- 1Rheumatology Department, Hospital Universitario La Princesa, Instituto de Investigación Sanitaria - Instituto Princesa (IIS-IP), Madrid, Spain
- 2Rheumatology Department, Hospital Universitario y Politécnico La Fé, Valencia, Spain
- 3Rheumatology Department, Hospital Clínico San Carlos, Instituto de Investigación Sanitaria San Carlos (IdiSSC), Madrid, Spain
- 4Rheumatology Department, Hospital General Universitario Gregorio Marañón, Madrid, Spain
- 5Instituto de Investigación Músculo-Esquelética (InMusc), Madrid, Spain
Objective: To compare the capacity of various disease activity indices to evaluate changes in function, IL-6 levels, and radiographic progression in early and established rheumatoid arthritis (RA).
Methods: Secondary data analysis of a clinical trial assessing the efficacy of tocilizumab in patients with established RA (ACT-RAY) and a longitudinal prospective register of early arthritis (PEARL). Targeted outcomes were changes in physical function, measured with the health assessment questionnaire (HAQ), IL-6 serum levels, and radiographic progression. The “Hospital Universitario La Princesa Index” (HUPI), DAS28 using erythrocyte sedimentation rate and SDAI were the disease activity indices compared. Models adjusted for age and sex were fitted for each outcome and index and ranked based on the R2 parameter and the quasi-likelihood under the independence model criterion.
Results: Data from 8,090 visits (550 patients) from ACT-RAY and 775 visits (534 patients) from PEARL were analyzed. The best performing models for HAQ were the HUPI (R2 = 0.351) and SDAI ones (R2 = 0.329). For serum IL-6 levels, the SDAI (R2 = 0.208) followed by the HUPI model (R2 = 0.205). For radiographic progression in ACT-RAY, the HUPI (R2 = 0.034) and the DAS28 models (R2 = 0.026) performed best whereas the DAS28 (R2 = 0.030) and HUPI models (R2 = 0.023) did so in PEARL.
Conclusions: HUPI outperformed other indices identifying changes in HAQ and radiographic progression and performed similarly to SDAI for IL-6 serum levels.
Introduction
Routine management of rheumatoid arthritis (RA) using the treat-to-target (1) and tight-control (2) strategies require validated tools to measure disease activity. The most frequently used measures in randomized clinical trials (RCT) are the disease activity score of 28 joints (DAS28) (3), the simplified disease activity index (SDAI) (4), and the clinical disease activity index (CDAI) (5). Although extensively validated, these indices exhibit some limitations. Different cohorts have shown that DAS28 and SDAI may be sex-biased, as they include a pain rating and erythrosedimentation rate (ESR), both usually higher in women. This potential bias could lead rheumatologists to over-treat women with RA (6, 7).
To overcome these limitations, the “Hospital Universitario La Princesa Index” (HUPI), was developed and validated (8–10). HUPI includes the same variables as DAS28 but its calculation can be done either with ESR, C-Reactive Protein (CRP), or both, depending on their availability, as a way to tackle missing data (8). This index developed and validated in an early arthritis cohort (8), has disease activity cut-offs with higher areas under the curve in comparison to DAS28, SDAI, and CDAI (9). HUPI's responsiveness was evaluated against the other disease activity indices in three different scenarios, namely an RCT and two different RA cohorts, including patients with early and established disease. The responsiveness was similar to that of DAS28-CRP and better than the remaining indices with response criteria that are more stringent than those of EULAR (10). Based on its psychometric properties, the 2019 update of the American College of Rheumatology recommended RA disease activity measures included HUPI among the indices that fulfil minimum standards for regular use in most clinical settings (11).
Nowadays, the importance of an early diagnosis and treatment in patients with RA is well-established (12, 13). However, to offer patients a tailored therapy aimed at improving efficacy and reducing side effects, we need reliable measures of what is happening now (assessment) and what will happen in the future (prediction). Accordingly, we hypothesized that HUPI's performance to identify unbiased changes in disease activity makes it more suitable to assess changes in relevant outcomes and surrogates of inflammation (14, 15). The objective of this study was to compare the capacity of HUPI and other indices to identify changes in (i) physical function, (ii) serum levels of interleukin-6 (IL-6), and (iii) radiographic progression in patients with early and established RA.
Methods
This study is a secondary data analysis of an early arthritis cohort and an RCT in established RA.
Study Population
The ACT-Ray Trial
The main characteristics of the ACT-RAY trial have been previously reported (16). In summary, this is a 3-year double-blind RCT designed to evaluate the efficacy and safety of tocilizumab (TCZ) plus methotrexate vs. TCZ monotherapy in patients with established RA with inadequate response to methotrexate. The study included patients fulfilling the ACR 1987 criteria with a DAS28 > 4.4 and erosive disease. Data on demographics, disease activity variables, and laboratory data were collected every 4 weeks from baseline until the end of the study. Since there were no statistically significant differences in clinical response between arms, we included all patients' data regardless of the allocation group up to week 52 when, according to the protocol, patients in sustained remission discontinued treatment with TCZ (16).
The PEARL Cohort
This prospective cohort has been previously described (9). In summary, PEARL includes incident cases of early arthritis, with one or more swollen joints for less than a year. Patients are referred by their treating rheumatologist to an early arthritis clinic, in which patients undergo 5 visits (at baseline, 26, 52, 104, and 260 weeks) per protocol performed by the same two rheumatologists, which guarantees consistency in clinical examination, particularly joint counts.
Demographics, disease activity measures, and radiological data are routinely recorded in standardized forms. In addition, biological samples are systematically collected. Patients are treated according to their treating rheumatologist's criteria.
For the present study, we included patients either meeting the 1987 ACR criteria for RA (17) or classified as having UA (18) at the 24-month follow-up visit, from cohort inception (2000) until June 2019.
Variables
Physical function: It was measured through the Health Assessment Questionnaire-Disability Index (HAQ) in both datasets. This self-reported questionnaire was administered at every follow-up visit using cross-cultural validated versions (19, 20).
Serum IL-6 Levels (pg/ml)
IL-6 had been previously measured in frozen serum samples from PEARL patients using an enzyme-linked immunoassay (Quantikine®HS ELISA, R&D Systems®) according to the manufacturer's instructions as previously described (21). The biobank of La Princesa University Hospital—Health Research Institute (ISS-IP) provided serum for this previous study. In the present work, we have used these previous serum IL-6 measurements as a surrogate for inflammation in the PEARL study, to analyze their relationship with the different indices studied.
IL-6 was measured as a surrogate for inflammation (14) only in the PEARL study, using an enzyme-linked immunoassay (Quantikine®HS ELISA, R&D Systems®) according to the manufacturer's instructions.
Radiographic Progression
Plain X-rays were available to measure radiographic progression using the Genant-Sharp score in ACT-RAY (22) and the modified-Sharp-Van der Heijde score (23) (applied only in hands) in PEARL. We analyzed only the Δ of erosions because we consider it more accurate to show changes only due to RA, as opposed to measuring changes in joint space narrowing that have been shown to be strongly associated with age, rather than disease activity (24). The variable Δ of erosions was calculated as the difference in the respective scores between baseline and the 52-week visit for ACT-RAY and the 104-week follow-up visit for PEARL.
Disease activity indices included DAS28-ESR, SDAI, and HUPI and were calculated as follows:
1. DAS28-ESR = 0.56*√(TJC28) + 0.28*√(SJC28) + 0.70* ln(ESR) + 0.014*(GDAPat). TJC28 and SJC28 refer to the count of tender and swollen joints in 28 joints while GDAPat does so for the patients' global disease assessment (25).
2. SDAI = TJC28 + SJC28 + CRP + GDAPat + GDAPhy. The latter refers to the physicians' global disease assessment (5).
3. HUPI is calculated as the sum of four variables (graded 0–3, see Supplementary Table 1): TJC28, SJC28, GDAPhy, and acute phase reactants (the average score value of ESR and CRP must be used if both are considered (9).
Categories of disease activity were established based on published cut-offs (5, 8, 25, 26).
Statistical Analysis
Data from each of the two studies were analyzed independently. Normally distributed variables were represented as mean and standard deviation (SD) and non-normally distributed variables as the median and interquartile range (IQR). Categorical variables were presented as numbers and proportions.
To assess the performance of HUPI, DAS28, and SDAI on explaining changes in the three mentioned outcomes, we developed models for each of them as dependent variable adjusting for known potential confounders, such as age and sex (27). Only patients without missing data in all of these variables were included for analysis. For all models, indices and age were standardized (centered and scaled by subtracting from each variable record the variable mean value and dividing the result by the standard deviation), thereby allowing comparisons.
Models with HAQ as a dependent variable were developed in ACT-RAY and fitted using Generalized Estimating Equations (GEE), nesting visits to each patient. An unstructured variance-covariance matrix for fixed and residual terms was used to avoid assumptions on the variance-covariance structure. Models were ranked according to the R2 parameter and the quasi-likelihood under the independence model criterion (QIC) (28). The model with the highest R2 and the lowest QIC was selected as the best-ranked one. This ranking was then validated in PEARL using the R2 parameter.
We used a similar approach to develop models for IL-6 serum levels as the dependent variable. As IL-6 levels were not collected in ACT-RAY, we used 80% of the PEARL population to establish the predicting model and the remaining 20% for its validation. This analysis was done with the R package “geepack” (29).
Finally, the models describing the relationship between Δ erosions and the different indices were developed independently for ACT-RAY and PEARL, because of the previously described differences in their measurement. For these models, we obtained the mean value of each disease activity index for the entire follow-up, rather than the score at every visit, as done in the previous models. These mean values were categorized as follows: remission = 0, low = 1, moderate = 2 and high activity = 3, according to their respective cut-offs (3). Models were ranked by the R2 parameter (R package stats) and the AIC (Akaike's Information Criterion) (30, 31), being the one with the highest R2 and the lowest AIC selected as the best-ranked model. The relative importance of each predictor was calculated by decomposing the R2 value of the model into components corresponding to each predictor (R package r2glmm) (32). Linear models were used to analyze HAQ and IL-6 and quadratic ones for radiographic progression due to better data adjustment. Statistical analyses were conducted using R version 3.6.3 (27).
Ethical Considerations
This is a secondary analysis of anonymized data from patients included in the ACT-RAY and PEARL studies. The ACT-RAY trial was approved by the Ethics committees of each participant center (see Acknowledgement section “ACT-RAY group”) and the PEARL study was approved by the Ethics Committee for Clinical Research at the Hospital Universitario de La Princesa (PI-518; March 28th, 2011). All patients had signed a written consent form before inclusion. Both studies were conducted according to the principles of the Helsinki Declaration (33).
Results
The analysis included 8,090 visits from 550 patients in ACT-RAY and 775 visits from 534 patients in PEARL. Nonetheless, different numbers of visits/patients were assessed for each model, based on the availability of data for the involved variables (see further details in each table). Patients' demographic and clinical characteristics are presented in Supplementary Table 2. In the complete sample (n = 1,084), 80% of patients were women, and 29% current smokers. Patients in the RCT presented higher HAQ and disease activity at baseline than their counterparts in the early arthritis cohort.
Comparative Analysis of Indices With HAQ as Outcome
The model fitted to explain HAQ adding HUPI as a predictor presented the highest R2 (0.351) and the lowest QIC (1989.790) compared to the models using SDAI (R2: 0.329; QIC: 2057.011) and DAS28 (R2: 0.325; QIC: 2070.581) indicating that the former explained 35% of the HAQ variance, while the latter two indices explained ~33%. In the same line, the β coefficient for HUPI was 0.365 vs. 0.344 and 0.343 for DAS28 and SDAI, respectively. The R2 parameters of all models remained similar in the validation cohort. Other parameters of each model are shown in Table 1 and the distribution of HAQ according to HUPI, DAS28, and SDAI in both study populations are shown in Figure 1 and Supplementary Figure 1.
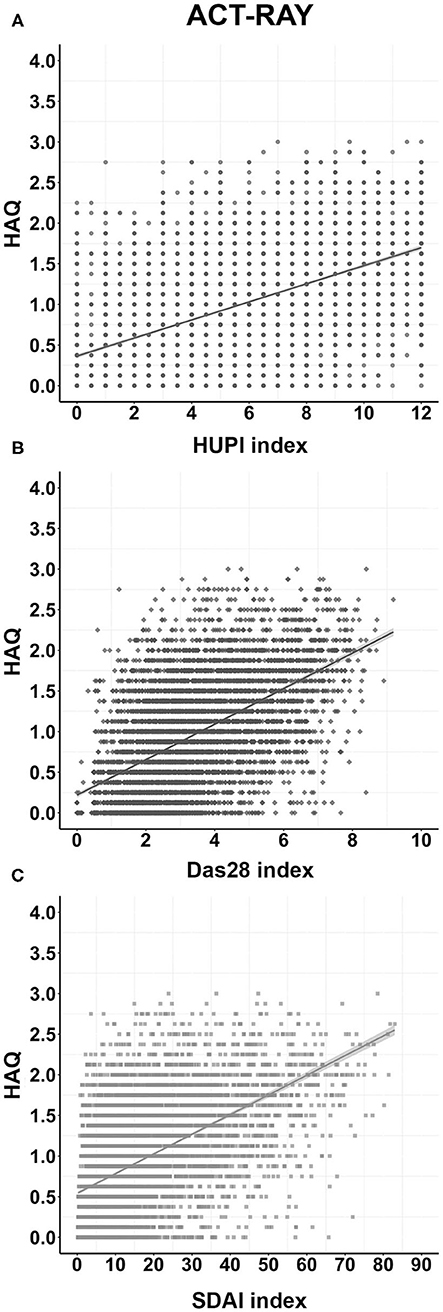
Figure 1. Distribution of HAQ according to each disease activity index in the ACT-RAY study through follow-up. (A), (B), and (C): Distributions according to the HUPI, DAS28, and SDAI index, respectively. Data are shown as dot-plots and their fitted linear prediction (line) with 95% confidence intervals (grey shadow).
Comparative Analysis of Indices With IL-6 Serum Levels as Outcome
Variations in the IL-6 serum level were initially modeled with a randomly split 80% of the PEARL population. In these initial models, IL-6 levels were better explained when including SDAI or HUPI as predictors, with R2 of 0.208 (QIC: 289.207) and 0.205 (QIC: 290.823), respectively, in comparison with an R2 of 0.190; QIC: 295.610 for DAS28 (Table 2). These results indicate that the former two explained ~21% of the variance, while the latter explained 19%. The β coefficient for SDAI was 0.363 vs. 0.345 and 0.337 for HUPI and DAS28, respectively. Of note, the R2 parameters of HUPI and DAS28 remained similar when applied to the validation cohort (the remaining 20% of the PEARL population), as opposed to the SDAI model, which changed from explaining ~21% in the initial population to 18% in the validation population (Table 2). It is also noteworthy that sex only reached significance in the DAS28 model. Additional data of the models are presented in Table 2 and the distribution of IL-6 serum levels according to each index scale in Figure 2.
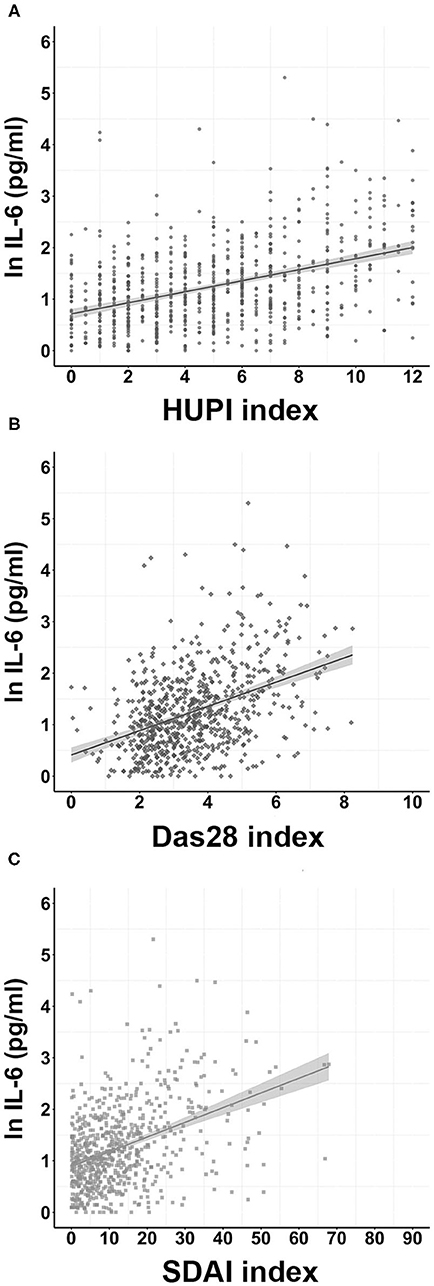
Figure 2. Distribution of IL-6 serum levels according to each disease activity index in the PEARL study through follow-up. (A), (B), and (C): Distributions according to the HUPI, DAS28, and SDAI index, respectively. IL-6 level values were transformed to their natural logarithm (nl). Data are shown as dot-plots and their fitted linear prediction (line) with 95% confidence intervals (grey shadow).
Comparative Analysis of Indices With Radiographic Progression as Outcome
As radiographic progression was evaluated using different methodologies in both studies, we ran separate comparative analyses. As shown in Table 3, when analyzing data from the ACT-RAY study, the model including HUPI as an explanatory variable showed the best performance (R2: 0.034; AIC:925.687), followed by the one with DAS28 (R2: 0.026; AIC: 928.793) and then the one using SDAI (R2: 0.017; AIC:932.347). These results indicate that the HUPI model explained slightly better the variance of Δ erosions (3.4%) than the models including DAS28 and SDAI (2.6 and 1.7%, respectively). When assessing partial R2 parameters for each explanatory variable, HUPI and HUPI2 explained 2% of the variance, while DAS28/DAS282 explained 1% and SDAI/SDAI2 0.2%. β coefficients for HUPI and HUPI2 were 1.472 and 1.632 vs. 1.675 and 0.266 for DAS28/DAS282 and 0.759 and −0.042 for SDAI/SDAI2, respectively. Additional data are shown in Table 3.
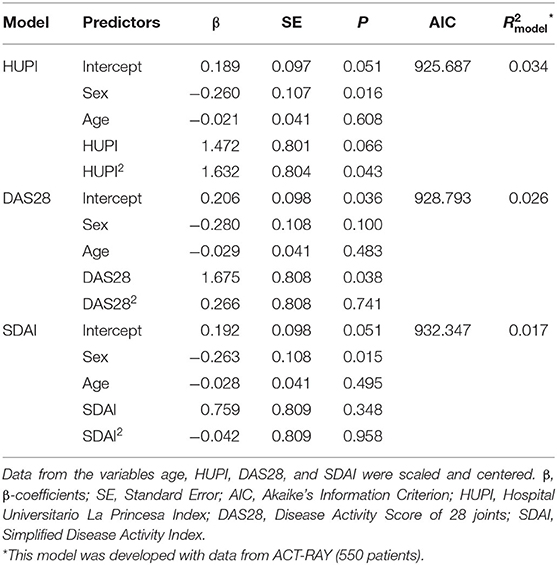
Table 3. Models for radiographic progression in the ACT-RAY study comparing the performance of different indices.
In contrast, when using data from PEARL, none of the models were associated with radiographic progression. Results were R2: 0.030 (0.010–0.150) AIC: 347.520) for DAS28, R2: 0.023 (0.008–0.138) AIC: 348.413 for HUPI, and R2: 0.018 (0.007–0.131) AIC: 348.955 for SDAI. The model including DAS28 explained ~3% of the variance, while those with HUPI and SDAI explained 2.3% and 1.8%, respectively. Partial R2 parameters show that DAS28/DAS282 explained 2.5% of the overall variance, while HUPI and SDAI explained 1.7 and 1.3%, respectively. Additional details are shown in Supplementary Table 3. The distribution of the variable Δ erosions in ACT-RAY and PEARL according to the different categories of each index is shown in Figure 3 and Supplementary Figure 2, respectively.
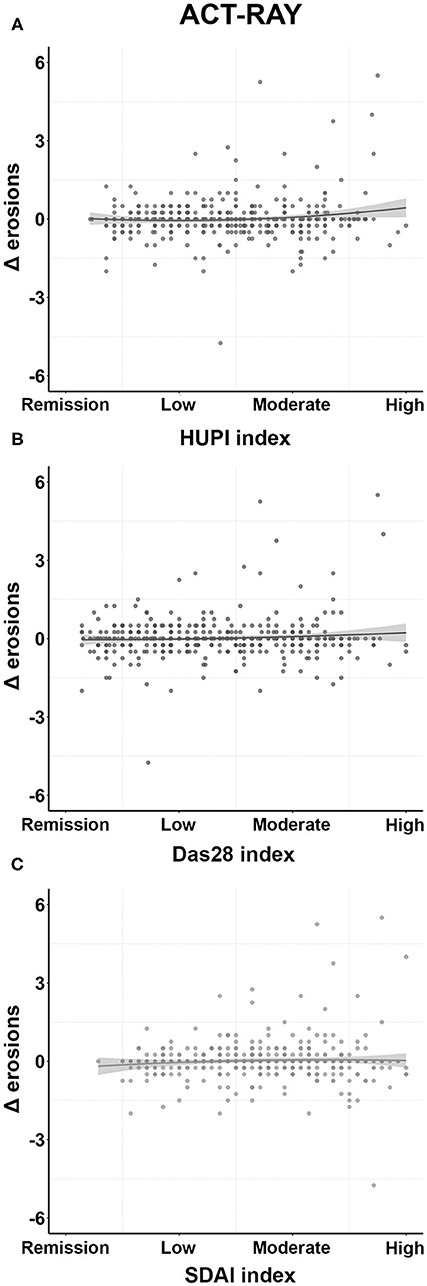
Figure 3. Distribution of Δ erosions according to the different disease activity indices in the ACT-RAY study. (A), (B), and (C): Distributions according to HUPI, DAS28, and SDAI categories, respectively. Disease activity values represent patients' mean disease activity through follow-up. Data are shown as dot-plots and their fitted linear prediction (line) with 95% confidence intervals (grey shadow).
Discussion
In this study, we evaluated the performance of HUPI in comparison to other traditional disease activity indices as explanatory variables for physical function decline measured by HAQ, inflammation, assessed by IL-6 serum levels, and radiographic progression measured by Δ erosions. Our results indicate that HUPI performed well with most outcomes studied, being the best in explaining the decline in physical function and radiographic progression (ACT-RAY) and second-best for IL-6 serum levels. Of note, all indices performed poorly with regard to radiographic progression, mainly because both populations showed modest changes in their respective radiographic scores, as expected for early diagnosed, intensively treated patients.
Even though the models containing HUPI did not outperform their counterparts in all comparisons, they were the most consistent in the different proposed scenarios. The SDAI models performed best for IL-6 changes, probably because the weight of CRP is high in SDAI but were the last ranked for Δ erosions. Similarly, DAS28 models worked best for explaining Δ erosions in PEARL but rated the worst for HAQ and IL-6.
The association between HAQ and traditional indices (DAS28, SDAI, and CDAI) has been previously analyzed in a study by Aletaha et al. (34) with two observational cohorts, one including patients with established RA, and another with early arthritis. These analyses showed moderate and similar correlations for all indices (r = 0.45–0.47) for the former, and weaker for the latter cohort (r = 0.26–0.31). Another study pooling data from three RCTs showed moderate to good correlations with HAQ for SDAI and CDAI at baseline and after 6 months of follow-up (r = 0.36–0.66) (4). These observations are consistent with our results: SDAI and DAS28 performed quite similarly on HAQ assessment. Nonetheless, our data support a slight superiority of HUPI.
The association between indices and IL-6 levels has also been previously analyzed in a study by Madhok et al. (35) showing a weak correlation (r = 0.3) with the Ritchie Activity Index. In our study, initial models including all three indices performed similarly, with little differences favoring those including SDAI (with R2 parameters ranging from 0.190 to 0.208). Notably, when validating these models with the 20% remaining data from PEARL, HUPI and DAS28 models performed better than SDAI.
Navarro-Compán et al. (36) summarized the association between disease activity indices and radiographic progression in a systematic review. The majority of studies reported a significant association, especially after adjustment by time. However, this review did not carry out comparative analyses between indices.
Aletaha et al. (34) assessed the linear correlation between time-averaged DAS28, SDAI, and CDAI and radiographic progression (measured with the Larsen score) after 3 years of follow-up, and found similar moderate correlations, with r coefficients ranging from 0.54 to 0.59. Of note, in this study, no GEE modeling was carried out. Klarenbeek et al. (37) using 5-year data from the BeST study, found similar results after assessing the association of different indices with radiographic progression, using the Sharp-van der Heijde score, and HAQ. These authors ran GEE models to analyze different scenarios for both outcomes and found that all associations were highly comparable. Despite the limited radiographic progression in ACT-RAY and PEARL, our results are in line with those previously described in the literature, favoring HUPI's performance to explain radiographic progression.
Our study has strengths, such as a study population including patients with both early and established RA, as well as a thorough statistical analysis. Nonetheless, it also presents some limitations, the most important being the low radiographic progression observed in both cohorts, which might have affected the performance of the three disease activity indices. This prevented us from establishing firm conclusions from the comparative analysis. Another limitation is the fact that IL-6 serum levels were only available from PEARL, something that limited the number of visits/patients assessed.
In conclusion, HUPI exhibits a slightly superior performance to identify physical function declines and radiographic progression than DAS28 and SDAI and detects changes in IL-6 serum levels similar to the other indices. This behavior is consistent in early and established RA. These new findings, in addition to the absence of sex bias and the possibility of its calculation either with CRP or ESR, reinforce the role of HUPI for research purposes.
Data Availability Statement
The data analyzed in this study is subject to the following licenses/restrictions: Data from the PEARL study can be requested to the corresponding author. Data from ACT-RAY were provided by Hoffmann-La Roche Ltd through a data-sharing agreement that does not allow for the public sharing of these data. The authors did not enjoy any special access privileges in gaining access to these data. Regarding the possibility that any other researcher would like to request data to replicate the reported study findings, Hoffmann-La Roche Ltd has implemented a Data Sharing policy to align with the ICMJE recommendations: “Qualified researchers may request access to individual patient-level data through the clinical study data request platform (www.clinicalstudydatarequest.com). Further details on Roche's criteria for eligible studies are available in https://clinicalstudydatarequest.com/Study-Sponsors/Study-Sponsors-Roche.aspx. For further details on Roche's Global Policy on the Sharing of Clinical Information and how to request access to related clinical study documents, see https://www.roche.com/research_and_development/who_we_are_how_we_work/clinical_trials/our_commitment_to_data_sharing.html”. Requests to access these datasets should be directed to ACT-RAY data: www.clinicalstudydatarequest.com. PEARL data: Isidoro Gonzalez-Alvaro, aXNpZG9yby5nYUBzZXI=.
Ethics Statement
This is a secondary analysis of anonymized data from patients included in the ACT-RAY and PEARL studies. The ACT-RAY trial was approved by the Ethics committees of each participant center and the PEARL study was approved by the Ethics Committee for Clinical Research at the Hospital Universitario de La Princesa (PI-518; March 28th, 2011). Both studies were conducted according to the principles of the Helsinki Declaration. The patients/participants provided their written informed consent to participate.
Author Contributions
IG-Á, SR-G, and NM contributed to conception and design of the study and organized the database. NM performed the statistical analysis and wrote a section of the manuscript. SR-G wrote the first draft of the manuscript. All authors contributed to manuscript revision, read, and approved the submitted version.
Funding
This study was funded by the Instituto de Salud Carlos III through the grant PI18/00371 and the RETICS Program RIER: RD16/0012/0004; RD16/0012/0011 (Co-funded by The European Regional Development Fund A way to make Europe). SR-G was funded by the Spanish Rheumatology Foundation (Grants for physician-researchers 2018–2021).
Conflict of Interest
The authors declare that the research was conducted in the absence of any commercial or financial relationships that could be construed as a potential conflict of interest.
Acknowledgments
The authors would like to thank Dr. Manuel Gomez-Gutierrez for his editing assistance.
The ACT-RAY Study Group
Lead authors: Dougados, M. CHU Paris Centre—Hôpital Cochin. France. E-mail: bWF4aW1lLmRvdWdhZG9zQGNjaC5hcC1ob3AtcGFyaXMuZnI=; Huizinga, T. Leiden University Medical Center, Netherlands. E-mail: VC5XLkouSHVpemluZ2FAbHVtYy5ubA==
Abu Shakra, M. Soroka Medical Center. Israel
Alberts, A. West Broward Rheumatology Associates, Inc. United States
Alperi Lopez, M. Hospital Univ. Central de Asturias. Spain
Amital, H. Chaim Sheba Medical Center. Israel
Aringer, M. Universitatsklinikum “Carl Gustav Carus”. Germany
Aslanidis, S. Hippokratio Hospital. Greece
Berenbaum, F. Hôpital Saint Antoine. France
Bijlsma, H. Academisch Medisch Centrum Utrecht. Netherlands
Blanco-Garcia, FJ. Complejo Hospitalario Universitario A Coruña. Spain
Bliddal, H. Frederiksberg Sygehus. Denmark
Borofsky, M. Clinical Research Center of Reading. United States
Brocq, O. Ch Princesse Grace. Monaco
Buldakov, S. Republican Clinicodiagnostic Center. Russian Federation
Cantini, F. Presidio Ospedaliero Misericordia e Dolce. Italy
Carreño-Perez, L. Hospital General Universitario Gregorio Marañon. Spain Chahade, W. Hospital Estadual do Servidor Publico. Brazil
Ciconelli, R. Universidade Federal de São Paulo. Brazil
Codreanu, C. Centrul de Boli Reumatismale Dr. Ioan Stoia. Romania
Dahlqvist, SR. Norrlands Universitary Hospital. Sweden
Damjanov, N. Institut Za Reumatologiju. Serbia
Diamantopoulos, A. Sørlandet Sykehus Kristiansand. Norway
Dimdina, L. Clinical University Hospital Gailezers. Latvia
Dimic, A. Institut Za Prevenciju, Lecenje I Rehabilitaciju. Serbia
Dorokhov, A. State Institution of Health Care—Territorial Clinical Hospital. Russian Federation
Dubikov, A. City Clinical Hospital # 2. Russian Federation
Fadienko, G. Glpu Tjumen Regional Clinical hospital #1. Russian Federation
Fanø, N. Sjællands Universitetshospital, Køge. Denmark
Ferreira, G. Hospital das Clinicas–UFMG. Brazil
Gabrielli, A. Uni Politecnica Delle Marche; Ist. Di Clinica Medica Generale Ematologia Ed Immunologia Clinica. Italy
Gaffney, K. Norfolk & Norwich Hospital. United Kingdom
Gaudin, P. Hopital Sud. France
Gerlag, DM. Academisch Medisch Centrum. Netherlands
Gerli, R. Osp S. Maria Misericordia Dip. Italy
Gonçalves, CR. Hospital das Clínicas–FMUSP. Brazil
Hansen, MS. Gentofte Hospital. Denmark
Hanvivadhanakul, P. Thammasat University Hospital. Thailand
Høili, C. Sykehuset Ostfold Moss HF. Norway
Hou, A. Inland Rheumatology; Clinical Trials, Inc. United States
Hunter, J. Gartnavel General Hospital. United Kingdom
Ilic, T. Clinical Centre of Vojvodina. Serbia
Ionescu, R. Spitalul Sf Maria. Romania
Kaine, J. Sarasota Arthritis Center. United States
Kakurina, N. Clinical Hospital of Daugavpils. Latvia
Kamalova, R. Republican clinical hospital. Russian Federation
Kelly, T. Innovative Health Research. United States
Knyazeva, L. GMU Kursk Regional Clinical Hospital. Russian Krumina Federation, L. L.Krumina GP practice. Latvia
Kurthen, R. Praxis Dr. med. Reiner Kurthen. Germany
Lagrone, RP. St. Thomas Hospital. United States
Lapadula, G. Ospedale Policlinico Di.M.I.M.P. Italy
Lavrentjevs, V. P.Stradins Clinical University Hospital. Latvia
Lawson, JG. Piedmont Arthritis Clinic. United States
Lazic, Z. Clinical Center Kragujevac. Serbia
Lejnieks, A. Rakus Clinic Linezers. Latvia
Levy, Y. Meir Medical Center. Israel
Lexberg, Å. Drammen sykehus Vestre Viken HF. Norway
Mader, R. Haemek Hospital. Israel
Mariette, X. Ch De Bicêtre. France
Markovits, D. Rambam Medical Center. Israel
Martin Mola, E. Htal. La Paz. Spain
Maugars, Y. Hopital Hotel Dieu Et Hme. France
Maymo Guarch, J. Hospital del Mar. Spain
Mazurov, VI. Sbei Of Hpe “Northwestern State Medical University N.A. I.I.Mechnikov”. Russian Federation
Mikkelsen, K. Revmatismesykehuset. Norway
Morovic Vergles, J. Clinical Hospital Dubrava. Croatia
Nabizadeh, S. Martina Hansen Hospital. Norway
Nanagara, R. Khon Kaen University. Thailand
Nasonov, EL. Fsbi “Scientific Research Institute of Rheumatology” Of Russian Academy Of Medical Sciences. Russian Federation
Navarro-Sarabia, F. Hospital Universitario Virgen Macarena. Spain
Neumann, T. Universitatsklinikum Jena. Germany
Novak, S. Rheumatology and Clinical Immunology. Croatia
Olech, E. Oklahoma Medical Research Foundation. United States
Oza, M. Arthritis/Osteoporosis Treatment Center. United States
Paran, D. Sourasky / Ichilov Hospital. Israel
Parsik, E. North Estonian Regional Hospital. Estonia
Pegram, S. Rheumatic Disease Clin Res Ctr. United States
Pombo-Suarez, M. Hospital Nuestra Señora de la Esperanza. Spain
Popova, T. Municipal Autonomous Institution of Healthcare “City Clinical Hospital #40”. Russian Federation
Puechal, X. Ch Du Mans. France
Raja, N. Agilence Arthritis and Osteoporosis Medical Center, Inc. United States
Ridley, D. St. Paul Rheumatology. United States
Rosner, I. Bnei Zion Medical Center. Israel
Rubbert-Roth, A. Klinik der Uni zu Ko ln. Germany
Rudin, A. Sahlgrenska Universitetssjukhuset. Sweden
Saraux, A. Hôpital La Cavale Blanche. France
Saulite-Kandevica, D. D.Saulite-Kandevica Private Practice. Latvia
Settas, L. Ahepa Hospital. Greece
Sfikakis, P. Laiko General Hospital. Greece
Sheeran, T. Cannock Chase Hospital. United Kingdom
Sizikov, A. FSBI Scientific Research Institute of Clinical Immunology of SB of RAMS. Russian Federation
Stamenkovic, D. Clinical Hospital Centre Rijeka. Croatia Stefanovic, D. Military Medical Academy. Serbia
Stolow, JB. Texas Arthritis Research Center. United States
Tan, AL. Chapel Allerton Hospital. United Kingdom
Tebib, J. Ch Lyon Sud. France
Tishler, M. Assaf Harofe. Israel
Tony, HP. Universitatsklinikum Würzburg. Germany
Troum, OM. United States
Uaratanawong, S. Vajira Hospital. Thailand
Ucar Angulo, E. Hospital de Basurto. Spain
Valenzuela, G. Berma Research Group. United States
van der Laken, K. VU Medisch Centrum. Netherlands
Van Laar, J. School of Clinical Medical Services. United Kingdom
Van RIEL, P.L.C.M. Akademisch Ziekenhuis St. Radboud. Netherlands Vasilopoulos, D. Hippocrateio Hospital of Athens. Greece
Veldi, T. East Tallinn Central Hospital. Estonia
Vinogradova, I. State Institution of Healthcare Ulyanovsk Regional Clinical Hospital. Russian Federation
Vosse, D. Academisch Ziekenhuis Maastricht. Netherlands
Wassenberg, S. Evangelisches Fachkrankenhaus. Germany
Weidmann, C. Medvin Clinical Research. United States
Weitz, M. Center For Arthritis. United States
Wollenhaupt, J. Scho n Klinik Hamburg-Eilbek Klinik für Rheumatologie. Germany Xavier, R. Hospital das Clinicas–UFRGS. Brazil
Yakupova, S. Kazan State Medical University. Russian Federation
Zagar, I. Klinicki Bolnicki Centar Zagreb. Croatia
Zavgorodnaja, T. P.Stradins Clinical University Hospital. Latvia
Zemerova, E. Khanty-Mansiysk Autonomous Area—Ugri Region Clinical Hospital. Russian Federation
Zisman, D. Carmel Hospital. Israel
Zonova, E. FSBI Scientific Research Institute of Clinical and Experimental Lymphology of SB of RAMS. Russian Federa.
Supplementary Material
The Supplementary Material for this article can be found online at: https://www.frontiersin.org/articles/10.3389/fmed.2021.669688/full#supplementary-material
References
1. Smolen JS, Landewé RBM, Bijlsma JWJ, Burmester GR, Dougados M, Kerschbaumer A, et al. EULAR recommendations for the management of rheumatoid arthritis with synthetic and biological disease-modifying antirheumatic drugs: 2019 update. Ann Rheum Dis. (2020) 79:685–99. doi: 10.1136/annrheumdis-2019-216655
2. Grigor C, Capell H, Stirling A, McMahon AD, Lock P, Vallance R, et al. Effect of a treatment strategy of tight control for rheumatoid arthritis (the TICORA study): a single-blind randomised controlled trial. Lancet. (2004) 364:263–9. doi: 10.1016/S0140-6736(04)16676-2
3. Wells G, Becker JC, Teng J, Dougados M, Schiff M, Smolen J, et al. Validation of the 28-joint Disease Activity Score (DAS28) and European League Against Rheumatism response criteria based on C-reactive protein against disease progression in patients with rheumatoid arthritis, and comparison with the DAS28 based on erythrocyte sedimentation rate. Ann Rheum Dis. (2009) 68:954–60. doi: 10.1136/ard.2007.084459
4. Smolen JS, Breedveld FC, Schiff MH, Kalden JR, Emery P, Eberl G, et al. A simplified disease activity index for rheumatoid arthritis for use in clinical practice. Rheumatology (Oxford). (2003) 42:244–57. doi: 10.1093/rheumatology/keg072
5. Aletaha D, Smolen J. The Simplified Disease Activity Index (SDAI) and the Clinical Disease Activity Index (CDAI): a review of their usefulness and validity in rheumatoid arthritis. Clin Exp Rheumatol. (2005) 23(5 Suppl. 39):S100–8.
6. Ahlmén M, Svensson B, Albertsson K, Forslind K, Hafström I. Influence of gender on assessments of disease activity and function in early rheumatoid arthritis in relation to radiographic joint damage. Ann Rheum Dis. (2010) 69:230–3. doi: 10.1136/ard.2008.102244
7. Castrejón Fernández I, Martínez-López JA, Ortiz García AM, Carmona Ortells L, García-Vicuña R, González-Álvaro I. Influence of gender on treatment response in a cohort of patients with early rheumatoid arthritis in the area 2 of Madrid. Reumatol Clin. (2010) 6:134–40. doi: 10.1016/j.reuma.2009.09.014
8. Castrejón I, Carmona L, Ortiz AM, Belmonte MA, Martínez-López JA, González-Álvaro I. Development and validation of a new disease activity index as a numerical sum of four variables in patients with early arthritis. Arthritis Care Res. (2013) 65:518–25. doi: 10.1002/acr.21854
9. Gonzalez-Alvaro I, Castrejon I, Ortiz AM, Toledano E, Castaneda S, Garcia-Vadillo A, et al. Cut-Offs and Response Criteria for the Hospital Universitario La Princesa Index (HUPI) and their comparison to widely-used indices of disease activity in rheumatoid arthritis. PLoS ONE. (2016) 11:e0161727. doi: 10.1371/journal.pone.0161727
10. Gonzalez-Alvaro I, Castrejon I, Carmona L, Act-Ray P, groups Es. The comparative responsiveness of Hospital Universitario Princesa Index and other composite indices for assessing rheumatoid arthritis activity. PLoS ONE. (2019) 14:e0214717. doi: 10.1371/journal.pone.0214717
11. England BR, Tiong BK, Bergman MJ, Curtis JR, Kazi S, Mikuls TR, et al. 2019 Update of the American college of rheumatology recommended rheumatoid arthritis disease activity measures. Arthritis Care Res. (2019) 71:1540–55. doi: 10.1002/acr.24042
12. van Steenbergen HW, da Silva JAP, Huizinga TWJ, van der Helm-van Mil AHM. Preventing progression from arthralgia to arthritis: targeting the right patients. Nat Rev Rheumatol. (2018) 14:32–41. doi: 10.1038/nrrheum.2017.185
13. Smolen JS, Breedveld FC, Burmester GR, Bykerk V, Dougados M, Emery P, et al. Treating rheumatoid arthritis to target: 2014 update of the recommendations of an international task force. Ann Rheum Dis. (2016) 75:3–15. doi: 10.1136/annrheumdis-2015-207524
14. Baillet A, Gossec L, Paternotte S, Etcheto A, Combe B, Meyer O, et al. Evaluation of serum interleukin-6 level as a surrogate marker of synovial inflammation and as a factor of structural progression in early rheumatoid arthritis: results from a French national multicenter cohort. Arthritis Care Res (Hoboken). (2015) 67:905–12. doi: 10.1002/acr.22513
15. Radner H, Chatzidionysiou K, Nikiphorou E, Gossec L, Hyrich KL, Zabalan C, et al. 2017 EULAR recommendations for a core data set to support observational research and clinical care in rheumatoid arthritis. Ann Rheum Dis. (2018) 77:476–9. doi: 10.1136/annrheumdis-2017-212256
16. Dougados M, Kissel K, Sheeran T, Tak PP, Conaghan PG, Mola EM, et al. Adding tocilizumab or switching to tocilizumab monotherapy in methotrexate inadequate responders: 24-week symptomatic and structural results of a 2-year randomised controlled strategy trial in rheumatoid arthritis (ACT-RAY). Ann Rheum Dis. (2013) 72:43–50. doi: 10.1136/annrheumdis-2011-201282
17. Arnett FC, Edworthy SM, Bloch DA, McShane DJ, Fries JF, Cooper NS, et al. The American Rheumatism Association 1987 revised criteria for the classification of rheumatoid arthritis. Arthritis Rheum. (1988) 31:315–24. doi: 10.1002/art.1780310302
18. Verpoort KN, van Dongen H, Allaart CF, Toes RE, Breedveld FC, Huizinga TW. Undifferentiated arthritis–disease course assessed in several inception cohorts. Clin Exp Rheumatol. (2004) 22(5 Suppl. 35):S12–7.
19. Cardiel MH, Abello-Banfi M, Ruiz-Mercado R, Alarcon-Segovia D. How to measure health status in rheumatoid arthritis in non-English speaking patients: validation of a Spanish version of the Health Assessment Questionnaire Disability Index (Spanish HAQ-DI). Clin Exp Rheum. (1993) 11:117–21.
20. Wolfe F. The determination and measurement of functional disability in rheumatoid arthritis. Arthritis Res Ther. (2002) 4(Suppl. 2):S11–5. doi: 10.1186/ar547
21. Lamana A, López-Santalla M, Castillo-González R, Ortiz AM, Martín J, García-Vicuña R, et al. The minor allele of rs7574865 in the STAT4 gene is associated with increased mRNA and protein expression. PLoS ONE. (2015) 10:e0142683. doi: 10.1371/journal.pone.0142683
22. Genant HK, Jiang Y, Peterfy C, Lu Y, Rédei J, Countryman PJ. Assessment of rheumatoid arthritis using a modified scoring method on digitized and original radiographs. Arthritis Rheum. (1998) 41:1583–90. doi: 10.1002/1529-0131(199809)41:9<1583::AID-ART8>3.0.CO;2-H
23. van der Heijde D. How to read radiographs according to the Sharp/van der Heijde method. J Rheumatol. (2000) 27:261–3.
24. Toledano E, Ortiz AM, Ivorra-Cortes J, Montes N, Beltran A, Rodríguez-Rodriguez L, et al. Are rheumatologists adhering to the concepts window of opportunity and treat-to-target? Earlier and more intense disease-modifying anti-rheumatic drug treatment over time in patients with early arthritis in the PEARL study. Clin Exp Rheumatol. (2018) 36:382–8.
25. Prevoo ML, van 't Hof MA, Kuper HH, van Leeuwen MA, van de Putte LB, van Riel PL. Modified disease activity scores that include twenty-eight-joint counts. Development and validation in a prospective longitudinal study of patients with rheumatoid arthritis. Arthritis Rheum. (1995) 38:44–8. doi: 10.1002/art.1780380107
26. Fransen J, van Riel PL. The disease activity score and the EULAR response criteria. Clin Exp Rheumatol. (2005) 23(5 Suppl. 39):S93–9.
27. Anderson JK, Zimmerman L, Caplan L, Michaud K. Measures of rheumatoid arthritis disease activity: Patient (PtGA) and Provider (PrGA) Global Assessment of Disease Activity, Disease Activity Score (DAS) and Disease Activity Score with 28-Joint Counts (DAS28), Simplified Disease Activity Index (SDAI), Clinical Disease Activity Index (CDAI), Patient Activity Score (PAS) and Patient Activity Score-II (PASII), Routine Assessment of Patient Index Data (RAPID), Rheumatoid Arthritis Disease Activity Index (RADAI) and Rheumatoid Arthritis Disease Activity Index-5 (RADAI-5), Chronic Arthritis Systemic Index (CASI), Patient-Based Disease Activity Score With ESR (PDAS1) and Patient-Based Disease Activity Score without ESR (PDAS2), and Mean Overall Index for Rheumatoid Arthritis (MOI-RA). Arthritis Care Res. (2011) 63 (Suppl. 11):S14–36. doi: 10.1002/acr.20621
28. Pan W. Akaike's information criterion in generalized estimating equations. Biometrics. (2001) 57:120–5. doi: 10.1111/j.0006-341X.2001.00120.x
29. Højsgaard S, Halekoh U, Yan J. The R package geepack for generalized estimating equations. J Stat Softw. (2006) 1:55775. doi: 10.18637/jss.v015.i02
30. Burnham K, Anderson DR. Model Selection and Inference: A Practical Information-Theoretic Approach. 2nd ed. New York (2002) (accessed July 8, 2020).
31. Team RC. R: A language and environment for statistical computing Vienna, Austria. R Foundation for Statistical Computing (2020). Available online at: https://www.R-project.org/ (accessed July 8, 2020).
32. Jaeger B. r2glmm: Computes R Squared for Mixed (Multilevel) Models. R package version 0.1.2 (2017). Available online at: https://CRAN.R-project.org/package=r2glmm (accessed July 8, 2020).
33. World Medical Association. World Medical Association Declaration of Helsinki: ethical principles for medical research involving human subjects. JAMA. (2013) 310:2191–4. doi: 10.1001/jama.2013.281053
34. Aletaha D, Nell VP, Stamm T, Uffmann M, Pflugbeil S, Machold K, et al. Acute phase reactants add little to composite disease activity indices for rheumatoid arthritis: validation of a clinical activity score. Arthritis Res Ther. (2005) 7:R796–806. doi: 10.1186/ar1740
35. Madhok R, Crilly A, Watson J, Capell HA. Serum interleukin 6 levels in rheumatoid arthritis: correlations with clinical and laboratory indices of disease activity. Ann Rheum Dis. (1993) 52:232–4. doi: 10.1136/ard.52.3.232
36. Navarro-Compan V, Gherghe AM, Smolen JS, Aletaha D, Landewe R, van der Heijde D. Relationship between disease activity indices and their individual components and radiographic progression in RA: a systematic literature review. Rheumatology (Oxford). (20150 54:994–1007. doi: 10.1093/rheumatology/keu413
37. Klarenbeek NB, Koevoets R, van der Heijde DM, Gerards AH, Ten Wolde S, Kerstens PJ, et al. Association with joint damage and physical functioning of nine composite indices and the 2011 ACR/EULAR remission criteria in rheumatoid arthritis. Ann Rheum Dis. (2011) 70:1815–21. doi: 10.1136/ard.2010.149260
Keywords: rheumatoid arthritis, outcome assessment (health care), statistical analysis, interleukin-6, radiographic progression, disease activity score
Citation: Rodriguez-García SC, Montes N, Ivorra-Cortes J, Triguero-Martinez A, Rodriguez-Rodriguez L, Castrejón I, Carmona L and González-Álvaro I (2021) Disease Activity Indices in Rheumatoid Arthritis: Comparative Performance to Detect Changes in Function, IL-6 Levels, and Radiographic Progression. Front. Med. 8:669688. doi: 10.3389/fmed.2021.669688
Received: 19 February 2021; Accepted: 30 April 2021;
Published: 31 May 2021.
Edited by:
Antonio Manzo, University of Pavia, ItalyReviewed by:
Susanna Proudman, University of Adelaide, AustraliaJoaquim Polido Pereira, Santa Maria Hospital, Portugal
Copyright © 2021 Rodriguez-García, Montes, Ivorra-Cortes, Triguero-Martinez, Rodriguez-Rodriguez, Castrejón, Carmona and González-Álvaro. This is an open-access article distributed under the terms of the Creative Commons Attribution License (CC BY). The use, distribution or reproduction in other forums is permitted, provided the original author(s) and the copyright owner(s) are credited and that the original publication in this journal is cited, in accordance with accepted academic practice. No use, distribution or reproduction is permitted which does not comply with these terms.
*Correspondence: Isidoro González-Álvaro, aXNpZG9yby5nYUBzZXIuZXM=