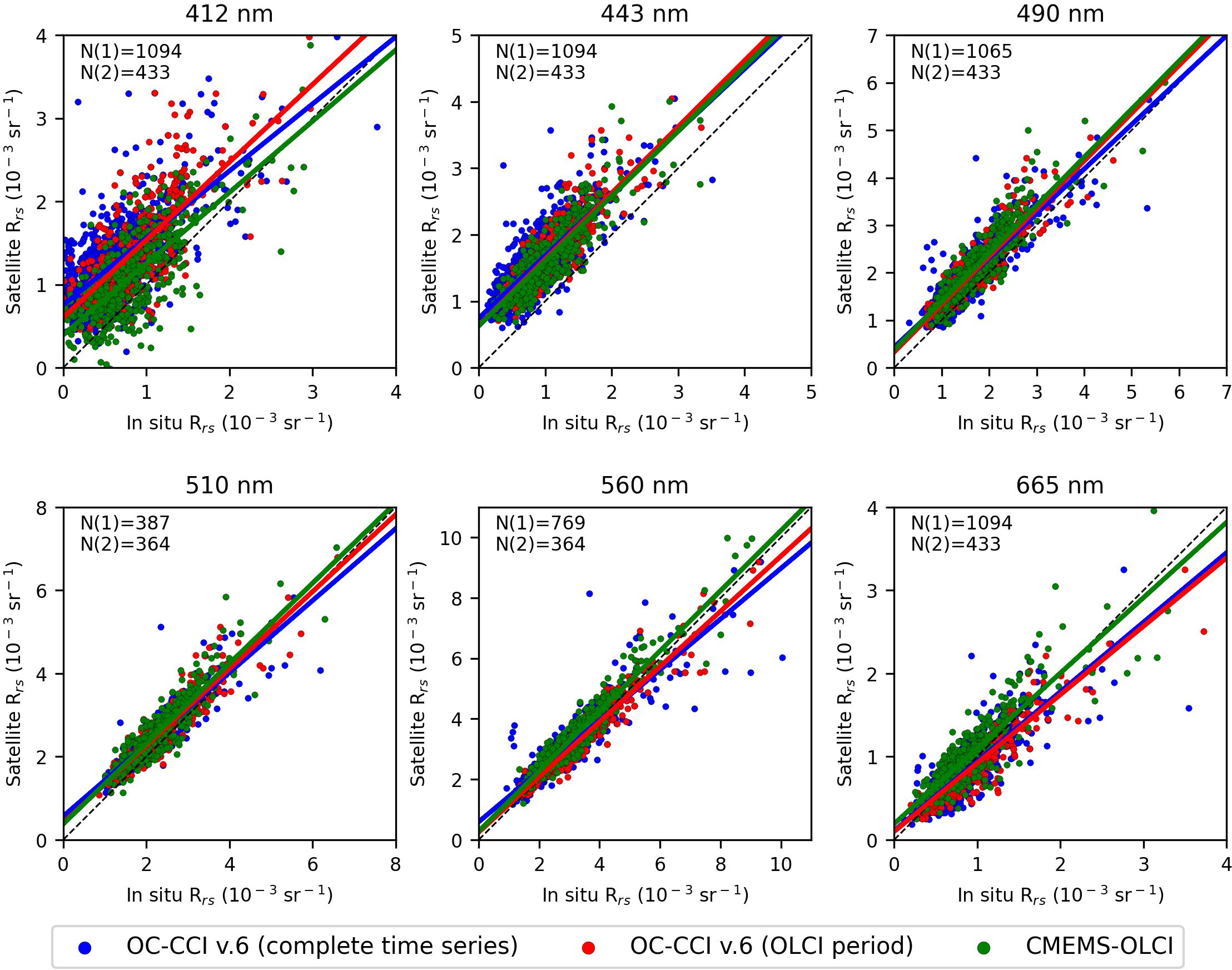
Figure 11 Scatter plots of match-ups between satellite level 3 and in-situ AERONET-OC Rrs measurements for six bands between 412 n and 665 nm. Data points and regression lines are color-coded by level-3 dataset (OC-CCI v.6—complete time series; OC-CCI v.6—OLCI period; CMEMS-OLCI). The dashed line represents the identity line (1:1 ratio). N(1) and N(2) are the number of match-ups available for the OC-CCI v.6 complete time series and for the OLCI period, respectively.
As observed in Figure 10, all the datasets show positive deviations with respect to the identity line between the 412-nm and 490-nm spectral range, with an offset more remarkable at 443 nm. Although comparable regression fits were achieved from all the datasets, CMEMS-OLCI seems to perform better at 412 nm and 665 nm.
Figure 12 shows the spectral variation of some metrics computed from the match-ups between satellite level 3 and AERONET-OC Rrs.
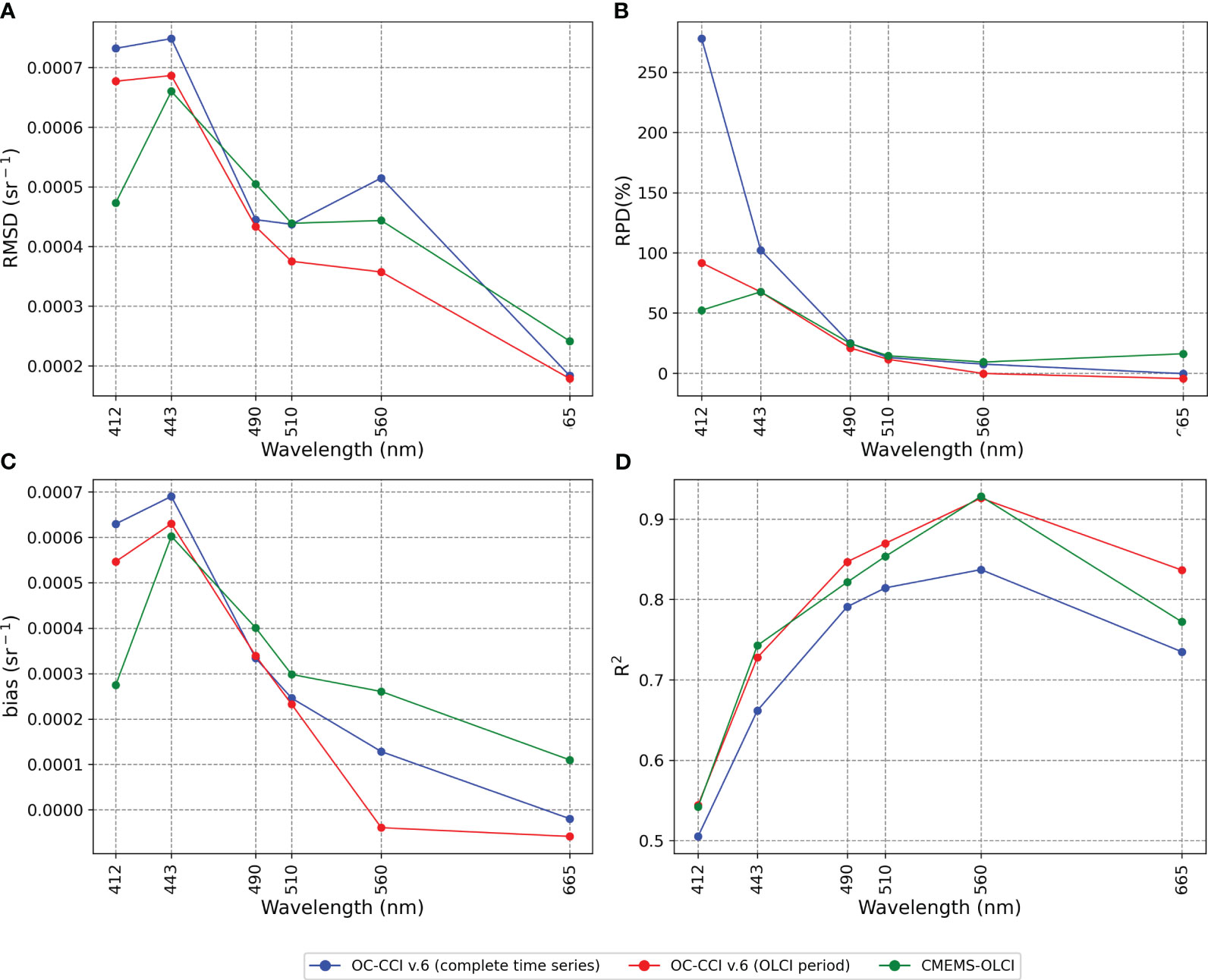
Figure 12 Spectral variation of the validation metrics computed from match-ups of level-3 datasets (OC-CCI v.6 complete time series; OC-CCI v.6—OLCI period; CMEMS-OLCI) and AERONET in-situ Rrs data. (A) RMSD (in Rrs units: sr−1). (B) Relative percent differences (%). (C) Bias (in Rrs units: sr−1). (D) Determination coefficient (R2, unitless).
Overall, all the metrics using the complete time series for OC-CCI v.6 are worse than those obtained only for the OLCI period, and this may be due to the differences in satellite missions being included in the OC-CCI v.6 time series and to differences related to the data availability from the three AERONET-OC sites.
Comparing CMEMS-OLCI and OC-CCI v.6 based on the metric computed with the common set of match-ups between 2016 and 2022, CMEMS-OLCI performs better at 412 nm and OC-CCI v.6 in the 490–665-nm spectral range in terms of RMSD, RPD, and bias, whereas the results are equivalent at 443 nm. In terms of correlation, similar R2 values were obtained for all the wavelengths except at 665 nm, with a higher R2 (0.84 vs. 0.77) for OC-CCI v.6. In summary, both level-3 datasets perform adequately in the 490–665-nm spectral range (R2: 0.75–0.95; RMSD< 0.005) and acceptable results at 443 nm (R2 ~ 0.75; RMSD< 0.007), whereas higher uncertainties are observed at 412 nm (Figure 12).
3.4 Chl-a validation
Chl-a concentrations were retrieved using the chl-aENS3 ensemble approach based on Rrs spectra from CMEMS-OLCI and OC-CCI v.6 and validated against co-collocated in-situ chl-a measurements from COMBINE and Alg@line datasets. Scatter plots and main metrics are shown in Figure 13, including the results from a common set of match-ups (2016–2019) for both satellite datasets and from all the valid match-ups (1997–2019) using the complete OC-CCI v.6 time series.
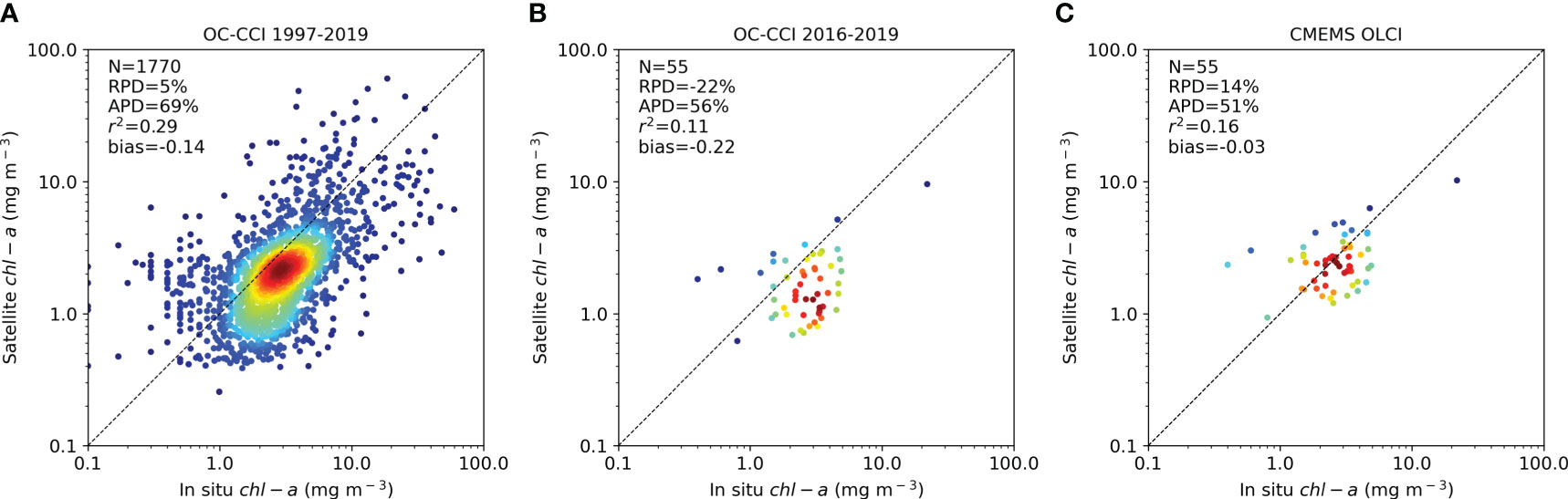
Figure 13 Scatter plots of match-ups between satellite-derived and in-situ log-transformed chl-a measurements. Satellite chl-a concentrations were retrieved using chl-aENS3. (A) all the valid match-ups (1997–2019) from OC-CCI v.6; (B) common match-ups (2016–2019) for OC-CCI v.6; and (C) CMEMS-OLCI. Data points are colored by density. The continuous line represents the 1:1 ratio.
Our dataset includes a total of 9,035 chl-a in-situ measurements from 1997 to 2019 (3,955 from Alg@line, 5,080 from COMBINE). For the OLCI period, only 1,047 measurements (325 from Alg@line, 722 from COMBINE) were available between 2016 and 2019. After applying the quality control (see Section 2.4), the number of valid match-ups for the OC-CCI v.6 (1997–2019) was 1,770, whereas for the OLCI period, validation for both CMEMS-OLCI and OC-CCI v.6 was based on a common set of 55 match-ups.
The results were quite comparable using the common set of match-ups, although, looking in detail, CMEMS-OLCI performs better for all performance metrics. As compared with CMEMS-OLCI, the results from the longer OC-CCI v.6 time series are characterized by a better fitness (R2 = 0.29) and a lower RPD (5%), but also a higher APD (69%) and a tendency toward underestimation (bias = −0.14). These negative deviations also appear in the results from the shorter OC-CCI v.6 time series (bias = −0.22) but are not observed in the CMEMS-OLCI dataset (bias = −0.03). Although metrics differences between both datasets could be explained by uncertainties associated with the input Rrs spectra, OLCI results should be interpreted with caution considering the lower number of match-ups (55 vs. 1,770) available for the OLCI period (from 2016 to 2019).
3.5 Comparison between CMEMS-OLCI and OC-CCI v.6 datasets
Figure 14 shows the scatter plots between OC-CCI v.6 and CMEMS-OLCI based on a set of co-collocated data points for the five Rrs bands involved in the chl-a retrieval as well as for the satellite-derived chl-a concentration (see Section 2.5).
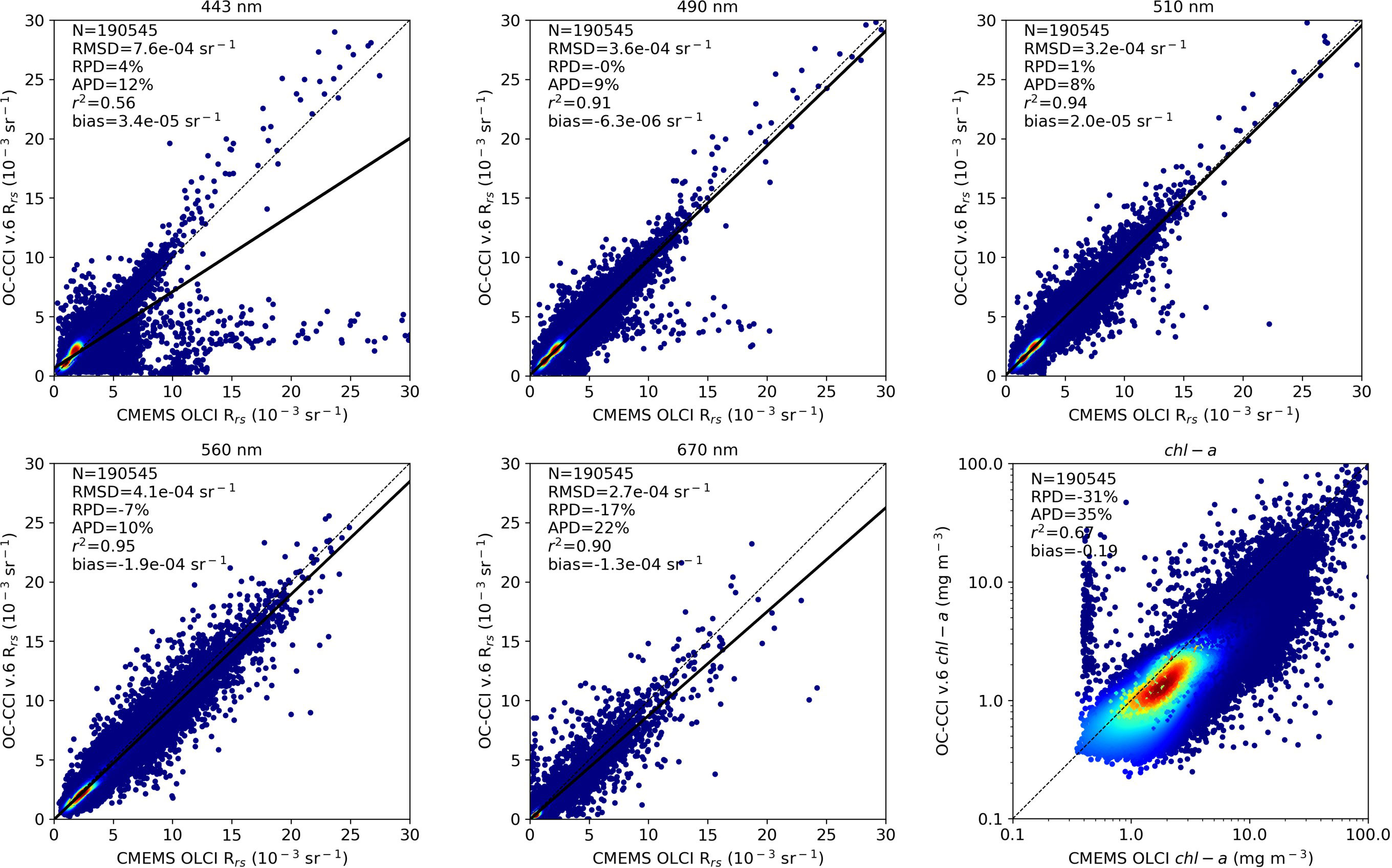
Figure 14 Scatter plots of co-collocated data points between OC-CCI v.6 and CMEMS-OLCI data points, including Rrs for five bands (443 nm, 490 nm, 510 nm, 560 nm, 670 nm) and chl-a concentration.
A good Rrs fitting with determination coefficients higher than 0.90 was observed in the 490–670-nm spectral range, whereas a poorer agreement and a higher dispersion, especially with low Rrs values (<5 10−3 sr−1), were found at 443 nm. Most of the data points fall around the identity line leading to a bias value close to zero (lower than 1.9 10−4 sr−1). In terms of percent error (APD and RPD), values were lower than 12% for all the bands except for 670 nm, whereas comparable RMSD values were also found for all the bands (between 2.7 10−4 and 7.6 10−4). Note that RMSD depends, to some extent, on the distribution range, so that the maximum was obtained at 443 nm (i.e., with the maximum range) and the minimum at 670 nm (minimum range) despite this band showing the maximum APD.
The scatter plots also show the data points deviating from the expected 1:1 ratio. The greatest deviation of the regression line with respect to the identity line is observed at 443 nm, caused by a significant number of points with low OC-CCI v.6 values (lower than 5 10−3 sr−1) but high CMEMS-OLCI Rrs (higher than 10 10−3 sr−1). Moreover, some erroneous data points with low CMEMS-OLCI Rrs (lower than 0.5 10−3 sr−1) but OC-CCI v.6 Rrs values approximately 5 10−3 sr−1 are also clearly visible in the scatter plot at 670 nm. These deviations are caused by problems with CMEMS-OLCI.
The chl-a concentration yielded an acceptable fitting (R2 = 0.67) but with a negative bias, meaning that chl-a values retrieved from OC-CCI v.6 tend to be lower than those from CMEMS-OLCI. In fact, most of the points are located below the identity line. Similar to Rrs at 443 nm and 670 nm, there is also a significant number of data points deviated from the expected 1:1 ratio, with concentrations ranging from 1.5 mg m−3 to 15 mg m−3 from OC-CCI v.6 but values lower than 0.5 mg m−3 when retrieved from CMEMS-OLCI.
4 Discussion
This study presented the introduction within the Copernicus Marine Service of the operational Rrs and chl-a datasets for the Baltic Sea from OLCI full resolution (300 m). Poor performances have been reported in the assessment of OLCI Rrs for the Baltic CDOM-dominated waters using both the EUMETSAT Operational Baseline (Zibordi et al., 2018; Zibordi et al., 2022) and the alternative atmospheric correction processing chain based on CR2CC (Cazzaniga et al., 2022). Hence, the first step was to select the best AC method to retrieve OLCI Rrs by comparing the accuracy of four processors using in-situ radiometric data from AERONET-OC sites and Alg@line shipborne hyperspectral radiometry as reference.
Our validation results from both in-situ sources (fixed platform and shipborne observations) show that POLYMER v.4.14 was the best option for the implementation in the processing chain for the new level-3 OLCI Rrs and chl-a datasets (see Section 3.2). In fact, it performs better not only in the 443–665-nm spectral range which includes the relevant bands for chl-a retrieval (Table 1), but also at 400 nm, 412.5 nm, and 778.75 nm. A greater variability in the metrics in the 673.75–708.75-nm spectral range (validated only with shipborne radiometry) and at 865 nm hinders the identification of the best-performing AC in this spectral range. Overall, performance differences are more remarkable in the blue spectral region (400–490 nm), especially in terms of correlation (Figures 6, 9). The main drawback for chl-a retrieval is the positive bias observed from both sources across the 412.5–665-nm spectral range.
Although validation results from AERONET-OC sites are expected to be more robust and reliable as in-situ data come from a stable platform with fewer uncertainties, metrics based on shipborne radiometry collected in 2016 were consistent and show the potential of this method to increase the number of match-ups providing data at other sites with different atmospheric and/or water conditions. According to Tilstone et al. (2022), differences between both in-situ sources could be mainly explained by two factors: 1) site differences—Baltic Sea waters are mainly dominated by CDOM, but ship trajectories could be more influenced by increases in chl-a concentrations due to phytoplankton blooms, whereas CDOM concentrations are generally higher in AERONET-OC sites; and 2) instruments and data processing—the differences in instruments (TriOS-RAMSES in the case of the hyperspectral shipborne radiometry; CIMEL-SeaPRISM for AERONET-OC) with their specific uncertainties by wavelength may be augmented by the fact that the data are processed with distinct methodologies. Moreover, in our study, shipborne radiometry is only available for validating Sentinel-3A in 2016, as AERONET-OC in-situ data extend from 2016 to 2022 enabling the validation of both Sentinel-3A and Sentinel-3B.
In our study, POLYMER results from AERONET-OC as compared with Alg@line in the 442.5–665-nm spectral range were better in terms of correlation (0.41–0.90 vs. 0.19–0.61), but slightly worse considering RMSD (2.6–7.0 10−4 sr−1 vs. 1.7–6.0 10−4 sr−1) or bias (1.3–6.4 10−4 sr−1 vs. 0.8–3.7 10−4 sr−1). As RPD are similar from the two sources (between 10% and 28% except for 442.5 nm), higher RMSD or bias values using AERONET-OC could also be related to its larger in-situ Rrs range and maximum values (see in-situ distribution in Figures 4, 7). Moreover, overall better results according to all the metrics were derived from AERONET-OC at 400 nm, 412.5 nm, and 778.75 nm (except for RMSD at 412.5 nm). At 400 nm, a negative bias was obtained from Alg@line (−2.7 10−4 sr−1) but a positive value (3.2 10−5 sr−1) from AERONET-OC, which could be related to differences in optical water characteristics: higher CDOM concentrations leading to lower Rrs (and higher bias) in AERONET-OC sites. In fact, only 21% of match-ups from AERONET-OC show Rrs greater than 1 10−3 sr−1 against 55% from the shipborne radiometry.
Another remarkable feature is the high RPD peak at 442 nm from shipborne radiometry, which is present in all the processors. This peak is explained by a small set of match-ups with very high RPD values (>200% with a maximum of approximately 23,000%) from 2 days (9 June 2016 and 28 July 2016). These high RPD values are caused by some outliers in the in-situ distribution at 442 nm characterized by very low Rrs values (0.06–0.86 10−3), as compared with the interquartile range between 1.25 10−3 and 1.75 10−3 sr−1 (Figure 7). These results evidence that results from shipborne radiometry could be improved by a stricter in-situ quality control (e.g., removing spectra with outliers). However, this refinement is out of the scope of this work as we use the dataset as a method to confirm the conclusions based on AERONET-OC data.
POLYMER also shows advantages in terms of coverage in comparison with other AC methods as by design it is able to deal with residual sun glint (Steinmetz and Ramon, 2018). As seen in Table 3, the number of valid match-ups based only on POLYMER flag mask bitmask (846 valid match-ups from AERONET-OC) or combining bitmask and IdePix (773 valid match-ups from AERONET-OC) is 30% higher than those using other processors. However, yielding more match-ups does not imply a better or worse performance of the validation results. In fact, datasets showed similar distributions, with close ranges and median values across the spectra. Table 4, based on AERONET-OC in-situ data, presents comparable values for R2, RMSD, or bias in the 412.5–665-nm spectral range, whereas expected higher uncertainties were observed at 400 nm or 778.75 nm. Note that bias was consistently lower across the whole spectra using only bitmask, especially in the blue, meaning that extra match-ups produced by POLYMER tend to show a lower bias.
Table 4 also shows the differences between the POLYMER validation metrics from Sentinel-3A and Sentinel-3B using in-situ data from AERONET-OC. Overall, results from Sentinel-3A are better considering all the metrics across the spectra, except for the bias between 620 nm and 778.75 nm. Differences are expected since match-up datasets do not show the same spatial–temporal coverage leading to different numbers of valid match-ups as Sentinel-3B is only available from 2018.
Our level-2 validation results agree with the findings in other works comparing AC algorithms over the Baltic Sea. Tilstone et al. (2022) assessed Sentinel-3A Rrs from WFR (pb 2.23–2.29 and OL_L2M.003), POLYMER v.4.14, and C2RCC using in-situ data from Alg@line shipborne radiometry (199 match-ups), Gustav Dalen Tower (5 match-ups), and Helsinki Lighthouse (4 match-ups), all the match-ups for only 2016. They found that POLYMER was the best-performing AC algorithm for six bands (412 nm, 443 nm, 490 nm, 560 nm, 665 nm, and 709 nm). Alikas et al. (2020) validated satellite OLCI Rrs from four AC processors (i.e., ALTNN, C2RCC, POLYMER, and WFR) against above-water field measurements collected from a research vessel over the coast of the Baltic Sea and Estonian Lakes in 2016. With a number of valid match-ups between 15 and 49 depending on the AC processor and filtering level, they reported POLYMER as the best suitable algorithm for all the OLCI bands except for 865 nm.
Since most of the valid match-ups (199 of 208) in Tilstone et al. (2022) are derived from the same dataset based on shipborne radiometry, metric values are expected to be similar to those reported in Section 3.2.2. In fact, RMSD values (2–6 10−4 sr−1 in this work; 3–7 10−4 sr−1 in Tilstone et al., 2022) or Pearson correlation coefficients (0.45–0.78 in this work; 0.38–0.6) follow a similar pattern. Differences are mainly observed in the bias, with negative values in Tilstone et al. (2022) (−4 10−4 to −1 10−4 sr−1) instead of the positive bias found in our work (8 10−5 to 4 10−4). This disparity could be explained because we applied a stricter validation protocol with results based on a dataset of common match-ups, leading to a considerably lower number of match-ups (107 vs. 199 in Tilstone et al.). Relaxing our protocol using only bitmask as flag band, the number of valid match-ups increases until 251 and bias tends to be lower (likewise using the AERONET-OC dataset), so that comparable negative bias values (−8.9 10−5 to −1.4 10−4) were found in the 490–665-nm spectral range.
Regarding the level-3 datasets, the in-situ distribution for the complete OC-CCI v.6 time series (Figure 10A) is characterized by lower Rrs values at 490 and 560 nm in comparison with the OLCI period (Figures 10B, C). Differences are more remarkable at 560 nm, with an upper quartile value approximately 3.5 10−3 sr−1 against a peak of almost 5 10−3 sr−1. These discrepancies could be explained by two facts. Firstly, differences in the optical water types related to the data availability from the three AERONET-OC sites: the complete time series include more spectra from Helsinki Lighthouse, whereas in-situ data from Irbe Lighthouse (available since 2018) become more predominant during the OLCI period as measurements at the Helsinki Lighthouse ended in 2019. Secondly, the results at 560 nm for the complete time series use in-situ data from the AERONET-OC band at 555 nm available between 2005 and 2011, introducing uncertainties associated with the band-shifting process. However, the results for the OLCI period are only based on the AERONET-OC band at 560 nm introduced in 2018 (Table 1).
Using the common set of match-ups, CMEMS-OLCI and multisensor OC-CCI v.6 show equivalent distributions and metrics (with some differences indicated in Section 3.3) notwithstanding the different spatial resolution (300 m vs. 1 km). This similar behavior could be explained because both datasets are based on the same AC processor, i.e., POLYMER (with the exception of SeaWiFS), and that from 2020 onward, OC-CCI v.6 is based only on OLCI from Sentinel-3A and Sentinel-3B, as MODIS-AQUA and VIIRS are included only until the end of 2019 (OC-CCI, 2022). As expected, the metrics from CMEMS-OLCI L3 (Figure 6) are very close to those from POLYMER OLCI L2 (Figure 12).
Our results based on the complete OC-CCI v.6 time series differ from the metrics for OC-CCI v4.2 reported in Brando et al. (2021). OC-CCI v.6 performs better in terms of correlation for the 412–490-nm spectral range, whereas R2 values are similar for the other bands, with more remarkable differences at 400 nm (0.51 vs. 0.05) and 442.5 nm (0.66 vs. 0.34). However, OC-CCI v4.2 shows lower positive bias values (0.1 10−4–0.8 10−4 sr−1 against 1.2 10−4–6.9 10−4 sr−1) and performs better in terms of APD or RPD in the 412–560-nm spectral range. Finally, OC-CCI v.6 shows lower bias, APD, and RPD at 665 nm.
The main differences between both OC-CCI versions explaining these discrepancies are the change of reference sensor from SeaWiFS to MERIS, the introduction of OLCI from Sentinel-3A and Sentinel-3B, and the shift of the green band from 555 nm to 560 nm. Moreover, the results in Brando et al. (2021) include 680 match-ups from 2005 to 2019, most of them from Gustav Dalen Tower and Helsinki Lighthouse, as the results from v.6 until 2022 introduce more in-situ data from Irbe Lighthouse. Note also that a stricter quality control (9 valid pixels in the extractions window instead of 4) was introduced in this work.
Table 4 shows the metrics from CMEMS-OLCI Rrs for the three sites. Overall, all of them are characterized by CDOM-dominated waters, and the metrics follow the same spectral pattern. The results from Gustav Dalen Tower and Helsinki Lighthouse lead to similar results, with more match-ups and a better adjustment (R2) from Gustav Dalen Tower. In the case of Irbe Lighthouse, the results show a poorer agreement at 412.5 nm and lower RMSD and bias in the 490–560-nm spectral range, which could be explained by a lower Rrs range.
OC-CCI v.6 chl-a retrievals show validation results (R2 = 0.29; RPD = 5%; APD = 69%; bias = −0.14), consistent with those reported for the previous version of the multisensor level-3 processing chain, i.e., OC-CCI v4.2 (Brando et al., 2021: R2 = 0.24; RPD = 41%; APD = 90%; bias = −0.78), with a better performance considering all the metrics. Overall, the effect of the positive bias observed in the 412–510-nm spectral range (Figures 12, 13) seems to be adequately handled by the ensemble approach.
Chl-a validation results from CMEMS-OLCI are similar to those from OC-CCI v.6 using the common set of match-ups (Figure 13). The most remarkable difference is the lower bias (−0.03 against −0.22), which is consistent with the bias decrease with the introduction of OLCI in the multisensor level-3 datasets from −0.78 (OC-CCI v.4.2, without OLCI, Brando et al., 2021) to −0.22 (OC-CCI v.6, with OLCI, this work). In any case, further research is required because of the small number of chl-a match-ups available for the OLCI period.
Scatter plots of co-collocated OC-CCI v.6 and CMEMS-OLCI data points (Figure 14) show better statistical figures for Rrs (except for 443 nm) than chl-a. Note that OC-CCI v.6 Rrs are derived from different space sensors and AC, whereas CMEMS-OLCI Rrs are obtained from Sentinel-A and Sentinel-B images processed with POLYMER v.4.14. With the exclusion of outliers, which are possibly related to the resolution and coverage of both datasets, most Rrs differences can be attributed to the specificities of data processing and absolute radiometric accuracy of the reference sensor (MERIS for OC-CCI v.6 and OLCI for CMEMS-OLCI).
An overall good agreement was found in the comparison between chl-a retrievals from CMEMS-OLCI and OC-CCI v.6, with a tendency of CMEMS-OLCI toward greater chl-a concentrations (Figure 14).
Nevertheless, the differences in Rrs are amplified in terms of chl-a retrieval. This is probably due to the non-linear nature of the MLP retrievals of chl-a and of the weights in the chl-aENS3 ensemble approach adopted in this study, as well as the underlying relationship between apparent and inherent optical properties in the Baltic Sea.
An issue is the presence of erroneous data points caused by underestimation in the chl-a retrievals from CMEMS-OLCI (<0.5 mg m−3) as compared with OC-CCI v.6 (1.5–15 mg m−3) (Figure 14). These wrong retrievals are caused by anomalously high OLCI Rrs values with a smaller range at 490 nm (4–5 10−3 sr−1 in CMEMS-OLCI against 0.01–3.5 10−3 sr−1 in OC-CCI v.6) and 510 nm (3–3.25 10−3 sr−1 in CMEMS-OLCI against 0.01–2.5 10−3 sr−1 in OC-CCI v.6). Note that these points are not clearly visible in the scatter plots in Figure 14.
Potential differences in the validation results between the available datasets (i.e., COMBINE and Alg@line, see Section 2.2.2), as well as differences in the analytical methods and protocols for the chl-a concentration estimation, were not considered in this study because of the limited number of match-ups, mainly for the OLCI period. It should be noted that Brando et al. (2021) reported higher uncertainty for the match-ups of their multisensor time series with the COMBINE measurements as compared with Alg@line water samples due to different sampling strategies and dynamic ranges of both data sources.
In our sampling to perform the comparative analysis (a point every 10 km, an image every 10 days, see Section 2.5), only 143 points (of 190,546) from 32 images (of 240) were identified as erroneous. For most of the images, only one erroneous point was found, with a maximum of 28 points on a single image. Despite this low impact, further research with a full sampling is required to evaluate the actual effect of these wrong pixels and to implement a flagging procedure.
Regarding wrong Rrs values with a lower impact on the chl-a results (e.g., at 442 nm or 670 nm, see Figure 14), the presence in our sampling is limited to a small number of points by image (often only one). For instance, we identified 216 erroneous points in 64 images at 442 nm and only 32 points in 18 images at 660 nm. In any case, likewise chl-a, a further masking could improve the mapping results.
5 Conclusions
In this study, the performance of four atmospheric correction processors for the Rrs retrieval from Sentinel-3 OLCI was assessed within the development of the regional ocean color processing chain for the Baltic Sea. The validations with the in-situ measurements collected at three AERONET-OC sites and those relying on the Alg@line shipborne hyperspectral radiometry show that POLYMER v.4.14 was the best-performing processor in terms of error and fitness in the visible spectral range, as well as spatial coverage. Results also document the relevance of shipborne radiometry to complement in-situ measurements from fixed sites, allowing for a larger spatial footprint across all subbasins.
POLYMER-derived Rrs spectra were thus employed to retrieve chl-a from OLCI full-resolution (300 m) data using the bio-optical ensemble scheme already introduced in the CMEMS processing chain for the Baltic Sea. Additionally, this study evaluated the operational Rrs and chl-a multiyear time series (from 1997 to 2022) for the Baltic Sea based on OC-CCI v.6.
The chl-a values retrieved from OC-CCI v.6 and OLCI Rrs using the same regional bio-optical ensemble scheme were compared with the in-situ chl-a measurements. Results confirm previous analyses undertaken within the CMEMS products assessments, even if the number of OLCI match-ups (2016–2019) was lower. A study extension is planned to include more recent in-situ measurements once available.
Finally, an overall good agreement was found in the comparison between chl-a retrievals from OLCI and OC-CCI v.6. However, differences between the Rrs bands used as input for the bio-optical ensemble scheme were amplified in terms of chl-a retrieval. A flagging strategy should be devised to identify and reduce the presence of erroneous data points in both datasets. Furthermore, a sensitivity analysis is then part of the future developments to analyze the response of the bio-optical ensemble by adding synthetic offsets and noise to input Rrs spectra and verify how it affects the chl-a retrieval.
Our results confirm that the quality of operational ocean color datasets presented in this study is suitable for studies on phytoplankton phenology, bloom occurrence, water quality monitoring, and eutrophication assessment in this threatened ecosystem.
Data availability statement
The datasets presented in this study can be found in online repositories. The names of the repository/repositories and accession number(s) can be found below: E.U. Copernicus Marine Service Information (CMEMS) Marine Data Store (MDS: marine.copernicus.eu): OCEANCOLOUR_BAL_BGC_L3_NRT_009_131 (Baltic Sea Ocean Colour Plankton, Reflectances, Transparency and Optics L3 NRT daily observations). E.U. Copernicus Marine Service Information (CMEMS). Marine Data Store (MDS). DOI: 10.48670/moi-00294 (Accessed on 10-JUL-2023)OCEANCOLOUR_BAL_BGC_L3_MY_009_133 (Baltic Sea Multiyear Ocean Colour Plankton, Reflectances and Transparency L3 daily observations). E.U. Copernicus Marine Service Information (CMEMS). Marine Data Store (MDS). DOI: 10.48670/moi-00296 (Accessed on 10-JUL-2023).
Author contributions
LG: Conceptualization, Data curation, Formal analysis, Software, Validation, Visualization, Writing – original draft. VB: Conceptualization, Formal analysis, Funding acquisition, Methodology, Project administration, Software, Supervision, Validation, Visualization, Writing – original draft. ADC: Data curation, Writing – review & editing. SC: Data curation, Software, Writing – review & editing. DD’A: Conceptualization, Funding acquisition, Methodology, Software, Supervision, Writing – review & editing. TK: Writing – review & editing. JA: Data curation, Writing – review & editing. TS: Writing – review & editing.
Funding
The author(s) declare financial support was received for the research, authorship, and/or publication of this article. This work has been performed in the context of the Ocean Colour Thematic Assembly Centre of the Copernicus Marine Environment and Monitoring Service (contract: 21001L02-COP-TAC OC-2200– Lot 2: Provision of Ocean Colour Observation Products (OC-TAC)) and the HYPERNETS project funded by the European Union’s Horizon 2020 research and innovation programme (Grant agreement no 775983).
Acknowledgments
EUMETSAT is acknowledged for the provision of the Copernicus Sentinel-3 full-resolution data. The Plymouth Marine Laboratory made available the OC-CCI v.6 Rrs spectra at 1 km resolution produced using the OC-CCI processor within the Ocean Colour Thematic Assembly Centre activities. The AERONET Team is acknowledged for the continuous effort in supporting the AERONET-OC subnetwork. Giuseppe Zibordi, Barbara Bulgarelli, and Frederic Melin from the Joint Research Center of the European Commission are acknowledged for establishing and maintaining the Irbe Lighthouse, Gustav Dalen Tower, and Helsinki Lighthouse AERONET-OC sites. ICES and HELCOM along with all single contributors are thanked for the COMBINE in-situ dataset. Scientists and technical personnel at SYKE are thanked for the acquisition of the Alg@line in-situ dataset. The ESA Sentinel Application Platform and Sentinel tool-box development teams are thanked for making the SNAP software freely available. We are grateful to Vega Forneris and Flavio Lapadula for maintaining the satellite data processing and the satellite data archive at CNR-ISMAR.
Conflict of interest
The authors declare that the research was conducted in the absence of any commercial or financial relationships that could be construed as a potential conflict of interest.
Publisher’s note
All claims expressed in this article are solely those of the authors and do not necessarily represent those of their affiliated organizations, or those of the publisher, the editors and the reviewers. Any product that may be evaluated in this article, or claim that may be made by its manufacturer, is not guaranteed or endorsed by the publisher.
References
Ahlman M., Alenius P., Attila J., Arnkil A., Arponen H., Below A., et al. (2020). Seurantakäsikirja suomen merenhoitosuunnitelman seurantaohjelmaan vuosille 2020–2026 (Manual for marine monitoring in Finland 2020–2026) (Helsinki, Finland: Suomen Ympäristökeskus).
Alikas K., Ansko I., Vabson V., Ansper A., Kangro K., Uudeberg K., et al. (2020). Consistency of radiometric satellite data over lakes and coastal waters with local field measurements. Remote Sens. 12 (4), 616. doi: 10.3390/rs12040616
Andersen J. H., Axe P., Backer H., Carstensen J., Claussen U., Fleming-Lehtinen V., et al. (2011). Getting the measure of eutrophication in the baltic sea: towards improved assessment principles and methods. Biogeochemistry 106, 137–156. doi: 10.1007/s10533-010-9508-4
Attilla J., Koponen S., Kallio K., Lindfors A., Kaitala S., Ylöstalo P. (2013). MERIS Case II water processor comparison on coastal sites of the northern Baltic Sea. Remote Sens. Environ. 128, 138–149. doi: 10.1016/j.rse.2012.07.009
Beltrán-Abaunza J. M., Kratzer S., Brockmann C. (2014). Evaluation of MERIS products from Baltic Sea coastal waters rich in CDOM. Ocean Sci. 10, 377–396. doi: 10.5194/os-10-377-2014
Berthon J.-F., Zibordi G. (2010). Optically black waters in the Northern Baltic Sea. Geophys. Res. Lett. 37, L09605. doi: 10.1029/2010GL043227
Blondeau-Patissier D., Gower J. F. R., Dekker A. G., Phinn S. R., Brando V. E. (2014). A review of ocean color remote sensing methods and statistical techniques for the detection, mapping and analysis of phytoplankton blooms in coastal and open oceans. Prog. Oceanogr. 123, 123–144. doi: 10.1016/j.pocean.2013.12.008
Brando V. E., Sammartino M., Colella S., Bracaglia M., Di Cicco A., D’Alimonte D., et al. (2021). Phytoplankton bloom dynamics in the baltic sea using a consistently reprocessed time series of multi-sensor reflectance and novel chlorophyll-a retrievals. Remote Sens. 13, 3071. doi: 10.3390/rs13163071
Brewin R. J., Sathyendranath S., Müller D., Brockmann C., Deschamps P. Y., Devred E., et al. (2015). The Ocean Colour Climate Change Initiative: III. A round-robin comparison on in-water bio-optical algorithms. Remote Sens. Environ. 162, 271–294. doi: 10.1016/j.rse.2013.09.016
Brockmann C., Doerffer R., Peters M., Stelzer K., Embacher S., Ruescas A. (2016). “Evolution of the C2RCC neural network for sentinel 2 and 3 for the retrieval of ocean colour products in normal and extreme optically complex waters,” in Proc. Living Planet Symposium. 740, 1–13. (Prague, Czech Republic: ESA).
Cazzaniga I., Zibordi G., Mélin F., Kwiatkowska E., Talone M., Dessailly D., et al. (2022). Evaluation of OLCI neural network radiometric water products. IEEE Trans. Geosci. Remote Sens. 19 (1-5), 1503405. doi: 10.1109/LGRS.2021.3136291
Concha J., Bracaglia M., Brando V. (2021). Assessing the influence of different validation protocols on Ocean Colour match-up analyses. Remote Sens. Environ. 259, 112415. doi: 10.1016/j.rse.2021.112415
D’Alimonte D., Kajiyama T., Saptawijaya A. (2016). Ocean color remote sensing of atypical marine optical cases. IEEE Trans. Geosci. Remote Sens. 54, 6574–6586. doi: 10.1109/TGRS.2016.2587106
D’Alimonte D., Zibordi G., Berthon J.-F., Canuti E., Kajiyama T. (2011). Bio-optical algorithms for european seas: performance and applicability of neural-net inversion schemes (Luxembourg: Publications Office of the European Union).
D’Alimonte D., Zibordi G., Kajiyama T., Berthon J.-F. (2014). Comparison between MERIS and Regional High-Level Products in European Seas. Remote Sens. Environ. 140, 378-395.
Darecki M., Stramski D. (2004). An evaluation of MODIS and seaWiFS bio-optical algorithms in the baltic sea. Remote. Sens. Environ. 89, 326–350. doi: 10.1016/j.rse.2003.10.012
Doerffer R., Schiller H. (2007). The MERIS Case 2 water algorithm. Int. J. Remote Sens. 28, 517–535. doi: 10.1080/01431160600821127
EUMETSAT (2019) EUMETSAT OCDB user manual. Copyright 2019, copernicus revision 763d8907 (Accessed December 11, 2023).
EUMETSAT (2021) Sentinel-3 OLCI L2 report for baseline collection OL_L2M_003 - EUM/RSP/REP/21/1211386 (Accessed December 11, 2023).
EUMETSAT (2022) Recommendations for Sentinel-3 OLCI Ocean Colour product validations in comparison with in situ measurements – Matchup Protocols (Accessed December 11, 2023).
Finni T., Kononen K., Olsonen R., Wallström K. (2001). The history of cyanobacterial blooms in the Baltic Sea. Ambio 30, 172–178. doi: 10.1579/0044-7447-30.4.172
Fleming V., Kaitala S. (2006). Phytoplankton spring bloom intensity index for the baltic sea estimated for the years 1992 to 2004. Hydrobiologia 554, 57–65. doi: 10.1007/s10750-005-1006-7
Fleming-Lehtinen V., Andersen J. H., Carstensen J., Łysiak-Pastuszak E., Murray C., Pyhälä M., et al. (2015). Recent developments in assessment methodology reveal that the Baltic Sea eutrophication problem is expanding. Ecol. Indic. 48, 380–388. doi: 10.1016/j.ecolind.2014.08.022
González Vilas L., Brando V. E., Concha A. J., Dogliotti A. I., Goyens C., Doxaran D. (2023) HYPERNETS multi-mission validation of water products V2. Deliverable D7.3, version 1.0, 24/04/2023 (Accessed December 11, 2023).
González Vilas L., Brando V. E., Concha A. J., Goyens C., Dogliotti A. I., Doxaran D., et al (2024). Validation of satellite water products based on Hypernets in situ data using a Match-up Database File (MDB) structure. Front. Remote Sens.
Groom S., Sathyendranath S., Ban Y., Bernard S., Brewi R., Brotas V., et al. (2019). Satellite ocean colour: current status and future perspective. Front. Mar. Sci. 6, 485. doi: 10.3389/fmars.2019.00485
Heiskanen A.-S., Bonsdorff E., Joas M. (2019). “Baltic sea: A recovering future from decades of eutrophication,” in Coasts and estuaries (Amsterdam, The Netherlands: Elsevier), 343–362.
HELCOM (2017). Manual for marine monitoring in the COMBINE programme of HELCOM (Helsinki, Finland: HELCOM).
HELCOM (2018). State of the baltic sea—Second HELCOM holistic assessment 2011–2016 (Helsinki, Finland: HELCOM).
Hieronymi M., Müller D., Doerffer R. (2017). The OLCI neural network swarm (ONNS): A bio-geo-optical algorithm for open ocean and coastal waters. Front. Mar. Sci. 4, 140. doi: 10.3389/fmars.2017.00140
Hjerne O., Hajdu S., Larsson U., Downing A. S., Winder M. (2019). Climate driven changes in timing, composition and magnitude of the Baltic Sea Phytoplankton Spring Bloom. Front. Mar. Sci. 6. doi: 10.3389/fmars.2019.00482
Kahru M., Elmgren R., Di Lorenzo E., Savchuk O. (2018). Unexplained interannual oscillations of cyanobacterial blooms in the Baltic Sea. Sci. Rep. 8, 6365. doi: 10.1038/s41598-018-24829-7
Kahru M., Horstmann U., Rud O. (1994). Satellite detection of increased cyanobacteria blooms in the Baltic Sea: Natural fluctuation or ecosystem change? Ambio 23, 469–472.
Kahru M., Savchuk O., Elmgren R. (2007). Satellite measurements of cyanobacterial bloom frequency in the Baltic Sea: Interannual and spatial variability. Mar. Ecol. Prog. Ser. 343, 15–23. doi: 10.3354/meps06943
Kaitala S., Zibordi G., Melin F., Seppälä J., Ylöstalo P. (2008). Coastal water monitoring and remote sensing products validation using ferrybox and above-water radiometric measurements. EARSeL eProceedings 7, 75–80.
Kajiyama T., D’Alimonte D., Zibordi G. (2019). Algorithms merging for the determination of chlorophyll-a concentration in the black sea. IEEE Geosci. Remote Sens. Lett. 16, 677–681. doi: 10.1109/LGRS.2018.2883539
Kratzer S., Moore G. (2018). Inherent optical properties of the Baltic Sea in comparison to other seas and oceans. Remote. Sens. 10, 418. doi: 10.3390/rs10030418
Kratzer S., Vinterhav C. (2010). Improvement of MERIS level 2 products in baltic sea coastal areas by applying the improved contrast between ocean and land processor (ICOL)—Data analysis and validation. Oceanologia 52, 211–236. doi: 10.5697/oc.52-2.211
Kyryliuk D., Kratzer S. (2019). Evaluation of sentinel-3A OLCI products derived using the case-2 regional coast colour processor over the baltic sea. Sensors 19, 3609. doi: 10.3390/s19163609
Lee Z., Carder K. L., Arnone R. A. (2014). Update of the quasi-analytical algorithm (QAA_V6) (Dartmouth, Canada: IOCCG).
Leppäranta M., Myrberg K. (2009). Physical oceanography of the baltic sea (Berlin/Heidelberg, Germany: Springer).
Le Traon P. Y., Reppucci A., Alvarez Fanjul E., Aouf L., Behrens A., Belmonte M., et al. (2019). From observation to information and users: the copernicus marine service perspective. Front. Mar. Sci. 6, 234. doi: 10.3389/fmars.2019.00234
Ligi M., Kutser T., Kallio K., Attila J., Koponen S., Paavel B., et al. (2017). Testing the performance of empirical remote sensing algorithms in the Baltic Sea waters with modelled and in situ reflectance data. Oceanologia 59, 57–68. doi: 10.1016/j.oceano.2016.08.002
Mélin F., Sclep G. (2015). Band shifting for ocean color multi-spectral reflectance data. Opt. Express 23, 2262. doi: 10.1364/OE.23.002262
OC-CCI (2022) Ocean colour climate change initiative (OC-CCI) – phase3. Product user guide for v6.0 dataset (Accessed December 11, 2023).
OCEANCOLOUR_BAL_BGC_L3_MY_009_133 (2023) E.U. Copernicus marine service information (CMEMS). Marine data store (MDS) (Accessed July 10, 2023).
Odermatt D., Gitelson A., Brando V. E., Schaepman M. (2012). Review of constituent retrieval in optically deep and complex waters from satellite imagery. Remote Sens. Environ. 118, 116–126. doi: 10.1016/j.rse.2011.11.013
Omstedt A., Elken J., Lehmann A., Piechura J. (2004). Knowledge of the Baltic Sea physics gained during the BALTEX and related programmes. Prog. Oceanogr. 63, 1–28. doi: 10.1016/j.pocean.2004.09.001
O’Reilly J. E., Werdell P. J. (2019). Chlorophyll algorithms for ocean color sensors—OC4, OC5 & OC6. Remote. Sens. Environ. 229, 32–47. doi: 10.1016/j.rse.2019.04.021
Pitarch J., Volpe G., Colella S., Krasemann H., Santoleri R. (2016). Remote sensing of chlorophyll in the baltic sea at basin scale from 1997 to 2012 using merged multi-sensor data. Ocean Sci. 12, 379–389. doi: 10.5194/os-12-379-2016
Qin P., Simis S. G. H., Tilstone G. H. (2017). Radiometric validation of atmospheric correction for MERIS in the Baltic Sea based on continuous observations from ships and AERONET-OC. Remote Sens. Environ. 200, 263–280. doi: 10.1016/j.rse.2017.08.024
Sathyendranath S., Brewin R., Brockmann C., Brotas V., Calton B., Chuprin A., et al. (2019). An ocean-colour time series for use in climate studies: The experience of the ocean-colour climate change initiative (OC-CCI). Sensors 19, 4285. doi: 10.3390/s19194285
Sathyendranath S., Groom S. B., Jackson T., Volpe G., Calton B. (2022). ESA Ocean colour climate change initiative-phase 3 climate assessment report (Accessed December 11, 2023).
Schroeder T., Behnert I., Schaale M., Fischer J., Doerffer R. (2007). Atmospheric correction algorithm for MERIS above case-2 waters. Int. J. Remote Sens. 28, 1469–1486. doi: 10.1080/01431160600962574
Schroeder T., Fischer J., Schaale M., Fell F. (2003). “Artificial-neural-network-based atmospheric correction algorithm: application to MERIS data,” in Proc. SPIE 4892, Ocean Remote Sensing and Applications. doi: 10.1117/12.467293
Schroeder T., Schaale M., Lovell J., Blondeau-Patissier D. (2022). An ensemble neural network atmospheric correction for Sentinel-3 OLCI over coastal waters providing inherent model uncertainty estimation and sensor noise propagation. Remote Sens. Environ. (SPIE) 270, 112848. doi: 10.1016/j.rse.2021.112848
Simis S. G. H., Olsson J. (2013). Unattended processing of shipborne hyperspectral reflectance measurements. Remote Sens. Environ. 135, 202–212. doi: 10.1016/j.rse.2013.04.001
Simis S., Qin P., Attila J., Kervinen M., Kallio K., Koponen S., et al. (2021). Baltic sea shipborne hyperspectral reflectance data from 2016 (1.0) (Zenodo), 5572537.
Simis S. G. H., YloÈstalo P., Kallio K. Y., Spilling K., Kutser T. (2017). Contrasting seasonality in optical-biogeochemical properties of the Baltic Sea. PloS One 12, e0173357. doi: 10.1371/journal.pone.0173357
Steinmetz F., Deschamps P.-Y., Ramon D. (2011). Atmospheric correction in presence of sun glint: application to MERIS. Opt. Express (SPIE) 19, 9783-9800. doi: 10.1364/OE.19.009783
Steinmetz F., Ramon D. (2018). “Sentinel-2 MSI and Sentinel-3 OLCI consistent ocean colour products using POLYMER,” in Proceedings of the remote sensing of the open and coastal ocean and inland waters(Honolulu, HI, USA).
Tilstone G. H., Pardo S., Simis S. G. H., Qin P., Selmes N., Dessailly D., et al. (2022). Consistency between satellite ocean colour products under high coloured dissolved organic matter absorption in the baltic sea. Remote Sens. 14 (1), 89. doi: 10.3390/rs14010089
Toming K., Kutser T., Uiboupin R., Arikas A., Vahter K., Paavel B. (2017). Mapping water quality parameters with sentinel-3 ocean and land colour instrument imagery in the baltic sea. Remote Sens. 9, 1070. doi: 10.3390/rs9101070
von Schuckmann K., Le Traon P.-Y., Smith N., Pascual A., Djavidnia S., Brasseur P., et al. (2022). Copernicus ocean state report, issue 6. J. Oper. Oceanogr. 15, s1–s220. doi: 10.1080/1755876X.2022.2095169
Warren M. A., Simis S. G. H., Martinez-Vicente V., Poser K., Bresciani M., Alikas K., et al. (2019). Assessment of atmospheric correction algorithms for the sentinel-2A multispectral imager over coastal and inland waters. Remote Sens. Environ. 225, 267–289. doi: 10.1016/j.rse.2019.03.018
Wasmund N., Tuimala J., Suikkanen S., Vandepitte L., Kraberg A. (2011). Long-term trends in phytoplankton composition in the western and central Baltic Sea. J. Mar. Syst. 87 (2), 145–159. doi: 10.1016/j.jmarsys.2011.03.010
Ylöstalo P., Seppälä J., Kaitala S., Maunula P., Simis S. (2016). Loadings of dissolved organic matter and nutrients from the Neva Riverinto the Gulf of Finland–Biogeochemical composition and spatial distribution within the salinity gradient. Mar. Chem. 186, 58–71. doi: 10.1016/j.marchem.2016.07.004
Zhang D., Lavender S., Muller J.-P., Walton D., Zou X., Shi F. (2018). MERIS observations of phytoplankton phenology in the Baltic Sea. Sci. Total. Environ. 642, 447–462. doi: 10.1016/j.scitotenv.2018.06.019
Zibordi G., Berthon J.-F., Mélin F., D’Alimonte D. (2011). Cross-site consistent in situ measurements for satellite ocean color applications: the biOMaP radiometric dataset. Remote Sens. Environ. 115, 2104–2115. doi: 10.1016/j.rse.2011.04.013
Zibordi G., Kwiatkowska E., Mélin F., Talone M., Cazzaniga I., Dessailly D., et al. (2022). Assessment of OLCI-A and OLCI-B radiometric data products across European seas. Remote Sens. Environ. 272, 112911. doi: 10.1016/j.rse.2022.112911
Zibordi G., Melin F., Berthon J.-F. (2018). A regional assessment of OLCI data products. IEEE Geosci. Remote Sens. Lett. 15, 1490–1494. doi: 10.1109/LGRS.2018.2849329
Keywords: ocean color, atmospheric correction, Baltic Sea, Sentinel-3 OLCI, chlorophyll-a, optically complex waters
Citation: González Vilas L, Brando VE, Di Cicco A, Colella S, D’Alimonte D, Kajiyama T, Attila J and Schroeder T (2024) Assessment of ocean color atmospheric correction methods and development of a regional ocean color operational dataset for the Baltic Sea based on Sentinel-3 OLCI. Front. Mar. Sci. 10:1256990. doi: 10.3389/fmars.2023.1256990
Received: 11 July 2023; Accepted: 22 December 2023;
Published: 16 January 2024.
Edited by:
Huizeng Liu, Shenzhen University, ChinaReviewed by:
Zhigang Cao, Chinese Academy of Sciences (CAS), ChinaJamie Shutler, University of Exeter, United Kingdom
Copyright © 2024 González Vilas, Brando, Di Cicco, Colella, D’Alimonte, Kajiyama, Attila and Schroeder. This is an open-access article distributed under the terms of the Creative Commons Attribution License (CC BY). The use, distribution or reproduction in other forums is permitted, provided the original author(s) and the copyright owner(s) are credited and that the original publication in this journal is cited, in accordance with accepted academic practice. No use, distribution or reproduction is permitted which does not comply with these terms.
*Correspondence: Luis González Vilas, bHVpcy5nb256YWxlenZpbGFzQGFydG92LmlzbWFyLmNuci5pdA==