- 1Trauma Research Center, Fourth Medical Center of the Chinese PLA General Hospital, Beijing, China
- 2Department of Burn Surgery, Changhai Hospital, Naval Medical University, Shanghai, China
- 3School of Mathematics and Statistics, Beijing Institute of Technology, Beijing, China
- 4School of Computer Science and Technology, Wuhan University of Technology, Wuhan, China
- 5Department of Cardiothoracic Surgery, Changzheng Hospital, Naval Medical University, Shanghai, China
- 6Medical Research and Biometrics Center, Fuwai Hospital, National Center for Cardiovascular Diseases, Chinese Academy of Medical Sciences and Peking Union Medical College, Beijing, China
- 7Department of General Surgery, First Medical Center of Chinese PLA General Hospital, Beijing, China
Introduction: The incidence of postoperative sepsis is continually increased, while few studies have specifically focused on the risk factors and clinical outcomes associated with the development of sepsis after surgical procedures. The present study aimed to develop a mathematical model for predicting the in-hospital mortality among patients with postoperative sepsis.
Materials and Methods: Surgical patients in Medical Information Mart for Intensive Care (MIMIC-III) database who simultaneously fulfilled Sepsis 3.0 and Agency for Healthcare Research and Quality (AHRQ) criteria at ICU admission were incorporated. We employed both extreme gradient boosting (XGBoost) and stepwise logistic regression model to predict the in-hospital mortality among patients with postoperative sepsis. Consequently, the model performance was assessed from the angles of discrimination and calibration.
Results: We included 3,713 patients who fulfilled our inclusion criteria, in which 397 (10.7%) patients died during hospitalization, and 3,316 (89.3%) patients survived through discharge. Fluid-electrolyte disturbance, coagulopathy, renal replacement therapy (RRT), urine output, and cardiovascular surgery were important features related to the in-hospital mortality. The XGBoost model had a better performance in both discriminatory ability (c-statistics, 0.835 vs. 0.737 and 0.621, respectively; AUPRC, 0.418 vs. 0.280 and 0.237, respectively) and goodness of fit (visualized by calibration curve) compared to the stepwise logistic regression model and baseline model.
Conclusion: XGBoost model has a better performance in predicting hospital mortality among patients with postoperative sepsis in comparison to the stepwise logistic regression model. Machine learning-based algorithm might have significant application in the development of early warning system for septic patients following major operations.
Introduction
Sepsis is a severe complication following major surgery and responsible for poor outcomes of postoperative patients by inducing multiple organ dysfunction and increasing in-hospital mortality. Although great progress has been made in the early recognition and therapeutic strategies, the incidence and mortality of septic complications remain unacceptably high (1–3). It has been documented that there are ~30% of septic patients after surgical procedures, and the number of patients who developed postoperative sepsis increases annually (4–6). Given the high incidence and poor prognosis, the Agency for Healthcare Research and Quality (AHRQ) defined the “postoperative sepsis” as a critical indicator for patients' safety, which mainly focused on preventable surgical complications and iatrogenic events after surgical procedures (7, 8).
Various evidences have demonstrated that immunocompromised state is strongly associated with the pathogenesis of postoperative sepsis (9). For example, impaired antigen presenting capacity of monocytes and dominant differentiation of type 2 helper T cells were all characterized in the animal models of postoperative sepsis (10–12). Meanwhile, researchers identified disparate gene expression profiles of whole blood cells from surgical patients with or without postoperative sepsis, and found that the expression patterns of interleukin (IL) 1β (IL-1β), tumor necrosis factor (TNF) superfamily, member 2, and CD3D were significantly different (13). However, the “Surviving Sepsis Campaign” (SSC) guidelines didn't provide distinctive treatments for postoperative sepsis (14). Moreover, there were insufficient clinical trials that specifically testified the guidelines in the postoperative sepsis cohort. Most of the studies examined the short-term mortality in septic patients admitted to emergency department or intensive care unit (ICU) that covered multiple types of sepsis (8, 15, 16). On the contrary, few studies specifically characterized the clinical outcomes of patients with postoperative sepsis.
In the present study, we aimed to establish a predictive model on in-hospital mortality among patients with postoperative sepsis. Given the limitation of conventional statistical methods in processing retrospective data that contained covariates of high correlation and inevitable missing values, we enrolled advanced machine learning algorithm, called extreme gradient boosting (XGBoost), to identify the important clinical features for predicting in-hospital mortality.
Materials and Methods
Database
Medical Information Mart for Intensive Care-III (MIMIC-III), a large online critical care database, was applied for the current study (17). Of note, MIMIC-III was a comprehensive dataset which contained clinical data of all the patients admitted to ICU of Beth Israel Deaconess Medical Center (BIDMC) in Boston, Massachusetts, from 2001 to 2012. In brief, it included more than fifty thousand distinct adult (aged >16 years) ICU patients and approximately eight thousand neonate cases. We had obtained the permission for accessing the database after the completion of “Protecting Human Research Participants,” an online training course launched by National Institutes of Health (NIH) (certification number: 32450965). We conducted this study in accordance with the Transparent Reporting of a multivariable prediction model for Individual Prognosis or Diagnosis (TRIPOD) recommendation (18).
Study Population
The selection of patients was based on “postoperative sepsis” criteria proposed by AHRQ combining with Sepsis 3.0 criteria, in which sepsis was diagnosed by sequential organ failure assessment (SOFA) score ≥2 plus documented or suspected infection (7, 19). Additionally, infection was confirmed in accordance with ICD-9 code in the MIMIC-III database. In this study, we included all patients (aged >18 years) who underwent surgical procedures prior to ICU admission and fulfilled Sepsis 3.0 criteria within 24 h post ICU admission. Patients were excluded even if they were in line with AHRQ selection criteria: (1) who had a principal or secondary diagnosis of sepsis or infection on admission; (2) who were diagnosed with cancer and had other immunocompromised state, including hematologic malignancies, HIV, prolonged usage of corticosteroids, and organ transplantation; (3) who were admitted to ICU with pregnancy, childbirth, or puerperium; (4) who stayed in hospital <4 days; (5) who had incomplete or unobtainable medical data records on admission.
Variables Extraction and Outcome Measurement
Clinical and laboratory variables were collected within the first 24 h after ICU admission. Demographic data was obtained, including age, gender, body mass index (BMI), and elective surgical type. Laboratory findings, including white blood cell (WBC) counts, hematocrit, platelet counts, glucose, lactate, creatinine, blood urea nitrogen (BUN), coagulation profile, chloride, potassium, sodium, bicarbonate, albumin, bilirubin, partial pressure of arterial oxygen (PaO2), partial pressure of arterial carbon dioxide (PaCO2), total CO2, and pH were incorporated. In addition, vital signs, including blood pressure, respiratory rates, heart rates, and body temperature were included. Comorbidities, such as congestive heart failure, cardiac arrhythmia, neurological disorders, diabetes, anemia, and obesity, were also recorded. Prognostic scoring systems, including SOFA score, Oxford Acute Severity of Illness Score (OASIS), Simplified Acute Physiology Score II (SAPSII), and Glasgow Coma Scale (GCS) were calculated and analyzed by using variables obtained in the first 24 h during admission. Notably, both the maximum and minimum values of some indicators were collected and analyzed for multiple measurements.
As severe data missing might render bias, all eligible predictors were screened, and variables with more than 30% missing values were not taken into subsequent model establishment. Correspondingly, we conducted multivariate imputation for variables with <30% missing values.
We chose in-hospital mortality as our primary endpoint, which was defined as survival status at hospital discharge. Patients without outcome information were excluded from the final cohort.
Statistical Analysis
Baseline characteristics of enrolled participants were presented and compared between survivors and non-survivors by applying either Student t-test, Chi-square test and Mann-Whitney U-test as appropriate. Continuous variables were characterized as mean (standardized differences [SD]) or median (interquartile range [IQR]), while categorical or ranked data were reported as count and proportion.
We employed stepwise logistic regression model to select predictors of in-hospital mortality. Both forward and backward directions were used in variable selection processes, in which Akaike Information Criterion (AIC) was applied as the selection criteria of the optimal model.
Furthermore, we applied Extreme Gradient Boosting (XGBoost) model to predict in-hospital death among patients with postoperative sepsis. XGBoost was a machine learning algorithm, which mainly functioned as iterative refit of weak classifier to residuals of previous models, meaning that the current weak classifier was generated based on previous one in order to optimize the predictive efficiency (20, 21). In each round of iteration, it focused more on misclassified observations. As eligible variables were included into the model, it outputted the importance score of each variable. Meanwhile, XGBoost could automatically process missing data through assigning a default direction to the null values. To reach the optimal model performance of XGBoost, we assessed and tuned the hyperparameters, including learning rates, maximum depth of a tree, number of estimators, alpha, and lambda. In this study, the original dataset was randomly divided into 5 subsets. One-fold was used as testing subset, while the other four-fold were processed to tune the hyperparameters, in which 25% were applied for calibration, and four-fold cross validation with grid search was conducted in remaining 75% of data. The hyperparameters with the highest area under the receiver operator characteristic curve (AUROC) were selected. The sufficiently tuned XGBoost hyperparameters were subsequently added back for training and calibrating the model, which was further validated in one held-out testing subset (22). Detailed process for tuning hyperparameters was provided in Supplemental Figure S1.
Model performance of both models was assessed in multiple dimensions. To test discriminatory ability, we used receiver operating characteristic (ROC) curve and c-statistic. Meanwhile, calibration plot revealed the correlation between observed and predicted risk, which was applied to evaluate the goodness of fit. The area under the precision-recall curve (AUPRC) provides a robust metric for unbalanced datasets, which has been a critical measure in assessing model performance. Given that, precision-recall curve with AUPRC, accuracy and recall were also applied to evaluate the performance of models. Of note, SOFA score that was commonly used for evaluating the severity of septic patients was assigned as a baseline model, and compared with stepwise logistic regression and XGBoost models as well. Aforementioned statistical analyses were performed by using IBM SPSS Statistics software (version 23.0), Python software (version 3.4.3), and R software (version 3.6.1). Two tailed P < 0.05 was deemed as statistical significance.
Results
Participants
Among 46,520 patients in the MIMIC-III database, 15,302 of them met with Sepsis 3.0 criteria. There were 4,653 potentially eligible adult patients (aged ≥18 years) who underwent surgical procedures prior to ICU admission. After excluded 940 patients in accordance with the AHRQ exclusion criteria, 3,713 patients were deemed to develop postoperative sepsis and were eventually incorporated into the study cohort, in which 397 (10.7%) patients died during hospitalization and 3,316 (89.3%) of them survived through discharge. The detailed information with regard to the enrollment and selection process was presented in Figure 1.
The comparison of baseline characteristics between survivors and non-survivors was summarized in Table 1. Notably, patients of the non-survivor group were much older than those of the survivor group (86.6 ± 59.2 vs. 74.6 ± 48.0; P = 0.001). As for the comorbidities, patients with postoperative sepsis who died during hospitalization had higher incidence of congestive heart failure (40.6 vs. 31.2%; P < 0.001), cardiac arrhythmias (48.1 vs. 39.4%; P = 0.001), renal failure (24.7 vs. 15.8%; P < 0.001), coagulopathy (30.5 vs. 13.0%; P < 0.001), and digestive disorders (16.9 vs. 7.8%; P < 0.001). The maximum respiratory rates (29.0 ± 8.1 vs. 27.7 ± 6.7; P = 0.002) were significantly higher in patients from the non-survivor group, while the minimum systolic blood pressure (BP) (84.1 ± 18.3 vs. 89.0 ± 16.2; P < 0.001), minimum diastolic BP (55.6 ± 9.7 vs. 58.3 ± 9.4; P < 0.001), and minimum mean BP (52.7 ± 14.1 vs. 56.5 ± 12.5; P < 0.001) were lower than those from the survivor group. Compared to survivors, non-survivors had higher levels in blood lactate (2.9 [IQR: 1.8, 5.8] vs. 2.3 [IQR: 1.5, 3.8]; P < 0.001), BUN (30 [IQR: 20, 46] vs. 21 [IQR: 15, 32]; P < 0.001), and creatinine (1.4 [IQR: 0.9, 2.4] vs. 1.1 [IQR: 0.8, 1.6]; P < 0.001). Additionally, higher international normalized ratio (INR) (1.9 ± 1.8 vs. 1.6 ± 1.1; P = 0.001), longer prothrombin time (PT) (16.1 [IQR: 14.1, 20.0] vs. 15.2 [IQR: 13.7, 17.3]; P < 0.001), and activated partial thromboplastin time (APTT) (39.5 [IQR: 30.6, 71.0] vs. 35.2 [IQR: 29, 48.2]; P < 0.001) were noted among patients in the non-survivor group when compared to those in the survivor group.
Stepwise Logistic Regression Model
We performed stepwise logistic regression analysis with both forward and backward methods, in which the classifier incorporated 36 variables into the final model. As shown in Table 2, it was found that female (odds ratio (OR), 0.65 [95% confidence interval [CI, 30.6–71.0]), patients with lower BMI (OR, 0.97 [95% CI, 0.95–0.99]), patients with higher PO2 (OR for with every 10% increment, 0.98 [95% CI, 0.96–0.99]), and oxygen saturation (SpO2) (OR, 0.78 [95% CI, 0.66–0.91]) had higher possibility to survive through discharge. Conversely, neurosurgery (OR, 2.53 [95% CI, 1.57–4.04]), the complication of multiple comorbidities, especially for coagulopathy (OR, 2.36 [95% CI, 1.49–3.68]), greater values of INR (OR, 1.75 [95% CI, 1.15–2.70]), and sodium (OR, 1.08 [95% CI, 1.03–1.13]) were responsible for increased risk of in-hospital death among ICU patients with postoperative sepsis. Furthermore, higher scores in several prognostic scoring systems, including SOFA (OR, 1.08 [95% CI, 1.01–1.16]), SAPS II (OR, 1.04 [95% CI, 1.02–1.06]), and OASIS (OR, 1.05 [95% CI, 1.03–1.08]), were linked to increased in-hospital mortality.
XGBoost Model
After tuning and grid search, the hyperparameters applied in the current XGBoost model were as follows: learning rates = 0.01, number of estimators = 1,000, maximum depth of a tree = 5, alpha = 0, and lambda = 0. The importance of feature was assigned by weight which was calculated by the number of times that a feature was used to split the data across all trees. Feature importance revealed the relative contribution of each variable on predicting the in-hospital mortality. As shown in Figure 2, the fluid-electrolyte disturbance and coagulopathy were the top ranked variables that were correlated with in-hospital death among patients with postoperative sepsis, followed by renal replacement therapy (RRT), urine output, cardiovascular surgery, and digestive disorders.
Evaluation of Model Performance
The discriminatory power of both stepwise logistic and XGBoost models was evaluated by using ROC analysis and c-statistics (calculated by AUROC) in the testing subset. The XGBoost had a significantly higher c-statistics compared to that of the stepwise logistic regression and baseline models (c-statistics, 0.835 vs. 0.737 and 0.621, respectively), suggesting a better discriminative capacity of XGBoost model (Figure 3A). As presented in Figure 3B, the XGBoost model also performed better in terms of precision-recall curve when compared to stepwise logistic regression and baseline models (AUPRC, 0.418 vs. 0.280 and 0.237, respectively). Besides, the accuracy for XGBoost and stepwise logistic models were 0.88 and 0.76, respectively. The recall for XGBoost and stepwise logistic models were 0.10 and 0.75, respectively. Meanwhile, as shown in Figure 4, the calibration curve of models showed that XGBoost presented a greater goodness of fit than logistic regression model and SOFA score.
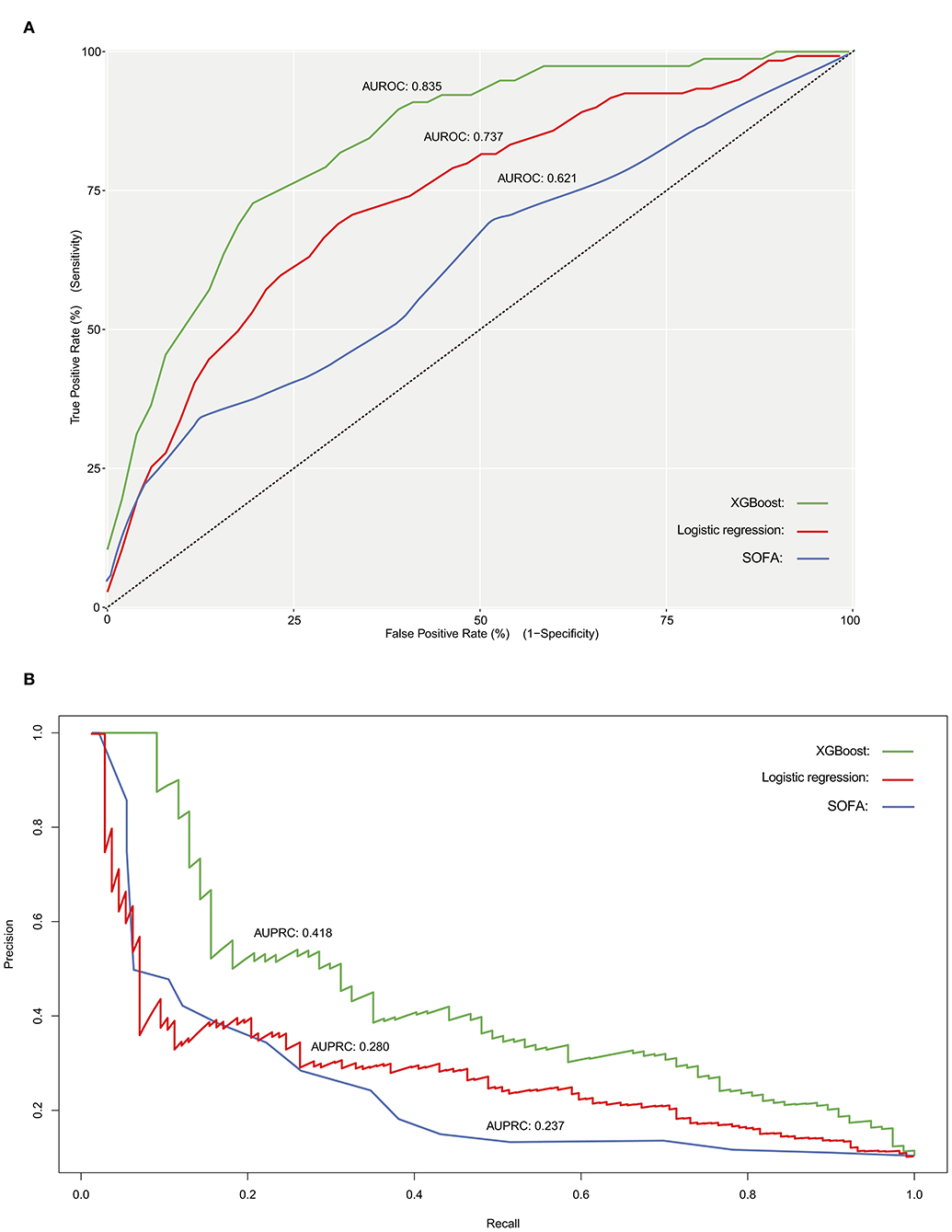
Figure 3. Receiver operating characteristic curve (A), and precision- recall curve (B) for evaluating the discriminatory ability of SOFA score (baseline model), stepwise logistic regression model as well as XGBoost model. AUROC, area under the receiver operator characteristic curve; AUPRC, area under the precision-recall curve.
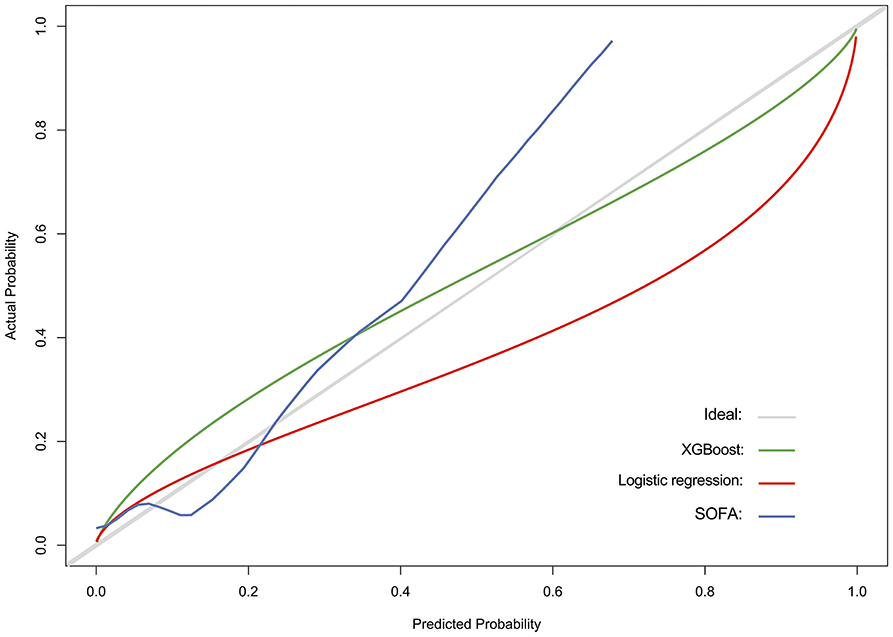
Figure 4. Calibration curve for assessing the goodness of fit for SOFA score (baseline model), stepwise logistic regression model, and XGBoost model.
Discussion
Major Findings
In the current study, we identified various clinical indicators that were associated with increased in-hospital mortality among ICU patients with postoperative sepsis. By applying sophisticated machine learning algorithm, we found that fluid-electrolyte disturbance, coagulopathy, RRT, urine output, and cardiovascular surgery were significant features for predicting in-hospital death. In addition, XGBoost model revealed a better performance in discrimination and calibration than that of the conventional stepwise logistic regression model.
Relation to Other Works
Plenty of evidence have indicated that the development of sepsis is critically involved in short-term and long-term mortality of postsurgical patients (8, 15, 23, 24). A large nationwide epidemiology of patients with elective surgery revealed an increased incidence of postoperative sepsis, ranging from 0.3% in 1997 to 0.9% in 2006, while they found that the in-hospital morality significantly decreased from 1997 to 2006 (44.4–30%) (23). Recently, Ou et al. conducted a population-based analysis in patients who underwent coronary artery bypass grafting (CABG) surgery, and they noticed that the incidence of postoperative sepsis was ~2%, and the mortality of those patients admitted to public hospital and private were 11.9% and 18.3%, respectively (15). In a retrospective analysis by Mørch et al., researchers focused on the clinical outcomes of patients who developed postoperative sepsis after hip fracture surgery. They documented a 30-day mortality of 15.8% among those patients, which was significantly higher than patients without postoperative sepsis (24). In our study, we identified an in-hospital mortality of 10.7% among ICU patients who developed postoperative sepsis. We observed an evident decline in mortality rates among surgical patients with sepsis over the past decades, while the morbidity rates showed sustained increase. The reduction of overall mortality rates might be attributed to the progress in perioperative care and extensive use of antibiotics. Meanwhile, the mortality of patients with postoperative sepsis was disparate from that of the other types of septic patients, which could be explained by different clinical settings and co-morbidities state.
Clinical Implications
The XGBoost model is capable of accurately predicting in-hospital death among patients with postoperative sepsis. Although several studies have identified the risk factors for the short-term or long-term mortality of septic patients following major operations, few of them establish feasible models to predict clinical outcomes of those patients. Unlike other types of sepsis, postoperative sepsis had some unique characteristics in both etiology and pathophysiology, which made it a specific subset (5). Therefore, it is of great importance to early recognize patients with postoperative sepsis who are at high risk of death and to identify preventable indicators. Since the recent advancements in machine learning techniques, the magnitude of variables and indicators that can be processed is largely enriched. Taken together, advanced machine learning algorithm allows us to establish a more optimal model that performed better in comparison to the conventional generalized linear models. By applying such models, physicians, and care givers could be alerted by the time when ICU patients are complicated with postoperative sepsis, thereby employing efficient yet personalized therapeutic strategies. Although the effectiveness of the XGBoost model had been validated in our study, the model was based on a single center retrospective database. Thus, further prospective cohort studies are required to evaluate the uniformity of this model.
Our results revealed that complication of coagulopathy and coagulation profile at ICU admission, including platelet counts, PT, APTT, and INR, were associated with increased in-hospital death among patients with postoperative sepsis. The occurrence of coagulopathy was commonly seen in septic patients, which was closely related to organ dysfunction and poor outcomes (25, 26). The activation of monocytes and endothelial cells was mainly characterized in the early phase of sepsis and resulted in massive exposure to tissue factors, thereby contributing to the over activation of coagulation and subsequent thrombin generation (27). Concomitantly, anticoagulant pathways, such as protein C system, were impaired by overexpression of proinflammatory cytokines (27). The imbalance between coagulation and anticoagulant pathways can be further augmented by surgical insults, and it leads to the upregulation of plasminogen activator inhibitor and subsequent hyperfibrinolysis (28, 29). Coagulation abnormalities have been reported to induce formation of microvascular clots and disseminated intravascular coagulation (DIC), further resulting in tissue ischemia and organ dysfunction (30, 31). Of note, majority of patients in our study had been exposed with cardiovascular surgery. Some of those patients might frequently receive anticoagulant agents, which could add to coagulation abnormalities. Early implementation of rotational thromboelastometry (ROTEM) and thrombelastography (TEG) appears to be beneficial for patients with postoperative sepsis who are at high risk of death (32, 33). As documented in large randomized controlled trials (RCTs), the administration of either antithrombin III or human recombinant thrombomodulin could improve short-term mortality among septic patients, but no trails specifically targeted patients with postoperative sepsis (34, 35). The results of our study suggested that secondary analyses of previously published RCTs and future large trails were both favorable for better recognition and treatment of septic patients following major operations. In addition, our models identified that fluid-electrolyte disturbance, sodium and chloride levels were associated with the in-hospital mortality, which could be explained by the deteriorative effects of acidosis on fibrin polymerization and clot integrity (28, 36).
From the present observation, we noticed that ICU patients underwent neurosurgery showed the highest in-hospital mortality compared to those with other types of surgery. Meanwhile, it revealed that neurosurgery was a robust predictor of in-hospital death among patients with postoperative sepsis. Neurosurgical procedures might bring about severe complications, including intracerebral hemorrhage, brain edema, and cerebral ischemia, which showed serious impacts on clinical outcomes of neurosurgical patients (37). Furthermore, neurosurgical insults could affect hypothalamic-pituitary-adrenal axis and hormonal generation, resulting in intractable immunosuppression (38). Therefore, well-performed neurocritical care is warranted for neurosurgical patients, especially for those with postoperative sepsis (39).
Limitations
There are some limitations to our study. Firstly, the current study was a single center retrospective analysis using publicly available database, which restricted us from identifying the causal relationship between variables and endpoints. Thus, prospective cohorts are needed for further validation. Secondly, there were several potential confounding variables that were unable to be assessed due to severe data missing and other reasons. However, some of the excluded variables might have predictive value for clinical outcomes. Thirdly, we employed XGBoost model, a machine learning-based algorithm that was not widely applied in clinical research. Although XGBoost had a significantly higher accuracy in predicting outcomes compared to generalized linear models, overfitting problem was inevitable. Given that, external validation was required to test its utility. Meanwhile, algorithm used other boosting strategy like Adaptive boosting (AdaBoost) were not tested in the current study, which might prevent us from developing more efficient model for predicting our endpoint. Finally, our study merely focused on the in-hospital mortality of patients with postoperative sepsis, while other outcomes, such as long-term mortality and readmission rates, were also important and needed further investigation.
Conclusions
In summary, these results suggest that some important features are potentially related to the in-hospital mortality among ICU patients with postoperative sepsis. The XGBoost model is capable of processing large amount of variables and further capturing these complicated relationships, which indeed performs better in mortality prediction compared to stepwise logistic model. Further validation of our model in external datasets can prompt us to early recognize patients with postoperative sepsis who are at high risk of death during hospitalization, and to implement timely yet efficient treatments.
Data Availability Statement
Publicly available datasets were analyzed in this study. This data can be found here: https://mimic.physionet.org.
Author Contributions
YYa, CR, and ZX conceived the analysis. RY and XJ extracted all data. XJ, YYu, and GWu undertook and refined the inclusion process. RY, CR, and YYu co-wrote the paper. RY, GWa, YZ, and LL undertook the statistical analyses. YL, PZ, and SZ were consulted for clinical issues. All authors contributed to and revised the final manuscript.
Funding
This work was supported by grants from the National Natural Science Foundation of China (Nos. 81730057, 81801935, and 81930057), the Key Project of Military Medical Innovation Program of Chinese PLA (No. 18CXZ026), and the National Key Research and Development Program of China (No. 2017YFC1103302).
Conflict of Interest
The authors declare that the research was conducted in the absence of any commercial or financial relationships that could be construed as a potential conflict of interest.
Acknowledgments
This manuscript has been released as a pre-print at [Research Square], Yao et al. (40).
Supplementary Material
The Supplementary Material for this article can be found online at: https://www.frontiersin.org/articles/10.3389/fmed.2020.00445/full#supplementary-material
Supplemental Figure S1. The process of tuning hyperparameters for XGBoost model.
References
1. Angus DC, Linde-Zwirble WT, Lidicker J, Clermont G, Carcillo J, Pinsky MR. Epidemiology of severe sepsis in the United States: analysis of incidence, outcome, and associated costs of care. Crit Care Med. (2001) 29:1303–10. doi: 10.1097/00003246-200107000-00002
2. Zafar Iqbal-Mirza S, Ciardo P, Julián-Jiménez A. Considerations on the epidemiology of community-acquired severe sepsis. Med Clin. (2017) 148:e3. doi: 10.1016/j.medcli.2016.09.025
3. Cecconi M, Evans L, Levy M, Rhodes A. Sepsis and septic shock. Lancet. (2018) 392:75–87. doi: 10.1016/S0140-6736(18)30696-2
5. Fried E, Weissman C, Sprung C. Postoperative sepsis. Curr Opin Crit Care. (2011) 17:396–401. doi: 10.1097/MCC.0b013e328348bee2
6. Rudd KE, Johnson SC, Agesa KM, Shackelford KA, Tsoi D, Kievlan DR, et al. Global, regional, and national sepsis incidence and mortality, 1990-2017: analysis for the Global Burden of Disease Study. Lancet. (2020) 395:200–11. doi: 10.1016/s0140-6736(19)32989-7
7. Bonventre JV, Yang L. Cellular pathophysiology of ischemic acute kidney injury. J Clin Invest. (2011) 121:4210–21. doi: 10.1172/jci45161
8. Ou L, Chen J, Hillman K, Flabouris A, Parr M, Assareh H, et al. The impact of post-operative sepsis on mortality after hospital discharge among elective surgical patients: a population-based cohort study. Crit Care. (2017) 21:34–34. doi: 10.1186/s13054-016-1596-7
9. Windsor AC, Klava A, Somers SS, Guillou PJ, Reynolds JV. Manipulation of local and systemic host defence in the prevention of perioperative sepsis. Br J Surg. (1995) 82:1460–7. doi: 10.1002/bjs.1800821106
10. Toft P, Dagnaes-Hansen F, Tønnesen E, Petersen MS. Influence of surgery and endotoxin-induced sepsis combined on natural killer cell activity, oxidative burst of granulocytes and antigen presentation capability of monocytes. Acta Anaesthesiol Scand. (2002) 46:405–10. doi: 10.1034/j.1399-6576.2002.460413.x
11. Angele MK, Chaudry IH. Surgical trauma and immunosuppression: pathophysiology and potential immunomodulatory approaches. Langenbecks Arch Surg. (2005) 390:333–41. doi: 10.1007/s00423-005-0557-4
12. Monneret G, Lepape A, Voirin N, Bohé J, Venet F, Debard AL, et al. Persisting low monocyte human leukocyte antigen-DR expression predicts mortality in septic shock. Intensive Care Med. (2006) 32:1175–83. doi: 10.1007/s00134-006-0204-8
13. Hinrichs C, Kotsch K, Buchwald S, Habicher M, Saak N, Gerlach H, et al. Perioperative gene expression analysis for prediction of postoperative sepsis. Clin Chem. (2010) 56:613–22. doi: 10.1373/clinchem.2009.133876
14. Rhodes A, Evans LE, Alhazzani W, Levy MM, Antonelli M, Ferrer R, et al. Surviving sepsis campaign: international guidelines for management of sepsis and septic shock: 2016. Intensive Care Med. (2017) 43:304–77. doi: 10.1007/s00134-017-4683-6
15. Ou L, Chen J, Flabouris A, Hillman K, Parr M, Bellomo R. Hospital variability of postoperative sepsis and sepsis-related mortality after elective coronary artery bypass grafting surgery. J Crit Care. (2018) 47:232–7. doi: 10.1016/j.jcrc.2018.07.018
16. Gabriel V, Grigorian A, Nahmias J, Pejcinovska M, Smith M, Sun B, et al. Risk factors for post-operative sepsis and septic shock in patients undergoing emergency surgery. Surg Infect. (2019) 20:367–72. doi: 10.1089/sur.2018.186
17. Johnson AEW, Pollard TJ, Shen L, Lehman L-WH, Feng M, Ghassemi M, et al. MIMIC-III, a freely accessible critical care database. Sci Data. (2016) 3:160035. doi: 10.1038/sdata.2016.35
18. Collins GS, Reitsma JB, Altman DG, Moons KGM. Transparent reporting of a multivariable prediction model for individual prognosis or diagnosis (TRIPOD): the TRIPOD statement. BMJ. (2015) 350:g7594. doi: 10.1136/bmj.g7594
19. Singer M, Deutschman CS, Seymour CW, Shankar-Hari M, Annane D, Bauer M, et al. The third international consensus definitions for sepsis and septic shock (sepsis-3). JAMA. (2016) 315:801–10. doi: 10.1001/jama.2016.0287
20. Zhang Z, Ho KM, Hong Y. Machine learning for the prediction of volume responsiveness in patients with oliguric acute kidney injury in critical care. Crit Care. (2019) 23:112–112. doi: 10.1186/s13054-019-2411-z
21. Zhang Z, Zhao Y, Canes A, Steinberg D, Lyashevska O, written on behalf of AME Big-Data Clinical Trial Collaborative Group. Predictive analytics with gradient boosting in clinical medicine. Ann. Transl. Med. (2019) 7:152. doi: 10.21037/atm.2019.03.29
22. Kilic A, Goyal A, Miller JK, Gjekmarkaj E, Tam WL, Gleason TG, et al. Predictive utility of a machine learning algorithm in estimating mortality risk in cardiac surgery. Ann Thor Surg. (2019) 109:1811–9. doi: 10.1016/j.athoracsur.2019.09.049
23. Bateman BT, Schmidt U, Berman MF, Bittner EA. Temporal trends in the epidemiology of severe postoperative sepsis after elective surgery: a large, nationwide sample. Anesthesiology. (2010) 112:917–25. doi: 10.1097/ALN.0b013e3181cea3d0
24. Mørch SS, Tantholdt-Hansen S, Pedersen NE, Duus CL, Petersen JA, Andersen CØ, et al. The association between pre-operative sepsis and 30-day mortality in hip fracture patients-A cohort study. Acta Anaesthesiol Scand. (2018) 62:1209–14. doi: 10.1111/aas.13160
25. Moore HB, Winfield RD, Aibiki M, Neal MD. Is coagulopathy an appropriate therapeutic target during critical illness such as trauma or sepsis? Shock. (2017) 48:159–67. doi: 10.1097/SHK.0000000000000854
26. Lyons PG, Micek ST, Hampton N, Kollef MH. Sepsis-associated coagulopathy severity predicts hospital mortality. Crit Care Med. (2018) 46:736–42. doi: 10.1097/CCM.0000000000002997
27. Levi M, van der Poll T. Coagulation and sepsis. Thromb Res. (2017) 149:38–44. doi: 10.1016/j.thromres.2016.11.007
28. Kozek-Langenecker SA. Coagulation and transfusion in the postoperative bleeding patient. Curr Opin Crit Care. (2014) 20:460–6. doi: 10.1097/MCC.0000000000000109
29. Meyer ASP, Meyer MAS, Sørensen AM, Rasmussen LS, Hansen MB, Holcomb JB, et al. Thrombelastography and rotational thromboelastometry early amplitudes in 182 trauma patients with clinical suspicion of severe injury. J Trauma Acute Care Surg. (2014) 76:682–90. doi: 10.1097/TA.0000000000000134
30. Ranieri VM, Thompson BT, Barie PS, Dhainaut J-F, Douglas IS, Finfer S, et al. Drotrecogin alfa (activated) in adults with septic shock. N Engl J Med. (2012) 366:2055–64. doi: 10.1056/NEJMoa1202290
31. Boral BM, Williams DJ, Boral LI. Disseminated intravascular coagulation. Am J Clin Pathol. (2016) 146:670–80. doi: 10.1093/ajcp/aqw195
32. Stensballe J, Ostrowski SR, Johansson PI. Viscoelastic guidance of resuscitation. Curr Opin Anaesthesiol. (2014) 27:212–8. doi: 10.1097/ACO.0000000000000051
33. Wikkelsø A, Wetterslev J, Møller AM, Afshari A. Thromboelastography (TEG) or thromboelastometry (ROTEM) to monitor haemostatic treatment versus usual care in adults or children with bleeding. Cochr Database Syst Rev. (2016) 2016:CD007871. doi: 10.1002/14651858.CD007871.pub3
34. Warren BL, Eid A, Singer P, Pillay SS, Carl P, Novak I, et al. Caring for the critically ill patient. High-dose antithrombin III in severe sepsis: a randomized controlled trial. JAMA. (2001) 286:1869–78. doi: 10.1001/jama.286.15.1869
35. Vincent J-L, Francois B, Zabolotskikh I, Daga MK, Lascarrou J-B, Kirov MY, et al. Effect of a recombinant human soluble thrombomodulin on mortality in patients with sepsis-associated coagulopathy: the SCARLET randomized clinical trial. JAMA. (2019) 321:1993–2002. doi: 10.1001/jama.2019.5358
36. Quaknine-Orlando B, Samama CM, Riou B, Bonnin P, Guillosson JJ, Beaumont JL, et al. Role of the hematocrit in a rabbit model of arterial thrombosis and bleeding. Anesthesiology. (1999) 90:1454–61. doi: 10.1097/00000542-199905000-00031
37. Adam EH, Füllenbach C, Lindau S, Konczalla J. Point-of-care coagulation testing in neurosurgery. Anasthesiol Intensivmed Notfallmed Schmerzther. (2018) 53:425–39. doi: 10.1055/s-0043-107754
38. Desborough JP. The stress response to trauma and surgery. Br J Anaesthesia. (2000) 85:109–17. doi: 10.1093/bja/85.1.109
39. Morris NA, Czeisler BM, Sarwal A. Simulation in neurocritical care: past, present, and future. Neurocrit Care. (2019) 30:522–33. doi: 10.1007/s12028-018-0629-2
Keywords: postoperative sepsis, intensive care unit, extreme gradient boosting, coagulation, prediction
Citation: Yao R, Jin X, Wang G, Yu Y, Wu G, Zhu Y, Li L, Li Y, Zhao P, Zhu S, Xia Z, Ren C and Yao Y (2020) A Machine Learning-Based Prediction of Hospital Mortality in Patients With Postoperative Sepsis. Front. Med. 7:445. doi: 10.3389/fmed.2020.00445
Received: 07 April 2020; Accepted: 06 July 2020;
Published: 11 August 2020.
Edited by:
Andrey V. Kozlov, Institute for Experimental and Clinical Traumatology (LBG), AustriaReviewed by:
Marcin Filip Osuchowski, Ludwig Boltzmann Institute for Experimental and Clinical Traumatology, AustriaZhongheng Zhang, Sir Run Run Shaw Hospital, China
Copyright © 2020 Yao, Jin, Wang, Yu, Wu, Zhu, Li, Li, Zhao, Zhu, Xia, Ren and Yao. This is an open-access article distributed under the terms of the Creative Commons Attribution License (CC BY). The use, distribution or reproduction in other forums is permitted, provided the original author(s) and the copyright owner(s) are credited and that the original publication in this journal is cited, in accordance with accepted academic practice. No use, distribution or reproduction is permitted which does not comply with these terms.
*Correspondence: Chao Ren, rc198@sina.com; Yong-ming Yao, c_ff@sina.com
†These authors have contributed equally to this work