- 1Biomedical and Mobile Health Technology Laboratory, Department of Health Sciences and Technology, ETH Zurich, Zurich, Switzerland
- 2Menrva Research Group, School of Mechatronic Systems Engineering, Simon Fraser University, Vancouver, BC, Canada
- 3Rady Faculty of Health Sciences, University of Manitoba, Winnipeg, MB, Canada
- 4Department of Electrical and Computer Engineering, University of British Columbia, Vancouver, BC, Canada
- 5Mechanical Engineering, Massachusetts Institute of Technology, Cambridge, MA, United States
- 6Department of Psychiatry, University of Massachusetts Medical School, Worcester, MA, United States
- 7Research Centre for Intelligent Healthcare, Coventry University, Coventry, United Kingdom
Introduction
The use of pulse oximetry data has grown significantly in recent years due to new applications of the technology and new wearable sensor platforms, as well as the widespread clinical demands of the ongoing coronavirus pandemic. The recent letter by Sjoding (1) (NEJM, Dec 2020) raising the effect of race (skin color) on pulse oximetry data has recently prompted the U.S. Food and Drug Administration (FDA) to exercise caution when using and interpreting pulse oximetry readings, with recommendation being given to following the trend in pulse oximeter readings rather than focusing on the absolute value of the readings alone (2). This finding is now being communicated to the nursing community as well (3).
The database referenced by Sjoding is one of many large pulse oximetry databases that are often used in clinical research to develop and decision support systems. In addition to the oxygen saturation values, there is now an increasing use of the morphological features of the pulse oximetry waveform which are being used, for example, to develop algorithms to predict blood pressure (4) as well as atherosclerosis (5) for use in patient monitoring and disease management. With the increasing use of these publicly available pulse oximetry databases, caution should be taken to prevent creating a bias in the resulting computer algorithms.
Methods
Prompted by the Sjoding letter, we proceeded to perform a demographic analysis of the main publicly available pulse oximetry databases. In particular, we were most focused on age distribution across these data sets, since it is well-known that the pulse waveform morphology changes significantly as a function of age and atherosclerosis. The result of this analysis, using freely accessible databases (from 2013 through 2021) consisting of pulse oximeter signal (called photoplethysmogram or PPG) signals is presented in Table 1. We classified publicly available databases into two different age categories namely, children (<16 years) and adults (≥16 years).
Discussion
As shown in Figure 1, there is a substantial difference in the number of subjects overall in all publicly available datasets between children and adults. This significant age bias could potentially impact algorithms developed to detect specific abnormalities. The morphology of the PPG waveform is typically different between children and adults, as shown in Figure 1. If a digital health solution is developed that combines a PPG sensor and an algorithm, then testing and evaluating over different age groups is essential to achieve reliability. Note that a significant difference (p < 0.05) between PPG waveform morphologies of different age groups (subjects younger than 30 years, 30–39 years, 40–49 years, and 50 years of age or older) was reported (17).
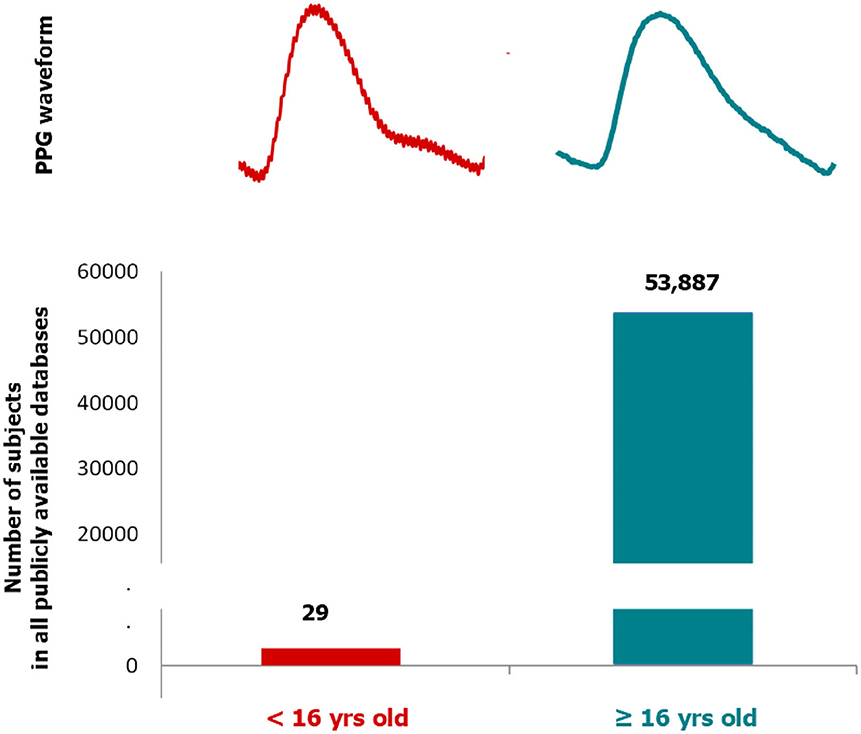
Figure 1. Number of subjects in publicly available pulse oximeter databases according to two age groups: children (<16 years) and adult (≥16 years). Two examples of photoplethysmogram waveforms collected via fingertip pulse oximetry probe (left: 15 years old and right: 50 years old) is shown for each age group. Note that the dicrotic notch is more salient in children compared to adults (i.e., the pulse becoming more triangulated with age).
On examining all of the 12 databases as reported in Table 1, there is only one database (i.e., CapnoBase database) that has data recorded from the children age group, specifically 29 out of 42 subjects. Referring to all the above-mentioned reasons, it can be clearly stated that there is an age bias while recording data, which makes the evolution of devices such as pulse oximeters and systems for detecting vascular disease more biased toward the age category (16 years and above).
Most of the machine learning algorithms, developed to detect abnormalities, are published on publicly available databases. Even the FDA-approved PPG-based devices use publicly available databases for validation. If the publicly available databases are biased in terms of age, it is expected that all these algorithms will be developed for a specific age group. This point, to our knowledge, is not addressed by the FDA yet, and it is essential to raise awareness so researchers can add “in adults” in their titles, for example, or in the discussion.
We argue that since pulse oximetry measurements are particularly susceptible to age, caution should be taken when using these data to create computer algorithms for patient monitoring or diagnosis.
Author Contributions
ME designed and led the study. ME, RF, HT, JA, RW, and CM conceived the study. All authors approved final manuscript.
Funding
This work was supported by Canada Research Chair program and the Canadian Institutes of Health Research (CIHR) agency.
Conflict of Interest
The authors declare that the research was conducted in the absence of any commercial or financial relationships that could be construed as a potential conflict of interest.
Publisher's Note
All claims expressed in this article are solely those of the authors and do not necessarily represent those of their affiliated organizations, or those of the publisher, the editors and the reviewers. Any product that may be evaluated in this article, or claim that may be made by its manufacturer, is not guaranteed or endorsed by the publisher.
References
1. Sjoding MW, Dickson RP, Iwashyna TJ, Gay SE, Valley TS. Racial bias in pulse oximetry measurement. N Engl J Med. (2020) 383:2477–8. doi: 10.1056/NEJMc2029240
2. The U.S. Food and Drug Administration. Pulse Oximeter Accuracy and Limitations: FDA Safety Communication. (2021). Available online at: https://www.fda.gov/medical-devices/safety-communications/pulse-oximeter-accuracy-and-limitations-fda-safety-communication. (accessed on March 01, 2020).
3. Pulse oximetry may be inaccurate in patients with darker skin. Am J Nurs. (2021) 121:16. doi: 10.1097/01.NAJ.0000742448.35686.f9
4. Elgendi M, Fletcher R, Liang Y, Howard N, Lovell NH, Abbott D, et al. The use of photoplethysmography for assessing hypertension. NPJ Digit Med. (2019) 2:60. doi: 10.1038/s41746-019-0136-7
5. Peltokangas M, Vehkaoja A, Huotari M, Verho J, Mattila VM, Röning J, et al. Combining finger and toe photoplethysmograms for the detection of atherosclerosis. Physiol Meas. (2017) 38:139–54. doi: 10.1088/1361-6579/aa4eb0
6. Pimentel MAF, Johnson AEW, Charlton PH, Birrenkott D, Watkinson PJ, Tarassenko L, et al. Toward a robust estimation of respiratory rate from pulse oximeters. IEEE Trans Biomed Eng. (2017) 64:1914–23. doi: 10.1109/TBME.2016.2613124
7. Jarchi D, Casson AJ. Description of a database containing wrist PPG signals recorded during physical exercise with both accelerometer and gyroscope measures of motion. Data. (2017) 2:1. doi: 10.3390/data2010001
8. Johnson AE, Pollard TJ, Shen L, Lehman LW, Feng M, Ghassemi M, et al. MIMIC-III, a freely accessible critical care database. Sci Data. (2016) 3:160035. doi: 10.1038/sdata.2016.35
9. Siam A, Abd El-Samie F, Abu Elazm A, El-Bahnasawy N, Elbanby G. Real-World PPG dataset. Mendeley Data. (2019). doi: 10.17632/yynb8t9x3d.1
10. Liang Y, Chen Z, Liu G, Elgendi M. A new, short-recorded photoplethysmogram dataset for blood pressure monitoring in China. Sci Data. (2018) 5:1–7. doi: 10.1038/sdata.2018.20
11. Reiss A, Indlekofer I, Schmidt P, Van Laerhoven K. Deep PPG: large-scale heart rate estimation with convolutional neural networks. Sensors. (2019) 19:3079. doi: 10.3390/s19143079
12. Schmidt P, Reiss A, Duerichen R, Marberger C, Van Laerhoven K. Introducing WESAD, a multimodal dataset for wearable stress and affect detection. in Proceedings of the 20th ACM International Conference on Multimodal Interaction. Boulder, CO (2018) p. 400–8. doi: 10.1145/3242969.3242985
13. Charlton PH, Bonnici T, Tarassenko L, Clifton DA, Beale R, Watkinson PJ. An assessment of algorithms to estimate respiratory rate from the electrocardiogram and photoplethysmogram. Physiol Meas. (2016) 37:610–26. doi: 10.1088/0967-3334/37/4/610
14. Zhang Z, Pi Z, Liu B. TROIKA: a general framework for heart rate monitoring using wrist-type photoplethysmographic signals during intensive physical exercise. IEEE Trans Biomed Eng. (2014) 62:522–31. doi: 10.1109/TBME.2014.2359372
15. Luque J, Cortès G., Segura C, Maravilla A, Esteban J, Fabregat J, et al. END-to-END photoplethysmography (PPG) based biometric authentication by using convolutional neural networks. In: 2018 26th European Signal Processing Conference (EUSIPCO). Rome (2018) p. 538–42. doi: 10.23919/EUSIPCO.2018.8553585
16. Karlen W, Raman S, Ansermino JM, Dumont GA. Multiparameter respiratory rate estimation from the photoplethysmogram. IEEE Trans Biomed Eng. (2013) 60:1946–53. doi: 10.1109/TBME.2013.2246160
Keywords: digital health, pulse oximetry, intensive care, elderly population, anesthesia, photoplethysmography, PPG signal analysis, PPG waveform
Citation: Elgendi M, Fletcher RR, Tomar H, Allen J, Ward R and Menon C (2021) The Striking Need for Age Diverse Pulse Oximeter Databases. Front. Med. 8:782422. doi: 10.3389/fmed.2021.782422
Received: 24 September 2021; Accepted: 08 November 2021;
Published: 01 December 2021.
Edited by:
Ovidiu Constantin Baltatu, Anhembi Morumbi University, BrazilReviewed by:
Colin K. Drummond, Case Western Reserve University, United StatesRossella Rizzo, Trinity College Dublin, Ireland
Branko Celler, University of New South Wales, Australia
Copyright © 2021 Elgendi, Fletcher, Tomar, Allen, Ward and Menon. This is an open-access article distributed under the terms of the Creative Commons Attribution License (CC BY). The use, distribution or reproduction in other forums is permitted, provided the original author(s) and the copyright owner(s) are credited and that the original publication in this journal is cited, in accordance with accepted academic practice. No use, distribution or reproduction is permitted which does not comply with these terms.
*Correspondence: Mohamed Elgendi, bW9lLmVsZ2VuZGlAaGVzdC5ldGh6LmNo