- 1Ocular Surface Research Group, Singapore Eye Research Institute, Singapore, Singapore
- 2Corneal and External Eye Disease Service, Singapore National Eye Centre, Singapore, Singapore
- 3Eye-Academic Clinical Programme, Office of Clinical, Academic and Faculty Affairs, Duke-NUS Medical School, Singapore, Singapore
- 4Department of Ophthalmology, Yong Loo Lin School of Medicine, National University of Singapore, Singapore, Singapore
- 5Singapore Centre for Environmental Life Sciences Engineering (SCELSE), Nanyang Technological University, Singapore, Singapore
- 6Lee Kong Chian School of Medicine (LKCMedicine), Nanyang Technological University, Singapore, Singapore
- 7School of Biological Sciences, Nanyang Technological University, Singapore, Singapore
- 8School of Medicine, Southern University of Science and Technology, Shenzhen, China
- 9Life Sciences Institute, National University of Singapore, Singapore, Singapore
- 10Centre for Marine Science and Innovation, School of Biological Earth and Environmental Sciences, University of New South Wales, Kensington, NSW, Australia
The ocular surface microbiome has implications for ocular surface inflammation and immunology. Previous shotgun metagenomics analyses were performed in China, showing results that differed according to environment and age. Patients with Sjogren’s syndrome were reported to have altered conjunctival microbiome, but such studies have not been done in milder dry eye. The aim of this study is to describe the conjunctival microbiome in people with mild dry eye in Singapore. Samples were collected from 14 participants with mild dry eye and 10 age-matched comparison participants recruited from Singapore National Eye Centre (SNEC) clinics. Shotgun metagenomic sequencing analysis was employed to evaluate the conjunctival microbiome composition. Proteobacteria formed the predominant phylum in the conjunctiva. As in a study from a coastal city in China, Achromobacter spp. was numerically most abundant. Compared to age-matched controls, the conjunctival microbial composition in mild dry eye was similar. Several microorganisms, including Streptococcus spp. increased in representation with age, and the abundance of Staphylococcus correlated with Schirmer readings. In addition, when cultured corneal epithelial cells were exposed to three strains of Achromobacter xylosoxidans, cytokines such as TNF-α and IL-6 were upregulated in the cell lysates and supernatants. Ourresults suggest that age is an important factor that affects composition of the conjunctival microbiome, and relative abundance of specific microorganism may vary according to the environment of the human host.
Introduction
The human ocular surface consists of the conjunctiva, corneal surfaces, the lid margin, tear secreting glands and outflow tracts. The ocular surface microbiome is important for several reasons. First, it has been linked to various human eye diseases such as dry eye (1–4), Sjogren’s syndrome (5, 6), allergic conjunctivitis (7, 8), trachoma (9) and infectious keratitis (10, 11). Second, microbial components in the eye are known to stimulate toll-like receptors on the ocular surface epithelial cells, thereby activating the ocular surface immune system (12–15). The conjunctival-associated lymphoid tissue and lymphatics in the conjunctiva (16–19) may play an analogous role to the Peyer’s patches in the small intestine for the regulation of immune tolerance (20–23). Topical probiotic eyedrops containing Lactobacillus acidophilus reduces severity of ocular allergies (24) in humans, while the use of topical eyedrops with Enterococcus faecium and Saccharomyces boulardii showed improvement in signs of dry eye in a clinical trial (25), suggesting that modulation of ocular surface inflammation via microbial strategies is possible. Furthermore, an oral probiotic formulation has been shown to reduce severity of experimental dry eye (26–28). Third, the role of ocular surface microorganisms in eyelid conditions like blepharitis and eye infections is well documented (29).
Conventional culture-based studies of the ocular surface have revealed a predominance of Gram-positive cocci such as coagulase-negative Staphylococci, Diphtheroids, and anaerobes, including Propionibacter acnes (30–32). Studies with 16S rRNA amplicon sequencing have reported a slightly different community profile, with more Gram-negative bacteria (4, 9, 33–35). Although 16S rRNA amplicon-based microbiome studies allowed a broad description of bacterial community, this method has low phylogenetic resolution at the species level (36). Shotgun metagenomic analysis sequences the whole community DNA, and allows the community profiling of the bacteria to species level (37–41). Furthermore, whole genome information enables analyses of metabolic and functional pathways. One such study reported that pathways related to transcription, lipid and amino acid metabolism were abundant in the healthy ocular surface microbiome (37). Previous studies using shotgun metagenomic methods showed that environmental factors impact healthy ocular surface microbiome (38). Since the previous studies using shotgun metagenomics were from China, it is necessary to replicate them in a different climate.
As inflammation is the most common cause of ocular surface disease, understanding how microbiomes relate to different types of inflammation is necessary for effective treatment. There are implications for clinical practice in ocular allergies, eyelid-induced ocular surface inflammation, contact lens wear, extended corticosteroid use, as well as prophylaxis against iatrogenic infections related to surgeries (31, 35, 42). It is known that SS patients have altered ocular surface microbiome, but this has not been studied in mild dry eye.
This study aimed to describe ocular surface microbiome in a group of people with mild dry eye and comparison participants living in Singapore, explore its association with demographic and parameters related to ocular surface health. In order to understand effect of the microbes to ocular surface inflammation, the impact of preponderant microbial species on cytokine expression by ocular surface epithelial cells was investigated.
Materials and methods
Participant recruitment
The study was approved by the SingHealth centralized Institutional Review Board, and complied with the tenets of the Declaration of Helsinki for human research. Informed written consent was obtained from all participants. Participants who had been diagnosed to have dry eye had a spectrum of results on clinical tests, with presence of dry eye symptoms and one clinical sign (either Schirmer I test results < 10 mm or NIBUT < 10 s or presence of corneal staining). Supplementary Table 1 showed clinical features of the participants. These dry eye cases were referred to as mild because all participants were level 1 except one with level 2 in the DEWS 2007 severity criteria (43). Participants with diabetes were excluded because they could have altered microbiomes (44, 45). Participants in the comparison group did not satisfy the above dry eye criteria and presented to Singapore National Eye Centre (SNEC) for an unrelated eye condition such as floaters.
The participants were evaluated with a questionnaire that identified risk factors of dry eye such as contact lens use, smoking (46). The use of artificial tears and contact lens wear are provided in Supplementary Table 2. None of the dry eye participants had treatment with prescription eye drops (corticosteroids, cyclosporine or antibiotics). The control group had no topical treatment including artificial tears and antibiotics, and no contact lens wear for the past 6 months.
Clinical examination
Keratograph (K) 5M (Oculus Inc., Arlington, WA) was used to assess the non-invasive tear breakup time (NIBUT). The machine’s algorithm monitored the tear film to document the time and site of tear film breakup. This technique analyzed reflection images of Placido rings at intervals after eye opening, to measure the smoothness of the tear-air interface. Tear irregularity presents as disruption of the images of the rings. A lower value of NIBUT indicates increased tear evaporation or greater tear instability.
Bulbar conjunctival and peri-limbal eye redness was also assessed by K5M (47). An image of the ocular surface under white light was acquired with the K5M and automatically given a standard grading for conjunctival hyperemia, ranging from 0 (no redness) to 4.0 (maximum redness). This analysis also provided separate measures of redness of the temporal and nasal conjunctiva.
Baseline tear secretion was measured by Schirmer’s test without anesthesia as described previously (48). A lower Schirmer I value indicates less tear secretion. The corneal fluorescein staining was performed and graded as previously described (46, 49).
Specimen collection
A drop of non-preserved tetracaine was firstly instilled into the conjunctival fornix. After the stinging sensation had resolved, a sterile cotton swab was used to collect the microbes from the lower conjunctival fornix using a gentle rolling action (up to eight strokes). The procedure was then repeated for the opposite eye. The cotton swabs from both eyes were combined and then soaked in 650 μl of DNA/RNA Shield (Zymo Research Corp., Irvine, CA) reagent, immediately homogenized for 30 s, transferred to ice for 1 min, and further homogenized for another 30 s. Homogenized samples were stored at 4°C until further processing (within 1 week). Total DNA was extracted with ZR-Duet DNA/RNA MiniPrep (Zymo Research, Irvine, CA). Empty swabs following the same procedure were used as control. An empty swab was an unused swab that was opened under the same room and conditions as the participants and then homogenized and processed as if it has been used on a participant.
Metagenomic library preparation and sequencing
DNA quality and quantity were determined using a 2100 Bioanalyzer and the Invitrogen PicoGreen assay, respectively. Library preparation was performed according to the Illumina TruSeq Nano DNA Sample preparation protocol. The samples were sheared on a Covaris S220 (Covaris, Woburn, MA, USA) to ∼450 bp, following the manufacturer’s recommendation, and each uniquely tagged with one of Illumina’s TruSeq LT DNA barcodes. Sequencing was performed on the Illumina HiSeq 2500 platform (Illumina, San Diego, CA, USA) resulting in an average of 56.18 million (49.29–77.79 M) 250 bp paired-end reads per sample. Sequence data were deposited in the Sequence Read Archive and are available under the BioProject PRJNA886972.
Read preprocessing
Illumina adaptors were removed using cutadapt (version 1.10). Low-quality reads were removed using the program “iu-filter-quality-minoche.” Overall, quality-trimmed reads represented 83% (81–86%) of DNA reads. Human reads were then removed from the dataset by aligning DNA reads to the human genome (h38 from NCBI: GCF_000001405.36_GRCh38.p10_genomic.fna.gz) using Bowtie2 (version 2.2.9). Overall, around 0.11 million (0.04–0.27 million) DNA reads were retained after quality filtering and removal of human reads.
Taxonomic and functional profiling of the ocular microbiome
Taxonomic composition of DNA reads was characterized by alignment against the NCBI non-redundant (NR) protein database (March 2016)1 using DIAMOND (version 0.7.10.59) with default parameters. The lowest common ancestor approach implemented in MEGAN6 (version CE_6_5_5, -ms 100 -supp 0 –sup 25 –pr –ps 2) was used to assign reads at the phylum, genus and species levels. Each aligned read was assigned a KEGG KO number using KEGG to GI mapping file generated using KEGG 01/04/2016 repository according to MEGAN manual.
Statistical analysis
Phylum, genus, species and KEGG count tables from the metagenomic dataset were exported from MEGAN6 and imported in R using the phyloseq package. Count tables were filtered to remove taxa/KO accounting for less than 10 sequences in total and observed in less than two samples (filter_taxa function). Taxonomic and functional tables were then rarefied to an even sequencing depth using the rarefy_even_depth function to allow robust comparison between samples. Microbial communities were characterized using alpha-diversity indices (number of observed taxa/K0 and Shannon diversity indices) and beta-diversity (Bray-Curtis dissimilarity) for taxonomic (phylum, genus and species levels) as well as functional datasets.
In order to investigate the correlation between microbial communities and ocular parameters, distance-based redundancy analysis models (db-RDA) were conducted between scaled ocular parameters and taxonomical or functional Bray-Curtis dissimilarities. The statistical significance was assessed by 999 permutations of the reduced model. Spearman correlations were conducted between ocular parameters and taxa/KO. Statistical significance of taxa/KO-group association was tested using the “signassoc function” from the “indicspecies” package. Sidak’s correction was applied for multiple testing.
Cell culture and treatment
Achromobacter xylosoxidans strains were obtained from ATCC (DSMZ, Braunschweig, Germany). Human corneal epithelial cells (H-CET) were cultured to near confluency in DMEM/F12 medium containing 5% FBS (Life Technology, Singapore) media, with passaging and maintenance of culture conditions as described in previous studies (50). Before experiments, cells were washed, seeded into serum-free DMEM/F12 medium and grown overnight.
For treatment experiments, the following conditions were tested in triplicates: (1) DMEM only: H-CET control; (2) DMEM + 1μg/ml LPS: H-CET activated with 1μg/ml LPS (Sigma) for 16 h; (3) Achromobacter xylosoxidans strain 1 or 2 or 3 + 1μg/mL LPS: H-CET activated with 1μg/mL LPS for 16 h, followed by infection of 1:100 MOI of the strain of Achromobacter for 2 h. The extracellular bacteria were then removed by washing three times with PBS and the H-CET were then incubated in DMEM for another 3 h; (4). The same conditions as in (3) were investigated, but without the addition of the LPS.
Cytokine analysis
The cell culture media and cells were collected separately for analysis. As for the cell culture media supernatant, the liquid was transferred to a new 1.5 ml Eppendorf tube, centrifuged at 13,000 g for 3 min. The supernatant was then filtered with 0.2 μm-filter. The cells were washed three times with 200 μL PBS/well, lysed by addition of 200 μL of ddH2O/well and scraped from the bottom of the well with a cell scraper. The cell lysates were transferred to a new 1.5 mL Eppendorf tube, centrifuged at 13,000 g for 3 min. The supernatant was then filtered with the 0.2 μm-filter.
Cell lysate protein concentrations were determined by the bicinchoninic acid method. The same volume of supernatant (25μL/well) and same amount of cell lysate proteins (29.5μg/well) from each sample were used for multiplex bead-based indirect immunofluorescent assay (Beadlyte; EMD Millipore, Billerica, MA, USA) as described previously (51). Each sample was triplicated. Levels of 15 cytokines (IL-1β, IL-2, IL-4, IL-6, IL-8, IL-10, IL-12, IL-13, IL-17, IFN-γ, TNF-α, IP-10, MCP, MIP1a, RANTES) were analyzed.
Results
There were no significant differences in age, gender or ethnicity of participants with dry eye and controls (Table 1). The dry eye participants recruited in this study were mainly mild in severity, with presence of dry eye symptoms and one clinical sign (either reduced Schirmer I test results or NIBUT or presence of corneal staining). Since the participants had an abnormal result in only one out of these tests, each test displayed a wide range of readings across the entire group (Table 1). Among the ocular surface clinical parameters, the extent of conjunctival redness was directly correlated to increased age. Age showed a bimodal distribution with one peak below and another above 40 years of age (Supplementary Figure 1).
Characterization of the microbiome
Sequencing reads were obtained in each of the 24 samples, as summarized in Table 2. Of 56,181,504 raw reads, most of the reads were human in origin and 1,246,495 were matched to identified microbial phyla. The top major phyla identified in these participants are shown in Supplementary Figure 2. The phylum Proteobacteria represents the most abundant phylum, followed by Bacteroidetes. The most common fungus phylum was Basidiomycota (which includes free living organisms and Cryptococcus). The bacterial phylum Firmicutes (which includes the Clostridia, Streptococcus, Staphylococcus and Lactobacillus) was significantly over-represented among the older (>40 years of age), compared to younger (<40 years of age) participants (Supplementary Figure 3). Bacterial reads outnumbered fungal and viral reads (data not shown).
The most abundant genus was Achromobacter, with the most common species identified as Achromobacter xylosoxidans (Supplementary Figure 4). There were no significant differences in abundance of any microbial genus, or species between dry eye and control participants, among the top 10 genera and species. The most abundant phylum and genus from the empty swabs control are showed in Supplementary Figure 5. Achromobacter was not among the top 10 genera in empty swab control samples.
Two clusters of participants were identified using principal component analysis (Figure 1), one large cluster (16 participants) and a small but more dispersed cluster of eight participants (MBD016LE, MBD036LE, MBC044LE, MBD037, MBD030LE, MBC022L, MBC023LE, MBD032LE). There were no significant differences in the dry eye parameters between participants of the two clusters, although participants of the smaller cluster (n = 7) were of an older age (p = 0.028). The ages in the two clusters were 39.6 ± 13.6 years and 53.5 ± 13.7 years, respectively. The smaller cluster (Figure 2 left) had an increased representation of Achromobacter spp. and a reduced proportion of a number of microbial species (e.g., Acidovorax temperans, Phenylobacterium zucineum, and Noviherbaspirillum spp.) compared to the larger cluster (Figure 2 right). Supplementary Table 3 listed all the bacteria that are under-represented in the smaller cluster.
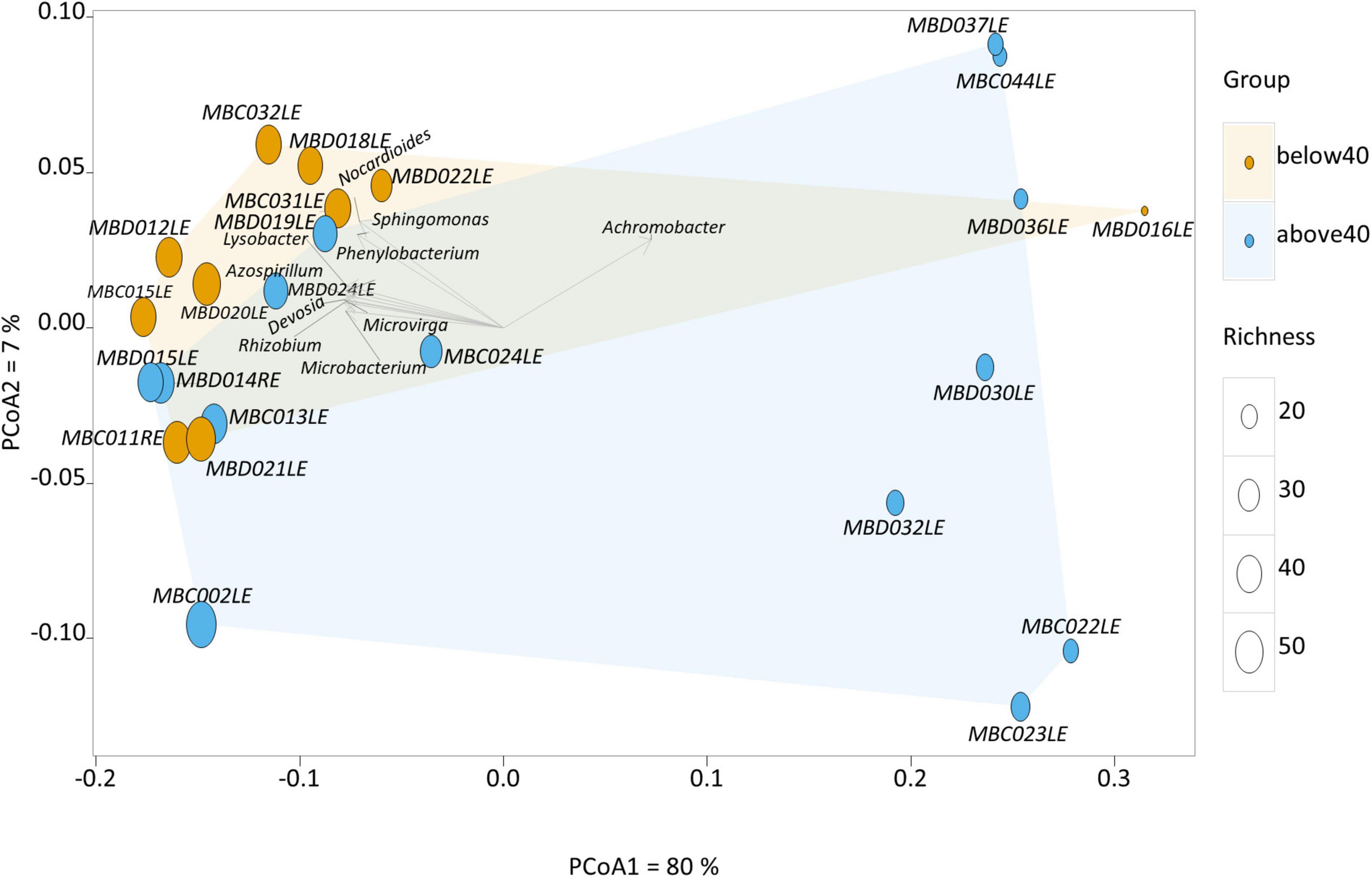
Figure 1. Principal component analysis of the human conjunctival microbiome composition. This scatter diagram shows each participant (symbol) along the first two principal components of the microbial gene analysis at the genus level. The age of the participant is displayed as either orange (younger) or blue (older), and richness is displayed as differing sizes of the symbols. Certain bacterial genera contribute significantly to loading scores in the first principal component (horizontal axis) illustrated in the scatter diagram.
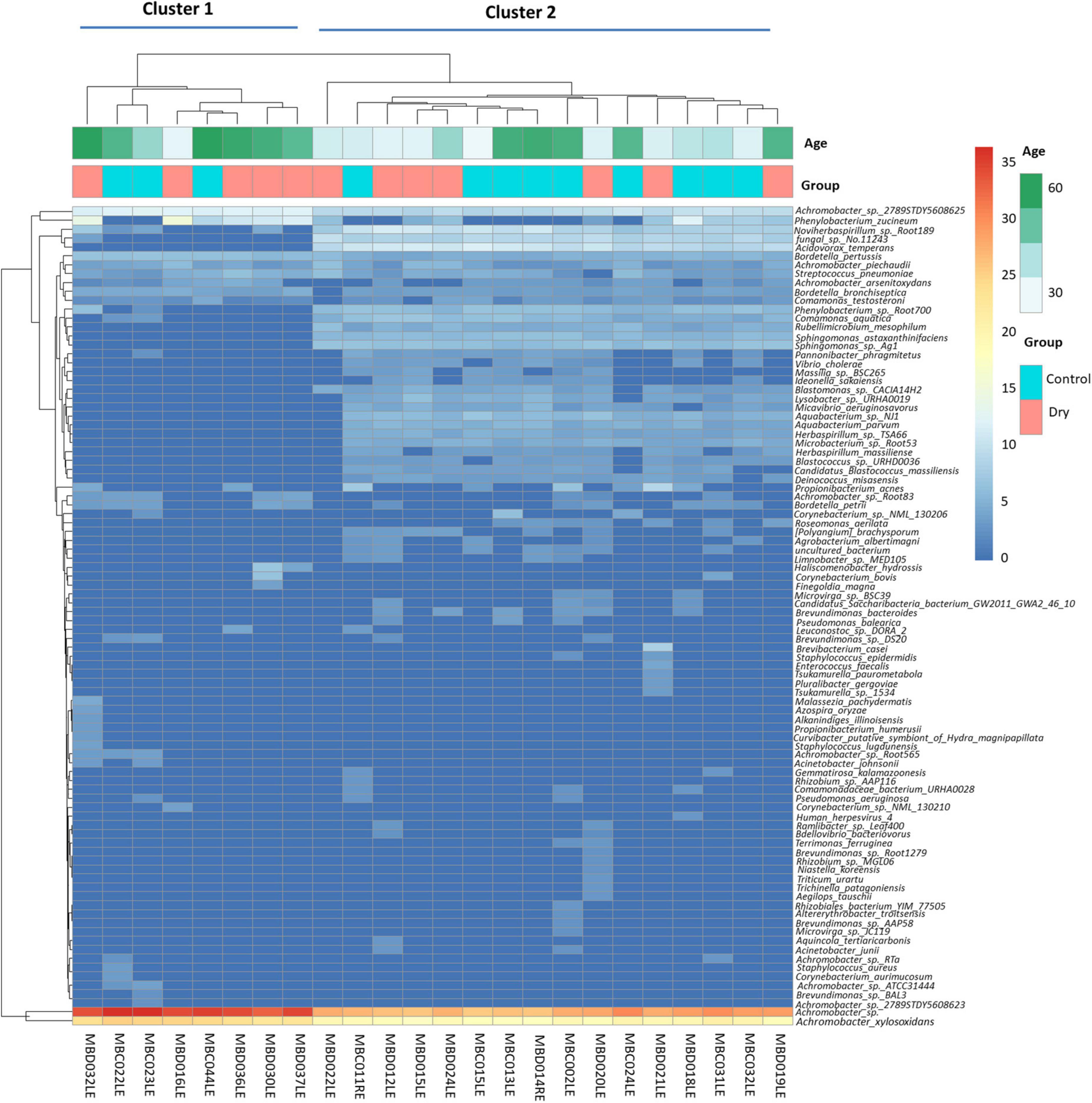
Figure 2. Heat maps showing hierarchical clustering of abundance of microbial species in the human conjunctival microbiome. This shows two distinct clusters (cluster 1: eight patients, and cluster 2: 16 patients). The identity of the species is indicated on the vertical axis and each column represents one participant. Horizontal axis annotation (top) shows the age of the participants and the dry eye category.
It is interesting to examine the bacteria under-represented in the smaller cluster in greater detail, as the age effect on this bacterial community may have physiological relevance, even though individual microbes may not play a significant role. On closer examination, this group of 23 bacterial species contain mainly bacteria from the phylum Proteobacteria, with the exception of four species. These exceptions were Deinococcus misasensis, Rubellimicrobium mesophilum and Candidatus Blastococcus massiliensis; a fourth species, Micavibrio aeruginosavorus which, is a known epibiotic obligate bacterial predator that feeds on potential disease-causing bacteria such as Pseudomonoas aeruginosa. A reduction in abundance of M. aerugonosavorus may have health effects, since the protective functions of M. aeruginosavorus may be reduced as its relative numerical composition is decreased.
Association with clinical parameters
In univariate analyses, we found significant correlations between abundance of certain microbial genera and clinical parameters (Figure 3). For example, older age was correlated with a higher abundance of Streptococcus spp. (p < 0.01), and a lower abundance of Staphylococcus was correlated with a reduced Schirmer’s test reading (p < 0.01, First 2 rows Figure 3A), which indicates the presence of aqueous tear deficient dry eye.
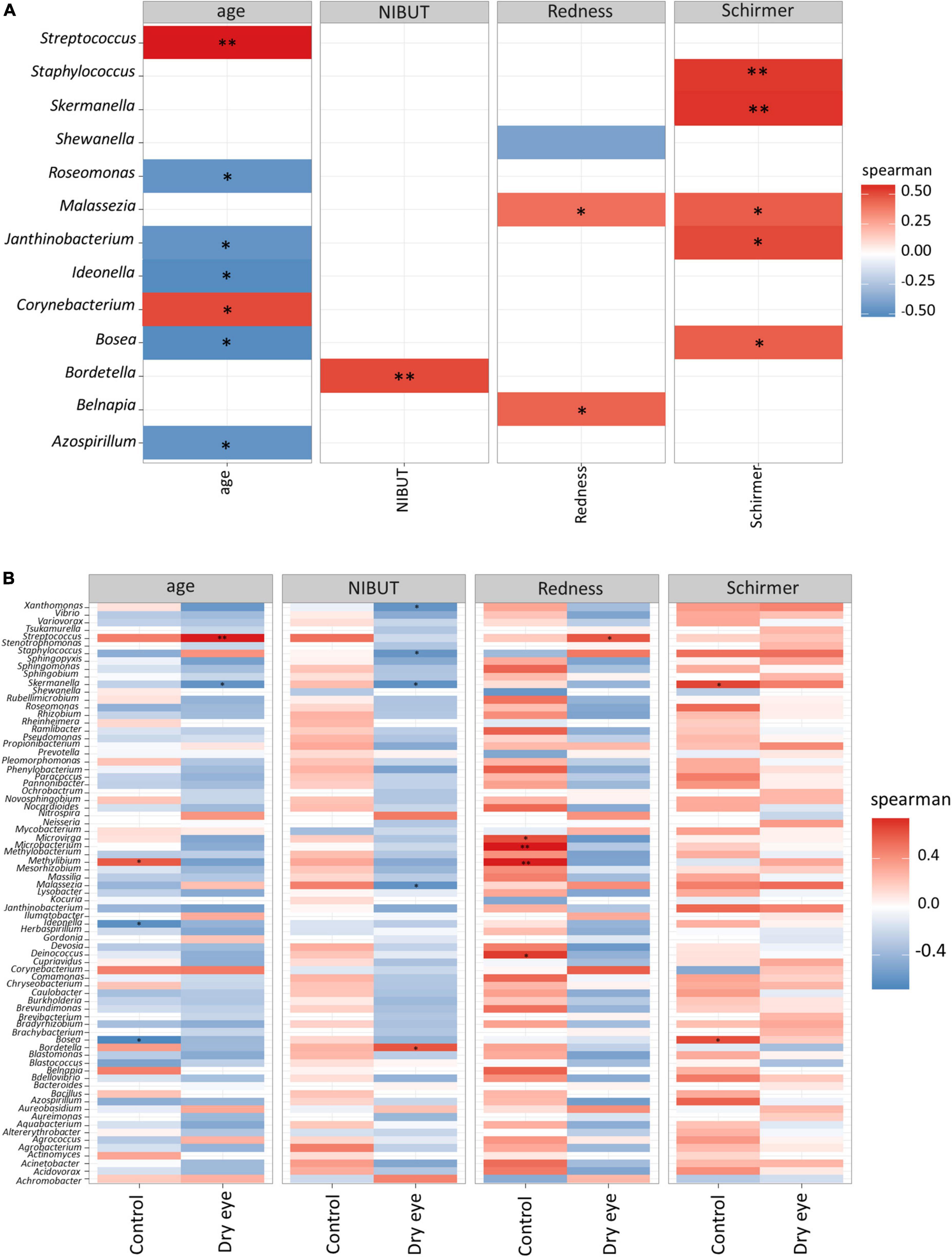
Figure 3. Correlation between microbial genera and demographic/clinical parameters. Each horizontal row indicates a unique microbial genus, and the horizontal axis indicates the correlation analysis with age, and three other clinical parameters. NIBUT: Non-invasive tear break up times (in seconds), redness: average temporal bulbar redness quantified automatically by Oculus Keratograph K5M; Schirmer: Schirmer I test results (mm over 5 min). The value of the Spearman correlation coefficient, which measures the strength and direction of association between two ranked variables, is color coded. Strong red color indicates positive correlation and strong blue color indicates negative or inverse correlation. Weak or faint color indicates weak or no correlation. (A) All participants included in analysis, and (B) Correlation stratified according to dry eye status (with or without dry eye). *p < 0.05; **p < 0.01.
Although Staphylococcus was not significantly correlated to age (second row Figure 3A), however, in older participants a few cases of Firmicutes (which included Staphylococcus) exceeded 10% relative abundance (Supplementary Figure 3). There is still a possibility that age may confound the relationship of Staphylococcus with Schirmer results, since it is well known that older age is weakly associated with reduced Schirmer readings (48, 52).
The fungal genus Malassezia also showed a similar, though less marked association with the Schirmer’s test result (p < 0.05, Figure 3A). Interestingly, when the same data were stratified by dry eye status, certain correlations remained in the dry eye group but not in the control group (Figure 3B). For example, the genus Streptococcus was highly correlated to age (p < 0.01), only in the dry eye group but not in the control group. On the other hand, the genus Microbacterium was increased in participants with more severe conjunctival redness (p < 0.01), but only in the control group, not for the dry eye participants.
At the species level, significant correlations were also observed (Figure 4) between the increased proportion of some species with older age and reduced proportion of other species with increased conjunctival redness (Figure 4A). When the results were stratified by dry eye status, some differential findings were observed between the control and dry eye groups (Figure 4B).
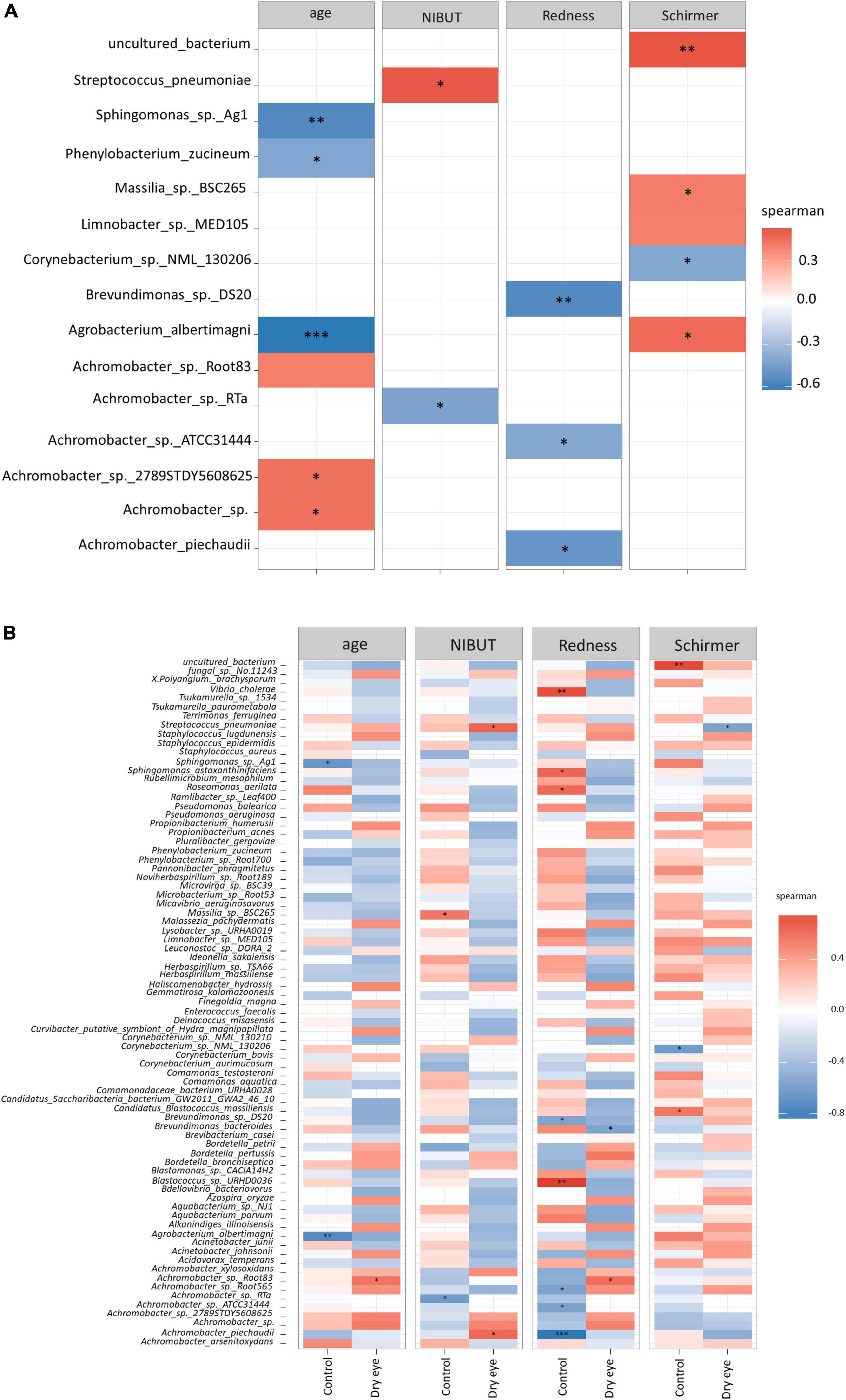
Figure 4. Correlation between microbial species and demographic/clinical parameters. Each horizontal row indicates a unique microbial species, and the horizontal axis indicates the correlation analysis with age, and three other clinical parameters. NIBUT: Non-invasive tear break up times (in seconds), Redness: Average temporal bulbar redness quantified automatically by Oculus Keratograph K5M; Schirmer: Schirmer I test results (mm over 5 min). The value of the Spearman correlation coefficient, which measures the strength and direction of association between two ranked variables, is color coded. Strong red color indicates positive correlation and strong blue color indicates negative or inverse correlation. Weak or faint color indicates weak or no correlation. (A) All participants included in analysis, and (B) Correlation stratified according to dry eye status (with or without dry eye). *p < 0.05; **p < 0.01; ***p < 0.001.
Next, we analyzed the abundance of functional genes not aligned with human genomes. The assessment of composition and abundance of functional genes can elucidate potential differences in microbial function. Such functional rather than taxonomic gene description can more accurately describe microbial community composition in specific host cohorts (53, 54). Our analysis revealed that in each of the samples, more than half the classified reads encode for genes related to metabolism, and of the remainder, about two thirds encoded for environmental/processing genes, while one third of the genes was associated with processing of genetic information. Approximately 20–30% of the assigned reads encoded for membrane transport, with 25% of these for ABC transporters (data not shown). Our analysis did not identify significant differences in the relative proportion of functional genes between the dry eye and control participants (data not shown).
Correlating the functional classifications with clinical parameters revealed that glutathione S-transferase [EC:2.5.1.18] was higher in the controls than in dry eye individuals (p = 0.01). The preprotein translocase subunit SecA (K03070) was positively correlated to the Schirmer’s test result (p < 0.001, Figure 5A and Supplementary Table 4), while the 3-oxoacyl-[acyl-carrier-protein] synthase III protein [EC:2.3.1.180] (K00648) was positively correlated to age (p < 0.001, Figure 5A). The DNA-directed RNA polymerase subunit beta [EC:2.7.7.6] (K03043) was positively correlated to conjunctival redness (p < 0.01, Figure 5B and Supplementary Table 5) in control participants without dry eye.
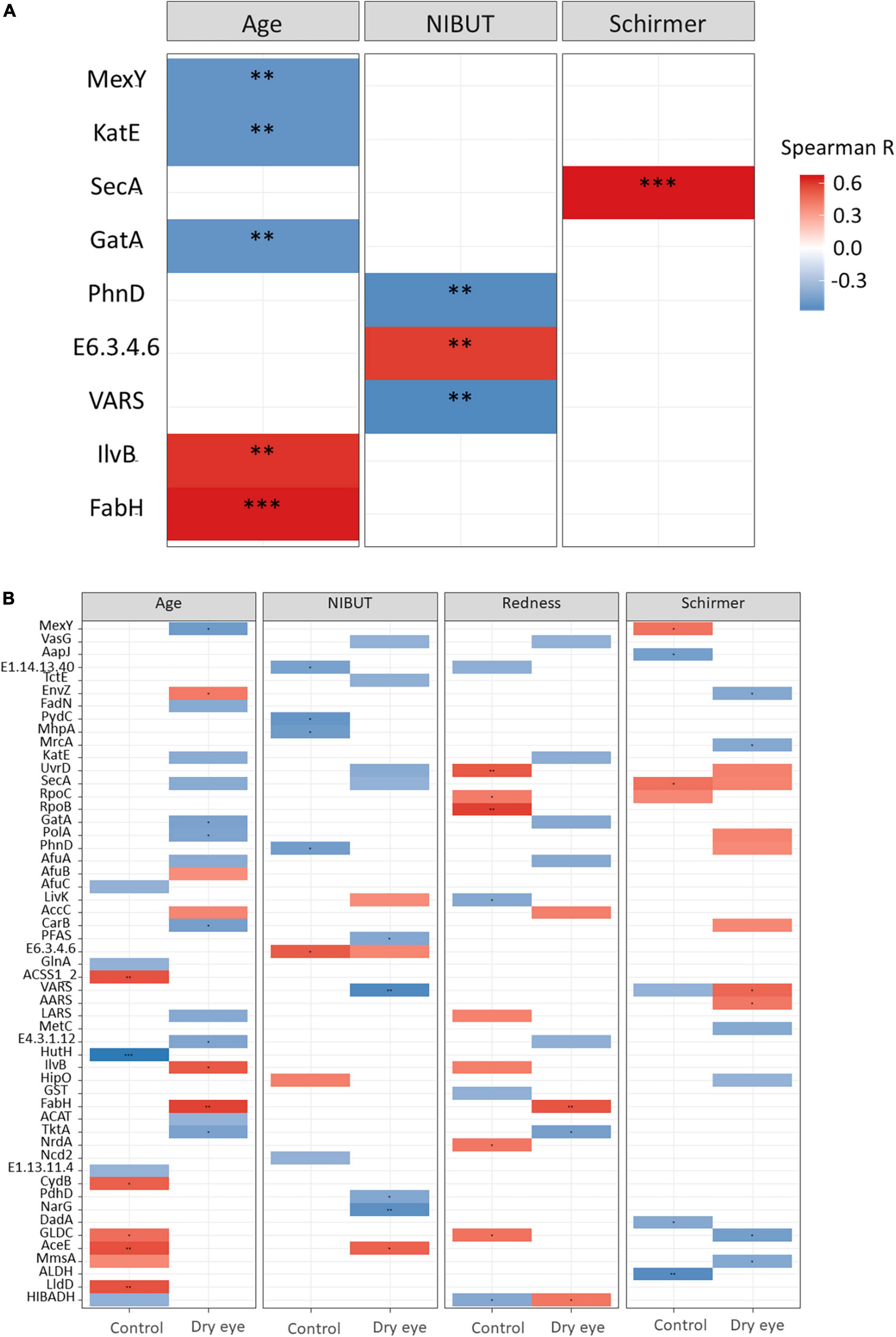
Figure 5. Results of functional gene analysis. (A) Correlation between KEGG functional classes and age, non-invasive tear break-up times (NIBUT) and Schirmer test for all participants. (B) Relationship between functional genes and age, NIBUT, conjunctival redness and Schirmer test, stratified by dry eye and control participants. The value of the Spearman correlation coefficient, which measures the strength and direction of association between two ranked variables, is color-coded. Red indicates positive correlation and blue indicates negative or inverse correlation. *p < 0.05; **p < 0.01; ***p < 0.001. Supplementary Tables 4, 5 listed the full names of KEGG functional classes.
Achromobacter effects the cytokine levels of human cornea epithelial cells
As Achromobacter species are the predominant microorganisms, the potential functional significance of Achromobacter in human ocular surface physiology was examined. To achieve this, human corneal epithelial cells (HCE-T) were cultured with three strains of A. xylosoxidans, the most abundant species.
Addition of any of the three strains of A. xylosoxidans to the culture medium of HCE-T cells induced the upregulation of inflammatory cytokines, including IL-6, IL-8, MCP-1, RANTES, TNF-α, and MIP-1α (Figures 6A–F). Two strains of A. xylosoxidans upregulated IFN-γ (Figure 6G), while only one strain upregulated IP-10 (Figure 6H). When LPS was added to simulate ocular surface stress and the simultaneous presence of other gram-negative bacteria, all three strains of A xylosoxidans further upregulated IL-6, IL-8, MCP-1 and RANTES (Figures 6A–D). Only strain 1 further upregulated TNF-α and IP-10 (Figure 6).
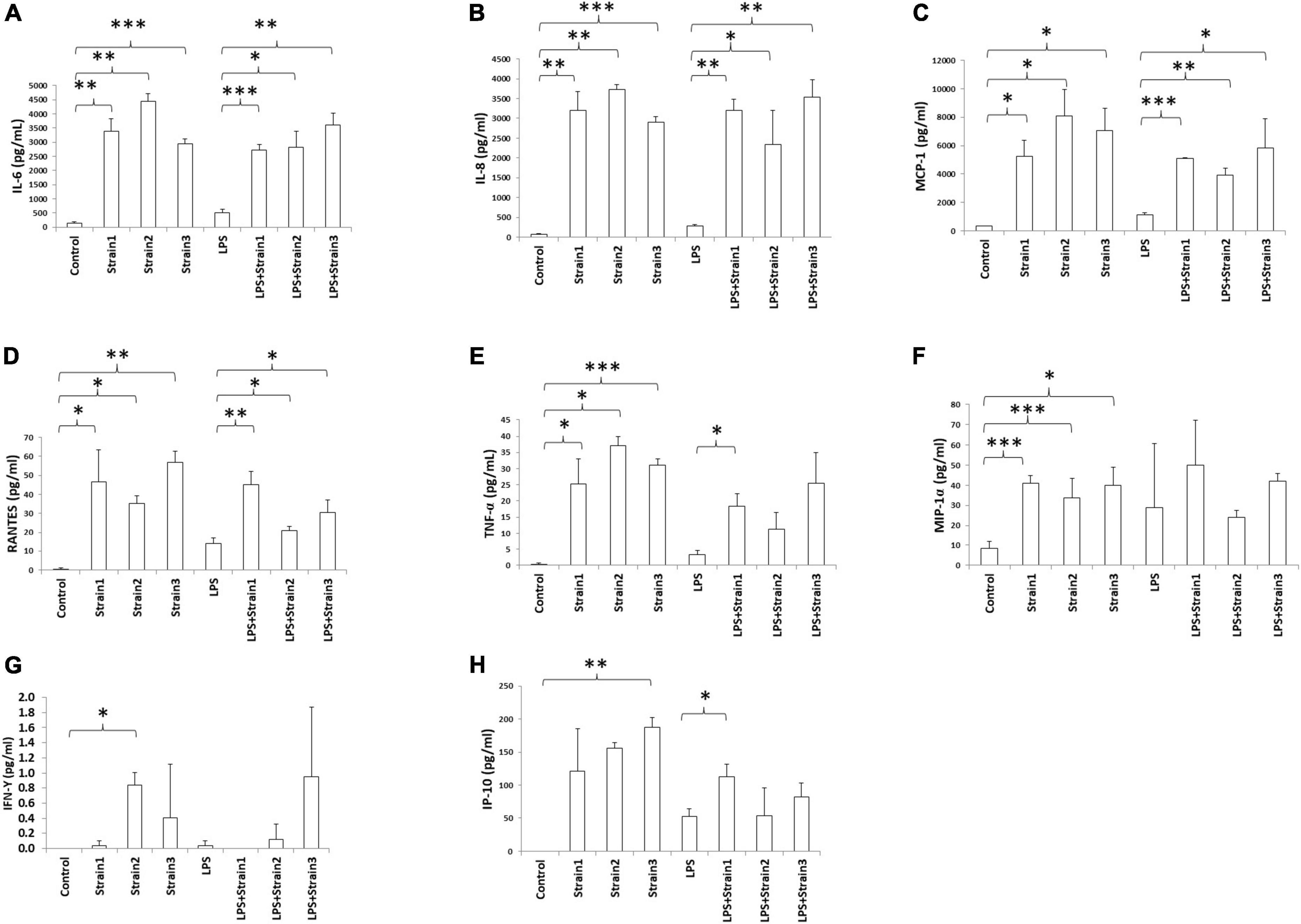
Figure 6. Achromobacter induced cytokine production in human corneal epithelial cells (serum free culture supernatant). (A) IL-6; (B) IL-8; (C) MCP-1; (D) RANTES; (E) TNF-α; (F) MIP-1α; (G) IFN-γ; (H) IP-10. IL, interleukin; TNF-α, tumor necrosis factor alpha; MIP, macrophage inhibitory protein; MCP, monocyte chemotactic protein; IFN, interferon; IP, interferon gamma-induced protein; RANTES, regulated on activation normal T cell expressed and secreted. The height of bars indicates the mean of three biological replicates. The error bars indicate one standard deviation *p < 0.05, **p < 0.01, ***p < 0.001.
The three strains of A. xylosoxidans, when added to the medium of HCE-T cells induced upregulation of MCP-1, RANTES and TNF-α intracellularly (Figures 7A–C). Strains 1 and 2 of A. xylosoxidans upregulated intracellular IL-6 and IL-8 (Figures 7D,E). Strains 1 and 3 upregulated IP-10 whereas only strain 1 upregulated IFN-γ (Figures 7F,G). When LPS was added concurrently, all the strains of A. xylosoxidans further upregulated MCP-1 and IL-8 (Figures 7A,E). Strains 1 and 3 further upregulated TNF-α (Figure 7C), whereas strain 1 further upregulated RANTES, IP-10 and IFN-γ (Figures 7B,F,G).
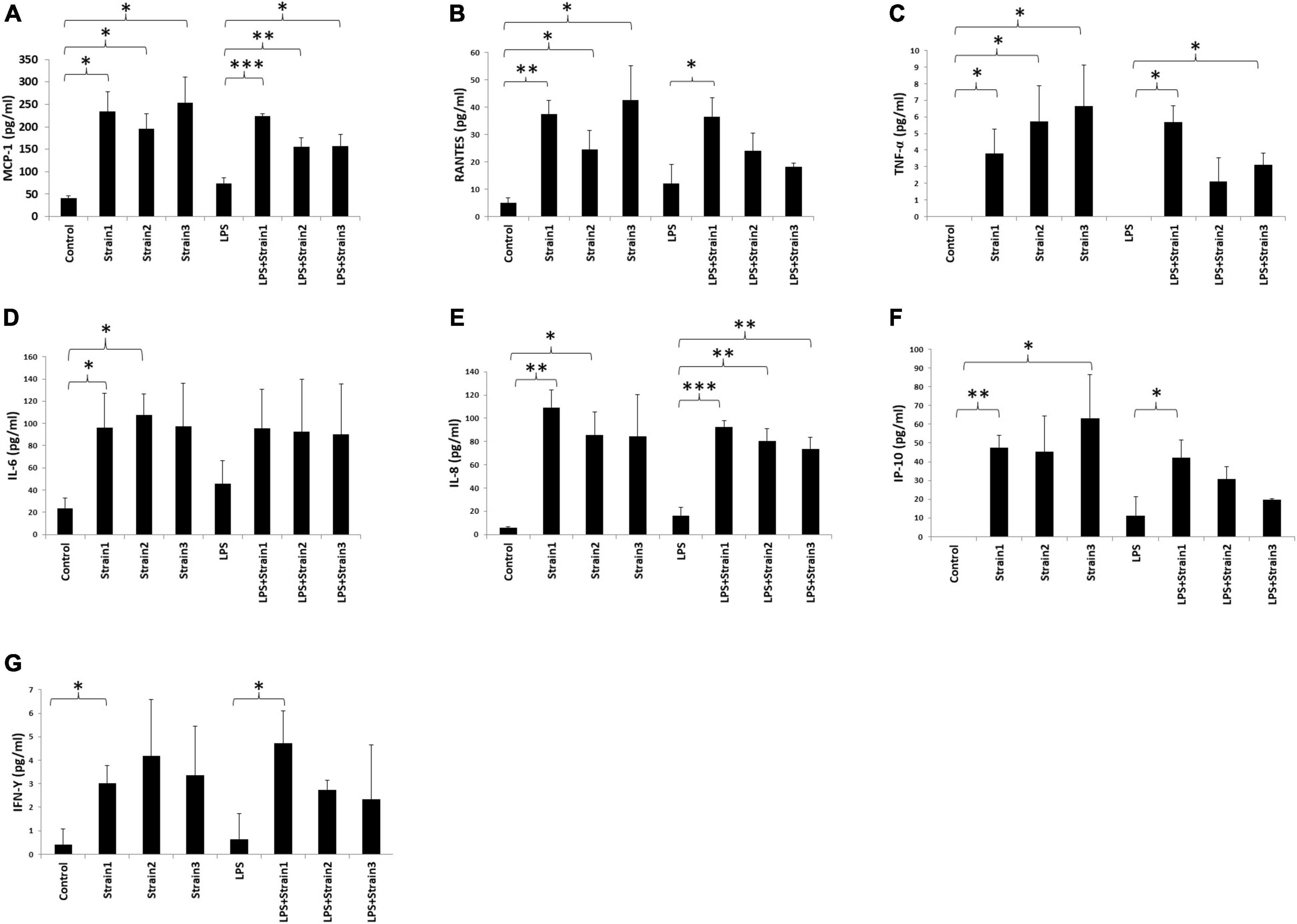
Figure 7. Achromobacter induced cytokine production in human corneal epithelial cells (cell lysates). (A) MCP-1; (B) RANTES; (C) TNF-upalpha; (D) IL-6; (E) IL-8; (F) IP-10; (G) IFN-γ. IL, interleukin; TNF, tumor necrosis factor; MIP, macrophage inhibitory protein; MCP, monocyte chemotactic protein; IFN, interferon; IP, interferon gamma-induced protein; RANTES, regulated on activation normal T cell expressed and secreted. The height of bars indicates the mean of three biological replicates. The error bars indicate one standard deviation *p < 0.05, **p < 0.01, ***p < 0.001.
Hence, addition of A. xyloxidans to corneal epithelial cells led to increased inflammatory cytokine production. In LPS-stimulated cells, cytokine production further increased when bacteria were present. An interesting finding was observed for the regulation of IP-10 and TNF-α by strain 2 and 3 of A. xylosoxidans (Figures 6, 7). Addition of LPS and A. xylosoxidans did not upregulate these cytokines to the same extent as adding only A. xylosoxidans. This suggests that LPS and these two strains of A. xylosoxidans may compete for the same cellular receptor in these epithelial cells.
Discussion
Here we describe a comprehensive study of ocular surface microbiome in participants with mild type of dry eye using shotgun metagenomics. Studies based on 16S rRNA sequencing have indicated that dry eye participants may have a distinct ocular surface microbial community (Supplementary Table 6). This study characterized conjunctival microbiomes with Achromobacter being the numerically dominant bacterial genus. A. xylosoxidans may regulate production of cytokines in corneal epithelial cells. Age has a significant influence on the ocular surface microbiome, with older participants showing an increase in the proportion of bacterial genera such as Achromobacter and Streptococcus, but a reduced proportion of up to 23 bacterial species, mostly from the phylum Proteobacteria. Although there was no significant difference in the composition of conjunctival microbiomes in mild dry eye and control participants, the proportion of certain microbial genera was correlated to tear function, for example, a reduced abundance of Staphylococcus was correlated to decreased Schirmer’s test scores.
During the past 5 years, there was a dramatic increase in studies describing microbial communities with metagenomic analysis. We compared our work with similar studies by conducting literature research in the Medline database using “ocular surface microbiome” as keywords. Only studies performing either 16S rRNA or shotgun metagenomics on human subjects were included. Studies profiling microbial compositions of healthy ocular surfaces are summarized in Table 3. These studies revealed a more diverse microbial community on human ocular surfaces than traditional culture methods. Similar to other mucosal surfaces, the ocular surface microbiota comprises Gram-positive and Gram-negative bacteria, virus and fungi.
Our finding that age is the primary factor affecting the conjunctival microbiome is in agreement with previous studies. In a study investigating ocular surface microbiome of healthy individuals, old and young participants were clearly separated in PCA analysis. Compared to the younger group, the older cohort had significantly greater abundance of several Streptococcus species, and also altered carbohydrate, lipid, nucleotide and amino metabolic pathways (41). Children above 6 months old had a higher abundance of Proteobacteria and reduced Firmicutes than toddlers (<6 months old) (55). Compared to adults, there was an increase of Streptococcus and Staphylococcus OTUs in children below 8 years old (56).
Recently, a study compared conjunctival microbiome compositions of healthy subjects from the three cities Beijing, Wenzhou and Guangzhou, which have distinct climates and diets (38). Shotgun metagenomic sequencing revealed that the conjunctival microbiome of Beijing participants showed distinct characteristics compared to Guangzhou and Wenzhou microbiomes, while there was no significant difference between Guangzhou and Wenzhou participants, suggesting that the environment shapes their conjunctival microbiota. Furthermore, for the participants who have traveled to a different city for at least 15 days, the conjunctival microbiome was markedly changed (38). These findings strongly supported an environmental impact on the composition of conjunctival microbiome. In our study, Achromobacter was the most abundant genus of the conjunctival microbiomes of all subjects. This finding was different from the outcome of the study by Deng et al. (38) possibly due to the different climate and diet in Singapore. In another study conducted in a coastal city from China, Achromobacter was one of the nine abundant genera in all patients tested (57).
In closed eye tears, the microbial ecology (determined by 16S sequencing) of tear samples from normal participants and from patients with mild dry eye showed no significant difference, but clear differences were observed between participants with mild and moderate dry eye (2). Therefore, that study supported our findings that our mild dry eye participants showed no alteration of microbiome from normal participants. If the mild dry eye cases don’t have a different microbiome from controls, it suggests that until more severe dry eye is reached, the level of immunoregulation on the ocular surface may not be drastically perturbed.
A. xylosoxidans is a Gram-negative aerobic, oxidase- and catalase-positive, motile bacterium with peritrichous flagella found in unsanitary conditions, soil and water. While the exact function of Achromobacter spp. in the normal eye is not known, they are likely commensals. In dry eye disease, it was one of the more variable genera (2). In fungal keratitis, it was also one of the most abundant genera detected (11). We found several proinflammatory cytokines to be upregulated when cultured human corneal epithelial cells were exposed to the three strains of this bacterium tested here, with the morphology of the cells remaining normal. Some of these dysregulated cytokines have been reported to be elevated in the tear fluid of people with dry eye (58). The TLR ligand LPS is the most abundant cell wall component of Gram-negative bacteria, including those of Achromobacter. LPS from different bacteria may compete for the same cellular receptors, so partially inhibitory relationships may exist between different Gram-negative bacteria.
Our hypothesis is that with increasing age, there is an altered immunoregulatory influence due to the change in composition of the microbiome. There is an increase in Achromobacter spp., and reduction in several other species of bacteria and fungi. Because of the change in the microbial ecosystem, there is reduced tolerance and increased prevalence of inflammatory conditions, such as dry eye. In fact, the increase in CD4+ T lymphocytes in the conjunctiva of older healthy people (59) is consistent with this concept. Some of the bacteria found to be reduced in the elderly, such as Micavibrio aeruginosavorus, normally feeds on pathogenic Pseudomonas aeruginosa (60). Hence, this finding may explain the increased susceptibility to ocular surface inflammation and infection in old age. A few redundant members of the bacterial microbiome can serve to maintain functionality of the community (61). Reduction of some of the 23 species listed in Supplementary Table 3 may not impact on conjunctival mucosal defense. Supplementary Tables 6, 7 summarize the studies of conjunctival microbiomes in dry eye and other ocular surface diseases, respectively.
Our study employed shotgun whole genome sequencing metagenomic analysis for characterizing the ocular microbiome. All participants for the eye microbiome investigation were subjected to standard characterization, including objective measurements of tear break up times. One of the limitations of the study is that we only examined superficial conjunctival fornix, and the results may not be applicable to microbiomes of the cornea or the bulbar conjunctiva. A study has shown that cornea and conjunctival microbiota are different (62). In addition, our experiments with Achromobacter were entirely in vitro, and it would be beneficial to evaluate the response induced by Achromobacter spp. inoculation on the ocular surface in animal disease models. We only tested the effect of Achromobacter spp. on human corneal epithelial cells as it was difficult to get conjunctival epithelial cells. We did not investigate whether the above effects on the cultured cells are specific to Achromobacter. Since the microbiome composition may be influenced by environmental and occupational factors, it may not be possible to extrapolate the results to participants from a different setting. It is not possible to delineate whether it is the external climate or the indoor conditions that shape this microbiome, though the China study that evaluated three cities suggest inter-center variability more than intra-center findings. Our sample size was small, it is possible that statistically significant differences may be revealed by larger sample sizes, but in the literature, similar sample sizes were able to detect changes in severe MGD (33, 63). Tetracaine was applied before sample collection. However, the possibility of tetracaine to introduce contaminant DNA is very low, since it was instilled from sterile unit dose (single use) vials which were discarded after application by each participant. Tetracaine was used for both the dry eye and comparison participants groups. Tetracaine may reduce diversity, but we believe the effect of tetracaine to diversity is limited.
Conclusion
In conclusion, we report the results derived from a comprehensive characterization of the ocular surface microbiome in participants with mild dry eye and control individuals. In the normal ocular microbiome, the phylum Proteobacteria dominates, with presence of Achromobacter spp. which increases in abundance with age. We also demonstrated that strains of A. xylosoxidans induced cytokine expression in basal and stressed epithelial cells. The alteration of the ocular surface microbial ecosystem with age may influence its susceptibility to inflammation.
Data availability statement
The data presented in this study are deposited in the NCBI Sequence Read Archive repository, accession number: PRJNA886972.
Ethics statement
The studies involving human participants were reviewed and approved by the SingHealth Centralized Institutional Review Board. The patients/participants provided their written informed consent to participate in this study.
Author contributions
LT and SK were the principle investigators of the study, provided study funding, participated in the study design, data interpretation, and wrote the manuscript. LT performed clinical assessment of the participants. LY, DD-M, and SS contributed to the preparation and acquisition of sequencing data. FC and RW participated in the bioinformatics analysis and the revision of the manuscript. AH and SC contributed to the acquisition of the Achromobacter treatment data and revision of the manuscript. All authors read and approved the final manuscript and contributed to the article and approved the submitted version.
Funding
This research was supported by the National Medical Research Council (NMRC) (NMRC/CSA/045/2012 and NMRC/CSA-SI/0017/2017).
Acknowledgments
We thank Sharon Yeo from Singapore Eye Research Institute for the participants recruitment.
Conflict of interest
The authors declare that the research was conducted in the absence of any commercial or financial relationships that could be construed as a potential conflict of interest.
Publisher’s note
All claims expressed in this article are solely those of the authors and do not necessarily represent those of their affiliated organizations, or those of the publisher, the editors and the reviewers. Any product that may be evaluated in this article, or claim that may be made by its manufacturer, is not guaranteed or endorsed by the publisher.
Supplementary material
The Supplementary Material for this article can be found online at: https://www.frontiersin.org/articles/10.3389/fmed.2022.1034131/full#supplementary-material
Footnotes
References
1. Andersson J, Vogt JK, Dalgaard MD, Pedersen O, Holmgaard K, Heegaard S. Ocular surface microbiota in patients with aqueous tear-deficient dry eye. Ocul Surf. (2021) 19:210–7. doi: 10.1016/j.jtos.2020.09.003
2. Willis KA, Postnikoff CK, Freeman A, Rezonzew G, Nichols K, Gaggar A, et al. The closed eye harbours a unique microbiome in dry eye disease. Sci Rep. (2020) 10:12035. doi: 10.1038/s41598-020-68952-w
3. Li Z, Gong Y, Chen S, Li S, Zhang Y, Zhong H, et al. Comparative portrayal of ocular surface microbe with and without dry eye. J Microbiol. (2019) 57:1025–32. doi: 10.1007/s12275-019-9127-2
4. Graham JE, Moore JE, Jiru X, Moore JE, Goodall EA, Dooley JS, et al. Ocular pathogen or commensal: a PCR-based study of surface bacterial flora in normal and dry eyes. Invest Ophthalmol Vis Sci. (2007) 48:5616–23. doi: 10.1167/iovs.07-0588
5. Kittipibul T, Puangsricharern V, Chatsuwan T. Comparison of the ocular microbiome between chronic Stevens-Johnson syndrome patients and healthy subjects. Sci Rep. (2020) 10:4353. doi: 10.1038/s41598-020-60794-w
6. Zilliox MJ, Gange WS, Kuffel G, Mores CR, Joyce C, de Bustros P, et al. Assessing the ocular surface microbiome in severe ocular surface diseases. Ocul Surf. (2020) 18:706–12. doi: 10.1016/j.jtos.2020.07.007
7. Liang Q, Li J, Zhang S, Liao Y, Guo S, Liang J, et al. Characterization of conjunctival microbiome dysbiosis associated with allergic conjunctivitis. Allergy. (2021) 76:596–600. doi: 10.1111/all.14635
8. Yau JW, Hou J, Tsui SKW, Leung TF, Cheng NS, Yam JC, et al. Characterization of ocular and nasopharyngeal microbiome in allergic rhinoconjunctivitis. Pediatr Allergy Immunol. (2019) 30:624–31. doi: 10.1111/pai.13088
9. Butcher RMR, Sokana O, Jack K, Kalae E, Sui L, Russell C, et al. Active trachoma cases in the solomon Islands have varied polymicrobial community structures but do not associate with individual non-chlamydial pathogens of the eye. Front Med. (2017) 4:251. doi: 10.3389/fmed.2017.00251
10. Shivaji S, Jayasudha R, Chakravarthy SK, SaiAbhilash CR, Sai Prashanthi G, Sharma S, et al. Alterations in the conjunctival surface bacterial microbiome in bacterial keratitis patients. Exp Eye Res. (2021) 203:108418. doi: 10.1016/j.exer.2020.108418
11. Ge C, Wei C, Yang BX, Cheng J, Huang YS. Conjunctival microbiome changes associated with fungal keratitis: metagenomic analysis. Int J Ophthalmol. (2019) 12:194–200.
12. McClure R, Massari P. TLR-dependent human mucosal epithelial cell responses to microbial pathogens. Front Immunol. (2014) 5:386. doi: 10.3389/fimmu.2014.00386
13. Marino A, Pergolizzi S, Lauriano ER, Santoro G, Spataro F, Cimino F, et al. TLR2 activation in corneal stromal cells by Staphylococcus aureus-induced keratitis. APMIS. (2015) 123:163–8. doi: 10.1111/apm.12333
14. Pearlman E, Sun Y, Roy S, Karmakar M, Hise AG, Szczotka-Flynn L, et al. Host defense at the ocular surface. Int Rev Immunol. (2013) 32:4–18. doi: 10.3109/08830185.2012.749400
15. Redfern RL, Barabino S, Baxter J, Lema C, McDermott AM. Dry eye modulates the expression of toll-like receptors on the ocular surface. Exp Eye Res. (2015) 134:80–9. doi: 10.1016/j.exer.2015.03.018
16. Knop E, Knop N. Influence of the eye-associated lymphoid tissue (EALT) on inflammatory ocular surface disease. Ocul Surf. (2005) 3(Suppl. 4):S180–6. doi: 10.1016/S1542-0124(12)70251-3
17. Knop E, Knop N. The role of eye-associated lymphoid tissue in corneal immune protection. J Anat. (2005) 206:271–85. doi: 10.1111/j.1469-7580.2005.00394.x
18. Knop N, Knop E. Conjunctiva-associated lymphoid tissue in the human eye. Invest Ophthalmol Vis Sci. (2000) 41:1270–9.
19. Knop N, Knop E. Regulation of the inflammatory component in chronic dry eye disease by the eye-associated lymphoid tissue (EALT). Dev Ophthalmol. (2010) 45:23–39. doi: 10.1159/000315017
20. Atarashi K, Tanoue T, Shima T, Imaoka A, Kuwahara T, Momose Y, et al. Induction of colonic regulatory T cells by indigenous Clostridium species. Science. (2011) 331:337–41. doi: 10.1126/science.1198469
21. Balfour Sartor R. Bacteria in Crohn’s disease: mechanisms of inflammation and therapeutic implications. J Clin Gastroenterol. (2007) 41(Suppl. 1):S37–43. doi: 10.1097/MCG.0b013e31802db364
22. Duck LW, Walter MR, Novak J, Kelly D, Tomasi M, Cong Y, et al. Isolation of flagellated bacteria implicated in Crohn’s disease. Inflamm Bowel Dis. (2007) 13:1191–201. doi: 10.1002/ibd.20237
23. Neish AS. Microbes in gastrointestinal health and disease. Gastroenterology. (2009) 136:65–80. doi: 10.1053/j.gastro.2008.10.080
24. Iovieno A, Lambiase A, Sacchetti M, Stampachiacchiere B, Micera A, Bonini S. Preliminary evidence of the efficacy of probiotic eye-drop treatment in patients with vernal keratoconjunctivitis. Graefes Arch Clin Exp Ophthalmol. (2008) 246:435–41. doi: 10.1007/s00417-007-0682-6
25. Chisari G, Chisari EM, Borzi AM, Chisari CG. Aging eye microbiota in dry eye syndrome in patients treated with Enterococcus faecium and Saccharomyces boulardii. Curr Clin Pharmacol. (2017) 12:7. doi: 10.2174/1574884712666170704145046
26. Yun SW, Son YH, Lee DY, Shin YJ, Han MJ, Kim DH. Lactobacillus plantarum and Bifidobacterium bifidum alleviate dry eye in mice with exorbital lacrimal gland excision by modulating gut inflammation and microbiota. Food Funct. (2021) 12:2489–97. doi: 10.1039/D0FO02984J
27. Moon J, Ryu JS, Kim JY, Im SH, Kim MK. Effect of IRT5 probiotics on dry eye in the experimental dry eye mouse model. PLoS One. (2020) 15:e0243176. doi: 10.1371/journal.pone.0243176
28. Choi SH, Oh JW, Ryu JS, Kim HM, Im SH, Kim KP, et al. IRT5 probiotics changes immune modulatory protein expression in the extraorbital lacrimal glands of an autoimmune dry eye mouse model. Invest Ophthalmol Vis Sci. (2020) 61:42. doi: 10.1167/iovs.61.3.42
29. Lee SH, Oh DH, Jung JY, Kim JC, Jeon CO. Comparative ocular microbial communities in humans with and without blepharitis. Invest Ophthalmol Vis Sci. (2012) 53:5585–93. doi: 10.1167/iovs.12-9922
30. Willcox MD. Characterization of the normal microbiota of the ocular surface. Exp Eye Res. (2013) 117:99–105. doi: 10.1016/j.exer.2013.06.003
31. Sankaridurg PR, Markoulli M, de la Jara PL, Harmis N, Varghese T, Willcox MD, et al. Lid and conjunctival micro biota during contact lens wear in children. Optom Vis Sci. (2009) 86:312–7. doi: 10.1097/OPX.0b013e318199d20c
32. Hori Y, Maeda N, Sakamoto M, Koh S, Inoue T, Tano Y. Bacteriologic profile of the conjunctiva in the patients with dry eye. Am J Ophthalmol. (2008) 146:729–34. doi: 10.1016/j.ajo.2008.06.003
33. de Paiva CS, Jones DB, Stern ME, Bian F, Moore QL, Corbiere S, et al. Altered mucosal microbiome diversity and disease severity in sjogren syndrome. Sci Rep. (2016) 6:23561. doi: 10.1038/srep23561
34. Dong Q, Brulc JM, Iovieno A, Bates B, Garoutte A, Miller D, et al. Diversity of bacteria at healthy human conjunctiva. Invest Ophthalmol Vis Sci. (2011) 52:5408–13. doi: 10.1167/iovs.10-6939
35. Shin H, Price K, Albert L, Dodick J, Park L, Dominguez-Bello MG. Changes in the eye microbiota associated with contact lens wearing. mBio. (2016) 7:e00198. doi: 10.1128/mBio.00198-16
36. Delbeke H, Younas S, Casteels I, Joossens M. Current knowledge on the human eye microbiome: a systematic review of available amplicon and metagenomic sequencing data. Acta Ophthalmol. (2021) 99:16–25. doi: 10.1111/aos.14508
37. Kang Y, Lin S, Ma X, Che Y, Chen Y, Wan T, et al. Strain heterogeneity, cooccurrence network, taxonomic composition and functional profile of the healthy ocular surface microbiome. Eye Vis. (2021) 8:6. doi: 10.1186/s40662-021-00228-4
38. Deng Y, Wen X, Hu X, Zou Y, Zhao C, Chen X, et al. Geographic difference shaped human ocular surface metagenome of Young Han Chinese From Beijing, Wenzhou, and Guangzhou Cities. Invest Ophthalmol Vis Sci. (2020) 61:47. doi: 10.1167/iovs.61.2.47
39. Kang Y, Zhang H, Hu M, Ma Y, Chen P, Zhao Z, et al. Alterations in the ocular surface microbiome in traumatic corneal ulcer patients. Invest Ophthalmol Vis Sci. (2020) 61:35. doi: 10.1167/iovs.61.6.35
40. Zhao F, Zhang D, Ge C, Zhang L, Reinach PS, Tian X, et al. Metagenomic profiling of ocular surface microbiome changes in meibomian gland dysfunction. Invest Ophthalmol Vis Sci. (2020) 61:22. doi: 10.1167/iovs.61.8.22
41. Wen X, Miao L, Deng Y, Bible PW, Hu X, Zou Y, et al. The influence of age and sex on ocular surface microbiota in healthy adults. Invest Ophthalmol Vis Sci. (2017) 58:6030–7. doi: 10.1167/iovs.17-22957
42. Dave SB, Toma HS, Kim SJ. Changes in ocular flora in eyes exposed to ophthalmic antibiotics. Ophthalmology. (2013) 120:937–41. doi: 10.1016/j.ophtha.2012.11.005
43. DEWS. The definition and classification of dry eye disease: report of the definition and classification subcommittee of the international dry eye workshop (2007). Ocul Surf. (2007) 5:75–92. doi: 10.1016/S1542-0124(12)70081-2
44. Karimsab D, Razak SK. Study of aerobic bacterial conjunctival flora in patients with diabetes mellitus. Nepal J Ophthalmol. (2013) 5:28–32. doi: 10.3126/nepjoph.v5i1.7818
45. Martins EN, Alvarenga LS, Höfling-Lima AL, Freitas D, Zorat-Yu MC, Farah ME, et al. Aerobic bacterial conjunctival flora in diabetic patients. Cornea. (2004) 23:136–42. doi: 10.1097/00003226-200403000-00006
46. Chong PQ, Yeo S, Too CL, Boo C, Tong L. Effects of wearing a daily disposable lens on tear film: a randomised controlled trial. Clin Exp Optom. (2016) 99:241–7. doi: 10.1111/cxo.12357
47. Downie LE, Keller PR, Vingrys AJ. Assessing ocular bulbar redness: a comparison of methods. Ophthalmic Physiol Opt. (2016) 36:132–9. doi: 10.1111/opo.12245
48. Tong L, Chaurasia SS, Mehta JS, Beuerman RW. Screening for meibomian gland disease: its relation to dry eye subtypes and symptoms in a tertiary referral clinic in singapore. Invest Ophthalmol Vis Sci. (2010) 51:3449–54. doi: 10.1167/iovs.09-4445
49. Fenner BJ, Tong L. Corneal staining characteristics in limited zones compared with whole cornea documentation for the detection of dry eye subtypes. Invest Ophthalmol Vis Sci. (2013) 54:8013–9. doi: 10.1167/iovs.13-12802
50. Araki-Sasaki K, Ohashi Y, Sasabe T, Hayashi K, Watanabe H, Tano Y, et al. An SV40-immortalized human corneal epithelial cell line and its characterization. Invest Ophthalmol Vis Sci. (1995) 36:614–21.
51. Tong L, Htoon HM, Hou A, Acharya RU, Tan JH, Wei QP, et al. Acupuncture and herbal formulation compared with artificial tears alone: evaluation of dry eye symptoms and associated tests in randomised clinical trial. BMJ Open Ophthalmol. (2018) 3:e000150. doi: 10.1136/bmjophth-2018-000150
52. Schein OD, Tielsch JM, Munõz B, Bandeen-Roche K, West S. Relation between signs and symptoms of dry eye in the elderly. A population-based perspective. Ophthalmology. (1997) 104:1395–401. doi: 10.1016/S0161-6420(97)30125-0
53. Hamady M, Knight R. Microbial community profiling for human microbiome projects: tools, techniques, and challenges. Genome Res. (2009) 19:1141–52. doi: 10.1101/gr.085464.108
54. The Human Microbiome Project Consortium. Structure, function and diversity of the healthy human microbiome. Nature. (2012) 486:207–14. doi: 10.1038/nature11234
55. Cavuoto KM, Banerjee S, Miller D, Galor A. Composition and comparison of the ocular surface microbiome in infants and older children. Transl Vis Sci Technol. (2018) 7:16. doi: 10.1167/tvst.7.6.16
56. Cavuoto KM, Mendez R, Miller D, Galor A, Banerjee S. Effect of clinical parameters on the ocular surface microbiome in children and adults. Clin Ophthalmol. (2018) 12:1189–97. doi: 10.2147/OPTH.S166547
57. Fan C, Yang B, Huang Y. Efficacy of 0.5% levofloxacin and 5.0% povidone-iodine eyedrops in reducing conjunctival bacterial flora: metagenomic analysis. J Ophthalmol. (2020) 2020:1780498. doi: 10.1155/2020/1780498
58. Hagan S, Tomlinson A. Tear fluid biomarker profiling: a review of multiplex bead analysis. Ocul Surf. (2013) 11:219–35. doi: 10.1016/j.jtos.2013.04.004
59. Williams GP, Denniston AK, Oswal KS, Tomlins PJ, Barry RJ, Rauz S, et al. The dominant human conjunctival epithelial CD8alphabeta+ T cell population is maintained with age but the number of CD4+ T cells increases. Age. (2012) 34:1517–28. doi: 10.1007/s11357-011-9316-3
60. Wang Z, Kadouri DE, Wu M. Genomic insights into an obligate epibiotic bacterial predator: micavibrio aeruginosavorus ARL-13. BMC Genomics. (2011) 12:453. doi: 10.1186/1471-2164-12-453
61. Van den Abbeele P, Van de Wiele T, Verstraete W, Possemiers S. The host selects mucosal and luminal associations of coevolved gut microorganisms: a novel concept. FEMS. (2011) 35:14. doi: 10.1111/j.1574-6976.2011.00270.x
62. Matysiak A, Kabza M, Karolak JA, Jaworska MM, Rydzanicz M, Ploski R, et al. Characterization of ocular surface microbial profiles revealed discrepancies between conjunctival and corneal microbiota. Pathogens. (2021) 10:405. doi: 10.3390/pathogens10040405
63. Dong X, Wang Y, Wang W, Lin P, Huang Y. Composition and diversity of bacterial community on the ocular surface of patients with meibomian gland dysfunction. Invest Ophthalmol Vis Sci. (2019) 60:4774–83. doi: 10.1167/iovs.19-27719
64. Huang Y, Yang B, Li W. Defining the normal core microbiome of conjunctival microbial communities. Clin Microbiol Infect. (2016) 22:.e7–643. doi: 10.1016/j.cmi.2016.04.008
65. Doan T, Akileswaran L, Andersen D, Johnson B, Ko N, Shrestha A, et al. Paucibacterial microbiome and resident DNA virome of the healthy conjunctiva. Invest Ophthalmol Vis Sci. (2016) 57:5116–26. doi: 10.1167/iovs.16-19803
66. Ozkan J, Nielsen S, Nielsen C, Diez-Vives M, Thomas T, Willcox M. Temporal stability and composition of the ocular surface microbiome. Sci Rep. (2017) 7:9880. doi: 10.1038/s41598-017-10494-9
67. Ozkan J, Coroneo M, Willcox M, Wemheuer B, Thomas T. Identification and visualization of a distinct microbiome in ocular surface conjunctival tissue. Invest Ophthalmol Vis Sci. (2018) 59:4268–76. doi: 10.1167/iovs.18-24651
68. Cavuoto KM, Galor A, Banerjee S. Anatomic characterization of the ocular surface microbiome in children. Microorganisms. (2019) 7:259. doi: 10.3390/microorganisms7080259
69. Suzuki T, Sutani T, Nakai H, Shirahige K, Kinoshita S. The microbiome of the meibum and ocular surface in healthy subjects. Invest Ophthalmol Vis Sci. (2020) 61:18. doi: 10.1167/iovs.61.2.18
Keywords: human microbiome, inflammation, ocular disease, dry eye, metagenomics
Citation: Tong L, Constancias F, Hou A, Chua SL, Drautz-Moses DI, Schuster SC, Yang L, Williams RBH and Kjelleberg S (2022) Shotgun metagenomic sequencing analysis of ocular surface microbiome in Singapore residents with mild dry eye. Front. Med. 9:1034131. doi: 10.3389/fmed.2022.1034131
Received: 06 September 2022; Accepted: 24 October 2022;
Published: 10 November 2022.
Edited by:
Wei Chi, Zhongshan Ophthalmic Center, Sun Yat-sen University, ChinaReviewed by:
Kyoung Yul Seo, Yonsei University Health System, South KoreaHun Lee, University of Ulsan, South Korea
Copyright © 2022 Tong, Constancias, Hou, Chua, Drautz-Moses, Schuster, Yang, Williams and Kjelleberg. This is an open-access article distributed under the terms of the Creative Commons Attribution License (CC BY). The use, distribution or reproduction in other forums is permitted, provided the original author(s) and the copyright owner(s) are credited and that the original publication in this journal is cited, in accordance with accepted academic practice. No use, distribution or reproduction is permitted which does not comply with these terms.
*Correspondence: Louis Tong, TG91aXMudG9uZy5oLnRAc2luZ2hlYWx0aC5jb20uc2c=