- 1Department of General Surgery, Shengjing Hospital, China Medical University, Shenyang, China
- 2Department of Health Management, Shengjing Hospital, China Medical University, Shenyang, China
Background: To develop and validate novel nomograms for better predicting the overall survival (OS) and cancer-specific survival (CSS) of patients with vulvar squamous cell cancer (VSCC).
Methods: A retrospective analysis using a population-based database between 2004 and 2016 was carried. A 10-fold cross-validation with 200 repetitions was used to choose the best fit multivariate Cox model based on the net-benefit of decision curve analysis. Net-benefit, Harrell's C concordance statistic (C-statistic) of calibration plot, and area under the receiver operating characteristic curve (AUC) were used to evaluate the model prediction accuracy. Nomograms of the OS and CSS were generated based on the best fit model.
Results: Of the 6,792 patients with VSCC, 5,094 (75%) and 1,698 (25%) were allocated to the training and validation cohort, respectively. All the variables were balanced between the training and validation cohorts. Age, insurance, tumor size, pathological grade, radiotherapy, chemotherapy, invasion depth, lymphadenectomy, sentinel lymph nodes biopsy, surgery, N stage, and M stage were in the best fit model for generating nomograms. The decision curve analysis, calibration plot, and receiver operating characteristic (ROC) curve show the better prediction performance of the model compared to previous studies. The C-statistics of our model for OS prediction are 0.80, 0.83, and 0.81 in the training, validation, and overall cohorts, respectively, while for CSS prediction are 0.83, 0.85, and 0.84. The AUCs for 3- and 5-year OS are the same and are 0.81, 0.83, and 0.81 in the training, validation, and overall cohorts, respectively. The AUCs for 3- and 5-year CSS are 0.78 and 0.80, 0.79 and 0.80, and 0.79 and 0.80 in those three cohorts.
Conclusions: Our model shows the best prediction accuracy of the OS and CSS for patients with vulvar cancer (VC), which is of significant clinical practice value.
Introduction
Primary vulvar cancer (VC) is a rare malignancy that accounts for about 5% of all gynecologic cancer cases, with more than 6,100 newly diagnosed cases yearly and a rising death rate trends in the United States, leading to more than 1,400 in 2020 to 1,500 in 2021 (1, 2). Furthermore, 90% of VC is squamous cell carcinoma (VSCC) (3).
The primary therapy for VSCC is surgical resection and radiotherapy with/without chemotherapy (3, 4). VC frequently spreads to the regional lymph nodes. The patients with regional lymph nodes involvement had worse survival (5). For VSCC with lymph node metastasis, lymphadenectomy and sentinel lymph nodes biopsy (SLNB) were both carried out. However, lymphadenectomy is associated with a high probability of complications (66–85%) that are the fundamental cause of death after surgery, such as wound breakdown, infection, lymphoceles, lymphedema, cellulitis, and erysipelas (6). After applying several new surgical techniques of lymphadenectomy in recent years, the morbidity after lymphadenectomy decreased in recent years but remains high (7). SLNB is less aggressive and has a lower complication occurrence rate and thus could prolong the survival of patients with VSCC, so it is preferred as the replacement of lymphadenectomy for well-selected patients with VSCC. Moreover, SLNB has a sensitivity of more than 95% to indicate lymph node involvement and a specialty of nearly 100% (8). So SLNB should be included as a predictor in nomograms for survival prediction. However, no nomograms for predicting the survival of the patients with VC have taken SLNB into account.
Nomograms for predicting the cancer-specific survival (CSS) of the patients with VC have been developed. For example, a nomogram based on age, American Joint Committee on Cancer (AJCC) T stage, invasion depth, margin status, and lymph nodes status had a Harrell's C concordance statistics (C-statistic) of 0.78 and 0.83 in the validation study and training study, respectively (9, 10). However, detection of margin status and the number of lymph nodes involved are difficult and highly influenced by the experience and imaging techniques of clinicians. Some studies even found that margin status was not associated with survival, probably due to the hardship of identifying margin status (11–13). A recently developed study comprising age, tumor size, pathological stage, metastasis, radiotherapy, chemotherapy, and surgery had a prediction accuracy of C-statistics of 0.81 for CSS prediction, without considering the invasion depth, SLNB, and N stage (14). Moreover, no nomograms have been developed to predict the overall survival (OS) of the patients with VC.
Therefore, we tried to develop novel nomograms for precisely predicting the OS and CSS for the patients with VC using a population-based database.
Materials and Methods
Data Source and Study Population
The patients with the International Classification of Diseases for Oncology, 3rd Edition (ICD-O-3) codes of C51.0, C51.1, C51.2, C51.8, C51.9, and the ICD-O-3 histology codes 8050-8084 (squamous cell carcinoma) were selected from the Surveillance, Epidemiology, and End Results (SEER) Program database of the National Cancer Institute from 2004 to 2016 (15). Moreover, the patients were excluded under the following conditions: (1) not squamous cell carcinoma; (2) not the only first primary tumor; (3) age at diagnosis <18 or more than 100 years old; and (4) not confirmed by positive histology.
The variables of primary site, year of diagnosis, insurance type, age, marital status, tumor size, pathological grade, radiotherapy, chemotherapy, historical stage, invasion depth, lymphadenectomy, sentinel lymph node biopsy, surgery, International Federation of Gynecology and Obstetrics (FIGO) stage, AJCC stage, AJCC T, N, and M stage were retrieved from the corresponding fields of the SEER database.
Outcomes
Overall survival was the primary outcome. CSS was the secondary outcome, calculated based on the patients whose death was attributable to vulvar cancer, while those who died of other reasons rather than VC were considered censors.
Statistical Analysis
The overall sample was randomly split into training (75%) and validation (25%) cohorts, with the constraints of keeping the proportion of death events similar between those two cohorts, following the Transparent Reporting of a multivariable prediction model for Individual Prognosis or Diagnosis (TRIPOD) guideline (16). The chi-square test was applied to test the balance of all the available variables between the training and validation groups. Within the training cohort, a 10-fold cross-validation with 200 repetitions was carried out to identify the best fit model based on the net benefit and Harrell's C-statistic, model with the largest average net benefit was considered as the best fit model. If the models have the same net benefit and C-statistic, the one with fewer variables is chosen.
After the best fit model had been identified, the model was refitted on the overall training cohort and validated on the validation cohort. The net benefit from the decision curve analysis (DCA), C-statistic of calibration plot, and areas under receiver operating characteristic curve (AUC) were used to measure the prediction performance of models. The 95% CI of C-statistic and AUC were calculated by bootstrap with 1,000 repetitions. The nomograms based on the best-fit model refitted on the overall sample (combination of training and validation cohort) were generated for 3- and 5-year OS and CSS.
Several multivariate Cox models such as all and part of the year of diagnosis, insurance status, age, race, marital status, primary site, historical stage, pathological grade, tumor size, invasion depth, surgery, radiotherapy, chemotherapy, FIGO stage, AJCC T, N and M stage were fitted and compared. Hazard ratio (HR) and their corresponding 95% CIs were calculated.
A two-tailed p-value of < 0.05 was considered statistically significant. All the statistics were performed in STATA 16.0 software (StataCorp, College Station, TX, USA).
Results
Baseline Characteristics
Figure 1 shows the sample selection procedure. Of the 6,792 patients in this study, 5,094 (75%) and 1,698 (25%) were randomly allocated into the training and validation groups. The primary site, year of diagnosis, insurance type, age, marital status, tumor size, pathological grade, radiotherapy, chemotherapy, historical stage, invasion depth, lymphadenectomy, sentinel lymph node biopsy, surgery, FIGO stage, AJCC stage, AJCC T, N and M stage were all balanced between the training and validation groups (all chi-square p > 0.05, Table 1).
Multivariate Cox Proportional Hazards Model Selection
Within the training cohort, a 10-fold cross-validation with 200 repetitions was carried out to choose the best fit model with the largest average net benefit. If two models have a similar average net benefit, the one with fewer variables was selected. Finally, the model comprising age, insurance, tumor size, pathological grade, radiotherapy, chemotherapy, invasion depth, lymphadenectomy, SLNB, surgery, N stage, and M stage was chosen for both predicting OS and CSS.
Effects of Variables in the Best Fit Model for OS and CSS
As shown in Tables 2, 3, within the training cohort, age, insurance, tumor size, pathological grade, chemotherapy, invasion depth, SLNB, surgery, and N stage and M stage were the factors significantly associated with OS and CSS (all p < 0.001). However, radiotherapy was not associated.
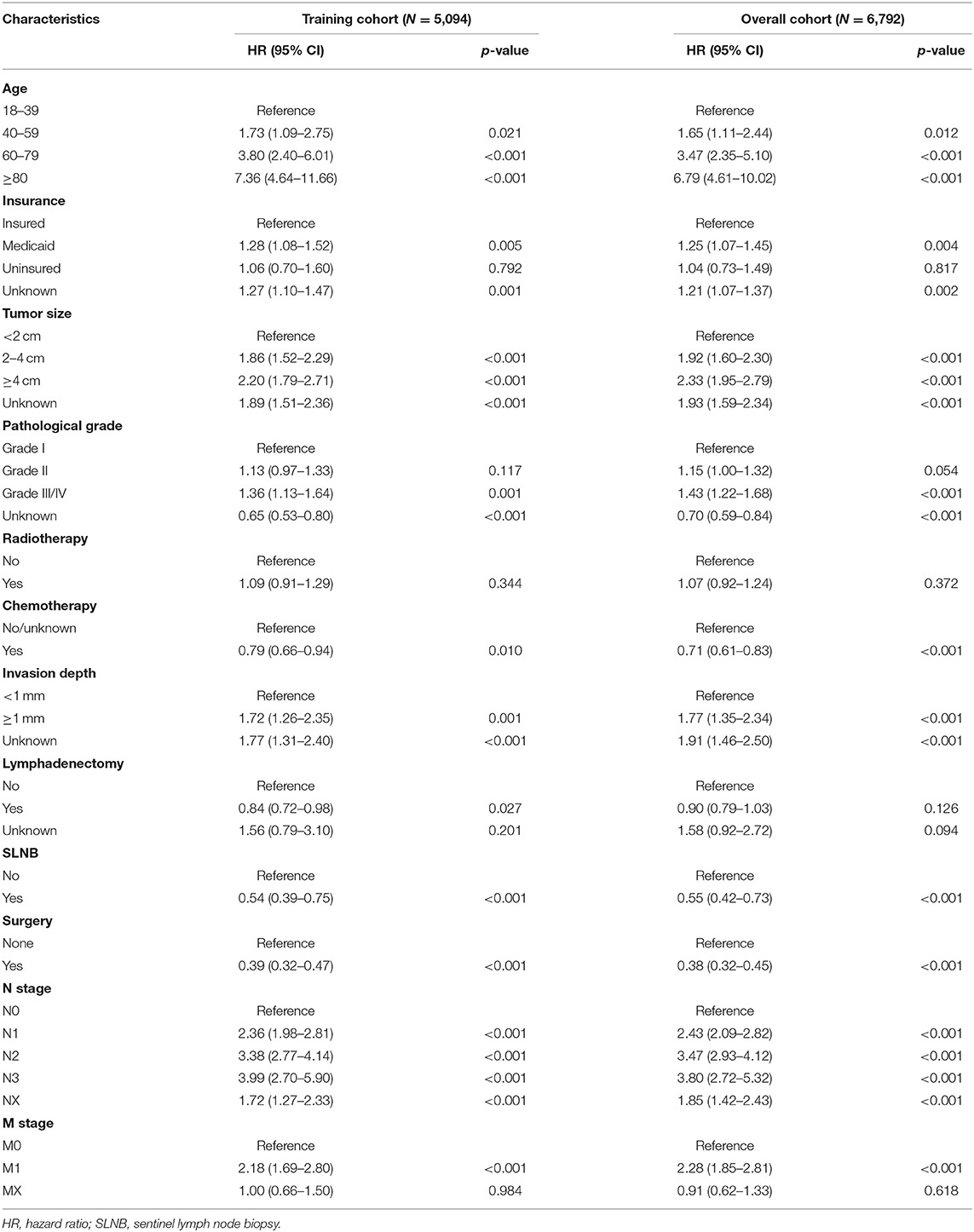
Table 3. The results of multivariate cox proportional hazard risk model for cancer-specific survival (CSS).
Model Prediction Accuracy
The DCA plot was shown in Figures 2, 3. Compared with the previously published nomograms with the best accuracy for CSS prediction (14), our model has the larger net benefit.
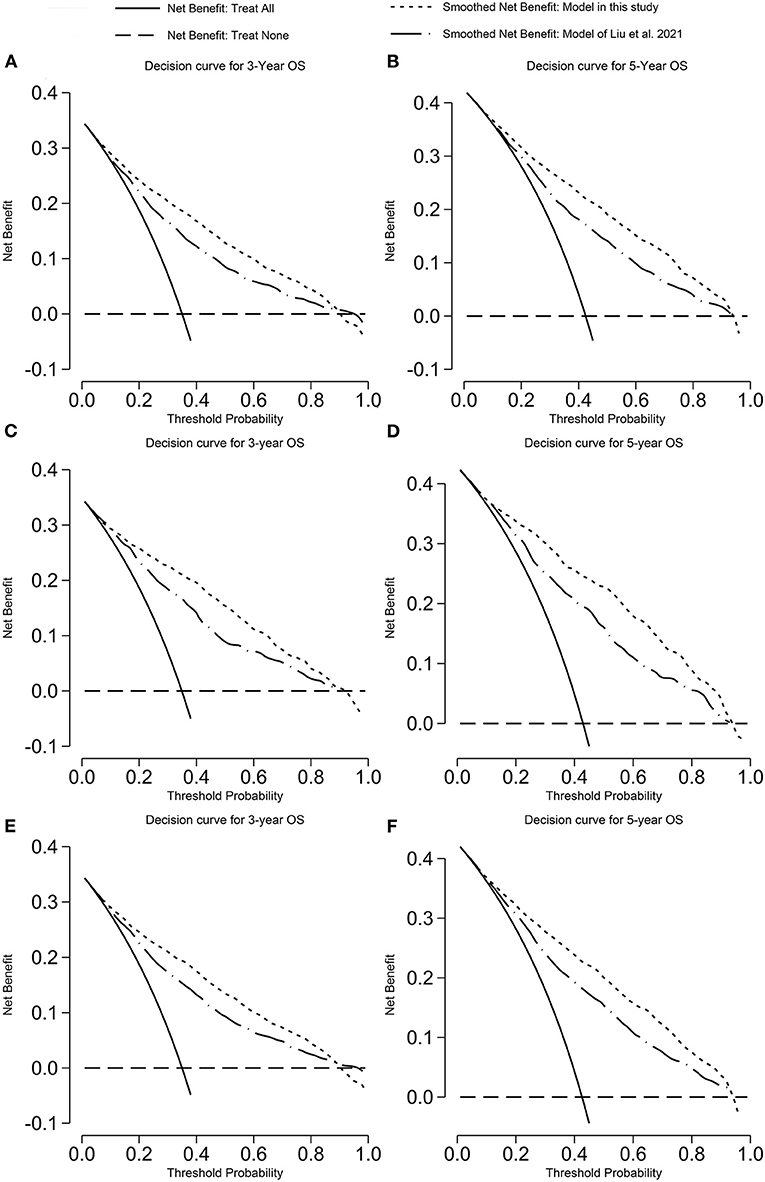
Figure 2. Decision curve analysis on the model predicting OS. (A) 3-years OS in the training cohort; (B) 5-years OS in the training cohort; (C) 3-years OS in the validation cohort; (D) 5-years OS in the validation cohort; (E) 3-years OS in the overall cohort; and (F) 5-years OS in the overall cohort. OS, overall survival.
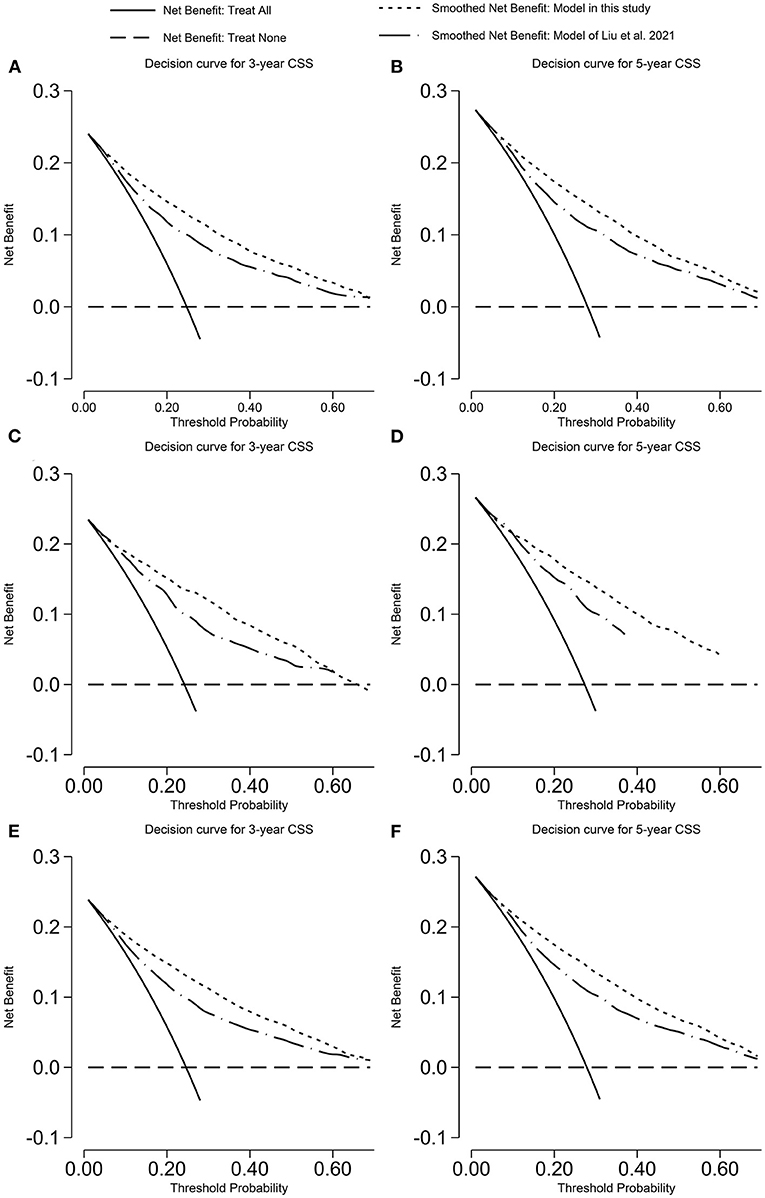
Figure 3. Decision curve analysis on the model predicting CSS. (A) 3-years CSS in the training cohort; (B) 5-years CSS in the training cohort; (C) 3-years CSS in the validation cohort; (D) 5-years CSS in the validation cohort; (E) 3-years CSS in the overall cohort; and (F) 5-years CSS in the overall cohort. CSS, cancer-specific survival.
The calibration plot was displayed in Figures 4, 5. The model has C-statistics of 0.80 (95% CI 0.79–0.81), 0.83 (95% CI 0.81–0.84), and 0.81 (95% CI 0.80–0.82) for the training cohort, validation cohort, and overall cohort, respectively, for predicting the OS. For predicting the CSS, those numbers are 0.83 (95% CI 0.82–0.84), 0.85 (95% CI 0.83–0.86), and 0.84 (95% CI 0.83–0.84).
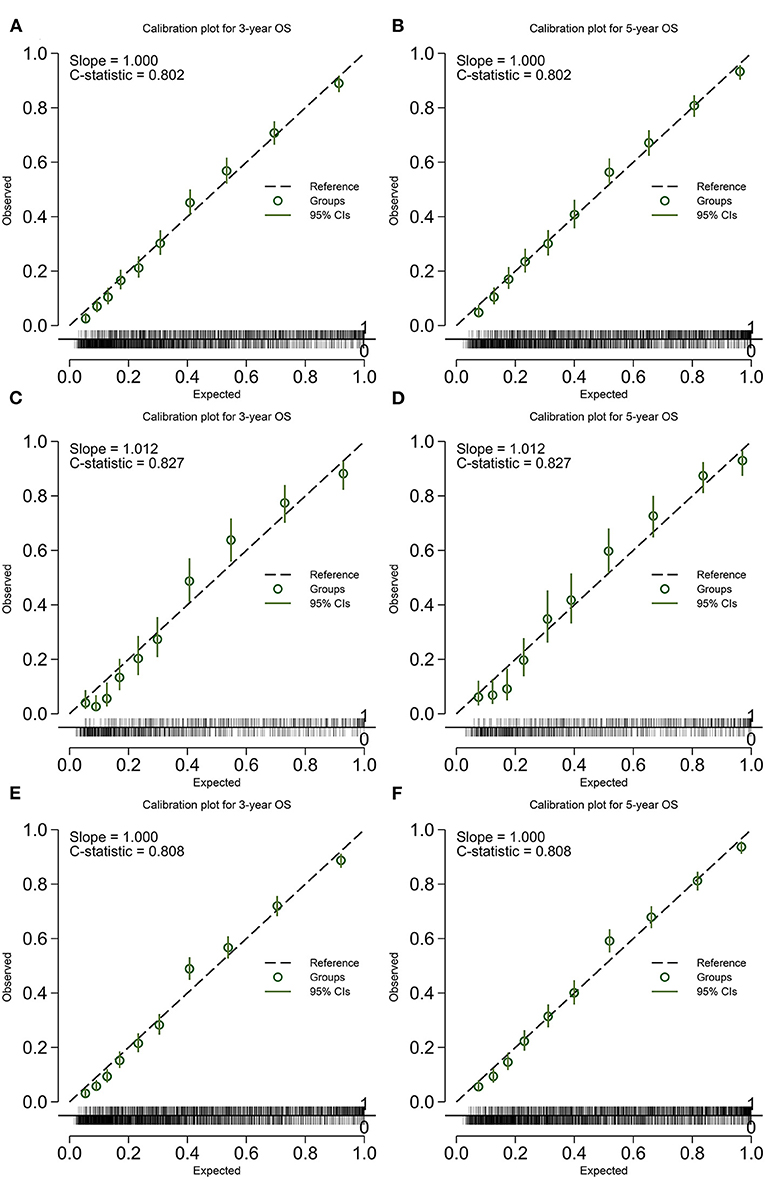
Figure 4. Calibration plot of the model predicting OS. (A) 3-years OS in the training cohort; (B) 5-years OS in the training cohort; (C) 3-years OS in the validation cohort; (D) 5-years OS in the validation cohort; (E) 3-years OS in the overall cohort; and (F) 5-years OS in the overall cohort. OS, overall survival.
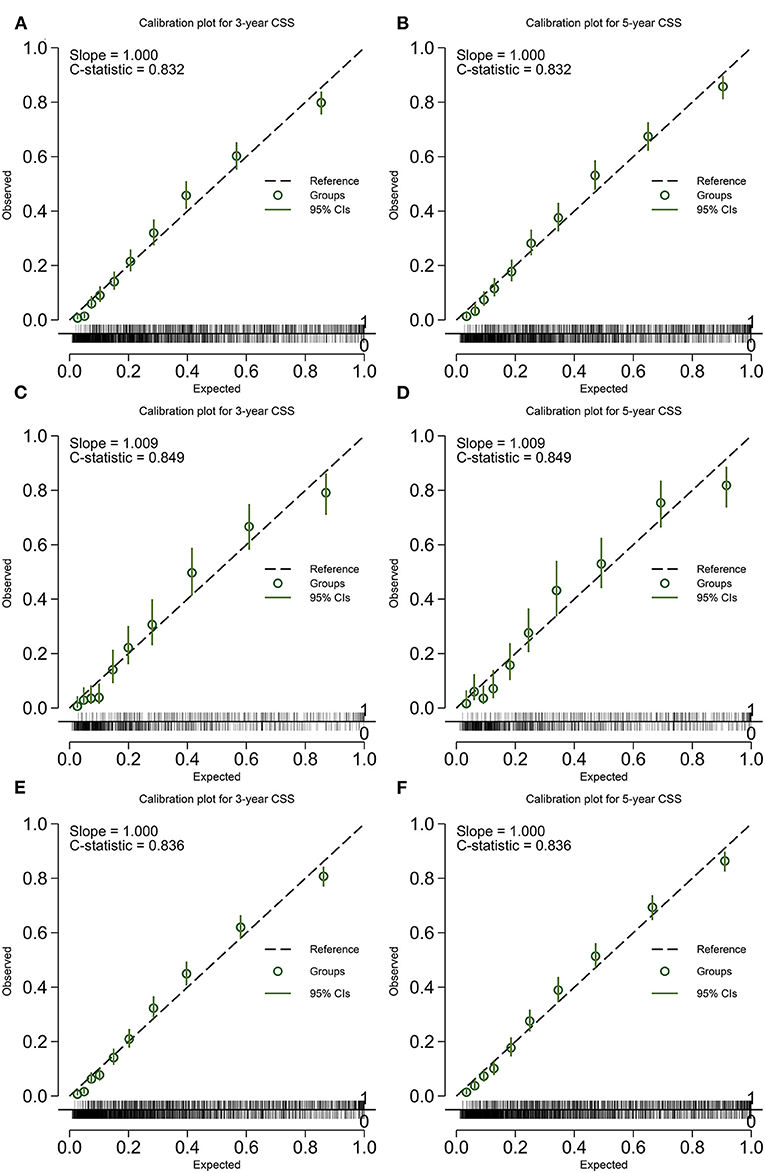
Figure 5. Calibration plot of the model predicting CSS. (A) 3-years CSS in the training cohort; (B) 5-years CSS in the training cohort; (C) 3-years CSS in the validation cohort; (D) 5-years CSS in the validation cohort; (E) 3-years CSS in the overall cohort; and (F) 5-years CSS in the overall cohort. CSS, cancer-specific survival.
The receiver operating characteristic (ROC) curves were illustrated in Figures 6, 7. The AUCs for the prediction of 3- and 5-year OS are 0.81 (95% CI 0.79–0.82) and 0.81 (95% CI 0.79–0.82), 0.83 (95% CI 0.81–0.86) and 0.83 (95% CI 0.81–0.85), and 0.81 (95% CI 0.80–0.83) and 0.81 (95% CI 0.80–0.82), respectively, for the training cohort, validation cohort, and the overall cohort. With reference to the prediction of CSS, those numbers are 0.78 (95% CI 0.77–0.80) and 0.80 (95% CI 0.78–0.81), 0.79 (95% CI 0.76–0.82) and 0.8 (95% CI 0.77–0.83), and 0.79 (95% CI 0.77–0.80) and 0.80 (95% CI 0.79–0.81), respectively.
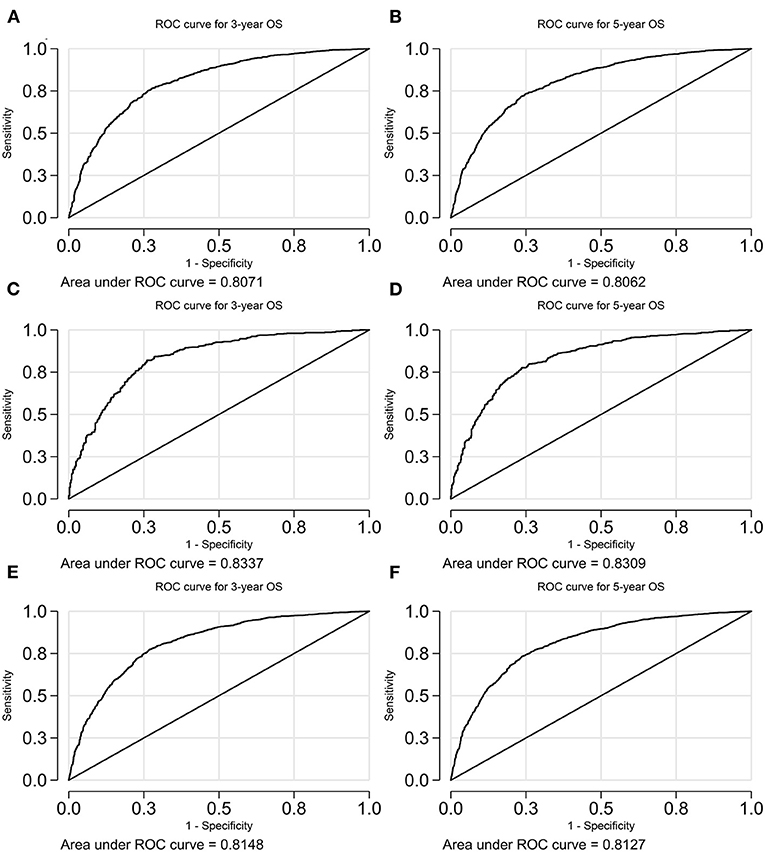
Figure 6. A receiver operating characteristic (ROC) curve of the model predicting OS. (A) 3-years OS in the training cohort; (B) 5-years OS in the training cohort; (C) 3-years OS in the validation cohort; (D) 5-years OS in the validation cohort; (E) 3-years OS in the overall cohort; and (F) 5-years OS in the overall cohort. OS, overall survival.
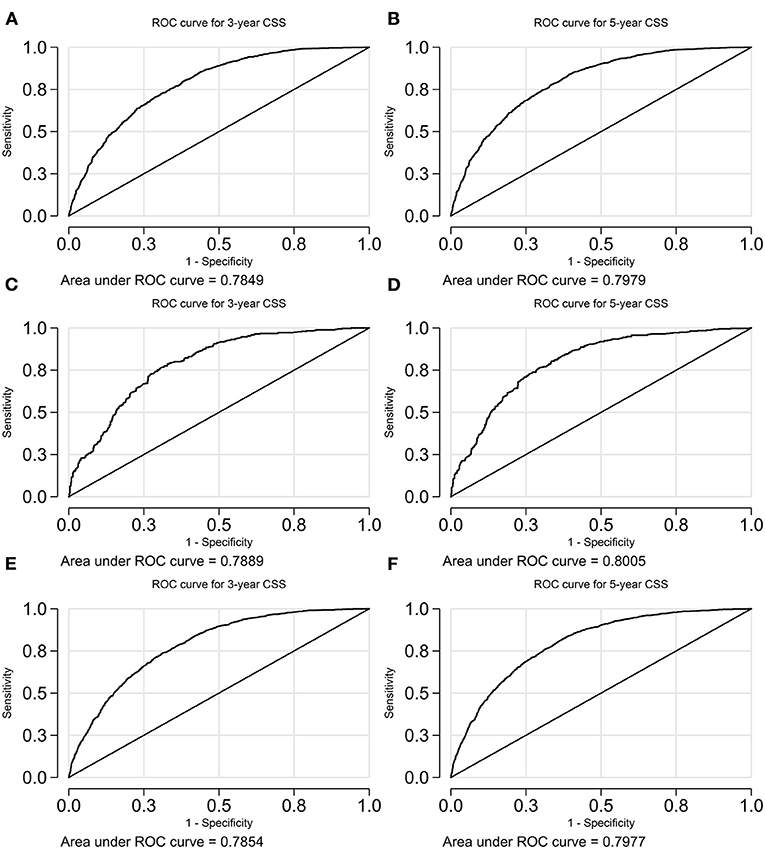
Figure 7. A ROC curve of the model predicting CSS. (A) 3-years CSS in the training cohort; (B) 5-years CSS in the training cohort; (C) 3-years CSS in the validation cohort; (D) 5-years CSS in the validation cohort; (E) 3-years CSS in the overall cohort; and (F) 5-years CSS in the overall cohort. CSS, cancer-specific survival.
Nomogram for Predicting 3- and 5-Year Survival
The best fit model was refitted on the overall cohort (combination of the training and validation cohort), and the result of the model was used to generate nomograms for predicting 3- and 5-year OS (Figure 8) and CSS (Figure 9).
Discussion
This study developed novel nomograms to predict the 3- and 5-year OS and CSS for the patients with VSCC aged 18–100 years, based on a cohort of 6,792 cases from a population-based multicenter database. To our knowledge, the novel nomograms in our study have the best prediction accuracy, with excellent clinical practice importance.
Compared with the previously developed nomograms for predicting CSS of the patients with VC, for CSS prediction, our model has the better net benefit and the largest C-statistics of 0.83, 0.85, and 0.84 in the training, validation, and overall cohort, respectively. Our model comprises of factors that are commonly inspected and easy to get in clinical practice. Moreover, we did not exclude cases with the variables having missed or unknown values, which expanded the applying range of our model.
In line with previous studies, our model comprises age, tumor size, pathologic grade, radiotherapy, chemotherapy, surgery, and M stage, which were significant factors associated with CSS and included in the previously generated nomograms (9, 10, 14). Moreover, the FIGO stage was not included in our final model, although it was the most prevalent stage system for gynecological cancers, similar to two studies (9, 10). The invasion depth and N stage were also included in the final model, which has been argued as an essential prognostic factor of VSCC (9, 10) but not included in a more recently published nomogram (14). The inclusion of radiotherapy tended to be associated with improved survival; although it is not significant in the final model, the addition of it increased the prediction accuracy of the model, contrary to a recent study in which radiotherapy was negatively associated with VSCC (14).
To our knowledge, the first unique characteristic of this study is that it is the first study that generated a nomogram for predicting 3- and 5-year OS of the patients with VSCC. The nomogram for OS prediction had a good prediction accuracy measured by C-statistics of 0.80, 0.83, and 0.81 in the training, validation, and overall cohorts, respectively. In our study, the models for predicting OS and CSS include the same variables. Accordingly, once the variables of predicting CSS have been determined, OS can be predicted, which intensifies the application of our nomograms. The second unique characteristic is that we included SLNB and lymphadenectomy in the novel nomograms, and those two variables were statistically significant in the best fit model, which led to the precise prediction of the survival and adaption to modern surgical technique development. SLNB and lymphadenectomy have never been integrated as a predictor in nomograms for predicting the survival of patients with VSCC. However, the beneficial role of SLNB in improving survival has been proved in previous studies (17–19). The inclusion of SLNB in the model has improved the prediction accuracy considerably. Age and N stage was the strongest predictors of OS and CSS in our model, followed by surgery, M stage, and tumor size.
Before applying those nomograms in clinical practice, several points need to clarify. First, we only included VC patients with squamous cell carcinoma in the training and validation procedures, and accordingly, those nomograms could only be used for patients with VSCC. Applying to other histological types of patients with VC is not suggested. Second, the models were built based on the patients with VC aged between 18 and 100 years old. Whether those can be expanded to patients older than 100 has not been straightforward; thus, expansion should be cautious. Third, the VC patients with other malignancies or not with VSCC as a first tumor were not included in the training and validation samples; accordingly, the novel nomograms should not be applied to those patients. In addition, the nomograms in this study should be preferred to be applied to the patients with just one malignancy of VSCC. Fourth, the patients with VSCC confirmed not by positive histology were not suitable for those nomograms due to the exclusion of those patients from the sample.
This study has some limitations. First, we could not obtain detailed information on radiotherapy and chemotherapy, for example, the drug agent and dose of chemotherapy and the intensity of radiotherapy. Thus, we could not control the impact of those factors on survival. Second, due to the nature of a retrospective study, there might be missing essential factors for predicting survival, which would lead to bias. Third, the usefulness of those nomograms may be limited to the United States because we used the SEER database, which only includes the United States population. Fourth, we could not carry out external validation because no patients with VSCC from a different population or within a single center could be available as a result of the extreme rareness of VC.
Though our study had some limitations, it generated the nomograms with the best prediction accuracy and, first, predicting the OS of the patients with VSCC. This study provides the novel nomograms for the clinicians to accurately predict the OS and CSS of the patients with VSCC and, consequently, clinicians could carry out more targeted therapy procedures.
Conclusions
The novel nomograms for predicting OS and CSS of the patients with VC have the best prediction accuracy, which is of significant clinical practice value.
Data Availability Statement
Publicly available datasets were analyzed in this study. This data can be found here: https://seer.cancer.gov/.
Ethics Statement
Ethical review and approval was not required for the study on human participants in accordance with the local legislation and institutional requirements. Written informed consent for participation was not required for this study in accordance with the national legislation and the institutional requirements.
Author Contributions
WZ: conceptualization, methodology, data curation, formal analyses, supervision, writing original draft preparation, writing, reviewing, and editing. YY: formal analyses, methodology, software, supervision, visualization, writing original draft preparation, writing, reviewing, and editing. All authors contributed to the article and approved the submitted version.
Conflict of Interest
The authors declare that the research was conducted in the absence of any commercial or financial relationships that could be construed as a potential conflict of interest.
Publisher's Note
All claims expressed in this article are solely those of the authors and do not necessarily represent those of their affiliated organizations, or those of the publisher, the editors and the reviewers. Any product that may be evaluated in this article, or claim that may be made by its manufacturer, is not guaranteed or endorsed by the publisher.
Acknowledgments
The authors acknowledge the efforts of the Surveillance, Epidemiology, and End Results (SEER) Program registries in the establishment of the SEER database. An ethical review process was not required for this study because the data were obtained from the SEER database, and we have signed the Data-use Agreement for the SEER 1975–2016 Research Data File.
Supplementary Material
The Supplementary Material for this article can be found online at: https://www.frontiersin.org/articles/10.3389/fmed.2021.777605/full#supplementary-material
References
1. Siegel RL, Miller KD, Jemal A. Cancer statistics, 2020. CA Cancer J Clin. (2020) 70:7–30. doi: 10.3322/caac.21590
2. Siegel RL, Miller KD, Fuchs HE, Jemal A. Cancer statistics, 2021. CA Cancer J Clin. (2021) 71:7–33. doi: 10.3322/caac.21654
3. Tan A, Bieber AK, Stein JA, Pomeranz MK. Diagnosis and management of vulvar cancer: a review. J Am Acad Dermatol. (2019) 81:1387–96. doi: 10.1016/j.jaad.2019.07.055
4. Dellinger TH, Hakim AA, Lee SJ, Wakabayashi MT, Morgan RJ, Han ES. Surgical management of vulvar cancer. J Natl Compr Canc Netw. (2017) 15:121–8. doi: 10.6004/jnccn.2017.0009
5. Papadia A, Ehm L, Gasparri ML, Wang J, Radan AP, Mueller MD. Unilateral versus bilateral lymph-nodal metastases and oncologic outcome in vulvar cancer patients. J Cancer Res Clin Oncol. (2020) 146:1877–81. doi: 10.1007/s00432-020-03196-9
6. Hinten F, van den Einden LC, Hendriks JC, van der Zee AG, Bulten J, Massuger LF, et al. Risk factors for short- and long-term complications after groin surgery in vulvar cancer. Br J Cancer. (2011) 105:1279–87. doi: 10.1038/bjc.2011.407
7. Pouwer AW, Arts HJ, van der Velden J, de Hullu JA. Limiting the morbidity of inguinofemoral lymphadenectomy in vulvar cancer patients; a review. Expert Rev Anticancer Ther. (2017) 17:615–24. doi: 10.1080/14737140.2017.1337513
8. Meads C, Sutton A, Małysiak S, Kowalska M, Zapalska A, Rogozinska E, et al. Sentinel lymph node status in vulval cancer: systematic reviews of test accuracy and decision-analytic model-based economic evaluation. Health Technol Assess. (2013) 17:1–216. doi: 10.3310/hta17600
9. Kim MK, Kim JW, Lee JM, Lee NW, Cha MS, Kim BG, et al. Validation of a nomogram for predicting outcome of vulvar cancer patients, primarily treated by surgery, in Korean population: multicenter retrospective study through Korean Gynecologic Oncology Group (KGOG-1010). J Gynecol Oncol. (2008) 19:191–4. doi: 10.3802/jgo.2008.19.3.191
10. Rouzier R, Preti M, Haddad B, Martin M, Micheletti L, Paniel BJ. Development and validation of a nomogram for predicting outcome of patients with vulvar cancer. Obstet Gynecol. (2006) 107:672–7. doi: 10.1097/01.AOG.0000198639.36855.e9
11. Te Grootenhuis NC, Pouwer AW, de Bock GH, Hollema H, Bulten J, van der Zee AGJ, et al. Margin status revisited in vulvar squamous cell carcinoma. Gynecol Oncol. (2019) 154:266–75. doi: 10.1016/j.ygyno.2019.05.010
12. Raimond E, Delorme C, Ouldamer L, Carcopino X, Bendifallah S, Touboul C, et al. Surgical treatment of vulvar cancer: impact of tumor-free margin distance on recurrence and survival. A multicentre cohort analysis from the francogyn study group. Eur J Surg Oncol. (2019) 45:2109–14. doi: 10.1016/j.ejso.2019.07.005
13. Woelber L, Griebel LF, Eulenburg C, Sehouli J, Jueckstock J, Hilpert F, et al. Role of tumour-free margin distance for loco-regional control in vulvar cancer-a subset analysis of the Arbeitsgemeinschaft Gynäkologische Onkologie CaRE-1 multicenter study. Eur J Cancer. (2016) 69:180–8. doi: 10.1016/j.ejca.2016.09.038
14. Liu J, Wang M. Development and validation of nomograms predicting cancer-specific survival of vulvar cancer patients: based on the Surveillance, Epidemiology, and End Results Program. Int J Gynaecol Obstet. (2021) 00:1–10. doi: 10.1002/ijgo.13722
15. Van Dyne EA, Henley SJ, Saraiya M, Thomas CC, Markowitz LE, Benard VB. Trends in human papillomavirus-associated cancers - United States, 1999-2015. MMWR Morbid Mortal Weekly Rep. (2018) 67:918–24. doi: 10.15585/mmwr.mm6733a2
16. Collins GS, Reitsma JB, Altman DG, Moons KG. Transparent Reporting of a multivariable prediction model for Individual Prognosis or Diagnosis (TRIPOD): the TRIPOD statement. Ann Intern Med. (2015) 162:55–63. doi: 10.7326/M14-0697
17. Collarino A, Fuoco V, Garganese G, Pereira Arias-Bouda LM, Perotti G, Manca G, et al. Lymphoscintigraphy and sentinel lymph node biopsy in vulvar carcinoma: update from a European expert panel. Eur J Nucl Med Mol Imag. (2020) 47:1261–74. doi: 10.1007/s00259-019-04650-8
18. Alhatem A, Lambert WC, Karanfilian K, Behbahani S, Heller D. Impact of partnership status on clinical outcomes of patients with vulvar squamous cell carcinoma and performance of sentinel lymph node biopsy. Int J Gynecol Cancer. (2020) 30:583–9. doi: 10.1136/ijgc-2019-001001
19. Zhou W, Bai Y, Yue Y. Safety and benefit of sentinel lymph nodes biopsy compared to regional lymph node dissection in primary vulvar cancer patients without distant metastasis and adjacent organ invasion: a retrospective population study. Front Oncol. (2021) 11:676038. doi: 10.3389/fonc.2021.676038
Keywords: invasion depth, lymphadenectomy, sentinel lymph node biopsy, nomogram, tumor size, vulvar cancer, N stage, M stage
Citation: Zhou W and Yue Y (2021) Developing and Validating Novel Nomograms for Predicting the Overall Survival and Cancer-Specific Survival of Patients With Primary Vulvar Squamous Cell Cancer. Front. Med. 8:777605. doi: 10.3389/fmed.2021.777605
Received: 15 September 2021; Accepted: 01 November 2021;
Published: 03 December 2021.
Edited by:
Vito Andrea Capozzi, University Hospital of Parma, ItalyReviewed by:
Luciano Monfardini, University of Parma, ItalyLuigi Della Corte, University of Naples Federico II, Italy
Copyright © 2021 Zhou and Yue. This is an open-access article distributed under the terms of the Creative Commons Attribution License (CC BY). The use, distribution or reproduction in other forums is permitted, provided the original author(s) and the copyright owner(s) are credited and that the original publication in this journal is cited, in accordance with accepted academic practice. No use, distribution or reproduction is permitted which does not comply with these terms.
*Correspondence: Yangyang Yue, eXVleXkxQHNqLWhvc3BpdGFsLm9yZw==